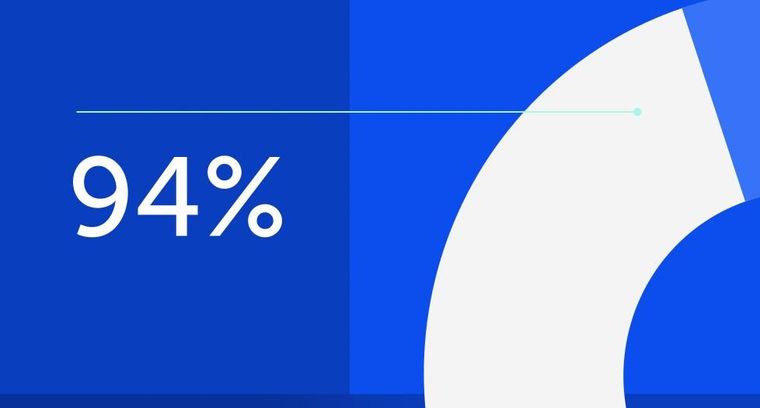
94% of researchers rate our articles as excellent or good
Learn more about the work of our research integrity team to safeguard the quality of each article we publish.
Find out more
PERSPECTIVE article
Front. Microbiol., 20 April 2023
Sec. Antimicrobials, Resistance and Chemotherapy
Volume 14 - 2023 | https://doi.org/10.3389/fmicb.2023.1134755
This article is part of the Research TopicResistant Pathogens: From Distribution to Emerging TherapiesView all 5 articles
The increasing number of chronic and life-threatening infections caused by antimicrobial resistant fungal isolates is of critical concern. Low DNA sequencing cost may facilitate the identification of the genomic profile leading to resistance, the resistome, to rationally optimize the design of antifungal therapies. However, compared to bacteria, initiatives for resistome detection in eukaryotic pathogens are underdeveloped. Firstly, reported mutations in antifungal targets leading to reduced susceptibility must be extensively collected from the literature to generate comprehensive databases. This information should be complemented with specific laboratory screenings to detect the highest number possible of relevant genetic changes in primary targets and associations between resistance and other genomic markers. Strikingly, some drug resistant strains experience high-level genetic changes such as ploidy variation as much as duplications and reorganizations of specific chromosomes. Such variations involve allelic dominance, gene dosage increments and target expression regime effects that should be explicitly parameterized in antifungal resistome prediction algorithms. Clinical data indicate that predictors need to consider the precise pathogen species and drug levels of detail, instead of just genus and drug class. The concomitant needs for mutation accuracy and assembly quality assurance suggest hybrid sequencing approaches involving third-generation methods will be utilized. Moreover, fatal fast infections, like fungemia and meningitis, will further require both sequencing and analysis facilities are available in-house. Altogether, the complex nature of antifungal resistance demands extensive sequencing, data acquisition and processing, bioinformatic analysis pipelines, and standard protocols to be accomplished prior to genome-based protocols are applied in the clinical setting.
Genome-based predictors of antimicrobial resistance (AMR) are not available for fungal pathogens. In contrast, the extensive knowledge of resistance markers is widely applied in bacterial isolates for the automated identification of the AMR genome section, the resistome. The performance accuracy of some of these methods equals antibiograms, the current standard in routine laboratories. Among AMR bacteria, the six ESKAPE organisms cause most intra-hospital cases (Rice, 2008). Resistome identification helps to rationally guide the treatment to prevent therapeutic failure and raise of future resistance levels in the principal bacterial pathogens.
Antifungal resistance arises by selective pressure after long treatment and/or wide utilization of antifungal clinical agents (Cowen, 2008) besides fungicides with agricultural purposes (Snelders et al., 2012). Prophylaxis is compromised by the several hurdles that prevent the development of vaccines to protect against fungal diseases (Oliveira et al., 2021). Therefore, despite resistance, control of fungal infections still exclusively relies on antimicrobial therapy. More than 95% clinical cases, including those refractory to treatment, are caused by three genera: Cryptococcus, Candida, and Aspergillus (Brown et al., 2012).
Several protocols have approached the bacterial resistome with different degrees of drug and pathogen inclusivity (Scaria et al., 2005; Liu and Pop, 2009; Gupta et al., 2014; de Man and Limbago, 2016; Alcock et al., 2020; Bortolaia et al., 2020). They commonly consist on a database of resistance determinants, a detection algorithm, and a controlled vocabulary scheme that connects the whole body of information to produce a readable outcome. Resistance markers are either the presence of genes (the “gene mode”), e.g., the mecA gene in beta-lactam-resistant Staphylococcus aureus, or specific polymorphisms in core genes (the “variant mode”), e.g., the S87W mutation in DNA Topoisomerase IV subunit A of fluoroquinolone-resistant Pseudomonas aeruginosa. However, higher biological complexity, multichromosomal organization, and lower omic data availability complicate the development of equivalent tools for eukaryotic pathogens as fungi or parasites (Leprohon et al., 2015).
Given the unquestionable interest on fungal resistome protocols, we objectively discuss here whether the construction of such tools is appropriate and realistic, and which aspects should be improved prior to meet their goals.
Resistome predictors are laborious initiatives only justified when provide evident clinical benefits. AMR bacteria show high virulence and national cost burden, associated with 5 million deaths and 1.3 direct causality in 2019 (Antimicrobial Resistance Collaborators, 2022). In 2017, the World Health Organization (WHO) presented a list with the top-12 bacterial pathogens (Tacconelli et al., 2018), which are extensively covered by resistome tools.
Fungal infections amount to 1.6 million deaths in developing countries (Almeida et al., 2019). Cryptococcus neoformans, Candida auris, Candida albicans, and Aspergillus fumigatus conform the critical priority group in the recently released Fungal Priority Pathogen List by the WHO.1 An increment of candidiasis caused by different Candida species has been observed (Lamoth et al., 2018), particularly C. auris in Europe (Kohlenberg et al., 2022). Fungal pathogens are gaining relevance in risk groups due to aging, AIDS, cancer chemotherapy, organ-transplanted patients, viral infections (flu and COVID-19), or ICU admission. Some cases can be deadly, such as systemic fungemia, pneumonia, and meningitis if not treated timely and properly. Candida is the fourth pathogen more often found in sepsis in the United States (Delaloye and Calandra, 2014).
Mortality and morbidity associated to AMR fungi is increasing (Perlin et al., 2017; Fisher et al., 2022; Gow et al., 2022; Figure 1A). The antimicrobial control of these infections is compromised by the small drug arsenal and genomic plasticity. Fungi are eukaryotic, i.e., molecularly similar to humans, which limits the number of antifungal family drugs to five. Only azoles, echinocandins, and polyenes are used to treat systemic infections. Genome plasticity facilitates the adaptive resistance to chemotherapeutical options during long treatments. Multidrug resistant phenotypes are also detected (Pfaller et al., 2012; Pham et al., 2014). In contrast to adaptive resistance, intrinsic resistance, caused by naturally occurring ancestral mutations, has also been detected (Chowdhary et al., 2018). Especially worrisome are the intrinsic pan-resistance observed in Lomentospora prolificans (Wu et al., 2020) and the emergence of fluconazole resistant Candida parapsilosis besides its intrinsic higher MICs to echinocandins (Trevijano-Contador et al., 2022). The therapeutic failure using the two preferred drug classes forces the prescription of alternative treatments such as amphotericin B, the antifungal with a broadest spectrum.
Figure 1. Occurrence of literature entries and number of genomes for AMR bacteria and fungi. (A) Number of PubMed (https://pubmed.ncbi.nlm.nih.gov/) counts during the 1990–2021 period using the “Antibiotic resistance” and “Antifungal resistance” search terms. (B) Number of entries concerning DNA reads and assembled genomes for the principal AMR bacteria and fungi in the SRA (https://www.ncbi.nlm.nih.gov/sra) and Assembly (https://www.ncbi.nlm.nih.gov/assembly) NCBI databases, respectively.
Fungal infections are usually treated by empirical therapy based on historical records or after applying phenotypical methods. Antifungal susceptibility testing involves the standardized exposition of the fungus to different concentrations of drug in liquid or solid media using reference protocols (CLSI and EUCAST). Therapeutic efficacy can be therefore predicted by quantitatively assessing the minimum inhibitory or effective concentrations. Clinical break points for some antifungals and species are available. Disadvantages of phenotypical methods include low reproducibility by subtle operational changes and potential absence of cutoff points. Besides, they are labor-intensive and time-consuming which has a direct impact in mortality (Garey et al., 2006; Chamilos et al., 2008).
Alternatively, the identification of mutations by sequencing outperforms MIC for antifungal therapy success (Shields et al., 2012; Alexander et al., 2013). However, only a limited number of genes can be sequenced by conventional Sanger sequencing or screened by real-time PCR (Durand et al., 2021). Resistance marker expression can be approached by MS MALDI-TOF (Vella et al., 2017; Maenchantrarath et al., 2022), which shows short turn-around-times but lacks of standards for universal application (Durand et al., 2021).
The polygenic nature of antifungal resistance (Gonçalves et al., 2016) favors the utilization of massive techniques. Cost-effective new generation sequencing (NGS) alone or complemented with better genome assemblies achieved by third-generation sequencing (TGS) facilitates obtaining whole-genome sequences. Genomics provides a view of the strain genetics at the highest, nucleotide-level, resolution, and the simultaneous evaluation of a multiplicity of factors (Garnaud et al., 2015; McTaggart et al., 2020; Schikora-Tamarit and Gabaldón, 2022b).
A central question is whether the current scientific knowledge includes enough fungal AMR mechanisms to fuel the development of efficient resistome predictors. Laboratory and clinical studies have indeed described numerous adaptive and intrinsic antifungal AMR processes with molecular precision.
Many point mutations linked to AMR have been characterized, which would be akin to the “variant mode” of bacterial resistome predictors. These include those in primary antifungal targets: the key enzyme for ergosterol biosynthesis sterol 14α demethylase for azoles, and glucan synthase for echinocandins. Many of these mutations produce residue changes in relevant (hot-spot) zones like drug binding pockets (Garcia-Effron et al., 2009). Mutations in non-primary target proteins can also produce resistance, such as in the ergosterol biosynthesis pathway to azoles (Alcazar-Fuoli and Mellado, 2012). Loss-of-function (LOF) resistance mutations, such as premature stop codons and insertion of transposable elements, have been described for instance in the ergosterol metabolism enzyme ERG3 (Lupetti et al., 2002; Ksiezopolska et al., 2021). Intrinsic AMR examples are the numerous polymorphisms in ERG11 and FKS proteins, causing azole and echinocandin resistance in C. auris (Muñoz et al., 2018) and C. parapsilosis (Garcia-Effron et al., 2008), respectively. Decrease of the intracellular drug concentration to ineffective levels can be achieved by gain-of-function (GOF) mutations in positive regulators of antifungal efflux pumps (Cannon et al., 2009). AMR phenotypes are favored in isolates with hypermutator behavior due to altered DNA repair systems (Healey et al., 2016). Resistant phenotypes caused by point mutations are usually only observed in diploid fungi when the sensitive allele is lost (Garnaud et al., 2015).
Expression-driven resistance can also be obtained by: (i) mutations in response elements of cis promoters of efflux pumps (Gaur et al., 2004); (ii) new promoters introduced by transposable elements (Hu et al., 2021); (iii) longer mRNA stability by enzymatic variants that increase polyadenylation (Manoharlal et al., 2010); and (iv) LOF mutations of lysine-acetylation enzymes of histones via epigenetic chromatin modification (Orta-Zavalza et al., 2013; Tscherner et al., 2015).
Fungal AMR often involve large chromosomal changes affecting copy number and expression regime of genes. Large changes include ploidy alterations, the combination of chromosomes into new ones, and the generation of isochromosomes (Selmecki et al., 2006, 2008; McTaggart et al., 2020). For example, chromosomal monosomies have been associated with tolerance to fluconazole and 5-flourocytosine (Yang et al., 2013; Uddin et al., 2021). The copy number of genes coding primary targets can be also increased by local duplication (Chow et al., 2020).
An essential issue for routine antifungal resistome is how many fungal AMR markers are detectable by WGS and with which degree of automation.
Intrinsic resistance involves precise taxon identification. For that, k-mers thresholds for species assignation have been reported (Gostinčar, 2020). Precise sub-species detection requires the application of phylogenomic tools or the standard sequence type, ST, scheme (Taylor and Fisher, 2003).
Point polymorphisms associated to AMR follow the classical “variant-mode” resistome principles. Illumina NGS technology meets quality and coverage standards enough to identify LOF and GOF residue changes, premature stop codons, small indels, and gene duplications (Garnaud et al., 2015). Protocols such as OVarFlow and SnpEff are available to call and study point and small indels in eukaryotic genomes (Bathke and Lühken, 2021; Cingolani, 2022). Fungal-specific and other tools have been already designed for this task, such as YMAP (Abbey et al., 2014). Deep coverages are also adequate to find heteroresistant subpopulations (Zhai et al., 2022). Homozygosity (~100% reads) and heterozygosity (~50% reads) are approachable using the proportion of mutated reads (Spettel et al., 2019).
Large chromosomal changes are harder to parametrize although they can be evaluated through tools like PerSVade (Schikora-Tamarit and Gabaldón, 2022a) or Assemblytics (Nattestad and Schatz, 2016). NGS permits proportional read count alteration in certain chromosomes or in the vicinity context of marker genes. Transposable elements are identifiable using sequence profiles from databases like Repbase (Kapitonov and Jurka, 2008). However, genome environment modifications through transposons and chromosomal rearrangements may require high-quality assemblies as those provided by TGS platforms.
The nature of some antifungal resistance modes is still too opaque to be investigated. The resulting alteration profiles by genomics can be a mixture of AMR causal, linked, suspected, or irrelevant changes. Players include drug targets, regulators, pathways, promoters, mRNA polyadenylation, and lysine acetylases. To assess the relevance of sequence variations, the number of sequenced genomes and the molecular knowledge of fungal pathogens should be strengthened (Figure 1B).
Beyond performance in the research environment, the ultimate goal of antifungal resistome predictors is their clinical application. Full advantage of genome-based tools has been taken for bacterial pathogens in the clinical laboratory in the recent years (Deurenberg et al., 2017).
Fungal resistome tools should be economically efficient, in particular in developing countries. The number of samples per NGS run should be optimized. The number of reads and assemblies obtained by NGS should satisfy quality metrics such as high genome coverage and a small contig number. Concerning TGS, nanopore flongle cells or multiplexing show lower fidelity but are cost-effective options for NGS-TGS hybrid approaches (Lipworth et al., 2020) or utilization in low- and middle-income countries. Expense can also be reduced by targeted resequencing (Spettel et al., 2019).
A principal hurdle in genomics is the downtime to obtain sequencing results. Long turnaround-times may be assumable for chronic non-critical superficial infections (like candiduria), but not for life-threatening ones (like fungemia, pneumonia or meningitis) that could be mortal within 24 h. The later demands in-house facilities as dependence of third externalized parties can substantially delay outcomes (Raven et al., 2018). Thus, the initial investment to purchase NGS facilities would be eventually profitable. Furthermore, nanopore technologies are relatively cheap and can be escalated up to 48 simultaneous cells.
The requirement of a high bioinformatic knowledge may also hamper the predictor utilization. Unexperienced laboratory staff should be trained to execute and interpret the provided software. On-line resources that provide human readable reports, such as Pathogenwatch2 and Microreact (Argimón et al., 2016), besides handy computational environments like Galaxy (Galaxy Community, 2022) may facilitate analyses for laboratories worldwide.
The wide application of genome-based therapy is still far for fungal infections. Worrying epidemiological data support the recent decision by the WHO of raising prioritization to control difficult-to-treat fungal pathogens, particularly opportunistic ones in immunocompromised patients. The reduced number of licensed antifungals highlights the relevance to discern susceptible phenotypes to prevent exhaustion of the therapeutic options. Accurate antifungal susceptibility methods are needed (Pristov and Ghannoum, 2019), which allows for the application of rational antifungal regimes. Over empirical multiple therapy, this may reduce long hospital admission timeframe, sequelae, death, high treatment cost, and future resistance (Cowen et al., 2014). Several antifungal resistance modes have been described at molecular detail and algorithms to potentially detect them are accessible. However, some intermediate milestones to develop resistome predictors are not straightforwardly achievable as fungal AMR present additional complex mechanisms respect to bacteria (Table 1). Here, we have objectively evaluated which specific steps are presently solved and which are pending prior to have equivalent tools to bacterial resistome predictors.
A strong limitation in the field is the incomplete understanding of some antifungal resistance modes. Fungal molecular biology is intricate and omic data is scarce. Moreover, fundamental knowledge of genetic mechanisms involved in resistance is currently biased toward C. albicans, which is not supported by recent epidemiological changes observed in this genus (Logan et al., 2020). Other primary pathogens, such as A. fumigatus (Bueid et al., 2010), remain comparatively neglected. Constraints also include polygenic resistant phenotypes, resulting from the confluence of several distributed markers, and the potential absence of a consensus reference strains. The completion in resistance modes, drugs, and species in cost- and time-efficient manner may benefit from multi-disciplinary consortia organized by the mycology community. Increases in the number of sequenced genomes, from multiple platforms, will permit to know the basal sequence variability, reveal previously unknown resistance determinants, precise typing, and virulence assessment. It should be noted that the contribution of databases to fungal resistome predictors ultimately depends on their quality. Likewise, experimental screenings showed a range of success in finding novel resistance modes (Snelders et al., 2008; Van Rhijn et al., 2021; Rhodes et al., 2022).
Compared to simpler monochromosomal bacteria, antifungal resistome software demands a substantial programming upgrade to cover a wide array of genetic events. Point residue mutations or premature stop codons just require simple gene detection and alignment. However, fungal AMR commonly involves gross chromosomal changes and multistep functional pathways. Algorithms to handle some of these issues are available but should be adapted to antifungal antecedents prior to their inclusion in pipelines. The software should operate on associated curated resources as databases that incorporate all reported advances on a regular basis. Such efforts require dedicated human staff and stable financing to prevent discontinuation. Pipelines should satisfy high standards prior approval for clinical decision-making in real patients.
Ideally, fungal resistome predictors would be fueled by the tripartite combination of raw reads, assemblies, and long-read approaches. The availability of in-house sequencing facilities and specialized bioinformatic staff will widen even more the differences also in time-turnaround terms. Consequentially, the sequencing costs and immediacy associated with fulminant infections will be prohibitive for tight budgets. We therefore envisage the screening of all resistance modes in routine will be progressively and unevenly, rather than universally, incorporated into healthcare centers.
Data sharing is facilitated by the standardization of genomic information. Cutoffs for sequencing and assembly quality metrics should be optimized for reproducible protocols that meet clinical-level criteria. The integration of data of different natures into a single system demands the development of a controlled language similar in spirit to the Antibiotic Resistance Ontology (Alcock et al., 2022). Such framework for terms and their connections must capture the correct hierarchical and granular nature of fungal AMR. For instance, the G54E mutation in CYP51A protects A. fumigatus against itraconazole, but not to voriconazole among the azole class (Leonardelli et al., 2017). Likewise, resistance can pertain to genus, species, or even sub-species, as the intrinsic azole resistant clade A observed in A. fumigatus (Rhodes et al., 2022). Markers should be causally associated to resistance through information about the protein involved, type of mutation (point, stop codon, and deletion), sequence position, location in hotspots, the exact resistance change observed, the GOF or LOF regime to exhibit resistance, and whether the resistant allele is phenotypically dominant or not. Taxon assignation plays a central role to infer the ploidy level and, subsequently, allelic dominance concerns, e.g., haploid for C. glabrata. For large changes, genetic environment descriptors of markers such as their chromosomal positioning and flanking genes should be considered. For regulatory issues, the promoter composition (integrity of responsive boxes) and the identification of adjacent transposable elements (type, orientation and integration sites) must be defined. Potential hypermutator behavior, heteroresistance indicators, and epigenetic changes should also be recorded. The degree of resistance may be binned into categories where high-level resistance may be achieved by the synergy of progressive multiple “intermediate” mechanism (Sasse et al., 2012) or by an unique event (Ferrari et al., 2009). Resistance determinants must be supported by literature identifiers, such as PMID or DOI. Clinical resistome initiatives will thus require strong data normalization and integration efforts.
Based on the above, the steps of the roadmap to follow recurrently may be: (i) exhaustive literature search for described resistance determinants where web-based specific and broad-purpose resources such as FunResDb (Weber et al., 2018) and MARDy (Nash et al., 2018) provide excellent starting material; (ii) conduction of extensive laboratory screenings (Dunyach et al., 2011; Rivero-Menendez et al., 2019) to map the mutational space leading to resistance (Schikora-Tamarit and Gabaldón, 2022b); (iii) generation of updated databases; and (iv) building resistome prediction pipelines.
Despite difficulty, the current status is, in our opinion, mature enough to warrant the design of fungal resistome pioneer tools. The first-generation algorithms should cover current data of Aspergillus, Candida, and Cryptococcus, and be further refined by more species, resistance modes, and quality criteria. Solving pending issues will be presumably cumbersome and demand a strong effort of the scientific community. Nevertheless, it will provide unprecedented performance for routine clinical management and surveillance of these challenging infections.
The original contributions presented in the study are included in the article, further inquiries can be directed to the corresponding authors.
AA-I and AM-G conceived the study, drafted the manuscript, revised the final version of the manuscript, and granted funding. All authors contributed to the article and approved the submitted version.
This study was supported by the Acción Estratégica en Salud from Fondo de Investigaciones Sanitarias, ISCIII, grants MPY 509/19 and PI20CIII/00043.
AA-I has received honoraria for educational talks of behalf of Gilead and Pfizer, outside of the submitted work.
The remaining author declares that the research was conducted in the absence of any commercial or financial relationships that could be construed as a potential conflict of interest.
All claims expressed in this article are solely those of the authors and do not necessarily represent those of their affiliated organizations, or those of the publisher, the editors and the reviewers. Any product that may be evaluated in this article, or claim that may be made by its manufacturer, is not guaranteed or endorsed by the publisher.
Abbey, D. A., Funt, J., Lurie-Weinberger, M. N., Thompson, D. A., Regev, A., Myers, C. L., et al. (2014). YMAP: a pipeline for visualization of copy number variation and loss of heterozygosity in eukaryotic pathogens. Genome Med. 6:100. doi: 10.1186/s13073-014-0100-8
Alcazar-Fuoli, L., and Mellado, E. (2012). Ergosterol biosynthesis in Aspergillus fumigatus: its relevance as an antifungal target and role in antifungal drug resistance. Front. Microbiol. 3:439. doi: 10.3389/fmicb.2012.00439
Alcock, B. P., Huynh, W., Chalil, R., Smith, K. W., Raphenya, A. R., Wlodarski, M. A., et al. (2022). CARD 2023: expanded curation, support for machine learning, and resistome prediction at the comprehensive antibiotic resistance database. Nucleic Acids Res. 51, D690–D699. doi: 10.1093/nar/gkac920
Alcock, B. P., Raphenya, A. R., Lau, T. T. Y., Tsang, K. K., Bouchard, M., Edalatmand, A., et al. (2020). CARD 2020: antibiotic resistome surveillance with the comprehensive antibiotic resistance database. Nucleic Acids Res. 48, D517–D525. doi: 10.1093/nar/gkz935
Alexander, B. D., Johnson, M. D., Pfeiffer, C. D., Jiménez-Ortigosa, C., Catania, J., Booker, R., et al. (2013). Increasing echinocandin resistance in Candida glabrata: clinical failure correlates with presence of FKS mutations and elevated minimum inhibitory concentrations. Clin. Infect. Dis. Off. Publ. Infect. Dis. Soc. Am. 56, 1724–1732. doi: 10.1093/cid/cit136
Almeida, F., Rodrigues, M. L., and Coelho, C. (2019). The still underestimated problem of fungal diseases worldwide. Front. Microbiol. 10:214. doi: 10.3389/fmicb.2019.00214
Antimicrobial Resistance Collaborators (2022). Global burden of bacterial antimicrobial resistance in 2019: a systematic analysis. Lancet Lond. Engl. 399, 629–655. doi: 10.1016/S0140-6736(21)02724-0
Argimón, S., Abudahab, K., Goater, R. J. E., Fedosejev, A., Bhai, J., Glasner, C., et al. (2016). Microreact: visualizing and sharing data for genomic epidemiology and phylogeography. Microb. Genomics 2:e000093. doi: 10.1099/mgen.0.000093
Bathke, J., and Lühken, G. (2021). OVarFlow: a resource optimized GATK 4 based open source variant calling workFlow. BMC Bioinformat. 22:402. doi: 10.1186/s12859-021-04317-y
Bortolaia, V., Kaas, R. S., Ruppe, E., Roberts, M. C., Schwarz, S., Cattoir, V., et al. (2020). ResFinder 4.0 for predictions of phenotypes from genotypes. J. Antimicrob. Chemother. 75, 3491–3500. doi: 10.1093/jac/dkaa345
Brown, G. D., Denning, D. W., Gow, N. A. R., Levitz, S. M., Netea, M. G., and White, T. C. (2012). Hidden killers: human fungal infections. Sci. Transl. Med. 4:165rv13. doi: 10.1126/scitranslmed.3004404
Bueid, A., Howard, S. J., Moore, C. B., Richardson, M. D., Harrison, E., Bowyer, P., et al. (2010). Azole antifungal resistance in Aspergillus fumigatus: 2008 and 2009. J. Antimicrob. Chemother. 65, 2116–2118. doi: 10.1093/jac/dkq279
Cannon, R. D., Lamping, E., Holmes, A. R., Niimi, K., Baret, P. V., Keniya, M. V., et al. (2009). Efflux-mediated antifungal drug resistance. Clin. Microbiol. Rev. 22, 291–321. doi: 10.1128/CMR.00051-08
Chamilos, G., Lewis, R. E., and Kontoyiannis, D. P. (2008). Delaying amphotericin B-based frontline therapy significantly increases mortality among patients with hematologic malignancy who have zygomycosis. Clin. Infect. Dis. Off. Publ. Infect. Dis. Soc. Am. 47, 503–509. doi: 10.1086/590004
Chow, N. A., Muñoz, J. F., Gade, L., Berkow, E. L., Li, X., Welsh, R. M., et al. (2020). Tracing the evolutionary history and global expansion of Candida auris using population genomic analyses. MBio :e03364–19:11. doi: 10.1128/mBio.03364-19
Chowdhary, A., Prakash, A., Sharma, C., Kordalewska, M., Kumar, A., Sarma, S., et al. (2018). A multicentre study of antifungal susceptibility patterns among 350 Candida auris isolates (2009-17) in India: role of the ERG11 and FKS1 genes in azole and echinocandin resistance. J. Antimicrob. Chemother. 73, 891–899. doi: 10.1093/jac/dkx480
Cingolani, P. (2022). Variant annotation and functional prediction: SnpEff. Methods Mol. Biol. Clifton NJ 2493, 289–314. doi: 10.1007/978-1-0716-2293-3_19
Galaxy Community (2022). The galaxy platform for accessible, reproducible and collaborative biomedical analyses: 2022 update. Nucleic Acids Res. 50, W345–W351. doi: 10.1093/nar/gkac247
Cowen, L. E. (2008). The evolution of fungal drug resistance: modulating the trajectory from genotype to phenotype. Nat. Rev. Microbiol. 6, 187–198. doi: 10.1038/nrmicro1835
Cowen, L. E., Sanglard, D., Howard, S. J., Rogers, P. D., and Perlin, D. S. (2014). Mechanisms of antifungal drug resistance. Cold Spring Harb. Perspect. Med. 5:a019752. doi: 10.1101/cshperspect.a019752
de Man, T. J. B., and Limbago, B. M. (2016). SSTAR, a stand-alone easy-to-use antimicrobial resistance gene predictor. mSphere 1:e00050-15. doi: 10.1128/mSphere.00050-15
Delaloye, J., and Calandra, T. (2014). Invasive candidiasis as a cause of sepsis in the critically ill patient. Virulence 5, 161–169. doi: 10.4161/viru.26187
Deurenberg, R. H., Bathoorn, E., Chlebowicz, M. A., Couto, N., Ferdous, M., García-Cobos, S., et al. (2017). Application of next generation sequencing in clinical microbiology and infection prevention. J. Biotechnol. 243, 16–24. doi: 10.1016/j.jbiotec.2016.12.022
Dunyach, C., Drakulovski, P., Bertout, S., Jouvert, S., Reynes, J., and Mallié, M. (2011). Fungicidal activity and morphological alterations of Candida albicans induced by echinocandins: study of strains with reduced caspofungin susceptibility. Mycoses 54, e62–e68. doi: 10.1111/j.1439-0507.2009.01834.x
Durand, C., Maubon, D., Cornet, M., Wang, Y., Aldebert, D., and Garnaud, C. (2021). Can we improve antifungal susceptibility testing? Front. Cell. Infect. Microbiol. 11:720609. doi: 10.3389/fcimb.2021.720609
Ferrari, S., Ischer, F., Calabrese, D., Posteraro, B., Sanguinetti, M., Fadda, G., et al. (2009). Gain of function mutations in CgPDR1 of Candida glabrata not only mediate antifungal resistance but also enhance virulence. PLoS Pathog. 5:e1000268. doi: 10.1371/journal.ppat.1000268
Fisher, M. C., Alastruey-Izquierdo, A., Berman, J., Bicanic, T., Bignell, E. M., Bowyer, P., et al. (2022). Tackling the emerging threat of antifungal resistance to human health. Nat. Rev. Microbiol. 20, 557–571. doi: 10.1038/s41579-022-00720-1
Garcia-Effron, G., Katiyar, S. K., Park, S., Edlind, T. D., and Perlin, D. S. (2008). A naturally occurring proline-to-alanine amino acid change in Fks1p in Candida parapsilosis, Candida orthopsilosis, and Candida metapsilosis accounts for reduced echinocandin susceptibility. Antimicrob. Agents Chemother. 52, 2305–2312. doi: 10.1128/AAC.00262-08
Garcia-Effron, G., Park, S., and Perlin, D. S. (2009). Correlating echinocandin MIC and kinetic inhibition of fks1 mutant glucan synthases for Candida albicans: implications for interpretive breakpoints. Antimicrob. Agents Chemother. 53, 112–122. doi: 10.1128/AAC.01162-08
Garey, K. W., Rege, M., Pai, M. P., Mingo, D. E., Suda, K. J., Turpin, R. S., et al. (2006). Time to initiation of fluconazole therapy impacts mortality in patients with candidemia: a multi-institutional study. Clin. Infect. Dis. Off. Publ. Infect. Dis. Soc. Am. 43, 25–31. doi: 10.1086/504810
Garnaud, C., Botterel, F., Sertour, N., Bougnoux, M.-E., Dannaoui, E., Larrat, S., et al. (2015). Next-generation sequencing offers new insights into the resistance of Candida spp. to echinocandins and azoles. J. Antimicrob. Chemother. 70, 2556–2565. doi: 10.1093/jac/dkv139
Gaur, N. A., Puri, N., Karnani, N., Mukhopadhyay, G., Goswami, S. K., and Prasad, R. (2004). Identification of a negative regulatory element which regulates basal transcription of a multidrug resistance gene CDR1 of Candida albicans. FEMS Yeast Res. 4, 389–399. doi: 10.1016/S1567-1356(03)00204-6
Gonçalves, S. S., Souza, A. C. R., Chowdhary, A., Meis, J. F., and Colombo, A. L. (2016). Epidemiology and molecular mechanisms of antifungal resistance in Candida and Aspergillus. Mycoses 59, 198–219. doi: 10.1111/myc.12469
Gostinčar, C. (2020). Towards genomic criteria for delineating fungal species. J. Fungi Basel Switz. 6:246. doi: 10.3390/jof6040246
Gow, N. A. R., Johnson, C., Berman, J., Coste, A. T., Cuomo, C. A., Perlin, D. S., et al. (2022). The importance of antimicrobial resistance in medical mycology. Nat. Commun. 13:5352. doi: 10.1038/s41467-022-32249-5
Gupta, S. K., Padmanabhan, B. R., Diene, S. M., Lopez-Rojas, R., Kempf, M., Landraud, L., et al. (2014). ARG-ANNOT, a new bioinformatic tool to discover antibiotic resistance genes in bacterial genomes. Antimicrob. Agents Chemother. 58, 212–220. doi: 10.1128/AAC.01310-13
Healey, K. R., Zhao, Y., Perez, W. B., Lockhart, S. R., Sobel, J. D., Farmakiotis, D., et al. (2016). Prevalent mutator genotype identified in fungal pathogen Candida glabrata promotes multi-drug resistance. Nat. Commun. 7:11128. doi: 10.1038/ncomms11128
Hu, M., Li, Z., Li, D., Zhao, J., Chen, Y., Wang, Z., et al. (2021). Long terminal repeat Retrotransposon Afut4 promotes azole resistance of Aspergillus fumigatus by enhancing the expression of sac1 gene. Antimicrob. Agents Chemother. 65:e0029121. doi: 10.1128/AAC.00291-21
Kapitonov, V. V., and Jurka, J. (2008). A universal classification of eukaryotic transposable elements implemented in Repbase. Nat. Rev. Genet. 9, 411–412. doi: 10.1038/nrg2165-c1
Kohlenberg, A., Monnet, D. L., and Plachouras, D. (2022). Increasing number of cases and outbreaks caused by Candida auris in the EU/EEA, 2020 to 2021. Euro Surveill. Bull. Eur. Sur Mal. Transm. Eur. Commun. Dis. Bull. 27:2200846. doi: 10.2807/1560-7917.ES.2022.27.46.2200846
Ksiezopolska, E., Schikora-Tamarit, M. À., Beyer, R., Nunez-Rodriguez, J. C., Schüller, C., and Gabaldón, T. (2021). Narrow mutational signatures drive acquisition of multidrug resistance in the fungal pathogen Candida glabrata. Curr. Biol. CB 31, 5314–5326.e10. doi: 10.1016/j.cub.2021.09.084
Lamoth, F., Lockhart, S. R., Berkow, E. L., and Calandra, T. (2018). Changes in the epidemiological landscape of invasive candidiasis. J. Antimicrob. Chemother. 73, i4–i13. doi: 10.1093/jac/dkx444
Leonardelli, F., Theill, L., Nardin, M. E., Macedo, D., Dudiuk, C., Mendez, E., et al. (2017). First itraconazole resistant Aspergillus fumigatus clinical isolate harbouring a G54E substitution in Cyp51Ap in South America. Rev. Iberoam. Micol. 34, 46–48. doi: 10.1016/j.riam.2016.05.005
Leprohon, P., Fernandez-Prada, C., Gazanion, É., Monte-Neto, R., and Ouellette, M. (2015). Drug resistance analysis by next generation sequencing in Leishmania. Int. J. Parasitol. Drugs Drug Resist. 5, 26–35. doi: 10.1016/j.ijpddr.2014.09.005
Lipworth, S., Pickford, H., Sanderson, N., Chau, K. K., Kavanagh, J., Barker, L., et al. (2020). Optimized use of Oxford nanopore flowcells for hybrid assemblies. Microb. Genomics 6:mgen000453. doi: 10.1099/mgen.0.000453
Liu, B., and Pop, M. (2009). ARDB--antibiotic resistance genes database. Nucleic Acids Res. 37, D443–D447. doi: 10.1093/nar/gkn656
Logan, C., Martin-Loeches, I., and Bicanic, T. (2020). Invasive candidiasis in critical care: challenges and future directions. Intensive Care Med. 46, 2001–2014. doi: 10.1007/s00134-020-06240-x
Lupetti, A., Danesi, R., Campa, M., Del Tacca, M., and Kelly, S. (2002). Molecular basis of resistance to azole antifungals. Trends Mol. Med. 8, 76–81. doi: 10.1016/s1471-4914(02)02280-3
Maenchantrarath, C., Khumdee, P., Samosornsuk, S., Mungkornkaew, N., and Samosornsuk, W. (2022). Investigation of fluconazole susceptibility to Candida albicans by MALDI-TOF MS and real-time PCR for CDR1, CDR2, MDR1 and ERG11. BMC Microbiol. 22:153. doi: 10.1186/s12866-022-02564-4
Manoharlal, R., Gorantala, J., Sharma, M., Sanglard, D., and Prasad, R. (2010). PAP1 [poly(a) polymerase 1] homozygosity and hyperadenylation are major determinants of increased mRNA stability of CDR1 in azole-resistant clinical isolates of Candida albicans. Microbiol. Read. Engl. 156, 313–326. doi: 10.1099/mic.0.035154-0
McTaggart, L. R., Cabrera, A., Cronin, K., and Kus, J. V. (2020). Antifungal susceptibility of clinical yeast isolates from a large Canadian reference laboratory and application of whole-genome sequence analysis to elucidate mechanisms of acquired resistance. Antimicrob. Agents Chemother. 64:e00402-20. doi: 10.1128/AAC.00402-20
Muñoz, J. F., Gade, L., Chow, N. A., Loparev, V. N., Juieng, P., Berkow, E. L., et al. (2018). Genomic insights into multidrug-resistance, mating and virulence in Candida auris and related emerging species. Nat. Commun. 9:5346. doi: 10.1038/s41467-018-07779-6
Nash, A., Sewell, T., Farrer, R. A., Abdolrasouli, A., Shelton, J. M. G., Fisher, M. C., et al. (2018). MARDy: mycology antifungal resistance database. Bioinforma. Oxf. Engl. 34, 3233–3234. doi: 10.1093/bioinformatics/bty321
Nattestad, M., and Schatz, M. C. (2016). Assemblytics: a web analytics tool for the detection of variants from an assembly. Bioinforma. Oxf. Engl. 32, 3021–3023. doi: 10.1093/bioinformatics/btw369
Oliveira, L. V. N., Wang, R., Specht, C. A., and Levitz, S. M. (2021). Vaccines for human fungal diseases: close but still a long way to go. NPJ Vaccines 6:33. doi: 10.1038/s41541-021-00294-8
Orta-Zavalza, E., Guerrero-Serrano, G., Gutiérrez-Escobedo, G., Cañas-Villamar, I., Juárez-Cepeda, J., Castaño, I., et al. (2013). Local silencing controls the oxidative stress response and the multidrug resistance in Candida glabrata. Mol. Microbiol. 88, 1135–1148. doi: 10.1111/mmi.12247
Perlin, D. S., Rautemaa-Richardson, R., and Alastruey-Izquierdo, A. (2017). The global problem of antifungal resistance: prevalence, mechanisms, and management. Lancet Infect. Dis. 17, e383–e392. doi: 10.1016/S1473-3099(17)30316-X
Pfaller, M. A., Castanheira, M., Lockhart, S. R., Ahlquist, A. M., Messer, S. A., and Jones, R. N. (2012). Frequency of decreased susceptibility and resistance to echinocandins among fluconazole-resistant bloodstream isolates of Candida glabrata. J. Clin. Microbiol. 50, 1199–1203. doi: 10.1128/JCM.06112-11
Pham, C. D., Iqbal, N., Bolden, C. B., Kuykendall, R. J., Harrison, L. H., Farley, M. M., et al. (2014). Role of FKS mutations in Candida glabrata: MIC values, echinocandin resistance, and multidrug resistance. Antimicrob. Agents Chemother. 58, 4690–4696. doi: 10.1128/AAC.03255-14
Pristov, K. E., and Ghannoum, M. A. (2019). Resistance of Candida to azoles and echinocandins worldwide. Clin. Microbiol. Infect. Off. Publ. Eur. Soc. Clin. Microbiol. Infect. Dis. 25, 792–798. doi: 10.1016/j.cmi.2019.03.028
Raven, K., Blane, B., Churcher, C., Parkhill, J., and Peacock, S. J. (2018). Are commercial providers a viable option for clinical bacterial sequencing? Microbe. Genomics 4:e000173. doi: 10.1099/mgen.0.000173
Rhodes, J., Abdolrasouli, A., Dunne, K., Sewell, T. R., Zhang, Y., Ballard, E., et al. (2022). Population genomics confirms acquisition of drug-resistant Aspergillus fumigatus infection by humans from the environment. Nat. Microbiol. 7, 663–674. doi: 10.1038/s41564-022-01091-2
Rice, L. B. (2008). Federal funding for the study of antimicrobial resistance in nosocomial pathogens: no ESKAPE. J. Infect. Dis. 197, 1079–1081. doi: 10.1086/533452
Rivero-Menendez, O., Navarro-Rodriguez, P., Bernal-Martinez, L., Martin-Cano, G., Lopez-Perez, L., Sanchez-Romero, I., et al. (2019). Clinical and laboratory development of Echinocandin resistance in Candida glabrata: molecular characterization. Front. Microbiol. 10:1585. doi: 10.3389/fmicb.2019.01585
Sasse, C., Dunkel, N., Schäfer, T., Schneider, S., Dierolf, F., Ohlsen, K., et al. (2012). The stepwise acquisition of fluconazole resistance mutations causes a gradual loss of fitness in Candida albicans. Mol. Microbiol. 86, 539–556. doi: 10.1111/j.1365-2958.2012.08210.x
Scaria, J., Chandramouli, U., and Verma, S. K. (2005). Antibiotic resistance genes online (ARGO): a database on vancomycin and beta-lactam resistance genes. Bioinformation 1, 5–7. doi: 10.6026/97320630001005
Schikora-Tamarit, M. À., and Gabaldón, T. (2022a). PerSVade: personalized structural variant detection in any species of interest. Genome Biol. 23:175. doi: 10.1186/s13059-022-02737-4
Schikora-Tamarit, M. À., and Gabaldón, T. (2022b). Using genomics to understand the mechanisms of virulence and drug resistance in fungal pathogens. Biochem. Soc. Trans. 50, 1259–1268. doi: 10.1042/BST20211123
Selmecki, A., Forche, A., and Berman, J. (2006). Aneuploidy and isochromosome formation in drug-resistant Candida albicans. Science 313, 367–370. doi: 10.1126/science.1128242
Selmecki, A., Gerami-Nejad, M., Paulson, C., Forche, A., and Berman, J. (2008). An isochromosome confers drug resistance in vivo by amplification of two genes, ERG11 and TAC1. Mol. Microbiol. 68, 624–641. doi: 10.1111/j.1365-2958.2008.06176.x
Shields, R. K., Nguyen, M. H., Press, E. G., Kwa, A. L., Cheng, S., Du, C., et al. (2012). The presence of an FKS mutation rather than MIC is an independent risk factor for failure of echinocandin therapy among patients with invasive candidiasis due to Candida glabrata. Antimicrob. Agents Chemother. 56, 4862–4869. doi: 10.1128/AAC.00027-12
Snelders, E., Camps, S. M. T., Karawajczyk, A., Schaftenaar, G., Kema, G. H. J., van der Lee, H. A., et al. (2012). Triazole fungicides can induce cross-resistance to medical triazoles in Aspergillus fumigatus. PLoS One 7:e31801. doi: 10.1371/journal.pone.0031801
Snelders, E., van der Lee, H. A. L., Kuijpers, J., Rijs, A. J. M. M., Varga, J., Samson, R. A., et al. (2008). Emergence of azole resistance in Aspergillus fumigatus and spread of a single resistance mechanism. PLoS Med. 5:e219. doi: 10.1371/journal.pmed.0050219
Spettel, K., Barousch, W., Makristathis, A., Zeller, I., Nehr, M., Selitsch, B., et al. (2019). Analysis of antifungal resistance genes in Candida albicans and Candida glabrata using next generation sequencing. PLoS One 14:e0210397. doi: 10.1371/journal.pone.0210397
Tacconelli, E., Carrara, E., Savoldi, A., Harbarth, S., Mendelson, M., Monnet, D. L., et al. (2018). Discovery, research, and development of new antibiotics: the WHO priority list of antibiotic-resistant bacteria and tuberculosis. Lancet Infect. Dis. 18, 318–327. doi: 10.1016/S1473-3099(17)30753-3
Taylor, J. W., and Fisher, M. C. (2003). Fungal multilocus sequence typing--it’s not just for bacteria. Curr. Opin. Microbiol. 6, 351–356. doi: 10.1016/s1369-5274(03)00088-2
Trevijano-Contador, N., Torres-Cano, A., Carballo-González, C., Puig-Asensio, M., Martín-Gómez, M. T., Jiménez-Martínez, E., et al. (2022). Global emergence of resistance to fluconazole and Voriconazole in Candida parapsilosis in tertiary hospitals in Spain during the COVID-19 pandemic. Open Forum Infect. Dis. 9:ofac605. doi: 10.1093/ofid/ofac605
Tscherner, M., Zwolanek, F., Jenull, S., Sedlazeck, F. J., Petryshyn, A., Frohner, I. E., et al. (2015). The Candida albicans histone Acetyltransferase Hat1 regulates stress resistance and virulence via distinct chromatin assembly pathways. PLoS Pathog. 11:e1005218. doi: 10.1371/journal.ppat.1005218
Uddin, W., Dhabalia, D., Prakash, S. M. U., and Kabir, M. A. (2021). Systematic truncations of chromosome 4 and their responses to antifungals in Candida albicans. J. Genet. Eng. Biotechnol. 19:92. doi: 10.1186/s43141-021-00197-0
Van Rhijn, N., Bromley, M., Richardson, M., and Bowyer, P. (2021). CYP51 Paralogue structure is associated with intrinsic azole resistance in fungi. MBio 12:e0194521. doi: 10.1128/mBio.01945-21
Vella, A., De Carolis, E., Mello, E., Perlin, D. S., Sanglard, D., Sanguinetti, M., et al. (2017). Potential use of MALDI-ToF mass spectrometry for rapid detection of antifungal resistance in the human pathogen Candida glabrata. Sci. Rep. 7:9099. doi: 10.1038/s41598-017-09329-4
Weber, M., Schaer, J., Walther, G., Kaerger, K., Steinmann, J., Rath, P.-M., et al. (2018). FunResDB-A web resource for genotypic susceptibility testing of Aspergillus fumigatus. Med. Mycol. 56, 117–120. doi: 10.1093/mmy/myx015
Wu, Y., Grossman, N., Totten, M., Memon, W., Fitzgerald, A., Ying, C., et al. (2020). Antifungal susceptibility profiles and drug resistance mechanisms of clinical Lomentospora prolificans isolates. Antimicrob. Agents Chemother. 64:e00318-20. doi: 10.1128/AAC.00318-20
Yang, F., Kravets, A., Bethlendy, G., Welle, S., and Rustchenko, E. (2013). Chromosome 5 monosomy of Candida albicans controls susceptibility to various toxic agents, including major antifungals. Antimicrob. Agents Chemother. 57, 5026–5036. doi: 10.1128/AAC.00516-13
Keywords: amphotericin B, antimicrobial target, Aspergillus, azoles, Candida, Cryptococcus, echinocandins, polyenes
Citation: Alastruey-Izquierdo A and Martín-Galiano AJ (2023) The challenges of the genome-based identification of antifungal resistance in the clinical routine. Front. Microbiol. 14:1134755. doi: 10.3389/fmicb.2023.1134755
Received: 30 December 2022; Accepted: 05 April 2023;
Published: 20 April 2023.
Edited by:
Florent Morio, Université de Nantes, FranceReviewed by:
Antonella Lupetti, University of Pisa, ItalyCopyright © 2023 Alastruey-Izquierdo and Martín-Galiano. This is an open-access article distributed under the terms of the Creative Commons Attribution License (CC BY). The use, distribution or reproduction in other forums is permitted, provided the original author(s) and the copyright owner(s) are credited and that the original publication in this journal is cited, in accordance with accepted academic practice. No use, distribution or reproduction is permitted which does not comply with these terms.
*Correspondence: Ana Alastruey-Izquierdo, YW5hYWxhc3RydWV5QGlzY2lpaS5lcw==; Antonio J. Martín-Galiano, bWdhbGlhbm9AaXNjaWlpLmVz
Disclaimer: All claims expressed in this article are solely those of the authors and do not necessarily represent those of their affiliated organizations, or those of the publisher, the editors and the reviewers. Any product that may be evaluated in this article or claim that may be made by its manufacturer is not guaranteed or endorsed by the publisher.
Research integrity at Frontiers
Learn more about the work of our research integrity team to safeguard the quality of each article we publish.