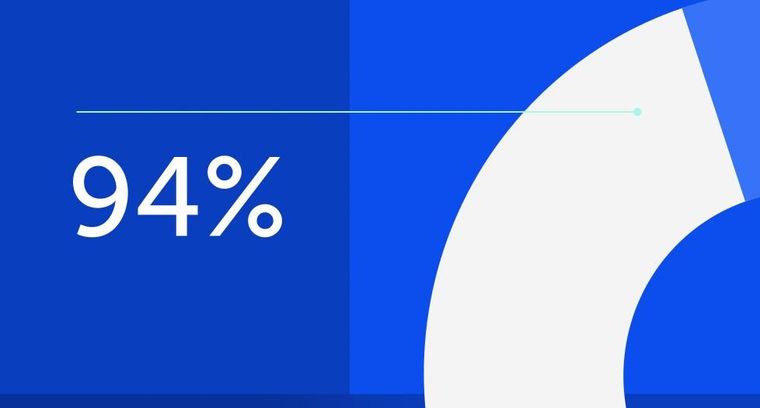
94% of researchers rate our articles as excellent or good
Learn more about the work of our research integrity team to safeguard the quality of each article we publish.
Find out more
ORIGINAL RESEARCH article
Front. Microbiol., 10 May 2023
Sec. Terrestrial Microbiology
Volume 14 - 2023 | https://doi.org/10.3389/fmicb.2023.1128853
This article is part of the Research TopicSoil Microbiome Community and Functional Succession Mechanism Driven by Different Factors in Agricultural EcologyView all 43 articles
Introduction: The composition and stability of soil fungal network are important for soil function, but the effect of trifluralin on network complexity and stability is not well understood.
Methods: In this study, two agricultural soils were used to test the impact of trifluralin on a fungal network. The two soils were treated with trifluralin (0, 0.84, 8.4, and 84 mg kg−1) and kept in artificial weather boxes.
Results and discussion: Under the impact of trifluralin, the fungal network nodes, edges, and average degrees were increased by 6–45, 134–392, and 0.169–1.468 in the two soils, respectively; however, the average path length was decreased by 0.304–0.70 in both soils. The keystone nodes were also changed in trifluralin treatments in the two soils. In the two soils, trifluralin treatments shared 219–285 nodes and 16–27 links with control treatments, and the network dissimilarity was 0.98–0.99. These results indicated that fungal network composition was significantly influenced. After trifluralin treatment, fungal network stability was increased. Specifically, the network robustness was increased by trifluralin with 0.002–0.009, and vulnerability was decreased by trifluralin with 0.0001–0.00032 in the two soils. Fungal network community functions were also impacted by trifluralin in both soils. Trifluralin significantly impacts the fungal network.
Microorganisms are important for soil substance and energy cycling. In a soil ecosystem, microbial species connect as an organic entity and connect with others through positive, negative, and neutral relationships (Faust and Raes, 2012; Coyte et al., 2015). In these complicated relationships, soil microorganisms perform functions of mineral and energy management and nutrient cycling (Montoya et al., 2006; Glaze et al., 2022). Therefore, interactions between microbes are vital for maintaining homeostasis in soil processes. The network has been increasingly used in soil ecology to evaluate complicated relationships of microbial species (Berry and Widder, 2014; Przulj and Malod-Dognin, 2016). For example, Wu reported that permafrost degradation reduced microbial network stability and increased carbon loss (Wu et al., 2021); Shen et al. analyzed the impact of plant diversity on soil fungal network stability and functions (Shen et al., 2022).
Pesticide is the most common means of agricultural production. However, it is also a disturbance factor in soil microbial connections (Du et al., 2022). Previous studies mostly focused on the topological indexes impacted by pesticides (Gao et al., 2018; Xun et al., 2021; Su et al., 2022), but the topological indexes are based on mathematical theory (Diestel, 2000), and this limited researchers in further understanding the impact of pesticides on the microbial network. Understanding the changed nodes in the network composition has important implications for soil community functions (Xun et al., 2021). In addition, the changed node, edge, and network dissimilarity are important for evaluating network changes (Poisot et al., 2012). Network stability is also an important network index, and it has been used to evaluate the resistance of the network to disturbance (Thebault and Fontaine, 2010; Yuan et al., 2021). However, no research has been carried out concerning node persistence, network composition, and network stability in pesticide-polluted soils based on the present literature, which limits researchers' understanding of the effect(s) of pesticides on the stability of soil microbial networks.
Trifluralin is a fluorinated dinitroaniline compound that has been used as a pre-emergence herbicide on cotton, alfalfa, and soybeans (Zhang, 2018). Approximately 4,400 tons is applied per year (Maggi et al., 2019), and the half-life is more than 375 days in soil (Karasali et al., 2017). Previous studies reported that trifluralin can influence soil microbial communities (Du et al., 2018). However, there is little understanding of the influence of trifluralin on the fungal network. In this study, we carried out a 3-month indoor experiment to evaluate the influence of trifluralin on the fungal network. Fungal network complexity, dissimilarity, stability, and related functions were analyzed to evaluate network changes. Network complexity includes the number of nodes and links, average degree of nodes, density, and clustering coefficient of a network. Stability evaluates the network resistance to interference, which has been estimated by robustness and vulnerability (Deng et al., 2012; Yuan et al., 2021). Microbial network dissimilarity was used to evaluate two network differences on the basis of shared nodes and edges (Poisot et al., 2012; Mo et al., 2021). Our aim was to clarify whether trifluralin could influence fungal network composition and stability.
A total of two soil samples were collected from the Langfang research base, Hebei Province (LF), and the Jiansanjiang reclamation area, Heilongjiang Province (JSJ). Based on soil particle diameter, soils from LF and JSJ were classified as silty loam soil and silty clay soil, respectively. In the LF soil, the content of organic matter, available P, available K, and pH were 25.8 g kg−1, 51.7 mg kg−1, 289 mg kg−1, and 7.24 mg kg−1, respectively. In JSJ soil, the content of organic matter, available P, available K, and pH were 18.0 g kg−1, 74.9 mg kg−1, 289.8 mg kg−1, and 7.07 mg kg−1, respectively. A 2-mm mesh was used to sieve the soils, and the soils were preincubated for 2 weeks (Trabue et al., 2006). Trifluralin purity was 98%, and it was dissolved in acetone (analytical grade; Beijing Chemical Company). In total, three concentrations of trifluralin in soils were used. The active ingredients of trifluralin in 1 kg of dry soil were 0.84, 8.4, and 84 mg, and they were corresponding to 1 (L), 10 (M), and 100 (H) times of the recommended application rate, respectively. The M level represents excessive use of pesticides in the field, and the H level represents extremely contaminated soil by pesticides (e.g., soil near a pesticide factory). The procedure of pesticide exposure is as follows: 50 g of soil and 100 μL pesticide solution were added to the dark brown bottles, and then thoroughly mixed for 15 min; after that, 200 g of soil was transferred to each bottle and thoroughly mixed for 15 min. A control treatment was also needed and this consisted of treatment with a solution lacking trifluralin. Each treatment was repeated three times. The soil concentration was 1.5 g cm−3 (GB/T31270.1-2014, 2014). Soil moister was kept at 50% using deionized water. The weighing method was used to determine the loss of water every 2 days, and the soil moisture was kept constant according to the loss of weight. The laboratory experiment was carried out for 3 months in an artificial climate box at 25°C. Sampling times were 7, 15, 30, 60, and 90 days after experimental establishment. The samples were stored at −80°C until analysis.
A PowerSoil Isolation Kit (Mo Bio Laboratories, Carlsbad, CA, USA) was used to extract soil microbial DNA. An ND-1000 spectrophotometer (NanoDrop Technologies) was used to analyze microbial DNA quality. The forward primer ITS3_KYO2 (5′-GATGAAGAACGYAGYRAA-3′) and reverse primer ITS4 (5′-TCCTCCGCTTATTGATATGC-3′) were used to amplify internally transcribed spacer (ITS; Tian et al., 2017). Microbial DNA was amplified using a PCR amplifier, and each amplification system contained 1.5 μL of each 10 μM primer, 100–300 ng DNA template, 5 μL of 2 mM dNTPs, 1 μL KOD-Plus-Neo enzyme (Toyobo, Shanghai, China), 5 μL of 10× PCR buffer for KOD-Plus-Neo, 3 μL of 25 mM MgSO4, and water to 50 μL. The temperature change steps were as follows: 94°C for 2 min, followed by 98°C for 10 s (for 35 cycles), 62°C for 30 s, and 68°C for 30 s, and the final extension temperature was 68°C for 10 min. Negative control with DNA solution was also settled. A PCR Purification Kit (Qiagen, Hilden, Germany) was used to purify PCR products after 1.5% agarose gel electrophoresis. An Illumina platform (Santiago, CA, United States) was used to sequence the purified PCR products using a 2 × 250 bp kit. USEARCH was used to process amplicon sequencing data (Edgar, 2010, 2013). The following rules were used to filter raw reads: (1) adaptors were cut, (2) reads which included more than 10% of unknown nucleotides were removed, and (3) reads that included < 80% of bases with quality (Q-value) > 20 were removed. Tags were assembled with clean reads according to more than 10 bp overlaps and <2% mismatch between paired-end reads. Clean data were clustered into operational taxonomic units (OTUs) with a similarity of 97%.
All co-occurrence networks were established on the basis of Pearson correlations of OTU abundances and performed on the Cytoscape platform using the CoNet plugin (Faust and Raes, 2016). Pearson's correlation was used to analyze the association of pairwise fungal OTUs with an absolute value of correlation coefficient (r) higher than 0.7. The topological indices were calculated using Gephi software include total nodes, total edges, average degrees, clustering coefficient, network density, and path length. Nodes in the network represent the OTUs in the network. The degrees of each node represents the connections of a node to others, and the average degree represents the main value of all degrees. Modularity based on the connections of nodes represents the level of a network divided into different modules. A network diagram was established on Gephi software.
The node's topological role was evaluated by among-module connectivity (Pi) and within-module connectivity (Zi; Guimerà and Nunes Amaral, 2005). The network nodes were classified as module hubs with Zi ≥ 2.5 and Pi < 0.62, connectors with Zi < 2.5 and Pi ≥ 0.62, and network hubs with Zi ≥ 2.5 and Pi ≥ 0.62 (Olesen et al., 2007; Chen et al., 2019). These three categories of nodes are referred to as keystone nodes (Banerjee et al., 2019; Röttjers and Faust, 2019). Upset plots were used to visualize shared keystone nodes between control and trifluralin treatment (Lex et al., 2014).
Network stability can be used to evaluate ecological system stability to disturbance (Thebault and Fontaine, 2010). Generally, it is usually evaluated by network robustness and vulnerability (Wu et al., 2021; Yuan et al., 2021). Robustness is the remaining proportion of nodes in the network after removing some nodes (Montesinos-Navarro et al., 2017). In this study, 0.05% of nodes in the network have been removed to simulate random species removal each time. Vulnerability is also an index used to evaluate network stability based on node removal (Yuan et al., 2021).
Network dissimilarity was used to evaluate the dissimilarity of two fungal networks in this study (Poisot et al., 2012; Mo et al., 2021). It was evaluated by shared nodes and edges of two different networks, and the shared nodes and edges were also used to evaluate dissimilarity (Poisot et al., 2012; Mo et al., 2021).
FUNGuild is a database of fungal functions, and it clusters almost all published studies on fungal functions (Nguyen et al., 2016). Based on fungal amplicon sequencing data and taxonomy, FUNGuild can be used to predict fungal functions. There are three categories of trophic modes of fungi, namely, pathotroph, saprotroph, and symbiotroph. Furthermore, these three categories can be divided for better evaluation of fungal functions. Saprotroph was divided into dung saprotroph, leaf saprotroph, plant saprotroph, soil saprotroph, and wood saprotroph; pathotroph was divided into animal pathogen, plant pathogen, fungal parasite, lichen parasite, bryophyte parasite, and endophyte; and symbiotroph was divided into ectomycorrhizal, ericoid mycorrhizal, and endophyte. Fungal network OTUs abundance was used to analyze the correlation of fungal community structure with fungal functions based on the mantal test (Duan et al., 2020).
A total of eight fungal networks were established for each treatment based on Pearson's correlation coefficients of fungal OTUs (Figure 1, Table 1) presents each network's topological indexes. In the networks, the nodes were assigned to four fungal phyla in LF soil and three fungal phyla in JSJ soil. Among these, the phyla Ascomycota and Basidiomycota were most abundant in both soils. Compared with the control, the total nodes, total links, and average degree were all increased by trifluralin. In LF soils, the total nodes, total links, and average degrees were increased by 11–45, 252–392, and 1.151–1.468 in trifluralin treatments, respectively; in JSJ soil, the total nodes, total links, and average degrees were increased by 6–29, 134–234, and 0.169–1.227 in trifluralin treatments, respectively. The average path length was decreased by 0.558, 0.304, and 0.70 in L, M, and H treatments in LF soils and by 0.562, 0.373, and 0.415 in L, M, and H treatments in JSJ soils.
Based on nodes' Zi and Pi, there were 93, 111, 86, and 96 keystone nodes in control, L, M, and H treatments in LF soil, respectively; there were 134, 118, 105, and 117 keystone nodes in control, L, M, and H treatments in JSJ soil, respectively. In LF soil, the shared keystone nodes were 35, 23, and 30 for the comparison of control-L, control-M, and control-H, respectively; in JSJ soil, they were 45, 37, and 36 for the comparison of control-L, control-M, and control-H, respectively (Figure 2).
Network dissimilarity is an effective tool to evaluate network similarity. According to Table 2, for the comparison of control-L, control-M, and control-H, the shared nodes were 231, 227, and 219 in LF soil and 285, 272, and 279 in JSJ soil, separately. The shared nodes accounted for a significant part of the total nodes in each network, but the shared links were significantly low for each comparison. Specifically, for the comparison of control-L, control-M, and control-H, shared links were 20, 16, and 27 in LF soil and 19, 26, and 23 in JSJ soil. According to the shared nodes and links, the network composition was significantly influenced by trifluralin. The dissimilarity was 0.97–0.99 between control and trifluralin treatments in both soils.
Table 2. Shared nodes and edges and dissimilarity between different fungal networks in the two soils.
On the basis of random species loss, the network robustness was increased by trifluralin in the two soils (Figure 3). In LF soil, it was increased by 0.009, 0.003, and 0.002 in L, M, and H, respectively. In JSJ soil, it was increased by 0.003, 0.006, and 0.007 in L, M, and H, separately. For vulnerability, it was decreased by trifluralin in both soils (Figure 3). In LF soil, it was decreased by 0.00032, 0.00022, and 0.00014 in L, M, and H, separately. In JSJ soil, it was decreased by 0.0001, 0.00015, and 0.00019 in L, M, and H, separately.
There were 11 guilds identified in this study, and they were dung saprotroph, endophyte, lichen parasite, fungal parasite, plant pathogen, animal pathogen, plant saprotroph, soil saprotroph, wood saprotroph, ectomycorrhizal, and mycorrhizal. The correlations of the fungal network community with functions are shown in Figure 4. In LF soil, the fungal community was significantly correlated with dung saprotroph in the control treatment; in H treatments, the fungal community was significantly correlated with dung saprotroph and wood saprotroph. In JSJ soil, the fungal community was significantly correlated with dung saprotroph in L treatment; in M treatments, the fungal community was significantly correlated with endophyte, lichen parasite, plant pathogen, animal pathogen, soil saprotroph, and wood saprotroph; in H treatment, the fungal community was significantly correlated with dung saprotroph and endophyte.
Figure 4. Relationships between fungal network communities and functions for each treatment in the two soils.
The soil microbial system is an organic entity, and fungi are important in the system. There are complicated relationships among them, which act as decomposers, nutrient moderators, mutualists, C-cycling mediators, and plant pathogens (Tedersoo et al., 2014). Microorganism network is a valuable way to analyze the complicated relationships and the influenced microorganism connection (Berry and Widder, 2014; Przulj and Malod-Dognin, 2016; Mo et al., 2021). Wu et al. (2021) studied the relationship between microbial stability and carbon loss through the network in alpine permafrost degradation. In the study of Mo et al. (2021), the authors found a slight salinity shift in microeukaryotic plankton communities' network stability. In the study of Vries, the fungal community was more stable than the bacterial community in the network (de Vries et al., 2018).
In this study, the increased network complexities in trifluralin treatments indicated that the relationships of fungal species were significantly impacted by trifluralin. In addition, these results also suggested that there were more connections with others caused by trifluralin. Mesosulfuron-methyl also increased the microorganism network average degree and network density in different soils (Du et al., 2021). In addition, the increased positive edges suggested that trifluralin induced relationships with mutualism, commensalism, parasitism, and neutralism predation more than previously reported (Faust and Raes, 2012; Coyte et al., 2015).
The increased network complexities also indicated that fungal network composition and dissimilarity were impacted. The results of shared nodes, shared edges, and dissimilarity between control and trifluralin treatments proved this suggestion. Network dissimilarity was first published by Poisot, and this index has also been used by other researchers to evaluate microbial network differences (Poisot et al., 2012; Mo et al., 2021; Liao et al., 2023). In the study of Liao et al. (2023), network dissimilarity was used to evaluate the difference between marine medaka gut and gill microbial networks; Mo reported that the microeukaryotic plankton networks in different salinity subtropical urban reservoirs were significantly different on the basis of shared nodes, shared edges, and network dissimilarity. These results suggested that fungal network composition was significantly impacted by trifluralin.
Soil microbial network stability is important for functions, ecosystem sustainability, and environmental protection (Coyte et al., 2015; Pan et al., 2023). In this study, fungal network stability was increased by trifluralin with increased robustness and decreased vulnerability in the two soils. These results indicated that the capacity of resisting interference of the fungal network was increased by trifluralin (McCann, 2000), but that also suggested that it was difficult for the fungal network to return to its original state. In addition, these influences also impact network functions. In LF soil, the impact on the correlations of the network community to functions was low. The influenced correlations of the network community to dung saprotroph in L and M treatments suggested that the capacity of decomposing livestock and poultry manure was impacted in LF soil (Hudson, 1984; Cannon and Kirk, 2008). In JSJ soil, the correlated functions were increased by trifluralin, suggesting that the network fungal community could play more functions after trifluralin treatment. Different from the profiles of correlations in LF soil, the fungal community was correlated with dung saprotroph in JSJ soil. These results suggested that the effects of trifluralin on network community functions were different in the two soils. Previous research also showed that fungal functions were sensitive to herbicides (Flores et al., 2014; Chen et al., 2022). Chen reported that Oxathiapiprolin significantly impacted fungal functions in an indoor experiment (Chen et al., 2022). Imazalil, clothianidin, and diazinon also impacted fungi's organic matter processing and energy cycling (Flores et al., 2014; Huang et al., 2021).
In this study network complexities, keystone node, composition, and stability were used to evaluate the impact of trifluralin on soil fungal networks. Trifluralin increased fungal network complexities in two studied soils. Correspondingly, fungal network composition and keystone nodes were also influenced. Fungal network stability was increased by trifluralin in the two soils, with increased robustness and decreased vulnerability. In addition, fungal functions related to network community were also impacted by trifluralin in both soils.
Publicly available datasets were analyzed in this study. This data can be found here: https://www.ncbi.nlm.nih.gov/biosample/?term=pengqiang%20du BioSample: SAMN08721648 to SAMN08721767.
HH and PD conceived and wrote this manuscript. HH, PD, and JH performed the bioinformatics analyses. ZZ, HX, XZ, and CZ revised this manuscript. All authors contributed to the article and approved the submitted version.
This research was funded by the National Natural Science Foundation of China, grant number: 30900443 and the State Key Laboratory for Managing Biotic and Chemical Threats to the Quality and Safety of Agroproducts, grant number: 2010DS700124-KF2008.
The authors declare that the research was conducted in the absence of any commercial or financial relationships that could be construed as a potential conflict of interest.
All claims expressed in this article are solely those of the authors and do not necessarily represent those of their affiliated organizations, or those of the publisher, the editors and the reviewers. Any product that may be evaluated in this article, or claim that may be made by its manufacturer, is not guaranteed or endorsed by the publisher.
Banerjee, S., Schlaeppi, K., and van der Heijden, M. G. A. (2019). Reply to “Can we predict microbial keystones?”. Nat. Rev. Microbiol. 17, 194–194. doi: 10.1038/s41579-018-0133-x
Berry, D., and Widder, S. (2014). Deciphering microbial interactions and detecting keystone species with co-occurrence networks. Front. Microbiol. 5, 219. doi: 10.3389/fmicb.2014.00219
Cannon, P. F., and Kirk, P. M. (2008). Fungal Families of the World [M]. Oxford University Press. doi: 10.1079/9780851998275.0000
Chen, L., Jiang, Y., Liang, C., Luo, Y., Xu, Q., Han, C., et al. (2019). Competitive interaction with keystone taxa induced negative priming under biochar amendments. Microbiome 7, 77. doi: 10.1186/s40168-019-0693-7
Chen, Y., Zhang, F., Huang, B., Wang, J., Huang, H., Song, Z., et al. (2022). Effects of oxathiapiprolin on the structure, diversity and function of soil fungal community. Toxics 10, 548. doi: 10.3390/toxics10090548
Coyte, K. Z., Schluter, J., and Foster, K. R. (2015). The ecology of the microbiome: Networks, competition, and stability. Science 350, 663–666. doi: 10.1126/science.aad2602
de Vries, F. T., Griffiths, R. I., Bailey, M., Craig, H., Girlanda, M., Gweon, H. S., et al. (2018). Soil bacterial networks are less stable under drought than fungal networks. Nat. Commun. 9, 3033. doi: 10.1038/s41467-018-05516-7
Deng, Y., Jiang, Y.-H., Yang, Y., He, Z., Luo, F., and Zhou, J. (2012). Molecular ecological network analyses. BMC Bioinformat. 13, 113. doi: 10.1186/1471-2105-13-113
Du, P., He, H., Wu, X., Xu, J., Dong, F., Liu, X., et al. (2021). Mesosulfuron-methyl influenced biodegradability potential and N transformation of soil. J. Hazard. Mater. 416, 125770. doi: 10.1016/j.jhazmat.2021.125770
Du, P., He, H., Zhou, L., Dong, F., Liu, X., and Zheng, Y. (2022). Different biodegradation potential and the impacted soil functions of epoxiconazole in two soils. J. Hazard. Mater. 422, 126787. doi: 10.1016/j.jhazmat.2021.126787
Du, P., Wu, X., Xu, J., Dong, F., Liu, X., and Zheng, Y. (2018). Effects of trifluralin on the soil microbial community and functional groups involved in nitrogen cycling. J. Hazard. Mater. 353, 204–213. doi: 10.1016/j.jhazmat.2018.04.012
Duan, X. Z., Sun, J. T., Wang, L. T., Shu, X. H., Guo, Y., Keiichiro, M., et al. (2020). Recent infection by Wolbachia alters microbial communities in wild Laodelphax striatellus populations. Microbiome 8, 104. doi: 10.1186/s40168-020-00878-x
Edgar, R. C. (2010). Search and clustering orders of magnitude faster than BLAST. Bioinformatics 26, 2460–2461. doi: 10.1093/bioinformatics/btq461
Edgar, R. C. (2013). UPARSE: Highly accurate OTU sequences from microbial amplicon reads. Nat. Methods 10, 996. doi: 10.1038/nmeth.2604
Faust, K., and Raes, J. (2012). Microbial interactions: From networks to models. Nat. Rev. Microbiol. 10, 538–550. doi: 10.1038/nrmicro2832
Faust, K., and Raes, J. (2016). CoNet app: Inference of biological association networks using Cytoscape. F1000Research 5, 1519–1519. doi: 10.12688/f1000research.9050.1
Flores, L., Banjac, Z., Farré, M., Larrañaga, A., Mas-Martí, E., Muñoz, I., et al. (2014). Effects of a fungicide (imazalil) and an insecticide (diazinon) on stream fungi and invertebrates associated with litter breakdown. Sci. Tot. Environ. 476–477, 532–541. doi: 10.1016/j.scitotenv.2014.01.059
Gao, W., Wu, H., Siddiqui, M. K., and Baig, A. Q. (2018). Study of biological networks using graph theory. Saudi J. Biol. Sci. 25, 1212–1219. doi: 10.1016/j.sjbs.2017.11.022
GB/T31270.1-2014 (2014). Test Guidelines on Environmental Safety Assessment for Chemical Pesticides-Part 16: Soil Microorganism Toxicity Test.
Glaze, T. D., Erler, D. V., and Siljanen, H. M. P. (2022). Microbially facilitated nitrogen cycling in tropical corals. ISME J. 16, 68–77. doi: 10.1038/s41396-021-01038-1
Guimerà, R., and Nunes Amaral, L. A. (2005). Functional cartography of complex metabolic networks. Nature 433, 895–900. doi: 10.1038/nature03288
Huang, W., Lu, Y., Chen, L., Sun, D., and An, Y. (2021). Impact of pesticide/fertilizer mixtures on the rhizosphere microbial community of field-grown sugarcane. Biotech 3, 11. doi: 10.1007/s13205-021-02770-3
Hudson, H. J. (1984). Dung Fungi an illustrated guide to coprophilous fungi in New Zealand. N. Zeal. J. Bot. 22, 593. doi: 10.1080/0028825X.1984.10425296
Karasali, H., Pavlidis, G., Marousopoulou, A., and Ambrus, A. (2017). Occurrence and distribution of trifluralin, ethalfluralin, and pendimethalin in soils used for long-term intensive cotton cultivation in central Greece. J. Environ. Sci. Health B 52, 719–728. doi: 10.1080/03601234.2017.1356678
Lex, A., Gehlenborg, N., Strobelt, H., Vuillemot, R., and Pfister, H. (2014). UpSet: Visualization of intersecting sets. IEEE Trans. Vis. Comput. Graph 20, 1983–1992. doi: 10.1109/TVCG.2014.2346248
Liao, X., Zhao, P., Hou, L., Adyari, B., Xu, E. G., Huang, Q., et al. (2023). Network analysis reveals significant joint effects of microplastics and tetracycline on the gut than the gill microbiome of marine medaka. J. Hazard. Mater. 442, 129996. doi: 10.1016/j.jhazmat.2022.129996
Maggi, F., Tang, F. H. M., la Cecilia, D., and McBratney, A. (2019). PEST-CHEMGRIDS, global gridded maps of the top 20 crop-specific pesticide application rates from 2015 to 2025. Sci. Data 6, 170. doi: 10.1038/s41597-019-0169-4
Mo, Y., Peng, F., Gao, X., Xiao, P., Logares, R., Jeppesen, E., et al. (2021). Low shifts in salinity determined assembly processes and network stability of microeukaryotic plankton communities in a subtropical urban reservoir. Microbiome 9, 128. doi: 10.1186/s40168-021-01079-w
Montesinos-Navarro, A., Hiraldo, F., Tella, J. L., and Blanco, G. (2017). Network structure embracing mutualism-antagonism continuums increases community robustness. Nat. Ecol. Evol. 1, 1661–1669. doi: 10.1038/s41559-017-0320-6
Montoya, J. M., Pimm, S. L., and Sole, R. V. (2006). Ecological networks and their fragility. Nature 442, 259–264. doi: 10.1038/nature04927
Nguyen, N. H., Song, Z., Bates, S. T., Branco, S., Tedersoo, L., Menke, J., et al. (2016). FUNGuild: An open annotation tool for parsing fungal community datasets by ecological guild. Fungal Ecol. 20, 241–248. doi: 10.1016/j.funeco.2015.06.006
Olesen, J. M., Bascompte, J., Dupont, Y. L., and Jordano, P. (2007). The modularity of pollination networks. Proc. Natl. Acad. Sci. U. S. A. 104, 19891–19896. doi: 10.1073/pnas.0706375104
Pan, C., Yu, W., Sun, C., Guo, J., Yu, Y., and Li, X. (2023). Saprotrophic fungi buffer the adverse effects of soil acidification on the soil nutrient supply ability of Chinese fir (Cunninghamia lanceolata) plantations. Eur. J. Soil Biol. 114, 103462. doi: 10.1016/j.ejsobi.2022.103462
Poisot, T., Canard, E., Mouillot, D., Mouquet, N., and Gravel, D. (2012). The dissimilarity of species interaction networks. Ecol. Lett. 15, 1353–1361. doi: 10.1111/ele.12002
Przulj, N., and Malod-Dognin, N. (2016). Network analytics in the age of big data. Science 353, 123–124. doi: 10.1126/science.aah3449
Röttjers, L., and Faust, K. (2019). Can we predict keystones? Nat. Rev. Microbiol. 17, 193. doi: 10.1038/s41579-018-0132-y
Shen, C., Wang, J., Jing, Z., Qiao, N.-H., Xiong, C., and Ge, Y. (2022). Plant diversity enhances soil fungal network stability indirectly through the increase of soil carbon and fungal keystone taxa richness. Sci. Tot. Environ. 818, 151737. doi: 10.1016/j.scitotenv.2021.151737
Su, G., Wang, Y., Ma, B., Deng, F., and Lin, D. (2022). Nanoscale zero-valent iron changes microbial co-occurrence pattern in pentachlorophenol-contaminated soil. J. Hazard. Mater. 438, 129482. doi: 10.1016/j.jhazmat.2022.129482
Tedersoo, L., Bahram, M., Põlme, S., Kõljalg, U., Yorou, N. S., Wijesundera, R., et al. (2014). Global diversity and geography of soil fungi. Science 346, 1256688. doi: 10.1126/science.1256688
Thebault, E., and Fontaine, C. (2010). Stability of ecological communities and the architecture of mutualistic and trophic networks. Science 329, 853–856. doi: 10.1126/science.1188321
Tian, X., Yang, T., He, J., Chu, Q., Jia, X., and Huang, J. (2017). Fungal community and cellulose-degrading genes in the composting process of Chinese medicinal herbal residues. Bioresour. Technol. 241, 374–383. doi: 10.1016/j.biortech.2017.05.116
Trabue, S. L., Palmquist, D. E., Lydick, T. M., and Singles, S. K. (2006). Effects of soil storage on the microbial community and degradation of metsulfuron-methyl. J. Agri. Food Chem. 54, 142–151. doi: 10.1021/jf0512048
Wu, M. H., Chen, S. Y., Chen, J. W., Xue, K., Chen, S. L., Wang, X. M., et al. (2021). Reduced microbial stability in the active layer is associated with carbon loss under alpine permafrost degradation. Proc. Natl. Acad. Sci. U. S. A. 118, 2025321. doi: 10.1073/pnas.2025321118
Xun, W., Liu, Y., Li, W., Ren, Y., Xiong, W., Xu, Z., et al. (2021). Specialized metabolic functions of keystone taxa sustain soil microbiome stability. Microbiome 9, 35. doi: 10.1186/s40168-020-00985-9
Yuan, M. M., Guo, X., Wu, L., Zhang, Y., Xiao, N., Ning, D., et al. (2021). Climate warming enhances microbial network complexity and stability. Nat. Climate Change 11, 343–U100. doi: 10.1038/s41558-021-00989-9
Keywords: trifluralin, fungi, network, dissimilarity, stability
Citation: He H, Huang J, Zhao Z, Xu H, Zheng X, Zhang C and Du P (2023) Fungal network composition and stability in two soils impacted by trifluralin. Front. Microbiol. 14:1128853. doi: 10.3389/fmicb.2023.1128853
Received: 21 December 2022; Accepted: 10 April 2023;
Published: 10 May 2023.
Edited by:
Bruno Tilocca, Magna Græcia University, ItalyReviewed by:
Jianqing Tian, Chinese Academy of Sciences (CAS), ChinaCopyright © 2023 He, Huang, Zhao, Xu, Zheng, Zhang and Du. This is an open-access article distributed under the terms of the Creative Commons Attribution License (CC BY). The use, distribution or reproduction in other forums is permitted, provided the original author(s) and the copyright owner(s) are credited and that the original publication in this journal is cited, in accordance with accepted academic practice. No use, distribution or reproduction is permitted which does not comply with these terms.
*Correspondence: Changpeng Zhang, Y3B6aGFuZzEyMTVAemFhcy5hYy5jbg==; Pengqiang Du, ZHVwZW5ncUAxNjMuY29t
Disclaimer: All claims expressed in this article are solely those of the authors and do not necessarily represent those of their affiliated organizations, or those of the publisher, the editors and the reviewers. Any product that may be evaluated in this article or claim that may be made by its manufacturer is not guaranteed or endorsed by the publisher.
Research integrity at Frontiers
Learn more about the work of our research integrity team to safeguard the quality of each article we publish.