- 1Instituto de Biomedicina y Biotecnología de Cantabria, Universidad de Cantabria—CSIC, Santander, Cantabria, Spain
- 2Department of Systems Biology, Centro Nacional de Biotecnología (CSIC), Madrid, Spain
- 3Interdisciplinary Platform for Sustainable Plastics towards a Circular Economy-Spanish National Research Council (SusPlast-CSIC), Madrid, Spain
Cyanobacteria are prokaryotic organisms that capture energy from sunlight using oxygenic photosynthesis and transform CO2 into products of interest such as fatty acids. Synechococcus elongatus PCC 7942 is a model cyanobacterium efficiently engineered to accumulate high levels of omega-3 fatty acids. However, its exploitation as a microbial cell factory requires a better knowledge of its metabolism, which can be approached by using systems biology tools. To fulfill this objective, we worked out an updated, more comprehensive, and functional genome-scale model of this freshwater cyanobacterium, which was termed iMS837. The model includes 837 genes, 887 reactions, and 801 metabolites. When compared with previous models of S. elongatus PCC 7942, iMS837 is more complete in key physiological and biotechnologically relevant metabolic hubs, such as fatty acid biosynthesis, oxidative phosphorylation, photosynthesis, and transport, among others. iMS837 shows high accuracy when predicting growth performance and gene essentiality. The validated model was further used as a test-bed for the assessment of suitable metabolic engineering strategies, yielding superior production of non-native omega-3 fatty acids such as α-linolenic acid (ALA). As previously reported, the computational analysis demonstrated that fabF overexpression is a feasible metabolic target to increase ALA production, whereas deletion and overexpression of fabH cannot be used for this purpose. Flux scanning based on enforced objective flux, a strain-design algorithm, allowed us to identify not only previously known gene overexpression targets that improve fatty acid synthesis, such as Acetyl-CoA carboxylase and β-ketoacyl-ACP synthase I, but also novel potential targets that might lead to higher ALA yields. Systematic sampling of the metabolic space contained in iMS837 identified a set of ten additional knockout metabolic targets that resulted in higher ALA productions. In silico simulations under photomixotrophic conditions with acetate or glucose as a carbon source boosted ALA production levels, indicating that photomixotrophic nutritional regimens could be potentially exploited in vivo to improve fatty acid production in cyanobacteria. Overall, we show that iMS837 is a powerful computational platform that proposes new metabolic engineering strategies to produce biotechnologically relevant compounds, using S. elongatus PCC 7942 as non-conventional microbial cell factory.
1. Introduction
Cyanobacteria are promising host organisms for the production of compounds with biotechnological applications (Santos-Merino et al., 2019). Their ability to utilize solar energy to fix CO2 makes them particularly attractive, especially in an era where the urge of development of sustainable biotechnological processes has gained an increased attention (Rajneesh et al., 2017). As bioproduction platforms, cyanobacteria offer several advantages when compared to plants and algae, such as higher photosynthetic efficiencies (Zahra et al., 2020) and ease of genetic manipulation (Berla et al., 2013). The model cyanobacterium Synechococcus elongatus PCC 7942 has been widely explored as a cell factory to produce several value-added compounds, including 2,3-butanediol (Oliver et al., 2013; Nozzi and Atsumi, 2015) and omega-3 fatty acids (Santos-Merino et al., 2018, 2022), among others.
Cyanobacteria are able to naturally produce short-chain omega-3 fatty acids, such as alpha-linolenic acid (ALA) and stearidonic acid (SDA). In the quest to find more sustainable, suitable, and economically viable hosts for the production of omega-3 fatty acids, cyanobacteria, and microalgae have emerged as alternative organisms to native (i.e., fish and plant oils and oleaginous microorganisms) and non-native sources (e.g., genetic engineering organisms) (Galán et al., 2019; Patel et al., 2020). By contrast, cyanobacteria are preferred organisms over microalgae due to their small genomes that generally facilitates manipulation and the availability of a large number of advanced genome editing tools for cyanobacterial genetic engineering (Vavitsas et al., 2021). In the last years, extensive research efforts have been focused on the metabolic engineering of cyanobacterial strains to enhance ALA and SDA production (Dong et al., 2016; Yoshino et al., 2017; Santos-Merino et al., 2018; Poole et al., 2020; Santos-Merino et al., 2022). In many of the cases, the enzymes directly involved in the synthesis of omega-3 fatty acids (i.e., desaturases) have been overexpressed to increase the production yields. There are only a couple of reports where other targets have been exploited to increase omega-3 fatty acids, such as enzymes involved in the saturated fatty acid synthesis (Santos-Merino et al., 2018, 2022) and the vesicle-inducing protein in plastids (Vipp1), a thylakoid membrane formation enhancer (Poole et al., 2020). Limited exploration has been done to identify additional targets in other competitive metabolic pathways with the aim to increase omega-3 fatty acid titers. One of the major obstacles to make omega-3 fatty acid production by engineered cyanobacteria practical and cost-effective is the low productivity levels achieved in these engineered strains (Shinde et al., 2022). Traditional metabolic engineering strategies are the most common avenues used to increase production yields in cyanobacteria. However, since these classical technologies are expensive, time-consuming, and labor-intensive processes, computational biology strategies are emerging as powerful tools to overcome these limitations (Xu C. et al., 2013; Gudmundsson and Nogales, 2021).
Genome-scale models (GEMs) are based on the annotated genome sequence and describe metabolic pathways as stoichiometric coefficients and mass balances of participating metabolites (Gudmundsson and Nogales, 2015). They can be used as computational test-bed to estimate metabolic fluxes using numerical optimization, thus offering a systems-biology tool not only to link genotype to phenotype but also to analyze and contextualize the metabolic capabilities of organisms (Oberhardt et al., 2009). GEMs have been successfully applied to analyze and guide the metabolism of cyanobacteria for production of several target compounds from CO2 (Nogales et al., 2013; Santos-Merino et al., 2019; Hendry et al., 2020). To date, three GEMs have been developed for S. elongatus PCC 7942: iSyf715 (Triana et al., 2014), iJB785 (Broddrick et al., 2016), and iJB792 (Broddrick et al., 2019). However, these existing models have paid little attention to fatty acid biosynthetic pathways, making it harder to use them as tool to analyze the potential of S. elongatus PCC 7942 toward the production of omega-3 fatty acids and related compounds. Then, an updated GEM with a high-quality annotation of fatty acid biosynthetic pathways is urgently required for accurately contextualizing fatty acid metabolism while predicting nutritional, physiological, and genetic scenarios for overproducing omega-3 fatty acids.
Full-facing this challenge, we present here iMS837, an updated GEM of S. elongatus PCC 7942 using iJB792 as a foundation (Broddrick et al., 2019). We validate the accuracy of our GEM using growth performance and gene essentiality predictions. The validated GEM was subsequently used to assess the production of omega-3 fatty, identifying the overexpression of fabF as key metabolic target to increase ALA production in agreement with published experimental data (Santos-Merino et al., 2018). Next, we used the model to predict possible engineered metabolic targets to enhance ALA production, identifying a set of ten additional knock-out metabolic targets that resulted in higher yields of this omega-3 fatty acid, albeit not growth-coupled. In addition, mixotrophic conditions using different carbon sources (i.e., glucose and acetate) were evaluated in silico, boosting the ALA yields obtained in both cases. These predictions will serve as a starting point for future efforts to design strains and conditions that will potentially improve omega-3 fatty acid production in cyanobacteria.
2. Results
2.1. Properties of the iMS837 GEM metabolic network
iMS837 was constructed using as a template the previously published GEM, iJB792 (Broddrick et al., 2019). We expanded iJB792 by adding new content, including 46 genes, 24 metabolites, and 29 reactions by means of a detailed manual curation based on literature legacy and by comparison with other published high-quality GEMs from cyanobacteria [i.e., iJN678 from Synechocystis, sp. PCC 6803 (Nogales et al., 2012)] and from heterotrophic bacteria [i.e., iJN1462 from Pseudomonas putida KT2440 (Nogales et al., 2020)] (Table 1; Supplementary Table S1). Overall, the largest portion of the new metabolic content of iMS837 was related with the fatty acid metabolism including 22 new reactions related to this subsystem (Figure 1A). In addition, other 11 reactions were included in iMS837, whereas four reactions related to the intracellular demands were removed. These new reactions demonstrate the uniqueness of iMS837, especially regarding the modeling of the fatty acid biosynthesis.
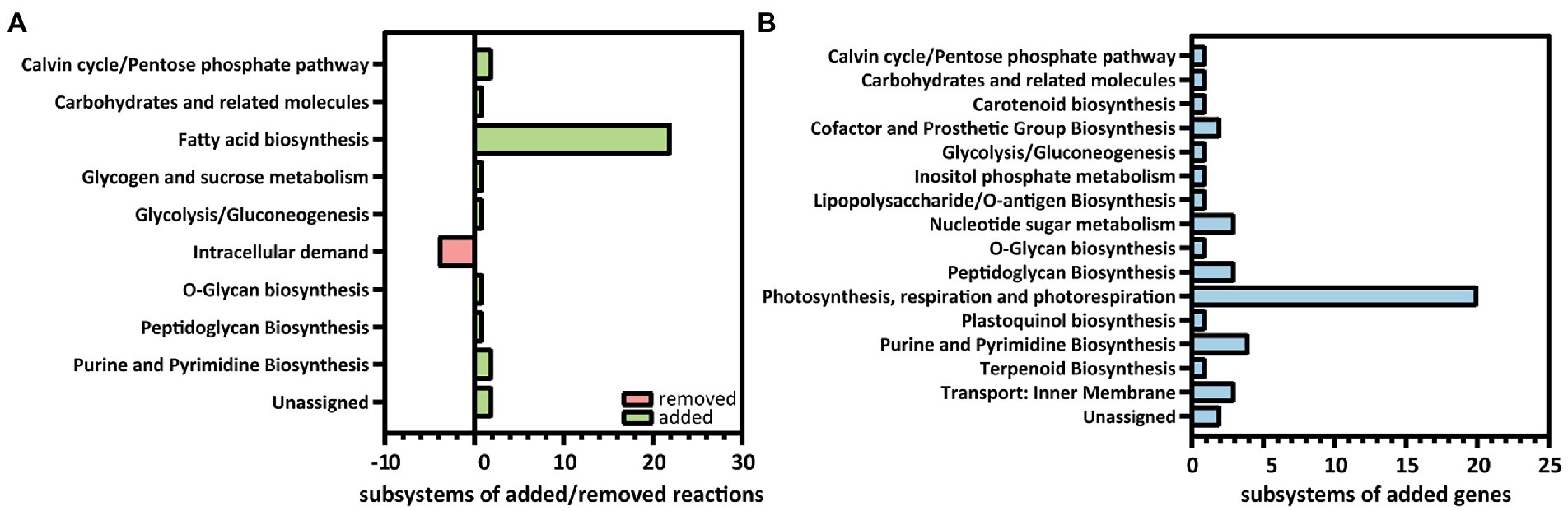
Figure 1. Distribution of reactions and genes added and removed to iMS837 based on functional subsystems. (A) Summary of reactions added and removed to iMS837. (B) Summary of genes added to iMS837. In both cases, the subsystems where changes have been made are only represented.
Beyond the important metabolic expansion done, the gene–protein-reaction (GPR) associations of several reactions were updated while others were corrected in order to improve the model accuracy (Supplementary Dataset 1). For example, reactions involved in glycogen synthesis and degradation were corrected while reactions related to electron transport chain (e.g., Cytochrome b6f complex, Cytochrome aa3 oxidase, Ferredoxin:NADPH oxidoreductase) were updated, among others. In addition, a meticulous analysis of orphan reactions included in iJB792 was carried out in order to identify the genes responsible for such reactions. We manually added 20 new GPRs, which were mainly involved in photosynthesis, respiration, and photorespiration processes (Figure 1B). The rest of the added genes (i.e., 26) belong to a large variety of subsystems as shown in Figure 1B. Finally, the biomass objective function (BOF) of iJB792 was also updated in iMS837 by removing those metabolites that were not necessary for growth (Supplementary Dataset 1). The modification of the BOF had associated changes in: (i) pigments and xanthophylls, (ii) cofactor pools, and (iii) lipids.
MEMOTE is a platform that has been developed to promote standardization of GEMs, as well as to assess quality control metrics in order to improve model reproducibility and applicability (Lieven et al., 2020). Therefore, we used MEMOTE tool in order to define the completeness, consistency, and interoperability of iMS837 when compared with previous models while analyzing potential flaws or shortcomings (Supplementary Dataset 2). The overall score for the model was 75% over the 20% estimated for iJB792, which suggest a very good level of completeness. The scores in annotation subcategories were increased by adding annotations and Systems Biology Ontology (SBO) terms to metabolites, reactions, and genes in the updated GEM which were not previously included in iJB792. The model scored 55% for the critical category of consistency, which represents accuracy in reaction stoichiometry, mass and charge balances, connectivity of metabolites, and reaction cycles. A major gap was found due the lack of annotation of outside references for some genes, metabolites, and reactions. This limitation only will have some impact when using automated tools or scripts; however, its accuracy and usability should not be affected. Taking together, the MEMOTE analysis demonstrated that iMS837 is a highly complete and detailed model that can be used as a reference for other GEM constructions.
2.2. Model validation using gene essentiality prediction
An increase in the number of genes, reactions and metabolites does not always indicate a higher-quality GEM. In order to validate the quality of iMS837, we conducted an extensive gene essentiality analysis of the genes included in this GEM by comparing the predicted results in silico with available essentiality experimental data for S. elongatus PCC 7942 (Rubin et al., 2015; Figure 2). We performed single-gene knockout simulations in iMS837 using COBRApy (Ebrahim et al., 2013). The model-based gene essentiality predictions showed an overall high-level of accuracy, 85.5% (Figure 2B). More specifically, the model was able to correctly assign 330 and 333 as essential and non-essential genes, respectively (Figure 2A; Supplementary Table S2). On the contrary, the level of discrepancy found between the predictions of iMS837 and the experimental data was pretty low. For instance, 75 genes were incorrectly predicted as essential while only 37 genes were predicted as essential but were found non-essential in vivo. Gene essentiality accuracy assignment in GEMs is often biased due large number of non-essential genes (Wei et al., 2013). Therefore, to avoid potential bias caused by such effect, we proceed to additionally compute the sensitivity (i.e., proportion of essential genes that have been correctly identified), specificity (i.e., proportion of true negatives that have been correctly predicted), and precision (i.e., the probability that the essential genes were predicted as essential) of our gene essentially prediction (Figure 2B). We found very high values for all these parameters, suggesting no significant bias, and corroborating the high capacity of iMS837 when predicting gene essentiality.
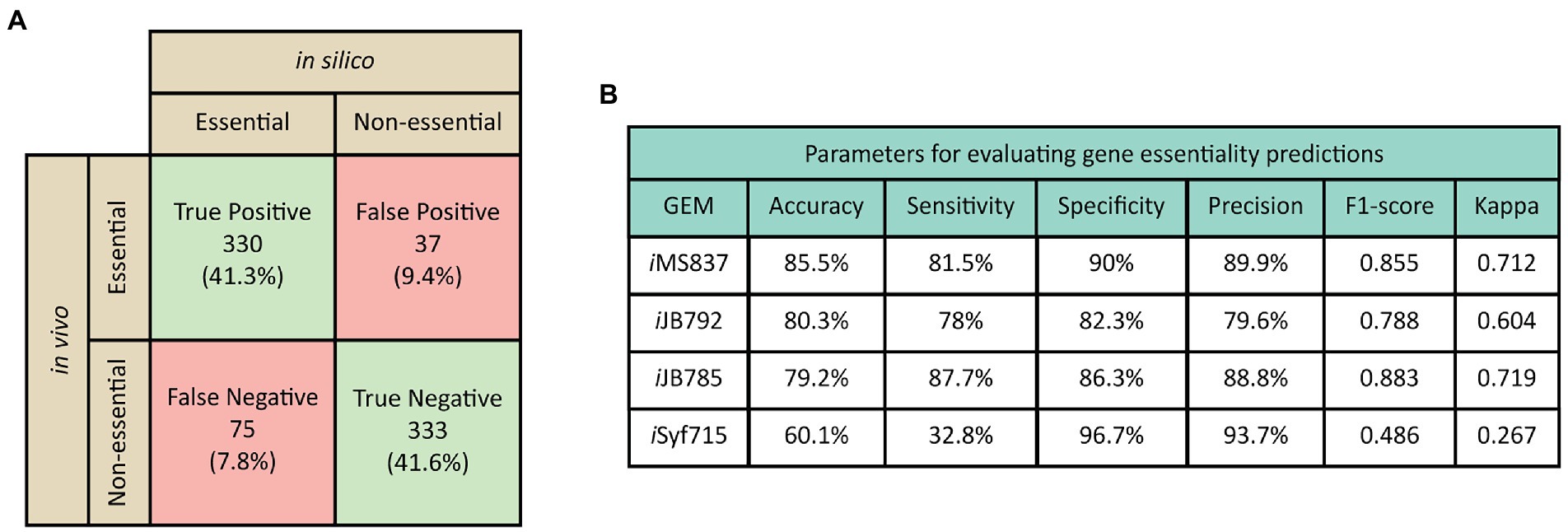
Figure 2. Comparison of gene essentially predictions to experimental results. (A) Contingency table of the results obtained for gene essentially predictions using iMS837. (B) Parameters used to estimate the performance of gene essentially predictions for all GEM of Synechococcus elongatus PCC 7942. Gene essentially results obtained in silico revealed a high level of accuracy (85.5%), sensitivity (81.5%), specificity (90%) and precision (89.9%) of iMS837. Genes with ambiguous essentiality results and not-analyzed in vivo were excluded from this analysis (Supplementary Table S2).
On the other hand, when comparing the level of accuracy of iMS837 with that from previous S. elongatus PCC 7942 GEMs, iJB792 (Broddrick et al., 2019), iJB785 (Broddrick et al., 2016), and iSyf715 (Triana et al., 2014), we found that iMS837 scored the highest in terms of accuracy, sensitivity, specificity, and precision (Figure 2B). Whereas iJB792, iJB785, and iSyf715 were able to correctly assign the 80.3, 79.2, and 60.1% of the genes included in the model as true essential and nonessential, the 80.3, 79.2, and 60.1% of the genes included in the model, iMS837 outperforms better that these three GEMs by correctly predicting 85.5% (Figure 2B; Supplementary Table S2). Overall, these results indicate that we have not only expanded the last published GEM of S. elongatus PCC 7942 by adding new reactions, metabolites and genes, but also, we have improved its accuracy by increasing its completeness.
2.3. System evaluation of S. elongatus PCC 7942 as a cell factory toward the production of omega-3 fatty acids
The capability of a GEM to provide accurate predictions of experimentally supported data of a target organism’s functional states is a key feature in order to assess the accuracy and completeness of the final reconstruction. Once iMS837’s accuracy and completeness were assessed, the model was ready to be used to characterize metabolic states underlying observed phenotypic functions and as a computational framework for metabolic engineering endeavors. In this regard, we further used iMS837 as a test-bed to systematically analyze S. elongatus PCC 7942 as a cell factory toward the production of ALA. In order to do that, we first included the non-native ALA synthesis pathways in the GEM of S. elongatus PCC 7942, a non-natural ALA producer strain. We used iJN678, a well-developed GEM from Synechocystis sp. PCC 6803 (Nogales et al., 2012) that includes this biosynthetic pathway, since this cyanobacterium is able to naturally produce omega-3 fatty acids (Tasaka et al., 1996). A total of 34 reactions and 28 metabolites were added to iMS837 in order to produce ALA and accumulate this omega-3 fatty acid in its membranes (Supplementary Table S3; Supplementary Dataset 1). Among the added reactions to produce ALA in S. elongatus PCC 7942, we included the desaturases DesA and DesB (Δ12- and Δ15-desaturases) and all the reactions involved in integrating this omega-3 in the phospholipids of cellular membranes.
In silico, the production capabilities of a given strain can be shown using production envelope plots, which represent all possible production rates of a selected metabolite and their associated feasible growth rates (Lewis et al., 2012). Production envelope for ALA production showed that, although feasible under a real nutritional scenario, the production of this omega-3 fatty acid is not coupled to growth (Supplementary Figure S1). In other words, for all the possible levels of ALA production, the model predicted a decrease in the maximal growth rate. This is not surprising since the synthesis of fatty acids is one of the most energetically expensive process among all the lipid membranes components (Zhang and Rock, 2009), and the heterologous production of ALA directly compete with cell growth (Chen et al., 2014). Cyanobacteria only produced unsaturated fatty acids in respond to drops in temperature to compensate for the decrease in membrane fluidity, conditions where they do not normally growth. In addition, it is well-established that cyanobacteria are only able to accumulate fatty acids at low temperatures, where the transcripts for desA and desB desaturases are more abundant (Sakamoto et al., 1997; Ludwig and Bryant, 2012).
Once demonstrated that the model was able to predict ALA production fluxes, we decided to explore in silico previously identified genetic interventions that led to a decrease and an increase in ALA yield (i.e., fabH deletion and fabF overexpression, respectively) (Santos-Merino et al., 2018). We used Markov chain Monte Carlo sampling to establish potential differences in the metabolic states between strains by comparing the allowed specific metabolic solution spaces (Schellenberger and Palsson, 2009). This flux sampling methodology allowed us to explore the feasible flux solutions in our metabolic network by generating probability distributions of steady-state reaction fluxes (Herrmann et al., 2019). We analyzed the fluxes of the reactions involved in the saturated and unsaturated fatty acid synthesis and their probability in each strain.
Firstly, we explored the flux distributions for strains with increased levels of FabF enzyme (3OAS180 reaction in iMS837): (i) FabF-UP-2x, with double flux for FabF reaction than the control strain; and (ii) FabF-UP-4x, with quadruple flux for FabF reaction than the control strain (Figure 3). We observed an overall increase in the flux of reactions involved in the saturated fatty acid synthesis pathway, proportional to the value of FabF flux. Same effect was observed in the flux of the DesC desaturase (DESAT18a). In addition, a large flux for DesA and DesB desaturases (DES::12 and DES::15, respectively) was observed for FabF-UP-4x, but the probability was lower than the minor fluxes observed FabF-UP-2x. These results agree with in vivo experimental data linking the overexpression of fabF with increased levels of C18:1 (the product of DESAT18a reaction) and ALA (Santos-Merino et al., 2018).
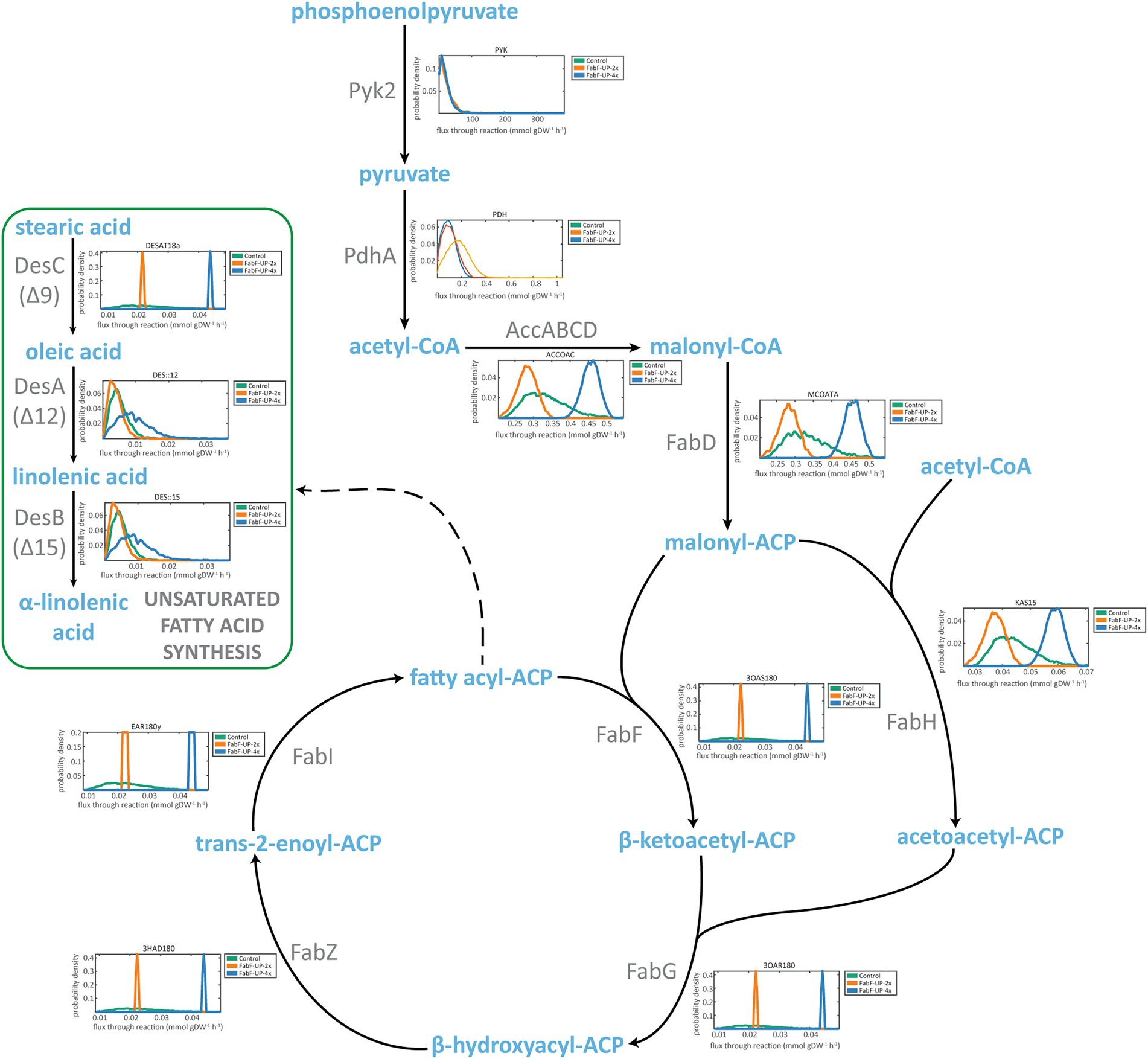
Figure 3. Flux sampling distributions of main reactions of ALA synthesis for in silico FabF overexpression mutants and predicted using iMS837. For each reaction, a plot of the probability density versus the predicted flux of the specific reaction is represented. In green, the results obtained for iMS837_ALA are represented (Control); in orange, an in silico designed strain that has double flux for FabF reaction (3OAS180) than iMS837_ALA (FabF-UP-2x); and in blue, an in silico designed strain that has quadruple flux for FabF reaction (3OAS180) than iMS837_ALA (FabF-UP-4x).
As a second scenario, we analyzed in silico the flux distributions for strains harboring down- and up-regulation of FabH fluxes (KAS15 reaction in iMS837) that we denominated FabH-DOWN and FabH-UP, respectively (Figure 4). For the FabH-UP sampling, it was predicted an increase in the flux of the reactions involved in the elongation cycle (FabF, FabG, FabZ, and FabI). As could be expected, we computed an increase of these fluxes for FabH-DOWN sampling. On the other hand, the flux of DesC desaturase (DESAT18a) was increased in FabH-UP, while it was almost zero for FabH-DOWN. Finally, FabH-UP and FabH-DOWN failed to increase the fluxes through DesA and DesB desaturases (DES::12 and DES::15, respectively). Overall, these results mainly agree with in vivo observations, showing no increased in ALA yields after modifications of the expression of fabH (Santos-Merino et al., 2018).
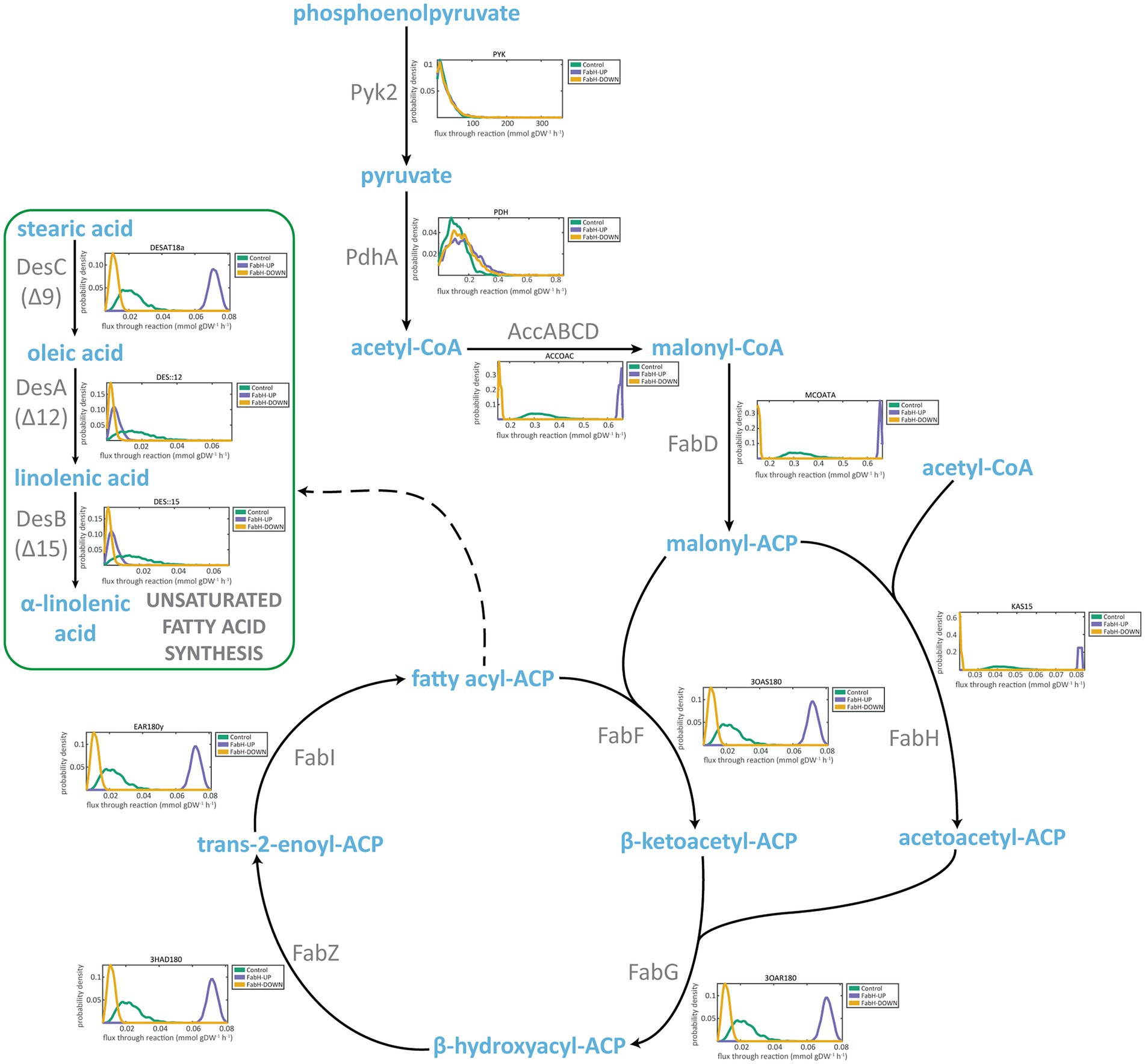
Figure 4. Flux sampling distributions of main reactions of ALA synthesis for in silico FabH overexpression and downregulation mutants and predicted using iMS837. For each reaction, a plot of the probability density versus the predicted flux of the specific reaction is represented. In green, the results obtained for iMS837_ALA are represented (Control); in purple, an in silico designed strain that has double flux for FabH reaction (KAS15) than iMS837_ALA (FabH-UP); and in yellow, an in silico designed strain that has half flux for FabH reaction (KAS15) than iMS837_ALA (FabH-DOWN).
2.4. Identification of potential gene overexpression targets to increase ALA yields
GEM gives us the advantage of speeding up the exploration and redesign of the metabolism of an organism toward the production of a given metabolite. In most cases, the upregulation of certain fluxes directly or indirectly involved in the biosynthetic pathway of a desired compound, results in improved yields. With the aim of identifying fluxes that could be upregulated to increase ALA production, we used flux scanning based on enforced objective function (FSEOF) (Park et al., 2012). This method scans changes in metabolic fluxes in response to an artificially enforced objective flux of the desired product formation. Using this algorithm, up to 70 target reactions were identified as a result of gradually increments in the ALA production reaction and the acceptance of up to 20% reduction in the biomass-producing reaction (Supplementary Table S4).
The FSEOF simulation results revealed that ALA production increased with the enhancement of the fatty acid biosynthetic pathways (Figure 5). All the reactions involved in the unsaturation and desaturation steps in the fatty acid biosynthesis were identified as potential overexpression targets. Following the logic of increasing the intermediates of ALA synthesis, we have previously overproduced in vivo FabF, FabH, FabD, FabZ, and FabG with the aim to improve ALA yields (Santos-Merino et al., 2018, 2022), with the exception of FabI. The overexpression of most of them did not increase C18:1 levels, the substrate for the sequential activity of DesA and DesB desaturases. Only the overproduction of FabF was able to successfully increase ALA yields. Little known of the regulation of fatty acid synthesis in cyanobacteria, that could affect to our experimental interventions in this pathway, as well as the in silico predictive capability of iMS837, which does not include information about regulation. Acetyl-CoA carboxylase, which catalyzes the first step of the saturated fatty acid biosynthesis, was also identified as a potential overexpression target to increase ALA production. Overexpression of this enzyme has been proven to be an effective way to increase the rate of saturated fatty acid synthesis in Synechocystis sp. PCC 6803 (Eungrasamee et al., 2019), as well as other Acetyl-CoA derived compounds, such as alkanes and alkenes (Tan et al., 2011; Wang et al., 2013). All together indicates that overexpression of Acetyl-CoA could be a feasible strategy to be implemented in vivo with the aim to increase ALA yields.
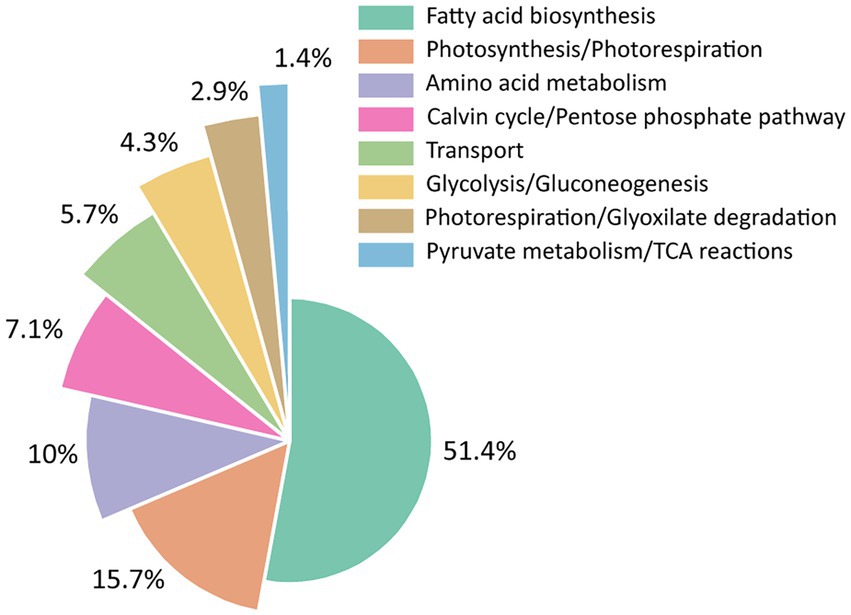
Figure 5. Distribution of reactions obtained with FSEOF algorithm based on functional subsystems. The reactions included in each subsystem are depicted in Supplementary Table S4.
In addition, the increase in the fluxes through the components of the photosynthetic electron transport chain was the second category of reactions that showed an increase in ALA production. It has been experimentally demonstrated that an increase in the light intensity leads to an increase in omega-3 fatty acids in Synechococcus sp. PCC 7002 (Sakamoto et al., 1997). Then, it is not surprising that reactions related to photosynthesis are the second-most represented in the results obtained with FSEOF. The third category of reactions that can be upregulated to increase ALA yields is the amino acid metabolism (Figure 5), including reactions involved in the synthesis of alanine, serine, glutamate, glutamine, and aspartate (Supplementary Table S4). In Arthrospira platensis, the supplementation of cultures with aspartate stimulated the accumulation of saturated fatty acids, possibly through enhanced de novo fatty acid biosynthesis (Fekrat et al., 2022). An increase of the in silico flux of reactions associated with amino acid metabolism could have the same role. Finally, it is important to highlight that in the category of reactions related to pyruvate metabolism and tricarboxylic acid cycle (TCA), the only reaction which flux should be increased is the one for the Acetyl-CoA synthetase (Supplementary Table S4). Acetyl-CoA is a key metabolic intermediate that links many metabolic processes, including the TCA cycle, amino acid metabolism, and fatty acid metabolism (Mills et al., 2020). It is possible that in order to increase the direct flux of Acetyl-CoA into the fatty acid synthesis, the flux of this key intermediate should be increased to avoid the competition between all the pathways that use it.
2.5. Identification of potential genetic interventions to improve ALA production
In addition to upregulation of reaction fluxes, gene knockout is one the most common strategies to improve microbial strains for producing desirable compounds. OptKnock (Burgard et al., 2003) and GDLS (Lun et al., 2009) are strain design algorithms commonly used to predict genetic manipulations for target overproduction. Both methods are based on constraint-based optimization processes to suggest reaction knockout interventions (constraining the metabolic flux of a reaction to zero) to increase targeted compound production while optimizing biomass yield and product yield. Once identified, the suggested reactions can be eliminated in vivo by knocking out one or more of the genes encoding the enzymes catalyzing the reaction.
Unfortunately, the application of GDLS and OptKnock algorithms failed identifying suitable knockout strategies for coupling ALA production to biomass synthesis. However, both algorithms were able to identify partial strategies harboring an increasing number of knockouts putatively resulting in ALA overproduction (Figure 6). Overall, and as could be expected, a higher number of knockouts resulted in a higher ALA production due the removal of competing pathways. Interestingly, Optknock was shown as the most efficient algorithm under the condition tested being able to identify more potential knockout target reactions than GDLS (Supplementary Figure S2; Table 2). Among the six reactions identified as potential target to improve ALA production by both algorithms, four of them were related to amino acid metabolism (ALAD_L, PSERT, PGCD, and PSP_L) and two were involved in the oxidative branch of the Pentose Phosphate Pathway (G6PDH2r and GND, respectively. As mentioned in the previous section, the synthesis of these amino acids outcompetes for Acetyl-CoA necessary for fatty acid biosynthesis. A similar explanation could be attributed to the other two common reactions obtained using OptKnock and GDLS, G6PDH2r and GND, since they are diverting glucose away to the formation of Acetyl-CoA. OptKnock was able to identify a reaction involved in the fatty acid synthesis as a potential target to be knocked out, ACOATA (Supplementary Figure S2; Table 2). This reaction is performed for FabH, an enzyme that failed in silico (Figure 4) and experimentally to increase ALA production (Santos-Merino et al., 2018).
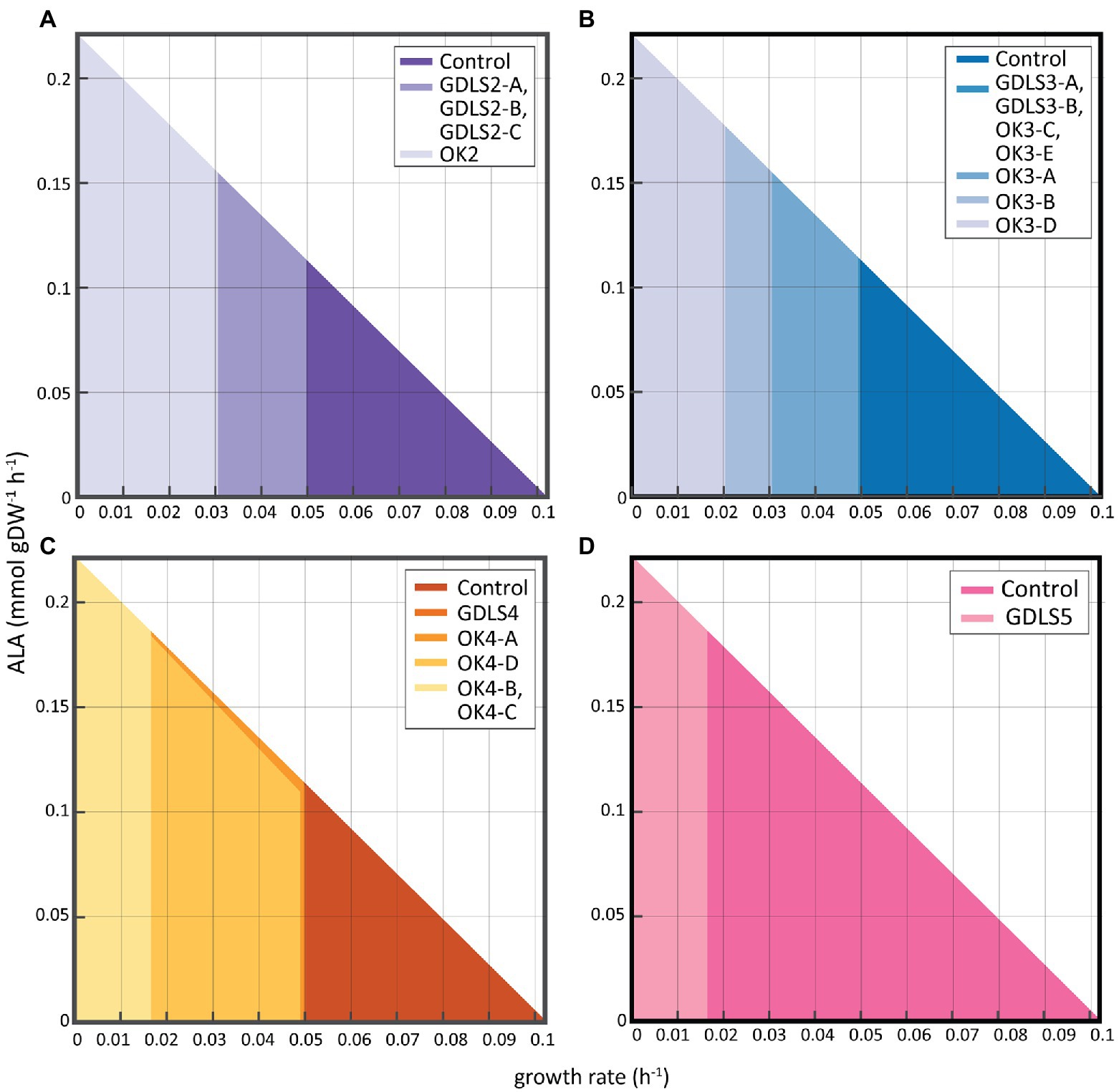
Figure 6. Production envelope for S. elongatus PCC 7942 mutants with enhanced ALA production obtained with GDLS and OptKnock. Maximum optimal production rate (mmol gDW−1 h−1) of ALA achievable with (A) two knockouts; (B) three knockouts; (C) four knockouts; and (D) five knockouts.
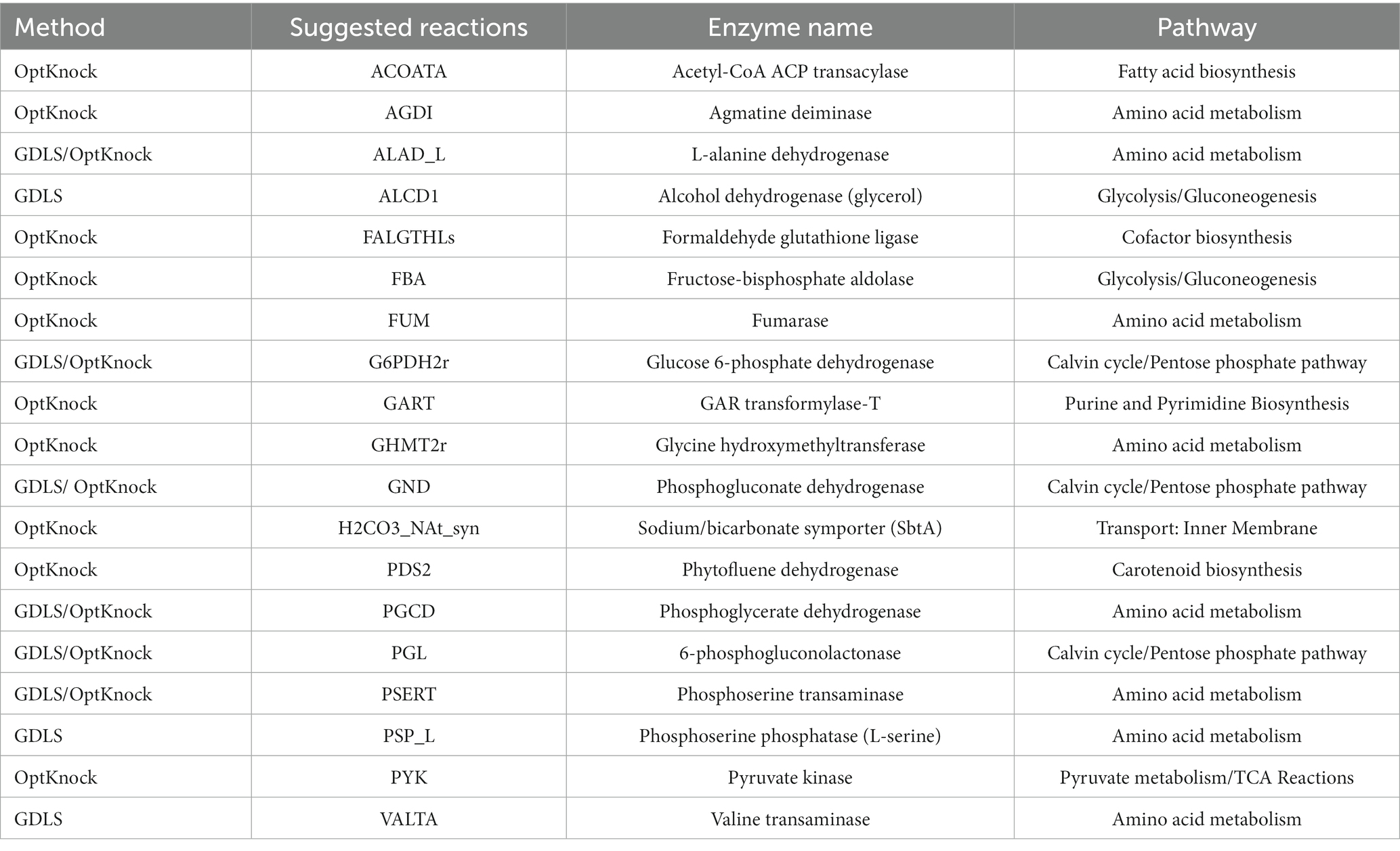
Table 2. Comparison of the reactions suggested by OptKnock and GDLS to be deleted in order to increase the production of ALA.
The optimal solutions for most of the single, double, triple, and quadruple knockouts were different for OptKnock and GDLS predictions (Supplementary Table S5; Figures 6A–C). Only in the case of some triple knockouts, the knockouts in the identified reactions gave identical optimal changes in the flux distribution (Supplementary Table S5; Figure 6B). In addition, only the GDLS algorithm was able to suggest optimal solutions by knocking out five reactions (Supplementary Table S5; Figure 6D). Finally, none of the algorithms was able to provide optimal solutions by applying single reaction knockout strategies.
Although growth coupled overproducing strategies are challenging due the low metabolic robustness of cyanobacteria (Nogales et al., 2013; Gudmundsson and Nogales, 2015), which could result in unfeasible genetic designs under the current scenario, we cannot rule out the possibility that the lack of success using GDLS and OptKnock is due to an insufficiently scrutinized metabolic space. To address a more systematic search, we performed a new strain designing analysis by using gcFront (Legon et al., 2022). gcFront is an algorithm that explores knockout strategies maximizing not only cell growth and product synthesis, but also the strength of production-to-growth coupling using a tri-level optimization. The incorporation of this last optimization parameter significantly reduces the search time with respect other strain designing algorithms such as OptKnock or GDLS, thus significantly speeding up the process. In addition, gcFront is based on a genetic algorithm approach, thus, it allows to perform a larger search in terms of number of knockouts. Unfortunately, we were not able to find growth-coupled ALA overproducing strategies even allowing up to 30 knockouts (data not shown). Taking together, these results confirmed the limited chance of designing growth-coupled ALA overproducing phenotypes using cyanobacteria under photoautotrophic conditions.
2.6. Exploring the production of ALA in S. elongatus PCC 7942 beyond photoautotrophic conditions
The lack of growth coupled ALA designs under photoautotrophic conditions encouraging us to explore alternative nutritional regimens, such as photomixotrophy. It has been previously demonstrated that photomixotrophic conditions can enhance growth performance of S. elongatus PCC 7942 while provide, at least in theory, a more robust metabolism increasing the metabolic space suitable to flux rerouting (Yan et al., 2012; Nogales et al., 2013). To address this goal, we constructed a set of condition-specific GEMs using as constraint the uptake of inorganic and organic carbon sources previously reported (Yan et al., 2012), as detailed in methods. Following this procedure, we construct a photoautotrophic model and two photomixotrophic GEMs harboring acetate and glucose consumption systems, respectively. The ALA production envelope of the condition-specific models revealed a significant higher metabolic space bounded by photomixotrophic conditions (Figure 7A). Glucose provided the highest metabolic solution space with up to double production of ALA and growth rate, whereas acetate provided a slightly chance of ALA production although it was not growth-coupled. This improved phenotypic performance under photomixotrophic conditions was not only due the presence of organic carbons as additional nutrients, but also to improved photosynthetic efficiencies (Figure 7B). In fact, we computed significant higher fluxes through Photosystem I and II reactions as well as a higher oxygen evolution and photon uptake under photomixotrophic conditions, completely agreeing experimental data (Yan et al., 2012). Subsequently, we used this expanded metabolic space to search for growth-coupled strategies using gcFront following identical setup than used under autotrophic conditions. Despite several attempts performed, we were not able to identify growth-coupled ALA-overproducing S. elongatus PCC 7942 strains neither using glucose nor acetate as organic carbon sources (data not shown). Therefore, we concluded that the single use of knockout strategy is not feasible to reroute carbon flux toward the production of ALA.
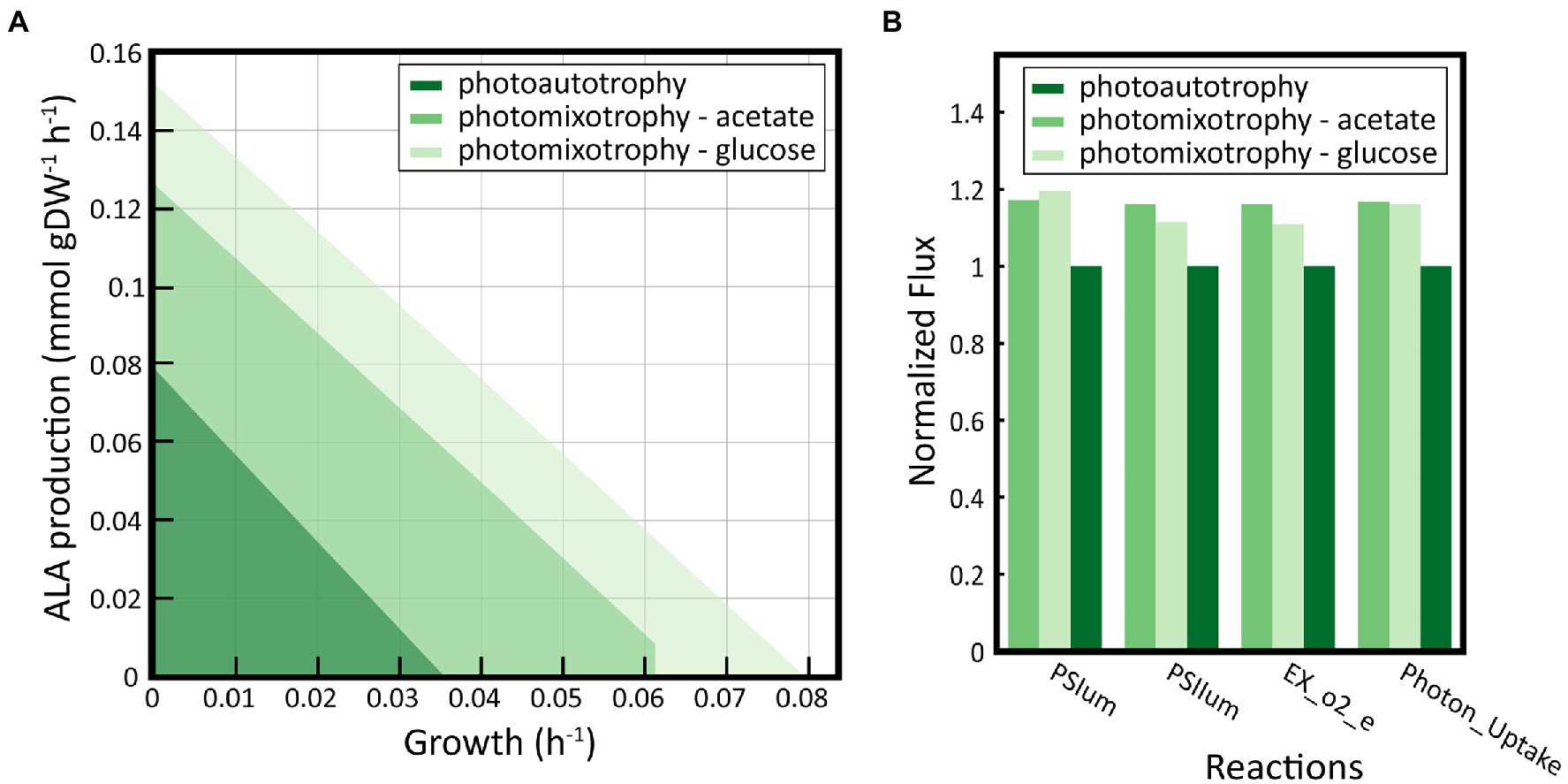
Figure 7. Exploration of the metabolic space under photomixotrophic conditions. (A) ALA production envelopes in photoautotrophic and photomixotrophic conditions using acetate or glucose as carbon source. The solution space increases under photomixotrophic conditions. (B) Evaluation of photosynthetic activity under photoautotrophic and photomixotrophic conditions. The flux of the reactions associated with the activity of Photosystem I and II (PSIum and PSIIum, respectively), the production of oxygen (EX_o2_e) and the flux oh photons (Photon_uptake) under photomixotrophic were simulated and normalized to the values obtained under photoautotrophic conditions.
To gain further insights on this hypothesis, we analyzed the metabolic flux of the reactions from central metabolism including Calvin–Benson–Bassham (CBB) cycle and TCA and those directly involved in ALA synthesis under photoautotrophic and photomixotrophic conditions (Supplementary Figures S3–S5). The detailed analysis of this flux distribution identified that the synthesis of ALA is limited since Acetyl-CoA pool is funneled almost exclusively to the synthesis of fatty acids. In fact, the metabolism of fatty acids via Acetyl-CoA is not connected with the production of other components of the biomass. Compounding the problem, the complete list of genes involved in fatty acid biosynthesis are essential under the three nutritional regimens analyzed, excluding the possibility to reroute carbon flux from fatty acid biosynthesis to ALA production via removing competitive pathways. Taking together, the reduced connectivity of Acetyl-CoA and the essentiality of genes surrounding fatty acid biosynthesis and ALA production explains, at great extent, the unfeasibility of designing growth coupled ALA overproducer S. elongatus PCC 7942 strains.
As a direct consequence, the only solution found increasing ALA production was the increase of the flux through the Δ12-desaturase (DES::12) reaction (Figure 8; Supplementary Figure S3), as it has been demonstrated in vivo (Sakamoto et al., 1997; Chen et al., 2014; Santos-Merino et al., 2018). Interestingly, while under photoautotrophic and glucose-driven photomixotrophic conditions the increase in DES::12 flux negatively impacted S. elongatus PCC 7942 growth rate (Figure 8; Supplementary Figure S5), we observed an increase in ALA production without any negative effect over the biomass using acetate-driven photomixotrophic conditions (Figure 8; Supplementary Figure S4). This is because, the drainage of the Acetyl-CoA pool toward the ALA production is replenished by increasing the uptake of acetate conditions. Therefore, our computational analysis expanding the metabolic space by feeding S. elongatus PCC 7942 with organic carbon sources, strongly suggested that such photomixotrophic conditions, especially using acetate as a carbon source, seem to be a promising strategy to increase ALA production in S. elongatus PCC 7942. However, these results will need to be further validated experimentally.
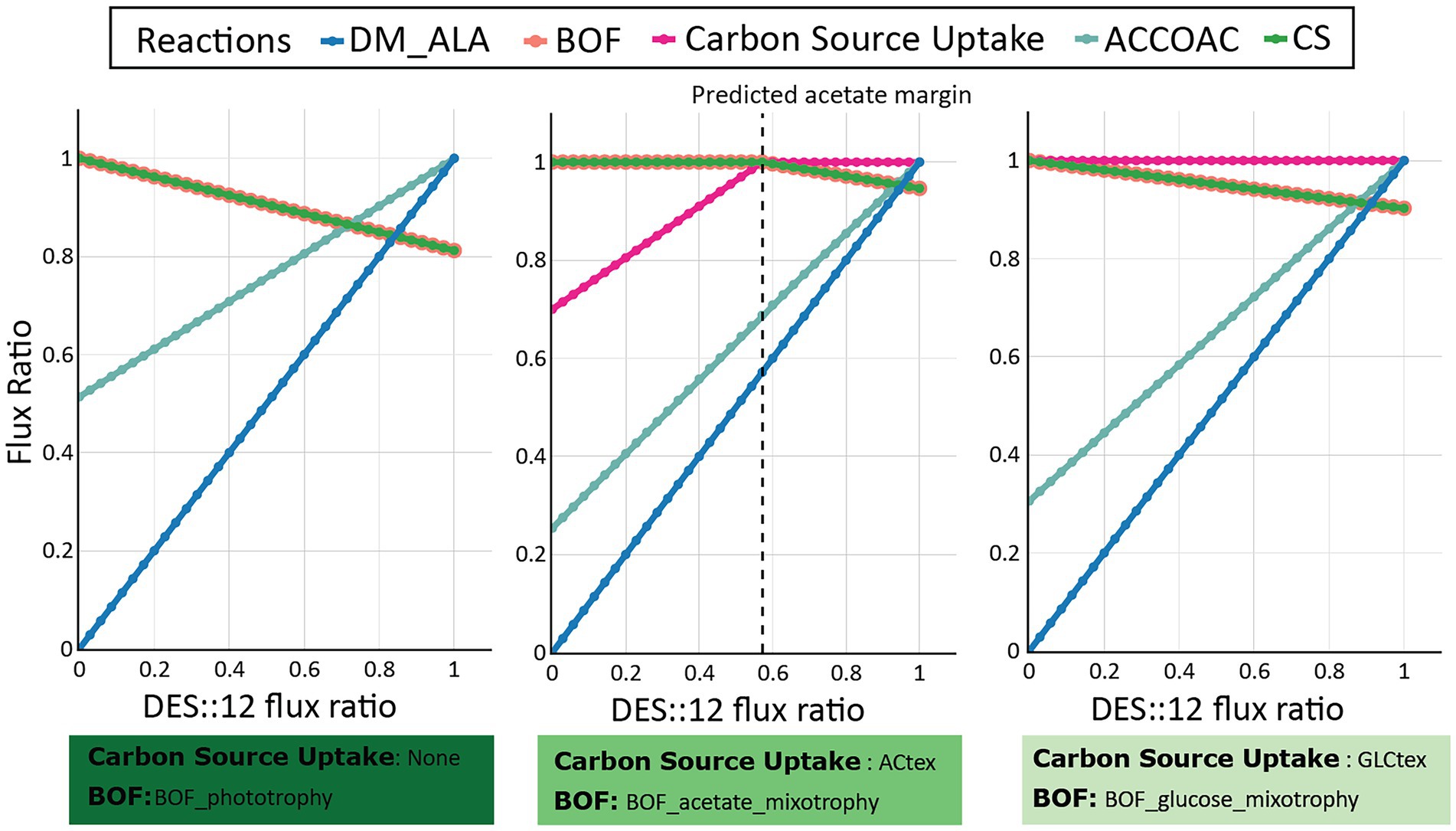
Figure 8. Flux variability analysis of Δ12-desaturase (DES::12) overexpression in different nutritional conditions. Impact of DES::12 overexpression on growth rate (BOF), ALA production (DM_ALA), and organic carbon uptake (Carbon Source Uptake) under photoautotrophic (left panel), photomixotrophic with acetate (middle panel), and photomixotrophic with glucose (right panel) conditions. Fluxes through some reactions of the fatty acid synthesis (ACCOAC) and TCA cycle (CS) were measured.
3. Discussion
We generated an updated GEM of S. elongatus PCC 792 with considerable improvements in model annotation and accuracy of essentiality prediction. The metabolic reaction and genome coverage of the reconstruction was expanded, the format and annotations were updated to be consistent with current best practices. Model improvements were quantified through various metrics such as accuracy of growth yield and ALA yield predictions as well as MEMOTE benchmarking. Overall, iMS837 has increased the coverage of the metabolic functionality of S. elongatus PCC 7942 and is one of the highest-quality cyanobacterial GEMs. While the updates included in iMS837 largely improved the model accuracy, there were still incorrect predictions about gene essentiality that were not able to be addressed. In S. elongatus PCC 7942, a significant proportion of genes has still an unknown function (Labella et al., 2020), with many of them presumably involved in processes relevant to the metabolism of the cyanobacteria. It makes it difficult to include new metabolic genes in the model, since there is a lack of information between GPR associations that will allow their identification. In addition, iMS837 only contains a small portion of the total genes of S. elongatus PCC 7942 genome (837 of 2,772 genes; Table 1) with an absence of regulation that could influence the correct prediction of their essentiality. However, despite all this missing information, iMS837 was able to achieve a high overall accuracy for predicting gene essentiality phenotypes (i.e., 85.5%) that is comparable to the performance of the well-curated E. coli models, iJO1366 (i.e., 93.4%) (Orth et al., 2011) and iML1515 (i.e., 93.4%) (Monk et al., 2017).
Although iMS837 only captures 30.2% of the protein coding genes of S. elongatus PCC 7942, this metabolic reconstruction was able to make correct computational predictions related to experimental data (i.e., gene essentiality and fatty acid biosynthesis). The correct functional annotation of the genes encoding metabolic enzymes involved in the fatty acid biosynthetic pathway helped with prediction involving enzymes of this pathway (Supplementary Dataset 1). An experimental effort to identify unknown GPR associations, as well as a constant improvement and updating of the GEM of S. elongatus PCC 7942 will improve its prediction capabilities and its use to generate new hypotheses and to identify promising targets for bioengineering applications (Esvelt and Wang, 2013). Integration of kinetics and omics data in GEMs will broaden their quality and application scopes to better understanding the metabolism of cyanobacteria (Gu et al., 2019). On the other hand, the incorporation of accurate and well-developed GEMs into Design-Build-Test-Learn cycles, together with the use of machine learning, will lead to a very powerful toolset for guiding metabolic engineering of cyanobacteria (Liao et al., 2022).
Metabolic models through the implementation of different algorithms are powerful tools to predict potential interventions that may improve the production of a specific compound. FSEOF and GDLS/OptKnock turned out as efficient systems to predict which gene overexpressions and knockouts, respectively, that might be potential targets to increase ALA production. FSEOF results suggest that the availability of saturated fatty acid pools is important for the synthesis of ALA (Figure 5; Supplementary Table S4). This hypothesis was tested previously in vivo demonstrated that FabF seems to be the limiting-rate step in this pathway (Santos-Merino et al., 2018). All the solutions obtained with GDLS, OptKnock and gcFront algorithms failed to produce mutants where ALA production is coupled with growth under photoautotrophic conditions (Figure 6).
It has been previously demonstrated that the carbon flux rerouting to obtain growth-coupled producer strains is more challenging under autotrophic conditions than under mixotrophic or heterotrophic conditions in cyanobacteria (Nogales et al., 2013; Wan et al., 2015). Photomixotrophic culture conditions has been successfully applied in S. elongatus PCC 7942 to efficiently increase the production of 2,3-butanediol (McEwen et al., 2013; Kanno et al., 2017), which has been also tested in silico using iJB792 (Broddrick et al., 2019). In addition, the use of acetate as carbon source has allowed to increase the production of poly-3-hydroxybutyrate (PHB) in different cyanobacterial strains (de Philippis et al., 1992; Wu et al., 2002; Sharma and Mallick, 2005; Panda et al., 2006; Khetkorn et al., 2016; Towijit et al., 2018). Here, we successfully demonstrated that the production of ALA can be also boosted in silico under photomixotrophic conditions using acetate or glucose as carbon sources (Figure 7A), and this strategy could be employed in vivo likely leading to the same result. The synthesis of PHB and ALA requires the same precursor, Acetyl-CoA. The stimulatory effect of acetate on ALA synthesis could be explained by the direct utilization of acetate to increase the intracellular Acetyl-CoA pool, as it has been previously speculated for PHB (de Philippis et al., 1992; Khetkorn et al., 2016).
Overall, we were able to find potential solutions in silico that were feasible to increase ALA production in S. elongatus PCC 7942 under phototrophic conditions, but in all the cases, they have a negative cost for the cells, negatively impacting their ability to grow. Omega-3 fatty acid synthesis takes place under a continuous supply of Acetyl-CoA and NADPH, which limits carbon flux through the biomass synthesis (Diao et al., 2020). Using iMS837, we were able to predict that there is a limitation of the flux of Acetyl-CoA that impedes maximizing ALA production without decreasing biomass yields (Supplementary Figure S3). Contrary to heterotrophic conditions, phototrophic growth promotes low level of Acetyl-CoA since reducing equivalents are provided by photosynthesis instead of TCA via oxidation of Acetyl-CoA. Therefore, Acetyl-CoA is mainly used as building block for fatty acids resulting a narrow window for ALA production (Supplementary Figure S3). The use of an organic carbon substrate to stimulate the production of Acetyl-CoA, such as acetate, was the only alternative option to balance the production of ALA and biomass. The utilization of acetate by S. elongatus PCC 7942 does not compete with TCA cycle and CO2 fixation activities (Supplementary Figure S4), but also it seems to stimulate S. elongatus PCC 7942 photosynthetic activity (Figure 7B), and potentially the NADPH production, needed for fatty acid production.
4. Conclusion
In this study, we provided and updated GEM of S. elongatus PCC 7942 by using information from the scientific literature and openly available databases, as well as data from well-annotated GEMs from other bacteria. The updated model, iMS837, comprised 837 genes, 887 reactions, and 801 metabolites. Following a series of growth simulations, the model was found to agree with published literature. The application of the updated GEM to investigate ALA production recapitulated phenotypes observed in literature, and the use of algorithms to identify potential reactions target to be overexpressed or eliminated can offer systematic strategies that would be difficult to delineate experimentally. In addition, photomixotrophic conditions were also identified as potential target to boost ALA production. This application of the updated reconstruction serves as an example of how GEMs can provide insights into non-intuitive metabolic engineering strategies to improve the production of industrially important metabolites. Ultimately, our computational in-depth analysis of iMS837 for ALA production provides an example of the systems-biology science iteration paradigm, by producing further hypothesis that need experimental follow-up to be validated.
5. Materials and methods
5.1. Development of an upgraded GEM of S. elongatus PCC 7942 named iMS837
The iMS837 model was developed using the published iJB792 model (Broddrick et al., 2019) as a starting point (Supplementary Dataset 3). Updates in iJB792 were made using Python and the COBRApy package (Ebrahim et al., 2013). A detailed description of all these changes can be found in the Supplementary Dataset 1 and Supplementary Table S1. The new generated model was denominated iMS837. All the scripts used in the methods section can be found in GitHub repository (https://github.com/MariaS87/GEM-Synechococcus-elongatus-PCC-7942-iMS837.git).
5.2. Flux balance analysis
Flux Balanced Analysis (FBA) uses linear programming to maximize an objective function while assuming no metabolite accumulation during cellular growth. We used FBA to evaluate the biomass production (growth prediction) once the biomass reaction was fixed as the objective function (BOF, Biomass Objective Function) (Orth et al., 2010). The result when executing FBA was the growth rate (h−1) predicted under the specified media conditions.
5.3. Model manipulation to produce ALA
iMS837 was modified to introduce the required reactions to produce ALA. A detailed list of the reactions and metabolites added is included in Supplementary Table S3. The new generated model was denominated iMS837_ALA (Supplementary Dataset 3).
5.4. Metabolic network simulations
iMS837 and iMS837_ALA models were analyzed using COBRA Toolbox v2.0 (Schellenberger et al., 2011) within the MATLAB environment (The MathWorks Inc.). Tomlab CPLEX (Tomlab Optimization Inc., San Diego, CA) and Gurobi (Gurobi Optimization Inc., Houston, TX) were used for solving the linear programming problems.
5.5. Gene essentiality predictions of iMS837
For growth simulation, the biomass equation (BOF) was set as the objective function. The analysis of gene essentiality was performed using the “single_gene_deletion” function of COBRApy (Supplementary Dataset 1; Ebrahim et al., 2013). If the growing rate of the knockout strain was lower than 10−3, the gene was defined as essential.
To evaluate the performance of our GEM to correctly predict gene essentiality, we employed a variety of statistical index-based methods, including: accuracy, sensitivity, specificity, precision, F1-score, and Cohen’s Kappa coefficient (van Stralen et al., 2009; Aromolaran et al., 2021). All the statistical metrics were computed based on the scores from true positives (TP), true negatives (TN), false positives (FP), and false negatives (FN). TP and TN occur when both the model prediction and the experimental data agree that a gene is essential and non-essential, respectively. FP occur when the model says a gene is essential, but experiments suggest otherwise, whereas FN occur when the model says a gene is non-essential, but experiments indicate that it is essential (Becker and Palsson, 2008). The aforementioned statistical index-based metrics are described from Equations 1–6 as follows:
The accuracy measures the degree of correctness of a model with respect to both positive and negative classes. The sensitivity estimates the proportion of essential genes that have been correctly identified, whereas the specificity measures the proportion of true negatives that have been correctly predicted. The precision calculates the probability that the essential genes are correctly predicted. The F1-score represents the harmonic mean between precision and sensitivity, combining these two parameters into a single measure (Ghasemian et al., 2022). Lastly, Cohen’s Kappa coefficient measures the degree of agreement between the output of experimental versus predicted essentiality data. If Kappa = 1, then the predictions are in perfect agreement with experimental data, and Kappa = 0 means there is no agreement between predictions and experimental data (Aromolaran et al., 2021).
5.6. Monte Carlo flux sampling
The distribution of feasible fluxes in the condition-specific models was calculated by Markov chain Monte Carlo sampling (Schellenberger and Palsson, 2009) implemented in COBRA package (Schellenberger et al., 2011).
5.7. Identification of gene overexpression targets for ALA overproduction
The identification of gene amplification targets was based on the strategy of flux scanning based on enforced objective flux (FSEOF) (Choi et al., 2010). We first simulated the growth behavior of the strain using FBA and set the biomass-producing reaction as the objective function. Then, the maximum theoretical ALA production was obtained by setting the ALA exchange reaction (EX_ALA(e)) as the objective function. In the next steps, this reaction was raised stepwise to reach 80% of the theoretical maximum.
5.8. Identification of potential knockout targets for ALA overproduction
OptKnock (Burgard et al., 2003) and GDLS (Lun et al., 2009) algorithms were implemented to predict potential genetic knockout manipulations that can lead to ALA overproduction. Whereas OptKnock uses bi-level optimization strategies to solve the conflict of cell growth and maximum bioengineering objective, the GDLS algorithm employs reduced metabolic models and predicts gene knockouts based on Gene–Protein-Reaction associations (Xu Z. et al., 2013). Before using these two algorithms, GEM was reduced, including only nonblocked reactions catalyzed by proteins whose genes are nonessential, reactions not involved in transport and reactions with known GPR associations. This step generated a ‘reduced’ model. These methods were accessible through the COBRA Toolbox v2.0 in MATLAB. For both optimization methods, ALA production flux was set as the optimization target. Each reaction elimination design solution was examined by making the identified changes on bounds to the reactions obtained with OptKnock or GDLS, and were plotted using metabolic production envelope that represents the accessible flux space onto the plane of growth rate versus the target’s production rate (Edwards et al., 2002). The suggested reactions obtained with these algorithms can be removed in vivo by knocking out one or more of the genes encoding the enzymes catalyzing the reaction.
In addition, the recently developed gcFront algorithm was also used to identify knockouts that growth-couple synthesis (Legon et al., 2022). gcFront uses a multiobjective genetic algorithm that identifies a Pareto front of designs that maximize growth rate, product synthesis and coupling strength and finds combinations of gene/reaction knockouts that will enforce growth coupling (Legon et al., 2022). Before applying this algorithm, GEMs need to be pre-processed to reduce the search space of reaction by removing the biomass reactions not assigned as objective and blocked reactions. All blocked reactions were identified using flux variability analysis (FVA) (Mahadevan and Schilling, 2003) as reactions unable to carry flux when the biomass was constraint to 20%. To reduce computation time by gcFront algorithm, the list of reactions previously identified with Optknock screening with 1–5 maximum number of knockouts was used. Then, gcFront was executed for each nutritional condition (i.e., phototrophic, photomixotrophic with acetate and photomixotrophic with glucose) using the parameter setup described in Table 3.
5.9. Generation of specific GEMs for photomixotrophic conditions from iMS837_ALA
Reactions for the uptake of glucose and acetate were added to iMS837_ALA. The experimental data obtained under photomixotrophic conditions with acetate and glucose in vivo (Yan et al., 2012) were used to introduce constraints to iMS837_ALA to simulate these conditions in silico. A summary of these constraints is depicted in Table 4. In addition, a biomass equation was specifically generated for photomixotrophic conditions with acetate and glucose, denominated BOF_acetate_photomixotrophy and BOF_glucose_photomixotrophy, respectively. In order to do that, a previously published protocol to generate biomass objective functions from experimental data was used (Lachance et al., 2019). Condition-specific macromolecular data related to protein, lipid, carbohydrate, and photosynthetic pigment composition were obtained for previously published data (Yan et al., 2012). In addition, transcriptomic data and lipid profiles were also needed and obtained from different literature sources (Rós et al., 2013; Xiong et al., 2015).
5.10. Generation of pathway maps for visualization of metabolic fluxes
Escher was used for visualizing the fluxes of the metabolic pathways involved in ALA synthesis (King et al., 2015). The Escher website was used to draw all the metabolic maps.
Data availability statement
The original contributions presented in the study are included in the article/Supplementary material, further inquiries can be directed to the corresponding authors.
Author contributions
MS-M and JN conceived and designed the study. MS-M and JN prepared the first draft of the manuscript. MS-M performed the metabolic network reconstruction. MS-M and AG-B performed model simulations. All the authors discussed the results and participated in the writing process.
Funding
This work was supported by the European Union’s Horizon 2020 Research and Innovation Programme under Grant agreement no. 101000733 (Promicon) by the Spanish Ministry of Science and Innovation (MICINN) grants RobExplode PID2019-108458RB-I00 (AEI/10.13039/501100011033) and TED2021-130689B-C33 to JN, and PID2020-117923GB-I00 to FdlC. Funding was likewise provided by CSIC’s Interdisciplinary Platform for Sustainable Plastics toward a Circular Economy+ (PTI-SusPlast+). MS-M was the recipient of a Ph.D. fellowship (BES-2012-057387) from Spanish Ministry of Economy and Competitiveness (MINECO).
Acknowledgments
The authors wish to thank David San León Granado for his help with Figure 2.
Conflict of interest
The authors declare that the research was conducted in the absence of any commercial or financial relationships that could be construed as a potential conflict of interest.
Publisher’s note
All claims expressed in this article are solely those of the authors and do not necessarily represent those of their affiliated organizations, or those of the publisher, the editors and the reviewers. Any product that may be evaluated in this article, or claim that may be made by its manufacturer, is not guaranteed or endorsed by the publisher.
Supplementary material
The Supplementary material for this article can be found online at: https://www.frontiersin.org/articles/10.3389/fmicb.2023.1126030/full#supplementary-material
References
Aromolaran, O., Aromolaran, D., Isewon, I., and Oyelade, J. (2021). Machine learning approach to gene essentiality prediction: a review. Brief. Bioinform. 22:bbab128. doi: 10.1093/bib/bbab128
Becker, S. A., and Palsson, B. O. (2008). Three factors underlying incorrect in silico predictions of essential metabolic genes. BMC Syst. Biol. 2:14. doi: 10.1186/1752-0509-2-14
Berla, B. M., Saha, R., Immethun, C. M., Maranas, C. D., Moon, T. S., and Pakrasi, H. B. (2013). Synthetic biology of cyanobacteria: unique challenges and opportunities. Front. Microbiol. 4:246. doi: 10.3389/fmicb.2013.00246
Broddrick, J. T., Rubin, B. E., Welkie, D. G., Du, N., Mih, N., Diamond, S., et al. (2016). Unique attributes of cyanobacterial metabolism revealed by improved genome-scale metabolic modeling and essential gene analysis. Proc. Natl. Acad. Sci. U. S. A. 113, E8344–E8353. doi: 10.1073/pnas.1613446113
Broddrick, J. T., Welkie, D. G., Jallet, D., Golden, S. S., Peers, G., and Palsson, B. O. (2019). Predicting the metabolic capabilities of Synechococcus elongatus PCC 7942 adapted to different light regimes. Metab. Eng. 52, 42–56. doi: 10.1016/j.ymben.2018.11.001
Burgard, A. P., Pharkya, P., and Maranas, C. D. (2003). Optknock: a bilevel programming framework for identifying gene knockout strategies for microbial strain optimization. Biotechnol. Bioeng. 84, 647–657. doi: 10.1002/bit.10803
Chen, G., Qu, S., Wang, Q., Bian, F., Peng, Z., Zhang, Y., et al. (2014). Transgenic expression of delta-6 and delta-15 fatty acid desaturases enhances omega-3 polyunsaturated fatty acid accumulation in Synechocystis sp. PCC6803. Biotechnol. Biofuels 7:32. doi: 10.1186/1754-6834-7-32
Choi, H. S., Lee, S. Y., Kim, T. Y., and Woo, H. M. (2010). In silico identification of gene amplification targets for improvement of lycopene production. Appl. Environ. Microbiol. 76, 3097–3105. doi: 10.1128/AEM.00115-10
De Philippis, R., Sili, C., and Vincenzini, M. (1992). Glycogen and poly-β-hydroxybutyrate synthesis in Spirulina maxima. Microbiology 138, 1623–1628.
Diao, J., Song, X., Guo, T., Wang, F., Chen, L., and Zhang, W. (2020). Cellular engineering strategies toward sustainable omega-3 long chain polyunsaturated fatty acids production: state of the art and perspectives. Biotechnol. Adv. 40:107497. doi: 10.1016/j.biotechadv.2019.107497
Dong, X., He, Q., Peng, Z., Yu, J., Bian, F., Li, Y., et al. (2016). Production of Î3-linolenic acid and stearidonic acid by Synechococcus sp. PCC7002 containing cyanobacterial fatty acid desaturase genes. Chin. J. Oceanol. Limnol. 34, 772–780. doi: 10.1007/s00343-016-4369-x
Ebrahim, A., Lerman, J. A., Palsson, B. O., and Hyduke, D. R. (2013). COBRApy: COnstraints-based reconstruction and analysis for python. BMC Syst. Biol. 7:74. doi: 10.1186/1752-0509-7-74
Edwards, J. S., Ramakrishna, R., and Palsson, B. O. (2002). Characterizing the metabolic phenotype: a phenotype phase plane analysis. Biotechnol. Bioeng. 77, 27–36. doi: 10.1002/bit.10047
Esvelt, K. M., and Wang, H. H. (2013). Genome-scale engineering for systems and synthetic biology. Mol. Syst. Biol. 9:641. doi: 10.1038/msb.2012.66
Eungrasamee, K., Miao, R., Incharoensakdi, A., Lindblad, P., and Jantaro, S. (2019). Improved lipid production via fatty acid biosynthesis and free fatty acid recycling in engineered Synechocystis sp. PCC 6803. Biotechnol. Biofuels 12:8. doi: 10.1186/s13068-018-1349-8
Fekrat, F., Nami, B., Hejazi, M. A., Ghaffari, M. R., and Shahbazi, M. (2021). Correlation network analysis of metabolites reveals the role of nitrogen-containing metabolic stressors in stimulating high-value compounds biosynthesis in Arthrospira platensis. J. Appl. Phycol. 9, 1967–1982. doi: 10.1007/s10811-022-02753-8
Galán, B., Santos-Merino, M., Nogales, J., De La Cruz, F., and García, J. L. (2019). “Microbial oils as nutraceuticals and animal feeds” in Health Consequences of Microbial Interactions with Hydrocarbons, Oils, and Lipids. ed. H. Goldfine (Cham: Springer International Publishing), 1–45.
Ghasemian, B., Shahabi, H., Shirzadi, A., Al-Ansari, N., Jaafari, A., Geertsema, M., et al. (2022). Application of a novel hybrid machine learning algorithm in shallow landslide susceptibility mapping in a mountainous area. Front. Environ. Sci. 10:897254. doi: 10.3389/fenvs.2022.897254
Gu, C., Kim, G. B., Kim, W. J., Kim, H. U., and Lee, S. Y. (2019). Current status and applications of genome-scale metabolic models. Genome Biol. 20:121. doi: 10.1186/s13059-019-1730-3
Gudmundsson, S., and Nogales, J. (2015). Cyanobacteria as photosynthetic biocatalysts: a systems biology perspective. Mol. BioSyst. 11, 60–70. doi: 10.1039/C4MB00335G
Gudmundsson, S., and Nogales, J. (2021). Recent advances in model-assisted metabolic engineering. Curr. Opin. Syst. Biol. 28:100392. doi: 10.1016/j.coisb.2021.100392
Hendry, J. I., Bandyopadhyay, A., Srinivasan, S., Pakrasi, H. B., and Maranas, C. D. (2020). Metabolic model guided strain design of cyanobacteria. Curr. Opin. Biotechnol. 64, 17–23. doi: 10.1016/j.copbio.2019.08.011
Herrmann, H. A., Dyson, B. C., Vass, L., Johnson, G. N., and Schwartz, J. M. (2019). Flux sampling is a powerful tool to study metabolism under changing environmental conditions. NPJ Syst. Biol. Appl. 5:32. doi: 10.1038/s41540-019-0109-0
Kanno, M., Carroll, A. L., and Atsumi, S. (2017). Global metabolic rewiring for improved CO2 fixation and chemical production in cyanobacteria. Nat. Commun. 8:14724. doi: 10.1038/ncomms14724
Khetkorn, W., Incharoensakdi, A., Lindblad, P., and Jantaro, S. (2016). Enhancement of poly-3-hydroxybutyrate production in Synechocystis sp. PCC 6803 by overexpression of its native biosynthetic genes. Bioresour. Technol. 214, 761–768. doi: 10.1016/j.biortech.2016.05.014
King, Z. A., Drager, A., Ebrahim, A., Sonnenschein, N., Lewis, N. E., and Palsson, B. O. (2015). Escher: a web application for building, sharing, and embedding data-rich visualizations of biological pathways. PLoS Comput. Biol. 11:e1004321. doi: 10.1371/journal.pcbi.1004321
Labella, J. I., Llop, A., and Contreras, A. (2020). The default cyanobacterial linked genome: an interactive platform based on cyanobacterial linkage networks to assist functional genomics. FEBS Lett. 594, 1661–1674. doi: 10.1002/1873-3468.13775
Lachance, J. C., Lloyd, C. J., Monk, J. M., Yang, L., Sastry, A. V., Seif, Y., et al. (2019). BOFdat: generating biomass objective functions for genome-scale metabolic models from experimental data. PLoS Comput. Biol. 15:e1006971. doi: 10.1371/journal.pcbi.1006971
Legon, L., Corre, C., Bates, D. G., and Mannan, A. A. (2022). gcFront: a tool for determining a Pareto front of growth-coupled cell factory designs. Bioinformatics 38, 3657–3659. doi: 10.1093/bioinformatics/btac376
Lewis, N. E., Nagarajan, H., and Palsson, B. O. (2012). Constraining the metabolic genotype-phenotype relationship using a phylogeny of in silico methods. Nat. Rev. Microbiol. 10, 291–305. doi: 10.1038/nrmicro2737
Liao, X., Ma, H., and Tang, Y. J. (2022). Artificial intelligence: a solution to involution of design-build-test-learn cycle. Curr. Opin. Biotechnol. 75:102712. doi: 10.1016/j.copbio.2022.102712
Lieven, C., Beber, M. E., Olivier, B. G., Bergmann, F. T., Ataman, M., Babaei, P., et al. (2020). MEMOTE for standardized genome-scale metabolic model testing. Nat. Biotechnol. 38, 272–276. doi: 10.1038/s41587-020-0446-y
Ludwig, M., and Bryant, D. A. (2012). Synechococcus sp. strain PCC 7002 Ttranscriptome: acclimation to temperature, salinity, oxidative stress, and mixotrophic growth conditions. Front. Microbiol. 3:354. doi: 10.3389/fmicb.2012.00354
Lun, D. S., Rockwell, G., Guido, N. J., Baym, M., Kelner, J. A., Berger, B., et al. (2009). Large-scale identification of genetic design strategies using local search. Mol. Syst. Biol. 5:296. doi: 10.1038/msb.2009.57
Mahadevan, R., and Schilling, C. H. (2003). The effects of alternate optimal solutions in constraint-based genome-scale metabolic models. Metab. Eng. 5, 264–276. doi: 10.1016/j.ymben.2003.09.002
Mcewen, J. T., Machado, I. M., Connor, M. R., and Atsumi, S. (2013). Engineering Synechococcus elongatus PCC 7942 for continuous growth under diurnal conditions. Appl. Environ. Microbiol. 79, 1668–1675. doi: 10.1128/AEM.03326-12
Mills, L. A., Mccormick, A. J., and Lea-Smith, D. J. (2020). Current knowledge and recent advances in understanding metabolism of the model cyanobacterium Synechocystis sp PCC 6803. Biosci. Rep. 40:BSR20193325.
Monk, J. M., Lloyd, C. J., Brunk, E., Mih, N., Sastry, A., King, Z., et al. (2017). iML1515, a knowledgebase that computes Escherichia coli traits. Nat. Biotechnol. 35, 904–908. doi: 10.1038/nbt.3956
Nogales, J., Gudmundsson, S., Knight, E. M., Palsson, B. O., and Thiele, I. (2012). Detailing the optimality of photosynthesis in cyanobacteria through systems biology analysis. Proc. Natl. Acad. Sci. U. S. A. 109, 2678–2683. doi: 10.1073/pnas.1117907109
Nogales, J., Gudmundsson, S., and Thiele, I. (2013). Toward systems metabolic engineering in cyanobacteria: opportunities and bottlenecks. Bioengineered 4, 158–163. doi: 10.4161/bioe.22792
Nogales, J., Mueller, J., Gudmundsson, S., Canalejo, F. J., Duque, E., Monk, J., et al. (2020). High-quality genome-scale metabolic modelling of pseudomonas putida highlights its broad metabolic capabilities. Environ. Microbiol. 22, 255–269. doi: 10.1111/1462-2920.14843
Nozzi, N. E., and Atsumi, S. (2015). Genome engineering of the 2,3-butanediol biosynthetic pathway for tight regulation in cyanobacteria. ACS Synth. Biol. 4, 1197–1204. doi: 10.1021/acssynbio.5b00057
Oberhardt, M. A., Palsson, B. O., and Papin, J. A. (2009). Applications of genome-scale metabolic reconstructions. Mol. Syst. Biol. 5:320. doi: 10.1038/msb.2009.77
Oliver, J. W., Machado, I. M., Yoneda, H., and Atsumi, S. (2013). Cyanobacterial conversion of carbon dioxide to 2,3-butanediol. Proc. Natl. Acad. Sci. U. S. A. 110, 1249–1254. doi: 10.1073/pnas.1213024110
Orth, J. D., Conrad, T. M., Na, J., Lerman, J. A., Nam, H., Feist, A. M., et al. (2011). A comprehensive genome-scale reconstruction of Escherichia coli metabolism--2011. Mol. Syst. Biol. 7:535. doi: 10.1038/msb.2011.65
Orth, J. D., Thiele, I., and Palsson, B. O. (2010). What is flux balance analysis? Nat. Biotechnol. 28, 245–248. doi: 10.1038/nbt.1614
Panda, B., Jain, P., Sharma, L., and Mallick, N. (2006). Optimization of cultural and nutritional conditions for accumulation of poly-beta-hydroxybutyrate in Synechocystis sp. PCC 6803. Bioresour. Technol. 97, 1296–1301. doi: 10.1016/j.biortech.2005.05.013
Park, J. M., Park, H. M., Kim, W. J., Kim, H. U., Kim, T. Y., and Lee, S. Y. (2012). Flux variability scanning based on enforced objective flux for identifying gene amplification targets. BMC Syst. Biol. 6:106. doi: 10.1186/1752-0509-6-106
Patel, A., Karageorgou, D., Rova, E., Katapodis, P., Rova, U., Christakopoulos, P., et al. (2020). An overview of potential oleaginous microorganisms and their role in biodiesel and omega-3 fatty acid-based industries. Microorganisms 8:434. doi: 10.3390/microorganisms8030434
Poole, L. B., Parsonage, D., Sergeant, S., Miller, L. R., Lee, J., Furdui, C. M., et al. (2020). Acyl-lipid desaturases and Vipp1 cooperate in cyanobacteria to produce novel omega-3 PUFA-containing glycolipids. Biotechnol. Biofuels 13:83. doi: 10.1186/s13068-020-01719-7
Rajneesh,, Singh, S. P., Pathak, J., and Sinha, R. P. (2017). Cyanobacterial factories for the production of green energy and value-added products: an integrated approach for economic viability. Renew. Sust. Energ. Rev. 69, 578–595. doi: 10.1016/j.rser.2016.11.110
Rós, P., Silva, C. S., Silva-Stenico, M. E., Fiore, M. F., and De Castro, H. F. (2013). Assessment of chemical and physico-chemical properties of cyanobacterial lipids for biodiesel production. Mar. Drugs 11, 2365–2381. doi: 10.3390/md11072365
Rubin, B. E., Wetmore, K. M., Price, M. N., Diamond, S., Shultzaberger, R. K., Lowe, L. C., et al. (2015). The essential gene set of a photosynthetic organism. Proc. Natl. Acad. Sci. U. S. A. 112, E6634–E6643. doi: 10.1073/pnas.1519220112
Sakamoto, T., Higashi, S., Wada, H., Murata, N., and Bryant, D. A. (1997). Low-temperature-induced desaturation of fatty acids and expression of desaturase genes in the cyanobacterium Synechococcus sp. PCC 7002. FEMS Microbiol. Lett. 152, 313–320. doi: 10.1111/j.1574-6968.1997.tb10445.x
Santos-Merino, M., Garcillan-Barcia, M. P., and De La Cruz, F. (2018). Engineering the fatty acid synthesis pathway in Synechococcus elongatus PCC 7942 improves omega-3 fatty acid production. Biotechnol. Biofuels 11:239. doi: 10.1186/s13068-018-1243-4
Santos-Merino, M., Gutiérrez-Lanza, R., Nogales, J., Garcia, J. L., and De La Cruz, F. (2022). Synechococcus elongatus PCC 7942 as a platform for bioproduction of omega-3 fatty acids. Life 12:810. doi: 10.3390/life12060810
Santos-Merino, M., Singh, A. K., and Ducat, D. C. (2019). New applications of synthetic biology tools for cyanobacterial metabolic engineering. Front. Bioeng. Biotechnol. 7:33. doi: 10.3389/fbioe.2019.00033
Schellenberger, J., and Palsson, B. O. (2009). Use of randomized sampling for analysis of metabolic networks. J. Biol. Chem. 284, 5457–5461. doi: 10.1074/jbc.R800048200
Schellenberger, J., Que, R., Fleming, R. M., Thiele, I., Orth, J. D., Feist, A. M., et al. (2011). Quantitative prediction of cellular metabolism with constraint-based models: the COBRA toolbox v2.0. Nat. Protoc. 6, 1290–1307. doi: 10.1038/nprot.2011.308
Sharma, L., and Mallick, N. (2005). Enhancement of poly-beta-hydroxybutyrate accumulation in Nostoc muscorum under mixotrophy, chemoheterotrophy and limitations of gas-exchange. Biotechnol. Lett. 27, 59–62. doi: 10.1007/s10529-004-6586-1
Shinde, S., Singapuri, S., Jiang, Z., Long, B., Wilcox, D., Klatt, C., et al. (2022). Thermodynamics contributes to high limonene productivity in cyanobacteria. Metab. Eng. Commun. 14:e00193. doi: 10.1016/j.mec.2022.e00193
Tan, X., Yao, L., Gao, Q., Wang, W., Qi, F., and Lu, X. (2011). Photosynthesis driven conversion of carbon dioxide to fatty alcohols and hydrocarbons in cyanobacteria. Metab. Eng. 13, 169–176. doi: 10.1016/j.ymben.2011.01.001
Tasaka, Y., Gombos, Z., Nishiyama, Y., Mohanty, P., Ohba, T., Ohki, K., et al. (1996). Targeted mutagenesis of acyl-lipid desaturases in Synechocystis: evidence for the important roles of polyunsaturated membrane lipids in growth, respiration and photosynthesis. EMBO J. 15, 6416–6425. doi: 10.1002/j.1460-2075.1996.tb01033.x
Towijit, U., Songruk, N., Lindblad, P., Incharoensakdi, A., and Jantaro, S. (2018). Co-overexpression of native phospholipid-biosynthetic genes plsX and plsC enhances lipid production in Synechocystis sp. PCC 6803. Sci. Rep. 8:13510.
Triana, J., Montagud, A., Siurana, M., Fuente, D., Urchueguia, A., Gamermann, D., et al. (2014). Generation and evaluation of a genome-scale metabolic network model of Synechococcus elongatus PCC7942. Meta 4, 680–698. doi: 10.3390/metabo4030680
Van Stralen, K. J., Stel, V. S., Reitsma, J. B., Dekker, F. W., Zoccali, C., and Jager, K. J. (2009). Diagnostic methods I: sensitivity, specificity, and other measures of accuracy. Kidney Int. 75, 1257–1263. doi: 10.1038/ki.2009.92
Vavitsas, K., Kugler, A., Satta, A., Hatzinikolaou, D. G., Lindblad, P., Fewer, D. P., et al. (2021). Doing synthetic biology with photosynthetic microorganisms. Physiol. Plant. 173, 624–638. doi: 10.1111/ppl.13455
Wan, N., Abernathy, M., Tang, J. K.-H., Tang, Y. J., and You, L. (2015). Cyanobacterial photo-driven mixotrophic metabolism and its advantages for biosynthesis. Front. Chem. Sci. Eng. 9, 308–316. doi: 10.1007/s11705-015-1521-7
Wang, W., Liu, X., and Lu, X. (2013). Engineering cyanobacteria to improve photosynthetic production of alka(e)nes. Biotechnol. Biofuels 6:69. doi: 10.1186/1754-6834-6-69
Wei, W., Ning, L. W., Ye, Y. N., and Guo, F. B. (2013). Geptop: a gene essentiality prediction tool for sequenced bacterial genomes based on orthology and phylogeny. PLoS One 8:e72343. doi: 10.1371/journal.pone.0072343
Wu, G. F., Shen, Z. Y., and Wu, Q. Y. (2002). Modification of carbon partitioning to enhance PHB production in Synechocystis sp. PCC6803. Enzym. Microb. Technol. 30, 710–715. doi: 10.1016/S0141-0229(02)00044-3
Xiong, Q., Feng, J., Li, S. T., Zhang, G. Y., Qiao, Z. X., Chen, Z., et al. (2015). Integrated transcriptomic and proteomic analysis of the global response of Synechococcus to high light stress. Mol. Cell. Proteomics 14, 1038–1053. doi: 10.1074/mcp.M114.046003
Xu, C., Liu, L., Zhang, Z., Jin, D., Qiu, J., and Chen, M. (2013). Genome-scale metabolic model in guiding metabolic engineering of microbial improvement. Appl. Microbiol. Biotechnol. 97, 519–539. doi: 10.1007/s00253-012-4543-9
Xu, Z., Zheng, P., Sun, J., and Ma, Y. (2013). ReacKnock: identifying reaction deletion strategies for microbial strain optimization based on genome-scale metabolic network. PLoS One 8:e72150. doi: 10.1371/journal.pone.0072150
Yan, R., Zhu, D., Zhang, Z., Zeng, Q., and Chu, J. (2012). Carbon metabolism and energy conversion of Synechococcus sp. PCC 7942 under mixotrophic conditions: comparison with photoautotrophic condition. J. Appl. Phycol. 24, 657–668. doi: 10.1007/s10811-011-9683-2
Yoshino, T., Kakunaka, N., Liang, Y., Ito, Y., Maeda, Y., Nomaguchi, T., et al. (2017). Production of omega3 fatty acids in marine cyanobacterium Synechococcus sp. strain NKBG 15041c via genetic engineering. Appl. Microbiol. Biotechnol. 101, 6899–6905. doi: 10.1007/s00253-017-8407-1
Zahra, Z., Choo, D. H., Lee, H., and Parveen, A. (2020). Cyanobacteria: review of current potentials and applications. Environments 7:13. doi: 10.3390/environments7020013
Keywords: cyanobacteria, Synechococcus elongatus PCC 7942, genome-scale metabolic model, strain-designing algorithms, α-linolenic acid
Citation: Santos-Merino M, Gargantilla-Becerra Á, de la Cruz F and Nogales J (2023) Highlighting the potential of Synechococcus elongatus PCC 7942 as platform to produce α-linolenic acid through an updated genome-scale metabolic modeling. Front. Microbiol. 14:1126030. doi: 10.3389/fmicb.2023.1126030
Edited by:
Xiaoming Tan, Hubei University, ChinaReviewed by:
Weiwen Zhang, Tianjin University, ChinaFei Gan, University of California, Berkeley, United States
Copyright © 2023 Santos-Merino, Gargantilla-Becerra, de la Cruz and Nogales. This is an open-access article distributed under the terms of the Creative Commons Attribution License (CC BY). The use, distribution or reproduction in other forums is permitted, provided the original author(s) and the copyright owner(s) are credited and that the original publication in this journal is cited, in accordance with accepted academic practice. No use, distribution or reproduction is permitted which does not comply with these terms.
*Correspondence: María Santos-Merino, c2FudG9zbTdAbXN1LmVkdQ==; Juan Nogales, ai5ub2dhbGVzQGNzaWMuZXM=
†Present address: María Santos-Merino, MSU-DOE Plant Research Laboratory, Michigan State University, East Lansing, MI, United States