- 1Pediatrics Research Institute of Hunan Province, Hunan Children’s Hospital, Changsha, China
- 2Academy of Pediatrics, Hengyang Medical School, University of South China, Hengyang, China
- 3Department of Emergency Center, Hunan Children’s Hospital, Changsha, China
- 4Department of Neonatology, Hunan Children’s Hospital, Changsha, China
Background and objective: Neonatal jaundice is a common clinical disease in neonates. Pathologic jaundice is more harmful to neonates. There are a few studies on the biomarkers of pathologic jaundice and the correlation between gut microbiota and clinical indices. Therefore, we aimed to reveal the characteristics of gut microbiota in pathologic jaundice, provide potential biomarkers for the diagnosis of pathologic jaundice, and find the correlation between gut microbiota and clinical indices.
Methods: Fourteen neonates with physiologic jaundice were recruited into a control group (Group A). Additionally, 14 neonates with pathologic jaundice were recruited into a case group (Group B). The microbial communities were analyzed using 16S rDNA sequencing. LEfSe and the differences in the relative abundance of gut microbiota were used to identify different bacteria among the two groups. The ROC curve was used to assess effective biomarkers for pathologic jaundice. Spearman’s rank-sum correlation coefficient was used to evaluate the correlation between gut microbiota and clinical indices.
Results: There were no differences in the total richness or diversity of gut microbiota between the two groups. At the phylum and genus levels, compared with the control group, Bacteroidetes (p = 0.002) and Braydrhizobium (p = 0.01) were significantly higher, while Actinobacteria (p = 0.003) and Bidfldobacterium (p = 0.016) were significantly lower in the case group. Bacteroidetes were valuable in differentiating pathologic jaundice from physiologic jaundice by the ROC curve, and the area under the ROC curve (AUC) value was 0.839 [95%CI (0.648–0.995)]. In the case group, Bacteroidetes were negatively associated with total bilirubin (TBIL) (p < 0.05). In the control group, Bacteroidetes were positively associated with TBIL (p < 0.05).
Conclusion: Bacteroidetes could be used as biomarkers to identify pathologic jaundice and Bacteroidetes are positively associated with bilirubin levels.
1. Introduction
Neonatal jaundice describes a condition in which an infant’s skin appears yellow by the accumulation of bilirubin (Dennery et al., 2001). It is a common clinical disease, that occurs in 80% of newborn babies (Rennie et al., 2010; Ng and How, 2015). Due to excessive generation of bilirubin, poor ability to deal with bilirubin, the insufficient serum albumin binding bilirubin ability, and increase of enterohepatic circulation influence, neonates cannot keep clear of bilirubin in the body in time, resulting in jaundice (Brits et al., 2018). Most the neonates with jaundice are relatively light conditions of physiologic jaundice (Fan and Xu, 2016). Pathologic jaundice is considered when jaundice appears early, increases too high or too fast, sustains for long terms, worsens progressively, or combines with pathologic factors (Boskabadi et al., 2020). Pathologic jaundice, if not treated in time, could cause bilirubin encephalopathy (kern jaundice) which leads to permanent damage to the nervous system and seriously affect the intellectual development and healthy growth of children (Fan and Xu, 2016). Moreover, pathologic jaundice will increase the risk of some diseases, such as childhood allergic diseases (Kuniyoshi et al., 2021), and autism spectrum disorder (Kujabi et al., 2021). Pathologic jaundice in neonates is caused by various factors, such as infection, genetic defects, and environmental factors (Akagawa et al., 2021).
Some studies have shown that the imbalance of gut microbiota, such as Firmicutes, Proteobacteria, Bifidobacteriales, Escherichia, and so on (Li et al., 2020; Akagawa et al., 2021; Ding et al., 2021), is one of the pathogenic factors of jaundice. Gut microbiota plays an important role in regulating bilirubin metabolism through enterohepatic circulation (Vitek et al., 2005). In the process of bilirubin metabolism, part of the conjugated bilirubin discharged into the intestine can be reduced to stercobilinogen and then discharged with the fecal under the action of gut microbiota. The other part can be hydrolyzed by intestinal β-glucuronidase (β-GD) to unconjugated bilirubin (Billing, 1978). Subsequently, unconjugated bilirubin can be reabsorbed through the intestinal wall by the portal vein and discharged into the intestine with bile acids after liver metabolism which forms enterohepatic circulation. However, the lack of gut microbiota transforming conjugated bilirubin in the intestine can cause the increase of bilirubin enterohepatic circulation (Sun et al., 2013), thus aggravating jaundice.
There are a few studies on the association and mechanism between gut microbiota and pathologic jaundice (Li et al., 2020). And there are a few studies on the biomarkers of pathologic jaundice and the correlation between gut microbiota and clinical indices (Zhou et al., 2019). Therefore, this study adopted the method of case–control study and the high-throughput 16S rDNA gene sequencing to detect the composition of gut microbiota in feces of neonates with physiologic jaundice and pathologic jaundice, and further reveal the characteristics of gut microbiota in neonatal pathologic jaundice, provide potential biomarkers for the diagnosis of pathologic jaundice, and find the correlation between gut microbiota and clinical indices.
2. Materials and methods
2.1. Study subjects
We selected 46 neonates with jaundice hospitalized in the Neonatology Department of Hunan Children’s Hospital from August 2018 to January 2019. According to the diagnostic criteria of neonatal physiologic jaundice and pathologic jaundice (Li and Feng, 2006; Shao et al., 2010), 25 neonates with physiologic jaundice were recruited into the control group and 21 neonates with pathologic jaundice were recruited into the case group. In the control group, three neonates with sepsis, two neonates with intracranial infection, and six neonates with digestive system diseases were excluded. Additionally, in the case group, four neonates with sepsis, one neonate with intracranial infection and, two neonates with digestive system diseases were excluded. Finally, there were 14 neonates with physiologic jaundice in the control group (Group A) and 14 neonates with pathologic jaundice in the case group (Group B). The recruitment process of all the subjects was shown in Figure 1. The study was approved by the Ethics Committee of Hunan Children’s Hospital (No. HCHLL-2018-64). Written informed consent was obtained from the parents and/or legal guardians of the enrolled children.
2.2. Fecal samples and general information collection
Fecal samples of neonates’ first defecation after hospitalization were collected by stool collection kit provided by Genesky Biotechnologies Inc., Shanghai, 201315 (China) and immediately frozen in ice boxes. The samples were then transported to the laboratory within 30 min and stored at −80°C. The hospitalization data of neonates were extracted from the medical record system. Collect neonates’ data of birth weightbirth weigh, age, gender, gestational age, delivery mode, the use of antibiotics, feeding status, maternal age during pregnancy, clinical diagnosis, C-reactive protein (CRP), total bilirubin (TBIL), direct bilirubin (DBIL), alanine aminotransferase (ALT), and aspartate aminotransferase (AST).
2.3. DNA extraction and high-throughput 16S rDNA gene sequencing
16S rDNA amplicon sequencing was performed by Genesky Biotechnologies Inc., Shanghai, 201315 (China). Total genomic DNA was extracted using the QIAamp Fast DNA Stool Mini Kit (QIAGEN ART.NO.56104). The integrity of genomic DNA was detected through agarose gel electrophoresis, and the concentration and purity of genomic DNA were detected through the Nanodrop 2000 and Qubit 3.0 Spectrophotometer. The V4–V5 hypervariable regions of the 16S rDNA gene were amplified with the primers 515F (5′-GTGCCAGCMGCCGCGG-3′) and 907R (5′-CCGTCAATTCMTTTR AGTTT-3′) (Xiong et al., 2012) and then sequenced using Illumina NovaSeq 6000 platform (Zhou et al., 2022). The sequencing data were deposited to NCBI’s Sequencing Read Archive with the accession ID PRJNA926124.
2.4. Gut microbial analysis
The raw read sequences were further filtered to remove adapter sequences, the primers, and low-quality reads by QIIME2 (Bolyen et al., 2019) and the cutadapt plugin to improve the accuracy of later analysis. The filtered sequences were clustered into operational taxonomic units (OTUs) of ≥97% similarity, and the sequence with the highest abundance was considered a representative sequence within each cluster (Qiu et al., 2022).
The species accumulation curve of the sample analyzed with QIIME2 was used to assess the rationality of sample content. Alpha diversity was evaluated using abundance indices and diversity indices. Chao 1 and ACE represent abundance and Shannon and Simpson represent diversity. Venn diagram which visualized with QIIME2 based on OTUs abundance was used to show the richness and similarity of gut microbiota composition within the groups. Principal component analysis (PCA) in Beta diversity (based on Bray–Curtis distance calculated with QIIME2) was used to evaluate community composition and structure of gut microbiota. Lefse analysis (Segata et al., 2011) was used to obtain species with significant differences in abundance between the control group and the case group by the linear discriminant analysis (LDA) histogram and the cladogram. The differences in the relative abundance of gut microbiota at phylum and genus levels were used to evaluate the differences in gut microbiota composition between the control group and the case group. The ROC curve calculated and displayed with R software (Version 4.1.3) was used to assess effective biomarkers for pathologic jaundice. Principal component analysis (Ringnér, 2008) based on the phylum and genus levels of gut microbiota was used to evaluate the value of their contribution to jaundice. Heatmap (Metsalu and Vilo, 2015) for Spearman’s rank-sum correlation coefficient was used to evaluate the correlation between gut microbiota and clinical indices.
2.5. Statistical analysis
The experimental data were analyzed by SPSS (Version 25) and R software (Version 4.1.3). For measurement data, if they were normal distribution (e.g., maternal age, Gestational age, TBIL, and Birth weight), they were expressed as mean ± SD (X ± S), and independent sample t-test was used for comparison difference between the two groups; if they were non-normal distribution (e.g., Age, CRP, ALT, AST, DBIL, Alpha diversity analysis index, and the relative abundance of bacteria), they were expressed as the median and interquartile range [M (P25,P75)], and rank sum test was used for comparison difference between the two groups. Categorical data were expressed as percentages, and the comparison differences between the two groups were evaluated by the chi-square test. The correlation between gut microbiota and clinical indices was evaluated by Spearman’s rank-sum correlation coefficient. Value of p < 0.05 was considered to be statistically significant.
3. Results
3.1. Clinical characteristics of the neonates
The clinical characteristics of the neonates are showed in Table 1. There were no significant differences in gender, feeding status, delivery mode, the use of antibiotics, age, maternal age, gestational age, birth weight, CRP, ALT, and AST between the two groups. The peak value of serum total bilirubin in the control group was 152.44 ± 38.24 μmol/L, and in the case group was 300.66 ± 105.97 μmol/L. There were significant differences between the two groups (p < 0.001).
3.2. Diversity analysis of gut microbiota
According to the species accumulation curve of the sample (Supplementary Figure 1), a total of 28 samples participated in the analysis in this study, and the sample size was sufficient, which could fully reflect the richness of the microbiota. Alpha diversity analysis among the two groups found that there were no significant differences in the alpha diversity index (Figure 2A). In the control group, the median and interquartile range of ACE index, Chao index, Shannon index, and Simpson index were 49.37 (27.93, 92.91), 43.50 (25.19, 86.55), 0.93 (0.43, 1.22), and 0.53 (0.35, 0.76), respectively. In the case group, the median and interquartile range of ACE index, Chao index, Shannon index, and Simpson index were 66.11 (38.71,169.56), 54.67 (30.43, 137.88), 1.02 (0.56, 1.18), and 0.46 (0.35, 0.76), respectively. Venn diagram showed that 97 out of 383 total OTUs were shared among the two groups (Figure 2B). Principal component analysis in Beta diversity found that the gut microbiota composition in the two groups had a high degree of similarity, and it could not be significantly distinguished from the serum bilirubin levels (Figure 2C).
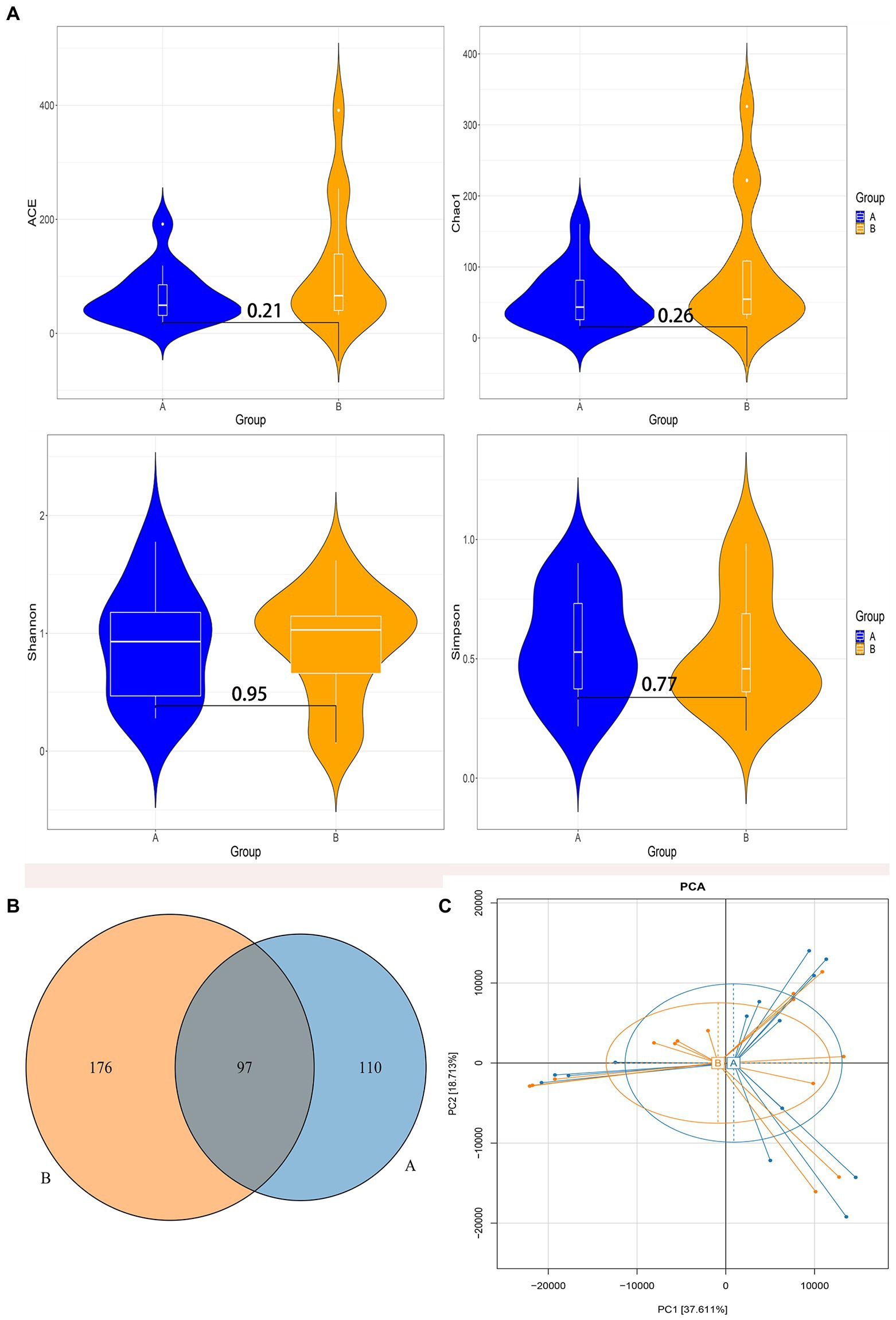
Figure 2. Diversity analysis of gut microbiota. (A) The comparison of the Alpha diversity index between the two groups. (B) Venn diagram of operational taxonomic units (OTUs) among the two groups. (C) Principal component analysis based on OTUs abundance table. Group A: the control group; Group B: the case group.
3.3. Differences in taxa between the control group and the case group
The LDA histogram showed eight bacteria (p, c, o, f, and g, respectively, representing phylum, class, order, family, and genus level) that were enriched in the fecal samples of the control group and ten bacteria that were enriched in the fecal samples of the case group (Figure 3A). Bacteroidetes, Bacterodales, Bacteroidia, Braydrhizobium, Rhizobiales, Braydrhizobiaceae, Planctomycetes, Planctomycetia, Cytophagales, and Cytophagia were abundant in the case group. As well as, Rothia, pelomonas, Bidfldobacteriales, Bidfldobacterium, Bidfldobacteriaceae, Actinobacteria (c), Actinobacteria (p), and Actinomycetales were abundant in the control group. From the LEfSe cladogram, we also found that these important bacteria were significantly abundant in the different groups (Figure 3B).
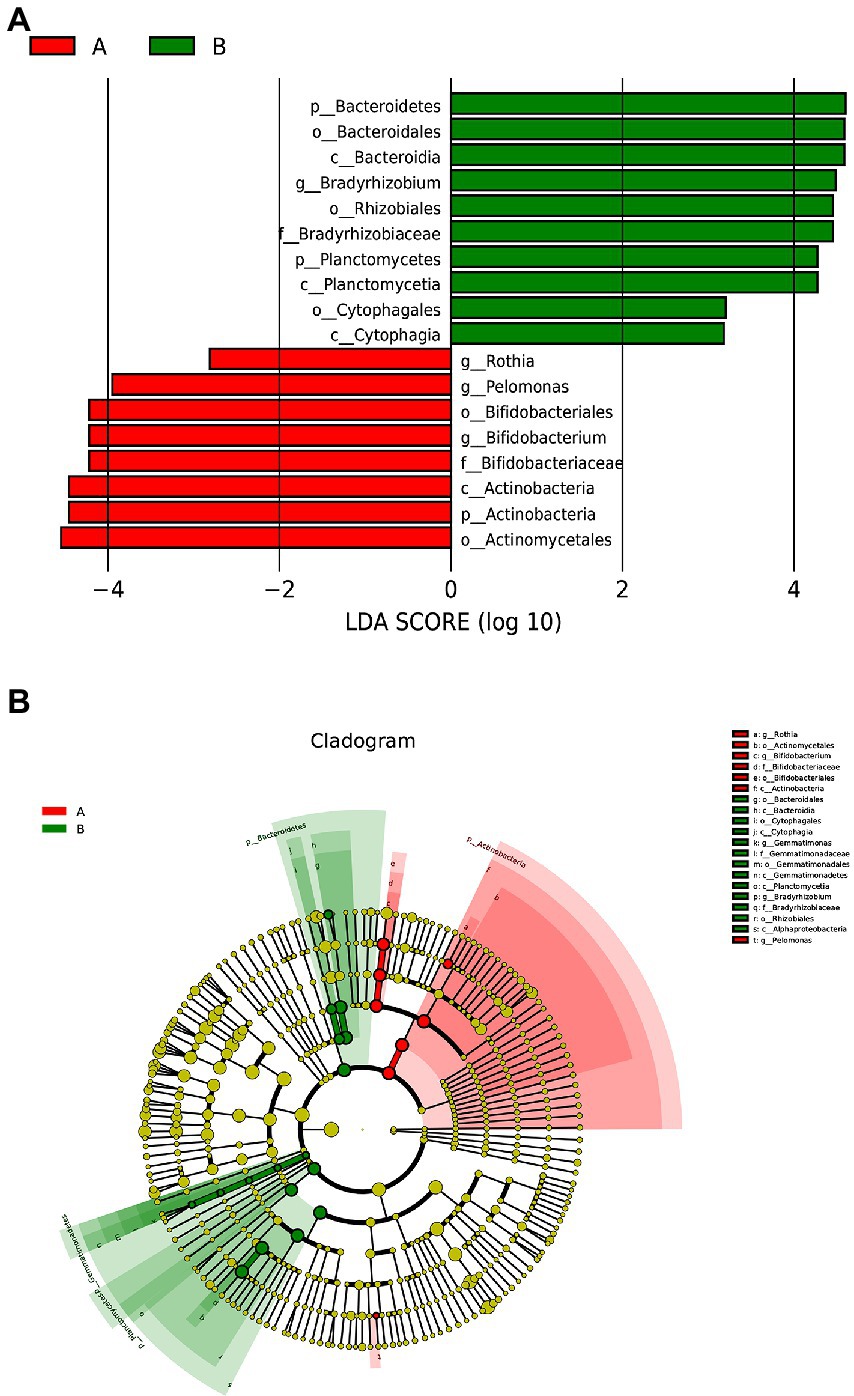
Figure 3. Lefse analysis between the two groups. (A) Histogram of LDA scores, showing significant differences in microbe type and abundance. LDA scores on the log 10 scale are indicated at the bottom. (B) Cladogram generated by LEfSe.
We mainly compared the differences in the taxa at the phylum and genus levels. At the phylum level (Figure 4), compared with the control group, the relative abundance of Bacteroidetes [0.00027 (0.00005, 0.00830) vs. 0.00015 (0, 0.00004), p = 0.002] and Planctomycetes [0.00005 (0, 0.001889) vs. 0(0, 0.00003), p = 0.015] were significantly higher in the case group, while Actinobacteria [0.00029(0.00005, 0.00074) vs. 0.01190(0.00049, 0.09004), p = 0.003] were significantly lower in the case group; At the genus level (Figure 5), compared with the control group, the relative abundance of Braydrhizobium [0.00003(0, 0.02207) vs. 0(0,0), p = 0.01] was significantly higher in the case group, while Bidfldobacterium [0.00003 (0, 0.00006) vs. 0.0006 (0.00002, 0.04192), p = 0.016] and Rothia [0(0, 0.00017) vs. 0.00015 (0.00003, 0.00109), p = 0.038] were significantly lower in the case group.
The ROC curve was used to assess effective biomarkers for pathologic jaundice (Figure 6). The results showed that a clear distinction between physiologic jaundice and pathologic jaundice could be achieved based on Bacteroidetes with the biggest AUC value of 0.839 [95%CI (0.648–0.995)], meaning that Bacteroidetes are effective biomarkers of pathologic jaundice. Besides, Actinobacteria, Bidfldobacterium, Rothia, Planctomycetes, and Bradyrhizobium with the area under the ROC curve (AUC) values of 0.827, 0.763, 0.724, 0.755, and 0.763 (Supplementary Figure 2).
Principal component analysis based on the phylum and genus levels of gut microbiota was used to evaluate the value of their contribution to jaundice (Figure 7). It showed that gut microbiota at phylum and genus levels could not separate the two groups. However, the differences in principal components at the phylum level were mainly contributed by Firmicutes and Proteobacteria (Figure 7A); the differences in principal components at the genus level was mainly contributed by Gemmatimonas (Figure 7B).
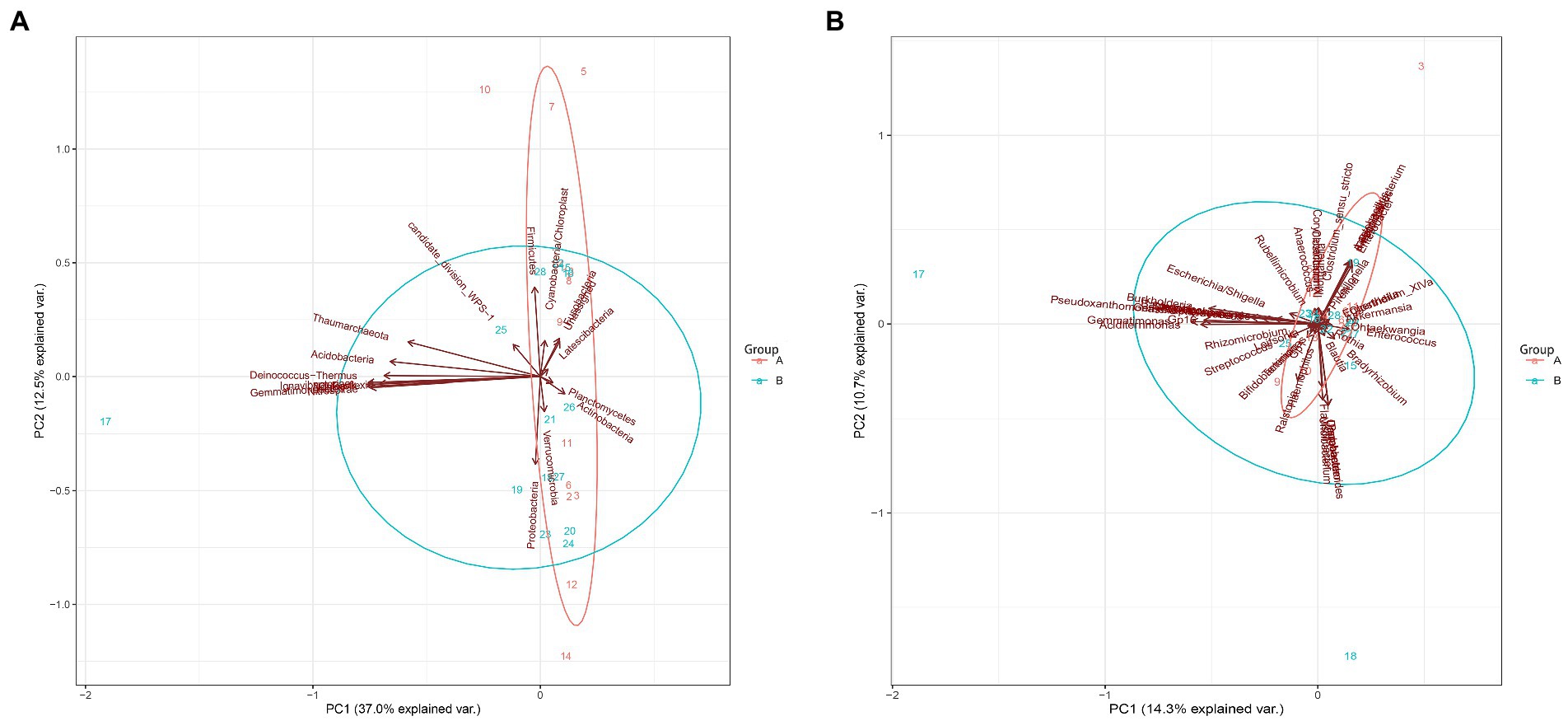
Figure 7. Principal component analysis between the control group and the case group at the phylum and genus levels. (A) The phylum level. (B) The genus levels.
3.4. Correlation between gut microbiota and clinical indices
In the case group (Figure 8), Bacteroidetes and Staphylococcus were negatively associated with TBIL; Escherichia/Shigella and Veillonella were positively associated with AST. There were significant differences in these phenomena (p < 0.05). In the control group (Supplementary Figure 3), Bacteroidetes were positively associated with TBIL; Pseudomonas were positively associated with DBIL; Acidobacteria were negatively associated with AST; and Rothia was negatively associated with ALT.
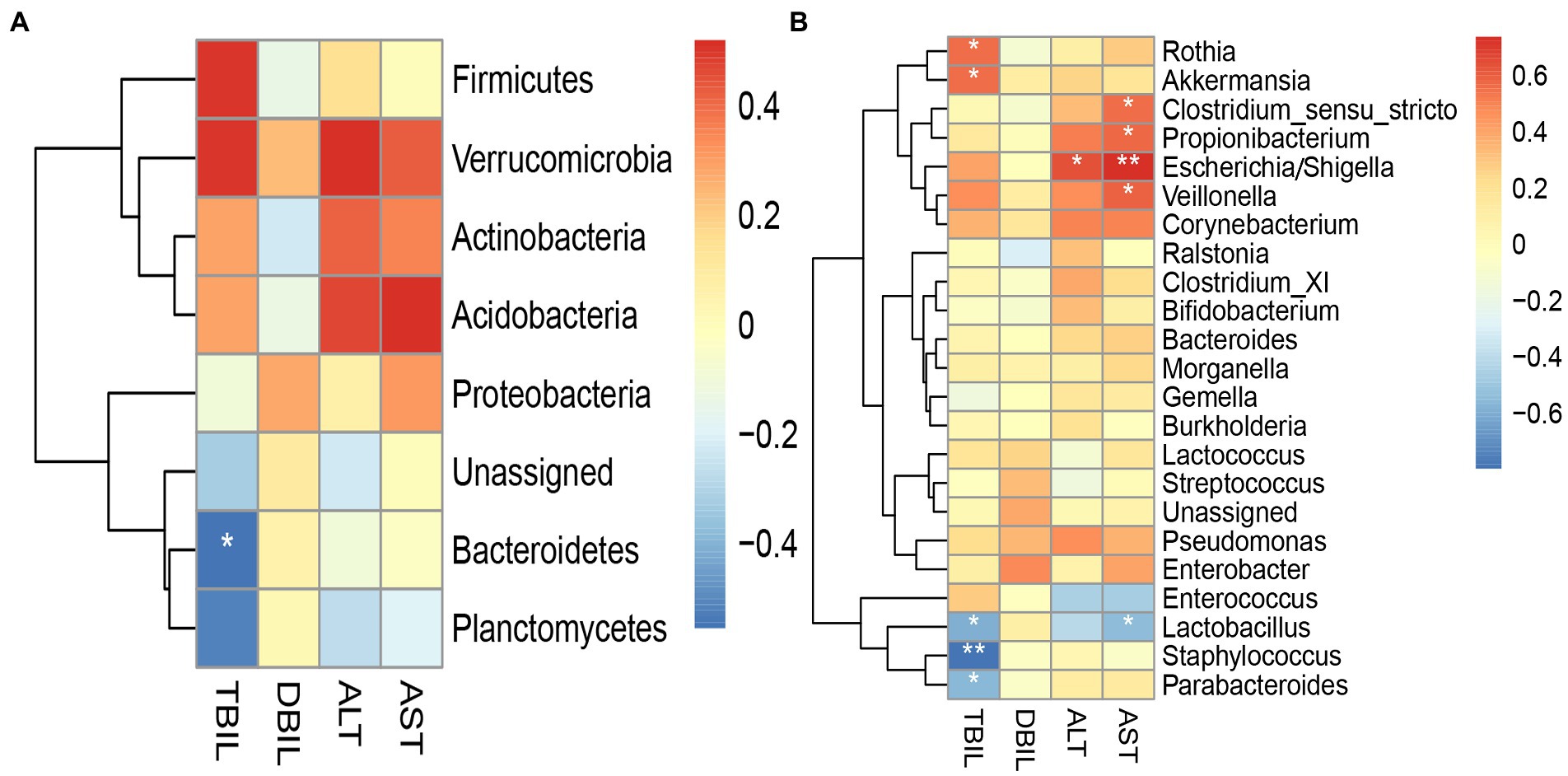
Figure 8. Heatmap for Spearman’s rank-sum correlation coefficient between gut microbiota and clinical indices in the case group. The red color on the heatmap indicates a positive correlation, while blue indicates a negative correlation. The darker the color, the larger the correlation coefficient. *value of p ≤ 0.05, **value of p ≤ 0.01. (A) Correlation between gut microbiota at the phylum level and clinical indices. (B) Correlation between gut microbiota at the genus level and clinical indices.
4. Discussion
In our study, we compared the difference in gut microbiota between 14 neonates with physiologic jaundice and 14 neonates with pathologic jaundice, and then explored the correlation between gut microbiota and clinical indices. Alpha diversity analysis and Beta diversity analysis showed that there were no differences in the total richness and diversity of gut microbiota between the two groups. At the phylum and genus levels, we found that Bacteroidetes, Planctomycetes, and Braydrhizobium were significantly abundant in the case group, while Actinobacteria, Rothia, and Bidfldobacterium were significantly abundant in the control group. Then we found that Bacteroidetes were most valuable in differentiating pathologic jaundice from physiologic jaundice by the ROC curve. Principal component analysis based on the phylum and genus levels found that the differences in principal components at the phylum level were mainly contributed by Firmicutes and Proteobacteria. According to the heatmap for Spearman’s rank-sum correlation coefficient of the case group, Escherichia/Shigella and Veillonella were positively associated with AST. Bacteroidetes and Staphylococcus were negatively associated with bilirubin levels. And in the control group, Bacteroidetes were positively associated with bilirubin levels.
Our results revealed some agreement with the majority of the data that are currently published. In our study, Alpha diversity analysis showed that there were no differences in the total richness and diversity of gut microbiota between the two groups. Akagawa et al. found that there were no significant differences in the total richness and diversity of gut microbiota between 26 neonates with jaundice and 17 neonates without jaundice through 16S rRNA gene sequencing (Akagawa et al., 2021). Beta diversity analysis showed that there was a greater similarity in gut microbiota between the two groups. Dong et al. using 16S rRNA gene sequencing found there were no differences in Beta diversity of gut microbiota between 141 children with jaundice and 160 children without jaundice (Dong et al., 2018). This may suggest that the diversity of gut microbiota is not associated with jaundice severity.
Lefse analysis found that Bacteroidetes, Planctomycetes, and Braydrhizobium were abundant in the case group, while Actinobacteria, Rothia, and Bidfldobacterium were abundant in the control group. Meanwhile, at the phylum and genus levels, we found that the Bacteroidetes, Planctomycetes, and Braydrhizobium were significantly higher and Actinobacteria, Rothia, and Bidfldobacterium were significantly lower in the case group. Zhou et al. found that Bacteroidetes significantly increased in neonates with cholestasis jaundice by shotgun metagenomic sequencing (Zhou et al., 2019), which was consistent with our study. Besides, there was a study finding that Bacteroides were higher in patients with cholestatic liver disease (Wu et al., 2020). Bacteroides have both positive and negative effects on the human body (Zafar and Saier, 2021). On the one hand, Bacteroides can ferment carbohydrates in the gut, producing fatty acids that provide an energy source for the host (Hooper et al., 2002). Moreover, Bacteroides thetaiotaomicron of Bacteroidetes can regulate the expression of some host genes, including those associated with nutrient absorption, mucosal barrier fortification, and production of angiogenic factors (Hooper et al., 2001). Among them, it plays an important role in maintaining the structure and function of the intestinal mucosal barrier (Martin-Gallausiaux et al., 2021). Bacteroides thetaiotaomicron is reported to induce the expression of the small proline-rich protein 2A (sprr2A), which is necessary to maintain the epithelial villus desmosomes (Lutgendorff et al., 2008). Additionally, B. thetaiotaomicron can produce an antibiotic Paneth cell protein (Ang4) that can kill certain pathogenic organisms (Wexler, 2007). One the other hand, Bacteroides fragilis of Bacteroidetes has the ultra-high ability to utilize nearby nutrients. It can use glycoproteins and glycolipids on the surface of host cells at the site of infection to compete for the utilization of human nutrients. Besides, B. fragilis has harmful effects on the human body by participating in tissue adhesion, preventing host immune response, and destroying tissue components (Wexler, 2007). Previous studies have shown that B. fragilis accounted for 50% of the pathogenic bacteria in neonatal bacteremia (Brook, 1990). Also, some studies have noted that the increased abundance of Bacteroides can cause chronic inflammatory effects and lead to the development of type 1 diabetes mellitus (T1DM), celiac disease and other diseases (Torun et al., 2021). In our study, Bacteroides were more abundant in the case group, which may cause intestinal inflammation most likely due to the increase of harmful bacteria B. fragilis. However, we could not identify the certain bacteria species due to technical limitation.
Braydrhizobium belongs to Proteobacteria, and Proteobacteria belongs to anaerobes or facultative anaerobes. The colonic epithelium is hypoxic, which indicates that there were changes in intestinal epithelium and mucosa in neonates with pathologic jaundice (Li et al., 2020). Studies have noted that there was severe impairment of intestinal mucosal barrier function in patients with obstructive jaundice (Parks et al., 1996). Akagawa et al. found that Bidfldobacterium significantly decreased in neonates with jaundice (Akagawa et al., 2021). Zhou et al. also found that Bidfldobacterium significantly decreased in neonates with cholestasis jaundice by shotgun metagenomic sequencing (Zhou et al., 2019). Bidfldobacterium belongs to Actinobacteria. In the process of galactooligosaccharides (GOS) utilization, Bifidobacteriales can convert GOS into galactose and UDP-glucose, and the latter can affect the glucuronic acid pathway in the liver. So, the reduction of Bifidobacteria can affect the production of conjugated bilirubin (Thongaram et al., 2017; Duan, 2018). Rothia also belongs to Actinobacteria. It can produce butyrate (Liang et al., 2021). Butyrate belongs to short-chain fatty acids (SCFAs) which can regulate inflammation and reduce the number of inflammatory macrophages and Th17 cells in the intestine (Jiang et al., 2016). It has been reported that SCFAs may lead to changes in the gut microbiota to some extent (Zheng et al., 2017). Duan et al. found that SCFAs significantly decreased in neonates with breast milk jaundice. Therefore, they hypothesized that the alteration of gut microbiota may affect the production of SCFAs, and then SCFAs affect bilirubin metabolism (Duan et al., 2021). Furthermore, the flagella of Rothia can repair the intestinal barrier, improve the intestinal ecosystem, and alleviate chronic inflammation (Seo et al., 2020). Therefore, it can be concluded that Bacteroidetes, Actinobacteria, Braydrhizobium, Rothia, and Bifidobacterium may affect bilirubin metabolism by causing intestinal inflammation, changing intestinal mucosal permeability, affecting glucuronic acid metabolism pathway, and antagonizing intestinal inflammation. By the ROC curve, we found that Bacteroidetes, Actinobacteria, Bifidobacterium, Rothia, Planctomycetes, and Bradyrhizobium had the significance of distinguishing pathologic jaundice from physiologic jaundice. Interestingly, Bacteroidetes were most valuable in differentiating pathologic jaundice from physiologic jaundice. Therefore, in the future clinical work, Bacteroidetes could be used as biomarkers to identify pathologic jaundice. At the same time, it could be used to determine whether the etiology of jaundice is endogenous or intestinal to a certain extent (Guo, 2017).
This study found that the differences in principal components at the phylum level were mainly contributed by Firmicutes and Proteobacteria. Studies have shown that gut microbiota in the neonatal period is mainly composed of Firmicutes and Proteobacteria (Jiménez et al., 2008). Firmicutes can produce butyrate (Fei et al., 2018), which plays an important role in intestinal conjunctivitis. It is the main energy source of the intestinal mucosa, and plays an important role in inhibiting colonic carcinogenesis, resisting inflammation, regulating oxidative stress, improving colonic defense barrier function, and promoting satiety (Hamer et al., 2008; Louis and Flint, 2009). By studying the changes in gut microbiota in rats with non-alcoholic fatty liver disease (NAFLD) model, Sun found that Proteobacteria significantly increased and were positively associated with serum aspartate aminotransferase (Sun, 2020). Previous studies have shown that Proteobacteria are related to the integrity of the intestinal mucosal barrier, and the increased abundance of Proteobacteria destroys the original intestinal mucosal barrier and increases intestinal permeability, thereby causing chronic intestinal inflammation and liver function damage (Jiang et al., 2015; Sun, 2020). In our study, Firmicutes decreased and Proteobacteria increased in the pathologic jaundice group, although there were no significant differences. The imbalance of Proteobacteria and Firmicutes may change intestinal permeability and cause chronic intestinal inflammation, then impair liver function and reduce liver processing capacity for bilirubin.
In the case group, the heatmap for Spearman’s rank-sum correlation coefficient showed that Escherichia/Shigella and Veillonella were positively associated with AST, while Bacteroidetes and Staphylococcus were negatively associated with bilirubin levels. Wu et al. found that Escherichia/Shigella and Veillonella increased in patients with cholestatic liver disease by 16S rRNA gene sequencing. Escherichia/Shigella and Veillonella are opportunistic pathogens, whose abundance will increase under the alteration of gut microbiota and eventually become the dominant bacterial genus in pathologic jaundice (Shen et al., 2017; Wu et al., 2020). The relatively high abundance of Veillonella might be used as a potential biomarker for the diagnosis of cholestatic liver disease (Wu et al., 2020). As we mentioned above, B. fragilis of Bacteroidetes may increase serum bilirubin levels by causing intestinal inflammation, and changing intestinal mucosal permeability. Some studies have shown that Staphylococcus can produce β-glucuronidase (β-GD) (Tang et al., 2021). In the intestine, β-GD can hydrolyze conjugated bilirubin into unconjugated bilirubin. Then, unconjugated bilirubin is absorbed by the intestinal mucosa, coming back to the liver, thereby promoting the serum bilirubin levels (Seyedi et al., 2019). And β-GD activity is positively associated with the abundance of Staphylococcus (Tang et al., 2021). However, this is contrary to the results of our experiment, which may be due to the fact that the bilirubin levels in the case group were determined by two factors. On the one hand, some neonates were recruited into the case group due to high bilirubin levels. One the other hand, the left neonates were recruited into the case group due to prolonged jaundice. So, we got a result that Bacteroidetes were positively associated with bilirubin levels in the control group. Because the diagnosis of physiologic jaundice is simply elevated bilirubin levels. It can be concluded that Escherichia/Shigella and Veillonella may become the dominant pathogens due to the imbalance of gut microbiota, causing changes in liver function. Bacteroidetes cause intestinal inflammation most likely due to the harmful bacteria B. fragilis, while Staphylococcus increases enterohepatic circulation by producing β-GD, which will cause an elevated serum bilirubin level.
There were some limitations in our study. Firstly, in this study, the control group recruited the subjects with physiologic jaundice, while the other studies mentioned above recruited the subjects with non-jaundice. There may be some differences in the gut microbiota between the physiologic jaundice group and the non-jaundice group. It is less supportive when discussing the pathogenicity with other studies. Secondly, although there were no significant differences in CRP between the two groups by baseline comparison. However, the individual subject has elevated CRP values, which may affect the development of gut microbiota. So, the confounding effects of infection have not been excluded. In the next step, healthy neonates will be selected as the research subjects. Additionally, although there was no difference in the rate of antibiotic use between the two groups in our study, antibiotic use can have an impact on gut microbiota. In the future studies about gut microbiota, we will study children who have not received antibiotics. Finally, we failed to follow up and continue to collect specimens for a longitudinal study to observe the changes and characteristics of gut microbiota.
5. Conclusion
Bacteroidetes, Planctomycetes, and Braydrhizobium were significantly abundant in the case group, while Actinobacteria, Rothia, and Bidfldobacterium were significantly abundant in the control group. Bacteroidetes were valuable in differentiating pathologic jaundice from physiologic jaundice by the ROC curve. Therefore, Bacteroidetes could be used as biomarkers to identify pathologic jaundice. Moreover, Bacteroidetes are positively associated with bilirubin levels. It affects bilirubin metabolism by causing intestinal inflammation most likely due to the harmful bacteria B. fragilis.
Data availability statement
The datasets presented in this study can be found in online repositories. The names of the repository/repositories and accession number (s) can be found at: https://www.ncbi.nlm.nih.gov/sra/PRJNA926124, PRJNA926124.
Ethics statement
The studies involving human participants were reviewed and approved by The Ethics Committee of Hunan Children’s Hospital (Changsha, China. No. HCHLL-2018-64). Written informed consent to participate in this study was provided by the participants’ legal guardian/next of kin.
Author contributions
JY and JQ contributed to the conception and design of the work and revised the manuscript for important intellectual content. RH, ZX, and JQ provided administrative support. GL, XP, YM, RH, and ZX provisioned the study materials or patients. JY, CZ, and YM collected and assembled the data. JY, SF, CZ, and JQ performed the statistical analysis and interpretation. JY drafted the manuscript. All authors contributed to the article and approved the submitted version.
Funding
This research was funded by the Science and Technology Department of Hunan Province (2020SK1014-3).
Acknowledgments
The authors thank all the participant children and their parents in the study and appreciate all of the support of the data collectors. All authors had access to the study data and reviewed and approved the final manuscript.
Conflict of interest
The authors declare that the research was conducted in the absence of any commercial or financial relationships that could be construed as a potential conflict of interest.
Publisher’s note
All claims expressed in this article are solely those of the authors and do not necessarily represent those of their affiliated organizations, or those of the publisher, the editors and the reviewers. Any product that may be evaluated in this article, or claim that may be made by its manufacturer, is not guaranteed or endorsed by the publisher.
Supplementary material
The Supplementary material for this article can be found online at: https://www.frontiersin.org/articles/10.3389/fmicb.2023.1122172/full#supplementary-material
References
Akagawa, S., Akagawa, Y., Yamanouchi, S., Teramoto, Y., Yasuda, M., Fujishiro, S., et al. (2021). Association of neonatal jaundice with gut dysbiosis characterized by decreased Bifidobacteriales. Meta 11:887. doi: 10.3390/metabo11120887
Billing, B. H. (1978). Twenty-five years of progress in bilirubin metabolism (1952–77). Gut 19, 481–491. doi: 10.1136/gut.19.6.481
Bolyen, E., Rideout, J. R., Dillon, M. R., Bokulich, N. A., Abnet, C. C., Al-Ghalith, G. A., et al. (2019). Reproducible, interactive, scalable and extensible microbiome data science using QIIME 2. Nat. Biotechnol. 37, 852–857. doi: 10.1038/s41587-019-0209-9
Boskabadi, H., Rakhshanizadeh, F., and Zakerihamidi, M. (2020). Evaluation of maternal risk factors in neonatal Hyperbilirubinemia. Arch. Iran. Med. 23, 128–140.
Brits, H., Adendorff, J., Huisamen, D., Beukes, D., Botha, K., Herbst, H., et al. (2018). The prevalence of neonatal jaundice and risk factors in healthy term neonates at national district hospital in Bloemfontein. Afr. J. Primary Health Care Family Med. 10, e1–e6. doi: 10.4102/phcfm.v10i1.1582
Dennery, P. A., Seidman, D. S., and Stevenson, D. K. (2001). Neonatal hyperbilirubinemia. N. Engl. J. Med. 344, 581–590. doi: 10.1056/NEJM200102223440807
Ding, J., Ma, X., Han, L., Zhao, X., Li, A., Xin, Q., et al. (2021). Gut microbial alterations in neonatal jaundice pre-and post-treatment. Biosci. Rep. 41. doi: 10.1042/BSR20210362
Dong, T., Chen, T., White, R. A. 3rd, Wang, X., Hu, W., Liang, Y., et al. (2018). Meconium microbiome associates with the development of neonatal jaundice. Clin. Transl. Gastroenterol. 9:182. doi: 10.1038/s41424-018-0048-x
Duan, M. (2018). Gut microbiota in infants with breast milk jaundice by high-throughput sequencing and metabonomics analysis. Doctor, Chongqing Medical University
Duan, M., Han, Z. H., Huang, T., Yang, Y., and Huang, B. (2021). Characterization of gut microbiota and short-chain fatty acid in breastfed infants with or without breast milk jaundice. Lett. Appl. Microbiol. 72, 60–67. doi: 10.1111/lam.13382
Fan, L. Y., and Xu, Y. H. (2016). The research progress of newborn bilirubin encephalopathy. J. Pediatr. Pharm. 22, 50–54. doi: 10.13407/j.cnki.jpp.1672-108X.2016.09.018
Fei, J., Luo, J., Zhang, X., Yu, X., and Ye, J. (2018). The role of short-chain fatty acids in the regulation of human energy metabolism by intestinal Flora. Chin. J. Diabetes Mellitus 5, 370–373. doi: 10.3760/cma.j.issn.1674-5809.2018.05.014
Guo, Z. (2017). Application of metagenomics in the studies of relationship between cut microbiota and human diseases. Doctor, China Agricultural University
Hamer, H. M., Jonkers, D., Venema, K., Vanhoutvin, S., Troost, F. J., and Brummer, R. J. (2008). Review article: the role of butyrate on colonic function. Aliment. Pharmacol. Ther. 27, 104–119. doi: 10.1111/j.1365-2036.2007.03562.x
Hooper, L. V., Midtvedt, T., and Gordon, J. I. (2002). How host-microbial interactions shape the nutrient environment of the mammalian intestine. Annu. Rev. Nutr. 22, 283–307. doi: 10.1146/annurev.nutr.22.011602.092259
Hooper, L. V., Wong, M. H., Thelin, A., Hansson, L., Falk, P. G., and Gordon, J. I. (2001). Molecular analysis of commensal host-microbial relationships in the intestine. Science 291, 881–884. doi: 10.1126/science.291.5505.881
Jiang, W., Wu, N., Wang, X., Chi, Y., Zhang, Y., Qiu, X., et al. (2015). Dysbiosis gut microbiota associated with inflammation and impaired mucosal immune function in intestine of humans with non-alcoholic fatty liver disease. Sci. Rep. 5:8096. doi: 10.1038/srep08096
Jiang, S., Xie, S., Lv, D., Zhang, Y., Deng, J., Zeng, L., et al. (2016). A reduction in the butyrate producing species Roseburia spp. and Faecalibacterium prausnitzii is associated with chronic kidney disease progression. Antonie Van Leeuwenhoek 109, 1389–1396. doi: 10.1007/s10482-016-0737-y
Jiménez, E., Marín, M. L., Martín, R., Odriozola, J. M., Olivares, M., Xaus, J., et al. (2008). Is meconium from healthy newborns actually sterile? Res. Microbiol. 159, 187–193. doi: 10.1016/j.resmic.2007.12.007
Kujabi, M. L., Petersen, J. P., Pedersen, M. V., Parner, E. T., and Henriksen, T. B. (2021). Neonatal jaundice and autism spectrum disorder: a systematic review and meta-analysis. Pediatr. Res. 90, 934–949. doi: 10.1038/s41390-020-01272-x
Kuniyoshi, Y., Tsujimoto, Y., Banno, M., Taito, S., and Ariie, T. (2021). Neonatal jaundice, phototherapy and childhood allergic diseases: an updated systematic review and meta-analysis. Pediatr. Allergy Immunol. 32, 690–701. doi: 10.1111/pai.13456
Li, Q., and Feng, Z. (2006). The latest guideline for neonatal jaundice produced by American academic of pediatrics. Chin. J. Appl. Clin. Pediatr. 21, 958–960. doi: 10.3969/j.issn.1003-515X.2006.14.043
Li, Y., Shen, N., Li, J., Hu, R., Mo, X., and Xu, L. (2020). Changes in intestinal Flora and Metabolites in neonates with breast Milk jaundice. Front. Pediatr. 8:177. doi: 10.3389/fped.2020.00177
Liang, S. K. E. C., Huang, H., Guan, J., Zhao, L., Li, B., et al. (2021). Butyrate-producing bacteria in the intestinal tract and the physiological function of their metabolite butyrate: a review. Microbiol. China 48, 948–959. doi: 10.13344/j.microbiol.china.200466
Louis, P., and Flint, H. J. (2009). Diversity, metabolism and microbial ecology of butyrate-producing bacteria from the human large intestine. FEMS Microbiol. Lett. 294, 1–8. doi: 10.1111/j.1574-6968.2009.01514.x
Lutgendorff, F., Akkermans, L. M., and Söderholm, J. D. (2008). The role of microbiota and probiotics in stress-induced gastro-intestinal damage. Curr. Mol. Med. 8, 282–298. doi: 10.2174/156652408784533779
Martin-Gallausiaux, C., Marinelli, L., Blottière, H. M., Larraufie, P., and Lapaque, N. (2021). SCFA: mechanisms and functional importance in the gut. Proc. Nutr. Soc. 80, 37–49. doi: 10.1017/s0029665120006916
Metsalu, T., and Vilo, J. (2015). ClustVis: a web tool for visualizing clustering of multivariate data using principal component analysis and heatmap. Nucleic Acids Res. 43, W566–W570. doi: 10.1093/nar/gkv468
Ng, M. C., and How, C. H. (2015). When babies turn yellow. Singap. Med. J. 56, 599–602; quiz 603. doi: 10.11622/smedj.2015167
Parks, R. W., Clements, W. D., Smye, M. G., Pope, C., Rowlands, B. J., and Diamond, T. (1996). Intestinal barrier dysfunction in clinical and experimental obstructive jaundice and its reversal by internal biliary drainage. Br. J. Surg. 83, 1345–1349. doi: 10.1002/bjs.1800831007
Qiu, J., Zhou, C., Xiang, S., Dong, J., Zhu, Q., Yin, J., et al. (2022). Association between trajectory patterns of body mass index change up to 10 months and early gut microbiota in preterm infants. Front. Microbiol. 13:828275. doi: 10.3389/fmicb.2022.828275
Rennie, J., Burman-Roy, S., and Murphy, M. S. (2010). Neonatal jaundice: summary of NICE guidance. BMJ 340:c2409. doi: 10.1136/bmj.c2409
Ringnér, M. (2008). What is principal component analysis? Nat. Biotechnol. 26, 303–304. doi: 10.1038/nbt0308-303
Segata, N., Izard, J., Waldron, L., Gevers, D., Miropolsky, L., Garrett, W. S., et al. (2011). Metagenomic biomarker discovery and explanation. Genome Biol. 12:R60. doi: 10.1186/gb-2011-12-6-r60
Seo, B., Jeon, K., Moon, S., Lee, K., Kim, W. K., Jeong, H., et al. (2020). Roseburia spp. abundance associates with alcohol consumption in humans and its administration ameliorates alcoholic fatty liver in mice. Cell Host Microbe 27, 25–40.e6. doi: 10.1016/j.chom.2019.11.001
Seyedi, R., Mirghafourvand, M., Dost, A. J., and al, e. (2019). Relationship between neonatal skin bilirubin level and severe jaundice with maternal, childbirth, and neonatal characteristics. Iran J. Neonatol. 10, 61–67. doi: 10.22038/ijn.2019.33282.1478
Shao, X., Ye, H., and Qiu, X. (2010). Practice of Neonatalogy. Beijing,China: Beijing: People's Medical Publishing House
Shen, F., Zheng, R.-D., Sun, X.-Q., Ding, W.-J., Wang, X.-Y., and Fan, J.-G. (2017). Gut microbiota dysbiosis in patients with non-alcoholic fatty liver disease. Hepatob. Pancreat. Dis. Int. 16, 375–381. doi: 10.1016/s1499-3872(17)60019-5
Sun, H. L. (2020). Study on the correlation between gut microbiota and IL-22 and LPS in NAFLD rats. Master, Yan’an University
Sun, Y., Zhou, B., and Miao, D. (2013). Research progress in pathogenesis of breast Milk jaundice. Med. Recapit. 19, 1963–1966. doi: 10.3969/j.issn.1006-2084.2013.11.014
Tang, W., Lu, H. Y., Sun, Q., and Xu, W. M. (2021). Characteristics of gut microbiota and its association with the activity of β-glucuronidase in neonates with hyperbilirubinemia. Chin. J. Contemp. Pediatr. 23, 677–683. doi: 10.7499/j.issn.1008-8830.2102039
Thongaram, T., Hoeflinger, J. L., Chow, J., and Miller, M. J. (2017). Prebiotic galactooligosaccharide metabolism by probiotic lactobacilli and Bifidobacteria. J. Agric. Food Chem. 65, 4184–4192. doi: 10.1021/acs.jafc.7b00851
Torun, A., Hupalowska, A., Trzonkowski, P., Kierkus, J., and Pyrzynska, B. (2021). Intestinal microbiota in common chronic inflammatory disorders affecting children. Front. Immunol. 12:642166. doi: 10.3389/fimmu.2021.642166
Vitek, L., Zelenka, J., Zadinova, M., and Malina, J. (2005). The impact of intestinal microflora on serum bilirubin levels. J. Hepatol. 42, 238–243. doi: 10.1016/j.jhep.2004.10.012
Wexler, H. M. (2007). Bacteroides: the good, the bad, and the nitty-gritty. Clin. Microbiol. Rev. 20, 593–621. doi: 10.1128/CMR.00008-07
Wu, L., Tang, L., Zhang, J., Lei, Y., Hu, S., Chen, Y., et al. (2020). Characteristics of gut microbiota and their correlation with clinical parameters in patients with cholestatic liver disease. J. Third Military Med. Univ. 44, 2251–2258. doi: 10.16016/j.1000-5404.202005245
Xiong, J., Liu, Y., Lin, X., Zhang, H., Zeng, J., Hou, J., et al. (2012). Geographic distance and pH drive bacterial distribution in alkaline lake sediments across Tibetan plateau. Environ. Microbiol. 14, 2457–2466. doi: 10.1111/j.1462-2920.2012.02799.x
Zafar, H., and Saier, M. H. Jr. (2021). Gut Bacteroides species in health and disease. Gut Microbes 13, 1–20. doi: 10.1080/19490976.2020.1848158
Zheng, X., Huang, F., Zhao, A., Lei, S., Zhang, Y., Xie, G., et al. (2017). Bile acid is a significant host factor shaping the gut microbiome of diet-induced obese mice. BMC Biol. 15:120. doi: 10.1186/s12915-017-0462-7
Zhou, C., Gong, S., Xiang, S., Liang, L., Hu, X., Huang, R., et al. (2022). Changes and significance of gut microbiota in children with focal epilepsy before and after treatment. Front. Cell. Infect. Microbiol. 12:965471. doi: 10.3389/fcimb.2022.965471
Keywords: Bacteroidetes , gut microbiota, pathologic jaundice, physiologic jaundice, neonate
Citation: You J, Qiu J, Li G, Peng X, Ma Y, Zhou C, Fang S, Huang R and Xiao Z (2023) The relationship between gut microbiota and neonatal pathologic jaundice: A pilot case-control study. Front. Microbiol. 14:1122172. doi: 10.3389/fmicb.2023.1122172
Edited by:
Marcelino Gutiérrez, Instituto de Investigaciones Científicas y Servicios de Alta Tecnología, PanamaReviewed by:
Shimeng Huang, Jiangsu Academy of Agricultural Sciences, ChinaShoji Tsuji, Kansai Medical University, Japan
Copyright © 2023 You, Qiu, Li, Peng, Ma, Zhou, Fang, Huang and Xiao. This is an open-access article distributed under the terms of the Creative Commons Attribution License (CC BY). The use, distribution or reproduction in other forums is permitted, provided the original author(s) and the copyright owner(s) are credited and that the original publication in this journal is cited, in accordance with accepted academic practice. No use, distribution or reproduction is permitted which does not comply with these terms.
*Correspondence: Rui Wen Huang, 846767295@qq.com; Zheng Hui Xiao, xiaozh888@126.com
†These authors have contributed equally to this work and share first authorship