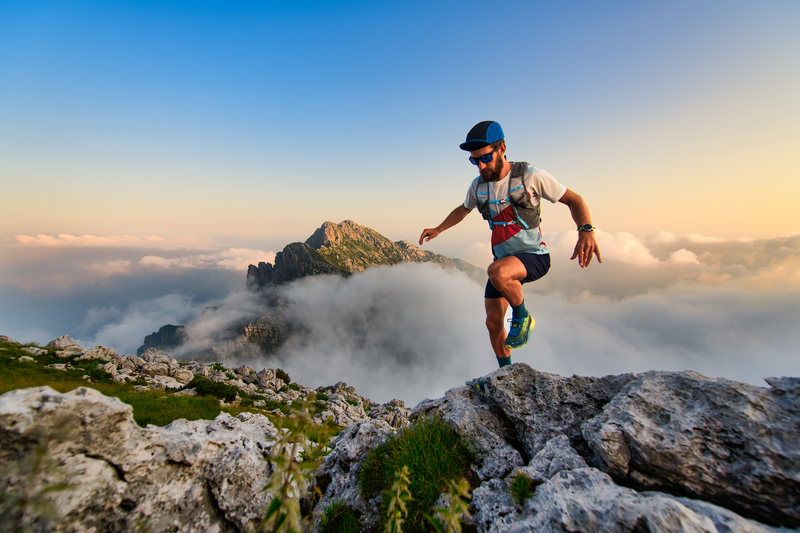
95% of researchers rate our articles as excellent or good
Learn more about the work of our research integrity team to safeguard the quality of each article we publish.
Find out more
ORIGINAL RESEARCH article
Front. Microbiol. , 03 February 2023
Sec. Systems Microbiology
Volume 14 - 2023 | https://doi.org/10.3389/fmicb.2023.1116592
This article is part of the Research Topic Computational and Systems Biology Methods for Elucidating Associations Between Cancer and Microbes View all 19 articles
Breast, ovarian, prostate, lung, and head/neck cancers are five solid cancers with complex interrelationships. However, the shared genetic factors of the five cancers were often revealed either by the combination of individual genome-wide association study (GWAS) approach or by the fixed-effect model-based meta-analysis approach with practically impossible assumptions. Here, we presented a random-effect model-based cross-cancer meta-analysis framework for identifying the genetic variants jointly influencing the five solid cancers. A comprehensive genetic correlation analysis (genome-wide, partitioned, and local) approach was performed by using GWAS summary statistics of the five cancers, and we observed three cancer pairs with significant genetic correlation: breast–ovarian cancer (rg = 0.221, p = 0.0003), breast–lung cancer (rg = 0.234, p = 7.6 × 10−6), and lung–head/neck cancer (rg = 0.652, p = 0.010). Furthermore, a random-effect model-based cross-trait meta-analysis was conducted for each significant cancer pair, and we found 27 shared genetic loci between breast and ovarian cancers, 18 loci between breast and lung cancers, and three loci between lung and head/neck cancers. Functional analysis indicates that the shared genes are enriched in human T-cell leukemia virus 1 infection (HTLV-1) and antigen processing and presentation (APP) pathways. Our study investigates the shared genetic links across five solid cancers and will help to reveal their potential molecular mechanisms.
Cancer has become one of the most fatal diseases and it poses a serious threat to human life and health. There have been ~18.1 million new cancer cases and 9.6 million cancer deaths each year (Bray et al., 2018). According to the prediction of the National Cancer Institute, the number of new cancer cases per year is expected to rise to 29.5 million, and the amount of cancer-related deaths will go up to 16.4 million by 2040. The high incidence of cancer has not only brought an enormous health burden to individuals but also caused heavy economic losses to countless families. Numerous pieces of evidence indicated widespread genetic pleiotropy and shared genetic basis among different cancers (Rashkin et al., 2020). As a few representative elements of solid cancer, breast, ovarian, prostate, lung, and head/neck cancers showed substantial heritability (ranging from 9 to 57%) in previous twin and family studies (Polderman et al., 2015; Mucci et al., 2016; Yu et al., 2017). Moreover, Jiang et al. (2019) quantified the pairwise genetic correlations of six solid cancers and found significant correlations between breast and ovarian cancers, breast and lung cancers, breast and colorectal cancers, and lung and head/neck cancers. The aforementioned conclusions demonstrate indirectly that these solid cancers may share inherited genetic mechanisms, which play important roles in cancer etiology. We would like to understand the shared genetic loci influencing the five solid cancers.
Genome-wide association studies (GWASs) have identified a number of susceptibility loci associated with each of the five solid cancers, ranging from dozens to hundreds (Buniello et al., 2019), but few of them overlap in at least two of these cancers. This indicates that rare pleiotropic loci are detected by cancer-specific GWAS. Identifying the shared genetic loci between diseases can help to reveal the underlying mechanisms driving disease etiology (Guo et al., 2020). There are mainly two strategies available to identify the shared loci in the previous literature. One strategy is based on the combination of GWASs and other scan analyses. For example, Ghoussaini et al. found pleiotropic loci located at 8q24, associated with breast, prostate, and other specific cancers by using this approach (Ghoussaini et al., 2008). Another strategy is based on a cross-cancer meta-analysis. For example, Kar et al. identified seven new loci shared by at least two of the three hormone-related cancers (breast, ovarian, and prostate); Fehringer et al. (2016) detected a novel pleiotropic locus 1q22 associated with both breast and lung cancers by performing a cross-cancer genome-wide analysis of breast, ovary, prostate, lung, and colorectal cancers. However, the pleiotropic loci identified by the above studies are still not sufficient, and this may due to the fact that the cross-cancer meta-analyses in the existing studies are based on the fix-effect model. The fix-effect model meta-analysis causes the loss of statistical power because it assumes the same real effect for each genetic variant in different studies, which is practically impossible and will inevitably yield inaccurate conclusions.
Random-effect model-based cross-trait meta-analysis methods can effectively account for the heterogeneous effect of each genetic variant by adding an additional variance term, addressing the shortcomings of fix-effect model-based meta-analysis. Here, we use the summary statistics of five solid cancers (breast, ovarian, prostate, lung, and head/neck) from the largest-to-date cancer-specific GWAS consortia, which include a total of 241,479 cases and 226,810 controls. We then estimate the genetic correlation between different cancer pairs. Furthermore, we conducted a cross-cancer meta-analysis to detect shared genetic loci between the cancer pairs using the current state-of-the-art random-effect model-based approach PLEIO (Pleiotropic Locus Exploration and Interpretation using Optimal test) (Lee et al., 2021), which enables us to properly account for the correlation of traits and the heterogeneity of variants. Finally, we perform functional analyses of pleiotropic variants to uncover the underlying biological mechanisms shared across the five solid cancers.
We used the most recent GWAS summary-level data from the Breast Cancer Association Consortium (BCAC) for breast cancer (122,977 cases and 105,974 controls) (Michailidou et al., 2017), the Ovarian Cancer Association Consortium (OCAC) for ovarian cancer (25,509 cases and 40,941 controls) (Phelan et al., 2017), the Prostate Cancer Association Group to Investigate Cancer Associated Alterations in the Genome (PRACTICAL) consortium for prostate cancer (79,148 cases and 61,106 controls) (Schumacher et al., 2018), the International Lung Cancer Consortium (ILCCO) for lung cancer (11,348 cases and 15,861 controls) (Wang et al., 2014), and the Oncoarray oral cavity and oropharyngeal cancer consortium for head/neck cancer (2,497 cases and 2,928 controls) (Lesseur et al., 2016).
To measure genome-wide genetic correlations for each cancer pair, we used the linkage disequilibrium (LD) score regression (LDSC) method (Schizophrenia Working Group of the Psychiatric Genomics Consortium et al., 2015). We applied pre-computed LD scores derived from ~1.2 million imputed variants from European populations that did not include the HLA region in the HapMap3 reference panel. LDSC controls for population structure using GWAS summary statistics without individual-level data.
We evaluated the partitioned genetic correlation across the five solid cancers within functional categories by using partitioned LDSC (ReproGen Consortium et al., 2015). We chose 11 functional categories as previously recommended (Zhu et al., 2019), including the DNase I digital genomic footprinting (DGF) region, DNase I hypersensitivity sites (DHSs), fetal DHS, intron, super-enhancer, transcription factor-binding sites (TFBS), transcribed region, and the histone markers H3K9ac, H3K4me1, H3K4me3, and H3K27ac from the Roadmap Epigenomics Project (Bernstein et al., 2010). Re-computed LD scores for variants classified in each particular annotation were used for estimating the cross-cancer genetic correlation within that functional group.
We estimated local genetic correlations between each pair of cancers in 1,703 pre-specified LD-independent regions using ρ-HESS (Shi et al., 2017). The goal of this method was to detect small contiguous regions of the genome in which the genetic associations of two traits are locally concordant, and to measure the local genetic correlation and p-values (pρ−HESS) between pairs of traits at local regions. Cancer pairs were considered to have genetic correlation at the local region if pρ−HESS passed the multiple testing correction (pρ−HESS < 0.05/1703).
For the cancer pairs with significant genome-wide genetic correlation, we conducted a pairwise cross-cancer meta-analysis by using PLEIO (Lee et al., 2021). The approach is based on a random-effect model, which can not only model genetic correlations across pairs of traits but can also correct for environmental correlations. It can seamlessly test multiple traits with various types by standardizing the effect sizes. Moreover, it maps pleiotropic loci through a variance component test and calculates statistical significance through an important sampling method. It overcomes the drawback of fixed-effect model methods such as ASSET (association analysis based on subsets) (Bhattacharjee et al., 2012). We conducted the cross-cancer meta-analysis on an Intel Xeon E5-2695 computer with the CPU operating at 2.10 GHz. This wastes ~10 min for each pair of cancers.
To separate the independent loci from the significant loci (p < 5 × 10−8), we used the clumping function in PLINK software (Purcell et al., 2007). SNPs with p < 1 × 10−5, an LD statistic r2>0.05, and a distance from the peak < 1,000 kb were assigned to the clump of that peak. Moreover, we set the NCBI human genome build 37 as the reference gene list.
We performed TWAS to identify gene–tissue pairs for each of the five solid cancers and used FUSION software based on the pre-computed 48 GTEx (version 7) tissue expression reference weights (Gusev et al., 2016). LD-reference data were derived from European descendants from the 1,000 Genomes Project. For each cancer, we conducted 48 TWASs, one tissue-cancer pair at a time. The false discovery rate (FDR) Benjamin–Hochberg procedure correction was used, and a result with an FDR < 0.05 was considered to be significant.
To validate our findings, we further conducted genome-wide genetic correlation analysis and cross-cancer meta-analysis of the five solid cancer GWAS datasets with the UK Biobank cohort from the IEU GWAS database project (Matthew et al., 2021): breast cancer (ID: ieu-b-4810), ovarian cancer (ID: ieu-b-4963), prostate cancer (ID: ieu-b-4809), lung cancer (ID: ieu-b-4954), and head/neck cancer (ID: ieu-b-4912). We applied the 1,000 Genomes Project variants (Phase 3) as the reference panel. The cross-cancer meta-analysis between each pair of replication datasets was implemented using the R software RE2C (Lee et al., 2017), which is another classical random-effect model-based method that tests heterogeneous effect size between individual summary statistics.
To gain biology insights from the shared risk genes, we performed Kyoto Encyclopedia of Genes and Genomes (KEGG) pathway analysis using the Enrichr web server (Kuleshov et al., 2016), which is a comprehensive resource for curated gene sets and a search engine that accumulates biological knowledge for further biological discoveries. The significant criterion is that the adjusted p-value is < 0.05.
We used STRING v10 (Szklarczyk et al., 2015) to analyze the PPI network. The basic assumption is that if two proteins are functionally associated, they may contribute to a common biological purpose. The interaction scores were derived from different sources, including experimentally determined interaction, database annotated information, and automated text mining knowledge.
A schematic overview of the present study is shown in Figure 1, that is, we estimated genome-wide, partitioned, and local genetic correlations of the five solid cancers. For the cancer pairs with significant genome-wide genetic correlation, we performed a cross-cancer meta-analysis to identify shared genetic loci. Finally, we conducted TWAS, pathway enrichment analysis, and PPI network analysis of the shared risk genes.
Among pairs of solid cancers, we found three pairs with positive genetic correlations at a significant threshold of p = 0.05: breast and ovarian cancers (rg = 0.221, p = 0.0003), breast and lung cancers (rg = 0.234, p = 7.6 × 10−6), and lung and head/neck cancers (rg = 0.652, p = 0.010). The remaining pairs do not show significant genetic correlations (Table 1).
In the partitioned genetic correlation analysis, we observed significant genetic correlation in all 11 functional categories for the breast–lung cancer pair, with only two exceptions: Intron and SuperEnhance for the lung–head/neck cancer pair. As to the breast–ovarian cancer pair, there is no significant signal in H3K27ac, H3K4me3, H3K9ac, and SuperEnhance. The partitioned genetic correlations range from 0.033 to 0.546 (Figure 2; Supplementary Table S1).
Figure 2. Partitioned genetic correlation between breast and ovarian cancers, breast and lung cancers, and lung and head/neck cancers. The vertical axis represents the genetic correlation rg, and the horizontal axis represents 11 functional categories. The asterisk represents significance (p < 0.05).
We conducted ρ-HESS to investigate whether specific regions had a genetic correlation between each pair of the five solid cancers. The results show that the breast–ovarian cancer pair has a strong local genetic correlation in the 2q33 region (chromosome 2: 201576284-202818637, p = 8.83 × 10−6) (Figure 3A). In addition, three regions, including the 9p21 region (chromosome 9: 20463534-22206559, p = 6.71 × 10−6), 10q26 region (chromosome 10: 123231465-123900545, p = 4.26 × 10−7), and 11q13 region (chromosome 11: 68005825-69516130, p = 4.90 × 10−6), are found to have strong local genetic correlations in the breast–prostate cancer pair (Figure 3B). We did not observe any significant local genetic correlations for the other cancer pairs.
Figure 3. Local genetic correlation and local SNP heritability between cancer pair. (A) Breast and ovarian cancers; (B) Breast and prostate cancers. For each subfigure, the top part represents local genetic correlation, the middle part represents local genetic covariance, blue or red highlights indicate significant local genetic correlation and covariance after multiple testing corrections, and the bottom part represents local SNP heritability for each trait.
In the cross-cancer meta-analysis, we identified 27 independent loci with a significant association between breast and ovarian cancers ( and single-trait p < 0.05, Table 2). The strongest pleiotropic signal is mapped to FGFR2 in the region 10q26.13 (rs1219648, ), a gene that has been altered in a number of patients with malignant solid tumors according to the AACR Project GENIE (The AACR Project GENIE Consortium et al., 2017). This SNP showed a pleiotropic association between breast and ovarian cancers according to a previous cross-cancer analysis (Kar et al., 2016). The second strongest signal is observed for chromosome 9q31.2 (rs630965, ). Patients with deletions on 9q31.2 may have delayed puberty (Iivonen et al., 2021). The third strongest signal observed on BNC2 (rs3814113, ) is a putative tumor suppressor gene in high-grade serous ovarian carcinoma, which impacted cell survival after oxidative stress (Cesaratto et al., 2016). Notably, four loci (rs7098100, rs4277389, rs4808616, and rs10069690) are not only significant after the meta-analysis but also reach a significant level in their original single-trait GWAS.
Table 2. Cross-trait meta-analysis result between breast and ovarian cancers (; single-trait p < 0.05).
For the breast–lung cancer pair, we detected 18 pleiotropic loci in the cross-cancer meta-analysis (Table 3). The most significant pleiotropic association is in the region 5q11.2 (rs16886181, ), and the mapped gene MAP3K1 regulates apoptosis, survival, migration, differentiation, and other functions, which suggests that it may be a target for cancer treatment (Pham et al., 2013). Moreover, we also found dense signals in the HIST1H gene family.
Table 3. Cross-trait meta-analysis result between breast and lung cancers (; single-trait p < 0.05).
A total of three loci were identified after conducting a meta-analysis of lung and head/neck cancers (Table 4). The first (rs380286, ) is mapped on CLPTM1L and MIR4457, genes encoding the catalytic subunit of human telomerase reverse transcriptase (McKay et al., 2017). The second (rs3117575, ) is in close proximity to ABHD16A and many other genes. ABHD16A is an emerging enzyme, mainly involved in lipid metabolism and intracellular signaling, leading to the metastasis of cancer (Xu et al., 2018). The third (rs2736100, ) is mapped on TERT, a gene that plays a central role in modulating telomerase activity in tumors (Colebatch et al., 2019).
Table 4. Cross-trait meta-analysis result between the lung and head/neck cancers (; single-trait p < 0.05).
To assess the association of gene expression in specific tissue between each pair of the five solid cancers, we performed TWAS. A total of 1,669 gene–tissue pairs are significantly associated with breast cancer after Benjamini–Hochberg correction (Supplementary Table S2), in addition to 418 gene–tissue pairs with ovarian cancer (Supplementary Table S3), 1,116 gene–tissue pairs with prostate cancer (Supplementary Table S4), 155 gene–tissue pairs with lung cancer (Supplementary Table S5), and 15 gene–tissue pairs with head/neck (Supplementary Table S6). Among them, 306 gene–tissue pairs are overlapped for the breast–ovarian cancer pair, and the tissues involved are scattered; however, a number of genes are almost concentrated in the clumping region of rs4277389 on chromosome 17, such as CRHR1, LRRC37A, and MAPT (Supplementary Table S7). Moreover, 23 gene–tissue pairs are overlapped for the breast–lung cancer pair, and most of the gene signals are observed in the 1q22 region, especially gene GBAP1, which is simultaneously significant in eight tissues (adipose, artery, breast, fibroblast cell, sigmoid colon, transverse colon, esophagus, and vagina) (Supplementary Table S7). In addition, one gene–tissue pair (CFB-pituitary) is overlapped for the lung–head/neck cancer pair (Supplementary Table S7).
In the replication analysis, we confirmed the significance of the genetic correlation between the breast and ovarian cancer pair (rg = 0.175, p = 0.0061), the breast and lung cancer pair (rg = 0.125, p = 0.0018), and the lung and head/neck cancer pair (rg = 0.506, p = 0.0005) in the UK Biobank. Then, we used cross-cancer meta-analysis (RE2C) to identify the shared genes between each of the three cancer pairs. For the breast–ovarian cancer pair, nine loci showed genome-wide significance. Of these, genes FGFR2, BNC2, ADAM29, ESR1, ATAD5, and TEFM were replicated when compared with their specific consortium results (Supplementary Table S8). Moreover, six loci demonstrated significance in the breast–lung cancer pair. Some genes were found to be replicated, such as MAP3K1 (rs12653202, ), HIST1H family (rs13214023, ), ASH1L (rs4971059, ), and ZMIZ1 (rs7904249, ) (Supplementary Table S9). In addition, we identified two loci shared in the lung–head/neck cancer pair, but neither was replicated (Supplementary Table S10).
We observed shared genes enriched in human T-cell leukemia virus 1 infection (HTLV-1) and antigen processing and presentation (APP) pathways. HTLV-1 was the first retrovirus discovered to cause adult T-cell leukemia (ATL), a highly aggressive blood cancer (Matsuoka and Jeang, 2011). The APP pathway is a key element for an efficient response to immune checkpoint inhibitor therapy, which can be exploited to enhance tumor immunogenicity and to increase the efficacy of immunotherapy. The use of immune checkpoint inhibitors has already shown significant clinical advances in a wide range of patients with cancer (D'Amico et al., 2022).
In total, we found 849 pairs of interaction in the PPI network (Supplementary Table S11). A total of 44 gene pairs have combined scores >0.95, in which the ESR1-NRIP1 pair has the highest score of 0.999. HIST1H family genes around the 6p22.1 region show strong interactions with high scores. We observed 26 genes with degrees >20, most of which are HIST1H family genes, in addition to ESR, HSPA4, TNF, and EHMT2 genes. HIST1H gene set expression was reported to be positively correlated with large tumor size, high grade, metastasis, and poor survival in patients with breast cancer (Liao et al., 2021), which were used as prognostic factors for survival prediction among patients with cervical cancer (Li et al., 2017). The PPI network for shared risk genes is shown in Figure 4.
In the present study, we conducted a comprehensive analysis measuring the genetic correlation of five solid cancers, leveraging summary statistics from the current largest GWAS cancer consortia. We found significant positive genome-wide genetic correlations in three cancer pairs: breast–ovarian cancer, breast–lung cancer, and lung–head/neck cancer. Although the correlation in the prostate–head/neck cancer pair was up to 0.139, it failed to reach a significant level.
In partitioned genetic correlation, we detected positive genetic correlation and statistical significance in most function regions of the genome for the three cancer pairs, which showed significance in LDSC. Among them, the transcribed region had the strongest magnitude and significance. Most of the susceptibility variants detected by GWAS are located in non-coding regions and affect most cancers by affecting gene expression (Sud et al., 2017). Histone markers, including H3K27ac, H3K4me1, H3K4me1, and H3K9ac, are important modifications that are associated with the dysregulation of many genes that play important roles in cancer development and progression (Kurdistani, 2007). Transcribed regions have diverse transcripts that impact cancer initiation and progression through several mechanisms of action (Gibert et al., 2022).
In the analysis of local genetic correlation, we identified a novel pleiotropic region (11q13) that showed a significant local genetic correlation between breast and prostate cancers. Although the 2q33 region was previously reported as a shared region for breast–ovarian and breast–prostate cancers (Jiang et al., 2019), we only observed the pleiotropic signal in the breast–ovarian cancer pair. In addition, the 9p21 and 10q26 regions we identified were indicated to share breast and prostate cancers (Jiang et al., 2019). However, we did not find any significant local correlation between the breast–lung cancer pair and the lung–head/neck cancer pair, which showed genome-wide statistical significance.
There are some common findings in the aforementioned three kinds of genetic correlation analyses. The three cancer pairs (breast–ovarian, breast–lung, and lung–head/neck), which were significant in genome-wide genetic association analysis, also showed strong significance in most functional categories in the partitioned genetic correlation analysis (Figure 2). In addition, the breast–ovarian cancer pair also showed strong significance in the 2q33 region in the local genetic correlation analysis (Figure 3A).
In the cross-cancer meta-analysis, we discovered 27 shared loci between breast and ovarian cancers, 18 shared loci between breast and lung cancers, and three shared loci between lung and head/neck cancers. Except for four of the shared loci that showed a significant association in trait-specific GWAS of two cancers, the others were newly discovered. In contrast, a previous study, which used the fixed-effect model-based approach ASSET, only identified one novel pleiotropic association at 1q22 involved in breast and lung cancers (Kar et al., 2016). This comparison demonstrated the high statistical power of the cross-cancer meta-analysis via the PLEIO test, which is based on a random-effect model.
In the TWAS analysis, we explored the significant gene–tissue pair in the five solid cancers by integrating GWAS summary statistics and GTEx tissue expression data. We identified 1,669 gene–tissue pairs associated with breast cancer at the transcriptome-wide level, in addition to 418 with ovarian cancer, 1,116 with prostate cancer, 155 with lung cancer, and 15 with head/neck cancer. Furthermore, we noticed that 306 gene–tissue pairs overlapped in the breast–ovarian cancer pair, 23 pairs overlapped in the breast–lung cancer pair, and one pair overlapped in the lung–head/neck cancer pair. These overlaps may implicate specific common regulations for biological function.
In the replication analysis, we found some shared genes in two independent cohorts, such as FGFR2 for the breast–ovarian cancer pair and MAP3K1 for the breast–lung cancer pair. Since there are more cases (tens of thousands) in specialized cohorts (such as BCAC for breast cancer) than those in the UK Biobank cohort (nearly 1,000), the small number of cases could affect the genetic correlation estimation; this may be the reason only a fraction of pleiotropic genes were found in UK Biobank replications.
The post-GWAS analyses enabled us to provide biological insights into the shared genes. We found that the shared genes were enriched in HTLV-1 and APP pathways via pathway enrichment analysis. In the PPI network analysis, we observed obvious aggregations around HIST1H family genes, which were proved to be used as prognostic factors for survival prediction among patients with cancer (Li et al., 2017).
There are some advantages of the present study. On the one hand, we conducted a cross-cancer meta-analysis using two large-scale cohorts for each cancer separately, which facilitated the detection of novel associations. On the other hand, we performed association analyses under two kinds of mainstream random-effect model-based methods, which confirmed some of the discoveries. We also point out the limitations of this study. First, the UK Biobank cohort cancers we used in our replication analysis are not independent because there may be some shared cases and substantial shared controls among these five solid cancers. Moreover, the identified pleiotropic loci can be divided into causal and non-causal, and further experiments are required to distinguish the causal loci and to study their biological function. Finally, our study focuses on identifying shared genetic factors across five solid cancers, and their shared environmental factors require further investigation.
Identifying the shared genetic loci across five solid cancers plays an important role in the etiology and pathogenesis of each cancer. Our study finds several significant genetic correlations in specific cancer pairs, and their corresponding pleiotropic variants are detected by a cross-cancer meta-analysis. We observe shared genes enriched in the human T-cell leukemia virus 1 infection (HTLV-1) and antigen processing and presentation (APP) pathways. These shared genes and pathways may help to provide clues for future drug development.
The original contributions presented in the study are included in the article/Supplementary material, further inquiries can be directed to the corresponding author.
HG: conceptualization, methodology, and software. HG, WC, TL, and YZ: writing the original draft preparation. HG, WC, YZ, and BH: writing, reviewing, and editing. All authors have read and agreed to the published version of the manuscript. All authors contributed to the article and approved the submitted version.
This research was funded by the Natural Science Foundation of Hubei Province (Grant No. 2022CFB942) and the Talent Introduction Project of Hubei Normal University in 2021 (Grant No. HS2021RC013).
The authors declare that the research was conducted in the absence of any commercial or financial relationships that could be construed as a potential conflict of interest.
All claims expressed in this article are solely those of the authors and do not necessarily represent those of their affiliated organizations, or those of the publisher, the editors and the reviewers. Any product that may be evaluated in this article, or claim that may be made by its manufacturer, is not guaranteed or endorsed by the publisher.
The Supplementary Material for this article can be found online at: https://www.frontiersin.org/articles/10.3389/fmicb.2023.1116592/full#supplementary-material
Bernstein, B. E., Stamatoyannopoulos, J. A., Costello, J. F., Ren, B., Milosavljevic, A., Meissner, A., et al. (2010). The NIH roadmap epigenomics mapping consortium. Nat. Biotechnol. 28, 1045–1048. doi: 10.1038/nbt1010-1045
Bhattacharjee, S., Rajaraman, P., Jacobs, K., Wheeler, W., Melin, B., Hartge, P., et al. (2012). A subset based approach improves power and interpretation for the combined analysis of genetic association studies of heterogeneous traits. Am. J. Hum. Genet. 90, 821–835. doi: 10.1016/j.ajhg.2012.03.015
Bray, F., Ferlay, J., Soerjomataram, I., Siegel, R. L., Torre, L. A., and Jemal, A. (2018). Global cancer statistics 2018: GLOBOCAN estimates of incidence and mortality worldwide for 36 cancers in 185 countries. CA Cancer J. Clin. 68, 394–424. doi: 10.3322/caac.21492
Buniello, A., MacArthur, J. A., Cerezo, M., Harris, L. W., Hayhurst, J., Malangone, C., et al. (2019). The NHGRI-EBI GWAS Catalog of published genome-wide association studies, targeted arrays and summary statistics 2019. Nucleic Acids Res. 47, D1005–D1012. doi: 10.1093/nar/gky1120
Cesaratto, L., Grisard, E., Coan, M., Zandona, L., De Mattia, E., Poletto, E., et al. (2016). BNC2 is a putative tumor suppressor gene in high-grade serous ovarian carcinoma and impacts cell survival after oxidative stress. Cell Death Dis. 7, e2374–e2374. doi: 10.1038/cddis.2016.278
Colebatch, A. J., Dobrovic, A., and Cooper, W. A. (2019). TERT gene: its function and dysregulation in cancer. J. Clin. Pathol. 72, 281–284. doi: 10.1136/jclinpath-2018-205653
D'Amico, S., Tempora, P., Melaiu, O., Lucarini, V., Cifaldi, L., Locatelli, F., et al. (2022). Targeting the antigen processing and presentation pathway to overcome resistance to immune checkpoint therapy. Front. Immunol. 13, 948297. doi: 10.3389/fimmu.2022.948297
Fehringer, G., Kraft, P., Pharoah, P. D., Eeles, R. A., Chatterjee, N., Schumacher, F. R., et al. (2016). Cross-cancer genome-wide analysis of lung, ovary, breast, prostate, and colorectal cancer reveals novel pleiotropic associations. Cancer Res. 76, 5103–5114. doi: 10.1158/0008-5472.CAN-15-2980
Ghoussaini, M., Song, H., Koessler, T., Al Olama, A. A., Kote-Jarai, Z., Driver, K. E., et al. (2008). Multiple loci with different cancer specificities within the 8q24 gene desert. J. Natl. Cancer Inst. 100, 962–966. doi: 10.1093/jnci/djn190
Gibert, M. K., Sarkar, A., Chagari, B., Roig-Laboy, C., Saha, S., Bednarek, S., et al. (2022). Transcribed ultraconserved regions in cancer. Cells 11, 1684. doi: 10.3390/cells11101684
Guo, H., An, J., and Yu, Z. (2020). Identifying shared risk genes for asthma, hay fever, and eczema by multi-trait and multiomic association analyses. Front. Genet. 11, 270. doi: 10.3389/fgene.2020.00270
Gusev, A., Ko, A., Shi, H., Bhatia, G., Chung, W., Penninx, B. W. J. H., et al. (2016). Integrative approaches for large-scale transcriptome-wide association studies. Nat. Genet. 48, 245–252. doi: 10.1038/ng.3506
Iivonen, A.-P., Karkinen, J., Yellapragada, V., Sidoroff, V., Almusa, H., Vaaralahti, K., et al. (2021). Kallmann syndrome in a patient with Weiss–Kruszka syndrome and a de novo deletion in 9q31.2. Eur. J. Endocrinol. 185, 57–66. doi: 10.1530/EJE-20-1387
Jiang, X., Finucane, H. K., Schumacher, F. R., Schmit, S. L., Tyrer, J. P., Han, Y., et al. (2019). Shared heritability and functional enrichment across six solid cancers. Nat. Commun. 10, 431. doi: 10.1038/s41467-019-12095-8
Kar, S. P., Beesley, J., Amin Al Olama, A., Michailidou, K., Tyrer, J., Kote-Jarai, Z., et al. (2016). Genome-wide meta-analyses of breast, ovarian, and prostate cancer association studies identify multiple new susceptibility loci shared by at least two cancer types. Cancer Discov. 6, 1052–1067. doi: 10.1158/2159-8290.CD-15-1227
Kuleshov, M. V., Jones, M. R., Rouillard, A. D., Fernandez, N. F., Duan, Q., Wang, Z., et al. (2016). Enrichr: a comprehensive gene set enrichment analysis web server 2016 update. Nucleic Acids Res. 44, W90–W97. doi: 10.1093/nar/gkw377
Kurdistani, S. K. (2007). Histone modifications as markers of cancer prognosis: a cellular view. Br. J. Cancer 97, 1–5. doi: 10.1038/sj.bjc.6603844
Lee, C. H., Eskin, E., and Han, B. (2017). Increasing the power of meta-analysis of genome-wide association studies to detect heterogeneous effects. Bioinformatics 14, i379–i388. doi: 10.1093/bioinformatics/btx242
Lee, C. H., Shi, H., Pasaniuc, B., Eskin, E., and Han, B. (2021). PLEIO: a method to map and interpret pleiotropic loci with GWAS summary statistics. Am. J. Hum. Genet. 108, 36–48. doi: 10.1016/j.ajhg.2020.11.017
Lesseur, C., Diergaarde, B., Olshan, A. F., Wunsch-Filho, V., Ness, A. R., Liu, G., et al. (2016). Genome-wide association analyses identify new susceptibility loci for oral cavity and pharyngeal cancer. Nat. Genet. 48, 1544–1550. doi: 10.1038/ng.3685
Li, X., Tian, R., Gao, H., Yang, Y., Williams, B. R. G., Gantier, M. P., et al. (2017). Identification of a histone family gene signature for predicting the prognosis of cervical cancer patients. Sci. Rep. 7, 16495. doi: 10.1038/s41598-017-16472-5
Liao, R., Chen, X., Cao, Q., Wang, Y., Miao, Z., Lei, X., et al. (2021). HIST1H1B promotes basal-like breast cancer progression by modulating CSF2 expression. Front. Oncol. 11, 780094. doi: 10.3389/fonc.2021.780094
Matsuoka, M., and Jeang, K.-T. (2011). Human T-cell leukemia virus type 1 (HTLV-1) and leukemic transformation: viral infectivity, Tax, HBZ and therapy. Oncogene 30, 1379–1389. doi: 10.1038/onc.2010.537
Matthew, S. L., Shea, J. A., Ben, E., Tom, R. G., Gibran, H., and Edoardo, M. (2021). The variant call format provides efficient and robust storage of GWAS summary statistics. Genome Biol. 22, 32. doi: 10.1186/s13059-020-02248-0
McKay, J. D., Hung, R. J., Han, Y., Zong, X., Carreras-Torres, R., Christiani, D. C., et al. (2017). Large scale association analysis identifies new lung cancer susceptibility loci and heterogeneity in genetic susceptibility across histological subtypes. Nat. Genet. 49, 1126–1132. doi: 10.1038/ng.3892
Michailidou, K., Lindstrom, S., Dennis, J., Beesley, J., Hui, S., Kar, S., et al. (2017). Association analysis identifies 65 new breast cancer risk loci. Nature 551, 92–94. doi: 10.1038/nature24284
Mucci, L. A., Hjelmborg, J. B., Harris, J. R., Czene, K., Havelick, D. J., Scheike, T., et al. (2016). Familial risk and heritability of cancer among twins in nordic countries. J. Am. Med. Assoc. 315, 68. doi: 10.1001/jama.2015.17703
Pham, T. T., Angus, S. P., and Johnson, G. L. (2013). MAP3K1: genomic alterations in cancer and function in promoting cell survival or apoptosis. Genes Cancer 4, 419–426. doi: 10.1177/1947601913513950
Phelan, C. M., Kuchenbaecker, K. B., Tyrer, J. P., Kar, S. P., Lawrenson, K., Winham, S. J., et al. (2017). Identification of 12 new susceptibility loci for different histotypes of epithelial ovarian cancer. Nat. Genet. 49, 680–691. doi: 10.1038/ng.3826
Polderman, T. J. C., Benyamin, B., de Leeuw, C. A., Sullivan, P. F., van Bochoven, A., Visscher, P. M., et al. (2015). Meta-analysis of the heritability of human traits based on fifty years of twin studies. Nat. Genet. 47, 702–709. doi: 10.1038/ng.3285
Purcell, S., Neale, B., Todd-Brown, K., Thomas, L., Ferreira, M. A., Bender, D., et al. (2007). PLINK: a tool set for whole-genome association and population-based linkage analyses. Am. J. Hum. Genet. 81, 559–575. doi: 10.1086/519795
Rashkin, S. R., Graff, R. E., Kachuri, L., Thai, K. K., Alexeeff, S. E., Blatchins, M. A., et al. (2020). Pan-cancer study detects genetic risk variants and shared genetic basis in two large cohorts. Nat. Commun. 11, 4423. doi: 10.1038/s41467-020-18246-6
ReproGen Consortium Schizophrenia Working Group of the Psychiatric Genomics Consortium, The RACI Consortium, Finucane, H. K. Bulik-Sullivan B. Gusev A. . (2015). Partitioning heritability by functional annotation using genome-wide association summary statistics. Nat. Genet. 47, 1228–1235. doi: 10.1038/ng.3404
Schizophrenia Working Group of the Psychiatric Genomics Consortium Bulik-Sullivan, B. K. Loh P.-R. Finucane H. K. Ripke S. Yang J. . (2015). LD score regression distinguishes confounding from polygenicity in genome-wide association studies. Nat. Genet. 47, 291–295. doi: 10.1038/ng.3211
Schumacher, F. R., Al Olama, A. A., Berndt, S. I., Benlloch, S., Ahmed, M., Saunders, E. J., et al. (2018). Association analyses of more than 140,000 men identify 63 new prostate cancer susceptibility loci. Nat. Genet. 50, 928–936. doi: 10.1038/s41588-018-0142-8
Shi, H., Mancuso, N., Spendlove, S., and Pasaniuc, B. (2017). Local genetic correlation gives insights into the shared genetic architecture of complex traits. Am. J. Hum. Genet. 101, 737–751. doi: 10.1016/j.ajhg.2017.09.022
Sud, A., Kinnersley, B., and Houlston, R. S. (2017). Genome-wide association studies of cancer: current insights and future perspectives. Nat. Rev. Cancer 17, 692–704. doi: 10.1038/nrc.2017.82
Szklarczyk, D., Franceschini, A., Wyder, S., Forslund, K., Heller, D., Huerta-Cepas, J., et al. (2015). STRING v10: protein–protein interaction networks, integrated over the tree of life. Nucleic Acids Res. 43, D447–D452. doi: 10.1093/nar/gku1003
The AACR Project GENIE Consortium The AACR Project GENIE Consortium, Andre, F. Arnedos M. Baras A. S. Baselga J. . (2017). AACR Project GENIE: powering precision medicine through an international consortium. Cancer Discov. 7, 818–831. doi: 10.1158/2159-8290.CD-17-0151
Wang, Y., McKay, J. D., Rafnar, T., Wang, Z., Timofeeva, M. N., Broderick, P., et al. (2014). Rare variants of large effect in BRCA2 and CHEK2 affect risk of lung cancer. Nat. Genet. 46, 736–741. doi: 10.1038/ng.3002
Xu, J., Gu, W., Ji, K., Xu, Z., Zhu, H., and Zheng, W. (2018). Sequence analysis and structure prediction of ABHD16A and the roles of the ABHD family members in human disease. Open Biol. 8, 180017. doi: 10.1098/rsob.180017
Yu, H., Frank, C., Sundquist, J., Hemminki, A., and Hemminki, K. (2017). Common cancers share familial susceptibility: implications for cancer genetics and counselling. J. Med. Genet. 54, 248–253. doi: 10.1136/jmedgenet-2016-103932
Keywords: solid cancers, summary statistics, shared genetic loci, meta-analysis, random effect model
Citation: Guo H, Cao W, Zhu Y, Li T and Hu B (2023) A genome-wide cross-cancer meta-analysis highlights the shared genetic links of five solid cancers. Front. Microbiol. 14:1116592. doi: 10.3389/fmicb.2023.1116592
Received: 05 December 2022; Accepted: 06 January 2023;
Published: 03 February 2023.
Edited by:
Lihong Peng, Hunan University of Technology, ChinaCopyright © 2023 Guo, Cao, Zhu, Li and Hu. This is an open-access article distributed under the terms of the Creative Commons Attribution License (CC BY). The use, distribution or reproduction in other forums is permitted, provided the original author(s) and the copyright owner(s) are credited and that the original publication in this journal is cited, in accordance with accepted academic practice. No use, distribution or reproduction is permitted which does not comply with these terms.
*Correspondence: Hongping Guo, Z3VvaG9uZ3BpbmdAaGJudS5lZHUuY24=
Disclaimer: All claims expressed in this article are solely those of the authors and do not necessarily represent those of their affiliated organizations, or those of the publisher, the editors and the reviewers. Any product that may be evaluated in this article or claim that may be made by its manufacturer is not guaranteed or endorsed by the publisher.
Research integrity at Frontiers
Learn more about the work of our research integrity team to safeguard the quality of each article we publish.