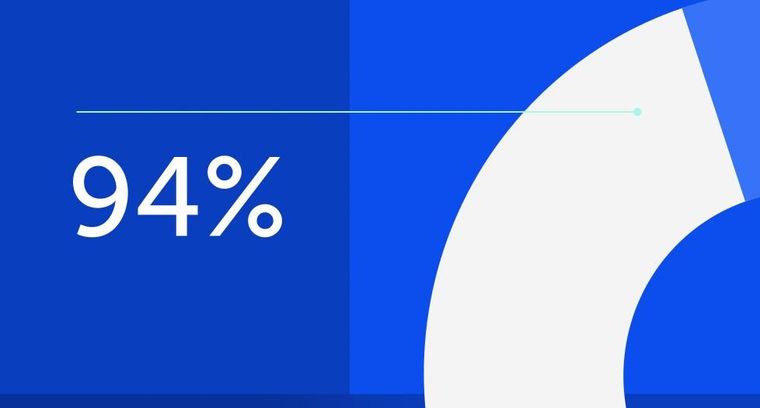
94% of researchers rate our articles as excellent or good
Learn more about the work of our research integrity team to safeguard the quality of each article we publish.
Find out more
ORIGINAL RESEARCH article
Front. Microbiol., 21 February 2023
Sec. Aquatic Microbiology
Volume 14 - 2023 | https://doi.org/10.3389/fmicb.2023.1110025
This article is part of the Research TopicMicrobial Diversity and Ecosystem Functioning in Fragmented Rivers WorldwideView all 16 articles
Rivers are often blocked by barriers to form different habitats, but it is not clear whether this change will affect the accumulation of N2O and CH4 in rivers. Here, low barriers (less than 2 m, LB) increased N2O concentration by 1.13 times and CH4 decreased by 0.118 times, while high barriers (higher than 2 m, less than 5 m high, HB) increased N2O concentration by 1.19 times and CH4 by 2.76 times. Co-occurrence network analysis indicated LB and HB can promote the enrichment of Cyanobium and Chloroflexi, further limiting complete denitrification and increasing N2O accumulation. The LB promotes methanotrophs (Methylocystis, Methylophilus, and Methylotenera) to compete with denitrifiers (Pseudomonas) in water, and reduce CH4 accumulation. While the HB can promote the methanotrophs to compete with nitrifiers (Nitrosospira) in sediment, thus reducing the consumption of CH4. LB and HB reduce river velocity, increase water depth, and reduce dissolved oxygen (DO), leading to enrichment of nirS-type denitrifiers and the increase of N2O concentration in water. Moreover, the HB reduces DO concentration and pmoA gene abundance in water, which can increase the accumulation of CH4. In light of the changes in the microbial community and variation in N2O and CH4 accumulation, the impact of fragmented rivers on global greenhouse gas emissions merits further study.
- Small barriers can promote the accumulation of N2O and CH4 in fragmented river.
- Enrichment of Cyanobium, Chloroflexi, nirS-denitrifiers increases N2O accumulation.
- Small barriers promote the methanotrophs to compete with Nitrosospira in sediment.
- Small barriers reduces DO concentration and pmoA gene abundance in water.
Natural rivers are typically characterized by their free-flowing, and they are often fragmented by barriers to free flow (Grill et al., 2019). Artificial interception (barriers) causes fragmentation and blockage of urban rivers (Grill et al., 2019), resulting in the formation of different habitats of rivers (Carpenter et al., 2011). There are more than 1 million barriers cause of rivers fragmentation (Belletti et al., 2020). Barriers higher than 15 m are rare, 68% had less than 2 m height and 91% had less than 5 m height (Belletti et al., 2020), leading to a major fragmentation caused by small barriers (Jones et al., 2019). However, the environmental effects of small barriers on rivers are not clear, especially whether it affects the accumulation of greenhouse gases in rivers.
There is growing evidence that urban river networks may be hot spots for greenhouse gas (N2O, CH4) emissions (Zhang W. et al., 2021). Inland waters are significant emitters of N2O (Gongqin et al., 2021), and the total estimated N2O from rivers is approximately 1.05 Tg N-N2O Yr−1 (global total emissions are 17.7 Tg N-N2O Yr−1; Frostegard et al., 2022). CH4 is generally considered to be a more important greenhouse gas than N2O (Stein, 2020; Neubauer, 2021). An increasing number of studies have shown that river interception significantly damages river continuity and flood pulsation (Wang et al., 2010), changed the fluxes and ecological dynamics of water and nutrients (Deemer et al., 2016) and affected the migration and transformation process of nitrogen and carbon.
The production of N2O is strongly affected by microorganisms and mainly involves the oxidation and reduction of active nitrogen (ammonia, nitrate, and nitrite; Babbin and Ward, 2013). N2O in rivers is thought to be formed in the bottom sediment, where a variety of microbes produce a large amount of N2O (Beaulieu et al., 2011). Incomplete denitrification is the main cause of N2O production in rivers (Clough et al., 2007; Babbin and Ward, 2013; Lansdown et al., 2015). Variation in river hydrological conditions affects undercurrent exchange, which in turn affects river water quality (such as the reactive nitrogen load) and ultimately N2O production (Marzadri et al., 2014). Given the complexity of N2O generation, N2O accumulation in different habitats of fragmented rivers is still uncertain, especially the effects on the structure and function of N2O accumulation-related microbial communities.
Biomethane sinks are composed mainly of methanophile microorganisms that use methane monooxygenase to oxidize CH4 to methanol using oxygen (Kallistova et al., 2017), which is then oxidized to formaldehyde, formic acid and carbon dioxide by a series of enzymes. It’s estimated that microbial oxidation can remove about 5% of CH4 emissions into the atmosphere each year (Stein, 2020). Current studies suggest that O2 is the factor that strongly affects microbial metabolism and CH4 emissions in river ecosystems (Kallistova et al., 2017). The aggregation of multiple microbial processes in river aerobic/anoxic zones suggests that these zones are highly dynamic in controlling CH4 fluxes and may be the most important region for CH4 mitigation. The riverine barriers slow the flow of fragmented rivers and increase the depth of water, which may lead to a decrease in the concentration of dissolved oxygen (DO) in the water. Changes in the DO concentration of fragmented rivers may lead to changes in microbial activity related to CH4 oxidation and thus affect CH4 accumulation in the rivers. Therefore, it is not clear if the effect of barriers over DO may lead to changes in CH4 oxidation, thus affecting CH4 accumulation.
In this study, Liangtan river (China) was studied as an example of fragmented river, where four habitats were delimited according to the height of the barriers between them. High-throughput sequencing was used to analyze the water and sediment microbial communities to explore how the barriers changed the microbial network structure in the four habitats. Quantitative PCR was used to analyze the changes in gene abundance of functional enzymes, further revealing the metabolic process of N2O and CH4 accumulation in different habitats of fragmented rivers.
The Liangtan River (29°26′–29°52′ N, 106°18′–106°24′ E) is a first-class tributary of the lower right bank of the Jialing River (Figure 1A). In a preliminary investigation, we found more than 15 barriers that led to fragmentation of the river. According to the barrier height, the Liangtan River can be divided into four habitats: pond (P) and stream (S) for low barriers (less than 2 m high, LB) and lake (L) and river (R) for high barriers (between 2 m and 5 m, HB; Figure 1; Fuller et al., 2015; Belletti et al., 2020).
Figure 1. According to the height of artificial barriers above the river (lower than 2 m or lower than 5 m), river channels are divided into four habitats. For LB (barriers less than 2 m high), the matrix habitat formed is called ‘pond’ (P), while the patch habitat is called ‘stream’(S). For HB (barriers less than 5 m high), matrix habitat formed by barriers is called ‘lake’ (L), while patch habitat is called ‘river’ (R). (A) Sampling locations of 20 sites on the Liangtan River. (B) Photographs of the sampling sites in this study. (C) Diagram of river channels division. (D) 20 water samples from four habitats were analyzed by Euclidean distance-based principal component analysis (PCA).
Samples were collected from the four habitats of the Liangtan River (December 2020 and August 2021). Sediment samples were obtained at a depth of 10 cm below the interface sediment: water, whereas water samples were obtained from a total of 20 sampling sites in the river channels. Sediments were immediately placed in sterile bags and sealed, placed on ice bags, shipped back to the laboratory within 12 h, and stored in a −80°C freezer. The water samples were divided into two parts. The first part was supplemented with HgCl2 (20 μg/mL) for subsequent determination of water quality indicators. The other part was returned to the laboratory within 12 h and filtered through a 0.22-μm glass fiber membrane to remove trapped microorganisms for subsequent high-throughput sequencing. Characteristics of the 20 sites studied (5 sites per habitat) in the Liangtan River are shown in Supplementary Table S1.
Electrical conductivity (EC), water temperature (T), and DO were determined by a YSI® ProODO DO meter, and the pH was determined by a YSI® 63 pH meter. Total organic carbon (TOC) was determined by a Shimazu® TOC-VWP analyzer (Shimadzu®, Japan). Chlorophyll a (Chl a) was extracted by ethanol and determined by spectrophotometry (Ortega et al., 2019). Total nitrogen (TN) was measured by the potassium persulfate oxidation method (Zhang L. Y. et al., 2021). Total phosphorus (TP) was extracted by HClO4-H2SO4 and measured by the molybdenum blue method. Nitrite nitrogen (NO2−-N) was determined by N-(1-)-ethylenediamine spectrophotometry. Nitrate nitrogen (NO3−-N) was measured by potassium peroxodisulfate solution spectrophotometric methods. Ammonium nitrogen (NH4+-N) was measured by spectrophotometry with a Nessler reagent (Ministry of Environmental Protection, 2012).
Total DNA was extracted from 0.5-g sediment samples using a Rapid Soil DNA Isolation Kit. The concentration of the extracted DNA was then quantified using an ND-2000C spectrophotometer (Nanodrop, Thermo Scientific, United States), and the extracted DNA was used for high-throughput sequencing. The V3-V4 hypervariable region of the 16S rRNA gene was amplified with 338F/806R universal primers (Supplementary Table S2), and each sample was identified. Specific PCR amplification conditions of the 16S rRNA gene were based on those described in previous studies (Junfeng et al., 2015). The resulting PCR products were then sequenced on an Illumina HiSeq 2000 platform. Data processing and statistical analysis were carried out (see the attachment for specific methods). The raw data of Illumina Miseq sequencing were submitted to the NCBI under the BioProject accession number PRJNA904922.
The concentrations of N2O and CH4 in water were measured using the static headspace method. In this study, the traditional method (Donis et al., 2017) was improved. Firstly, a syringe is used to extract 200 mL water sample from the water sampler. The sample should be extracted slowly during the collection process to avoid bubbles. Extract 100 mL nitrogen from an air bag containing high purity nitrogen (99.999% purity) (500 mL air bag) to form an air chamber above the syringe (the same syringe used to collect water sample). Hold the syringe and shake it up and down for 3 min to achieve a balance between the gas–liquid phase. Push the gas in the syringe into the prepared vacuum air bag (300 mL air bag) for preservation. Record the temperature of the water after the shake. The preserved headspace samples were returned to the laboratory and the concentration of N2O and CH4 was determined by gas chromatography.
16S rRNA and the abundance of functional genes of denitrifying bacteria were detected by qPCR using the Majorbio Cloud Platform (Shanghai). The functional genes were mainly divided into nitrite reductase (nir), NO reductase (nor), N2O reductase (nos), and particulate methane monooxygenase (pmo). Three repeats of qPCR were performed on each sample using an ABI 7500 sequence detection system with the SYBR method (Applied Biosystems, Canada). Primer sequences are listed in Supplementary Table S2. qPCR-specific tests were performed using a melting profile and gel electrophoresis to reduce the possibility of overestimating gene abundances. In addition, each qPCR reaction consisted of negative control, in which no DNA template was added. The abundance of functional genes was converted to the number of copies of functional genes per gram of dry sediment or per milliliter of water. The efficiency was assumed to be 100% for DNA extracted from sediment or water samples (Zheng et al., 2019).
One-way ANOVA and Tukey’s post hoc tests were used to evaluate the differences in microbial diversity and abundance, structure, and environmental factors among habitat types. The above statistical analyses were conducted using PASW Statistics 18 software (IBM SPSS Inc., Chicago, United States). The molecular ecological networks of habitats S, P, R, and L were constructed to analyze the microbial community structure. Linear discriminant analysis (LDA) was used to estimate biomarkers of the four habitats based on analysis software (LEfSe). Based on Origin, a heatmap was drawn to analyze the microbial abundance related to the production of N2O and CH4 in the four habitats. To further elucidate the direct and indirect effects of environmental factors and microbial communities on N2O concentrations and CH4 concentrations, we conducted path analyses using the maximum likelihood estimation method. Gephi version 0.8.2 was used for network visualization and modular analysis. According to Banerjee et al. (2018), keystone species were identified using the following thresholds: genera with high mean degree (>30) and low betweenness centrality (<700) in the network. Path coefficients, R2, direct and indirect effects, and model fit parameters were calculated using R studio.
Samples were taken from five randomly selected points in each habitat (Figures 1A–C). Through principal component cluster analysis of physical and chemical indexes of four habitats, it was found that the four habitats could be distinguished well, which indicated that it was appropriate to divide the different habitats of Liangtan River (Figure1D). The abundance and composition at the phylum level of microbial communities from water and sediment was analyzed for each habitat (S, P, R, and L; Supplementary Figure S1). A total of 14,481,930 high-quality bacterial 16S rRNA gene sequences and 30,863 operational taxonomic units (OTUs) were obtained by high-throughput sequencing.
The relative abundances of the phyla Proteobacteria, Bacteroidota, and Cyanobacteria were higher in water (17.6–76.5%, 2.8–57.9%, and 0.2–41.4%, respectively) than in sediment (7.0–45.8%, 1.3–9.2%, and 0.2–4.2%, respectively). In contrast, the relative abundances of Chloroflexi, Firmicutes, Acidobacteriota, Verrucomicrobiota, and Desulfobacterota were higher in sediment (4.3–35.3%, 2.0–31.1%, 2.2–14.8%, 1.5–10.0%, and 0.1–9.0%, respectively) than in water (0.05–6.5%, 0.7–13.5%, 0.03–0.9%, 0.1–4.5%, and 0.06–1.0%, respectively, Supplementary Figure S2). Most of these biomarkers were abundant in the sediment of S and R. There were 31 and 102 biomarkers (p < 0.05, LDA > 2.0) in water and sediment, respectively (Figure 2). The classes Acetobacteraceae, Blastocatellales, Microtrichales, and Pseudomonadales were the main biomarkers in sediment samples from S. Species belonging to Rhodobacterales, Xanthomonadales, and Chitinophagales dominated in sediment samples from R. Flavobacteriaceae and Fusobacteriaceae species were most abundant in water samples from S. Species affiliated with Veillonellales, Prevotellaceae, Nocardioidaceae, and Lachnospirales were abundant in water samples from R (Figure 2). The number of biomarkers was lower in L and P. In L, the classes Ncardioidaceae and Micrococcales were the main biomarkers in sediment, and Saccharimonadales was dominant in water. In P, the classes Norank, Fusobacteriales, and Prolixibacteraceae were the main biomarkers in sediment, whereas RBG-13-54-9, Chitinophagales, and PeM15 were dominant in water.
Figure 2. The abundant biomarkers in water (A) and sediment (B) obtained for four habitats. All detected taxa, with a relative abundance of >0.5% in at least one sample, were assigned to phyla (outermost), classes, orders, families, and genera (innermost) and were used to determine the taxa or clades most likely to explain differences between the four habitats.
The topological structures of the bacterial communities at the OTU level are shown in Figure 3. Regarding the sediment samples, the percentage of Chloroflexi nodes increased in P compared with that in S, while the node ratio in Proteobacteria and Actinobacteriota decreased. Similarly, the percentage of Chloroflexi nodes increased in P compared with that in S, while the node ratio in Proteobacteria and Actinobacteriota decreased.
Figure 3. Co-occurrence networks and their topological properties of different bacterial communities in the water and sediment.
Then we further analyzed the variation of ecological network parameters in the four habitats. The two networks were defined according to the same threshold, and the corresponding statistical results are shown in Supplementary Table S3. In water, the modularity of the S bacterial network (1.734) was lower than that of the P bacterial network (3.147). In S, the resulting networks consisted of 334 nodes linked by 2,809 edges, which consisted of 1,816 positive correlations and 993 negative correlations. In P, the resulting networks consisted of 287 nodes linked by 2,105 edges, which consisted of 1,210 positive correlations and 895 negative correlations. Compared with R, the modularity of L was lower. In R, the resulting networks consisted of 320 nodes linked by 2,812 edges, which consisted of 1,759 positive correlations and 1,042 negative correlations. In L, the resulting networks consisted of 300 nodes linked by 2,816 edges, which consisted of 1,764 positive correlations and 1,052 negative correlations. The presence of barriers will reduce the number of nodes, and positive or negative correlations in the microbial network, leading to the estrangement of microbial connections in the water.
In sediments, the modularity of S was higher than that of P, and that of L was higher than that of R. In S, the network consisted of 370 nodes and 3,756 edges. The network showed 2,293 positive correlations, which was higher than the negative correlations (1,463 green lines). In P, the network consisted of 388 nodes and 4,789 edges. The network showed 2,967 positive correlations and 1,822 negative correlations. In R, the network consisted of 409 nodes and 4,128 edges. The network showed 2,730 positive correlations and 1,428 negative correlations. In L, the network consisted of 373 nodes and 4,733 edges. The network showed 2,735 positive correlations and 1,999 negative correlations. This suggested that the presence of obstacles may lead to closer connections of microbial networks in sediments.
In water, the abundances of anammox bacteria and nitrifiers were low, and denitrifiers (Pseudomonas) and methanotrophs (Methylocystis) were more abundant (Figure 4A). The denitrifying bacterial abundance of P was higher than that of S, and that of L was higher than that of R. The methanotrophs abundance of S was higher than that of P, and that of L was higher than that of R. In the sediment, the abundances of anammox bacteria and denitrifiers were low, the nitrifiers (Nitrosospira) and methanotrophs (Methylophilus, Methylocystis, Methylocystis) were more abundant (Figure 4B). The nitrifying bacterial abundance of S was higher than that of P, and that of R was higher than that of L. The methanotrophs abundance of S was higher than that of P, and that of L was higher than that of R.
Figure 4. Heatmap of anammox bacteria, nitrifiers, denitrifiers and methanotrophs (average abundance in all samples) of water and sediment in four habitats.
The concentrations of dissolved N2O in S, P, R, and L were 0.014–0.029, 0.014–0.037, 0.019–0.078, and 0.012–0.11 μmol/L, respectively (Figure 5A). Compared with S, the concentration of N2O in P showed an increasing trend. The concentration of N2O in L was higher than that in R. LB and HB can increase the N2O concentration in rivers by 1.13 and 1.29 times, respectively. The concentrations of dissolved CH4 in S, P, R, and L were 0.30–4.18, 0.04–0.67, 0.03–2.4, and 0.03–34.0 μmol/L, respectively (Figure 5B). The CH4 concentration in S was higher than that in P, and that in L was higher than that in R, it can be inferred that that LB reduces CH4 accumulation and HB increase it. LB reduced the CH4 concentration by 0.118 times, while HB increased it by 2.76 times.
Next, we conducted path analyses to further elucidate the direct and indirect effects of environmental factors and microbial communities on N2O concentrations and CH4 concentrations. DO and NO3−-N could regulate the concentration of N2O in water both directly and indirectly (Figure 6A). The regulation of DO to N2O is reverse, while that of NO3−-N is positive. DO (β = −0.18, standardized coefficient) directly impacted the concentration of N2O. NO3−-N was the most significant parameter (β = 0.76, standardized coefficient) influencing the concentration of N2O. NO3−-N indirectly through water denitrifying communities (β = 1.32, standardized coefficient) impacted the concentration of N2O. Furthermore, TN indirectly through sediment nitrifying bacterial community (β = 0.41, standardized coefficient) impacted the concentration of N2O. Moreover, DO directly (β = −0.35, standardized coefficient), and indirectly through soil pH (β = −0.31, standardized coefficient), impacted the concentration of CH4 (Figure 6B).
Figure 6. Path diagrams estimating the direct and indirect effects of environmental factors and microbial communities on N2O concentrations (A) and CH4 concentrations (B). Solid lines demonstrate significant effects (p < 0.05), and dashed lines indicate insignificant effects. Numbers adjacent to the arrows are standardized path coefficients. Single headed arrows refer to unidirectional causal relationships. Denitrifying community (W): abundance of denitrifiers in water. Nitrifying community (S): abundance of nitrifiers in sediment. Methane-oxidizing community (W): abundance of methanotrophs in water. Methane-oxidizing community (S): abundance of methanotrophs in sediment.
As can be seen from the above analysis, the LB and HB promote the enrichment of denitrifiers in the P and L habitat. To further verify our hypothesis, the abundances of functional enzyme genes of denitrifiers of water were analyzed in four habitats. The abundances of nirS, nirK, and nosZ in water and sediment were counted (Supplementary Tables S6–S9). To estimate the abundance contribution of different genes in the denitrification pathway to N2O accumulation, the nisS/nirK and (nisS + nirK)/nosZ copy number ratios were analyzed (Figure 7). The nirS/nirK of P and L was higher than that of S and R, suggesting that the LB and HB promote the enrichment of nirS-type denitrifiers, and then affect the accumulation of N2O. In sediments (nirS + nirK)/nosZ copy number ratios were decreased in P and L compared with S and R, suggesting that the LB and HB promoted the nosZ enrichment in sediments.
Figure 7. Gene ratios of functional enzymes in water and sediment of four habitats. (A) nirS/nirK copy number ratios in water. (B) (nirS + nirK)/nosZ copy number ratios in sediment. (C) pmoA/16S rRNA copy number ratios in water. (D) pmoA/16S rRNA copy number ratios in sediment. The bacteria represent the abundance of the total bacterial 16S rRNA gene.
Then, the abundances of pmoA in water and sediment were counted (Supplementary Tables S6–S9). To estimate the contribution of functional genes to the abundance of CH4 accumulation in CH4 oxidation, we analyzed the pmoA/16S rRNA copy number ratio in water and sediment of four habitats (Figure 7). pmoA is a key enzyme gene for CH4 oxidation, which reduce CH4 accumulation (Lieberman and Rosenzweig, 2004; Vrieze and Verstraete, 2016). In water, the pmoA/16S rRNA copy number ratio of R was the highest, while in sediment, the pmoA/16S rRNA copy number ratio of P was the highest. These results further suggest that the presence of small barriers affects CH4 accumulation by altering the activities of enzymes involved in methane oxidation.
LB and HB lead to lower flow rates and increased water depth in fragmented rivers, which change environmental variables and affect N2O and CH4 accumulation. The small barrier leads to an increase in the concentration of NO3−-N (Supplementary Tables S4, S5), which promotes the accumulation of N2O in P and L. Both LB and HB reduce river velocity, leading to an increase in the water bed contact area per unit of water volume, and an increase in the diffusion efficiency of NO3−-N at the river-sediment interface (Mulholland et al., 2008). This is consistent with previous work showing that nitrate concentrations strongly affect the production of N2O (Meyer et al., 2008). With few exceptions, the nitrate concentration in sediment and surface water is positively correlated with the production of N2O (Quick et al., 2019). The positive correlation between N2O and NO3−-N might stem from incomplete denitrification (Beaulieu et al., 2011; Abed et al., 2013; Jung et al., 2019). LB and HB promoted the enrichment of denitrifiers in the water of P and L respectively, which may lead to an increase in incomplete denitrification under unit nitrogen load (Mulholland et al., 2008).
Moreover, in most rivers, the overlying water above the surface of the sediment is anoxic and carries dissolved oxygen that continuously seeps into the sediment (Xia et al., 2018). The depth of dissolved oxygen penetration into sediment is affected by sediment roughness, porosity, and connectivity (Xia et al., 2018). LB and HB lead to increased water depth and decreased DO concentration in the river (Supplementary Figure S3), which is conducive to incomplete denitrification.
In addition, the higher abundance of nitrifiers (Nitrosospira) in the lotic habitats (S and R) suggest that the LB and HB would inhibit the growth of nitrifiers. The main reason is that the barriers reduce the DO in the river, thus inhibiting the growth of nitrifiers (aerobic bacteria). At present, there are still many uncertainties about the relationship between nitrifiers and N2O accumulation (Beaulieu et al., 2010). According to our results, the decrease of nitrifiers promotes N2O accumulation to a certain extent in river surface sediments.
According to the CH4 concentration in four habitats, the CH4 concentration in P was lower than in S. The main reason was that the abundance of methanotrophs in P was higher than that in S, resulting in a large amount of CH4 consumption. It can be seen that the LB reduces CH4 accumulation. Compared with R, the CH4 concentration in L was higher. The potential reason was that the concentration of DO in L was reduced, which was conducive to the generation of CH4 under anaerobic conditions. It can be seen that the HB increases the accumulation of CH4, these results were consistent with other literature reports (He et al., 2021).
Denitrifiers and methanotrophs were abundant in the four habitats and significantly affected N2O production and CH4 oxidation, respectively. Hence, a co-occurrence network analysis was conducted to identify the cooperative and competitive relationships among denitrifiers, methanotrophs, and other microorganisms. In networks of P, Proteobacteria was still the first dominant phylum, and the second dominant phylum was Cyanobacteria. Cyanobium_PCC-6307 is a genus with high content of Cyanobacteria phylum. It has been reported that the abundance of denitrifiers has a competitive relationship with non-diazotrophic Cyanobium sp. (Song et al., 2022), so it is further speculated that LB increases the abundance of Cyanobium, further limits complete denitrification and increases N2O accumulation. Interestingly, denitrifiers (Pseudomonas) and methanotrophs (Methylocystis, Methylophilus, Methylotenera), were dominant nodes in water. Previous studies have also reported low N2O and high CH4 fluxes in rivers, which they suggest are due to competition (Deemer et al., 2016). The potential cause is the production of a copper chelator (methanobactin) by methanotrophs during the CH4 cycle (Spirito et al., 2016), which has been shown to effectively compete for copper from denitrifiers, thereby increasing N2O production while reducing CH4 emissions (Chang et al., 2018). Similarly, the availability of copper is particularly important for nosZ expression and activity, since copper is required for the active site of the enzyme (Gaimster et al., 2018). nosZ will compete with methanotrophs for copper ions, thus reducing N2O production and increasing CH4 accumulation. This is consistent with our results that the ratio of nosZ and concentration of CH4 in S and L is higher than that of P and R (Supplementary Figure S4).
In sediments, the Chloroflexi was the dominant bacteria in P and L habitats, it was speculated that the accumulation of Chloroflexi may promote the accumulation of N2O. It has been reported that bacteria attached to Chloroflexi may be highly active protein degradation, breaking down the extracellular peptides bound to the extracellular polymer matrix and simultaneously breathing nitrate to produce nitrite (Lawson et al., 2017). The increase of nitrite can promote denitrification (Quick et al., 2019), and thus increase the accumulation of N2O, which further proves that the barriers can increase the accumulation of N2O. Unlike in water, nitrifiers (Nitrosospira) and methanotrophs (Methylocystis, Methylophilus, Methylotenera), were dominant nodes in sediments. It has been reported that nitrifiers can be inhibited by methanotrophs due to competition between nitrifiers and methanotrophs for available oxygen and inorganic nitrogen (Megmw and Knowles, 1987). As LB and HB reduce DO concentration, nitrifiers in P and L were more severely inhibited compared with S and R, resulting in the stronger activity of methanotrophs. On the other hand, ammoxidation microorganisms may consume CH4, thereby reducing the amount of CH4 oxidized by methanotrophs (Bodelier and Laanbroek, 2004), and thus competing with methanotrophs for CH4. Therefore, the decrease of CH4 content in habitats P and R was also related to the nitrifiers.
In order to further analyze the accumulation patterns of N2O and CH4 in fragmented rivers, the potential metabolic pathways of N2O and CH4 in different habitats were further analyzed. Compared with microbial functional groups, the metabolism between functional enzyme genes can better reveal the coupling relationship of element metabolic processes in environmental media. First, the potential N2O metabolism pathways of microorganisms in four habitats were analyzed. The analysis of the metabolic processes of nitrogenous microorganisms in four habitats revealed that the content of enzymes ([EC:1.7.7.2]) involved in nitrate transformation processes was higher in P compared with S in water (Figure 8), and this promoted the generation of N2O. This further proves that LB promotes the generation of N2O. The expression of enzyme genes involved in the dissimilatory nitrate reduction to ammonium (DNRA) process (Li et al., 2019) ([EC:1.7.1.15], [EC:1.7.7.1], [EC:1.7.2.2]) was higher in S than in P in sediment. The results indicated that more NO2-was converted into NH4+ in S, which reduced the possibility of N2O formation. The content of enzymes ([EC:1.7.2.6]) involved in nitrate transformation processes was higher in L compared with R in water and sediment, and indirectly promotes the generation of N2O. The expression of enzyme genes involved in the DNRA process ([EC:1.7.1.15]) was higher in R than in L in sediment. The results indicated that more NO2− was converted into NH4+ in R, which reduced the possibility of N2O formation. Some studies have shown that the growth rate of a new class of non-denitrifying N2O reductants may be slow, but the isolated strains have great metabolic flexibility, enabling them to grow through DNRA (Sanford et al., 2012; Jones et al., 2014), which plays an important role in N2O reduction. It indicates that both LB and HB can promote the DNRA process, thus increasing the accumulation of N2O.
Figure 8. KEGG pathways and functional genes related to N2O and CH4 metabolism in the water (A) and sediment (B) of four habitats. DNRA, dissimilatory nitrate reduction to ammonium.
The analysis of the metabolic processes revealed that the content of enzymes (EC:1.17.1.10; Li et al., 2019) involved in CH4 oxidation processes was higher in P compared with S in sediment (Figure 8), and directly reduced the generation of CH4. It further shows that LB inhibits CH4 accumulation by promoting CH4 oxidation. The enzymes (EC:1.14.18.3; Zhang et al., 2020) involved in CH4 oxidation processes were higher in R compared with L in water and sediment, and directly reduced the accumulation of CH4. It further shows that HB increases CH4 accumulation by inhibiting CH4 oxidation. Some studies have speculated that aerobic CH4 oxidation coupled to denitrification process and anaerobic nitrite-dependent CH4 oxidation processes in river sediments (Zhang et al., 2020). It was speculated that the barriers may affect these two processes and thus the accumulation of N2O and CH4.
In summary, we preliminarily sorted out the accumulation patterns of N2O and CH4 in fragmented rivers (Figure 9). It was found that N2O accumulated continuously with the increase of barriers height, and the potential reasons were related to enrichment of Cyanobium, Chloroflexi, and nirS-type denitrifiers, as well as the increase of NO3−-N concentration and decrease of DO concentration in water (Figure 9). The reason for the decrease of CH4 concentration caused by the LB may be related to the compete with Pseudomonas in water. The HB can lead to the accumulation of CH4, which was largely related to the methanotrophs to compete with Nitrosospira in sediment and the decrease of DO concentration.
Figure 9. Accumulation patterns of N2O and CH4 in fragmented rivers. The water concentration of N2O increases with the height of barriers. The barriers (less than 2 m) inhibit CH4 accumulation, while barriers (higher than 2 m, less than 5 m high) promote CH4 accumulation. The darker the arrow, the higher the solute concentration.
In this study, the small barriers lead to the formation of different habitats, and the microbial structure and network also change. Both LB and HB reduce river velocity, leading to an increase in the water bed contact area per unit of water volume, and enrichment of nirS-type denitrifiers in water, promoting the accumulation of N2O. The LB and HB lead to the increase of water depth, which reduces the DO concentration, relieves the inhibition of oxygen on nitrate reductase activity, and promotes the accumulation of N2O.
In addition, the LB leads to an increase in the methanotrophs abundance in water, and an increase in the abundance of the pmoA gene in sediments, which reduces the accumulation of CH4. Moreover, the HB reduces DO concentration and pmoA gene abundance in water, which can increase the accumulation of CH4.
The datasets presented in this study can be found in online repositories. The names of the repository/repositories and accession number(s) can be found in the article/Supplementary material.
C-YX, HL, QL, and ZL designed the experiments. C-YX, HL, and QL performed the experiments. C-YX and ZL analyzed the data and wrote the manuscript. L-HL was involved in interpretation of results, and figures and table arrangement. All authors revised the manuscript, read, and approved the final manuscript.
The authors gratefully acknowledge the support from the National Key Research and Development Program of China (2022YFC3203504), the National Natural Science Foundation of China (42207243), the Chongqing Science and Technology Bureau (cstc2020jcyj18511jqX0010, sl202100000123), and China Three Gorges Corporation.
We thank Majorbio for bioinformatics and analysis. The sequencing of all samples in this study was performed by the Shanghai Majorbio Bio-pharm Technology Co. Ltd. The data were analyzed on the online platform of Majorbio Cloud Platform (www.majorbio.com).
The authors declare that the research was conducted in the absence of any commercial or financial relationships that could be construed as a potential conflict of interest.
All claims expressed in this article are solely those of the authors and do not necessarily represent those of their affiliated organizations, or those of the publisher, the editors and the reviewers. Any product that may be evaluated in this article, or claim that may be made by its manufacturer, is not guaranteed or endorsed by the publisher.
The Supplementary material for this article can be found online at: https://www.frontiersin.org/articles/10.3389/fmicb.2023.1110025/full#supplementary-material
Abed, R. M. M., Lam, P., de Beer, D., and Stief, P. (2013). High rates of denitrification and nitrous oxide emission in arid biological soil crusts from the Sultanate of Oman. ISME J. 7, 1862–1875. doi: 10.1038/ismej.2013.55
Babbin, A. R., and Ward, B. B. (2013). Controls on nitrogen loss processes in Chesapeake bay sediments. Environ. Sci. Technol. 47, 4189–4196. doi: 10.1021/es304842r
Banerjee, S., Schlaeppi, K., and van der Heijden, M. G. A. (2018). Keystone taxa as drivers of microbiome structure and functioning. Nat. Rev. Microbiol. 16, 567–576. doi: 10.1038/s41579-018-0024-1
Beaulieu, J. J., Shuster, W. D., and Rebholz, J. A. (2010). Nitrous oxide emissions from a large, impounded river: the Ohio River. Environ. Sci. Technol. 44, 7527–7533. doi: 10.1021/es1016735
Beaulieu, J. J., Tank, J. L., Hamilton, S. K., Wollheim, W. M., Hall, R. O., Mulholland, P. J., et al. (2011). Nitrous oxide emission from denitrification in stream and river networks. Proc. Natl. Acad. Sci. U.S.A. 108, 214–219. doi: 10.1073/pnas.1011464108
Belletti, B., de Leaniz, C. G., Jones, J., Bizzi, S., Boerger, L., Segura, G., et al. (2020). More than one million barriers fragment Europe’s rivers. Nature 588, 436–441. doi: 10.1038/s41586-020-3005-2
Bodelier, P. L. E., and Laanbroek, H. J. (2004). Nitrogen as a regulatory factor of methane oxidation in soils and sediments. FEMS Microbiol. Ecol. 47, 265–277. doi: 10.1016/S0168-6496(03)00304-0
Carpenter, S. R., Stanley, E. H., and Vander Zanden, M. J. (2011). “State of the world’s freshwater ecosystems: physical, chemical, and biological changes,” in Series Annual Review of Environment and Resources. eds. A. Gadgil and D. M. Liverman, vol. 36 (Literature type Review), 75–99.
Chang, J., Gu, W., Park, D., Semrau, J. D., DiSpirito, A. A., and Yoon, S. (2018). Methanobactin from methylosinus trichosporium OB3b inhibits N2O reduction in denitrifiers. ISME J. 12, 2086–2089. doi: 10.1038/s41396-017-0022-8
Clough, T. J., Addy, K., Kellogg, D. Q., Nowicki, B. L., Gold, A. J., Groffman, P. M., et al. (2007). Dynamics of nitrous oxide in groundwater at the aquatic–terrestrial interface. Global Change Biol. 13, 1528–1537. doi: 10.1111/j.1365-2486.2007.01361.x
Deemer, B. R., Harrison, J. A., Li, S., Beaulieu, J. J., Delsontro, T., Barros, N., et al. (2016). Greenhouse gas emissions from reservoir water surfaces: a new global synthesis. Bioscience 66, 949–964. doi: 10.1093/biosci/biw117
Donis, D., Flury, S., Stockli, A., Spangenberg, J. E., Vachon, D., and McGinnis, D. F. (2017). Full-scale evaluation of methane production under oxic conditions in a mesotrophic lake. Nat. Commun. 8:1661. doi: 10.1038/s41467-017-01648-4
Frostegard, A., Vick, S. H. W., Lim, N. Y. N., Bakken, L. R., and Shapleigh, J. P. (2022). Linking meta-omics to the kinetics of denitrification intermediates reveals pH-dependent causes of N2O emissions and nitrite accumulation in soil. ISME J. 16, 26–37. doi: 10.1038/s41396-021-01045-2
Fuller, M. R., Doyle, M. W., and Strayer, D. L.,(2015). Causes and consequences of habitat fragmentation in river networks. In Year in Ecology and Conservation Biology, A. G. Power and R. S. Ostfeld (Eds). New York, NY: New York Academy of Sciences
Gaimster, H., Alston, M., Richardson, D. J., Gates, A. J., and Rowley, G. (2018). Transcriptional and environmental control of bacterial denitrification and N2O emissions. FEMS Microbiol. Lett. 365:fnx277. doi: 10.1093/femsle/fnx277
Gongqin, W., Xinghui, X., Shaoda, L., Sibo, Z., Weijin, Y., et al. (2021). Distinctive patterns and controls of nitrous oxide concentrations and fluxes from urban inland waters. Environ. Sci. Technol. 55, 8422–8431. doi: 10.1021/acs.est.1c00647
Grill, G., Lehner, B., Thieme, M., Geenen, B., Tickner, D., Antonelli, F., et al. (2019). Mapping the world’s free-flowing rivers. Nature 569, 215–221. doi: 10.1038/s41586-019-1111-9
He, D., Xiao, J., Wang, D., Liu, X., Fu, Q., Li, Y., et al. (2021). Digestion liquid based alkaline pretreatment of waste activated sludge promotes methane production from anaerobic digestion. Water Res. 199:117198. doi: 10.1016/j.watres.2021.117198
Jones, J., Borger, L., Tummers, J., Jones, P., Lucas, M., Kerr, J., et al. (2019). A comprehensive assessment of stream fragmentation in Great Britain. Sci. Total Environ. 673, 756–762. doi: 10.1016/j.scitotenv.2019.04.125
Jones, C. M., Spor, A., Brennan, F. P., Breuil, M.-C., Bru, D., Lemanceau, P., et al. (2014). Recently identified microbial guild mediates soil N2O sink capacity. Nat. Clim. Chang. 4, 801–805. doi: 10.1038/nclimate2301
Junfeng, L., Junyi, Z., Liyang, L., Yucai, F., Lianshuo, L., Yunfeng, Y., et al. (2015). Annual periodicity in planktonic bacterial and archaeal community composition of eutrophic Lake Taihu. Sci. Rep. 5:15488. doi: 10.1038/srep15488
Jung, M. Y., Gwak, J. H., Rohe, L., Giesemann, A., Kim, J. G., Well, R., et al. (2019). Indications for enzymatic denitrification to N2O at low pH in an ammonia-oxidizing archaeon. ISME J. 13, 2633–2638. doi: 10.1038/s41396-019-0460-6
Kallistova, A. Y., Merkel, A. Y., Tarnovetskii, I. Y., and Pimenov, N. V. (2017). Methane formation and oxidation by prokaryotes. Microbiology 86, 671–691. doi: 10.1134/S0026261717060091
Lansdown, K., Heppell, C. M., Trimmer, M., Binley, A., Heathwaite, A. L., Byrne, P., et al. (2015). The interplay between transport and reaction rates as controls on nitrate attenuation in permeable, streambed sediments. J. Geophys. Res. 120, 1093–1109. doi: 10.1002/2014JG002874
Lawson, C. E., Wu, S., Bhattacharjee, A. S., Hamilton, J. J., McMahon, K. D., Goel, R., et al. (2017). Metabolic network analysis reveals microbial community interactions in anammox granules. Nat. Commun. 8:15416. doi: 10.1038/ncomms15416
Li, H., Chi, Z. F., Li, J. L., Wu, H. T., and Yan, B. X. (2019). Bacterial community structure and function in soils from tidal freshwater wetlands in a Chinese delta: potential impacts of salinity and nutrient. Sci. Total Environ. 696:134029. doi: 10.1016/j.scitotenv.2019.134029
Lieberman, R. L., and Rosenzweig, A. C. (2004). Biological methane oxidation: regulation, biochemistry, and active site structure of particulate methane monooxygenase. Crit. Rev. Biochem. Mol. 39, 147–164. doi: 10.1080/10409230490475507
Marzadri, A., Tonina, D., Bellin, A., and Tank, J. L. (2014). A hydrologic model demonstrates nitrous oxide emissions depend on streambed morphology. Geophys. Res. Lett. 41, 5484–5491. doi: 10.1002/2014GL060732
Megmw, S. R., and Knowles, R. (1987). Active methanotrophs suppress nitrification in a Humisol. Biol. Fertil. Soils 4, 205–212. doi: 10.1007/BF00270942
Meyer, R. L., Allen, D. E., and Schmidt, S. (2008). Nitrification and denitrification as sources of sediment nitrous oxide production: a microsensor approach. Mar. Chem. 110, 68–76. doi: 10.1016/j.marchem.2008.02.004
Ministry of Environmental Protection (2012). Soil-Determination of Ammonium, Nitrite and Nitrate by Extraction With Potassium Chloride Solution-Spectrophotometric Methods. China Environmental Science Press: Beijing
Mulholland, P. J., Helton, A. M., Poole, G. C., Hall, R. O., Hamilton, S. K., Peterson, B. J., et al. (2008). Stream denitrification across biomes and its response to anthropogenic nitrate loading. Nature 452, 202–205. doi: 10.1038/nature06686
Neubauer, S. C. (2021). Global warming potential is not an ecosystem property. Ecosystems 24, 2079–2089. doi: 10.1007/s10021-021-00631-x
Ortega, S. H., González-Quijano, C. R., Casper, P., Singer, G. A., and Gessner, M. O. (2019). Methane emissions from contrasting urban freshwaters: rates, drivers, and a whole-city footprint. Glob. Chang. Biol. 25, 4234–4243. doi: 10.1111/gcb.14799
Quick, A. M., Reeder, W. J., Farrell, T. B., Tonina, D., Feris, K. P., and Benner, S. G. (2019). Nitrous oxide from streams and rivers: a review of primary biogeochemical pathways and environmental variables. Earth Sci. Rev. 191, 224–262. doi: 10.1016/j.earscirev.2019.02.021
Sanford, R. A., Wagner, D. D., Wu, Q., Chee-Sanford, J. C., Thomas, S. H., Cruz-Garcia, C., et al. (2012). Unexpected nondenitrifier nitrous oxide reductase gene diversity and abundance in soils. Proc. Natl. Acad. Sci. U.S.A. 109, 19709–19714. doi: 10.1073/pnas.1211238109
Song, K., Senbati, Y., Li, L., Zhao, X., Xue, Y., and Deng, M. (2022). Distinctive microbial processes and controlling factors related to indirect N2O emission from agricultural and urban rivers in Taihu watershed. Environ. Sci. Technol. 56, 4642–4654. doi: 10.1021/acs.est.1c07980
Spirito, A. A., Semrau, J. D., Murrell, J. C., Gallagher, W. H., Dennison, C., and Vuilleumier, S. (2016). Methanobactin and the link between copper and bacterial methane oxidation. Microbiol. Mol. Biol. R. 80, 387–409. doi: 10.1128/MMBR.00058-15
Stein, L. Y. (2020). The long-term relationship between microbial metabolism and greenhouse gases. Trends Microbiol. 28, 500–511. doi: 10.1016/j.tim.2020.01.006
Vrieze, J., and Verstraete, W. (2016). Perspectives for microbial community composition in anaerobic digestion: from abundance and activity to connectivity. Environ. Microbiol. 18, 2797–2809. doi: 10.1111/1462-2920.13437
Wang, F., Yu, Y., Liu, C., Wang, B., Wang, Y., and Guan, J. (2010). Dissolved silicate retention and transport in cascade reservoirs in Karst area, Southwest China. Sci. Total Environ. 408, 1667–1675. doi: 10.1016/j.scitotenv.2010.01.017
Xia, X., Zhang, S., Li, S., Zhang, L., Wang, G., Zhang, L., et al. (2018). The cycle of nitrogen in river systems: sources, transformation, and flux. Environ Sci. Proc. Imp. 20, 863–891. doi: 10.1039/C8EM00042E
Zhang, L. Y., Delgado-Baquerizo, M., Shi, Y., Liu, X., Yang, Y. F., and Chu, H. Y. (2021). Co-existing water and sediment bacteria are driven by contrasting environmental factors across glacier-fed aquatic systems. Water Res. 198:117139. doi: 10.1016/j.watres.2021.117139
Zhang, M., Li, Y., Sun, Q., Chen, P., and Wei, X. (2020). Correlations of functional genes involved in methane, nitrogen and sulfur cycling in river sediments. Ecol. Indic. 115:106411. doi: 10.1016/j.ecolind.2020.106411
Zhang, W., Li, H., Xiao, Q., and Li, X. (2021). Urban rivers are hotspots of riverine greenhouse gas (N2O, CH4, CO2) emissions in the mixed-landscape Chaohu lake basin. Water Res. 189:116624. doi: 10.1016/j.watres.2020.116624
Keywords: river fragmentation, greenhouse gases, microbial community, habitats, low barriers
Citation: Xing C-Y, Li H, Li Q, Lu L-H and Li Z (2023) Shifts in composition and function of bacterial communities reveal the effect of small barriers on nitrous oxide and methane accumulation in fragmented rivers. Front. Microbiol. 14:1110025. doi: 10.3389/fmicb.2023.1110025
Received: 28 November 2022; Accepted: 30 January 2023;
Published: 21 February 2023.
Edited by:
Yu Wang, Guangzhou University, ChinaReviewed by:
Yao Chen, Chongqing Jiaotong University, ChinaCopyright © 2023 Xing, Li, Li, Lu and Li. This is an open-access article distributed under the terms of the Creative Commons Attribution License (CC BY). The use, distribution or reproduction in other forums is permitted, provided the original author(s) and the copyright owner(s) are credited and that the original publication in this journal is cited, in accordance with accepted academic practice. No use, distribution or reproduction is permitted which does not comply with these terms.
*Correspondence: Zhe Li, ✉ bGl6aGVAY2lnaXQuYWMuY24=
Disclaimer: All claims expressed in this article are solely those of the authors and do not necessarily represent those of their affiliated organizations, or those of the publisher, the editors and the reviewers. Any product that may be evaluated in this article or claim that may be made by its manufacturer is not guaranteed or endorsed by the publisher.
Research integrity at Frontiers
Learn more about the work of our research integrity team to safeguard the quality of each article we publish.