- 1Department of Respiratory Medicine, National Key Clinical Specialty, Branch of National Clinical Research Center for Respiratory Disease, Xiangya Hospital, Central South University, Changsha, China
- 2Center of Respiratory Medicine, Xiangya Hospital, Central South University, Changsha, China
- 3Hunan Engineering Research Center for Intelligent Diagnosis and Treatment of Respiratory Disease, Changsha, China
- 4National Clinical Research Center for Geriatric Disorders, Xiangya Hospital, Changsha, China
- 5Clinical Research Center for Respiratory Diseases in Hunan Province, Changsha, China
- 6Nosocomial Infection Control Center, Xiangya Hospital, Central South University, Changsha, China
- 7First Department of Thoracic Medicine, Hunan Cancer Hospital, The Affiliated Cancer Hospital of Xiangya School of Medicine, Central South University, Changsha, China
Background: Delayed diagnosis further increases the mortality of invasive candidiasis (IC) in intensive care unit (ICU) patients. This study aimed to develop and validate a score based on novel serological biomarkers and clinical risk factors for predicting IC in immunocompetent ICU patients.
Methods: We retrospectively collected clinical data and novel serological markers on admission to ICU. Multivariate logistic regression was used to identify the risk factors associated with IC, which were adopted to establish a scoring system.
Results: Patients with IC had a higher C-reactive protein-to-albumin ratio (CAR) and neutrophil-to-lymphocyte ratio (NLR) and lower prognostic nutritional index than those without IC. The NLR, CAR, sepsis, total parenteral nutrition, 1,3-β-D-glucan (BDG)-positivity, and Sequential Organ Failure Assessment score were identified as independent risk factors for IC by multivariate logistic regression analysis and entered into the final scoring system. The area under receiver operating characteristic curve of the score were 0.883 and 0.892, respectively, in the development and validation cohort, higher than Candida score (0.883 vs.0.730, p < 0.001).
Conclusion: We established a parsimonious score based on NLR, CAR, BDG-positivity, and clinical risk factors, which can accurately identify IC in ICU patients to give treatment on time and reduce mortality.
Introduction
Invasive candidiasis (IC) refers to a bloodstream (termed candidemia) and deep-seated infection with Candida spp. (Pappas et al., 2016; Bassetti et al., 2019). Candidemia is the second most common nosocomial bloodstream infection, with a mortality rate of 49% (McCarty et al., 2021). Approximately 1/3 of candidemia cases occur in the intensive care unit (ICU), and IC is the most common fungal disease in ICU patients (Logan et al., 2020). Patients admitted to the ICU have numerous risk factors significantly associated with IC, such as wide use of broad-spectrum antibiotics (Thomas-Ruddel et al., 2022), prolonged duration of corticosteroid use, post-aggressive immune paralysis (Jung et al., 2021), and higher utilization of intravenous catheters (Kullberg and Arendrup, 2015; Pappas et al., 2018). The overall mortality rate of ICU-associated IC is up to 55%, and has even been documented at 98% when septic shock develops (Kullberg and Arendrup, 2015). Previous studies and guidelines have mostly focused on immunodeficient patients, such as those with hematological malignancies and solid organ transplants (Donnelly et al., 2020). However, most patients in the ICU are immunocompetent at the onset of infection (Bassetti et al., 2021). Therefore, there is a lack of strong evidence to guide early recognition and timely initial antifungal therapy for IC among immunocompetent ICU patients.
Notably, delayed antifungal treatment can further increase the mortality of ICU-related IC, especially when not initiated 24–48 h after the first positive culture (Playford et al., 2016). Most ICU patients have hemodynamic instability, thrombocytopenia, or coagulation disorders, which may complicate tissue sampling. Furthermore, current pathogen detection methods for IC have limitations and cannot achieve timely and accurate diagnosis at the onset of infection. Culture-based techniques require more than 1–2 days to obtain results and have low sensitivity (Clancy and Nguyen, 2013). The most common non-culture-based method, testing for 1,3-β-D-glucan (BDG), has narrow specificity and sensitivity (Lamoth et al., 2012). Multiplex Candida real-time polymerase chain reaction (PCR) and metagenomics second-generation gene sequencing have great advantages in etiological diagnosis; however, they have some limitations, such as cost and the need for skilled personnel, and it is difficult to carry out routine etiological examinations (Bassetti et al., 2019). Therefore, the early recognition of IC in ICU patients is problematic. Hence, it is necessary and urgent to construct a tailored, prompt, and easy-to-use diagnostic clinical model.
Candida invades the human body and activates innate immune cells to produce many proinflammatory cytokines and chemokines (Pappas et al., 2018). Previous studies have found that plasma levels of inflammatory cytokines [Interleukin-1β (IL-1β), Tumor necrosis factor-α (TNF-α), and Interferon-γ (IFN-γ)] and clinical inflammatory markers [C-reactive protein (CRP) and neutrophils] are significantly increased in patients with IC (Chumpitazi et al., 2014; Akin et al., 2015; Wunsch et al., 2021). Furthermore, several previous studies have shown that patients with IC in the ICU are at a high risk of malnutrition, which is associated with poor clinical prognosis (Piazza et al., 2004; Lee et al., 2021). Therefore, patients with IC in the ICU exhibit extremely severe systemic inflammation and poor nutritional status. Novel serological markers derived from a combination of two or more laboratory indicators, such as the neutrophil-to-lymphocyte ratio (NLR), platelet-to-lymphocyte ratio (PLR), monocyte-to-lymphocyte ratio (MLR), CRP-to-albumin ratio (CAR), and systemic immune-inflammation index (SII), represent inflammation and immune response (Xue et al., 2020). The prognostic nutritional index (PNI) symbolizes the nutritional status of the patients (Xue et al., 2020). However, changes in these novel serological biomarkers and their clinical value in IC remain unclear. In this retrospective study, we aimed to evaluate the associations between novel serological biomarkers, disease severity, and prognosis and to develop a simple predictive scoring system based on clinical risk factors and new biomarkers for the early diagnosis of IC in ICU patients to guide timely initial antifungal therapy.
Methods
Study setting and patients enrollment
We retrospectively collected data on consecutive patients admitted to the ICU at Xiangya Hospital of Central South University from January 2018 to July 2022. The inclusion criteria were as follows: (1) age ≥ 18 years and (2) critical illness requiring intensive care. We excluded patients with an expected length of stay in the ICU of less than 48 h, pregnancy, fungal infections other than Candida species on admission, insufficient medical records and immunocompromised patients [a. transplant recipients (solid organ or hematopoietic stem cell transplant); b. hematological malignancies; c. neutropenia (peripheral absolute neutrophil count <500 cells/mm3); d. immunodeficiency (primary immunodeficiency or acquired immunodeficiency caused by immunosuppressive drugs, chemotherapy, and human immunodeficiency virus)]. A total of 1,841 ICU patients participated in the study, including 84 patients with IC. The flowchart of this retrospective study is shown in Figure 1. This study was approved by the Ethics Committee of the Xiangya Hospital, Central South University (No. 202104005). Due to the retrospective nature of the study and the anonymous processing of data prior to analysis, the Ethics Committee waived the requirement for informed consent. The study strictly complied with the Declaration of Helsinki.
Criteria for diagnosis
IC was diagnosed by experienced respiratory physicians according to the criteria outlined by the European Organization for Research on Treatment of Cancer and Mycology Study Group Education and Research Consortium (EORTC/MSGERC) (Bassetti et al., 2021) based on clinical manifestations consistent with IC, plus one of the following: (1) sterile tissue obtained by needle aspiration or biopsy showing budding cells consistent with Candida species by histopathology, cytopathology, or direct microscopic examination; (2) sterile specimens obtained through sterile procedures (such as pleural effusion, ascites, and cerebrospinal fluid) showing Candida spp.; or (3) positive peripheral blood culture for Candida. A high corticosteroid dose was defined as prednisone ≥20 mg/day (Kluge et al., 2021). A history of broad-spectrum antibiotics means that the patient has received antibiotics for more than 10 days in the last month against both gram-positive and gram-negative bacteria (Gu et al., 2021).
Clinical data collection
The baseline clinical characteristics and laboratory test results of each patient were extracted from the electronic medical system. A team of experienced respiratory clinicians independently collected, reviewed, and analyzed clinical data. Demographic and clinical characteristics at admission to the ICU included age, sex, smoking status, vital signs (body temperature, heart rate, respiratory rate, and blood pressure), oxygenation index, Sequential Organ Failure Assessment (SOFA score), comorbidities, laboratory test findings (routine blood test, liver and kidney function tests, BDG, and CRP). All baseline laboratory examinations were completed within 24 h after ICU admission. And other IC-associated risk factors include mechanical ventilation methods, vasopressors, continuous renal replacement therapies (CRRT), total parenteral nutrition (TPN), presence of urinary catheter or central venous catheter (CVC), and administration of a high dose of corticosteroid or a broad spectrum of antibiotic. The primary clinical outcome of this study was the 30-day mortality after ICU admission. Secondary outcomes included length of hospital stay, length of ICU stay, and hospitalization expenses.
New serological markers
These new serological markers are composed of two or more clinical laboratory indicators. The novel markers and their formulas are as follows (Li et al., 2021): (1) CAR = CRP(mg/L) /albumin(g/L), (2) NLR = neutrophil counts(109/L) /lymphocyte counts(109/L), (3) PNI = 10 × albumin(g/L) + 5 × lymphocyte count(109/L), (4) MLR = monocyte counts(109/L) /lymphocyte counts(109/L), (5) PLR = platelet counts(109/L) /lymphocyte counts(109/L), (6) SII = platelet count(109/L) × neutrophil count (109/L) /lymphocyte count (109/L).
Statistical analysis
Continuous variables were represented as mean ± standard deviation (SD) or median (range) and compared using Student’s t-test and Mann–Whitney U test. Categorical data were expressed as frequencies and percentages and compared using the chi-square test or Fisher’s exact test. The study population was randomly divided into development and validation cohorts at a ratio of 7:3. Variables demonstrating possible risk factors for IC were transformed from continuous variables into categorical variables using cut-off values generated from receiver operating characteristic (ROC) analysis. Significant variables in the univariate logistic regression analysis were entered into multivariate forward stepwise logistic regression analysis. Final significant indicators were included in the predictive scoring system. The weighed point of each variable was defined by the corresponding β coefficient, which was divided by the absolute value of the smallest β coefficient and rounded to the nearest integer. The area under the curve (AUC) was used to evaluate the performance of the scoring system. The calibration was checked by plotting the actual probabilities and model-predicted probabilities of the IC. The fitness of the model was calculated using the Hosmer–Lemeshow test (p > 0.05, good fitness). The aforementioned validation methodologies were conducted in both the development and validation cohorts. The optimal cutoff values were determined by calculating the Youden index. The sensitivity, specificity, positive predictive value (PPV), negative predictive value (NPV), positive likelihood ratio (LR+), and negative likelihood ratio (LR−) were calculated. All statistical tests were two-tailed, and differences were considered statistically significant at p < 0.05. Statistical analysis was performed using SPSS (version 26.0; SPSS Company, Chicago, IL, United States) and R Statistical Software 4.1.3 (R Foundation for Statistical Computing, Vienna, Austria). Figures were drawn using GraphPad Prism version 9.00 software and R Statistical Software 4.1.3.
Results
Demographic and clinical characteristics of the study population
A total of 1,841 ICU patients were enrolled in the study, including 1,289 in the development cohort and 552 in the validation cohort (Figure 1). The demographic and clinical characteristics of the two cohorts are shown in Supplementatry Table S1. There were no significant differences in the baseline clinical characteristics and novel serological marker levels between the two cohorts. The overall incidence of IC was 4.56% (84/1,841).
Patients in the IC group had higher rates of diabetes mellitus (DM), sepsis, and solid tumor than those in the non-IC group (Table 1; p < 0.05). The mean arterial pressure and oxygenation index were significantly lower in the IC group. Higher proportions of patients with IC received life-sustaining treatments, indwelling catheters, immunoglobulins, and broad-spectrum antibiotics than patients without IC (p < 0.05). With regard to disease severity, patients with IC had significantly higher SOFA scores than those without IC (p < 0.001). With reference to clinical outcomes, the 30-day mortality rate was significantly higher in the IC group than in the non-IC group (21.43% vs. 13.15%, p = 0.03). Furthermore, patients with IC had a longer hospital stay (14.5 vs. 9 days, p < 0.001) and ICU stay (10 vs. 7 days, p < 0.001) than those without IC. In addition, the hospitalization cost of patients with IC was significantly higher than that of patients without IC.
Novel serological biomarkers for the severity of disease and clinical outcomes
Figure 2 shows the differences in the novel serological biomarker levels between the IC and non-IC groups. CAR [4.08 (1.78–6.62) vs. 2.23 (0.55–4.36), p < 0.001] and NLR [14.50 (8.67–23.60) vs. 10.02 (5.58–18.50), p = 0.002] were significantly higher and PNI [272 (247–310) vs. 307 (272–346), p < 0.001] was much lower in the IC group than in the non-IC group. The MLR, PLR, and SII levels did not differ between the two groups. The total study population was divided into the survivor and non-survivor groups according to the 30-day mortality rates. Then we found CAR [3.78 (1.62–7.10) vs. 2.28 (0.55–3.96), p < 0.001], NLR [16.67 (9.00–28.00) vs. 9.40 (5.20–16.66), p < 0.001], MLR [0.89 (0.50–1.50) vs. 0.74 (0.45–1.13), p = 0.002], PLR [320.00 (162.50–538.00) vs. 257.50 (151.74–420.95), p = 0.003], and SII [2725.00 (1273.47–5382.55) vs. 1746.00 (868.65–3587.25), p < 0.001] were significantly higher in non-survivors than in survivors, while PNI [281.00 (254.97–312.50) vs. 311.00 (274.85–348.68), p < 0.001] was significantly lower in non-survivors than in survivors (Figure 3). Correlation analysis was performed to correlate the SOFA scores with novel biomarker levels. As shown in Figure 4, CAR, NLR, and MLR were positively correlated with SOFA scores (p < 0.001), and PNI and PLR were inversely correlated with SOFA scores (p < 0.001).
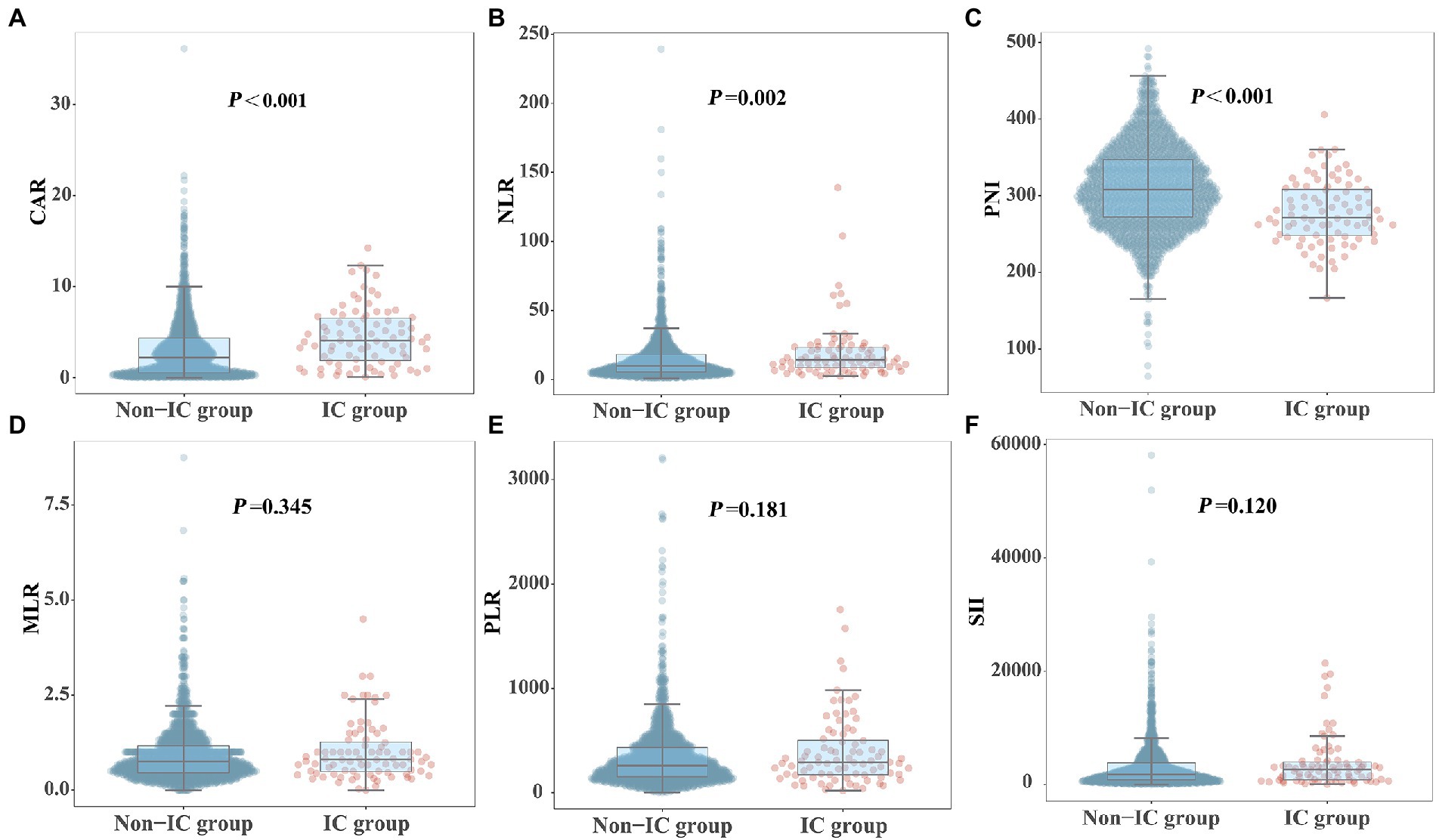
Figure 2. Comparison of novel serological biomarkers between IC group and non-IC group. (A) CAR, (B) NLR, (C) PNI, (D) MLR, (E) PLR, (F) SII. Data were presented as medians (IQR). Statistical significance was calculated by Mann–Whitney U test. P values indicated differences between patients in IC and non-IC group.
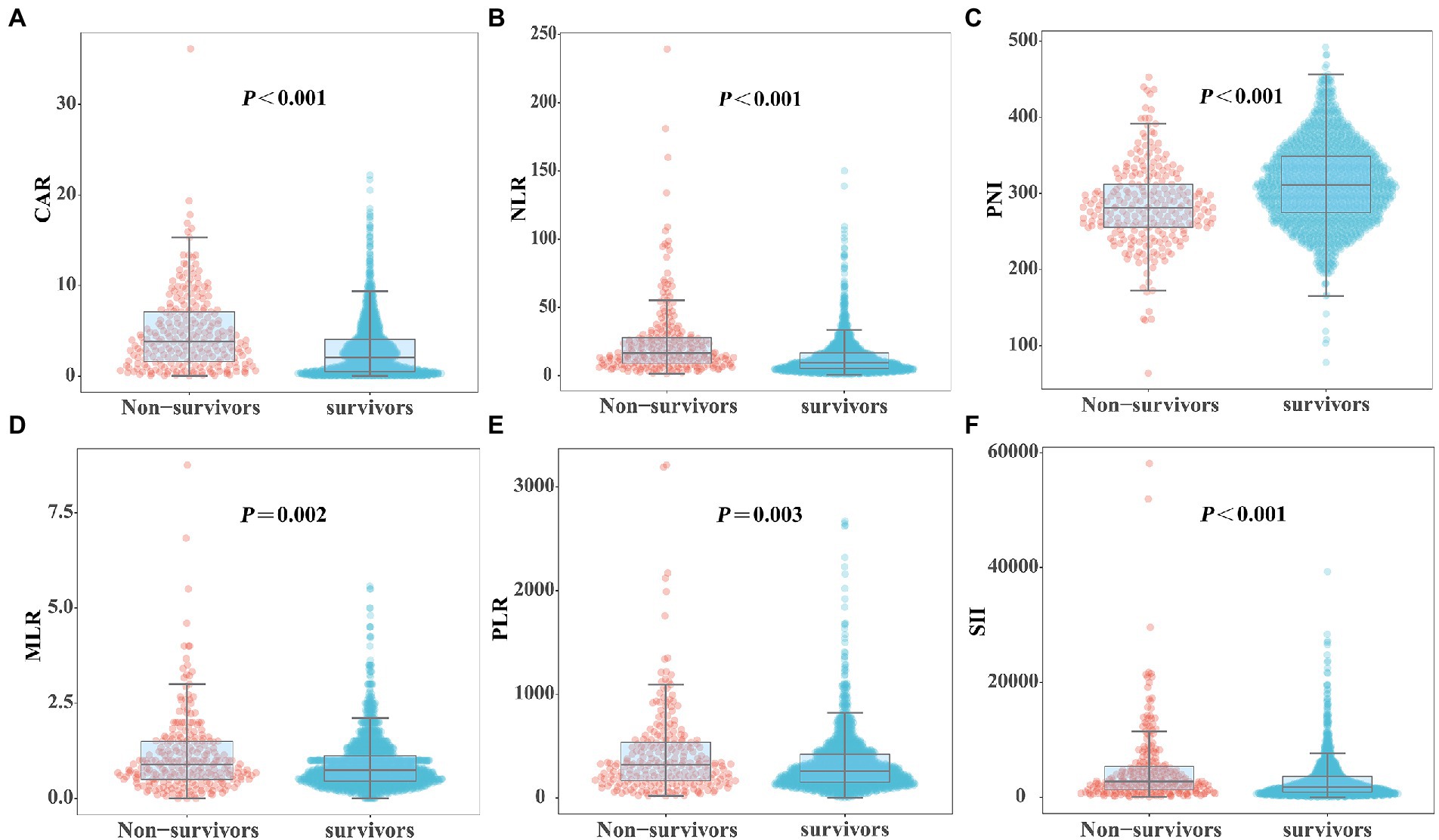
Figure 3. Comparison of novel serological biomarkers between survivors and non-survivors groups. (A) CAR, (B) NLR, (C) PNI, (D) MLR, (E) PLR, (F) SII. Data were presented as medians (IQR). Statistical significance was calculated by Mann–Whitney U test. p values indicated differences between patients in non-survivors and survivors group.
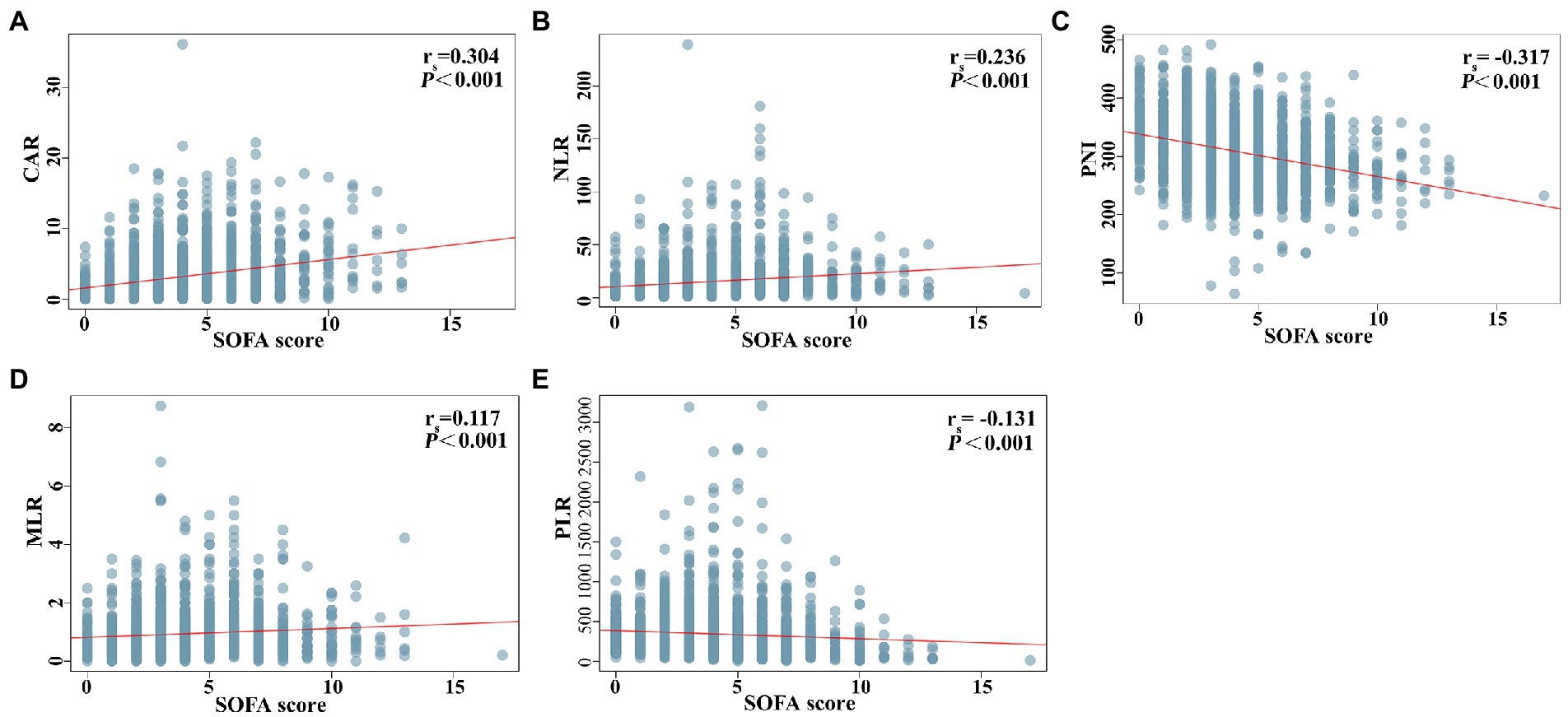
Figure 4. Correlation analysis between novel serological biomarkers and SOFA score. (A) CAR, (B) NLR, (C) PNI, (D) MLR, (E) PLR. Correlations between variables were analyzed with Spearman’s coefficients.
Development of the scoring system
The results of univariate and multivariate logistic regression analyzes in the development cohort are presented in Tables 2, 3. Variables demonstrating possible risk factors for IC were transformed from continuous variables into categorical variables using cut-off values generated from ROC analysis with the best Youden index. The best cutoff points for NLR, PNI, CAR, and SOFA scores were 8.65, 281.25, 4.31, and 4.5, respectively, for predicting IC. Thus, high NLR, low PNI, high CAR, and high SOFA scores were defined as ≥8.65, < 281.25, ≥4.31, and ≥ 4.5, respectively. Sixteen significant factors in the univariate analysis, including DM, sepsis, mean arterial pressure, oxygenation index, invasive mechanical ventilation, vasopressor, CRRT, TPN, urinary catheter, CVC, broad-spectrum antibiotics, SOFA score, BDG positivity, high NLR, low PNI, and high CAR, were included in the multivariate forward stepwise logistic regression analysis. Multivariate logistic regression analysis showed that sepsis (OR: 2.065, 95% CI: 1.073–3.973, p = 0.030), TPN (OR: 2.034, 95% CI: 1.015–4.078, p = 0.045), broad-spectrum antibiotics (OR: 3.376, 95% CI: 1.231–9.264, p = 0.018), BDG-positivity (OR: 14.320, 95% CI: 7.653–26.795, P < 0.001), high NLR (OR: 2.608, 95% CI: 1.212–5.609, p = 0.014), high CAR (OR: 1.952, 95% CI: 1.045–3.646, p = 0.036), and high SOFA score (OR: 1.922, 95% CI: 1.016–3.634, p = 0.045) were risk factors for IC in the final model. Then, we divided each β coefficient by the smallest β coefficient value and assigned the rounded integer to each variable, constructing a scoring system (Table 3).
Validation of the scoring system
The predictive scoring system performed well with an AUC of 0.883 (95% CI: 0.842–0.924, p < 0.001) in the development cohort and an AUC of 0.892 (95% CI, 0.826–0.958, p < 0.001) in the validation cohort (Figure 5). Moreover, the predictive power of the overall model was superior to that of a single variable in both the development and validation cohorts (Figure 5). The scoring system also showed good calibration in both the development and validation cohorts (Hosmer-Lemeshow p = 0.300 and 0.623, respectively), as depicted in the calibration plot (Figure 6). Furthermore, the estimated risk of IC was highly correlated with the observed risk (Spearman’s contingency coefficient = 0.723, p < 0.001).
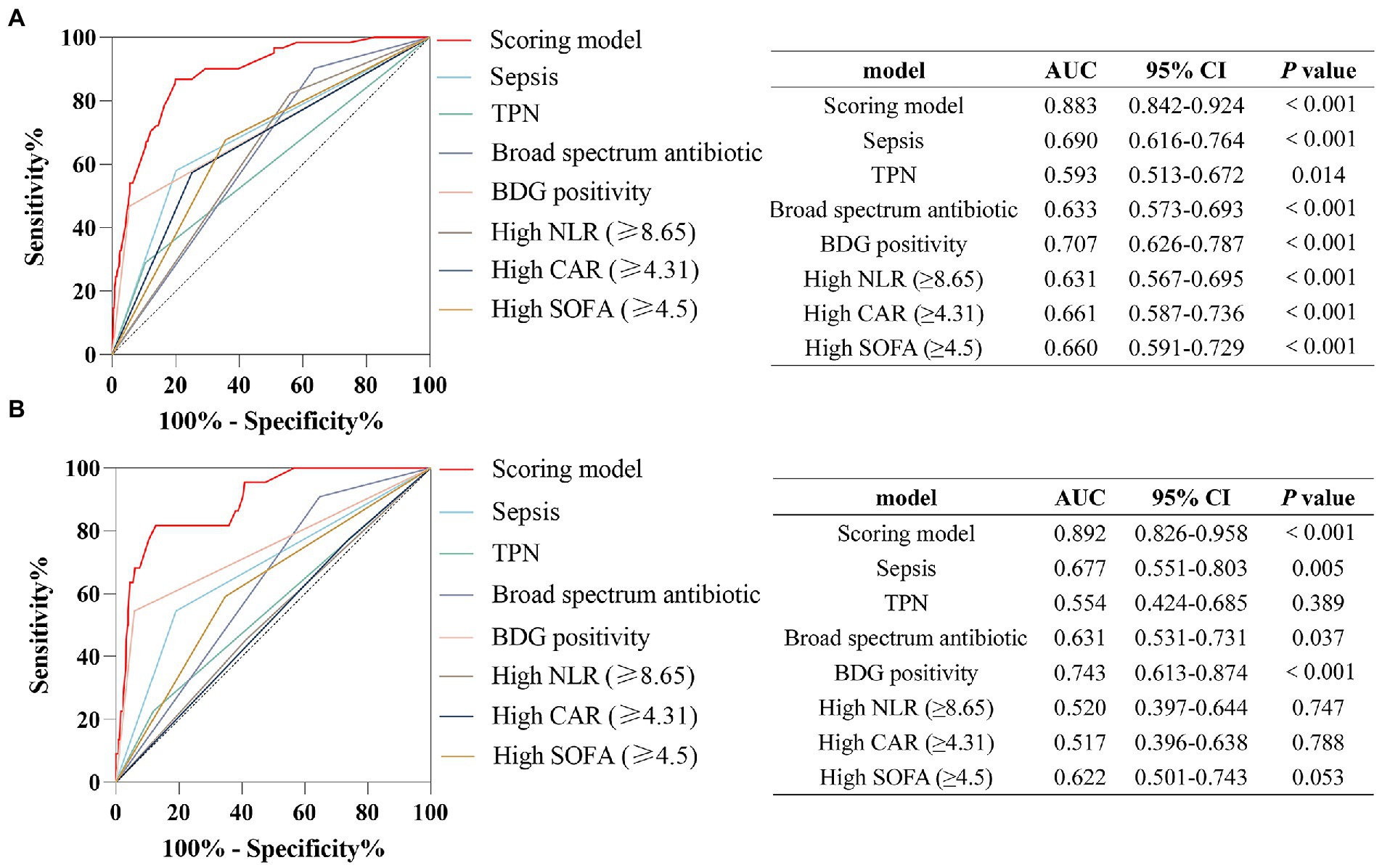
Figure 5. Receiver operating characteristic curves of the predictive model and single variables for the prediction of IC risk in (A) the development cohort, (B) the validation cohort.
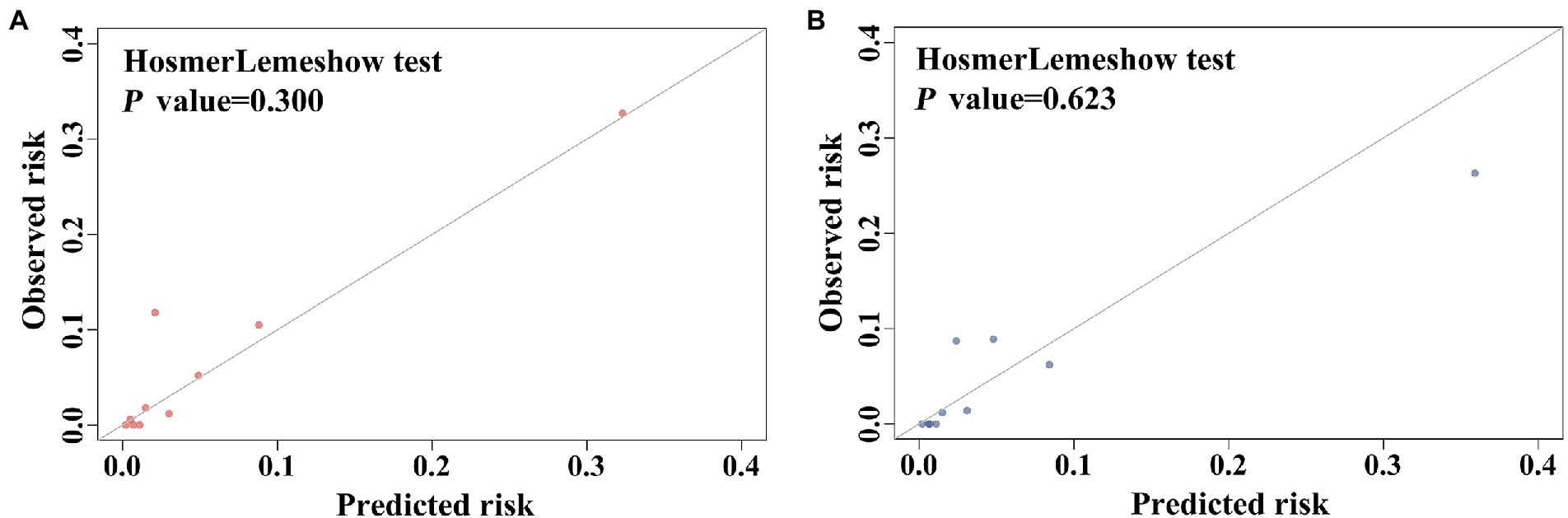
Figure 6. Calibration performance of the scoring model in (A) the development cohort, (B) the validation cohort.
Clinical performance of the scoring system
The patients were divided into low-(≤3 points), medium-(4–7 points), and high-risk (≥8 points) groups. In the development cohort, 725 cases were included in the low-risk group, with six developing IC (0.828%). A total of 452 cases were included in the medium-risk group, with 35 developing IC (7.743%). Fifty cases were included in the high-risk group, with 20 developing IC (40.000%). In the validation cohort, the low-risk group comprised 307 patients, with one developing IC (0.326%). The medium-risk group comprised 204 patients, with 14 developing IC (6.863%). The high-risk group comprised 27 patients, with seven developing IC (25.926%; Figure 7). Table 4 presents the detailed clinical implications of the scoring system for different score thresholds. According to the ROC analysis, the cut-off point of the scoring system was 4.5 points. The sensitivity and specificity for ≥4 points were 91.57 and 60.94%, respectively; the PPV and NPV were 10.37 and 99.32%, respectively, with an LR+ of 2.34 and LR− of 0.14. The sensitivity and specificity of ≥5 points were 83.13 and 78.40%, respectively; the PPV and NPV were 15.94 and 98.95%, respectively, with an LR+ of 3.84 and LR− of 0.22.
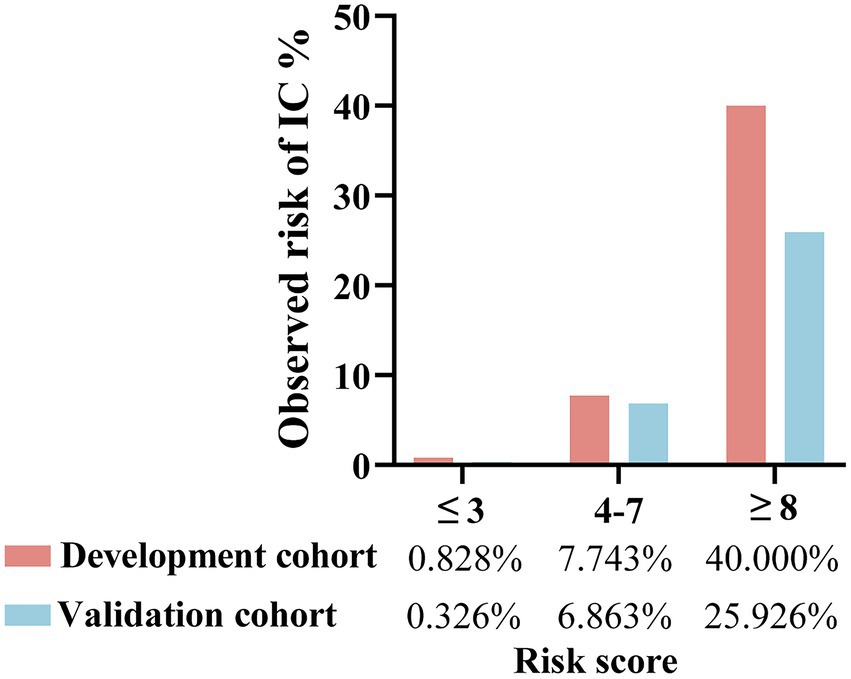
Figure 7. Risk groups according to risk score of the predictive scoring system and observed risk of IC in the development and validation cohorts. ≤3 points refers to low-risk group; 4–7 points refers to medium-risk group; ≥8 points refers to high-risk group.
Diagnostic value of the scoring system
Previous studies have shown that a high Candida score is associated with an increased risk of IC and is commonly used for diagnosing IC (Leon et al., 2009; Barantsevich and Barantsevich, 2022). We generated ROC curves to evaluate the diagnostic value of the Candida score and the predictive scoring system for the early diagnosis of IC among immunocompetent critically ill patients. The AUCs were 0.730 (95% CI: 0.667–0.792, p < 0.001) for the Candida score and 0.883 (95% CI: 0.842–0.924, p < 0.001) for the scoring system (Figure 8). As shown in Figure 8, the AUC of the scoring model was larger than that of the Candida score (0.883 vs. 0.730, z-statistic, 4.229, p < 0.001).
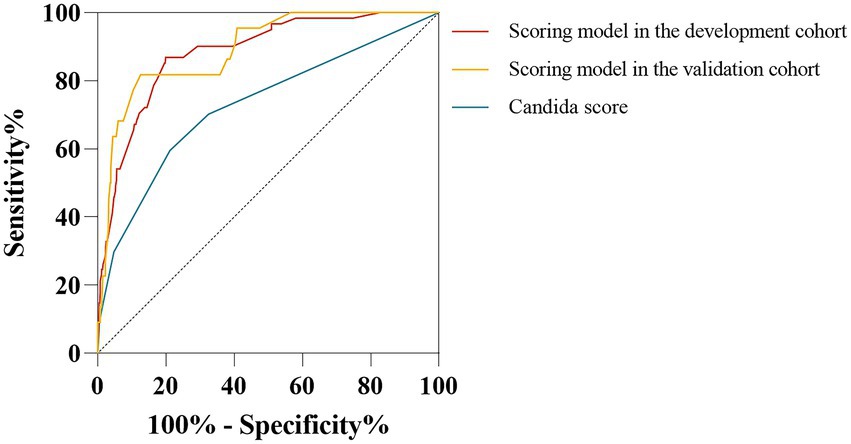
Figure 8. Comparison of receiver operating characteristic curves for IC risk between the scoring model and Candida score. The AUC of the scoring model was similar between the development cohort and validation cohort (0.883 vs. 0.892, p = 0.825). AUC of the scoring model was higher than the Candida score (0.883 vs. 0.730, Z statistic = 4.229, p < 0.001; 0.892 vs. 0.730, Z-statistic = 3.592, p < 0.001).
Discussion
At present, the guidelines and studies related to IC are mainly focused on people with immunodeficiency but less on immunocompetent populations (Donnelly et al., 2020). However majorities of critically ill patients are immunocompetent (Bassetti et al., 2021). Thus, early diagnosis of IC remains challenging among immunocompetent, critically ill patients, resulting in delayed antifungal treatment and increased mortality. Clinicians urgently need a tailored and prompt diagnostic method to recognize IC in ICU patients and instruct timely antifungal therapy. In this study, we found that new biomarkers, CAR, NLR, and PNI, were significantly altered in patients with IC. Furthermore, these biomarkers were significantly associated with disease severity and prognosis of ICU patients. Finally, we developed a scoring system for the early diagnosis of IC in immunocompetent patients in the ICU. The scoring system, based on sepsis, TPN, broad-spectrum antibiotics, high SOFA score, BDG positivity, high NLR, and high CAR, had a good identifiable value for IC, with an AUC of 0.883.
The burden of invasive fungal disease in ICU patients is increasing, of which 80% is caused by Candida species (Bassetti et al., 2017). In this study, the incidence of IC in immunocompetent ICU patients was 4.56% (84/1841), similar to previous studies (Zhang et al., 2022). The all-cause mortality of IC is reported to be approximately 49% (Kollef et al., 2012). However, the overall mortality rate of IC in our study population was 21.4% (18/84), which was lower than the published data. This may be related to the exclusion of immunodeficient patients in our study. In addition, we showed that patients with IC had a significantly higher disease severity score, 30-day mortality rate, hospitalization expenses, and longer hospital and ICU lengths of stay than those non-IC patients. Therefore, patients with IC have a higher mortality and socioeconomic burden.
Delayed antifungal treatments could further increase the mortality of ICU patients with IC (Martin-Loeches et al., 2019). Therefore, timely diagnosis of IC is crucial to guide targeted antifungal therapy and to improve the prognosis of ICU patients. Nevertheless, early diagnosis of IC in the ICU setting remains difficult. First, tissue biopsy, the gold standard for diagnosing IC, is difficult to accomplish in ICU patients because of their unstable vital signs and/or coagulation disorders. Second, noninvasive diagnostic methods have their respective limitations. For example, BDG is a cell wall component of Candida, which can be continuously released into blood in the process of IC (Clancy and Nguyen, 2018; Yoshida, 2021). So the detection of BDG is a common diagnostic method for IC. However, the detection methodologies are diverse, such as turbidimetry, colorimetry, or chemiluminescence immunoassay (Clancy and Nguyen, 2018; Yoshida, 2021). And colorimetry includes endpoint colorimetry and dynamic colorimetry, while turbidimetry also includes endpoint turbidimetry and dynamic turbidimetry. So the thresholds of BDG testing are different, ranging from 11 pg./ml to 95 pg./ml, making it difficult to reach a consensus (White et al., 2019; Bamba et al., 2021; Lamoth et al., 2021; Lass-Florl et al., 2021). Additionally, BDG detection has a high false-positive rate (Karageorgopoulos et al., 2011; Haydour et al., 2019) and can be influenced by blood product perfusion, Candida spp. colonization, and disturbed GI mucosa (Logan et al., 2020).
Critically ill IC patients exhibit extremely severe systemic inflammation and poor nutritional status. Novel markers derived from a combination of two or more laboratory indicators, such as NLR, PLR, MLR, CAR, and SII, represent inflammation and immune responses (Xue et al., 2020). Meanwhile, PNI symbolizes the nutritional status (Xue et al., 2020). Higher levels of NLR, PLR, MLR, CAR, and SII and lower levels of PNI are correlated with the severity of various diseases, such as COVID-19, cancer, and rheumatic diseases (Gasparyan et al., 2019; Fois et al., 2020; Huang et al., 2020; Nost et al., 2021). In our study, we found that NLR and CAR were higher in patients with IC, whereas PNI was lower. Correlation analysis showed that CAR, NLR, and MLR were positively correlated with SOFA scores, while PNI and PLR were reversed. Furthermore, CAR, NLR, MLR, PLR, and SII were higher and PNI was lower in the non-survivor group. Therefore, our study revealed that these new biomarkers are closely related to IC, and the disease severity and clinical outcomes of ICU patients. In addition, multivariate logistic regression analysis showed that high NLR (OR: 2.608, 95% CI: 1.212–5.609, p = 0.014) and high CAR (OR: 1.952, 95% CI: 1.045–3.646, p = 0.036) were independent risk factors for IC. Therefore, NLR and CAR may be potential biomarkers for IC.
The most common mycosis in ICU patients is IC (Pappas et al., 2018). The risk factors for IC are closely related to the patient’s physical condition, disease, and iatrogenic treatment. At present, common risk factors for IC include long-term use of corticosteroids, broad-spectrum antibiotics, TPN, indwelling CVC, any type of dialysis, invasive mechanical ventilation, and surgery (Pappas et al., 2018; Martin-Loeches et al., 2019). ICU patients are more likely to be exposed to these risk factors, which leads to a higher incidence of IC in ICU patients. Our study also demonstrated that TPN and broad-spectrum antibiotic were independent risk factors for IC. As for TPN, lipid and glucose contained in nutrient solutions could propagate biofilm formation of Candida spp. (Kuwahara et al., 2010). Furthermore, blocked intestinal peristalsis leads to changes in the intestinal epithelial barrier function and promotes the translocation of intestinally attached Candida into the bloodstream (Pierre, 2017). Broad-spectrum antibiotics would increase the colonization rate of Candida spp. and change the interaction between bacteria and fungi, leading to enhanced pathogenicity of Candida (Guinan et al., 2019). In addtion, our study found that sepsis is a risk factor for IC. Previous studies have suggested that patients with sepsis are more likely to receive long-term antibiotics and invasive medical devices, which are definite risk factors for IC (Meng et al., 2017; Wang et al., 2021). Moreover, sepsis induces immune paralysis, predisposing the host to Candida infection (Jung et al., 2021).
Based on traditional BDG, combined biomarker NLR, CAR, and classical clinical risk factors (sepsis, TPN, broad-spectrum antibiotic, and SOFA), we established a bedside risk-scoring model for predicting the risk of IC. The model performed excellently with an AUC of 0.883 in the development cohort and was well validated in the validation cohort (AUC = 0.892). The estimated score was highly positively related to the actual risk of IC. Furthermore, the discriminative value of our scoring model was higher than that of the Candida scoring system. The above parameters included in the model are available, economical, and reliable in clinical practice and are usually included in routine admission examinations among ICU patients. Thus, this scoring model can be extensively used, even in grassroots hospitals. Therefore, it is convenient for physicians to evaluate the risk of IC for patients at the bedside.
However, our study has some limitations. First, this was a single-center retrospective study; therefore, we lacked another hospital validation cohort to validate our scoring system. However, the validation in our study exhibited a satisfactory AUC and calibration in both the development and validation cohorts, even with a higher discriminative value than the Candida score. Second, although the scoring system can early and accurately diagnose IC among ICU patients, the clinical benefits of the clinical management strategy based on our scoring system need to be confirmed. In the future, a larger prospective cohort involving multicenter hospitals is needed to evaluate the clinical performance of our scoring system.
Conclusion
In conclusion, our results demonstrated that NLR, CAR, and PNI were significantly associated with IC and disease severity in immunocompetent critically ill patients. We established a parsimonious score based on NLR, CAR, clinical risk factors (sepsis, TPN, broad-spectrum antibiotic), SOFA, and BDG, which can diagnose IC in immunocompetent ICU patients early and accurately to guide clinical physicians to initiate antifungal therapy at an early stage and improve the prognosis of IC.
Data availability statement
The raw data supporting the conclusions of this article will be made available by the authors, without undue reservation.
Ethics statement
The studies involving human participants were reviewed and approved by the Ethics Committee of the Xiangya Hospital, Central South University (No. 202104005). Written informed consent for participation was not required for this study in accordance with the national legislation and the institutional requirements.
Author contributions
YL and PP conceived, supervised the study and approved the final version of manuscript. YL revised the manuscript. PP provided funds. WL and GC analyzed data, prepared figures, tables and drafted the manuscript. FL and HY provided codes for statistical analysis. YC, RL, CS, and HL collected data. All authors read and approved the final manuscript.
Funding
This study was supported by Key R&D Program of Hunan Province (No. 2022SK2038), Project Program of National Clinical Research Center for Geriatric Disorders (Xiangya Hospital, Grant No. 2020LNJJ05), National Natural Science Foundation of China (No. 82000089, No. 82100100 and No. 82200099), Hunan Natural Science Youth Foundation (No.2022JJ40810 and No. 2022JJ40775), The National Key Clinical Specialist Construction Programs of China (No. z047-02), Scientific research project of Hunan Health Commission (No. 202103020612) and Science and Technology Projects in Guangzhou (No. 202201010012).
Acknowledgments
We thank all the patients and their families involved in this study. We are gratefully acknowleage the medical staff of Xiangya Hospital, Central South University. We also appreciate the reviewers for their helpful comments on this paper.
Conflict of interest
The authors declare that the research was conducted in the absence of any commercial or financial relationships that could be construed as a potential conflict of interest.
Publisher’s note
All claims expressed in this article are solely those of the authors and do not necessarily represent those of their affiliated organizations, or those of the publisher, the editors and the reviewers. Any product that may be evaluated in this article, or claim that may be made by its manufacturer, is not guaranteed or endorsed by the publisher.
Supplementary material
The Supplementary material for this article can be found online at: https://www.frontiersin.org/articles/10.3389/fmicb.2023.1097574/full#supplementary-material
References
Akin, H., Akalin, H., Budak, F., Ener, B., Ocakoğlu, G., Gürcüoğlu, E., et al. (2015). Alterations of serum cytokine levels and their relation with inflammatory markers in candidemia. Med. Mycol. 53, 258–268. doi: 10.1093/mmy/myu084
Bamba, Y., Nagano, K., Moro, H., Ogata, H., Hakamata, M., Shibata, S., et al. (2021). Efficacy of the new beta-D-glucan measurement kit for diagnosing invasive fungal infections, as compared with that of four conventional kits. PLoS One 16:e255172. doi: 10.1371/journal.pone.0255172
Barantsevich, N., and Barantsevich, E. (2022). Diagnosis and treatment of invasive candidiasis. Antibiotics (Basel) 11:718. doi: 10.3390/antibiotics11060718
Bassetti, M., Azoulay, E., Kullberg, B. J., Ruhnke, M., Shoham, S., Vazquez, J., et al. (2021). EORTC/MSGERC definitions of invasive fungal diseases: summary of activities of the intensive care unit working group. Clin. Infect. Dis. 72, S121–S127. doi: 10.1093/cid/ciaa1751
Bassetti, M., Garnacho-Montero, J., Calandra, T., Kullberg, B., Dimopoulos, G., Azoulay, E., et al. (2017). Intensive care medicine research agenda on invasive fungal infection in critically ill patients. Intensive Care Med. 43, 1225–1238. doi: 10.1007/s00134-017-4731-2
Bassetti, M., Giacobbe, D. R., Vena, A., and Wolff, M. (2019). Diagnosis and treatment of Candidemia in the intensive care unit. Semin. Respir. Crit. Care Med. 40, 524–539. doi: 10.1055/s-0039-1693704
Chumpitazi, B. F., Lebeau, B., Faure-Cognet, O., Hamidfar-Roy, R., Timsit, J. F., Pavese, P., et al. (2014). Characteristic and clinical relevance of Candida mannan test in the diagnosis of probable invasive candidiasis. Med. Mycol. 52, 462–471. doi: 10.1093/mmy/myu018
Clancy, C. J., and Nguyen, M. H. (2013). Finding the "missing 50%" of invasive candidiasis: how nonculture diagnostics will improve understanding of disease spectrum and transform patient care. Clin. Infect. Dis. 56, 1284–1292. doi: 10.1093/cid/cit006
Clancy, C. J., and Nguyen, M. H. (2018). Diagnosing Invasive Candidiasis. J. Clin. Microbiol. 56:e01909. doi: 10.1128/JCM.01909-17
Donnelly, J. P., Chen, S. C., Kauffman, C. A., Steinbach, W. J., Baddley, J. W., Verweij, P. E., et al. (2020). Revision and update of the consensus definitions of invasive fungal disease from the European Organization for Research and Treatment of cancer and the mycoses study group education and research consortium. Clin. Infect. Dis. 71, 1367–1376. doi: 10.1093/cid/ciz1008
Fois, A. G., Paliogiannis, P., Scano, V., Cau, S., Babudieri, S., Perra, R., et al. (2020). The systemic inflammation index on admission predicts in-hospital mortality in COVID-19 patients. Molecules 25:5725. doi: 10.3390/molecules25235725
Gasparyan, A. Y., Ayvazyan, L., Mukanova, U., Yessirkepov, M., and Kitas, G. D. (2019). The platelet-to-lymphocyte ratio as an inflammatory marker in rheumatic diseases. Ann. Lab. Med. 39, 345–357. doi: 10.3343/alm.2019.39.4.345
Gu, Y., Ye, X., Liu, Y., Wang, Y., Shen, K., Zhong, J., et al. (2021). A risk-predictive model for invasive pulmonary aspergillosis in patients with acute exacerbation of chronic obstructive pulmonary disease. Respir. Res. 22:176. doi: 10.1186/s12931-021-01771-3
Guinan, J., Wang, S., Hazbun, T. R., Yadav, H., and Thangamani, S. (2019). Antibiotic-induced decreases in the levels of microbial-derived short-chain fatty acids correlate with increased gastrointestinal colonization of Candida albicans. Sci. Rep. 9:8872. doi: 10.1038/s41598-019-45467-7
Haydour, Q., Hage, C. A., Carmona, E. M., Epelbaum, O., Evans, S. E., Gabe, L. M., et al. (2019). Diagnosis of Fungal Infections. A Systematic Review and Meta-Analysis Supporting American Thoracic Society Practice Guideline. Ann. Am. Thorac. Soc. 16, 1179–1188. doi: 10.1513/AnnalsATS.201811-766OC
Huang, Z., Fu, Z., Huang, W., and Huang, K. (2020). Prognostic value of neutrophil-to-lymphocyte ratio in sepsis: a meta-analysis. Am. J. Emerg. Med. 38, 641–647. doi: 10.1016/j.ajem.2019.10.023
Jung, B., Le Bihan, C., Portales, P., Bourgeois, N., Vincent, T., Lachaud, L., et al. (2021). Monocyte human leukocyte antigen-DR but not beta-D-glucan may help early diagnosing invasive Candida infection in critically ill patients. Ann. Intensive Care 11:129. doi: 10.1186/s13613-021-00918-1
Karageorgopoulos, D. E., Vouloumanou, E. K., Ntziora, F., Michalopoulos, A., Rafailidis, P. I., and Falagas, M. E. (2011). β-D-glucan assay for the diagnosis of invasive fungal infections: a meta-analysis. Clin. Infect. Dis. 52, 750–770. doi: 10.1093/cid/ciq206
Kluge, S., Strauss, R., Kochanek, M., Weigand, M. A., Rohde, H., and Lahmer, T. (2021). Aspergillosis: emerging risk groups in critically ill patients. Med. Mycol. 60:myab064. doi: 10.1093/mmy/myab064
Kollef, M., Micek, S., Hampton, N., Doherty, J. A., and Kumar, A. (2012). Septic shock attributed to Candida infection: importance of empiric therapy and source control. Clin. Infect. Dis. 54, 1739–1746. doi: 10.1093/cid/cis305
Kullberg, B. J., and Arendrup, M. C. (2015). Invasive Candidiasis. N. Engl. J. Med. 373, 1445–1456. doi: 10.1056/NEJMra1315399
Kuwahara, T., Shimono, K., Kaneda, S., Tamura, T., Ichihara, M., and Nakashima, Y. (2010). Growth of microorganisms in total parenteral nutrition solutions containing lipid. Int. J. Med. Sci. 7, 101–109. doi: 10.7150/ijms.7.101
Lamoth, F., Akan, H., Andes, D., Cruciani, M., Marchetti, O., Ostrosky-Zeichner, L., et al. (2021). Assessment of the role of 1,3-beta-d-glucan testing for the diagnosis of invasive fungal infections in adults. Clin. Infect. Dis. 72, S102–S108. doi: 10.1093/cid/ciaa1943
Lamoth, F., Cruciani, M., Mengoli, C., Castagnola, E., Lortholary, O., Richardson, M., et al. (2012). Beta-glucan antigenemia assay for the diagnosis of invasive fungal infections in patients with hematological malignancies: a systematic review and meta-analysis of cohort studies from the third European conference on infections in leukemia (ECIL-3). Clin. Infect. Dis. 54, 633–643. doi: 10.1093/cid/cir897
Lass-Florl, C., Samardzic, E., and Knoll, M. (2021). Serology anno 2021-fungal infections: from invasive to chronic. Clin. Microbiol. Infect. 27, 1230–1241. doi: 10.1016/j.cmi.2021.02.005
Lee, Y. C., Chen, Y. C., Wang, J. T., Wang, F. D., Hsieh, M. H., Hii, I. M., et al. (2021). Impact of nutritional assessment on the clinical outcomes of patients with non-albicans Candidemia: a multicenter study. Nutrients 13:3218. doi: 10.3390/nu13093218
Leon, C., Ruiz-Santana, S., Saavedra, P., Galvan, B., Blanco, A., Castro, C., et al. (2009). Usefulness of the "Candida score" for discriminating between Candida colonization and invasive candidiasis in non-neutropenic critically ill patients: a prospective multicenter study. Crit. Care Med. 37, 1624–1633. doi: 10.1097/CCM.0b013e31819daa14
Li, Y., Li, H., Song, C., Lu, R., Zhao, Y., Lin, F., et al. (2021). Early prediction of disease progression in patients with severe COVID-19 using C-reactive protein to albumin ratio. Dis. Markers 2021:6304189. doi: 10.1155/2021/6304189
Logan, C., Martin-Loeches, I., and Bicanic, T. (2020). Invasive candidiasis in critical care: challenges and future directions. Intensive Care Med. 46, 2001–2014. doi: 10.1007/s00134-020-06240-x
Martin-Loeches, I., Antonelli, M., Cuenca-Estrella, M., Dimopoulos, G., Einav, S., De Waele, J. J., et al. (2019). ESICM/ESCMID task force on practical management of invasive candidiasis in critically ill patients. Intensive Care Med. 45, 789–805. doi: 10.1007/s00134-019-05599-w
McCarty, T. P., White, C. M., and Pappas, P. G. (2021). Candidemia and invasive candidiasis. Infect. Dis. Clin. N. Am. 35, 389–413. doi: 10.1016/j.idc.2021.03.007
Meng, M., Klingensmith, N. J., and Coopersmith, C. M. (2017). New insights into the gut as the driver of critical illness and organ failure. Curr. Opin. Crit. Care 23, 143–148. doi: 10.1097/MCC.0000000000000386
Nost, T. H., Alcala, K., Urbarova, I., Byrne, K. S., Guida, F., Sandanger, T. M., et al. (2021). Systemic inflammation markers and cancer incidence in the UK biobank. Eur. J. Epidemiol. 36, 841–848. doi: 10.1007/s10654-021-00752-6
Pappas, P. G., Kauffman, C. A., Andes, D. R., Clancy, C. J., Marr, K. A., Ostrosky-Zeichner, L., et al. (2016). Clinical practice guideline for the Management of Candidiasis: 2016 update by the Infectious Diseases Society of America. Clin. Infect. Dis. 62, e1–e50. doi: 10.1093/cid/civ933
Pappas, P. G., Lionakis, M. S., Arendrup, M. C., Ostrosky-Zeichner, L., and Kullberg, B. J. (2018). Invasive candidiasis. Nat. Rev. Dis. Primers. 4:18026. doi: 10.1038/nrdp.2018.26
Piazza, O., Boccia, M. C., Iasiello, A., Storti, M. P., Tufano, R., and Triassi, M. (2004). Candidemia in intensive care patients. Risk factors and mortality. Minerva Anestesiol. 70, 63–69.
Pierre, J. F. (2017). Gastrointestinal immune and microbiome changes during parenteral nutrition. Am. J. Physiol. Gastrointest. Liver Physiol. 312, G246–G256. doi: 10.1152/ajpgi.00321.2016
Playford, E. G., Lipman, J., Jones, M., Lau, A. F., Kabir, M., Chen, S. C., et al. (2016). Problematic dichotomization of risk for intensive care unit (ICU)-acquired invasive candidiasis: results using a risk-predictive model to categorize 3 levels of risk from a multicenter prospective cohort of Australian ICU patients. Clin. Infect. Dis. 63, 1463–1469. doi: 10.1093/cid/ciw610
Thomas-Ruddel, D. O., Schlattmann, P., Pletz, M., Kurzai, O., and Bloos, F. (2022). Risk factors for invasive Candida infection in critically ill patients: a systematic review and meta-analysis. Chest 161, 345–355. doi: 10.1016/j.chest.2021.08.081
Wang, Y., Zhang, Y., McGuire, T. M., Hollingworth, S. A., Van Driel, M. L., Cao, L., et al. (2021). ICU Patients' antibiotic exposure and Triazole-resistance in invasive candidiasis: parallel analysis of aggregated and individual data. Front. Pharmacol. 12:586893. doi: 10.3389/fphar.2021.586893
White, S. K., Walker, B. S., Hanson, K. E., and Schmidt, R. L. (2019). Diagnostic accuracy of beta-d-glucan (Fungitell) testing among patients with hematologic malignancies or solid organ tumors: a systematic review and meta-analysis. Am. J. Clin. Pathol. 151, 275–285. doi: 10.1093/ajcp/aqy135
Wunsch, S., Zurl, C., Strohmaier, H., Meinitzer, A., Rabensteiner, J., Posch, W., et al. (2021). Longitudinal evaluation of plasma cytokine levels in patients with invasive candidiasis. J Fungi (Basel) 7:101. doi: 10.3390/jof7020101
Xue, G., Gan, X., Wu, Z., Xie, D., Xiong, Y., Hua, L., et al. (2020). Novel serological biomarkers for inflammation in predicting disease severity in patients with COVID-19. Int. Immunopharmacol. 89:107065. doi: 10.1016/j.intimp.2020.107065
Yoshida, M. (2021). Development of a method of measuring beta-D-glucan and its use in preemptive therapy for invasive fungal infections. Int. J. Mol. Sci. 22:9265. doi: 10.3390/ijms22179265
Zhang, J., Cheng, W., Li, D., Chen, J., Zhao, G., Wang, H., et al. (2022). Development and validation of a risk score for predicting invasive candidiasis in intensive care unit patients by incorporating clinical risk factors and lymphocyte subtyping. Front. Cell. Infect. Microbiol. 12:829066. doi: 10.3389/fcimb.2022.829066
Keywords: invasive candidiasis, scoring system, immunocompetent, novel serological biomarkers, critically ill patients
Citation: Li W, Chen G, Lin F, Yang H, Cui Y, Lu R, Song C, Li H, Li Y and Pan P (2023) A scoring system based on novel biomarkers and clinical risk factors to predict invasive candidiasis in immunocompetent critically ill patients. Front. Microbiol. 14:1097574. doi: 10.3389/fmicb.2023.1097574
Edited by:
Sameh S. M. Soliman, University of Sharjah, United Arab EmiratesReviewed by:
Zarrin Basharat, Independent Researcher, Islamabad, PakistanFrancesco Giuseppe De Rosa, University of Turin, Italy
Copyright © 2023 Li, Chen, Lin, Yang, Cui, Lu, Song, Li, Li and Pan. This is an open-access article distributed under the terms of the Creative Commons Attribution License (CC BY). The use, distribution or reproduction in other forums is permitted, provided the original author(s) and the copyright owner(s) are credited and that the original publication in this journal is cited, in accordance with accepted academic practice. No use, distribution or reproduction is permitted which does not comply with these terms.
*Correspondence: Pinhua Pan, cGluaHVhcGFuNjY4QGNzdS5lZHUuY24=; Yi Li, bGl5aXd4MjAxMEBjc3UuZWR1LmNu
†These authors have contributed equally to this work