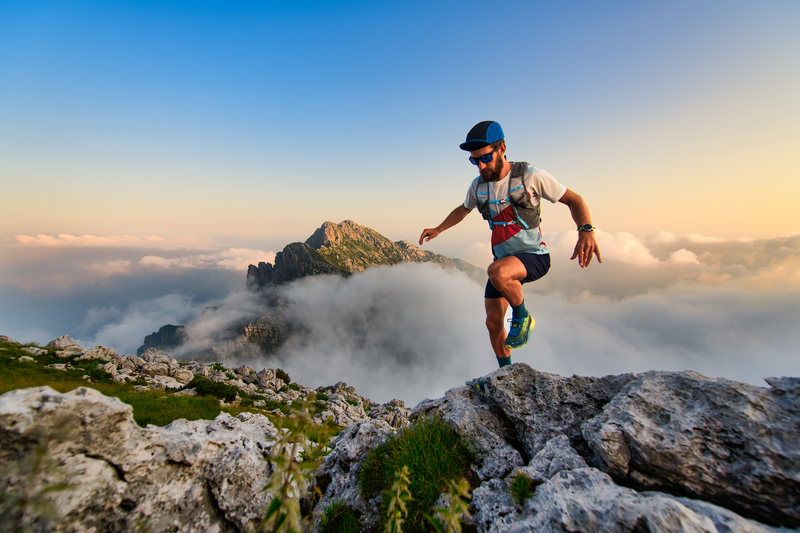
94% of researchers rate our articles as excellent or good
Learn more about the work of our research integrity team to safeguard the quality of each article we publish.
Find out more
ORIGINAL RESEARCH article
Front. Microbiol. , 24 March 2023
Sec. Microbe and Virus Interactions with Plants
Volume 14 - 2023 | https://doi.org/10.3389/fmicb.2023.1076522
This article is part of the Research Topic Plant Disease Management in the Post-Genomic Era: from Functional Genomics to Genome Editing, Volume II View all 9 articles
Introduction: Oomycetes cause several damaging diseases of plants and animals, and some species also act as biocontrol agents on insects, fungi, and other oomycetes. RNA silencing is increasingly being shown to play a role in the pathogenicity of Phytophthora species, either through trans-boundary movement of small RNAs (sRNAs) or through expression regulation of infection promoting effectors.
Methods: To gain a wider understanding of RNA silencing in oomycete species with more diverse hosts, we mined genome assemblies for Dicer-like (DCL), Argonaute (AGO), and RNA dependent RNA polymerase (RDRP) proteins from Phytophthora plurivora, Ph. cactorum, Ph. colocasiae, Pythium oligandrum, Py. periplocum, and Lagenidium giganteum. Moreover, we sequenced small RNAs from the mycelium stage in each of these species.
Results and discussion: Each of the species possessed a single DCL protein, but they differed in the number and sequence of AGOs and RDRPs. SRNAs of 21nt, 25nt, and 26nt were prevalent in all oomycetes analyzed, but the relative abundance and 5’ base preference of these classes differed markedly between genera. Most sRNAs mapped to transposons and other repeats, signifying that the major role for RNA silencing in oomycetes is to limit the expansion of these elements. We also found that sRNAs may act to regulate the expression of duplicated genes. Other sRNAs mapped to several gene families, and this number was higher in Pythium spp., suggesting a role of RNA silencing in regulating gene expression. Genes for most effector classes were the source of sRNAs of variable size, but some gene families showed a preference for specific classes of sRNAs, such as 25/26 nt sRNAs targeting RxLR effector genes in Phytophthora species. Novel miRNA-like RNAs (milRNAs) were discovered in all species, and two were predicted to target transcripts for RxLR effectors in Ph. plurivora and Ph. cactorum, indicating a putative role in regulating infection. Moreover, milRNAs from the biocontrol Pythium species had matches in the predicted transcriptome of Phytophthora infestans and Botrytis cinerea, and L. giganteum milRNAs matched candidate genes in the mosquito Aedes aegypti. This suggests that trans-boundary RNA silencing may have a role in the biocontrol action of these oomycetes.
RNA silencing is a form of gene regulation mediated by non-coding small RNAs (sRNAs), usually 18–32 nt in length (Hannon, 2002; Huang et al., 2019). RNA silencing is a conserved biological process involving three protein classes: Dicers or Dicer-like enzymes (DCLs), Argonaute proteins (AGOs) and RNA-dependent RNA polymerases (RDRPs). DCLs cleave long double stranded RNA (dsRNA) precursors (Bartel, 2009), producing double stranded sRNAs which are then unwound and loaded onto a protein complex called the RNA-induced silencing complex (RISC), where AGOs mediate the recognition of sequences complementary to sRNAs (Ma et al., 2018). Targeted mRNAs are cleaved by AGOs and degraded, or their translation is inhibited. RDRPs can amplify the silencing signal by driving production of additional sRNAs from the fragments of cleaved transcripts (Zong et al., 2009; Maniar and Fire, 2011). In some organisms, specific classes of AGOs can mediate the silencing of sRNA-complementary DNA sequences through methylation and other chromatin modifications (Holoch and Moazed, 2015).
Oomycetes are a group of organisms phenotypically similar to filamentous fungi but phylogenetically close to diatoms and brown algae (Gunderson et al., 1987; Thines, 2014). Many oomycetes are plant pathogens (Kamoun et al., 2015) and the most impactful of them is Phytophthora infestans, causal agent of potato late blight. This disease was the cause of the Irish famine in the nineteenth century and it continues to threaten potato production today (Haverkort et al., 2008). The genus Phytophthora also includes other pathogenic species, such as Phytophthora cactorum causing leather rot and crown rot on strawberries (Eikemo and Stensvand, 2015), Phytophthora colocasiae causing leaf blight of taro (Shakywar et al., 2013) and Phytophthora plurivora affecting roots in beech, oak and rhododendron (Jung et al., 2000; Jung, 2009; Lilja et al., 2011). Some oomycetes are also pathogens of animals, causing diseases on fish, crustaceans, and mammals (Phillips et al., 2008; Derevnina et al., 2016). Apart from the pathogens, certain oomycete species can be used as biocontrol agents (BCAs) against insects and plant pathogens. For example, Pythium oligandrum and Pythium periplocum can protect plants from fungal and oomycete pathogens and promote plant growth and fitness (Paul, 1999; Bělonožníková et al., 2022), while Lagenidium giganteum is a known parasite of the larvae of several mosquito species (Kerwin, 2007).
The genes involved in the RNA silencing mechanism have been identified in Phytophthora and their role in gene expression regulation was demonstrated (Vetukuri et al., 2011, 2012; Fahlgren et al., 2013; Qutob et al., 2013). Each of the Phytophthora spp. analysed to date possesses one DCL protein, at least four AGO proteins, and a single RDRP. Ph. infestans possesses five AGO proteins, divided into two clades (Vetukuri et al., 2011). Fahlgren et al. (2013) also identified a putative second DCL in Phytophthora, but this protein is more similar in sequence to Drosha proteins. Sequencing of sRNAs from Phytophthora spp. has revealed at least two major size classes (21 nt and 25/26 nt) of sRNAs (Vetukuri et al., 2012; Fahlgren et al., 2013; Qutob et al. 2013), although longer sRNAs of over 30 nt are also present (Vetukuri et al., 2012). Generation of sRNA size classes depends on their genomic locus of origin, as a higher proportion of 21 nt sRNAs mapped to inverted repeats and genes, presumably controlled at the post-transcriptional level, while sRNAs of 25–26 nt mapped predominantly to transposable elements and are suggested to control their transcription by inducing epigenetic modifications (Fahlgren et al., 2013; Jia et al., 2017). In addition, it was observed that silencing of specific elements of the RNA silencing pathway affects the production of sRNAs of precise sizes. For example silencing of Dcl1 affected 21 nt sRNA production, while silencing of Ago4 or Ago5 affected 32 nt sRNAs, which seem to be generated in a DCL-independent manner (Vetukuri et al., 2012). Similarly, AGO1 is mostly associated with 21 nt sRNAs with a 5′ cytosine, while AGO4 binds mostly 25–26 nt sRNAs with a 5′ uracil (Åsman et al., 2016). Furthermore, the Ph. infestans clade 2 AGOs (AGO3, AGO4 and AGO5) do not have the typical catalytic amino acids in their PIWI domain, which normally mediates the cleavage of RNA (Nakanishi et al., 2012; Swarts et al., 2014), and it was suggested by Åsman et al. (2016) that these AGOs could mediate RNA regulation in a cleavage-independent way.
Small RNAs were observed to map to several Crinkle and Necrosis (CRN) and RxLR effectors of Ph. infestans, Ph. parasitica and Phytophthora sojae (Vetukuri et al., 2012; Jia et al., 2017; Wang et al., 2019). Since these two effector classes are known for their essential roles in overcoming plant immune responses (Anderson et al., 2015; Amaro et al., 2017; Liu et al., 2019), it is likely that RNA silencing has an important role in the regulation of key pathogenicity systems during the induction of Phytophthora diseases and evasion of detection by resistance proteins. Furthermore, sRNAs from oomycetes can occasionally be transferred directly to host organisms and carry out a role in virulence by “hijacking” the RNA silencing mechanism of their host, as was proven in Hyaloperonospora arabidopsidis infecting Arabidopsis thaliana (Dunker et al., 2020). Cross-kingdom and cross-species RNA silencing is not only observed in pathogens, but it is quickly being discovered or predicted in a number of biocontrol organisms. For example, Beauveria bassiana can use the milRNA bba-milR1 to attenuate the immune response of its host Anopheles stephensi (a mosquito vector of malaria parasites) (Cui et al., 2019), while many sRNAs of the mycoparasite Clonostachys rosea, another fungal species, were predicted to target virulence factors in Botrytis cinerea and Fusarium graminearum (Piombo et al., 2021, 2022). However, the potential for cross-species RNA silencing has never been assessed in biocontrol oomycetes to date.
The aim of this study was to analyze RNA silencing components and sRNA populations of oomycetes with diverse lifestyles, for which RNA silencing mechanisms have so far been unexplored, to investigate whether sRNAs are a major determinant of oomycete lifestyles, ranging from plant pathogenic to fungus and mosquito parasitic. The species to be analysed here are L. giganteum, a parasite of several mosquito species, the mycoparasites Py. oligandrum and Py. periplocum, and the Phytophthora plant pathogens Ph. cactorum, Ph. colocasiae and Ph. plurivora. Lagenidium giganteum has been used as a BCA against mosquitoes, while Py. oligandrum and Py. periplocum are used against plant pathogenic fungi and oomycetes (Paul, 1999; Bělonožníková et al., 2022). We also included the fungal biocontrol species C. rosea as an outgroup for comparisons. To achieve the aim we: (1) identified genes coding for DCLs, AGOs and RDRPs in the considered genomes, (2) performed sRNA sequencing of these species and analyzed the sRNA populations to ascertain differences in size, sequence bias and mapping between different sRNA populations, with emphasis on sRNAs mapping to known effector classes, (3) predicted milRNAs and milRNA targets, with a focus on putative cross-species targets with potential role in the biocontrol action of L. giganteum, Py. oligandrum and Py. periplocum. The results presented constitute an initial survey of how RNA silencing can affect the regulation of genes involved in pathogenesis and biocontrol in oomycetes, paving the way for practical applications in the field (Kalyandurg et al., 2021).
The genomes used in this project are C. rosea IK726 (GCA_902827195.1), L. giganteum ARSEF 373 (GCA_002286825.1), Ph. cactorum P414 (GCA_016864655.1), Ph. colocasiae 7,290 (GCA_002288995), Py. oligandrum CBS 530.74 (Kushwaha et al., 2017a), Py. periplocum CBS 532.74 (Kushwaha et al., 2017b) and Ph. plurivora AV1007 (GCA_002247145.1). As gene predictions were not available for L. giganteum, Ph. colocasiae, Ph. plurivora, Py. oligandrum and Py. periplocum, genes were predicted using Augustus v3.4 (Stanke et al., 2006) and MAKER v3.01.1 (Campbell et al., 2014; Supplementary Files 1, 2). Species-specific parameters for Augustus were obtained by Augustus online training using available proteins from other oomycete species (Stanke and Morgenstern, 2005). Single copy genes present in all the considered species were identified with Orthofinder v2.5.2 (Emms and Kelly, 2019), concatenated and aligned with mafft v7.453 (Katoh and Standley, 2013) with the “-auto” option to select the best parameters. A phylogenetic tree was then obtained using iqtree2 v2.1.3 (Nguyen et al., 2015) with the option “-m MFP” to select the best model. The trees were visualized with FigTree v1.4.4 (Rambaut, 2018).
The predicted proteomes of the considered species were annotated with InterProScan v5.46–81.0 (Jones et al., 2014). Proteins having both the ribonuclease III domain (IPR000999) and the Dicer dimerisation domain (IPR005034) were considered to be DCLs. Proteins having both the PAZ domain (IPR003100) and PIWI domain (IPR003165) were considered to be AGO proteins. Proteins having the eukaryotic type RDRP domain (IPR007855) were considered to be RDRPs.
Each category of proteins was aligned with mafft v7.453 (Katoh and Standley, 2013) with the “--maxiterate 1,000 --localpair” options, and a phylogenetic tree with 1,000 bootstraps was constructed using iqtree2 v2.1.3 (Nguyen et al., 2015) with the option “-m MFP” to select the best model. Both alignment and phylogenetic tree construction were run using only the conserved domains of interest (IPR000999, IPR005034, IPR003100, IPR003165, IPR007855). The trees were visualized with FigTree v1.4.4 (Rambaut, 2018) and they were rooted at C. rosea.
Ph. colocasiae, Ph. cactorum, Ph. plurivora, Py. oligandrum, and Py. periplocum were individually cultured in liquid V8 juice media and L. giganteum on PYG liquid medium (Domnas et al., 1977) for 3 days at 20°C and collected by gravity filtration. The different culture samples were snap frozen in liquid nitrogen and kept at −70°C until RNA extraction. Total RNA was obtained using the Ambion mirVana miRNA isolation kit according to the manufacturer’s instructions (Invitrogen, Waltham, MA), and RNA quality was assessed using Agilent Bioanalyzer chips (Agilent Technologies, Santa Clara, CA). The sequencing was carried out at the SciLife sequencing facility in Stockholm using an Illumina HiSeq2500 sequencing platform in High Output mode, SR 1x50bp. FastQC v0.11.3 (Andrews, 2010) was used to examine the quality of raw reads.
Adapters, low quality and low complexity reads were removed with bbduk v38.86 (Bushnell, 2019) using the following options:
Bbduk.sh in = raw.fq out = clean.fq ref. = adapters.fa ktrim = r k = 23 mink = 11 hdist = 1 qtrim = r trimq = 10 maq = 10 minlen = 18 entropymask = f entropy = 0.5 entropywindow = 18 entropyk = 5.
The resulting reads were checked with FastQC v0.11.3 (Andrews, 2010). Afterwards, “reformat.sh” from the BBTools package v38.86 (Bushnell, 2019) was used to remove reads shorter than 18 bp or longer than 32 bp, and structural RNAs (rRNAs, tRNAs, snoRNAs and snRNAs) were removed using SortMerRNA v4.3.4 (Kopylova et al., 2012). The resulting reads were mapped to the respective genomes using bowtie v1.0.0 (Langmead, 2010) with the following options:
bowtie -S -p 10 -k 101 -v 1 -m 20 --best --strata bowtie_index reads.fq output.sam.
FeatureCounts v2.0.0 (Liao et al., 2014) was used to determine the number of reads mapped to various genomic features, using the following options:
featureCounts --fracOverlap 0.7 -O --fraction -g Parent -t genomic_feature_of_interest -a file.gff -o counts.txt BAMfiles.
InterProScan v5.46.81.0 (Jones et al., 2014) was used to predict proteases. Diamond and HMMer were used on the dbCAN and dbCAN_sub databases through the dbCAN2 metaserver, and any gene predicted as a CAZyme by one of these programs was considered a CAZyme in subsequent analyses (Buchfink et al., 2015; Prakash et al., 2017; Zhang et al., 2018). Putative effectors were determined from these groups by predicting secretion using the procedures recommended previously (Gogleva et al., 2018). SignalP v4.0 (Petersen et al., 2011) was used to predict proteins possessing a signal peptide for secretion. Proteins with transmembrane domains were identified with TMHMM v2 (Krogh et al., 2001) and excluded from the analysis of effectors. TargetP v2 was then used to exclude proteins targeted to mitochondria (Almagro Armenteros et al., 2019) and PredGPI was used to remove proteins with a GPI anchor (Pierleoni et al., 2008). CRN and RxLR effectors were specifically predicted using effectR (Tabima and Grünwald, 2019).
Duplicated genes were determined with BLAST, comparing every proteome to itself with minimum identity of 85% and minimum query coverage of 90%. Antisense overlapping locations were determined with an ad hoc Python script using the Pandas module (McKinney, 2010), setting promoters to be 500 bp in length using promoter_extractor.1 When a promoter would have overlapped the exons of the previous gene on the same strand the promoter length was set so it commenced after the previous gene. The coordinates of introns and untranslated region (UTR) sequences (when available from gene prediction) were included in the gff files using, respectively, the programs “add_utrs_to_gff”2 and GenomeTools with the “-addintrons” option (Gremme et al., 2013).
Transposons and repeat sequences were predicted with RepeatModeler v.2.0.1 (Flynn et al., 2020) and coordinates were obtained through RepeatMasker v. 4.1.1 (Smit et al., 2015). The data was analyzed using the Python module Pandas (McKinney, 2010) and visualization was carried out with the Python module Seaborn (Bisong, 2019).
milRNAs were predicted with mirdeep2 v.2.0.0.7 (Friedländer et al., 2012) and they were retained only if they were present in at least 10 counts on average in every sample of the considered species. Targets were predicted using TargetFinder and psRNAtarget, as well as TAPIR and psRobot through the online sRNAtoolbox (Bo and Wang, 2005; Bonnet et al., 2010; Wu et al., 2012; Rueda et al., 2015; Dai et al., 2018). Predictions supported by at least two programs were considered in further analysis. Prediction of candidate transboundary milRNA targets was carried out using the same programs for milRNAs of Py. oligandrum and Py. periplocum. Available transcripts of Ph. infestans (GCF_000142945.1) and B. cinerea (GCF_000143535.2) were used as putative targets, and only predictions supported by all four software packages were considered. Prediction of candidate transboundary targets for milRNAs of L. giganteum was performed using the animal-dedicated tools Miranda, PITA and TargetScan, used online through the sRNAtoolbox (Rueda et al., 2015). The targets were 3' UTR regions of insect host Aedes aegypti (GCF_002204515.2), where coordinates were derived from the gff file using “add_utrs_to_gff” (see Footnote 2). Only predictions confirmed by all three software packages were considered.
We performed domain prediction in the species of interest to identify the components of the RNA silencing mechanism. All of the oomycete genomes analysed contained a single gene encoding a DCL, while the number of AGO and RDRP coding genes was variable (Table 1). The number of AGO coding genes was lowest in Py. periplocum (two genes), while Ph. plurivora had six AGO coding genes. One AGO coding gene, similar to Ago1 and/or Ago2 from Ph. infestans (clade 1) was identified in the analysed species, with the exception of two genes in L. giganteum. All other AGOs were similar to Ago3/4/5 from Ph. infestans (clade 2). A single RDRP gene was predicted in the species analysed, with the exception of two and five genes in Py. periplocum and L. giganteum, respectively. Some of the predicted DCL proteins show a P-loop NTPase fold (IPR027417) or a DEAD box helicase domain (IPR011545), in addition to the Dicer dimerization domain (IPR005034) and the ribonuclease III domain (IPR000999). Similarly, all AGOs have a combination of protein argonaute N-terminal domain (IPR032474), Argonaute linker 1 and linker 2 domains (IPR014811, IPR032472) and Argonaute mid domain (IPR032473), in addition to the PAZ (IPR003100) and PIWI (IPR003165) domains. All the domains detected in the considered proteins are listed in Supplementary Table 1.
Table 1. Copy number of genes coding for DCLs, AGOs and RDRPs in the species considered in this study.
Phylogenetic analysis showed that DCLs clustered according to the expected evolutionary relationships between the species (Figure 1), with both Pythium spp. clustering together and separately from the Phytophthora spp. Both of these groups clustered separately from C. rosea, while L. giganteum clustered with the Pythium spp. (Figure 2A). AGOs clustered in two main clades according to their similarity to AGO1/2 or AGO 3/4/5 of Ph. infestans, again with a clear separation from C. rosea. In each of these two clusters, Pythium AGOs were separate to those from Phytophthora, and L. giganteum proteins were closer to those from Pythium (Figure 2B). Contrary to the DCL and AGO phylogenies, L. giganteum RDRP proteins clustered separately from both Pythium and Phytophthora RDRPs, and were closer to those from C. rosea (Figure 2C).
Figure 1. Phylogenetic tree illustrating phylogenetic relationships of the species of interest, based on the concatenation of all single-copy proteins present in every species. Rapid bootstrap support (≥60%) values from 100,000 iterations are associated with nodes.
Figure 2. Phylogenetic tree illustrating phylogenetic relationships of: DCL proteins (A), AGO proteins (B) and RDRP proteins (C). The two AGO clades are separated by red lines. Bootstrap support (≥60%) values from 1,000 iterations are associated with nodes.
A second class of DCL proteins, similar to Ph. infestans DCL2, was also detected in the considered oomycetes. This class has two RNaseIII domains but no Dicer dimerization domain and it is more similar to the Drosha protein. All the considered Pythium spp. and Phytophthora spp. had one gene belonging to this class in their genomes. Pythium DCL2 proteins clustered separately to those from Phytophthora. L. giganteum was the only exception as it had two genes coding for DCL2 proteins; one was similar to Phytophthora DCL2 and the other was separate to the DCL proteins of the other oomycetes (Supplementary Figure 1).
Sequencing of sRNAs produced between 7.9 and 60 million high-quality reads for each sample. After removal of structural RNAs (ribosomal RNAs, transfer RNAs, small nuclear RNAs and small nucleolar RNAs), as well as reads shorter than 18 or longer than 32 nt, the final numbers of sequences ranged between 6.6 million and 27.1 million reads (Supplementary Table 2). L. giganteum yielded the highest proportion of usable reads (between 70 and 83% of the reads were kept when filtering for size and non-structural RNAs), while Py. periplocum had the lowest proportion (between 13 and 14% of total reads were usable).
Average length distribution of sRNAs revealed that all oomycete species analysed exhibited clear peaks at 21 nt and 25/26 nt, but with differences in the level of these peaks. The Phytophthora spp. analysed had a further distinct sRNA size peak at 31 nt. L. giganteum had an overall size distribution and abundance pattern that appeared most similar to the Phytophthoras. 25/26 nt sRNAs represented 40% of reads in Ph. cactorum and Ph. colocasiae, 50% in Ph. plurivora and 75% in L. giganteum. Pythium spp. had smaller peaks for sRNAs of 21, 26 and 32 nt, and none of them amounted to more than 15% of the reads (Figure 3A).
Figure 3. Characteristics of sRNAs identified in Pythium spp., Phytophthora spp., Clonostachys rosea and Lagenidium giganteum. (A) Read length distribution. (B) 5′ base distribution. (C) 5′ base distribution based on sRNA size class.
In C. rosea and Pythium spp. samples around 30% of sRNAs had an adenine or uracil at the 5′ end. Approximately 20% of C. rosea sRNAs had a 5′ guanine and cytosine. In the Pythium spp., approximately 20% of sRNAs had a 5′ guanine, while approximately 15% had a 5′ cytosine. In comparison, in all Phytophthora spp. there was a clear preference for uracil at the 5′ end of sRNAs, up to 54% in Ph. plurivora. This phenomenon was even more pronounced in L. giganteum, in which 88% of sRNAs had a 5′ uracil (Figure 3B). In all oomycete species assessed here, the prevalence of uracil at the 5′ end increased for sRNAs of 25/26 nt, with the most extreme example being approximately 95% of sRNAs in this size class in L. giganteum (Figure 3C). While guanine was the least preferred 5′ base for 21 nt and 25/26 nt sRNAs, especially in Phytophthora and L. giganteum, it was typically the most common 5′ base for longer sRNAs of 31/32 nt, with the exception of L. giganteum which had cytosine as the most common base at the 5′ end of 31 nt sRNAs. In the two Pythium spp., the 21 nt sRNAs exhibited similar preference for a 5′ adenine or uracil (30–40%), with lesser proportions of cytosine and guanine (10–20%). In contrast, 21 nt sRNAs from Phytophthora and L. giganteum exhibited a preference for 5′ uracil (45–50%), a strong bias against guanine (less than 10%), and a higher proportion of 5′ cytosine (20–30%) (Figure 3C).
Mapping of sRNAs to the respective genomes revealed that a majority of sRNAs originated from intergenic regions. This portion of the reads was highest (more than 80%) in C. rosea, while it was lowest in Py. oligandrum and Py. periplocum amounting to less than 50% of the total sRNA reads. Among the reads mapping to genetic features (Figure 4), sRNA reads from oomycetes mapped more frequently to exons than to promoters or introns, while in the ascomycete C. rosea a higher proportion of sRNAs mapped to promoters. Most of the reads assigned to exons were assigned to CDS regions. UTRs could only be predicted for C. rosea, Ph. colocasiae, Py. oligandrum and Py. periplocum, and in every case the 3’ UTR had more sRNAs mapped to it than the 5’ UTR. Ph. cactorum and Ph. colocasiae had similar mapping statistics, with exons/CDSs as the source of most sRNAs, followed by promoters and then introns. Ph. plurivora, however, had more sRNAs mapping to introns than to promoters, and the same was true for L. giganteum. In the two Pythium spp. Py. oligandrum had similar numbers of sRNAs mapping to promoters and introns, while in Py. periplocum promoters were the origin of almost double the sRNAs mapping to introns. Even after normalizing according to the number of reads of each length, 25 and 26 nt sRNAs were still the most likely classes mapping to genes in Ph. cactorum and Ph. plurivora, while a peak at 21 nt was also present in L. giganteum and Ph. colocasiae and peaks at 21 nt and 31 nt were present in the Pythium spp. (Figure 5A).
Figure 4. Proportion of sRNA reads mapped to different genic locations of the respective species. Error bars indicate the standard deviation between the replicates. The percentage is calculated on the total number of reads mapping to genes.
Figure 5. Number of reads mapping to transcripts. Data normalized according to total kb extent of the transcriptome in the genome and to millions of reads of the specific sRNA size for the sample. Error bars indicate the standard deviation. (A) All transcripts. (B) Duplicated transcripts.
In the analysed oomycete species, a higher number of sRNAs mapped to duplicated genes, ranging from 25 to 50 counts per kb of duplicated genes per million reads of the 21, 25 and 26 nt sRNAs compared to 2–12 sRNA counts when all genes were considered (Figure 5B). The sRNA size classes most likely to be mapped to duplicated genes were the same which mapped to single genes. The number of 25 nt and 26 nt sRNAs mapping to duplicated transcripts was particularly high in Ph. plurivora, with peaks of over 200 counts per kb of duplicated genes per million reads (Figure 5B). GO term enrichment analysis of duplicated genes from oomycetes species used in this study showed enrichment of the GO term “zinc ion binding” (GO:0008270). In addition, the GO term “FMN binding” (GO:0010181) was enriched in the duplicated genes of both Pythium spp., but not in the Phytophthora spp. (Supplementary Table 3).
Among the species analysed, Py. oligandrum and Py. periplocum had the highest amount of antisense overlapping regions (79 kb - 100 kb per Mb), followed by Ph. colocasiae at 55 kb per Mb (Figure 6A). However, after normalization, according to the length of these regions, Ph. cactorum and Py. oligandrum sRNAs showed higher mapping (350 sRNAs per kb) to antisense overlapping regions per million mapped reads, followed by Py. periplocum and Ph. colocasiae with 279 and 190, respectively. In C. rosea, L. giganteum and Ph. plurivora, between 130 and 50 sRNAs were mapped per kb of antisense overlapping region (Figure 6B).
Figure 6. Mapping of sRNAs to antisense overlapping regions. (A) Extent of antisense overlapping regions in the analyzed species. (B) Number of sRNA reads mapping to antisense overlapping regions. Data normalized according to millions of reads per sample, length of the genomes and length of the antisense overlapping regions. Error bars indicate the standard deviation. (C) Length distribution of sRNAs mapping to antisense overlapping regions.
The most frequent length for oomycete sRNAs mapping to antisense overlapping regions was 25–26 nt in L. giganteum and the Phytophthora spp., while these peaks were not present in the Pythium spp. All oomycete species analysed also had a second peak at 21 nt, while the length distribution of C. rosea reads was very different from that of the oomycetes and presented no distinct peaks, even though the most common length for sRNAs mapping to antisense overlapping regions was 18 bp (Figure 6C). The 31 nt peak observed in the total sRNA population was absent in all species analysed when sRNA were mapped to antisense overlapping regions.
Transposons and repetitive regions were more frequent in Ph. cactorum (around 279 kb repeat/transposon per Mb of genome), followed by Ph. colocasiae at more than 200 kb/Mb and then C. rosea, L. giganteum, Ph. plurivora, Py. periplocum, and Py. oligandrum, with the last three having between 50 and 100 kb/Mb (Figure 7A). The most abundant repeat/transposon in Phytophthora spp. were LTRs, in particular those of classes Copia and Gypsy, followed by DNA transposons. In Pythium spp., on the other hand, around 85% of transposons and repeated sequences were unclassified, with the most common recognized class being simple repeats (Figure 7B). The extent of repeated regions was not a good indicator of the number of sRNAs mapping to them. In particular, L. giganteum was by far the species with the highest number of sRNA reads mapping to repeats/transposons, reaching almost 14,000 reads per kb of repeats per Mb of genome, normalized also according to sequencing depth. The analysed Phytophthora spp. ranged between 4,500 and 6,000 sRNAs per kb/Mb of repeats, with Ph. plurivora reaching a similar value to the other two Phytophthora spp. despite having much less of its genome composed of repeat/transposon sequences. Pythium spp. and C. rosea, however, had less than 1,000 sRNAs per kb/Mb of repeat/transposon. (Figure 7C). Most of the sRNAs mapping to transposons and repetitive regions of oomycetes had a length of 25–26 nt, with the exception of Ph. cactorum which had the major peak at 31 nt. Moreover, Ph. colocasiae, Ph. plurivora and both Pythium spp. also had a peak at 21 nt. C. rosea had a different distribution from the oomycetes and had peaks at 18–20 nt and 23 nt (Figure 7D).
Figure 7. Identification of transposons and repeated sequences with RepeatModeler. (A) Extent of transposons and repeated sequences in the analyzed species. (B) Families of transposons identified in the genomes of the species of interest. (C) Number of sRNA reads mapping to transposons and repeated sequences. Data normalized according to millions of reads per sample, length of the genomes and length of the repetitive regions. Error bars indicate the standard deviation. (D) Length distribution of sRNA mapping to transposons and repeated sequences.
We predicted CRNs and RxLR genes in the genomes of interest, as these are two important classes of effectors in oomycete plant pathogens (Anderson et al., 2015; Amaro et al., 2017). Genes encoding CRNs were detected in every oomycete species analysed except Py. oligandrum, with the highest number identified in Ph. cactorum (230 putative CRN coding genes), while 85 genes coding for CRNs were detected in L. giganteum (Table 2). After normalizing according to the length of regions covered by these genes, the sizes of sRNAs mapping to predicted CRNs appeared to be species-specific, with 21 nt sRNAs more likely to map to these transcripts in Ph. colocasiae and Py. periplocum, while 25 nt and 26 nt sRNAs were more common in Ph. plurivora, and 21, 25 and 25 nt sRNAs were equally likely to target CRNs in L. giganteum and Ph. cactorum (Figure 8A).
Table 2. Number of putative secreted proteins, including effectors, predicted in fungal and oomycete species analyzed in this study.
Figure 8. Number of sRNA reads mapping to putative effectors detected in the species of interest. Data normalized according to total kb extent of the respective effector area in the genome and to millions of reads of the specific sRNA size for the sample. Error bars indicate the standard deviation. (A) CRNs. (B) RxLRs.
Genes coding for proteins with putative RxLR motifs, on the other hand, were predicted in every species, again with the highest number (373 genes) detected in Ph. cactorum. In Phytophthora spp., the amount of 25–26 nt sRNAs mapping to these effectors was proportionally higher than for other sizes of sRNAs (Figure 8B), although in Ph. colocasiae 21 nt sRNAs were almost as likely to target RxLRs as 25 and 26 nt sRNAs (Figure 8B). The Pythium spp. had a low number of putative RxLRs (20 to 27), and they were on average more targeted by sRNAs than those in Phytophthora. These genes encoded proteins with the typical RxLR-EER motifs, but lacked predicted signal peptides, had no homology with any previously described RxLR effectors (Tabima and Grünwald, 2019), and it is likely they perform other functions in the Pythium spp., as the RxLR motif can sometimes occur by chance. This is likely the case also for the few RxLR genes identified in C. rosea and L. giganteum.
Secreted CAZymes often have roles in interactions with the host organism. Ph. cactorum and Ph. plurivora had the highest number of these proteins (276 and 223, respectively), while Py. oligandrum and Py. periplocum had 140 and 119, respectively. Considering the sRNAs that mapped to genes encoding secreted CAZymes, 56% of Py. periplocum sRNAs mapped to GH17 (1,3-β-glucosidases), which is considerably higher compared to Phytophthora spp. sRNAs (12% on average). In Phytophthora spp., a higher proportion of sRNA reads (19%) mapped to AA17 (copper-dependent lytic polysaccharide monooxygenases), followed by GH17. These two classes were the origin of most sRNAs even in L. giganteum. Interestingly, in Ph. colocasiae and Ph. cactorum more than 10% of sRNAs mapping to secreted CAZymes mapped to GH30 (xylanases and glucosidases) and PL3 (pectate lyases), while these were almost absent in Ph. plurivora, which had instead more sRNAs mapping to GH3 (glucosidases, xylosidases and alpha-L-arabinofuranosidases) and CE8 (pectin methylesterases) CAZymes (Figure 9A). Both Pythium spp. had proportionally more sRNAs of all sizes mapping to genes encoding secreted CAZymes, when compared with the Phytophthora spp. (Figure 9B). The largest proportion of reads mapping to secreted CAZymes were 31 nt length in both Pythium spp., and 32 nt sRNAs more commonly mapped to CAZyme coding genes than 21, 25 or 26 nt sRNAs in Py. oligandrum. In the Phytophthora spp., Ph. colocasiae had the highest number of sRNAs mapping to genes encoding secreted CAZymes, with a predominance of 31 nt and 32 nt sRNAs, while all size classes except 31 nt were equally present in Ph. cactorum. In Ph. plurivora, 21, 31 and 32 nt sRNAs were present in similar quantities. A low number of reads mapped to secreted CAZymes in C. rosea and L. giganteum compared to Pythium or Phytophthora spp.
Figure 9. Distribution of the sRNA reads mapping to secreted CAZyme families in the species of interest (A). Number of reads mapping to secreted CAZymes (B), proteases (C) and secreted proteins not predicted to be CAZymes, proteases, CRNs or RxLRs (D). Data normalized according to total kb extent of the respective gene area in the genome and to millions of reads of the specific sRNA size for the sample. Error bars indicate the standard deviation.
Among the oomycete species analysed, L. giganteum had the highest number of secreted proteases (386), followed by Py. oligandrum with 168, while the species with the lowest number was Ph. plurivora with 43. As with CAZymes, Pythium spp. had proportionally more sRNAs mapping to secreted proteases, when compared with the other species (Figure 9C). Secreted proteases were associated mostly with 21 nt sRNAs in Py. periplocum and 21 and 31 nt sRNAs in Py. oligandrum. 31 nt and 32 nt sRNAs were more prevalent in Ph. colocasiae and Ph. plurivora, while 21, 25, 26 and 32 nt sRNAs were equally likely to map to a secreted protease gene in Ph. cactorum. 21, 25 and 26 nt sRNAs were the most prevalent size classes mapping to genes coding for secreted proteases in L. giganteum.
Other secreted protein coding genes were also the origin of more sRNAs in Pythium spp., especially 31 nt sRNAs in Py. oligandrum and 21 nt sRNAs in Py. periplocum (Figure 9D). sRNAs of 31 nt and 32 nt were proportionally more abundant in Ph. colocasiae, while 21 nt sRNAs were prevalent in Ph. plurivora and L. giganteum, and 21 nt and 31 nt sizes were prevalent in Ph. cactorum.
milRNA prediction resulted in the identification of 38 putative milRNAs from the insect parasitic oomycete L. giganteum, which is a markedly higher number compared to the number of milRNAs identified in mycoparasitic Py. oligandrum (five milRNAs) and Py. periplocum (three milRNAs). Among the Phytophthora spp. a higher number of milRNAs were predicted in the taro leaf blight pathogen Ph. colocasiae (13 milRNAs) compared to two and three milRNAs in Ph. cactorum and Ph. plurivora, respectively. These milRNAs were novel and unique to the respective species with the exception of pol_mir_1-ppe_mir_1, which was present in both Py. periplocum and Py. oligandrum. The precursors of the majority of milRNAs were located in intergenic regions (Supplementary Table 4). Additional information on the predicted milRNAs is presented in Supplementary Table 4.
milRNAs normally perform their regulating action by inducing the degradation of target transcripts. Between two and 25 endogenous milRNA targets were predicted for each species (Table 3; Supplementary Table 5). The detected milRNAs were predicted to control a variety of genes, including a dynein protein of Ph. colocasiae (PCOL_00008544-RA), a CRN effector of L. giganteum (g26638.t1), an RxLR effector of Ph. plurivora (g9339.t1) and one of Ph. cactorum (Pcac1_g11221.t1). This last transcript shows high similarity (qcov >90% and ID >85%) to transcript U17742 identified in Ph. cactorum strain 10,300, upregulated in germinating cysts with germ tubes compared to mycelium and zoospores (Chen et al., 2018).
Some milRNAs are known to be exported to interacting organisms and perform their regulation activity in host cells (Wang et al., 2016; Cui et al., 2019), therefore we predicted putative transboundary targets for our species of interest. MilRNA Ppe_mir_2 of Py. periplocum was predicted to target three genes of Phytophthora infestans (PITG_00939, PITG_03209, PITG_13437), encoding a putative diphthine-ammonia ligase, an oomycete-specifi c conserved hypothetical protein, and a metalloprotease, respectively, suggesting the potential for cross-species RNA silencing regulation in the biocontrol action of Py. periplocum. The same milRNA also had four putative gene targets in B. cinerea (Supplementary Table 5), consisting of two membrane proteins, one protein with a zinc finger C2H2-type domain (BCIN_04g03280) and Bccch1, a calcium channel involved in vegetative growth in conditions of low extracellular calcium (Harren and Tudzynski, 2013). These targets were copredicted for the same milRNA by four separate prediction programs, and no endogenous target was similarly copredicted for these Py. periplocum milRNAs. However, eight endogenous targets were copredicted by three programs for ppe_mir_2 (Supplementary Table 5). MilRNA Ppe_mir_1, on the other hand, had no endogenous gene targets co-predicted by three or four programs, but one putative transboundary gene target in B. cinerea, a putative GTPase activating protein (BCIN_07g03380) copredicted as a target by all four of the programs used (Supplementary Table 5). The same type of co-prediction was performed for milRNAs of L. giganteum and targets of Aedes aegypti, resulting in 5227 transcripts identified as putative targets.
This study into RNA silencing components in the oomycetes represents the broadest overview to date and reveals commonalities and some specific differences among this group of filamentous microbes. All oomycetes analysed here had DCLs, AGOs and RDRPs in their predicted proteomes.
The first step in RNA silencing involves cleavage of long dsRNA substrates into sRNAs by DCL RNaseIII proteins. Each oomycete analysed here had one DCL protein. In a previous study, a second, more divergent DCL containing two RNaseIII domains was identified from Ph. infestans, Ph. sojae and Ph. ramorum (Fahlgren et al., 2013). However, BLASTP similarity searches with this protein in this study revealed that it is most similar to Drosha proteins. Previous studies with Ph. infestans showed that DCL1 is associated with the generation of 21 nt sRNAs (Vetukuri et al., 2012). While DCL2 may be more similar to Drosha proteins, the presence of two RNaseIII domains might suggest its involvement in generation of longer sRNAs such as the 25/26 nt class. However, all the oomycete species considered in this study had a homolog of Ph. infestans DCL2 (with the exception of L. giganteum, which had two), irrespective of the differences detected in sRNA length distribution. Linking oomycete DCL2 with a specific size of sRNA remains to be determined experimentally.
Oomycete AGOs are divided into two clades (Vetukuri et al., 2011; Fahlgren et al., 2013; Åsman et al., 2016; Jia et al., 2017). Clade 1 comprises closely related proteins that have the four catalytic amino acids in their PIWI domain, which allows them to mediate target cleavage. Clade 2 contains a more diverse grouping of AGO proteins and does not have this group of catalytic amino acids. Instead, clade 2 AGOs are suggested to mediate mRNA regulation in a cleavage-independent way (Åsman et al., 2016). Clade 1 is furthermore associated mostly with 21 nt sRNAs and a preference for cytosine at the 5′ end, as well as with a post-transcriptional regulation role, while clade 2 is associated with 25/26 nt sRNAs, a preference for uracil at the 5′ end, and a regulation role at the transcriptional level (Vetukuri et al., 2012; Fahlgren et al., 2013; Åsman et al., 2016; Jia et al., 2017). In plants like A. thaliana there is also a similar separation, with AGO3 and AGO4 binding mostly 24 nt sRNAs and mediating transcriptional silencing through epigenetic modifications, while other AGOs bind 21 nt sRNAs and mediate post-transcriptional gene silencing (Wang et al., 2011; Zhang et al., 2016). Ph. infestans has two AGOs belonging to clade 1 (Ago1/2) and three belonging to clade 2 (Ago3-5) (Vetukuri et al., 2011), while the Phytophthora spp. analyzed in this study have only one clade 1 and three to five clade 2 AGOs. The relatedness of the Phytophthora AGOs, and the distance to the lower number of more diverse AGOs in Pythium and L. giganteum, suggests that the Phytophthora sequences have undergone diversification since the split from other Pythiaceae. It remains to be determined how this relates to functioning of RNA silencing in Pythium and L. giganteum, but may explain the different abundances of different sRNAs sizes, especially those of 25/26 nt.
It was expected that the Phytophthora spp. would have major groupings of sRNAs at 21 nt and 25/26 nt. While a third group of sRNAs of 32 nt in length was observed in the work of Vetukuri et al., (2012) with Ph. infestans, we could not observe a peak at exactly this length for any Phytophthora spp. investigated in this study. However, there was a clear peak at 31 nt in each of the Phytophthora spp., and elevated numbers of 32 nt sRNAs were present in both Py. oligandrum and Py. periplocum. These longer sRNAs have been shown to be genuine in Ph. infestans and could be detected by Northern blot hybridisation analysis (Vetukuri et al., 2012).
L. giganteum, surprisingly, showed both a higher peak of 25–26 nt sRNAs and a more highly pronounced preference for 5′ uracil than the three Phytophthora spp., despite having an equal number of AGOs of clade 1 and 2. This could be due to the action of five RDRPs, a class of enzyme with only one or two members in all the other oomycetes analysed. It is possible that the combination of enzymes in L. giganteum preferentially produce sRNAs of 25–26 nt in length. The L. giganteum RDRPs are also peculiar in the fact that they are more similar to the ascomycetous C. rosea proteins than to the RDRPs of the other oomycetes. However, using BLAST we were able to identify similar RDRPs in other oomycete genera like Aphanomyces, Achlya and Saprolegnia (data not presented).
In accordance with previous studies, the sRNAs mapping to transposons and repeat-rich regions belonged mostly to the 25/26 nt size class, reinforcing the hypothesis that oomycetes use this class of RNAs to control transposons in their genomes (Åsman et al., 2016; Jia et al., 2017). However, in all species except Ph. cactorum and L. giganteum a second peak is present at 21 nt. Simple repeats are often the origin of 21 bp sRNAs in Ph. infestans (Fahlgren et al., 2013), and it is possible that they are the origin of the peak at this length for the species investigated here. Moreover, almost 50% of the repeat/transposon mapping sRNAs were of 31 nt in Ph. cactorum, a characteristic not shared by other Phytophthora species. Interestingly, this peak was unique to Ph. cactorum despite its similarity in repeat/transposon length and composition to Ph. colocasiae, that instead had a length distribution of sRNAs mapping to repeat/transposon closer to Ph. plurivora. This latter Phytophthora sp. had proportionally less Gypsy and other LTRs, more simple repeats and a total amount of repeats/transposons closer to Pythium species. This suggests that Ph. cactorum has a unique sRNA-dependent regulation mechanism based on sRNAs of 31 bp, which has not yet been explored. Pythium spp., on the other hand, had around six times less sRNAs mapping to repeat/transposon, in respect to Phytophthora spp., suggesting a functional difference in the role of RNA silencing among oomycetes.
Clonostachys rosea, the only non-oomycete included, had a very low amount of sRNA reads mapping to transposons despite having a similar quantity of them in its genome, compared with the analyzed oomycetes. Furthermore, most of the sRNAs mapping to C. rosea transposons were 18, 19, 20 or 23 nt in length, suggesting that fungi control transposons and repeat-rich genomic regions in different ways to oomycetes.
Oomycete genomes are characterized by having islands of densely packed genes, separated by long tracts of repeat-rich and gene sparse sequence. Oomycete gene promoters are very compact, and promoter regions frequently overlap on opposite DNA strands (Roy et al., 2013). Similarly, 3′ ends of genes can also be very close, and possibly overlap. The same study, which focused on Ph. infestans, also revealed that neighbouring genes were typically not activated at the same lifecycle stage, including those that were transcribed in antisense from small intergenic promotors. This may be a strategy that has evolved in oomycetes to regulate the transcriptional activity of densely packed gene space, while avoiding heterochromatin formation from RNA silencing mechanisms. Antisense overlapping regions were also a source of 25/26 nt sRNAs, even if a second peak was observable at 21 nt for all the oomycete species tested. The origin of some 21 nt sRNAs from the overlapping region of two adjacent genes has already been observed by Jia et al., (2017) in Ph. parasitica. In the current study, the species with the greatest extent of antisense overlapping regions were those with more sRNAs mapping to them, even after normalizing according to region length. The only exception was Ph. cactorum which, although it had only 23 kb per Mb of genome constituted by antisense overlapping regions, against the 105 kb/Mb of Py. oligandrum, was the species with the highest proportional number of sRNAs mapping to them. It should be noted that, while sRNAs are generated from these genomic antisense regions, the number of sRNAs from these regions is dramatically lower than that found for transposons.
CRNs and RxLRs are two large classes of effectors associated with pathogenicity of Phytophthora spp. (Anderson et al., 2015; Amaro et al., 2017; Liu et al., 2019). It has been observed in Ph. infestans that CRN genes are the source of mainly 21 nt sRNAs, while RxLRs are associated with the mapping of 25–26 nt sRNAs (Vetukuri et al., 2012; Fahlgren et al., 2013). Among the identified CRNs, less than 10% were predicted to be secreted via a conventional signal peptide, endoplasmic reticulum-Golgi route. If the CRNs without a predicted signal peptide are indeed extracellular, it would suggest that this class of effectors are secreted via non-conventional secretion mechanisms. Considering all the predicted CRNs, we found that 21 nt sRNAs are the most likely to map to CRNs in Ph. colocasiae, as was observed in Ph. infestans (Vetukuri et al., 2012; Fahlgren et al., 2013), while 25 nt and 26 nt were the most likely sRNA classes in Ph. plurivora and 21 nt, 25 nt, 26 nt and 32 nt had around the same frequency in Ph. cactorum. This was after normalizing each sRNA count according to the frequency of that sRNA size, meaning that 21 nt were not the dominant class of sRNAs mapping to CRNs in Ph. cactorum and Ph. plurivora even after considering that there were less 21 nt sRNAs than 25–26 nt. This suggests a high variability in sRNA mapping to this effector class in Phytophthora spp., which is also confirmed by the results of Jia et al. in Ph. parasitica (Jia et al., 2017) in which 53% of CRNs were associated with 25–26 nt sRNAs and not 21 nt. In comparison, both secreted and non-secreted RxLRs were more associated with 25-26 nt sRNAs in all Phytophthora spp., confirming the results of previous studies (Vetukuri et al., 2012; Fahlgren et al., 2013).
All other protein classes normally considered to contain effectors (secreted CAZymes, secreted proteases and other secreted proteins) had on average more sRNAs mapping to them in Pythium spp. than in other oomycetes. Both Pythium spp. had more sRNAs mapping to putative effectors compared to typical genes, while in both Phytophthora spp. and L. giganteum this number was lower for putative effector coding genes than for other genes. This suggests that effector production is generally not regulated by RNA silencing in these species, outside of specific effector classes like CRNs. Furthermore, it was interesting to observe that L. giganteum had more than double the number of secreted proteases (386) than Py. oligandrum (168), which was the oomycete species with the second highest amount. This may reflect the fact that a dedicated array of proteases is necessary to efficiently penetrate the cuticle of the insect hosts infected by L. giganteum.
Many families of CAZymes in oomycetes are composed of multiple members that have arisen by gene duplication (Ospina-Giraldo et al., 2010; Horowitz and Ospina-Giraldo, 2015; Liang et al., 2020; Sabbadin et al., 2021). In general, glycoside hydrolase family 17 (GH17) β-1,3-glucosidases are involved in cell wall biogenesis and modification, which is a prerequisite for cell growth and development (Beauvais and Latgé, 2018). Moreover, CAZymes of this class have been observed to be highly expressed during the early, mid and late stages of lupin infection by Ph. parasitica, and in all life stages in Ph. kernoviae (Blackman et al., 2015; Wang et al., 2021). Their involvement in both infection and regular growth can be explained by the fact that oomycete cell walls contain a high proportion of β-1,3-glucans, and plant cell walls can contain β-1,3-glucans as callose in response to pathogen infection. The high proportion of sRNAs mapping to genes coding for this class of CAZymes suggests that the careful regulation of these genes is crucial for normal growth and development in oomycetes. The significant differences in proportion of sRNAs mapping to GH17 coding genes between Phytophthora spp. and Pythium spp. is probably due to the evolutionary history of this GH family in oomycetes. Gene expression regulation of certain genes of this class is also reported in the fungal plant pathogen Cladosporium fulvum during late-stage infection of its host plant (Ökmen et al., 2019). Ökmen et al. found that a GH17 enzyme was expressed only during the late-stage of infection. Its activity released a damage-associated molecular pattern (DAMP) which induced the hypersensitive response and consequent reduction of fungal growth if the gene was constitutively expressed. In this light, our results might suggest that RNA silencing contributes to the tight regulation of GH17 proteins in the analyzed oomycetes, allowing them to influence cell wall biosynthesis, degrade plant cell walls and affect the induction of defense in plant hosts, a phenomenon fundamental for the action of both pathogens and biocontrol organisms. In both Py. periplocum and Py. oligandrum GH17 CAZymes have also been observed to be differentially regulated during specific stages of the interaction with Ph. infestans (Liang et al., 2020), therefore these β-1,3-glucosidases likely have a role during host parasitism, further explaining the high amount of sRNAs mapping to them in the analyzed Pythium species.
Another class of CAZyme with a consistent number of sRNAs mapping to it was AA17, an oomycete-specific family which was consequently not identified in C. rosea. This class had the highest number of sRNAs mapping to it in Phytophthora spp. and L. giganteum, and it is formed of copper-dependent lytic polysaccharide monooxygenases which are the most-induced CAZymes during early stages of Ph. infestans infection of tomato (Sabbadin et al., 2021). In Phytophthora spp. these enzymes can facilitate host penetration by cleaving pectin and disrupting the plant cell wall network, while possibly interfering with host immunity (Sabbadin et al., 2021). It is possible that there are also other substrate specificities in this CAZyme family. In the P. infestans genome, AA17 proteins form clusters of closely related (likely duplicated) genes. The high amount of sRNA mapping to them in all the oomycetes analysed suggest that RNA silencing has a role in their regulation and importance, even in Pythium spp. and L. giganteum.
The remaining secreted CAZyme classes predicted to be most affected by RNA silencing were all involved in hemicellulose degradation, but they differed between individual species. GH30 (xylanases and glucosidases) and PL3 (pectate lyases) originated more sRNAs in Ph. cactorum and Ph. colocasiae, while GH3 (glucosidases, xylosidases and alpha-L-arabinofuranosidases) and CE8 (pectin methylesterase) were more represented in Ph. plurivora, suggesting different hemicellulose degradation capabilities in these species.
Novel milRNAs were predicted for all the species analysed, but only one was shared between different species (Py. oligandrum and Py. periplocum), suggesting that most milRNAs have a species-specific function in oomycetes. This would also explain why known Ph. infestans milRNA miR8788 (Hu et al., 2022) was not detected in our dataset. MilRNA pca_mir_1 of Ph. cactorum was predicted to target the transcript Pcac1_g11221.t1, coding for the RxLR effector KAG2778520.1. The homolog of this transcript in Ph. cactorum strain 10,300, called U17742, was observed to be upregulated in germinating cysts with germ tubes, compared to mycelium and zoospores, and it is therefore possible that milRNA pca_mir_1 could be involved in the fine-tuning of the infection process (Chen et al., 2018). During the prediction of cross-species targets, Py. periplocum milRNA Ppe_mir_2 was predicted to target Ph. infestans gene PITG_13437, coding for a metalloprotease of family M16C. Several metalloproteases have been characterized as virulence factors for Ph. infestans (Schoina et al., 2021), and the potential for targeting enzymes of this class by Py. periplocum milRNAs suggests the possibility for this oomycete to use cross-kingdom RNA silencing as a component of its biocontrol action. The same milRNA was also predicted to target four B. cinerea transcripts with no predicted role in disease induction, and therefore it could have a function in transboundary RNA silencing against this fungal plant pathogen.
The prediction of putative cross-species targets was also performed for L. giganteum and its mosquito host A. aegypti, but animal-based target prediction software programs often search only for a 2–9 nt complementarity region and they produce a high number of false positives (Peterson et al., 2014; Riffo-Campos et al., 2016). In this case we predicted 5,227 putative A. aegypti targets for the milRNAs of L. giganteum, and expression, localization and binding analyses are necessary to reduce this number.
Oomycete species cause disease on a wide variety of host organisms. Their pathogenicity arsenal includes many protein effectors and can also include trans-boundary RNA silencing. To date, the proteins that generate sRNAs, and sRNA populations, have only been explored in a few species of Phytophthora. Here we extended the survey of RNA silencing proteins and sRNAs in three more species of Phytophthora, two Pythium spp., and L. giganteum. Our results show that, while all the species surveyed contain a single DCL protein, there was greater diversity in AGO and RDRP proteins that may be reflected in the sRNA populations. The major role for RNA silencing in the oomycetes appears to be for controlling the expansion of mobile elements in their genomes, but further roles may also be to limit gene expression from duplicated copies of genes, and regulation of effector gene expression, a process which seems more frequent in Pythium spp. than other oomycetes. Moreover, specific gene families seem to be targeted by precise sRNA sizes, such as effectors of the RxLR class which are predominantly targeted by 25/26 nt sRNAs in Phytophthora spp. The discovery of novel milRNAs from the biocontrol species Py. periplocum and L. giganteum with putative gene targets in hosts reveals the potential role for trans-boundary sRNA transport during biocontrol interactions.
The datasets presented in this study can be found in online repositories. The names of the repository/repositories and accession number(s) can be found in the article/Supplementary material.
RV, MD, and SW planned the study. RV prepared samples for sRNA sequencing. EP performed sRNA data analysis. EP, BK, and PS performed the phylogenetic analyses. EP wrote the first draft of the manuscript. RV and MD secured the funding. All authors contributed to the article and approved the submitted version.
This work was financially supported by the Swedish Research Council for Environment, Agricultural Sciences and Spatial Planning (FORMAS; grant numbers 2018–01420 and 2019–01316), Novo Nordisk Fonden (0074727), the Swedish Research Council (2019–04270), and Carl Tryggers Stiftelse för Vetenskaplig Forskning (CTS 19: 82, CTS 20: 464).
The authors acknowledge support from the National Genomics Infrastructure in Genomics Production Stockholm funded by Science for Life Laboratory, the Knut and Alice Wallenberg Foundation and the Swedish Research Council and SLU’s Centre for Biological Control. SW acknowledges funding from the Scottish Government Rural and Environment Science and Analytical Services (RESAS) Division. SW acknowledges funding from the Scottish Government Rural and Environment Science and Analytical Services (RESAS) Division.
The authors declare that the research was conducted in the absence of any commercial or financial relationships that could be construed as a potential conflict of interest.
All claims expressed in this article are solely those of the authors and do not necessarily represent those of their affiliated organizations, or those of the publisher, the editors and the reviewers. Any product that may be evaluated in this article, or claim that may be made by its manufacturer, is not guaranteed or endorsed by the publisher.
The Supplementary material for this article can be found online at: https://www.frontiersin.org/articles/10.3389/fmicb.2023.1076522/full#supplementary-material
1. ^https://github.com/EdoardoPiombo/promoter_extractor
2. ^https://github.com/dpryan79/Answers/tree/master/bioinfoSE_3181
Almagro Armenteros, J. J., Salvatore, M., Emanuelsson, O., Winther, O., von Heijne, G., Elofsson, A., et al. (2019). Detecting sequence signals in targeting peptides using deep learning. Life Sci. Alliance 2:e201900429. doi: 10.26508/lsa.201900429
Amaro, T. M. M. M., Thilliez, G. J. A., Motion, G. B., and Huitema, E. (2017). A perspective on CRN proteins in the genomics age: evolution, classification, delivery and function revisited. Front. Plant Sci. 8:99. doi: 10.3389/fpls.2017.00099
Anderson, R. G., Deb, D., Fedkenheuer, K., and McDowell, J. M. (2015). Recent progress in RXLR effector research. Mol. Plant-Microbe Interact. 28, 1063–1072. doi: 10.1094/MPMI-01-15-0022-CR
Andrews, S. (2010). FastQC: a quality control tool for high throughput sequence data. Available at: https://www.bioinformatics.babraham.ac.uk/projects/fastqc/
Åsman, A. K. M., Fogelqvist, J., Vetukuri, R. R., and Dixelius, C. (2016). Phytophthora infestans Argonaute 1 binds micro RNA and small RNA s from effector genes and transposable elements. New Phytol. 211, 993–1007. doi: 10.1111/nph.13946
Bartel, D. P. (2009). MicroRNAs: target recognition and regulatory functions. Cells 136, 215–233. doi: 10.1016/j.cell.2009.01.002
Bělonožníková, K., Hýsková, V., Chmelík, J., Kavan, D., Čeřovská, N., and Ryšlavá, H. (2022). Pythium oligandrum in plant protection and growth promotion: secretion of hydrolytic enzymes, elicitors and tryptamine as auxin precursor. Microbiol. Res. 258:126976. doi: 10.1016/j.micres.2022.126976
Bisong, E. (2019). “Matplotlib and Seaborn” in Building machine learning and deep learning models on google cloud platform (Springer), 151–165.
Blackman, L. M., Cullerne, D. P., Torrena, P., Taylor, J., and Hardham, A. R. (2015). RNA-Seq analysis of the expression of genes encoding cell wall degrading enzymes during infection of lupin (Lupinus angustifolius) by Phytophthora parasitica. PLoS One 10:e0136899. doi: 10.1371/journal.pone.0136899
Bo, X., and Wang, S. (2005). TargetFinder: a software for antisense oligonucleotide target site selection based on MAST and secondary structures of target mRNA. Bioinformatics 21, 1401–1402. doi: 10.1093/bioinformatics/bti211
Bonnet, E., He, Y., Billiau, K., and Van de Peer, Y. (2010). TAPIR, a web server for the prediction of plant microRNA targets, including target mimics. Bioinformatics 26, 1566–1568. doi: 10.1093/bioinformatics/btq233
Buchfink, B., Xie, C., and Huson, D. H. (2015). Fast and sensitive protein alignment using DIAMOND. Nat. Methods 12, 59–60. doi: 10.1038/nmeth.3176
Bushnell, B. (2019). BBTools: a suite of fast, multithreaded bioinformatics tools designed for analysis of DNA and RNA sequence dData. Jt. Genome Inst. Berkeley, CA, 2018.
Campbell, M. S., Holt, C., Moore, B., and Yandell, M. (2014). Genome annotation and curation using MAKER and MAKER-P. Curr. Protoc. Bioinforma. 48, 4–11. doi: 10.1002/0471250953.bi0411s48
Chen, X.-R., Huang, S.-X., Zhang, Y., Sheng, G.-L., Zhang, B.-Y., Li, Q.-Y., et al. (2018). Transcription profiling and identification of infection-related genes in Phytophthora cactorum. Mol. Gen. Genomics. 293, 541–555. doi: 10.1007/s00438-017-1400-7
Cui, C., Wang, Y., Liu, J., Zhao, J., Sun, P., and Wang, S. (2019). A fungal pathogen deploys a small silencing RNA that attenuates mosquito immunity and facilitates infection. Nat. Commun. 10, 4298–4210. doi: 10.1038/s41467-019-12323-1
Dai, X., Zhuang, Z., and Zhao, P. X. (2018). psRNATarget: a plant small RNA target analysis server (2017 release). Nucleic Acids Res. 46, W49–W54. doi: 10.1093/nar/gky316
Derevnina, L., Petre, B., Kellner, R., Dagdas, Y. F., Sarowar, M. N., Giannakopoulou, A., et al. (2016). Emerging oomycete threats to plants and animals. Philos. Trans. R. Soc. B Biol. Sci. 371:20150459. doi: 10.1098/rstb.2015.0459
Domnas, A. J., Srebro, J. P., and Hicks, B. F. (1977). Sterol requirement for zoospore formation in the mosquito-parasitizing fungus Lagenidium giganteum. Mycologia 69, 875–886. doi: 10.1080/00275514.1977.12020142
Dunker, F., Trutzenberg, A., Rothenpieler, J. S., Kuhn, S., Pröls, R., Schreiber, T., et al. (2020). Oomycete small RNAs bind to the plant RNA-induced silencing complex for virulence. Elife 9:e56096. doi: 10.7554/eLife.56096
Eikemo, H., and Stensvand, A. (2015). Resistance of strawberry genotypes to leather rot and crown rot caused by Phytophthora cactorum. Eur. J. Plant Pathol. 143, 407–413. doi: 10.1007/s10658-015-0685-9
Emms, D. M., and Kelly, S. (2019). OrthoFinder: phylogenetic orthology inference for comparative genomics. Genome Biol. 20, 1–14. doi: 10.1186/s13059-019-1832-y
Fahlgren, N., Bollmann, S. R., Kasschau, K. D., Cuperus, J. T., Press, C. M., Sullivan, C. M., et al. (2013). Phytophthora have distinct endogenous small RNA populations that include short interfering and microRNAs. PLoS One 8:e77181. doi: 10.1371/journal.pone.0077181
Flynn, J. M., Hubley, R., Goubert, C., Rosen, J., Clark, A. G., Feschotte, C., et al. (2020). RepeatModeler2 for automated genomic discovery of transposable element families. Proc. Natl. Acad. Sci. U. S. A. 117, 9451–9457. doi: 10.1073/pnas.1921046117
Friedländer, M. R., MacKowiak, S. D., Li, N., Chen, W., and Rajewsky, N. (2012). MiRDeep2 accurately identifies known and hundreds of novel microRNA genes in seven animal clades. Nucleic Acids Res. 40, 37–52. doi: 10.1093/nar/gkr688
Gogleva, A., Drost, H.-G., and Schornack, S. (2018). SecretSanta: flexible pipelines for functional secretome prediction. Bioinformatics 34, 2295–2296. doi: 10.1093/bioinformatics/bty088
Gremme, G., Steinbiss, S., and Kurtz, S. (2013). Genome tools: a comprehensive software library for efficient processing of structured genome annotations. IEEE/ACM Trans. Comput. Biol. Bioinforma. 10, 645–656. doi: 10.1109/TCBB.2013.68
Gunderson, J. H., Elwood, H., Ingold, A., Kindle, K., and Sogin, M. L. (1987). Phylogenetic relationships between chlorophytes, chrysophytes, and oomycetes. Proc. Natl. Acad. Sci. U. S. A. 84, 5823–5827. doi: 10.1073/pnas.84.16.5823
Harren, K., and Tudzynski, B. (2013). Cch1 and Mid1 are functionally required for vegetative growth under low-calcium conditions in the phytopathogenic ascomycete Botrytis cinerea. Eukaryot. Cell 12, 712–724. doi: 10.1128/EC.00338-12
Haverkort, A. J., Boonekamp, P. M., Hutten, R., Jacobsen, E., Lotz, L. A. P., Kessel, G. J. T., et al. (2008). Societal costs of late blight in potato and prospects of durable resistance through cisgenic modification. Potato Res. 51, 47–57. doi: 10.1007/s11540-008-9089-y
Holoch, D., and Moazed, D. (2015). RNA-mediated epigenetic regulation of gene expression. Nat. Rev. Genet. 16, 71–84. doi: 10.1038/nrg3863
Horowitz, B. B., and Ospina-Giraldo, M. D. (2015). The pectin methylesterase gene complement of Phytophthora sojae: structural and functional analyses, and the evolutionary relationships with its oomycete homologs. PLoS One 10:e0142096. doi: 10.1371/journal.pone.0142096
Hu, X., Persson Hodén, K., Liao, Z., Åsman, A., and Dixelius, C. (2022). Phytophthora infestans Ago1-associated miRNA promotes potato late blight disease. New Phytol. 233, 443–457. doi: 10.1111/nph.17758
Huang, C. Y., Wang, H., Hu, P., Hamby, R., and Jin, H. (2019). Small RNAs – big players in plant-microbe interactions. Cell Host Microbe 26, 173–182. doi: 10.1016/j.chom.2019.07.021
Jia, J., Lu, W., Zhong, C., Zhou, R., Xu, J., Liu, W., et al. (2017). The 25–26 nt small RNAs in Phytophthora parasitica are associated with efficient silencing of homologous endogenous genes. Front. Microbiol. 8:773. doi: 10.3389/fmicb.2017.00773
Jones, P., Binns, D., Chang, H. Y., Fraser, M., Li, W., McAnulla, C., et al. (2014). InterProScan 5: genome-scale protein function classification. Bioinformatics 30, 1236–1240. doi: 10.1093/bioinformatics/btu031
Jung, T. (2009). Beech decline in Central Europe driven by the interaction between Phytophthora infections and climatic extremes. For. Pathol. 39, 73–94. doi: 10.1111/j.1439-0329.2008.00566.x
Jung, T., Blaschke, H., and Oßwald, W. (2000). Involvement of soilborne Phytophthora species in central European oak decline and the effect of site factors on the disease. Plant Pathol. 49, 706–718. doi: 10.1046/j.1365-3059.2000.00521.x
Kalyandurg, P. B., Sundararajan, P., Dubey, M., Ghadamgahi, F., Zahid, M. A., Whisson, S. C., et al. (2021). Spray-Induced Gene Silencing as a Potential Tool to Control Potato Late Blight Disease. Phytopathology 111, 2168–2175. doi: 10.1094/PHYTO-02-21-0054-SC
Kamoun, S., Furzer, O., Jones, J. D. G., Judelson, H. S., Ali, G. S., Dalio, R. J. D., et al. (2015). The top 10 oomycete pathogens in molecular plant pathology. Mol. Plant Pathol. 16, 413–434. doi: 10.1111/mpp.12190
Katoh, K., and Standley, D. M. (2013). MAFFT multiple sequence alignment software version 7: improvements in performance and usability. Mol. Biol. Evol. 30, 772–780. doi: 10.1093/molbev/mst010
Kerwin, J. L. (2007). Oomycetes: Lagenidium giganteum. J. Am. Mosq. Control Assoc. 23, 50–57. doi: 10.2987/8756-971X(2007)23[50:OLG]2.0.CO;2
Kopylova, E., Noé, L., and Touzet, H. (2012). SortMeRNA: fast and accurate filtering of ribosomal RNAs in metatranscriptomic data. Bioinformatics 28, 3211–3217. doi: 10.1093/bioinformatics/bts611
Krogh, A., Larsson, B., Von Heijne, G., and Sonnhammer, E. L. L. (2001). Predicting transmembrane protein topology with a hidden Markov model: application to complete genomes. J. Mol. Biol. 305, 567–580. doi: 10.1006/jmbi.2000.4315
Kushwaha, S. K., Vetukuri, R. R., and Grenville-Briggs, L. J. (2017a). Draft genome sequence of the mycoparasitic oomycete Pythium oligandrum strain CBS 530.74. Genome Announc. 5, e00346–e00317. doi: 10.1128/genomeA.00346-17
Kushwaha, S. K., Vetukuri, R. R., and Grenville-Briggs, L. J. (2017b). Draft genome sequence of the mycoparasitic oomycete Pythium periplocum strain CBS 532.74. Genome Announc. 5, e00057–e00017. doi: 10.1128/genomeA.00057-17
Langmead, B. (2010). Aligning short sequencing reads with bowtie. Curr. Protoc. Bioinforma Chapter 11:Unit 11.7. doi: 10.1002/0471250953.bi1107s32
Liang, D., Andersen, C. B., Vetukuri, R. R., Dou, D., and Grenville-Briggs, L. J. (2020). Horizontal gene transfer and tandem duplication shape the unique CAZyme complement of the mycoparasitic oomycetes Pythium oligandrum and Pythium periplocum. Front. Microbiol. 11:581698. doi: 10.3389/fmicb.2020.581698
Liao, Y., Smyth, G. K., and Shi, W. (2014). FeatureCounts: an efficient general purpose program for assigning sequence reads to genomic features. Bioinformatics 30, 923–930. doi: 10.1093/bioinformatics/btt656
Lilja, A., Rytkönen, A., and Hantula, J. (2011). Introduced pathogens found on ornamentals, strawberry and trees in Finland over the past 20 years. Agric. Food Sci. 20, 74–85. doi: 10.2137/145960611795163051
Liu, L., Xu, L., Jia, Q., Pan, R., Oelmüller, R., Zhang, W., et al. (2019). Arms race: diverse effector proteins with conserved motifs. Plant Signal. Behav. 14:1557008. doi: 10.1080/15592324.2018.1557008
Ma, X., Zuo, Z., Shao, W., Jin, Y., and Meng, Y. (2018). The expanding roles of Argonautes: RNA interference, splicing and beyond. Brief. Funct. Genomics 17, 191–197. doi: 10.1093/bfgp/elx045
Maniar, J. M., and Fire, A. Z. (2011). EGO-1, a C. elegans RdRP, modulates gene expression via production of mRNA-templated short antisense RNAs. Curr. Biol. 21, 449–459. doi: 10.1016/j.cub.2011.02.019
McKinney, W. (2010). Data structures for statistical computing in python. In Proceedings of the 9th Python in Science Conference 445, 51–56. doi: 10.25080/Majora-92bf1922-00a
Nakanishi, K., Weinberg, D. E., Bartel, D. P., and Patel, D. J. (2012). Structure of yeast Argonaute with guide RNA. Nature 486, 368–374.
Nguyen, L. T., Schmidt, H. A., Von Haeseler, A., and Minh, B. Q. (2015). IQ-TREE: a fast and effective stochastic algorithm for estimating maximum-likelihood phylogenies. Mol. Biol. Evol. 32, 268–274. doi: 10.1093/molbev/msu300
Ökmen, B., Bachmann, D., and De Wit, P. J. G. M. (2019). A conserved GH17 glycosyl hydrolase from plant pathogenic Dothideomycetes releases a DAMP causing cell death in tomato. Mol. Plant Pathol. 20, 1710–1721. doi: 10.1111/mpp.12872
Ospina-Giraldo, M. D., Griffith, J. G., Laird, E. W., and Mingora, C. (2010). The CAZyome of Phytophthora spp.: a comprehensive analysis of the gene complement coding for carbohydrate-active enzymes in species of the genus Phytophthora. BMC Genomics 11, 525–516. doi: 10.1186/1471-2164-11-525
Paul, B. (1999). Pythium periplocum, an aggressive mycoparasite of Botrytis cinerea causing the gray mould disease of grape-vine. FEMS Microbiol. Lett. 181, 277–280. doi: 10.1111/j.1574-6968.1999.tb08855.x
Petersen, T. N., Brunak, S., Von Heijne, G., and Nielsen, H. (2011). SignalP 4.0: discriminating signal peptides from transmembrane regions. Nat. Methods 8, 785–786. doi: 10.1038/nmeth.1701
Peterson, S. M., Thompson, J. A., Ufkin, M. L., Sathyanarayana, P., Liaw, L., and Congdon, C. B. (2014). Common features of microRNA target prediction tools. Front. Genet. 5:23. doi: 10.3389/fgene.2014.00023
Phillips, A. J., Anderson, V. L., Robertson, E. J., Secombes, C. J., and Van West, P. (2008). New insights into animal pathogenic oomycetes. Trends Microbiol. 16, 13–19. doi: 10.1016/j.tim.2007.10.013
Pierleoni, A., Martelli, P. L., and Casadio, R. (2008). PredGPI: a GPI-anchor predictor. BMC Bioinformatics 9, 1–11. doi: 10.1186/1471-2105-9-392
Piombo, E., Raju Vetukuri, R., Sundararajan, P., Kushwaha, S., Funck Jensen, D., Karlsson, M., et al. (2022). Comparative small RNA and Degradome sequencing provides insights into antagonistic interactions in the biocontrol fungus Clonostachys rosea. Appl. Environ. Microbiol. 88:e0064322. doi: 10.1128/aem.00643-22
Piombo, E., Vetukuri, R. R., Broberg, A., Kalyandurg, P. B., Kushwaha, S., Funck Jensen, D., et al. (2021). Role of dicer-dependent RNA interference in regulating mycoparasitic interactions. Microbiol. Spectr. 9, e01099–e01021. doi: 10.1128/Spectrum.01099-21
Prakash, A., Jeffryes, M., Bateman, A., and Finn, R. D. (2017). The HMMER web server for protein sequence similarity search. Curr. Protoc. Bioinforma. 60, 3–15. doi: 10.1002/cpbi.40
Qutob, D., Patrick Chapman, B., and Gijzen, M. (2013). Transgenerational gene silencing causes gain of virulence in a plant pathogen. Nat. Commun. 4:1349.
Rambaut, A. (2018). FigTree v. 1.4.4. Available at: http://tree.bio.ed.ac.uk/software/figtree/
Riffo-Campos, Á. L., Riquelme, I., and Brebi-Mieville, P. (2016). Tools for sequence-based miRNA target prediction: what to choose? Int. J. Mol. Sci. 17:1987. doi: 10.3390/ijms17121987
Roy, S., Kagda, M., and Judelson, H. S. (2013). Genome-wide prediction and functional validation of promoter motifs regulating gene expression in spore and infection stages of Phytophthora infestans. PLoS Pathog. 9:e1003182. doi: 10.1371/journal.ppat.1003182
Rueda, A., Barturen, G., Lebrón, R., Gómez-Martín, C., Alganza, Á., Oliver, J. L., et al. (2015). SRNAtoolbox: an integrated collection of small RNA research tools. Nucleic Acids Res. 43, W467–W473. doi: 10.1093/nar/gkv555
Sabbadin, F., Urresti, S., Henrissat, B., Avrova, A. O., Welsh, L. R. J., Lindley, P. J., et al. (2021). Secreted pectin monooxygenases drive plant infection by pathogenic oomycetes. Science 373, 774–779. doi: 10.1126/science.abj1342
Schoina, C., Rodenburg, S. Y. A., Meijer, H. J. G., Seidl, M. F., Lacambra, L. T., Bouwmeester, K., et al. (2021). Mining oomycete proteomes for metalloproteases leads to identification of candidate virulence factors in Phytophthora infestans. Mol. Plant Pathol. 22, 551–563. doi: 10.1111/mpp.13043
Shakywar, R. C., Pathak, S. P., Mahesh, P., Tomar, K. S., and Hem, S. (2013). Developmental behaviour of leaf blight of taro caused by Phytophthora colocasiae. Vegetos 26, 167–170. doi: 10.5958/j.2229-4473.26.1.024
Stanke, M., Keller, O., Gunduz, I., Hayes, A., Waack, S., and Morgenstern, B. (2006). AUGUSTUS: ab initio prediction of alternative transcripts. Nucleic Acids Res. 34, W435–W439. doi: 10.1093/nar/gkl200
Stanke, M., and Morgenstern, B. (2005). AUGUSTUS: a web server for gene prediction in eukaryotes that allows user-defined constraints. Nucleic Acids Res. 33, W465–W467. doi: 10.1093/nar/gki458
Swarts, D. C., Makarova, K., Wang, Y., Nakanishi, K., Ketting, R. F., Koonin, E. V., et al. (2014). The evolutionary journey of Argonaute proteins. Nat. Struct. Mol. Biol. 21, 743–753.
Tabima, J. F., and Grünwald, N. J. (2019). effectR: an expandable R package to predict candidate RxLR and CRN effectors in oomycetes using motif searches. Mol. Plant-Microbe Interact. 32, 1067–1076. doi: 10.1094/MPMI-10-18-0279-TA
Thines, M. (2014). Phylogeny and evolution of plant pathogenic oomycetes—a global overview. Eur. J. Plant Pathol. 138, 431–447. doi: 10.1007/s10658-013-0366-5
Vetukuri, R. R., Åsman, A. K. M., Tellgren-Roth, C., Jahan, S. N., Reimegård, J., Fogelqvist, J., et al. (2012). Evidence for small RNAs homologous to effector-encoding genes and transposable elements in the oomycete Phytophthora infestans. PLoS One 7:e51399. doi: 10.1371/journal.pone.0051399
Vetukuri, R. R., Avrova, A. O., Grenville-Briggs, L. J., van West, P., Söderbom, F., Savenkov, E. I., et al. (2011). Evidence for involvement of dicer-like, Argonaute and histone deacetylase proteins in gene silencing in Phytophthora infestans. Mol. Plant Pathol. 12, 772–785. doi: 10.1111/j.1364-3703.2011.00710.x
Wang, Q., Li, T., Zhong, C., Luo, S., Xu, K., Gu, B., et al. (2019). Small RNAs generated by bidirectional transcription mediate silencing of RXLR effector genes in the oomycete Phytophthora sojae. Phytopathol. Res. 1, 1–11. doi: 10.1186/s42483-019-0026-6
Wang, S., Vetukuri, R. R., Kushwaha, S. K., Hedley, P. E., Morris, J., Studholme, D. J., et al. (2021). Haustorium formation and a distinct biotrophic transcriptome characterize infection of Nicotiana benthamiana by the tree pathogen Phytophthora kernoviae. Mol. Plant Pathol. 22, 954–968. doi: 10.1111/mpp.13072
Wang, M., Weiberg, A., Lin, F. M., Thomma, B. P. H. J., Huang, H.Da, and Jin, H. (2016). Bidirectional cross-kingdom RNAi and fungal uptake of external RNAs confer plant protection. Nat. Plants 2, 16151–16110. doi: 10.1038/nplants.2016.151
Wang, H., Zhang, X., Liu, J., Kiba, T., Woo, J., Ojo, T., et al. (2011). Deep sequencing of small RNAs specifically associated with Arabidopsis AGO1 and AGO4 uncovers new AGO functions. Plant J. 67, 292–304. doi: 10.1111/j.1365-313X.2011.04594.x
Wu, H.-J., Ma, Y.-K., Chen, T., Wang, M., and Wang, X.-J. (2012). PsRobot: a web-based plant small RNA meta-analysis toolbox. Nucleic Acids Res. 40, W22–W28. doi: 10.1093/nar/gks554
Zhang, Z., Liu, X., Guo, X., Wang, X.-J., and Zhang, X. (2016). Arabidopsis AGO3 predominantly recruits 24-nt small RNAs to regulate epigenetic silencing. Nat. Plants 2, 1–7. doi: 10.1038/nplants.2016.49
Zhang, H., Yohe, T., Huang, L., Entwistle, S., Wu, P., Yang, Z., et al. (2018). DbCAN2: a meta server for automated carbohydrate-active enzyme annotation. Nucleic Acids Res. 46, W95–W101. doi: 10.1093/nar/gky418
Keywords: Phytophthora, Pythium, Lagenidium giganteum, miRNA, sRNA, transposons, RNA silencing, biocontrol
Citation: Piombo E, Kelbessa BG, Sundararajan P, Whisson SC, Vetukuri RR and Dubey M (2023) RNA silencing proteins and small RNAs in oomycete plant pathogens and biocontrol agents. Front. Microbiol. 14:1076522. doi: 10.3389/fmicb.2023.1076522
Received: 21 October 2022; Accepted: 03 March 2023;
Published: 24 March 2023.
Edited by:
Sabrina Sarrocco, University of Pisa, ItalyReviewed by:
Alfredo Herrera-Estrella, National Polytechnic Institute of Mexico (CINVESTAV), MexicoCopyright © 2023 Piombo, Kelbessa, Sundararajan, Whisson, Vetukuri and Dubey. This is an open-access article distributed under the terms of the Creative Commons Attribution License (CC BY). The use, distribution or reproduction in other forums is permitted, provided the original author(s) and the copyright owner(s) are credited and that the original publication in this journal is cited, in accordance with accepted academic practice. No use, distribution or reproduction is permitted which does not comply with these terms.
*Correspondence: Ramesh Raju Vetukuri, UmFtZXNoLlZldHVrdXJpQHNsdS5zZQ==; Mukesh Dubey, TXVrZXNoLkR1YmV5QHNsdS5zZQ==
†These authors have contributed equally to this work and share last authorship
Disclaimer: All claims expressed in this article are solely those of the authors and do not necessarily represent those of their affiliated organizations, or those of the publisher, the editors and the reviewers. Any product that may be evaluated in this article or claim that may be made by its manufacturer is not guaranteed or endorsed by the publisher.
Research integrity at Frontiers
Learn more about the work of our research integrity team to safeguard the quality of each article we publish.