- 1School of Mathematics and Statistics, Hubei Normal University, Huangshi, China
- 2School of Computational Science and Electronics, Hunan Institute of Engineering, Xiangtan, China
People with coronavirus disease 2019 (COVID-19) have different mortality or severity, and this clinical outcome is thought to be mainly attributed to comorbid cardiovascular diseases. However, genetic loci jointly influencing COVID-19 and cardiovascular disorders remain largely unknown. To identify shared genetic loci between COVID-19 and cardiac traits, we conducted a genome-wide cross-trait meta-analysis. Firstly, from eight cardiovascular disorders, we found positive genetic correlations between COVID-19 and coronary artery disease (CAD, Rg = 0.4075, P = 0.0031), type 2 diabetes (T2D, Rg = 0.2320, P = 0.0043), obesity (OBE, Rg = 0.3451, P = 0.0061), as well as hypertension (HTN, Rg = 0.233, P = 0.0026). Secondly, we detected 10 shared genetic loci between COVID-19 and CAD, 3 loci between COVID-19 and T2D, 5 loci between COVID-19 and OBE, and 21 loci between COVID-19 and HTN, respectively. These shared genetic loci were enriched in signaling pathways and secretion pathways. In addition, Mendelian randomization analysis revealed significant causal effect of COVID-19 on CAD, OBE and HTN. Our results have revealed the genetic architecture shared by COVID-19 and CVD, and will help to shed light on the molecular mechanisms underlying the associations between COVID-19 and cardiac traits.
Introduction
The coronavirus disease 2019 (COVID-19) arises from severe acute respiratory syndrome coronavirus 2 (SARS-CoV-2) infection, and it rapidly outbreak since November 2019 and recently become a public health emergency of international concern (The Severe Covid-19 Gwas Group, 2020). Up to now, there have been more than 170 million confirmed cases and nearly 3.9 million deaths globally. However, its etiology is not fully understood.
People with COVID-19 have different mortality or severity, and the clinical outcome are worse in patients with cardiovascular related disorders, which suggests the comorbidity of COVID-19 and cardiovascular diseases (CVD) (Guan et al., 2020b). More evidences showed the concordant result (Guan et al., 2020a; Ruan et al., 2020; Sisnieguez et al., 2020; Wang et al., 2020; Yang et al., 2020). On the one hand, it was reported that hypertension (21.1%) and diabetes (9.7%) ranked as the top two most prevalent comorbidities for COVID-19 (Wang et al., 2020). The odd ratios of hypertension (2.36) and coronary heart disease (3.42) were larger than 1 when comparing severe COVID-19 patients to non-severe cases (Yang et al., 2020). On the one hand, genome-wide association studies (GWAS) have identified several associated-variants involved in COVID-19 and cardiovascular disease-related traits. For example, a gene known as ERI3 has been associated with COVID-19 related mortality, coronary artery disease and type 2 diabetes (MacArthur et al., 2017). Moreover, COVID-19 cardiovascular epidemiology showed that nearly 12% of COVID-19 cases have been found to have sustained cardiac injuries, COVID-19 might have a direct and indirect effect on the cardiovascular system (Tajbakhsh et al., 2021). The etiologic agent of COVID-19 can infect the heart, vascular tissues, and circulating cells through the host cell receptor for the viral spike protein (Chung et al., 2021). All the above studies lead us to wonder whether the comorbidity between COVID-19 and CVD is due to the potential shared genetic factors. However, there is few genetic study to reveal the common genetic architecture between COVID-19 and CVD. To this end, the goal of this study was to identify genetic loci shared between COVID-19 and cardiac traits by conducting a large-scale genome-wide cross-trait meta-analysis, and provide more knowledge about common molecular mechanisms of them.
Our study mainly includes three parts. Firstly, we estimated both the overall and local genetic correlation between COVID-19 and eight cardiac traits, including coronary artery disease (CAD), type 2 diabetes (T2D), hypertension (HTN), obesity (OBE), high-density lipoproteins (HDL), low-density lipoproteins (LDL), triglycerides (TC), and total cholesterol (TG). Secondly, we carried out a large-scale cross-trait meta-analysis to identify shared genetic loci between trait pairs that showed significant genetic correlation in the first part of the study. Finally, we conducted transcriptome-wide association study (TWAS), pathway enrichment analysis and Mendelian randomization (MR) analysis to obtain more biological insight.
The overall study design is shown in Figure 1.
Materials and methods
Data sources
The GWAS summary statistic for COVID-19 was extracted from the Genetics of Mortality in Critical Care (GenOMICC) study, which performed GWAS on 2244 critically ill patients with COVID-19 in 208 UK intensive care units (Pairo-Castineira et al., 2021). We downloaded the summary statistic with European cases vs UK Biobank controls in this study. We also retrieved the summary statistics of eight cardiac traits in the following public available datasets. The summary statistic for CAD was from the Coronary ARtery DIsease Genome Wide Replication and Meta-analysis plus The Coronary Artery Disease Genetics (CARDIoGRAMplusC4D) Consortium (60,801 cases and 123,504 controls) (Nikpay et al., 2015). The summary statistic for T2D was from the Diabetes Genetics Replication and Meta-Analysis (DIAGRAM) Consortium (26,676 cases and 132,532 controls) (Scott et al., 2017). The summary statistic for OBE was from the Genetic Investigation of ANthropometric Traits (GIANT) Consortium (32,858 cases and 65,839 controls) (Berndt et al., 2013). The summary statistic for HTN was from the Genome wide association study ATLAS (GWASATLAS) database (99,665 cases and 189,642 controls) (Watanabe et al., 2019). The summary statistics for four lipid traits (LDL, HDL, TC, and TG) were from the Global Lipids Genetics Consortium (GLGC) Consortium (188,577 samples) (Willer et al., 2013). The details of each summary statistic dataset are provided in Supplementary Table 1.
Genome-wide genetic correlation analysis
We employed the high-definition likelihood methodology (Ning et al., 2020) to estimate the genetic correlation between COVID-19 and eight cardiac traits. This approach provides more accurate estimation by fully accounting for linkage disequilibrium (LD) information across the whole genome. The χ2 statistic of single nucleotide polymorphisms (SNPs) in high LD regions is higher than that of those in low LD regions, and similar results are observed by replacing one study test statistic with the product of two z-scores in the study. We used the reference panel with imputed HapMap3 SNPs, which are based on genotypes in UK Biobank.
Local genetic correlation
We applied ρ-HESS (Shi et al., 2017) to investigate whether COVID-19 and cardiac traits show local genetic correlation. ρ-HESS quantifies the correlation between traits at each LD-independent region of the genome due to genetic variation. A total of approximately 1.5 Mb was used for estimating local genetic heritabilities and genetic covariances from independent LD blocks. We chose the cardiac traits that showed significant genetic correlation with COVID-19 in this analysis, thus, four pairs of traits were included (COVID-19 and CAD, COVID-19 and T2D, COVID-19 and OBE, COVID-19 and HTN). Notice that we removed the empty loci (with no SNP in it) in each local region in ρ-HESS.
Cross-trait meta-analysis
We conducted a large-scale cross-trait meta-analysis to identify genetic loci shared between severe COVID-19 and cardiac traits that showed significant genetic correlation, using PLEIO framework (Lee et al., 2021). PLEIO is a summary-statistics approach to mapping pleiotropic loci in a multiple trait analysis, either binary, quantitative, independent or correlated traits. Besides, this method can maximize power by adequately modeling the genetic architectures (genetic correlation and heritability) and control false positive rate by accounting for environmental correlation. SNPs with Pmeta < 5 × 10–8 and trait-specific P < 0.05 were considered to be significant for both traits. We performed the operations on a computer of Intel Xeon E5-2695 CPU 2.10 GHz. For each disease pair, it will waste 8–10 mins for the standardization of raw summary statistics first, and then about 2 mins for the identification of pleiotropic loci with PLEIO.
The independent loci were identified using the clumping function of PLINK (version 1.9) tool (Purcell et al., 2007) with clumping parameters p1 = 5 × 10–8, p2 = 1 × 10–5, r2 = 0.1, and kb = 500, that is, SNPs with p value less than 1 × 10–5, r2 greater than 0.1 and distance less than 500 kb from the peak will be assigned to the clump with that peak. Distance to the nearest gene was calculated using NCBI human genome build37 gene annotation.
Transcriptome-wide association study
We performed transcriptome-wide association study (TWAS) to detect gene expression associations in specific tissues for COVID-19 and cardiac traits, using FUSION software (Gusev et al., 2016) based on 43 Genotype-Tissue Expression Project (GTEx: version 6) tissue expression weights. FUSION is a powerful strategy that uses cis-regulated gene expression measurements to identify genes associated with complex traits through large-scale summary statistics. TWAS p values for each trait were corrected for multiple testing by using Benjamini-Hochberg’s False Discovery Rate (FDR) procedure (FDR < 0.05).
Pathway enrichment analysis
To obtain biological insight for shared risk genes that were identified from cross-trait meta-analysis, we used Enrichr tool (Kuleshov et al., 2016) to perform Kyoto Encyclopedia of Genes and Genomes (KEGG) pathway enrichment analysis. The Benjamini-Hochberg procedure was used on p value to account for multiple testing.
Mendelian randomization analysis
In order to examine the causal relationships between COVID-19 and cardiac traits, we conducted MR analysis using MR-PRESSO test (Verbanck et al., 2018). The MR-PRESSO method estimates exposure effects in multi-instrument MR using SNPs significantly associated with exposure, as well as horizontal pleiotropy in multi-instrument MR utilizing summary statistics. Instruments were constructed using LD-independent SNPs with p values lower than 5 × 10–8.
Results
Overall and local genetic correlations between coronavirus disease 2019 and cardiac traits
We estimated the genetic correlation between COVID-19 and eight cardiac traits using high-definition likelihood method. Four out of eight cardiac traits showed strong and significant genetic correlation with COVID-19. There was the strongest genetic correlation between COVID-19 and CAD (Rg = 0.4075, P = 0.0031), followed by T2D and HTN in a similar magnitude (Rg = 0.232, P = 0.0043 and Rg = 0.233, P = 0.0026, respectively). Moreover, a positive genetic correlation was also found with COVID-19 in OBE (Rg = 0.3451, P = 0.0061). However, no significant genetic correlation was found between COVID-19 and four lipid traits (LDL, HDL, TC, and TG). The detailed results of genetic correlation are displayed in Table 1.
Due to the significant genetic correlation between COVID-19 and four cardiac traits (CAD, T2D, OBE, and HTN), we conducted ρ-HESS to explore whether there is a genetic correlation between COVID-19 and cardiac traits in certain regions of the genome. Result of the COVID-19/CAD trait pair showed that the 19p13.2 region (chromosome 19: 9238393-11284028) had strong local genetic correlation (P = 3.76 × 10–6). Besides, result of the COVID-19/T2D trait pair showed strong local genetic correlation (P = 1.39 × 10–7) in the 4q21.23 region (chromosome 4: 83372593-84799656). We did not find significant local genetic correlations for neither COVID-19/HTN nor COVID-19/OBE trait pair (Figure 2).
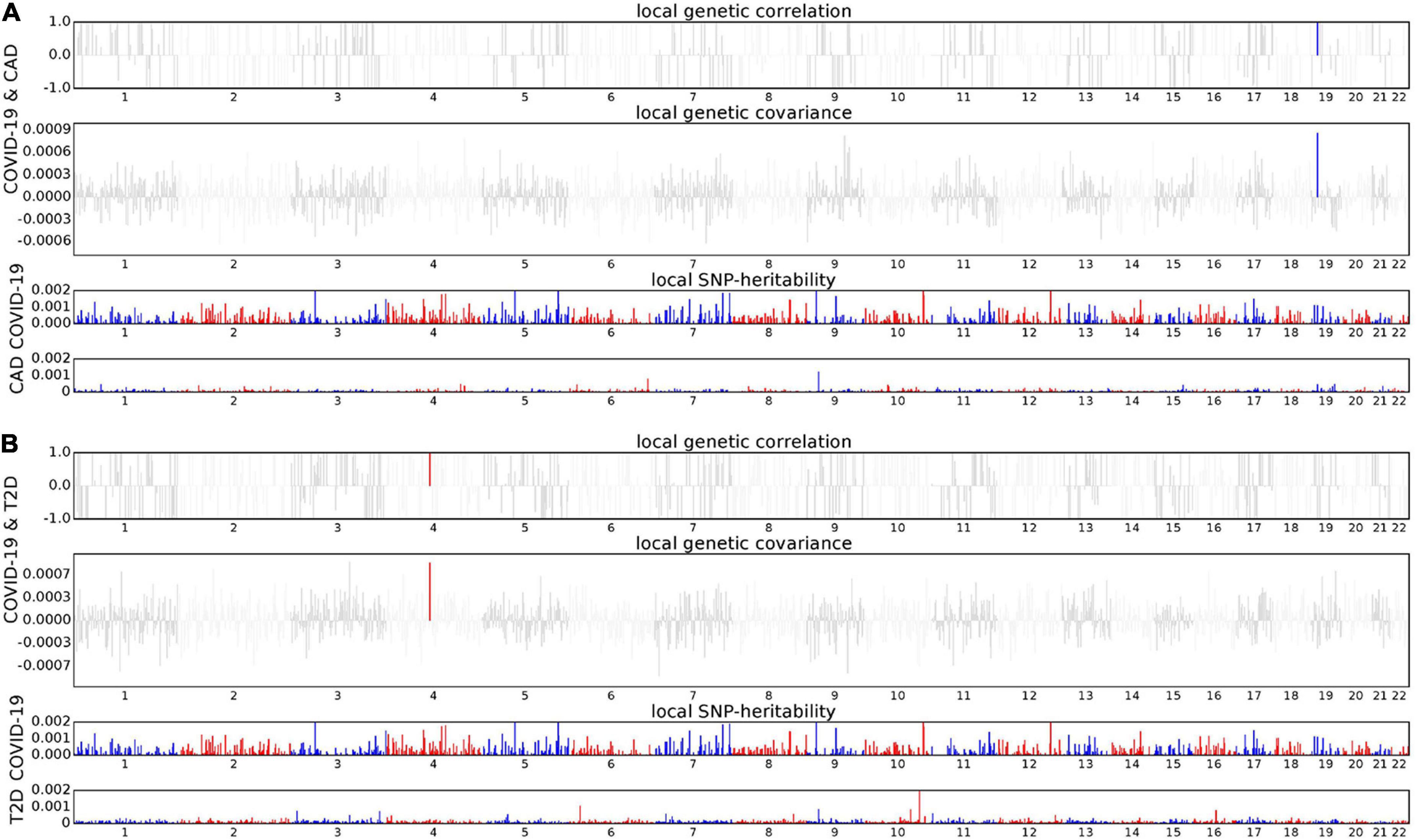
Figure 2. Local genetic correlation and local SNP-heritability between COVID-19 and CAD (A), T2D (B), respectively. For each subfigure, the top part represents local genetic correlation, the middle part represents local genetic covariance, and blue or red highlights indicate significant local genetic correlation and covariance after multiple testing correction, the bottom part represents local SNP heritability for each trait.
Cross-trait meta-analysis results between coronavirus disease 2019 and cardiac traits
We performed a large-scale genome-wide cross-trait meta-analysis to improve the statistical power to identify shared genetic loci between COVID-19 and four cardiac traits that show significant genetic correlations. We considered SNPs with Pmeta < 5 × 10–8 and trait-specific P < 0.05 to be significant for both COVID-19 and cardiac traits. Based on these criteria, we identified 39 independent loci significantly associated with COVID-19 and cardiac traits, of which eight loci failed to be detected in trait-specific GWAS of COVID-19 and cardiac traits (Tables 2, 3).
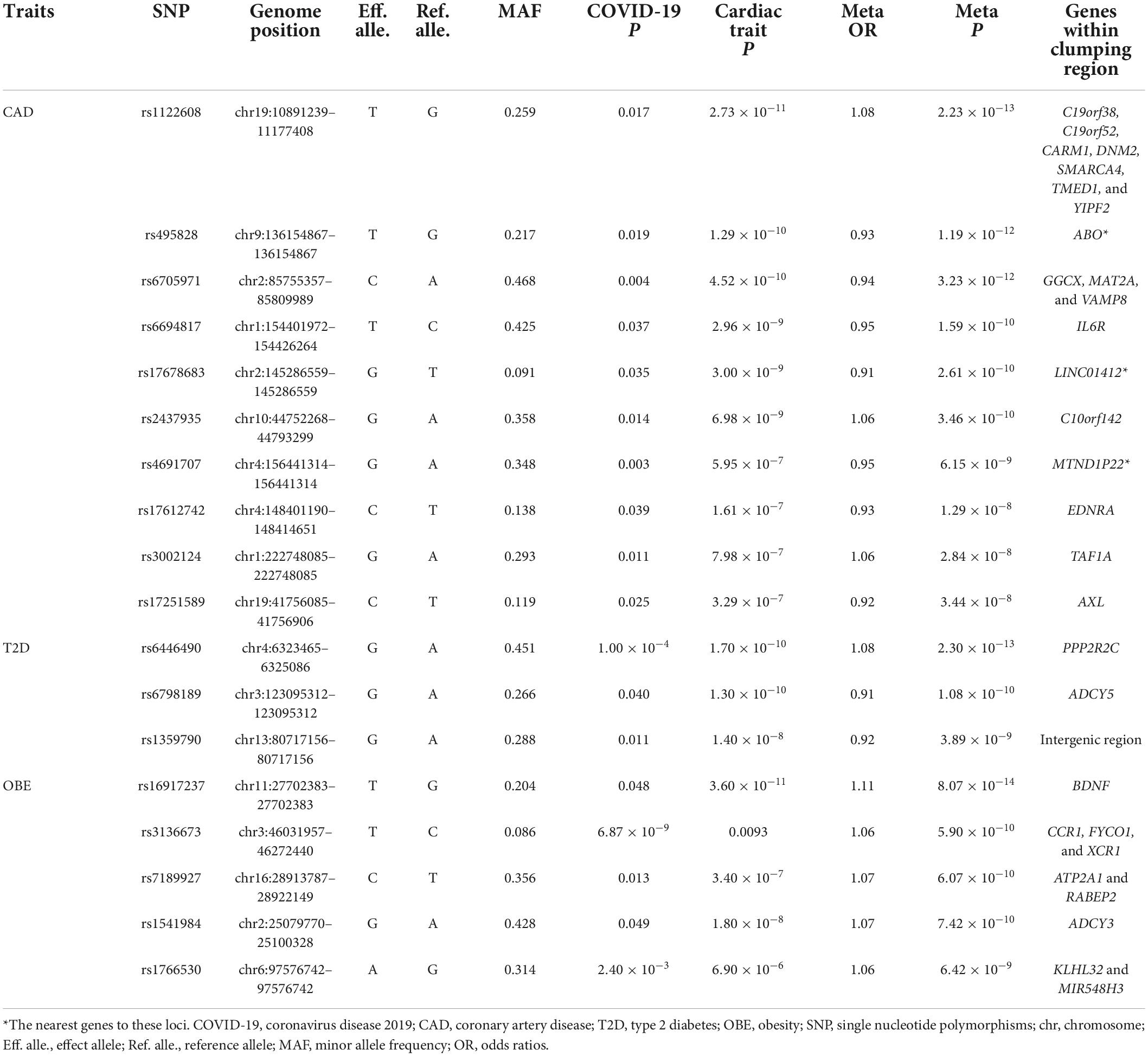
Table 2. Cross-trait meta-analysis results between coronavirus disease 2019 and CAD, T2D, and OBE (Pmeta < 5 × 10–8; single trait P < 0.05).
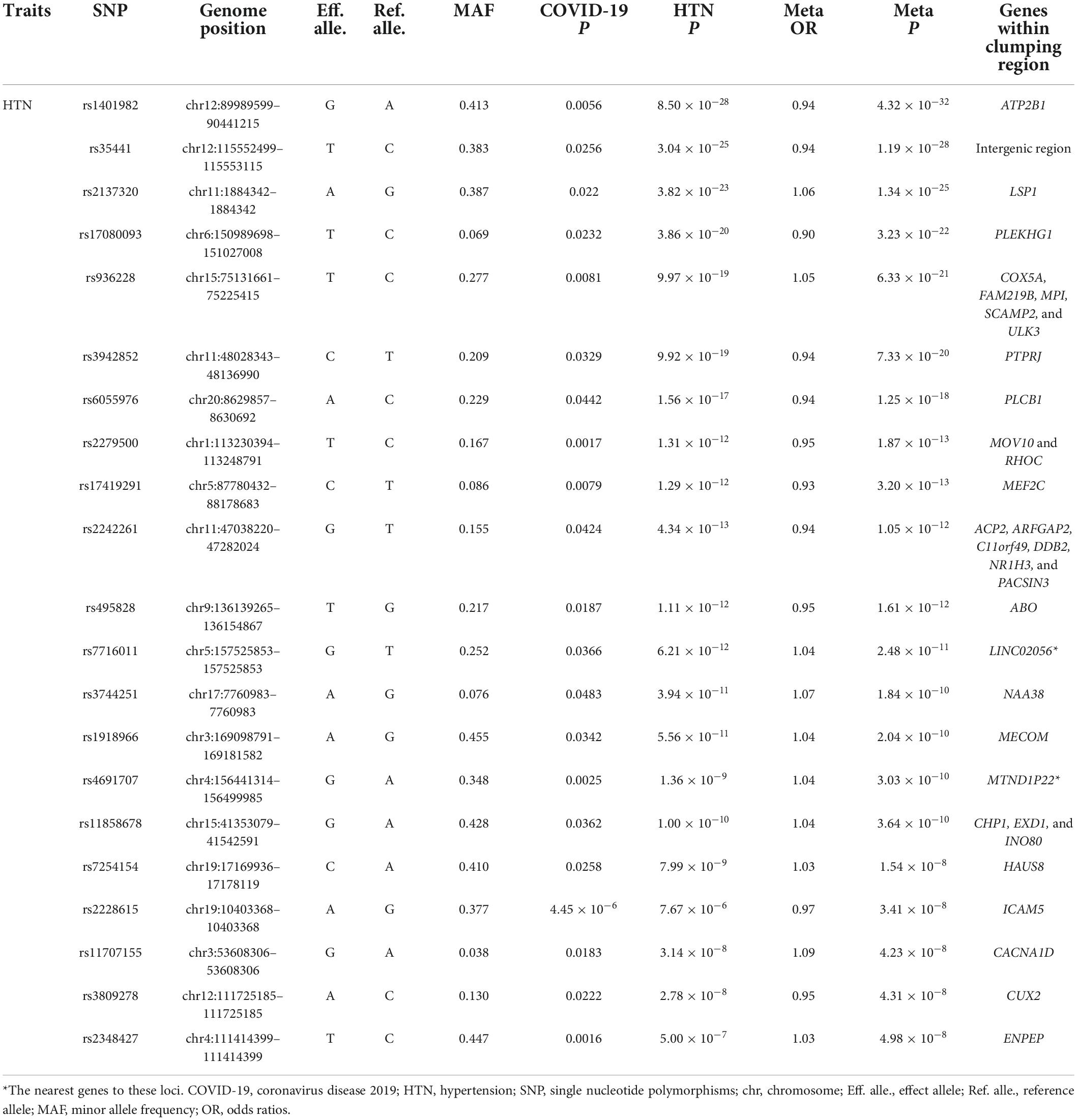
Table 3. Cross-trait meta-analysis result between coronavirus disease 2019 and HTN (Pmeta < 5 × 10–8; single trait P < 0.05).
We observed two overlapped significant loci in the cross-trait meta-analysis of COVID-19/CAD and COVID-19/HTN. The first association signal was 9q34.2 (index SNP: rs495828, Pmeta = 1.19 × 10–12 for COVID-19/CAD; Pmeta = 1.61 × 10–12 for COVID-19/HTN). This locus was located at the ABO blood group, which contributed to the immunopathogenesis of SARS-CoV-infection (The Severe Covid-19 Gwas Group, 2020). Similarly, it was concluded that group A individuals had a higher risk of COVID-19 respiratory failure while group O individuals had a protective effect via blood type-specific analysis (Deleers et al., 2021). The other locus (index SNP: rs4691707, Pmeta = 6.15 × 10–9 for COVID-19/CAD; Pmeta = 3.03 × 10–10 for COVID-19/HTN) was in the intergenic region closet to the MTND1P22 gene, which may have a role in transcription regulation.
In addition to rs495828 and rs4691707, a further eight loci were identified to be associated with COVID-19 and CAD (Table 2). The strongest association signal (index SNP: rs1122608, Pmeta = 2.23 × 10–13) was found near gene SMARCA4 on chromosome 19, which was previously reported to regulate atherosclerosis (Ma et al., 2019) and play a protective role to against the risk of HTN (Xiong et al., 2014).
Three loci were identified in a cross-trait meta-analysis of COVID-19 and T2D (Table 2). The first locus (index SNP: rs6446490, Pmeta = 2.30 × 10–13) was mapped on PPP2R2C, a gene that increased insulin resistance (Daily et al., 2019). The second locus represented by rs6798189 (Pmeta = 1.08 × 10–10) was mapped on ADCY5, a gene coupled glucose to insulin secretion in human islets (Hodson et al., 2014). The third locus (index SNP: rs1359790, Pmeta = 3.89 × 10–9) located in intergenic region, which was previously reported to be associated with T2D (Flannick et al., 2019).
We also found five significant loci that were associated with both COVID-19 and OBE (Table 2). The top locus (index SNP: rs16917237, Pmeta = 8.07 × 10–14) was mapped on BDNF, a gene was not only associated with body mass index but also CAD (Winkler et al., 2015; van der Harst and Verweij, 2018). The second locus (index SNP: rs3136673, Pmeta = 5.90 × 10–10) was originally significant associated with COVID-19 (P = 6.87 × 10–9), the mapped gene CCR1 involved in heart and blood communication in cardiac diseases.
In the cross-trait meta-analysis of COVID-19 and HTN, we identified 21 significant loci (Table 3). One of the most important loci is characterized by the ATP2B1 gene (index SNP: rs1401982, Pmeta = 4.32 × 10–32), which plays a key role in regulating blood pressure by altering calcium handling and vasoconstriction in vascular smooth muscle cells (Wain et al., 2011).
Results of transcriptome-wide association analysis, pathway enrichment analysis, and Mendelian randomization analysis
To identify association between COVID-19 and cardiac traits with gene expression in specific tissue, we performed TWAS in 43 GTEx tissues. A total of 20 gene-tissue pairs were significantly associated with COVID-19, in addition to 263 gene-tissue pairs with CAD, 142 gene-tissue pairs with T2D, 2030 gene-tissue pairs with HTN, and 256 gene-tissue pairs with OBE (Supplementary Tables 2–6). There is no gene-tissue pair overlapped between COVID-19 and the four cardiac traits in TWAS.
To investigate the biological pathways represented by shared genes, we assessed enrichment of shared genes between COVID-19 and cardiac traits. KEGG pathway enrichment analysis revealed cGMP-PKG signaling pathway as the most significant pathway, as well as other signaling pathways and secretion pathways (Figure 3).
We identified three significant causal relationships by using MR-PRESSO test, including the effect of COVID-19 on CAD (causal estimate = 0.0045, P = 3.70 × 10–6), OBE (causal estimate = 0.0494, P = 1.86 × 10–4), and HTN (causal estimate = 0.0019, P = 1.20 × 10–6). However, we did not observed causal effect of COVID-19 on T2D (causal estimate = –0.0026, P = 0.2281; Supplementary Table 7).
Discussion
To the best of our knowledge, this is the study to identify shared genetic architecture between COVID-19 and cardiac traits. Specifically, we found substantial and significant genetic correlation between COVID-19 and CAD, T2D, OBE, and HTN. These findings are consistent with the study which estimated the genetic correlation by LD score regression method (Chang et al., 2021), and further confirmed the fact that patients with certain underlying medical conditions (such as CAD, T2D, OBE, and HTN) are at increased risk for poor outcome in COVID-19 (Richardson et al., 2020).
In the original GWAS summary statistics, there were hundreds to thousands of significant loci (P < 5 × 10–8) in each of these diseases. However, no shared genetic locus was found between COVID-19 and any of the four cardiac traits. After cross-trait meta-analysis, we identified 10 shared loci between COVID-19 and CAD, three shared loci between COVID-19 and T2D, five shared loci between COVID-19 and OBE, and 21 shared locus between COVID-19 and HTN. This series of comparative data highlights the superiority of cross-trait meta-analysis. These shared genetic loci could be used to predict the occurrence of COVID-19 as well as the abnormal cardiac traits. In addition, we identified eight loci that failed to reach significance in trait-specific GWAS, demonstrating cross-trait meta-analysis’ excellent statistical power similarly.
We performed GWAS-Catalog analysis to understand whether the shared genes have been reported in the previous studies (Supplementary Table 8). Gene ABO, mapped by the locus rs495828 in 9q34.2 region, was reported to be associated with COVID-19, CAD, OBE and HTN (Covid-19 Host Genetics Initiative., 2021). Additionally three genes (CCR1, FYCO1, and XCR1) were not only associated with COVID-19, but also at least two cardiac traits (Shelton et al., 2021). Beyond them, other shared genes were newly found. In terms of gene function, ABO, which determines blood type, may affect COVID-19 disease severity, but there was no evidence to confirm ABO blood group influences risk of COVID-19 infection or outcome (Lehrer and Rheinstein, 2021). Genes CCR1, FYCO1, and XCR1 were involved in T-cell and dendritic-cell function (Kaser, 2020).
In the local genetic correlation analysis between COVID-19 and cardiac traits that showed significant genetic correlation, we found that SMARCA4 region to have genetic correlation between COVID-19 and CAD, which was also identified by cross-trait meta-analysis. SMARCA4 is a well-known gene associated with CAD, and it mediated nucleosome remodeling which was considered another epigenetic mechanism that can affect the course of COVID-19 (Peng et al., 2020; Shirvaliloo, 2021). Moreover, we also identified HELQ region to be significantly associated with COVID-19 and T2D. HELQ is predominantly known for its ATP-dependent helicase activity and participation in DNA repair.
Post-GWAS function analyses provided biological insights into the shared genes between COVID-19 and four cardiac traits. In TWAS analysis, we detected 20 significant gene-tissue pair associated with COVID-19, 263 with CAD, 142 with T2D, 256 with OBE and 2030 with HTN. Of these, none of the gene-tissue pair significantly associated with COVID-19 and cardiac traits. In addition, we also performed GTEx tissue enrichment analysis, and did not identify any enrichment signal in tissues. These results suggest that the distribution of pleiotropic genes between COVID-19 and cardiac traits is scattered and not limited to a specific tissue. Moreover, KEGG pathway enrichment analysis showed that the shared genes enriched in some signaling pathways and secretion pathways, such as cGMP-PKG signaling pathway, pancreatic secretion and insulin secretion. The recent studies reported that signaling pathways significantly related to COVID-19 (Messina et al., 2021; Wang et al., 2021), and secretion pathways significantly related to cardiovascular diseases (Chae and Kwon, 2019).
Our MR analysis showed causal effect of COVID-19 on CAD, OBE, and HTN, these findings supported the idea that the genetic correlation of polygenic diseases may be due to both causality and pleiotropy (van Rheenen et al., 2019). Moreover, there is no causal relationship between COVID-19 and T2D, this result indicated the shared genetic effect between COVID-19 and T2D is more likely to be pleiotropic effect, rather than causal effect or mechanism.
As well as genetic factors, environmental factors and lifestyle also play an important role in the comorbidity of COVID-19 and cardiac traits. Although there are many studies on screening anti-SARS-CoV-2 drugs and discoverying potential therapeutic drugs for COVID-19 (Zhou et al., 2020; Peng et al., 2021; Shen et al., 2022; Tian et al., 2022), home quarantine and staying away from infection prevention, vaccination, appropriate immunomodulatory diet and drugs that modulate cardiovascular system are currently the most effective approach to prevention (Lotfi et al., 2020).
We also acknowledge some limitations of our work. First, we restricted the analysis to the participants of European ancestors to avoid population stratification, so the findings may not be applicable to general populations. Second, we observed some positive genetic correlation between COVID-19 and TG as well as negative genetic correlation between COVID-19 and HDL, but they failed to reach the standard significant level. The genetic relationship between severe COVID-19 and lipid traits deserves further study. Third, although large sample cohorts were used in this study, we did not perform replication with other COVID-19 cohorts, which would be meaningful to confirm our findings.
Conclusion
In conclusion, our genome-wide cross-trait meta-analysis confirmed the association between COVID-19 and cardiovascular disorders. Investigation of the shared genetic loci between COVID-19 and cardiac traits can be helpful to understand the common biological mechanisms underlying the comorbidity.
Data availability statement
The original contributions presented in this study are included in the article/Supplementary material, further inquiries can be directed to the corresponding author.
Author contributions
HG conceived the project, conducted data analysis, and wrote the manuscript. HW performed the methodology and software. TL participated in the discussion and revised the manuscript. All authors contributed to the article and approved the submitted version.
Funding
This research was funded by Natural Science Foundation of Hubei Province (Grant No. 2021CFB197), Young Talents Project of Scientific Research Plan of Hubei Provincial Department of Education (Grant No. Q20212506), and Talent Introduction Project of Hubei Normal University in 2021 (Grant No. HS2021RC013).
Acknowledgments
The authors would like to thank the reviewers and editors for the valuable comments and suggestions.
Conflict of interest
The authors declare that the research was conducted in the absence of any commercial or financial relationships that could be construed as a potential conflict of interest.
Publisher’s note
All claims expressed in this article are solely those of the authors and do not necessarily represent those of their affiliated organizations, or those of the publisher, the editors and the reviewers. Any product that may be evaluated in this article, or claim that may be made by its manufacturer, is not guaranteed or endorsed by the publisher.
Supplementary material
The Supplementary Material for this article can be found online at: https://www.frontiersin.org/articles/10.3389/fmicb.2022.993933/full#supplementary-material
References
Berndt, S. I., Gustafsson, S., Mägi, R., Ganna, A., Wheeler, E., Feitosa, M. F., et al. (2013). Genome-wide meta-analysis identifies 11 new loci for anthropometric traits and provides insights into genetic architecture. Nat. Genet. 45, 501–512. doi: 10.1038/ng.2606
Chae, C. W., and Kwon, Y. W. (2019). Cell signaling and biological pathway in cardiovascular diseases. Arch. Pharmacal. Res. 42, 195–205. doi: 10.1007/s12272-019-01141-0256
Chang, X., Li, Y., Nguyen, K., Qu, H., Liu, Y., Glessner, J., et al. (2021). Genetic correlations between COVID-19 and a variety of traits and diseases. Innovation 2:100112. doi: 10.1016/j.xinn.2021.100112
Chung, M. K., Zidar, D. A., Bristow, M. R., Cameron, S. J., Chan, T., Harding, C. V., et al. (2021). COVID-19 and cardiovascular disease: From bench to beside. Circul. Res. 128, 1214–1236. doi: 10.1161/CIRCRESAHA.121.317997
Covid-19 Host Genetics Initiative. (2021). Mapping the human genetic architecture of COVID-19. Nature 600, 472–477. doi: 10.1038/s41586-021
Daily, J., Liu, M., and Parkb, S. (2019). High genetic risk scores of SLIT3, PLEKHA5 and PPP2R2C variants increased insulin resistance and interacted with coffee and caffeine consumption in middle-aged adults. Nut. Metabolism Cardiovasc. Dis. 29, 79–89. doi: 10.1016/j.numecd.2018.09
Deleers, M., Breiman, A., Daubie, V., Maggetto, C., Barreau, I., Besse, T., et al. (2021). Covid-19 and blood groups: ABO antibody levels may also matter. Int. J. Infect. Dis. 104, 242–249. doi: 10.1016/j.ijid.2020.12.025
Flannick, J., Mercader, J. M., Fuchsberger, C., Udler, M. S., Mahajan, A., Wessel, J., et al. (2019). Exome sequencing of 20791 cases of type 2 diabetes and 24440 controls. Nature 570, 71–76. doi: 10.1038/s41586-019-1231-2
Guan, W., Liang, W., He, J., and Zhong, N. (2020a). Cardiovascular comorbidity and its impact on patients with COVID-19. Eur. Respiratory J. 55:2001227. doi: 10.1183/13993003.01227-2020
Guan, W., Liang, W., Zhao, Y., Liang, H., Chen, Z., Li, Y., et al. (2020b). Comorbidity and its impact on 1590 patients with COVID-19 in china: A nationwide analysis. Eur. Respiratory J. 55:2000547. doi: 10.1183/13993003.00547-2020
Gusev, A., Ko, A., Shi, H., Bhatia, G., Chung, W., Penninx, B. W. J. H., et al. (2016). Integrative approaches for large-scale transcriptome-wide association studies. Nat. Genet. 48, 245–252. doi: 10.1038/ng.3506
Hodson, D. J., Mitchell, R. K., Marselli, L., Pullen, T. J., Brias, S. G., Semplici, F., et al. (2014). ADCY5 couples glucose to insulin secretion in human islets. Diabetes 63, 3009–3021. doi: 10.2337/db13-1607
Kaser, A. (2020). Genetic risk of severe Covid-19. New England J. Med. 383, 1590–1591. doi: 10.1056/NEJMe2025501
Kuleshov, M. V., Jones, M. R., Rouillard, A. D., Fernandez, N. F., Duan, Q., Wang, Z., et al. (2016). Enrichr: A comprehensive gene set enrichment analysis web server 2016 update. Nucleic Acids Res. 44:W90–W97. doi: 10.1093/nar/gkw377
Lee, C. H., Shi, H., Pasaniuc, B., Eskin, E., and Han, B. (2021). PLEIO: A method to map and interpret pleiotropic loci with GWAS summary statistics. Am. J. Hum. Genet. 108, 36–48. doi: 10.1016/j.ajhg.2020.11.017
Lehrer, S., and Rheinstein, P. H. (2021). ABO blood groups, COVID-19 infection and mortality. Blood Cells, Mol. Dis. 89:102571. doi: 10.1016/j.bcmd.2021.102571
Lotfi, M., Hamblin, M. R., and Reza, N. (2020). COVID-19: Transmission, prevention, and potential therapeutic opportunities. Clin. Chimica Acta 508, 254–266. doi: 10.1016/j.cca.2020.05.044
Ma, H., He, Y., Bai, M., Zhu, L., He, X., Wang, L., et al. (2019). The genetic polymorphisms of ZC3HC1 and SMARCA4 are associated with hypertension risk. Mol. Genet. Genomic Med. 7:e942. doi: 10.1002/mgg3.942
MacArthur, J., Bowler, E., Cerezo, M., Gil, L., Hall, P., Hastings, E., et al. (2017). The new NHGRI-EBI Catalog of published genome-wide association studies (GWAS Catalog). Nucleic Acids Res. 45:D896–D901. doi: 10.1093/nar/gkw1133
Messina, F., Giombini, E., Montaldo, C., Sharma, A. A., Zoccoli, A., Sekaly, R.-P., et al. (2021). Looking for pathways related to COVID-19: Confirmation of pathogenic mechanisms by SARS-CoV-2-host interactome. Cell Death Dis. 12:788. doi: 10.1038/s41419-021-03881-8
Nikpay, M., Goel, A., Won, H.-H., Hall, L. M., Willenborg, C., Kanoni, S., et al. (2015). A comprehensive 1000 Genomes-based genome-wide association meta-analysis of coronary artery disease. Nat. Genet. 47, 1121–1130. doi: 10.1038/ng.3396
Ning, Z., Pawitan, Y., and Shen, X. (2020). High-definition likelihood inference of genetic correlations across human complex traits. Nat. Genet. 52, 859–864. doi: 10.1038/s41588-020-0653-y
Pairo-Castineira, E., Clohisey, S., Klaric, L., Bretherick, A. D., Rawlik, K., Pasko, D., et al. (2021). Genetic mechanisms of critical illness in COVID-19. Nature 591, 92–98. doi: 10.1038/s41586-020-03065-y
Peng, L., Shen, L., Xu, J., Tian, X., Liu, F., Wang, J., et al. (2021). Prioritizing antiviral drugs against SARS-CoV-2 by integrating viral complete genome sequences and drug chemical structures. Sci. Rep. 11:6248. doi: 10.1038/s41598-021-83737-5
Peng, L., Tian, X., Shen, L., Kuang, M., Li, T., Tian, G., et al. (2020). Identifying effective antiviral drugs against SARS-CoV-2 by drug repositioning through virus-drug association prediction. Front. Genet. 11:577387. doi: 10.3389/fgene.2020.577387
Purcell, S., Neale, B., Todd-Brown, K., Thomas, L., Ferreira, M. A. R., Bender, D., et al. (2007). PLINK: A tool set for whole-genome association and population-based linkage analyses. Am. J. Hum. Genet. 81, 559–575. doi: 10.1086/519795
Richardson, S., Hirsch, J. S., Narasimhan, M., Crawford, J. M., McGinn, T., Davidson, K. W., et al. (2020). Presenting characteristics, comorbidities, and outcomes among 5700 patients hospitalized with COVID-19 in the New York city area. J. Am. Med. Assoc. 323, 2052–2059. doi: 10.1001/jama.2020.6775
Ruan, Q., Yang, K., Wang, W., Jiang, L., and Song, J. (2020). Clinical predictors of mortality due to COVID -19 based on an analysis of data of 150 patients from Wuhan. China. Intensive Care Med. 46, 846–848. doi: 10.1007/s00134-020-05991-x
Scott, R. A., Scott, L. J., Mägi, R., Marullo, L., Gaulton, K. J., Kaakinen, M., et al. (2017). An expanded genome-wide association study of type 2 diabetes in europeans. Diabetes 66, 2888–2902. doi: 10.2337/db16-1253
Shelton, J. F., Shastri, A. J., Ye, C., Weldon, C. H., Filshtein-Sonmez, T., Coker, D., et al. (2021). Transancestry analysis reveals genetic and nongenetic associations with COVID-19 susceptibility and severity. Nat. Genet. 53, 801–808. doi: 10.1038/s41588-021-00854-7
Shen, L., Liu, F., Huang, L., Liu, G., Zhou, L., and Peng, L. (2022). VDA-RWLRLS: An anti-SARS-CoV-2 drug prioritizing framework combining an unbalanced bi-random walk and Laplacian regularized least squares. Comput. Biol. Med. 140:105119. doi: 10.1016/j.compbiomed.2021.105119
Shi, H., Mancuso, N., Spendlove, S., and Pasaniuc, B. (2017). Local genetic correlation gives insights into the shared genetic architecture of complex traits. Am. J. Hum. Genet. 101, 737–751. doi: 10.1016/j.ajhg.2017.09.022
Shirvaliloo, M. (2021). Epigenomics in COVID-19, the link between DNA methylation, histone modifications and SARS-CoV-2 infection. Epigenomics 13:10. doi: 10.2217/epi-2021-0057
Sisnieguez, C. E. L., Espeche, W. G., and Salazar, M. R. (2020). Arterial hypertension and the risk of severity and mortality of COVID-19. Eur. Respiratory J. 55:2001148. doi: 10.1183/13993003.01148-2020
Tajbakhsh, A., Gheibi Hayat, S. M., Taghizadeh, H., Akbari, A., Inabadi, M., Savardashtaki, A., et al. (2021). COVID-19 and cardiac injury: Clinical manifestations, biomarkers, mechanisms, diagnosis, treatment, and follow up. Exp. Rev. Anti Infect. Therapy 19, 345–357. doi: 10.1080/14787210
The Severe Covid-19 Gwas Group. (2020). Genome-wide association study of severe Covid-19 with respiratory failure. N. England J. Med. 383, 1522–1534. doi: 10.1056/NEJMoa2020283
Tian, X., Shen, L., Gao, P., Huang, L., Liu, G., Zhou, L., et al. (2022). Discovery of potential therapeutic drugs for COVID-19 through logistic matrix factorization with kernel diffusion. Front. Microbiol. 13:740382. doi: 10.3389/fmicb.2022.740382
van der Harst, P., and Verweij, N. (2018). Identification of 64 novel genetic loci provides an expanded view on the genetic architecture of coronary artery disease. Circul. Res. 122, 433–443. doi: 10.1161/CIRCRESAHA.117.312086
van Rheenen, W., Peyrot, W. J., Schork, A. J., Lee, S. H., and Wray, N. R. (2019). Genetic correlations of polygenic disease traits: From theory to practice. Nat. Rev. Genet. 20, 567–581. doi: 10.1038/s41576-019-0137-z
Verbanck, M., Chen, C.-Y., Neale, B., and Do, R. (2018). Detection of widespread horizontal pleiotropy in causal relationships inferred from Mendelian randomization between complex traits and diseases. Nat. Genet. 50, 693–698. doi: 10.1038/s41588-018-0099-7
Wain, L. V., Verwoert, G. C., O’Reilly, P. F., Shi, G., Johnson, T., Johnson, A. D., et al. (2011). Genome-wide association study identifies six new loci influencing pulse pressure and mean arterial pressure. Nat. Genet. 43, 1005–1011. doi: 10.1038/ng.922
Wang, D., Hu, B., Hu, C., Zhu, F., Liu, X., Zhang, J., et al. (2020). Clinical characteristics of 138 hospitalized patients with 2019 novel coronavirus–infected pneumonia in Wuhan, China. J. Am. Med. Assoc. 323, 1061–1069. doi: 10.1001/jama.2020.1585
Wang, J., Wang, C., Shen, L., Zhou, L., and Peng, L. (2021). Screening potential drugs for COVID-19 based on bound nuclear norm regularization. Front. Genet. 12:749256. doi: 10.3389/fgene.2021.749256
Watanabe, K., Stringer, S., Frei, O., Mirkov, M. U., de Leeuw, C., Polderman, T. J., et al. (2019). A global overview of pleiotropy and genetic architecture in complex traits. Nat. Genet. 51, 1339–1348. doi: 10.1038/s41588-019-0481-0
Willer, C. J., Schmidt, E. M., Sengupta, S., Peloso, G. M., Gustafsson, S., Kanoni, S., et al. (2013). Discovery and refinement of loci associated with lipid levels. Nat. Genet. 45, 1274–1283. doi: 10.1038/ng.2797
Winkler, T. W., Justice, A. E., Graff, M., Barata, L., Feitosa, M. F., Chu, S., et al. (2015). The influence of age and sex on genetic associations with adult body size and shape: A large-scale genome-wide interaction study. PLoS Genet. 570:e1005378. doi: 10.1371/journal.pgen.1005378
Xiong, X., Xu, C., Zhang, Y., Li, X., Wang, B., Wang, F., et al. (2014). BRG1 variant rs1122608 on chromosome 19p13.2 confers protection against stroke and regulates expression of pre-mRNA-splicing factor SFRS3. Hum. Genet. 133, 499–508. doi: 10.1007/s00439-013-1389-x
Yang, J., Zheng, Y., Gou, X., Pu, K., Chen, Z., Guo, Q., et al. (2020). Prevalence of comorbidities and its effects in patients infected with SARS-CoV-2: A systematic review and meta-analysis. Int. J. Infect. Dis. 94, 91–95. doi: 10.1016/j.ijid.2020.03.017
Keywords: COVID-19, cardiovascular diseases, shared genetics, meta-analysis, GWAS
Citation: Guo H, Li T and Wen H (2022) Identifying shared genetic loci between coronavirus disease 2019 and cardiovascular diseases based on cross-trait meta-analysis. Front. Microbiol. 13:993933. doi: 10.3389/fmicb.2022.993933
Received: 14 July 2022; Accepted: 24 August 2022;
Published: 15 September 2022.
Edited by:
Lihong Peng, Hunan University of Technology, ChinaReviewed by:
Jiyuan Hu, New York University, United StatesXuehai Hu, Huazhong Agricultural University, China
Xie Xianhua, Gannan Normal University, China
Copyright © 2022 Guo, Li and Wen. This is an open-access article distributed under the terms of the Creative Commons Attribution License (CC BY). The use, distribution or reproduction in other forums is permitted, provided the original author(s) and the copyright owner(s) are credited and that the original publication in this journal is cited, in accordance with accepted academic practice. No use, distribution or reproduction is permitted which does not comply with these terms.
*Correspondence: Hongping Guo, guohongping@hbnu.edu.cn