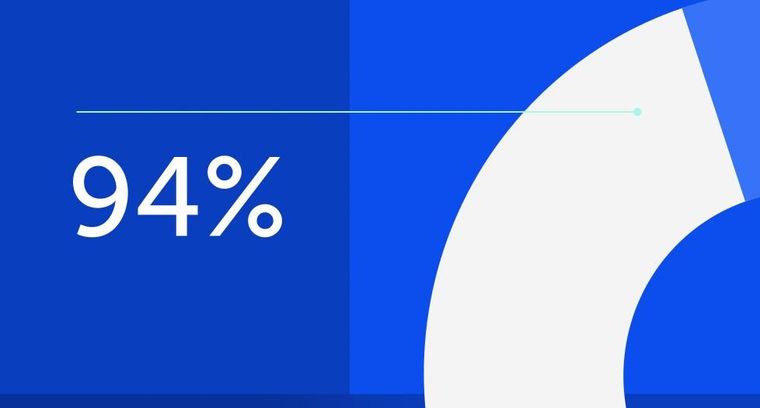
94% of researchers rate our articles as excellent or good
Learn more about the work of our research integrity team to safeguard the quality of each article we publish.
Find out more
ORIGINAL RESEARCH article
Front. Microbiol., 27 September 2022
Sec. Evolutionary and Genomic Microbiology
Volume 13 - 2022 | https://doi.org/10.3389/fmicb.2022.993879
Aging is a complex multifactorial process that greatly affects animal health. Multi-omics analysis is widely applied in evolutionary biology and biomedical research. However, whether multi-omics can provide sufficient information to reveal comprehensive changes in aged non-human primates remains unclear. Here, we explored changes in host–microbe interactions with aging in Chinese rhesus macaques (Macaca mulatta lasiota, CRs) using multi-omics analysis. Results showed marked changes in the oral and gut microbiomes between young and aged CRs, including significantly reduced probiotic abundance and increased pathogenic bacterial abundance in aged CRs. Notably, the abundance of Lactobacillus, which can metabolize tryptophan to produce aryl hydrocarbon receptor (AhR) ligands, was decreased in aged CRs. Consistently, metabolomics detected a decrease in the plasma levels of AhR ligands. In addition, free fatty acid, acyl carnitine, heparin, 2-(4-hydroxyphenyl) propionic acid, and docosahexaenoic acid ethyl ester levels were increased in aged CRs, which may contribute to abnormal fatty acid metabolism and cardiovascular disease. Transcriptome analysis identified changes in the expression of genes associated with tryptophan metabolism and inflammation. In conclusion, many potential links among different omics were found, suggesting that aged CRs face multiple metabolic problems, immunological disorders, and oral and gut diseases. We determined that tryptophan metabolism is critical for the physiological health of aged CRs. Our findings demonstrate the value of multi-omics analyses in revealing host–microbe interactions in non-human primates and suggest that similar approaches could be applied in evolutionary and ecological research of other species.
Aging is a complex multifactorial process involving many biological pathways and molecules (Ahadi et al., 2020; Bunning et al., 2020). Aging is also a major risk factor for many chronic diseases (Lehallier et al., 2019). Therefore, a deeper understanding of aging could provide new insights into the mechanisms of age-related diseases to promote health and longevity (Alpert et al., 2019; Lehallier et al., 2019). As such, increasing studies have explored the processes and mechanisms of aging at the genomic (Kenyon, 2010; Hou et al., 2020), transcriptomic (Peters et al., 2015; Bryois et al., 2017; Balliu et al., 2019), proteomic (Lehallier et al., 2019), and metabolomic levels (Bunning et al., 2020; Hou et al., 2020). However, given the complex involvement of genetic, environmental, and lifestyle factors, the molecular changes that occur with aging remain poorly understood (López-Otín et al., 2013; Ahadi et al., 2020; Bunning et al., 2020).
In addition to molecular changes during aging, the host microbiome, consisting of diverse microbial communities, plays an important role in host physiology and metabolism (Sommer and Bäckhed, 2013; Manara et al., 2019). In particular, the gut microbiota is increasingly regarded as an ‘invisible organ’ in animals (Hill et al., 2017) and is associated with host physiology, immunity, neurological function, metabolism, and disease (Gilbert et al., 2018; Kurilshikov et al., 2021). Recent studies have also provided new insights into gut microbial trajectories associated with aging (Wilmanski et al., 2021; Zhang et al., 2021). For example, the gut microbiota develops rapidly from birth until 3 to 5 years of age (Hill et al., 2017; Roswall et al., 2021; Wilmanski et al., 2021), followed by a long period of relatively stability, then gradual changes with aging (O’Toole and Jeffery, 2015). Wilmanski et al. (2021) reported increasing compositional uniqueness of the gut microbiome as a component of healthy aging. Therefore, identifying age-related patterns in the gut microbiota may be useful for monitoring gut microbiome health and thus host health (Wilmanski et al., 2021).
Oral microbiota is also important because the oral cavity serves as the initial entry point for oral and gut microbial colonization (Xiao et al., 2020). In addition, oral bacteria are implicated in oral and dental health as well as many diseases that affect the elderly, such as non-oral cancers, cardiovascular disease, and Alzheimer’s disease (Hezel and Weitzberg, 2015; Olsen and Singhrao, 2015; Shoemark and Allen, 2015; Fan et al., 2018; Xiao et al., 2020). To date, however, most studies have focused on oral microbiome changes associated with oral disease (Chen et al., 2020; Nóvoa et al., 2020; Baker et al., 2021), with changes during aging still largely unexplored.
Research related to human aging presents several challenges, including ethical and practical difficulties in recruiting large cohorts for long-term research, long human lifespans, and differences in medical interventions, diet, and socioeconomic circumstances (Janiak et al., 2021). Non-human model organisms, such as roundworms, mice, and rats, can provide considerable data regarding the aging process (Kenyon, 2010; Campisi et al., 2019). However, given the distant evolutionary relationships between such species and humans, findings may not be directly transferable to human aging. In contrast, considering their close relationship to humans (Gibbs et al., 2007) and similar anatomy, physiology, and behavior (Lan et al., 2020; Yan et al., 2020), non-human primates, such as rhesus macaques (Macaca mulatta, RMs), are well-suited animal models and have been successfully used in aging-related studies (Mattison et al., 2017; Campisi et al., 2019; Zhou et al., 2020).
Studies have indicated that multi-omics analysis can effectively detect molecular changes during aging (Price et al., 2017; Schüssler-Fiorenza Rose et al., 2019; Zhou et al., 2019; Ahadi et al., 2020; Hou et al., 2020). Here, we used a multi-omics approach to profile the blood transcriptome and metabolome as well as the gut and oral microbiome in young adult (7–9 years old) and aged (>20 years old) Chinese rhesus macaques (Macaca mulatta lasiota, CRs). We examined the dynamics of molecular and microbiome changes and explored the changes in host–microbe interactions that occur during aging. This study should provide useful information to better understand and maintain the health of aged CRs and provide new insights into the mechanisms of aging.
We collected anal swabs, oral swabs, and whole peripheral blood samples from young (n = 9, ages: 7–9 years) and old (n = 10, ages: 20–28 years) semi-captive CRs housed at Sichuan Green-House Biotech Co., Ltd. (Meishan, Sichuan, China) in a 2000-m2 open-air enclosure. All macaques were housed under the same conditions and fed the same diet.
PAXgene Blood RNA tubes were used to collect fresh blood samples. The samples were first stored at room temperature (18–20°C) for 4 h, then transferred to −20°C for 24 h, and finally preserved at −80°C until RNA extraction. Other blood samples were centrifuged on site to extract supernatants, which were then stored at −80°C for subsequent metabolome analysis and inflammatory factor measurement.
This study was approved by the Ethics Committee of the College of Life Sciences, Sichuan University, China (No. 20200327012 and No. 20210308001). All guidelines of the Management Committee of Experimental Animals of Sichuan Province, China (SYXK-Sichuan, 2019–192) regarding sample collection and use were strictly followed.
Details on methods used are reported in our previous study (Lan et al., 2020). In brief, RNA samples were sent to Novogene (Beijing, China) for sequencing using the Illumina NovaSeq 6,000 platform with a paired-end sequencing length of 150 bp. The NGS QC Toolkit v2.3.3 (Patel and Jain, 2012) and HISAT2 v2.1.0 (Kim et al., 2015) were used for quality control and read mapping, and StringTie v1.3.6 (Pertea et al., 2015) was used to assemble transcriptomes and obtain raw read counts for each gene and transcript. Based on expression values, we used the DESeq2 R package (Love et al., 2014) to perform differential expression analysis. The DEGs were selected based on adjusted p ≤ 0.05 and log2 fold-change ≥1, resulting in 124 DEGs in the two age categories. Gene Ontology (GO) and Kyoto Encyclopedia of Genes and Genomes (KEGG) enrichment analyses were performed using g: Profiler (Raudvere et al., 2019) to functionally classify DEGs, with a Benjamini-Hochberg false discovery rate (FDR) ≤ 0.05 considered significant.
The collected blood supernatants were sent to MetWare (Chengdu, China) for targeted metabolite analysis. After thawing the blood sample on ice, 300 μl of pure methanol was added to 50 μl of sample to remove blood protein. The samples were then centrifuged at 12000 g and 4°C several times (centrifuged 10 min → collected the supernatant → centrifuged 5 min → stored at 20° for 30 min → centrifuged 3 min). In total, 150 μl of supernatant was analyzed using high-performance liquid chromatography-electrospray tandem mass spectrometry (HPLC-ESI-MS/MS; UPLC, ExionLC AD; MS, QTRAP 6500 + System, Sciex). All chemicals used, including methanol (Merck), acetonitrile (Merck), formic acid (Aladdin), and standards (BioBioPha/Sigma-Aldrich), were chromatographically pure. Analyst v1.6.3 was used to analyze mass spectrometry data, in a qualitative analysis of the metabolites according to the retention time of the detected substance and secondary spectral data based on the MetWare database.
Each metabolite was subjected to a two-tailed unpaired t-test assuming unequal variances and using the q-value package (for total metabolites) to correct for false discovery.
Total DNA from the swabs was extracted using a Tiangen DNA Stool Mini Kit (Tiangen Biotech Co., Ltd., China) and sent to Novogene (Beijing, China) for sequencing using the Illumina NovaSeq 6,000 platform with a paired-end sequencing length of 150 bp. After sequencing, adapters and low-quality raw reads were removed using Trimmomatic based on a four-base wide sliding window, with average quality per base >20 and minimum length 90 bp (Bolger et al., 2014). The CR potential sequences were removed using Bowtie2 (Langmead and Salzberg, 2012) as part of the KneadData pipeline1 with the RM reference genome (assembly Mmul_10). De novo assembly of the metagenomes from the quality-filtered Illumina reads was performed using MEGAHIT (Li et al., 2015) with the option “-t 96 –m 0.95 --min-contig-len 300.” Separate assembly of each sample was applied, rather than co-assembly of all samples, the advantages and disadvantages of which have been discussed previously (Pasolli et al., 2019). Gene prediction was performed using Prodigal (Hyatt et al., 2010) with the option “-p meta –g 11.” Non-redundant gene sets with thresholds of 95% similarity and 90% coverage of query sequences were constructed with CD-HIT (Fu et al., 2012) with the option “-c 0.95-aS 0.90.” The non-redundant genes were further translated into amino acid sequences. The amino acid sequences were aligned using DIAMOND (Buchfink et al., 2015) with the option “--id 80% --query-cover 70% --evalue 1e-5” in the Carbohydrate-Active enZYmes (CAZy) database (Lombard et al., 2014). Quantification of the non-redundant genes in each metagenome was performed using Salmon (Patro, 2017) with the option “--meta.” Total abundance of each gene type was determined by total abundance of all genes mapped to the same gene type. Gene family and microbial metabolic pathway abundances were assessed using HUMANn3 (Franzosa, 2018) with the ChocoPhlAn and UniRef90 EC filtered databases (Suzek et al., 2007), and were normalized by copies per million (CPMs). Taxonomic labels of metagenomic sequences were assigned using Kraken2 (Wood and Salzberg, 2014) with the option “--use-mpa-style.” Taxon abundances were normalized by relative abundance. Differences in taxon, functional gene, and metabolic pathway abundances were determined using Linear discriminant analysis effect size (LEfSe) analysis (Segata et al., 2011). Antibiotic resistance genes (ARGs) were quantified using ShortBRED (Kaminski et al., 2015). In brief, the shortbred_identify.py script was used to produce a FASTA file of markers using the ARG sets in the Comprehensive Antibiotic Resistance Database (CARD; Alcock et al., 2019) as proteins of interest and UniRef90 sequences as reference proteins, and the shortbred_quantify.py script was used to quantify the abundance of ARGs in the metagenomes. The ARG and gut microbiome abundance network was drawn using Cytoscape (Otasek et al., 2019).
The taxonomic abundance table generated using Kraken2 was used as input for QIIME2 (Bolyen et al., 2019). The QIIME2 diversity plugin was used to calculate alpha (α) diversity (within sample diversity; Bolyen et al., 2019). The QIIME2 plugin DEICODE (Martino et al., 2019) was used to calculate beta diversity with feature loadings.
To assess possible relationships between microbial genera, DEGs, and differentially expressed metabolites (DEMs), Spearman correlation analysis was performed based on the combined dataset of all variables. As an example, to determine whether microbe M is related to host genes X, Y, and Z, respectively, we calculated the correlation between the abundance of M and expression levels of X, Y, and Z in the samples, thereby obtaining three correlation values for microbe M, i.e., M ~ X, M ~ Y, and M ~ Z. This was carried out for all microbes and DEGs (and metabolites), with the top 100 relationships showing the highest relevance selected for display. All value of ps were corrected using the python model statsmodels. Stats. multitest. Multipletests (Supplementary Table 2).
To quantify the levels of interleukin 21 (IL-21) and interleukin 27 (IL-27) in the two groups, enzyme-linked immunosorbent assays (ELISA) were performed using an ELISA kit (JM-10921 M2 and JM-10916 M2, Jingmei, Jiangsu, China). Data were analyzed using Wilcoxon rank tests, with p < 0.05 considered significant.
To characterize differences in gut microbial composition, we compared relative abundance of gut bacteria between old and young CRs using the Wilcoxon rank sum test, which showed enrichment in different bacterial lineages. At the genus level, Prevotella, Faecalibacterium, and Lactobacillus were dominant in both groups (Figure 1A). Compared to the young group, the relative abundances of Lactobacillus, Lactiplantibacillus and Limosilactobacillus were lower in the old group (p < 0.05 and LDA > 2), while the relative abundances of Salmonella, Odoribacter, Ornithobacterium, Tamlana, and Sarcina were higher in the old group (p < 0.05 and LDA > 2; Figure 1B). Comparing α-diversity, results showed no significant differences in the four indices (ACE, Chao1, Shannon, and Simpson) between the two groups (p > 0.05; Figure 1C).
Figure 1. Metagenomics analysis of gut microbiota. (A) Top 10 most abundant genera in gut microbiome between young and old groups. (B) Differential analysis of gut microbial composition in young and old groups. (C) Alpha (α) diversity estimates between young and old groups. (D) Differential analysis of gut microbial function in young and old groups. (E) Differential analysis of gut microbial ARGs in young and old groups. (F) Differential analysis of gut microbial CAZy enzyme in young and old groups. p < 0.05 was considered significant.
To characterize the global function of the gut microbiome and compare functional differences between young and old CRs, the abundances of microbial gene families and metabolic pathways were quantified using HUMANn3. Results indicated that the young CR gut microbiome was mainly enriched in aerobactin biosynthesis-related pathways such as L-lysine biosynthesis II, O-antigen building blocks biosynthesis, and pyrimidine deoxyribonucleotides biosynthesis, while the old CR gut microbiome was primarily enriched in L-glutamate and L-glutamine biosynthesis, ketogenesis, and petroselinate biosynthesis. Interestingly, the homolactic fermentation pathway was significantly enriched in the young CRs compared to the old CRs, while the opposite pattern was observed in the oral microbiome (Figure 1D).
Identification and quantification of ARGs showed that the tetracycline resistance tetW gene, was more abundant in the gut microbiome of old CRs than young CRs (p < 0.05; Figure 1E). Furthermore, carbohydrate esterases (CEs) and auxiliary activities (AAs) of the CAZy enzyme family were significantly down-regulated in aged CRs, indicating decreased carbohydrate metabolism in the older monkeys (p < 0.05; Figure 1F).
We also identified and characterized differences in the oral microbiota of young and old CRs. Unlike the gut microbiota, however, the distribution of dominant microflora in the oral cavity of young and old CRs was similar, while the less abundant microflora showed considerable differences (Figure 2A). At the genus level, nine genera were significantly more abundant in the young CRs (p < 0.05 and LDA > 2), including Ottowia, Simonsiella, Streptobacillus. In contrast, 26 genera were more abundant in the old CRs (p < 0.05 and LDA > 2), including Veillonella, Filifactor, Paenibacillus, Olsenella, Dialister, Sporomusa, and Bifidobacterium (Figure 2B). No significant differences were found in α-diversity, consistent with the gut microbiota results (Figure 2C).
Figure 2. Metagenomics analysis of oral microbiota. (A) Top 10 most abundant genera in oral microbiome between young and old groups. (B) Differential analysis of oral microbial composition in young and old groups. (C) Alpha (α) diversity estimates between young and old groups. (D) Differential analysis of oral microbial function in young and old groups. (E) Differential analysis of oral microbial ARGs in young and old groups. p < 0.05 was considered significant.
In addition to microbial composition, oral microbiota function and ARGs showed significant differences between young and old CRs. The young CR oral microbiome was enriched in several metabolic-related pathways, including CDP-diacylglycerol biosynthesis I/II, superpathway of glycolysis and Entner-Doudoroff, and superpathway of L-lysine, L-threonine, and L-methionine biosynthesis I. In contrast, the old CR oral microbiome was primarily enriched in homolactic fermentation, pyruvate fermentation to propanoate I, and glycolysis-related pathways (Figure 2D). For oral microbiota ARGs, the tet (L) gene, encoding resistance to tetracycline antibiotic, and the ADC-76 gene, encoding resistance to cephalosporin, were more abundant in old CRs than in young CRs (p < 0.05; Figure 2E). However, there were no significant differences in the six CAZy enzyme family modules between the two groups (p > 0.05).
After removing unqualified samples, whole peripheral blood samples from 18 CRs (10 old and eight young CRs) were collected and secondary metabolite species were determined by HPLC-ESI-MS/MS. All metabolites were annotated using KEGG. Based on the local metabolite database, qualitative and quantitative mass spectrometry analyses were conducted on the sample metabolites. In total, 644 metabolites were identified, including 14 sugars, 56 amino acids and their derivatives, and 45 organic acids and their derivatives. All raw data for the detected metabolites are shown in Supplementary Table 1.
Based on certain criteria (p < 0.05 and variable importance in projection (VIP) > 1), 53 significant DEMs were identified between the old and young groups (26 up-regulated and 27 down-regulated in old CRs; Figures 3A,B). Both CARs and FFAs were the major up-regulated DEMs in old CRs and included carnitine C18: 3, carnitine C20: 2, hexadecanoic acid (C16: 0), palmitoleic acid (C16: 1), and cis-11,14-eicosadienoic acid (C20: 2), which were enriched in the arginine biosynthesis, ABC transporter, amyotrophic lateral sclerosis, and biosynthesis of amino acid pathways. Indole and its derivatives, organic acid and its derivatives, and phenolic acids were the top three classes down-regulated in the old group and included 3-indolepropionic acid, indole-3-carboxaldehyde, indoleacrylic acid, and 3-hydroxyanthranilic acid. However, the down-regulated DEMs were not enriched in any pathway (Figures 3C,D).
Figure 3. Blood metabolome and blood transcriptome analyses. (A) Volcano plots of metabolomes between young and old groups. (B) Partial least squares discriminant analysis (PLS-DA) and orthogonal partial least squares discriminant analysis (OPLS-DA) score plots based on metabolic profiles. (C) Differential abundance of blood metabolites in young and old groups (VIP ≥ 1, p < 0.05). (D) Enrichment analysis of differentially abundant pathways in young and old groups (p < 0.05). (E) Volcano plots of DEGs in young and old groups (log fold-change ≥1, p < 0.05). (F) GO and KEGG pathway enrichment analyses of up-regulated DEGs in old group (p < 0.05). (G) GO and KEGG pathway enrichment analyses of down-regulated DEGs of old group (p < 0.05).
Whole peripheral blood transcriptomes of 15 CRs (nine old and six young CRs) were sequenced. The 524.5 million clean reads obtained after removing adaptor sequences and low-quality reads were aligned to the rhesus macaque reference genome (MMUL_10) separately with an average mapping rate of 95.55% per sample. All samples were processed using the same bioinformatics pipeline. After removing low-expression genes, the reads were assembled into 19,902 known genes.
In total, 124 genes were identified as DEGs between the groups (Figure 3E), including 44 up-regulated DEGs and 80 down-regulated DEGs in the old group compared to the young group. To clarify the biological roles of the DEGs, we performed GO and KEGG pathway enrichment analyses of the up-regulated and down-regulated DEGs separately. For GO enrichment analysis, the old down-regulated DEGs were mainly enriched in protein binding-or nucleotide binding-related molecular function (MF) categories, such as heat shock protein binding (GO: 0031072) and adenyl nucleotide binding (GO: 0030554). In the biological process categories, the old down-regulated DEGs were mainly enriched in immune response and interferon signaling pathways, such as interleukin-27-mediated signaling pathway (GO: 0070106), defense response to bacterium (GO: 0042742), Toll-like receptor 3 signaling pathway (GO: 0034138), and type I interferon signaling pathway (GO: 0060337). Based on KEGG enrichment analysis, the old CR down-regulated DEGs were significantly enriched in nine pathways, including NOD-like receptor signaling pathway (KEGG: 04621) and antigen processing and presentation (KEGG: 04612; Figure 3G). For analysis of old CR up-regulated DEGs, only GO terms related to vitamin D biosynthesis were enriched, including regulation of calcidiol 1-monooxygenase activity (GO: 0060558) and vitamin D biosynthetic process (GO: 0042368; Figure 3F).
To reveal host–microbe interactions, metabolites were used as the core factor to link differential microbial genera, DEMs, and DEGs. We performed Spearman correlation analysis, which generated a correlation network between the variables that differed significantly between the young and old CRs (Figure 4A). The correlation network between differential gut microbial genera and DEMs contained 36 nodes and 53 edges, and each of the top gut microbial genera had associations with more than four differential metabolites were Sarcina, Lactiplantibacillus, Limosilactobacillus, Odoribacter, and Lactobacillus (Figure 4B). As shown in Figure 4C, the correlation network between differential oral microbial genera and DEMs contained 51 nodes and 72 edges, and each of the top oral microbial genera associated with more than four differential metabolites were Acetobacterium, Filifactor, Intestinibaculum, Megasphaera, Ottowia, and Veillonella. The correlation network between DEGs and DEMs contained 64 nodes and 99 edges. We found that six DEGs, which each of them had more than four associations with differential metabolites, including FBN2, ENSMMUG00000063631, ENSMMUG00000041057, IFNG, TPH1, and CIB2 (Figure 4D).
Figure 4. Association analysis among multi-omics. (A) Correlation network of variables that differed significantly between young and old groups of each omics. (B) Correlation network of differential gut microbial genera and DEMs. (C) Correlation network of differential oral microbial genera and DEMs. (D) Correlation network of DEGs and DEMs. DEGs: differentially expressed genes; DEMs: differentially expressed metabolites; B_DEMi: differentially expressed oral microbiota; M_DEMi: differentially expressed gut microbiota. All nodes and edges of correlation networks, with lines indicating significant correlations (p < 0.05). Blue lines indicate positive correlation and green lines indicate negative correlation.
Analyses related to tryptophan metabolism appeared in each omics data. Thus, we next focused on the correlations between tryptophan metabolites (3-indolepropionic acid, indole-3-carboxaldehyde, indoleacrylic acid, and 3-indolebutyric acid), differential microbial genera, and DEGs. The DEGs showing the strongest association with differential tryptophan metabolites were IFNG and ENSMMUG00000059005. The differential gut microbial genera showing the strongest associations with differential tryptophan metabolites were Sarcina and Lactobacillus, and the differential oral microbial genus showing the strongest association with differential tryptophan metabolites was Slackia (Table 1).
We determined the levels of immune factors IL-21 and IL-27 using an ELISA kit. As shown in Supplementary Figure S1, the level of IL-21 was significantly higher in the old group (p < 0.05), consistent with the transcriptome results, while the level of IL-27 showed no significant differences between the two groups (p > 0.05).
As CRs exhibit similar molecular and phenotypic changes to humans during aging, they are considered an excellent model species for studying cardiovascular (Zhou et al., 2020) and microbiota-associated human diseases (Chen et al., 2018; Janiak et al., 2021). Currently, our understanding of how the oral and gut microbiomes change in aged CRs and how these changes affect host gene expression and physiology during aging remains limited. Most previous studies on molecular changes during aging in non-human primates have been based on single omics data, e.g., transcriptome (Li et al., 2019; Yan et al., 2020; Zhou et al., 2020) and 16S rRNA gene amplicon profiling (Chen et al., 2018; Ebersole et al., 2020; Janiak et al., 2021). In the present study, we evaluated host–microbe interactions in aged CRs using a multi-omics approach.
first identified changes in oral and gut microbiome composition and function. The gut microbiota in young and old CRs was dominated by Prevotella, Faecalibacterium, and Lactobacillus, consistent with previous reports (Chen et al., 2018; Janiak et al., 2021). At the genus level, Prevotella was the most abundant in both young and old CRs, as found in humans on non-westernized diets associated with carbohydrates and fiber (Wu et al., 2011; Tett et al., 2019). Lactobacillus showed high abundance in both young and old CRs but was significantly more abundant in the young group. Based on 16S V4 rRNA amplicon sequences, Janiak et al. (2021) also detected significant differences in the relative abundance of Lactobacillus between infant and non-infant CRs, but not between other post-infancy age groups, suggesting that Lactobacillus may play an important role in digesting milk. Lactobacillus species are beneficial bacteria in the gut of humans and other mammals (Jia et al., 2010; Rossi et al., 2016; Valeriano et al., 2017), identified as important probiotics for gut health (Spinler et al., 2008). Lactobacillus species can inhibit the overgrowth of pathogens in the gut by producing various antibiotic factors, such as organic acid, hydrogen peroxide, and bacteriocin (Reid and Burton, 2002; Spinler et al., 2008; Linninge et al., 2019). Therefore, the significantly higher abundance of probiotics in young CRs may contribute to better gut health maintenance. The homolactic fermentation pathway was also enriched in the gut of young CRs, which inhibits the growth of intestinal saprophytes and ensures a healthy gut (Zhang et al., 2019).
In contrast, we identified two highly abundant genera (Salmonella and Sarcina) in the gut of old CRs associated with intestinal issues. Salmonella species are common food-borne pathogens (Bula-Rudas et al., 2015; Rivera-Chávez and Bäumler, 2015). In addition, Sarcina species can cause abomasal bloat and death in livestock, especially sheep and goats, and are associated with certain human diseases, including emphysema gastritis and gastric ulcers (Lam-Himlin et al., 2011). Functional analysis indicated that the L-glutamine biosynthesis III (PWY-6549) and L-glutamate and L-glutamine biosynthesis (PWY-5505) pathways were enriched in old CRs. These two pathways produce L-glutamine, which can induce cancer cells to undergo rapid growth (Daye and Wellen, 2012). Taken together, the significant decrease in probiotics and significant increase in pathogenic bacteria in old CRs may induce a variety of intestinal and other diseases.
Compared with the gut microbiome, the oral microbiome of CRs shows marked similarities to that of humans (Janiak et al., 2021). Similarly, we found that most of the top 15 genera in the oral microbiome of CRs were also core genera in the oral microbiome of healthy humans, e.g., Streptococcus, Fusobacterium, Haemophilus, Porphyromonas, and Gemella (Aas et al., 2008; Zaura et al., 2009; Krishnan et al., 2017; Chen et al., 2018). We also found a higher abundance of oral pathogens in old CRs, such as Bifidobacterium. Bifidobacterium is normally considered a probiotic, producing a variety of organic acids, including lactic acid, and showing beneficial effects, such as reducing the number of harmful bacteria in the gut (Gill et al., 2001; Manome et al., 2019). However, oral Bifidobacterium is recognized as a novel caries-associated bacterium, especially in children (Aas et al., 2008; Tanner et al., 2011; Kaur et al., 2013). In humans, frequently detected Bifidobacterium species in the oral microbiome include B. dentium (Munson et al., 2004) and B. longum (Nyvad and Kilian, 1990; Aas et al., 2008; Mantzourani et al., 2009). We detected 23 Bifidobacterium species in the oral microbiome of CRs, with B. adolescentis and B. longum found in higher abundance in old CRs than in young CRs. This is consistent with our observation that almost all aged individuals sampled showed evidence of caries or other oral disease. Functional pathway analysis showed that the homolactic fermentation pathway, which is related to lactic acid formation, was enriched in the oral microbiome of the old group. Lactic acid in the oral cavity is not beneficial, with long-term presence associated with tooth damage and corrosion (Jijakli and Jensen, 2019). Functional analysis also indicated that the L-glutamine biosynthesis III (PWY-6549) and L-glutamate and L-glutamine biosynthesis (PWY-5505) pathways were enriched in the oral and gut microbiomes of old CRs. Thus, the microbial characteristics of both the oral and gut microbiomes changed with age, likely increasing health risks for the host (Ahadi et al., 2020; Wilmanski et al., 2021).
Filifactor alocis, Selenomonas, and Veillonella, which are associated with periodontal diseases (Aruni et al., 2011; Barzilai et al., 2012; Antezack et al., 2021), were more abundant in the old CRs. Filifactor alocis has been used as a diagnostic marker for periodontitis due to its unique ability to tolerate oxidative stress and generate a strong pro-inflammatory response (Aruni et al., 2011; Moffatt et al., 2011). Filifactor alocis is associated with gingivitis, gestational diabetes, and oral squamous cell carcinoma (Gogeneni et al., 2015; Yang et al., 2018), but has not been found in healthy individuals (Schulz et al., 2019). Selenomonas is also associated with gingivitis and may be a potential biomarker for the onset of periodontitis (Tanner et al., 1996, 1998; Socransky et al., 1998; Antezack et al., 2021). Oral Veillonella is closely related to dental pulp, periapical infection, and chronic periodontitis, and can cause caries in cooperation with Streptococcus mutans (Barzilai et al., 2012). Comparing the oral microbiomes of young and old CRs, we identified several bacteria related to human oral diseases that were more abundant in aged CRs. These results suggest that aged CRs may face similar dental issues as humans, and thus could be an excellent model for studying oral diseases in humans.
We next investigated metabolite and gene expression levels in the plasma metabolome and blood transcriptome. Metabolomics is a popular approach to study aging, as metabolic changes are central to the aging process (Barzilai et al., 2012; López-Otín et al., 2013; Hou et al., 2020; Wilmanski et al., 2021). Metabolomics also reflects both genetic and nongenetic factors, as metabolites are influenced by the host, microbial activity, and environmental exposure (Rinschen et al., 2019; Bunning et al., 2020). In recent years, metabolomics has been successfully applied in the study of human aging, identifying many age-related biomarkers and biological pathways (Tanner et al., 1998; López-Otín et al., 2013; Price et al., 2017; Hou et al., 2020; Antezack et al., 2021; Wilmanski et al., 2021). However, few studies have explored metabolomics in non-human primates. Here, plasma metabolomics revealed significant differences at the single metabolite level between young and old CRs. We found that indole and its derivatives (indole-3-carboxaldehyde, 3-indolepropionic acid, and indoleacrylic acid), produced from tryptophan metabolism (Natividad et al., 2018), were significantly decreased in old CRs. These microbial tryptophan metabolites act as AhR ligands to increase IL-22 secretion (Zelante et al., 2013; Lamas et al., 2016). AhR is widely distributed in different mammalian cells and produces different effects by binding to different ligands, thus playing a vital role in immune and inflammatory responses, with its deletion leading to an increase in pro-inflammatory cytokines (Alexeev et al., 2018). Previous research has shown that the gut microbiota of Anopheline mosquitoes promotes anti-parasitic responses by participating in tryptophan metabolism (Feng et al., 2022).
In addition to indole and its derivatives, a variety of FFAs were also found in the aged CRs, including cis-11, 14-cicosadienoic acid (C20: 2), linoleic acid (C18: 2N6C), and hexadecanoic acid (C16: 0). Compared with other saturated FFAs, hexadecanoic acid (palmitic acid) is found at higher concentrations in human plasma (Richieri and Kleinfeld, 1995). High concentrations of palmitic acid can enhance serine 307 phosphorylation of insulin receptor substrate 1 (IRS1) through multiple mechanisms, leading to insulin resistance (Wei et al., 2018). High levels of palmitic acid can also induce excessive reactive oxygen species (ROS) production, resulting in mitochondrial and endoplasmic reticulum stress and fatty acid metabolism disorders (Tumova et al., 2016). Therefore, fatty acid metabolism disorders may occur in aged CRs.
Dysregulation of carnitine homeostasis is a prominent feature of fatty acid metabolism disorders (Bene et al., 2018). We detected higher abundance of carnitine in the old CR group. One of the main functions of carnitine is to transport long-chain fatty acids from the cytoplasm across the mitochondrial membrane into the mitochondrial matrix for β-oxidation, thereby generating cellular energy (Bene et al., 2018). Dysfunction in fatty acid metabolism can lead to a substantial accumulation of free carnitine and CARs (Möder et al., 2003; Zhang et al., 2014). Indeed, we found that the most significant changes in plasma metabolites in the aged CRs were the increases in free carnitine and CARs, including carnitine C18: 3, carnitine C6: 0 isomer 1, carnitine C8: 0, carnitine C20: 2, and carnitine C7: 1 isomer 1, further suggesting that older CRs may experience disturbances in fatty acid metabolism. This finding is similar to that reported in human studies showing that lipid metabolism disturbance can increase abnormal acylcarnitine levels, leading to obesity or diabetes (Mihalik et al., 2010).
The top three DEMs showing higher abundance in the aged CRs were all related to cardiovascular function and blood coagulation. Heparin is a commonly used anticoagulant for the prevention and treatment of venous thrombosis (Gray et al., 2008). 2-(4-hydroxyphenyl) propionic acid (2-HPPA CPD) can act on cyclooxygenase (COX) to regulate platelet aggregation (Yamanishi et al., 2008). Elevated levels of docosahexaenoic acid ethyl ester (DHA-EE) in the body can increase DHA levels, which play an important role in neurological and cardiac function (Martínez et al., 2000; Valenzuela et al., 2005), and thus DHA-EE intake may delay damage caused by aging. These results are consistent with our previous study, which showed that aged CRs have shorter coagulation times and higher coagulation factor II (FII) and VIII (FVIII) activity compared to young CRs (Zhou et al., 2020). The above results indicate that aged CRs may face the same problems as older humans, and thus are an appropriate model species for studying human cardiovascular disease (Zhou et al., 2020).
Consistent with the metagenome and metabolome results, transcriptome analysis detected several DEGs (TPH1, IFNG, IL-21, and IL-27) and signaling pathways related to inflammation and tryptophan metabolism. Tryptophan hydroxylase 1 (TPH1) is mainly expressed in enterochromaffin and other non-neuronal cells (Walther et al., 2003) and is the rate-limiting enzyme encoded by TPH1 for tryptophan metabolism into serotonin (5-HT; Billing et al., 2019). 5-HT is a key neurotransmitter in the central nervous system and plays a role in controlling mood, sleep, and anxiety, and regulating gastrointestinal motility (Martin et al., 2017; Keating and Spencer, 2019). High levels of 5-HT and TPH1 are observed in certain metabolic diseases (e.g., NAFLD and type 2 diabetes; Crane et al., 2015). Interferon-γ (IFN-γ), which is encoded by IFNG, is involved in tryptophan metabolism. Notably, IFN-γ can induce the synthesis of indoleamine-2, 3-dioxygenase, a tryptophan-catabolizing enzyme, converting tryptophan into kynurenine and reducing the level of tryptophan in plasma (Munn et al., 2005). IFN-γ is produced by natural killer (NK) and T cells and is considered a pro-inflammatory factor due to its strong macrophage activation potential (Seder et al., 1993). IL-21, which is encoded by IL-21, is secreted by follicular helper T cells (Tfh), peripheral helper T cells (Tph), and helper T cells (Th17). Stimulating T cell receptor (TCR) signals induces IL-21 production and IFN-γ release (Apetoh et al., 2010). In addition, IL-21 can promote the differentiation of CD4+ T cells into Th17 cells, which are robust producers of IL-21 (Manel et al., 2008). Dendritic cells (DCs) can express Toll-like receptors (TLRs), which can recognize cell wall components such as lipopolysaccharide, peptidoglycan, and lipoprotein. Therefore, TLRs are regarded as a bridge for DC activation caused by microbial components (Kawai and Akira, 2011). DCs also produce IL-27 in response to TLR activation (Molle et al., 2010). IL-27, which is encoded by IL-27, was originally thought to be a pro-inflammatory cytokine due to its structural homology with IL-12 and its ability to trigger IFN-γ production. However, Kastelein et al. (2007) found that IL-27 inhibits Th1, Th2, and Th17 cell responses and limits central nervous system inflammation. IL-27 also promotes the differentiation of type 1 regulatory T cells (Tr1 cells), which produce IL-10 to inhibit inflammation (Stumhofer et al., 2007). Our results showed that the expression levels of IL-21 and IFN-γ increased, whereas the expression level of IL-27 decreased in aged CRs. Furthermore, the Toll-like receptor TLR1: TLR2 and Toll-like receptor 3 signaling pathways, which recognize viruses and bacteria, were down-regulated in the aged CRs. In addition, the vitamin D biosynthetic process pathway, which directly and indirectly inhibits antigen processing and presentation and Th1 cell activation (Bartels et al., 2010), was up-regulated in aged CRs. These changes suggest that aged CRs may be more prone to tryptophan metabolism disorder and inflammation than young CRs.
Using multi-omics analysis, we observed many potential links among the detected differential microbiota, metabolites, and genes. We found that changes in metabolite composition in aged CRs were primarily caused by decreased abundance of beneficial genera (Lactobacillus, Lactiplantibacillus, and Limosilactobacillus) and increased abundance of pathogenic genera. Changes in metabolite composition may also have contributed to the increase in oral pathogenic genera (Filifactor and Veillonella). Among the DEGs associated with DEMs, IFNG and TPH1 were both related to tryptophan metabolism. Correlation analysis showed that IFNG was strongly associated with tryptophan metabolites, consistent with studies showing that IFN-γ can accelerate tryptophan metabolism (Munn et al., 2005). At the same time, Lactobacillus in the gut was strongly associated with tryptophan metabolites, consistent with studies showing that Lactobacillus can metabolize tryptophan to produce indole-3-carboxaldehyde (Natividad et al., 2018). Zhang et al. (2022a,b) also found that Lactobacillus in the honeybee gut modulates host learning and memory behaviors via tryptophan metabolism regulation.
Based on our results, tryptophan metabolism appears to be critical for the physiological health of aged CRs (Figure 5). Most tryptophan obtained from food is absorbed in the small intestine and plays an important role in immune regulation and neuronal activity (Martin et al., 2017; Keating and Spencer, 2019). We found the key gene TPH1 involved in 5-HT synthesis and IFN-γ involved in tryptophan convert into kynurenine were up-regulated, suggesting the increase in 5-HT and the decrease in circulating tryptophan in old CRs. In our study, the down-regulation of SLC7A8, which is involved in tryptophan transport, further confirmed the decrease in circulating tryptophan (Tina et al., 2019). Studies in healthy older people have shown that low circulating tryptophan is associated with several diseases, including neurodegenerative diseases, olfactory dysfunction, and immunological disorders (Denz et al., 1993; Iwagaki et al., 1995; Widner et al., 1999, 2000; Adachi et al., 2017).
Figure 5. Dysregulation of tryptophan metabolism in aged CRs. Tryptophan obtained from food is absorbed in the small intestine. Up-regulation of key gene TPH1, which is involved in 5-HT synthesis, and up-regulation of IFN-γ, which is involved in the conversion of tryptophan into kynurenine, suggest a decrease in circulating tryptophan in aged CRs. On the other hand, in the colon, tryptophan is metabolized by gut microbiota to produce indole-3-carboxaldehyde, 3-indolepropionic acid, and indoleacrylic acid. Our results showed that Lactobacillus abundance decreased in old CRs, leading to a decrease in AhR ligands in the plasma. Down-regulation of the Toll-like receptor TLR1:TLR2 and Toll-like receptor 3 signaling pathways and up-regulation of vitamin D biosynthetic process pathway suggested that activity of DCs decreased, which may lead to a decrease in IL-27 expression and an increase in IL-21 expression. The decrease in microbial tryptophan metabolites may affect intestinal mucosal barrier integrity, allowing gut pathogens (such as Salmonella and Sarcina) to invade the host. Thus, these changes can induce the occurrence of inflammation and various gut diseases. Increased FFAs can lead to inflammation and accumulation of CARs, which may induce various metabolic diseases in aged CRs. CRs: Chinese rhesus macaques; AhR: Aryl hydrocarbon receptor; DCs: Dendritic cells; FFA: Free fatty acids; CAR: Acyl carnitine.
We found that the abundance of Lactobacillus, which is involved in tryptophan metabolism, decreased in aged CRs, leading to a decrease in AhR ligands (indoleacrylic acid, 3-indolepropionic acid, and indole-3-carboxaldehyde) in the plasma and down-regulation of the Toll-like receptor TLR1: TLR2 and Toll-like receptor 3 signaling pathways. Furthermore, IL-21 levels in the blood of aged CRs showed an increase. Based on these changes, we hypothesized that the decrease in Lactobacillus may eventually lead to an increase in IL-21 by affecting AhR and DCs. In addition, up-regulation of the vitamin D biosynthetic process pathway may affect antigen processing and presentation. As a result, host defenses against bacteria and viruses are reduced, which may result in gut and oral pathogen invasion (such as Salmonella, Sarcina, Filifactor, Treponema, Selenomonas, and Veillonella), leading to various gut and oral diseases.
Studies have shown that a lack of tryptophan can affect intestinal mucosal homeostasis, resulting in impaired intestinal immunity and altered gut microbial communities (Gao et al., 2018). Decreased tryptophan suggests that the intestinal mucosal barrier integrity was likely impaired in the aged CRs. Symptoms of intestinal barrier dysfunction and low-grade inflammation are common in metabolic diseases (Everard and Cani, 2013). Indeed, the increase in FFA and CAR accumulation indicated abnormal fatty acid metabolism, and the decrease in heparin, 2-HPPA CPD, and DHA-EE indicated cardiovascular disease in the aged CRs.
In conclusion, we applied transcriptomics, metagenomics, and metabolomics to study host–microbe interactions in aged CRs. Based on this multi-omics approach, we identified many potential links among differential microbiota, metabolites, and genes, indicating that aged CRs will likely experience multiple metabolic problems, immune disorders, and gut and oral diseases. Notably, we found that tryptophan metabolism is critical for the physiological health of aged CRs. Therefore, precise health management and care of aged CRs should be considered. Although the sample size was not large, our research showed that the multi-omics approach is robust and can reveal host–microbe interactions in non-human primates in the rapidly evolving fields of species conservation and molecular ecology. Thus, this approach could be applied to address species conservation and molecular ecology in other species.
The raw data of transcriptomes and metagenomes have been submitted to the China National GeneBank DataBase (CNGBdb) with the accession number CNP0002963. The identified metabolites were listed in the Supplementary Table.
This study was approved by the Ethics Committee of College of Life Sciences, Sichuan University (Nos. 20200327012 and 20210308001). We strictly obeyed the guidelines of the management committee of experimental animals of Sichuan Province, China (SYXK-Sichuan, 2019-192) in the sample collection and utility protocols.
JX, YL, XW, and JW collected the samples. JX, YL, XW, XL, JW, and KS performed the bioinformatics analyses. JX and XW performed the experiments. JX, YL, XW, and ZF wrote the manuscript. JL, BY, and MS revised the manuscript. MS and ZF designed and supervised the study. All authors contributed to the article and approved the submitted version.
This work was supported by the National Natural Science Foundation of China (Nos. 32070413 and 81900966).
The authors declare that the research was conducted in the absence of any commercial or financial relationships that could be construed as a potential conflict of interest.
All claims expressed in this article are solely those of the authors and do not necessarily represent those of their affiliated organizations, or those of the publisher, the editors and the reviewers. Any product that may be evaluated in this article, or claim that may be made by its manufacturer, is not guaranteed or endorsed by the publisher.
The Supplementary material for this article can be found online at: https://www.frontiersin.org/articles/10.3389/fmicb.2022.993879/full#supplementary-material
Aas, J. A., Griffen, A. L., Dardis, S. R., Lee, A. M., Olsen, I., Dewhirst, F. E., et al. (2008). Bacteria of dental caries in primary and permanent teeth in children and Young adults. J. Clin. Microbiol. 46, 1407–1417. doi: 10.1128/JCM.01410-07
Adachi, Y., Shimodaira, Y., Nakamura, H., Imaizumi, A., Mori, M., Kageyama, Y., et al. (2017). Low plasma tryptophan is associated with olfactory function in healthy elderly community dwellers in Japan. BMC Geriatr. 17:239. doi: 10.1186/s12877-017-0639-5
Ahadi, S., Zhou, W., Schüssler-Fiorenza Rose, S. M., Sailani, M. R., Contrepois, K., Avina, M., et al. (2020). Personal aging markers and ageotypes revealed by deep longitudinal profiling. Nat. Med. 26, 83–90.
Alcock, B. P., Raphenya, A. R., Lau, T. T. Y., Tsang, K. K., Bouchard, M., Edalatmand, A., et al. (2019). CARD 2020: antibiotic resistome surveillance with the comprehensive antibiotic resistance database. Nucleic Acids Res. 48, D517–D525. doi: 10.1093/nar/gkz935
Alexeev, E. E., Lanis, J. M., Kao, D. J., Campbell, E. L., Kelly, C. J., Battista, K. D., et al. (2018). Microbiota-derived Indole metabolites promote human and murine intestinal homeostasis through regulation of Interleukin-10 receptor. Am. J. Pathol. 188, 1183–1194. doi: 10.1016/j.ajpath.2018.01.011
Alpert, A., Pickman, Y., Leipold, M., Rosenberg-Hasson, Y., Ji, X., Gaujoux, R., et al. (2019). A clinically meaningful metric of immune age derived from high-dimensional longitudinal monitoring. Nat. Med. 25, 487–495. doi: 10.1038/s41591-019-0381-y
Antezack, A., Boxberger, M., Ben Khedher, M., La Scola, B., and Monnet-Corti, V. (2021). Isolation and description of Selenomonas timonae sp. nov., a novel Selenomonas species detected in a gingivitis patient. Int. J. Syst. Evol. Microbiol. 71:5040. doi: 10.1099/ijsem.0.005040
Apetoh, L., Quintana, F. J., Pot, C., Joller, N., Xiao, S., Kumar, D., et al. (2010). The aryl hydrocarbon receptor interacts with c-Maf to promote the differentiation of type 1 regulatory T cells induced by IL-27. Nat. Immunol. 11, 854–861. doi: 10.1038/ni.1912
Aruni, A. W., Roy, F., and Fletcher, H. M. (2011). Filifactor alocis has virulence attributes that can enhance its persistence under oxidative stress conditions and mediate invasion of epithelial cells by Porphyromonas gingivalis. Infect. Immun. 79, 3872–3886. doi: 10.1128/IAI.05631-11
Baker, J. L., Morton, J. T., Dinis, M., Alvarez, R., Tran, N. C., Knight, R., et al. (2021). Deep metagenomics examines the oral microbiome during dental caries, revealing novel taxa and co-occurrences with host molecules. Genome Res. 31, 64–74. doi: 10.1101/gr.265645.120
Balliu, B., Durrant, M., Goede, O. de, Abell, N., Li, X., Liu, B., et al. (2019). Genetic regulation of gene expression and splicing during a 10-year period of human aging. Genome Biol. 20:230. doi: 10.1186/s13059-019-1840-y
Bartels, L. E., Hvas, C. L., Agnholt, J., Dahlerup, J. F., and Agger, R. (2010). Human dendritic cell antigen presentation and chemotaxis are inhibited by intrinsic 25-hydroxy vitamin D activation. Int. Immunopharmacol. 10, 922–928. doi: 10.1016/j.intimp.2010.05.003
Barzilai, N., Huffman, D. M., Muzumdar, R. H., and Bartke, A. (2012). The critical role of metabolic pathways in aging. Diabetes 61, 1315–1322. doi: 10.2337/db11-1300
Bene, J., Hadzsiev, K., and Melegh, B. (2018). Role of carnitine and its derivatives in the development and management of type 2 diabetes. Nutr & Diabetes 8:8. doi: 10.1038/s41387-018-0017-1
Billing, L. J., Larraufie, P., Lewis, J., Leiter, A., Li, J., Lam, B., et al. (2019). Single cell transcriptomic profiling of large intestinal enteroendocrine cells in mice – identification of selective stimuli for insulin-like peptide-5 and glucagon-like peptide-1 co-expressing cells. Molecular Metabolism 29, 158–169. doi: 10.1016/j.molmet.2019.09.001
Bolger, A. M., Lohse, M., and Usadel, B. (2014). Trimmomatic: a flexible trimmer for Illumina sequence data. Bioinformatics 30, 2114–2120. doi: 10.1093/bioinformatics/btu170
Bolyen, E., Rideout, J. R., Dillon, M. R., Bokulich, N. A., Abnet, C. C., Al-Ghalith, G. A., et al. (2019). Reproducible, interactive, scalable and extensible microbiome data science using QIIME 2. Nat. Biotechnol. 37, 852–857. doi: 10.1038/s41587-019-0209-9
Bryois, J., Buil, A., Ferreira, P. G., Panousis, N. I., Brown, A. A., Viñuela, A., et al. (2017). Time-dependent genetic effects on gene expression implicate aging processes. Genome Res. 27, 545–552. doi: 10.1101/gr.207688.116
Buchfink, B., Xie, C., and Huson, D. H. (2015). Fast and sensitive protein alignment using DIAMOND. Nat. Methods 12, 59–60. doi: 10.1038/nmeth.3176
Bula-Rudas, F. J., Rathore, M. H., and Maraqa, N. F. (2015). Salmonella infections in childhood. Advances in Pediatrics 62, 29–58. doi: 10.1016/j.yapd.2015.04.005
Bunning, B. J., Contrepois, K., Lee-McMullen, B., Dhondalay, G. K. R., Zhang, W., Tupa, D., et al. (2020). Global metabolic profiling to model biological processes of aging in twins. Aging Cell 19:e13073. doi: 10.1111/acel.13073
Campisi, J., Kapahi, P., Lithgow, G. J., Melov, S., Newman, J. C., and Verdin, E. (2019). From discoveries in ageing research to therapeutics for healthy ageing. Nature 571, 183–192.
Chen, W., Jiang, Q., Yan, G., and Yang, D. (2020). The oral microbiome and salivary proteins influence caries in children aged 6 to 8 years. BMC Oral Health 20:295. doi: 10.1186/s12903-020-01262-9
Chen, Z., Yeoh, Y. K., Hui, M., Wong, P. Y., Chan, M. C. W., Ip, M., et al. (2018). Diversity of macaque microbiota compared to the human counterparts. Sci. Rep. 8:15573. doi: 10.1038/s41598-018-33950-6
Crane, J. D., Palanivel, R., Mottillo, E. P., Bujak, A. L., Wang, H., Ford, R. J., et al. (2015). Inhibiting peripheral serotonin synthesis reduces obesity and metabolic dysfunction by promoting brown adipose tissue thermogenesis. Nat. Med. 21, 166–172. doi: 10.1038/nm.3766
Daye, D., and Wellen, K. E. (2012). Metabolic reprogramming in cancer: unraveling the role of glutamine in tumorigenesis. Semin. Cell Dev. Biol. 23, 362–369. doi: 10.1016/j.semcdb.2012.02.002
Denz, H., Orth, B., Weiss, G., Herrmann, R., Huber, P., Wachter, H., et al. (1993). Weight loss in patients with hematological neoplasias is associated with immune system stimulation. Clin. Investig. 71, 37–41. doi: 10.1007/BF00210961
Ebersole, J., Kirakodu, S., Chen, J., Nagarajan, R., and Gonzalez, O. A. (2020). Oral microbiome and gingival Transcriptome profiles of ligature-induced periodontitis. J. Dent. Res. 99, 746–757. doi: 10.1177/0022034520906138
Everard, A., and Cani, P. D. (2013). Diabetes, obesity and gut microbiota. Best Pract. Res. Clin. Gastroenterol. 27, 73–83. doi: 10.1016/j.bpg.2013.03.007
Fan, X., Alekseyenko, A. V., Wu, J., Peters, B. A., Jacobs, E. J., Gapstur, S. M., et al. (2018). Human oral microbiome and prospective risk for pancreatic cancer: a population-based nested case-control study. Gut 67, 120–127. doi: 10.1136/gutjnl-2016-312580
Feng, Y., Peng, Y., Song, X., Wen, H., An, Y., Tang, H., et al. (2022). Anopheline mosquitoes are protected against parasite infection by tryptophan catabolism in gut microbiota. Nat. Microbiol. 7, 707–715. doi: 10.1038/s41564-022-01099-8
Franzosa, E. A. (2018). Species-level functional profiling of metagenomes and metatranscriptomes. Nat. Methods 15, 962–968. doi: 10.1038/s41592-018-0176-y
Fu, L., Niu, B., Zhu, Z., Wu, S., and Li, W. (2012). CD-HIT: accelerated for clustering the next-generation sequencing data. Bioinformatics 28, 3150–3152. doi: 10.1093/bioinformatics/bts565
Gao, J., Xu, K., Liu, H., Liu, G., Bai, M., Peng, C., et al. (2018). Impact of the gut microbiota on intestinal immunity mediated by tryptophan metabolism. Front. Cell. Infect. Microbiol. 8:13. doi: 10.3389/fcimb.2018.00013
Gibbs, R. A., Rogers, J., Katze, M. G., Bumgarner, R., Weinstock, G. M., Mardis, E. R., et al. (2007). Evolutionary and biomedical insights from the rhesus macaque genome. Science 316, 222–234. doi: 10.1126/science.1139247
Gilbert, J. A., Blaser, M. J., Caporaso, J. G., Jansson, J. K., Lynch, S. V., and Knight, R. (2018). Current understanding of the human microbiome. Nat. Med. 24, 392–400. doi: 10.1038/nm.4517
Gill, H. S., Rutherfurd, K. J., Cross, M. L., and Gopal, P. K. (2001). Enhancement of immunity in the elderly by dietary supplementation with the probiotic Bifidobacterium lactis HN019. Am. J. Clin. Nutr. 74, 833–839. doi: 10.1093/ajcn/74.6.833
Gogeneni, H., Buduneli, N., Ceyhan-Öztürk, B., Gümüş, P., Akcali, A., Zeller, I., et al. (2015). Increased infection with key periodontal pathogens during gestational diabetes mellitus. J. Clin. Periodontol. 42, 506–512. doi: 10.1111/jcpe.12418
Gray, E., Mulloy, B., and Barrowcliffe, T. (2008). Heparin and low-molecular-weight heparin. Thromb. Haemost. 99, 807–818. doi: 10.1160/TH08-01-0032
Hezel, M., and Weitzberg, E. (2015). The oral microbiome and nitric oxide homoeostasis. Oral Dis. 21, 7–16. doi: 10.1111/odi.12157
Hill, C. J., Lynch, D. B., Murphy, K., Ulaszewska, M., Jeffery, I. B., O’Shea, C. A., et al. (2017). Evolution of gut microbiota composition from birth to 24 weeks in the INFANTMET cohort. Microbiome 5:55x.
Hou, Y.-C. C., Yu, H.-C., Martin, R., Cirulli, E. T., Schenker-Ahmed, N. M., Hicks, M., et al. (2020). Precision medicine integrating whole-genome sequencing, comprehensive metabolomics, and advanced imaging. Proc. Natl. Acad. Sci. U. S. A. 117, 3053–3062. doi: 10.1073/pnas.1909378117
Hyatt, D., Chen, G.-L., LoCascio, P. F., Land, M. L., Larimer, F. W., and Hauser, L. J. (2010). Prodigal: prokaryotic gene recognition and translation initiation site identification. BMC Bioinformatics 11:119. doi: 10.1186/1471-2105-11-119
Iwagaki, H., Hizuta, A., Tanaka, N., and Orita, K. (1995). Decreased serum tryptophan in patients with cancer cachexia correlates with increased serum Neopterin. Immunol. Investig. 24, 467–478. doi: 10.3109/08820139509066843
Janiak, M. C., Montague, M. J., Villamil, C. I., Stock, M. K., Trujillo, A. E., DePasquale, A. N., et al. (2021). Age and sex-associated variation in the multi-site microbiome of an entire social group of free-ranging rhesus macaques. Microbiome 9:68.
Jia, J., Frantz, N., Khoo, C., Gibson, G. R., Rastall, R. A., and McCartney, A. L. (2010). Investigation ofthe faecal microbiota associated with canine chronic diarrhoea. FEMS Microbiol. Ecol. 71, 304–312. doi: 10.1111/j.1574-6941.2009.00812.x
Jijakli, K., and Jensen, P. A. (2019). Metabolic modeling of Streptococcus mutans reveals complex nutrient requirements of an Oral pathogen. mSystems 4, e00529–e00519. doi: 10.1128/mSystems.00529-19
Kaminski, J., Gibson, M. K., Franzosa, E. A., Segata, N., Dantas, G., and Huttenhower, C. (2015). High-specificity targeted functional profiling in microbial communities with ShortBRED. PLoS Comput. Biol. 11:e1004557. doi: 10.1371/journal.pcbi.1004557
Kastelein, R. A., Hunter, C. A., and Cua, D. J. (2007). Discovery and biology of IL-23 and IL-27: related but functionally distinct regulators of inflammation. Annu. Rev. Immunol. 25, 221–242. doi: 10.1146/annurev.immunol.22.012703.104758
Kaur, R., Gilbert, S. C., Sheehy, E. C., and Beighton, D. (2013). Salivary levels of Bifidobacteria in caries-free and caries-active children: salivary Bifidobacteria in children. Int. J. Paediatr. Dent. 23, 32–38. doi: 10.1111/j.1365-263X.2011.01220.x
Kawai, T., and Akira, S. (2011). Toll-like receptors and their crosstalk with other innate receptors in infection and immunity. Immunity 34, 637–650. doi: 10.1016/j.immuni.2011.05.006
Keating, D. J., and Spencer, N. J. (2019). What is the role of endogenous gut serotonin in the control of gastrointestinal motility? Pharmacol. Res. 140, 50–55. doi: 10.1016/j.phrs.2018.06.017
Kim, D., Langmead, B., and Salzberg, S. L. (2015). HISAT: a fast spliced aligner with low memory requirements. Nat. Methods 12, 357–360. doi: 10.1038/nmeth.3317
Krishnan, K., Chen, T., and Paster, B. (2017). A practical guide to the oral microbiome and its relation to health and disease. Oral Dis. 23, 276–286. doi: 10.1111/odi.12509
Kurilshikov, A., Medina-Gomez, C., Bacigalupe, R., Radjabzadeh, D., Wang, J., Demirkan, A., et al. (2021). Large-scale association analyses identify host factors influencing human gut microbiome composition. Nat. Genet. 53, 156–165. doi: 10.1038/s41588-020-00763-1
Lamas, B., Richard, M. L., Leducq, V., Pham, H.-P., Michel, M.-L., Da Costa, G., et al. (2016). CARD9 impacts colitis by altering gut microbiota metabolism of tryptophan into aryl hydrocarbon receptor ligands. Nat. Med. 22, 598–605. doi: 10.1038/nm.4102
Lam-Himlin, D., Pai, R. K., Eshleman, J. R., Bhagavan, B., and Anders, R. A. (2011). Sarcina organisms in the gastrointestinal tract: a Clinicopathologic and molecular study. Am. J. Surg. Pathol. 35, 1700–1705. doi: 10.1097/PAS.0b013e31822911e6
Lan, Y., Wang, J., Yang, Q., Tang, R.-X., Zhou, M., Lei, G.-L., et al. (2020). Blood transcriptome analysis reveals gene expression features of breast-feeding rhesus macaque (Macaca mulatta) infants. Zool. Res. 41, 431–436. doi: 10.24272/j.issn.2095-8137.2020.044
Langmead, B., and Salzberg, S. L. (2012). Fast gapped-read alignment with bowtie 2. Nat. Methods 9, 357–359. doi: 10.1038/nmeth.1923
Lehallier, B., Gate, D., Schaum, N., Nanasi, T., Lee, S. E., Yousef, H., et al. (2019). Undulating changes in human plasma proteome profiles across the lifespan. Nat. Med. 25, 1843–1850.
Li, D., Liu, C.-M., Luo, R., Sadakane, K., and Lam, T.-W. (2015). MEGAHIT: an ultra-fast single-node solution for large and complex metagenomics assembly via succinct de Bruijn graph. Bioinformatics 31, 1674–1676. doi: 10.1093/bioinformatics/btv033
Li, M.-L., Wu, S.-H., Zhang, J.-J., Tian, H.-Y., Shao, Y., Wang, Z.-B., et al. (2019). 547 transcriptomes from 44 brain areas reveal features of the aging brain in non-human primates. Genome Biol. 20:258.
Linninge, C., Xu, J., Bahl, M. I., Ahrné, S., and Molin, G. (2019). Lactobacillus fermentum and lactobacillus plantarum increased gut microbiota diversity and functionality, and mitigated Enterobacteriaceae, in a mouse model. Benefic. Microbes 10, 413–424. doi: 10.3920/BM2018.0074
Lombard, V., Golaconda Ramulu, H., Drula, E., Coutinho, P. M., and Henrissat, B. (2014). The carbohydrate-active enzymes database (CAZy) in 2013. Nucleic Acids Res. 42, D490–D495. doi: 10.1093/nar/gkt1178
López-Otín, C., Blasco, M. A., Partridge, L., Serrano, M., and Kroemer, G. (2013). The hallmarks of aging. Cells 153, 1194–1217. doi: 10.1016/j.cell.2013.05.039
Love, M. I., Huber, W., and Anders, S. (2014). Moderated estimation of fold change and dispersion for RNA-seq data with DESeq2. Genome Biol. 15:550. doi: 10.1186/s13059-014-0550-8
Manara, S., Asnicar, F., Beghini, F., Bazzani, D., Cumbo, F., Zolfo, M., et al. (2019). Microbial genomes from non-human primate gut metagenomes expand the primate-associated bacterial tree of life with over 1000 novel species. Genome Biol. 20:299. doi: 10.1186/s13059-019-1923-9
Manel, N., Unutmaz, D., and Littman, D. R. (2008). The differentiation of human TH-17 cells requires transforming growth factor-β and induction of the nuclear receptor RORγt. Nat. Immunol. 9, 641–649. doi: 10.1038/ni.1610
Manome, A., Abiko, Y., Kawashima, J., Washio, J., Fukumoto, S., and Takahashi, N. (2019). Acidogenic potential of Oral Bifidobacterium and its high fluoride tolerance. Front. Microbiol. 10:1099. doi: 10.3389/fmicb.2019.01099
Mantzourani, M., Gilbert, S. C., Sulong, H. N. H., Sheehy, E. C., Tank, S., Fenlon, M., et al. (2009). The isolation of Bifidobacteria from Occlusal carious lesions in children and adults. Caries Res. 43, 308–313. doi: 10.1159/000222659
Martin, A. M., Young, R. L., Leong, L., Rogers, G. B., Spencer, N. J., Jessup, C. F., et al. (2017). The diverse metabolic roles of peripheral serotonin. Endocrinology 158, 1049–1063. doi: 10.1210/en.2016-1839
Martínez, M., Vázquez, E., García-Silva, M. T., Manzanares, J., Bertran, J. M., Castelló, F., et al. (2000). Therapeutic effects of docosahexaenoic acid ethyl ester in patients with generalized peroxisomal disorders. Am. J. Clin. Nutr. 71, 376s–385s. doi: 10.1093/ajcn/71.1.376s
Martino, C., Morton, J. T., Marotz, C. A., Thompson, L. R., Tripathi, A., Knight, R., et al. (2019). A novel sparse compositional technique reveals microbial perturbations. mSystems 4, e00016–e00019. doi: 10.1128/mSystems.00016-19
Mattison, J. A., Colman, R. J., Beasley, T. M., Allison, D. B., Kemnitz, J. W., Roth, G. S., et al. (2017). Caloric restriction improves health and survival of rhesus monkeys. Nat. Commun. 8:14063.
Mihalik, S. J., Goodpaster, B. H., Kelley, D. E., Chace, D. H., Vockley, J., Toledo, F. G. S., et al. (2010). Increased levels of plasma Acylcarnitines in obesity and type 2 diabetes and identification of a marker of Glucolipotoxicity. Obesity 18, 1695–1700. doi: 10.1038/oby.2009.510
Möder, M., Kießling, A., Löster, H., and Brüggemann, L. (2003). The pattern of urinary acylcarnitines determined by electrospray mass spectrometry: a new tool in the diagnosis of diabetes mellitus. Anal. Bioanal. Chem. 375, 200–210. doi: 10.1007/s00216-002-1654-7
Moffatt, C. E., Whitmore, S. E., Griffen, A. L., Leys, E. J., and Lamont, R. J. (2011). Filifactor alocis interactions with gingival epithelial cells: F. alocis and gingival epithelial cells. Mol Oral Microbiol 26, 365–373. doi: 10.1111/j.2041-1014.2011.00624.x
Molle, C., Goldman, M., and Goriely, S. (2010). Critical role of the IFN-stimulated gene factor 3 complex in TLR-mediated IL-27p28 gene expression revealing a two-step activation process. J.Immunol. 184, 1784–1792. doi: 10.4049/jimmunol.0902005.
Munn, D. H., Sharma, M. D., Baban, B., Harding, H. P., Zhang, Y., Ron, D., et al. (2005). GCN2 kinase in T cells mediates proliferative arrest and Anergy induction in response to Indoleamine 2,3-Dioxygenase. Immunity 22, 633–642. doi: 10.1016/j.immuni.2005.03.013
Munson, M. A., Banerjee, A., Watson, T. F., and Wade, W. G. (2004). Molecular analysis of the microflora associated with dental caries. J. Clin. Microbiol. 42, 3023–3029. doi: 10.1128/jcm.42.7.3023-3029.2004
Natividad, J. M., Agus, A., Planchais, J., Lamas, B., Jarry, A. C., Martin, R., et al. (2018). Impaired aryl hydrocarbon receptor ligand production by the gut microbiota is a key factor in metabolic syndrome. Cell Metab. 28, 737–749.e4. doi: 10.1016/j.cmet.2018.07.001
Nóvoa, L., del Sánchez, M. C., Blanco, J., Limeres, J., Cuenca, M., Marín, M. J., et al. (2020). The subgingival microbiome in patients with down syndrome and periodontitis. JCM 9:2482. doi: 10.3390/jcm9082482
Nyvad, B., and Kilian, M. (1990). Microflora associated with experimental root surface caries in humans. Infect. Immun. 58, 1628–1633. doi: 10.1128/iai.58.6.1628-1633.1990
O’Toole, P. W., and Jeffery, I. B. (2015). Gut microbiota and aging. Science 350, 1214–1215. doi: 10.1126/science.aac8469
Olsen, I., and Singhrao, S. K. (2015). Can oral infection be a risk factor for Alzheimer’s disease? J. Oral Microbiol. 7:29143. doi: 10.3402/jom.v7.29143
Otasek, D., Morris, J. H., Bouças, J., Pico, A. R., and Demchak, B. (2019). Cytoscape automation: empowering workflow-based network analysis. Genome Biol. 20:185. doi: 10.1186/s13059-019-1758-4
Pasolli, E., Asnicar, F., Manara, S., Zolfo, M., Karcher, N., Armanini, F., et al. (2019). Extensive unexplored human microbiome diversity revealed by over 150,000 genomes from Metagenomes spanning age, geography, and lifestyle. Cells 176, 649–662.e20. doi: 10.1016/j.cell.2019.01.001
Patel, R. K., and Jain, M. (2012). NGS QC toolkit: a toolkit for quality control of next generation sequencing data. PLoS One 7:e30619. doi: 10.1371/journal.pone.0030619
Patro, R. (2017). Salmon provides fast and bias-aware quantification of transcript expression. Nat. Methods 14, 417–419. doi: 10.1038/nmeth.4197
Pertea, M., Pertea, G. M., Antonescu, C. M., Chang, T.-C., Mendell, J. T., and Salzberg, S. L. (2015). StringTie enables improved reconstruction of a transcriptome from RNA-seq reads. Nat. Biotechnol. 33, 290–295. doi: 10.1038/nbt.3122
Peters, M. J., Joehanes, R., Pilling, L. C., Schurmann, C., Conneely, K. N., Powell, J., et al. (2015). The transcriptional landscape of age in human peripheral blood. Nat. Commun. 6:8570. doi: 10.1038/ncomms9570
Price, N. D., Magis, A. T., Earls, J. C., Glusman, G., Levy, R., Lausted, C., et al. (2017). A wellness study of 108 individuals using personal, dense, dynamic data clouds. Nat. Biotechnol. 35, 747–756. doi: 10.1038/nbt.3870
Raudvere, U., Kolberg, L., Kuzmin, I., Arak, T., Adler, P., Peterson, H., et al. (2019). G:profiler: a web server for functional enrichment analysis and conversions of gene lists (2019 update). Nucleic Acids Res. 47, W191–W198. doi: 10.1093/nar/gkz369
Reid, G., and Burton, J. (2002). Use of lactobacillus to prevent infection by pathogenic bacteria. Microbes Infect. 4, 319–324. doi: 10.1016/S1286-4579(02)01544-7
Richieri, G. V., and Kleinfeld, A. M. (1995). Unbound free fatty acid levels in human serum. J. Lipid Res. 36, 229–240. doi: 10.1016/S0022-2275(20)39899-0
Rinschen, M. M., Ivanisevic, J., Giera, M., and Siuzdak, G. (2019). Identification of bioactive metabolites using activity metabolomics. Nat. Rev. Mol. Cell Biol. 20, 353–367. doi: 10.1038/s41580-019-0108-4
Rivera-Chávez, F., and Bäumler, A. J. (2015). The pyromaniac inside you: salmonella metabolism in the host gut. Annu. Rev. Microbiol. 69, 31–48. doi: 10.1146/annurev-micro-091014-104108
Rossi, M., Martínez-Martínez, D., Amaretti, A., Ulrici, A., Raimondi, S., and Moya, A. (2016). Mining metagenomic whole genome sequences revealed subdominant but constant lactobacillus population in the human gut microbiota. Environ. Microbiol. Rep. 8, 399–406. doi: 10.1111/1758-2229.12405
Roswall, J., Olsson, L. M., Kovatcheva-Datchary, P., Nilsson, S., Tremaroli, V., Simon, M.-C., et al. (2021). Developmental trajectory of the healthy human gut microbiota during the first 5 years of life. Cell Host Microbe 29, 765–776.e3.
Schulz, S., Porsch, M., Grosse, I., Hoffmann, K., Schaller, H.-G., and Reichert, S. (2019). Comparison of the oral microbiome of patients with generalized aggressive periodontitis and periodontitis-free subjects. Arch. Oral Biol. 99, 169–176. doi: 10.1016/j.archoralbio.2019.01.015
Schüssler-Fiorenza Rose, S. M., Contrepois, K., Moneghetti, K. J., Zhou, W., Mishra, T., Mataraso, S., et al. (2019). A longitudinal big data approach for precision health. Nat. Med. 25, 792–804. doi: 10.1038/s41591-019-0414-6
Seder, R. A., Gazzinelli, R., Sher, A., and Paul, W. E. (1993). Interleukin 12 acts directly on CD4+ T cells to enhance priming for interferon gamma production and diminishes interleukin 4 inhibition of such priming. Proc. Natl. Acad. Sci. U. S. A. 90, 10188–10192. doi: 10.1073/pnas.90.21.10188
Segata, N., Izard, J., Waldron, L., Gevers, D., Miropolsky, L., Garrett, W. S., et al. (2011). Metagenomic biomarker discovery and explanation. Genome Biol. 12:R60. doi: 10.1186/gb-2011-12-6-r60
Shoemark, D. K., and Allen, S. J. (2015). The microbiome and disease: reviewing the links between the Oral microbiome, aging, and Alzheimer’s disease. J. Alzheimers Dis. 43, 725–738. doi: 10.3233/JAD-141170
Socransky, S. S., Haffajee, A. D., Cugini, M. A., Smith, C., and Kent, R. L. (1998). Microbial complexes in subgingival plaque. J. Clin. Periodontol. 25, 134–144. doi: 10.1111/j.1600-051X.1998.tb02419.x
Sommer, F., and Bäckhed, F. (2013). The gut microbiota — masters of host development and physiology. Nat. Rev. Microbiol. 11, 227–238. doi: 10.1038/nrmicro2974
Spinler, J. K., Taweechotipatr, M., Rognerud, C. L., Ou, C. N., Tumwasorn, S., and Versalovic, J. (2008). Human-derived probiotic lactobacillus reuteri demonstrate antimicrobial activities targeting diverse enteric bacterial pathogens. Anaerobe 14, 166–171. doi: 10.1016/j.anaerobe.2008.02.001
Stumhofer, J. S., Silver, J. S., Laurence, A., Porrett, P. M., Harris, T. H., Turka, L. A., et al. (2007). Interleukins 27 and 6 induce STAT3-mediated T cell production of interleukin 10. Nat. Immunol. 8, 1363–1371. doi: 10.1038/ni1537
Suzek, B. E., Huang, H., McGarvey, P., Mazumder, R., and Wu, C. H. (2007). UniRef: comprehensive and non-redundant UniProt reference clusters. Bioinformatics 23, 1282–1288. doi: 10.1093/bioinformatics/btm098
Tanner, A. C. R., Kent, R. L., Holgerson, P. L., Hughes, C. V., Loo, C. Y., Kanasi, E., et al. (2011). Microbiota of severe early childhood caries before and after therapy. J. Dent. Res. 90, 1298–1305. doi: 10.1177/0022034511421201
Tanner, A., Kent, R., Maiden, M. F. J., and Taubman, M. A. (1996). Clinical, microbiological and immunological profile of healthy, gingivitis and putative active periodontal subjects. J. Periodontal Res. 31, 195–204. doi: 10.1111/j.1600-0765.1996.tb00484.x
Tanner, A., Maiden, M. F. J., Macuch, P. J., Murray, L. L., and Kent, R. L. (1998). Microbiota of health, gingivitis, and initial periodontitis. J. Clin. Periodontol. 25, 85–98. doi: 10.1111/j.1600-051X.1998.tb02414.x
Tett, A., Huang, K. D., Asnicar, F., Fehlner-Peach, H., Pasolli, E., Karcher, N., et al. (2019). The Prevotella copri complex comprises four distinct clades underrepresented in westernized populations. Cell Host Microbe 26, 666–679.e7. doi: 10.1016/j.chom.2019.08.018
Tina, E., Prosén, S., Lennholm, S., Gasparyan, G., Lindberg, M., and Göthlin Eremo, A. (2019). Expression profile of the amino acid transporters SLC7A5, SLC7A7, SLC7A8 and the enzyme TDO2 in basal cell carcinoma. Br. J. Dermatol. 180, 130–140. doi: 10.1111/bjd.16905
Tumova, J., Andel, M., and Trnka, J. (2016). Excess of free fatty acids as a cause of metabolic dysfunction in skeletal muscle. Physiol. Res. 65, 193–207. doi: 10.33549/physiolres.932993
Valenzuela, A., Valenzuela, V., Sanhueza, J., and Nieto, S. (2005). Effect of supplementation with Docosahexaenoic acid ethyl Ester and sn-2 Docosahexaenyl Monoacylglyceride on plasma and erythrocyte fatty acids in rats. Ann. Nutr. Metab. 49, 49–53. doi: 10.1159/000084177
Valeriano, V. D. V., Balolong, M. P., and Kang, D.-K. (2017). Probiotic roles of lactobacillus sp. in swine: insights from gut microbiota. J. Appl. Microbiol. 122, 554–567. doi: 10.1111/jam.13364
Walther, D. J., Peter, J.-U., Bashammakh, S., Hörtnagl, H., Voits, M., Fink, H., et al. (2003). Synthesis of serotonin by a second tryptophan hydroxylase isoform. Science 299:76. doi: 10.1126/science.1078197
Wei, W., An, X.-R., Jin, S.-J., Li, X.-X., and Xu, M. (2018). Inhibition of insulin resistance by PGE1 via autophagy-dependent FGF21 pathway in diabetic nephropathy. Sci. Rep. 8:9. doi: 10.1038/s41598-017-18427-2
Widner, B., Leblhuber, F., Walli, J., Tilz, G. P., Demel, U., and Fuchs, D. (1999). “Degradation of tryptophan in neurodegenerative disorders,” in Tryptophan, serotonin, and melatonin advances in experimental medicine and biology. eds. G. Huether, W. Kochen, T. J. Simat, and H. Steinhart (Boston, MA: Springer US), 133–138.
Widner, B., Leblhuber, F., Walli, J., Tilz, G. P., Demel, U., and Fuchs, D. (2000). Tryptophan degradation and immune activation in Alzheimer’s disease. J. Neural Transm. 107, 343–353. doi: 10.1007/s007020050029
Wilmanski, T., Diener, C., Rappaport, N., Patwardhan, S., Wiedrick, J., Lapidus, J., et al. (2021). Gut microbiome pattern reflects healthy ageing and predicts survival in humans. Nat. Metab. 3, 274–286. doi: 10.1038/s42255-021-00348-0
Wood, D. E., and Salzberg, S. L. (2014). Kraken: ultrafast metagenomic sequence classification using exact alignments. Genome Biol. 15:R46. doi: 10.1186/gb-2014-15-3-r46
Wu, G. D., Chen, J., Hoffmann, C., Bittinger, K., Chen, Y.-Y., Keilbaugh, S. A., et al. (2011). Linking long-term dietary patterns with gut microbial Enterotypes. Science 334, 105–108. doi: 10.1126/science.1208344
Xiao, J., Fiscella, K. A., and Gill, S. R. (2020). Oral microbiome: possible harbinger for children’s health. Int. J. Oral Sci. 12:12. doi: 10.1038/s41368-020-0082-x
Yamanishi, Y., Araki, M., Gutteridge, A., Honda, W., and Kanehisa, M. (2008). Prediction of drug-target interaction networks from the integration of chemical and genomic spaces. Bioinformatics 24, i232–i240. doi: 10.1093/bioinformatics/btn162
Yan, C.-C., Zhang, X.-S., Zhou, L., Yang, Q., Zhou, M., Zhang, L.-W., et al. (2020). Effects of aging on gene expression in blood of captive Tibetan macaques (Macaca thibetana) and comparisons with expression in humans. Zool. Res. 41, 557–563. doi: 10.24272/j.issn.2095-8137.2020.092
Yang, C.-Y., Yeh, Y.-M., Yu, H.-Y., Chin, C.-Y., Hsu, C.-W., Liu, H., et al. (2018). Oral microbiota community dynamics associated with Oral squamous cell carcinoma staging. Front. Microbiol. 9:862. doi: 10.3389/fmicb.2018.00862
Zaura, E., Keijser, B. J., Huse, S. M., and Crielaard, W. (2009). Defining the healthy “core microbiome” of oral microbial communities. BMC Microbiol. 9:259. doi: 10.1186/1471-2180-9-259
Zelante, T., Iannitti, R. G., Cunha, C., De Luca, A., Giovannini, G., Pieraccini, G., et al. (2013). Tryptophan Catabolites from microbiota engage aryl hydrocarbon receptor and balance mucosal reactivity via Interleukin-22. Immunity 39, 372–385. doi: 10.1016/j.immuni.2013.08.003
Zhang, Z., Mu, X., Cao, Q., Shi, Y., Hu, X., and Zheng, H. (2022a). Honeybee gut lactobacillus modulates host learning and memory behaviors via regulating tryptophan metabolism. Nat. Commun. 13:2037. doi: 10.1038/s41467-022-29760-0
Zhang, Z., Mu, X., Shi, Y., and Zheng, H. (2022b). Distinct roles of honeybee gut bacteria on host metabolism and neurological processes. Microbiol Spectr 10:e0243821. doi: 10.1128/spectrum.02438-21
Zhang, N., Wang, Y., Kan, J., Wu, X., Zhang, X., Tang, S., et al. (2019). In vivo and in vitro anti-inflammatory effects of water-soluble polysaccharide from Arctium lappa. Int. J. Biol. Macromol. 135, 717–724. doi: 10.1016/j.ijbiomac.2019.05.171
Zhang, X., Zhang, C., Chen, L., Han, X., and Ji, L. (2014). Human serum acylcarnitine profiles in different glucose tolerance states. Diabetes Res. Clin. Pract. 104, 376–382. doi: 10.1016/j.diabres.2014.04.013
Zhang, X., Zhong, H., Li, Y., Shi, Z., Ren, H., Zhang, Z., et al. (2021). Sex-and age-related trajectories of the adult human gut microbiota shared across populations of different ethnicities. Nat Aging 1, 87–100.
Zhou, W., Sailani, M. R., Contrepois, K., Zhou, Y., Ahadi, S., Leopold, S. R., et al. (2019). Longitudinal multi-omics of host–microbe dynamics in prediabetes. Nature 569, 663–671. doi: 10.1038/s41586-019-1236-x
Keywords: non-human primates, multi-omics, oral and gut microbiome, blood transcriptome and metabolome, aging
Citation: Xu J, Lan Y, Wang X, Shang K, Liu X, Wang J, Li J, Yue B, Shao M and Fan Z (2022) Multi-omics analysis reveals the host–microbe interactions in aged rhesus macaques. Front. Microbiol. 13:993879. doi: 10.3389/fmicb.2022.993879
Received: 14 July 2022; Accepted: 08 September 2022;
Published: 27 September 2022.
Edited by:
Zhigang Zhang, Yunnan University, ChinaCopyright © 2022 Xu, Lan, Wang, Shang, Liu, Wang, Li, Yue, Shao and Fan. This is an open-access article distributed under the terms of the Creative Commons Attribution License (CC BY). The use, distribution or reproduction in other forums is permitted, provided the original author(s) and the copyright owner(s) are credited and that the original publication in this journal is cited, in accordance with accepted academic practice. No use, distribution or reproduction is permitted which does not comply with these terms.
*Correspondence: Meiying Shao, c2hhb21laXlpbmcyMDEzQDE2My5jb20=; Zhenxin Fan, enhmYW5Ac2N1LmVkdS5jbg==
†These authors have contributed equally to this work
Disclaimer: All claims expressed in this article are solely those of the authors and do not necessarily represent those of their affiliated organizations, or those of the publisher, the editors and the reviewers. Any product that may be evaluated in this article or claim that may be made by its manufacturer is not guaranteed or endorsed by the publisher.
Research integrity at Frontiers
Learn more about the work of our research integrity team to safeguard the quality of each article we publish.