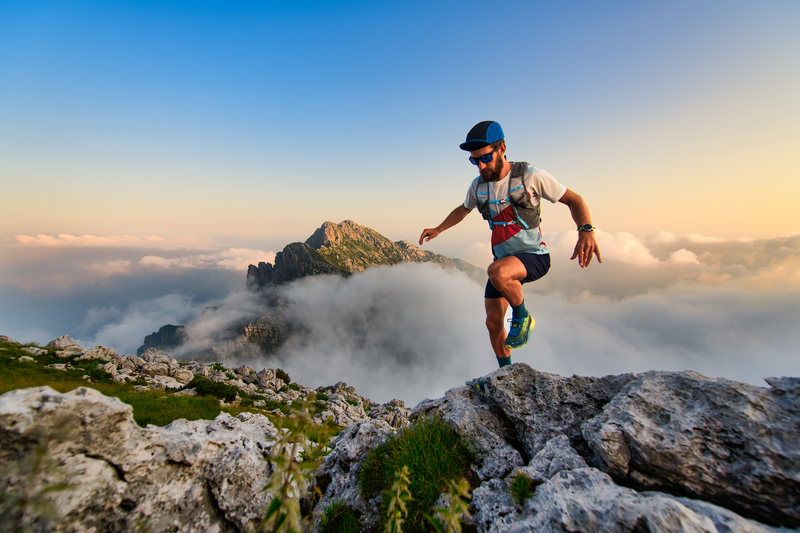
94% of researchers rate our articles as excellent or good
Learn more about the work of our research integrity team to safeguard the quality of each article we publish.
Find out more
ORIGINAL RESEARCH article
Front. Microbiol. , 25 August 2022
Sec. Microbiotechnology
Volume 13 - 2022 | https://doi.org/10.3389/fmicb.2022.992816
This article is part of the Research Topic Application of Microbial Technology in Ecological Remediation of Mines View all 6 articles
Vegetation recovery is an important marker of ecosystem health in the mining area. Clarifying the influence of vegetation recovery on the characteristics of soil microbial community and its assembly process can improve our understanding of the ecological resilience and self-maintaining mechanism in the open-pit mining area. For this purpose, we employed MiSeq high-throughput sequencing coupled with null model analysis to determine the composition, molecular ecological network characteristics, key bacterial and fungal clusters, and the assembly mechanism of the soil microbial communities in shrubs (BL), coniferous forest (CF), broad-leaved forests (BF), mixed forest (MF), and the control plot (CK, the poplar plantation nearby that had been continuously grown for over 30 a without disturbance). The results showed that the vegetation restoration model had a significant influence on the α-diversity of the microbial community (p < 0.05). Compared with CK, Sobs and Shannon index of MF and CF have increased by 35.29, 3.50, and 25.18%, 1.05%, respectively, whereas there was no significant difference in the α-diversity of fungal community among different vegetation restoration types, Actinobacteria, Chloroflexi, Proteobacteria, and Acidobacteria were the dominant phyla. The diversity of the first two phyla was significantly higher than those of CK. However, the diversity of the last two phyla was dramatically lower than those of CK (p < 0.05). Ascomycota and Basidiomycota were dominant phyla in the fungal community. The abundance and diversity of Ascomycota were significantly higher than those of CK, while the abundance and diversity of the latter were considerably lower than those of CK (p < 0.05). The stochastic process governed the assembly of the soil microbial community, and the contribution rate to the bacterial community construction of CK, CF, BF, and MF was 100.0%. Except for MF, where the soil fungal community assembly was governed by the deterministic process, all other fungal communities were governed by the stochastic process. Proteobacteria and Acidobacteria are key taxa of the bacterial network, while Mortierellales, Thelebolales, Chaetothyriales, and Hypocreales are the key taxa of the fungal network. All these results might provide the theoretical foundation for restoring the fragile ecosystem in the global mining region.
Open-pit mining can cause extremely severe damage to the ecosystem on the earth surface. Soil and vegetation damage is widespread in the open-pit mining area (Wang et al., 2016). The local eco-environment is usually altered, which further affects the biodiversity and functional stability of the ecosystem (Li et al., 2014; Baldrian, 2017). The Loess Plateau, an ecologically vulnerable region, produces about 30% of the world’s total coal. Mining has aggravated surface collapse and water and soil loss, leading to soil depletion and vegetation degradation (Festin et al., 2019; Gastauer et al., 2019). All these ecological phenomena have severely restricted regional sustainable development. Ecological repair and governance are pressing issues at the moment. Vegetation recovery measures the degree of ecological restoration in the ecologically damaged mining areas (Crouzeilles et al., 2016; Zhao et al., 2021). Taking full advantage of the self-recovery ability of the soil–plant system is vital for building a relatively stable ecosystem (Bahram et al., 2020; Yang et al., 2020). The Loess Plateau is featured by low precipitation and poor water-and fertility-retaining abilities, especially in the reclaimed soils of the mining area. Vegetation restoration is a highly challenging task because this region has prevalent “small old trees,” and a persistent drought may cause extensive wilting and death of trees. In addition, soil ecosystem conservation and resilience reconstruction tend to be neglected during the manual repair of some mining areas. As a result, the reconstructed soils are too underdeveloped to satisfy the growth needs of higher plants, such as trees. Given the above, stimulating the natural resilience and promoting the self-repair of reconstructed soils are essential for vegetation restoration in the mining areas (Yang et al., 2018; Liu et al., 2019).
In recent years, there has been growing scholarly attention towards the utilization of microbes in soil-vegetation system restoration. Microbes are the most extensively distributed and active constituents in the earth’s surface ecosystem, driving substance cycling and nutrient conversion (Shi et al., 2016; Baldrian, 2017). Microbes are regulators of and catalysts for belowground ecological processes. Therefore, recognizing the interactions between soil microbial species and the mechanism of mutual feedback between microbes and plants can improve the understanding of the functional diversity and roles of the microbial community (Barberan et al., 2012; Faust and Raes, 2012). Wei et al. found that metabolic activities such as Acetobacter respiration and nitrification in soil were closely related to vegetation types. Sharrar et al. (2020) confirmed that the Acidobacteria generally existed in the grassland soils of grass, which had the highest biosynthetic potential capacity. It was also mentioned that the enrichment degree of Actinobacteria in the grassland was significantly higher than that in the trees, while the subtaxa with the highest biosynthetic potential were enriched in the hills. Some scholars have already studied the features of soil microbial communities after vegetation restoration in the Loess Plateau (Banerjee et al., 2016, 2019; Deng et al., 2016). They are mainly concerned with the composition of the soil microbial community and its correlations with environmental factors (Banerjee et al., 2016; Xu et al., 2022). However, the assembly mechanism of complex microbial communities is rarely discussed. A null model based on β-diversity indices (βNTI and RCbray) was developed to quantify the process of microbial community assembly (Graham et al., 2017). The model offers a tool to quantitatively elucidate the ecological mechanism of soil microbial co-occurrence under different vegetation restoration models and assess the soil microbial community assembly and succession process (Barberan et al., 2012). Considering the soil microbial community, the deterministic and stochastic processes are two mutually complementary mechanisms that play important roles. The deterministic process is determined by non-biological (e.g., pH value and temperature) and biological factors (e.g., competition among individuals of different species and predator–prey relationship). More specifically, the deterministic process comes into play by differentiating preferences and adaptability of microbes to different habitats. Microbial communities vary with the vegetation restoration model and land use type (Zhang et al., 2011, 2016; Thomson et al., 2015). The natural history and physiology of different vegetation types also vary (Eviner, 2004), resulting in the formation of a unique soil environment and biotic communities (Zhao et al., 2021). By contrast, the stochastic process highlights the roles of dispersal and ecological drift (Zhou and Ning, 2017). The soil microbial community can track the changes in the aboveground plant community (Peay et al., 2013). The influence of specific plant species on the composition of the soil microbial community is closely related to the soil type (Bulgarelli et al., 2012). The soil-vegetation interaction is a highly complex process during the ecological restoration of the mining area; it is not only restricted by local natural environment elements but also influenced by foreign soils and alien plants. These two sources of influence control the resilience of the soil-vegetation system (Zhou and Ning, 2017; Mori et al., 2018). Understanding the soil-vegetation interaction is significant for the natural restoration and health of the mining area ecosystem (Mori et al., 2018).
Microbial network analysis is a valuable tool for studying the complex relationship between the species (Barberan et al., 2012; Faust and Raes, 2012) and identifying the interactions between microbial clusters and the key populations (Deng et al., 2016; Nuccio et al., 2016). Microbial network analysis can help monitor and evaluate key microbial clusters and changes in relevant ecological functions (Banerjee et al., 2016; Shi et al., 2018). One study has shown that deterministic factors have a more significant impact on a larger spatial scale (Shi et al., 2018). So, the question is: Is the microbial community assembly in reconstructed soils in the mining area of the Loess Plateau governed by the deterministic process? Or, is the stochastic process exerting the governing effect? Finding an answer to this question can facilitate understanding ecosystem resilience regulation and ecosystem self-maintaining mechanism reconstruction in the ecologically damaged mining area. In the present study, we focused on the reclaimed forests at the Antaibao Open-Pit Mine dumping site in Pingshuo, Loess Plateau. Forty samples were collected from four vegetation types and one control and examined for structural and functional changes in soil microbial communities under different vegetation restoration models. The purpose was to explore the differentiation in the microbial molecular ecological network, key microbial clusters, and their interactions under different vegetation restoration models. This study tried to uncover the influence of vegetation restoration on the soil microbial community assembly in the mining area and the working mechanism. This study offers new insights into the natural restoration of the ecosystem in the ecologically damaged mining area.
China is the country with the largest coal consumption in the world, accounting for more than 50% of the global coal energy consumption. Most of the theoretical and technical research on mine ecological restoration is concentrated in the middle- and low-altitude areas. The difficulty and effect of restoration depend on natural conditions. The selection of vegetation species in the environment, restrictive factors, material ratio, slope protection mechanism, and restoration effect evaluation will be the key links in the future research on ecological restoration of mine slopes. The south dumping site of the Antaibao open-pit mine is located in Shuozhou, Shanxi Province (112°10′–113°30′E, 39°23′–30°37′N; Figure 1). This mining area has a typical temperate continental semi-arid monsoon climate, with an annual average precipitation of 426.7 mm and an annual average temperature of 5.6°C. However, the precipitation is mostly concentrated from June to September, and the annual average precipitation reaches 2,150 mm. The primary soil type is chestnut soil, with the sand, loam, and clay components accounting for 2.3%, 65.9%, and 31.8%, respectively. The bulk weight of the soil ranged between 1.27 and 1.74 g·cm−3, and the organic matter content was found to be low. This dumping site covers an area of 180.5 hm2 and has an altitude of 1,465 m. The land reclamation first began in 1992 through layer-by-layer bulldozing and compaction based on height difference, followed by backfilling foreign soils to a depth of 50–60 cm. The reclaimed region is designed as a stepped terrain with an alternation of terraces and side slopes and a relative height of 140 m (Li et al., 2014). The primary plants grown for restoration included: Pinus tabulaeformis, Robinia pseudoacacia, Elm, Willow, Pinus tabulaeformis + Elm, Caragana, and Seabuckthorn. Stipa sareptana and Elymus dahuricus were aerially seeded.
From July 29 to 30, 2020, we collected soil samples from the shrubs (BL) where Caragana species were dominant, coniferous forest (CF) comprising Pinus tabulaeformis alone, broadleaved forest (BF) comprising Robinia pseudoacacia alone, mixed forest (MF) composed of Pinus tabulaeformis + Elm, and unreclaimed plot (CK). For the collection of each sample, about 200 g of topsoil was dug up from a depth of 0–10 cm at each of the five points chosen randomly. The five parts were combined into a 1,000 g compound sample. Eight duplicates were set up for each vegetation type, and 40 samples were collected in total. The plant roots and gravel were removed on site. The soil samples were mixed adequately, sealed under sterile conditions, and stored in the car fridge at −20°C. Parts of the fresh soil samples were directly refrigerated in the foam boxes, sealed, and mailed to Majorbio Bio-pharm Technology (Shanghai) for sequencing.
Total RNA was extracted from the soil samples according to the instruction provided with the E.Z.N.A.® soil kit (Omega Bio-Tek, Norcross, GA, United States). DNA concentration and purity were determined using a NanoDrop 2000 spectrophotometer. The DNA quality was assessed by 1% agarose gel electrophoresis. For the soil bacteria, the V3-V4 region of 16S rDNA was amplified by polymerase chain reaction (PCR) using primers 338F (5′-ACTCCTACGGGAGGCAGCAG-3′; Xu et al., 2016) and 806R (5′-GGACTACHVGGGTWTCTAAT-3′; Adams et al., 2013). For the soil fungi, the ITS1-ITS2 region of the rRNA gene was amplified by PCR using primers ITS1F (5′-CTTGGTCATTTAGAGGAAGTAA-3′) and ITS2R (5′-GCTGCGTTCTTCATCGATGC-3′). The following were the steps of PCR: Predenaturation at 95°C for 3 min, 27 cycles of denaturation at 95°C for 30 s, annealing at 55°C for 30 s, extension at 72°C for 45 s, and a final extension at 72°C for 10 min (PCR instrument: ABI GeneAmp® 9700). A 20 μl reaction system consisted of 4 μl *5FastPfu buffer, 2 μl 2.5 mM dNTPs, 0.8 μl primer (5 μM), 0.4 μl FastPfu polymerase, and 10 ng DNA template. The PCR products were resolved by 2% agarose gel electrophoresis and purified using the AxyPrep DNA Gel Extraction Kit (Axygen Biosciences, Union City, CA, United States). The proteins were eluted with Tris–HCl and assessed for their quality by 2% agarose gel electrophoresis. Quantification was done using QuantiFluor™ -ST (Promega, United States). The PE 2*300 library was constructed using the purified amplification products on the Illumina MiSeq platform (Illumina, San Diego, United States) following the standard operating procedure. Sequencing was done on the Illumina MiSeq (PE300) sequencing platform (Majorbio, Shanghai).
After performing quality control for the original sequencing data using Trimmomatic, the reads were merged using the FLASH software. However, after the quality control and merging of the reads, PCR and sequencing errors might still exist in the optimized sequences; hence, the optimized data were further processed using the sequencing denoising algorithm DADA2 or Deblur to eliminate these errors as much as possible and obtain the amplicon sequence variants (ASVs). The sequence and abundance information, as represented by ASVs, were used for the subsequent taxonomic analysis of the species, microbial community diversity analysis, and species composition analysis. The original 16S rRNA sequence data of bacteria and fungi are already stored in the NCBI database (accession numbers PRJNA725677 and PRJNA725679, respectively).
The molecular ecological network analysis (MENA) was constructed using the Network Analyses Pipeline.1 The ASVs were subjected to the 1og10 transformation. The correlation matrix was computed and converted into a similarity matrix based on Spearman’s correlation coefficient (Deng et al., 2016). According to the random matrix theory, the optimal similarity threshold was selected from the reciprocal adjacency matrix of the similarity matrix. The intensity of connection between each pair of nodes was coded using the adjacency matrix. The ecological communities were predicted by analyzing the nearest neighbor spacing distribution of eigenvalues (Zhou et al., 2010; Deng et al., 2012). The network typology properties were described by calculating the average clustering coefficient (avgCC), average path distance (APD), graph density (GD), and modularity (M). The role of each node in the typology was determined based on two attributes, intramodular connectivity (Zi) and intermodular connectivity (Pi; Guimera and Amaral, 2005). Using the thresholds for different levels of Zi and Pi as recommended by MENA (Zhou et al., 2010; Derocles et al., 2018), all species were divided into four topological roles: peripherals (Zi < 2.5, Pi < 0.62), module hubs (Zi > 2.5, Pi < 0.62), connectors (Zi < 2.5, Pi > 0.62), and network hubs (Zi > 2.5, Pi > 0.62). The network was visually analyzed using the Gephi software.
The influence of the vegetation restoration model on soil microbial diversity was assessed by one-way analysis of variance (ANOVA) and the least significant difference (LSD) test. The microbial alpha-diversity analysis, PCoA, and analysis of species differences were conducted on the Majorbio Cloud platform.2 Based on the Bray-Curtis distance, PCoA was employed to assess the overall structural differences of the soil microbial community. The difference in relative abundance on the order level was assessed using the Kruskal–Wallis H test. The null model was used to analyze the ecological process of the microbial communities and the microbial community assembly on the Galaxy/DengLab platform3 (Stegen et al., 2013). Each ASV on the dataset was analyzed through Reconstruction of Unobserved States (PICRUSt2) to predict the metabolic status of the bacterial community.4 Based on KO (KEGG orthologs) levels 1 and 2, the relative abundance of functional traits and functional genes were analyzed to determine the differences among the treatment. We first calculated the parameters, such as the β-nearest taxon index (βNTI) and the Raup-Crick matrix (RCbray). When |βNTI| > 2, the community assembly was a deterministic process, and when |βNTI| < 2, the community assembly was a primarily stochastic process. Meanwhile, RCbray > 0.95, RCbray < −0.95, and |RCbray| < 0.95 corresponded to dispersal limitation, homogenizing dispersal, and drift, respectively (Zhou and Ning, 2017).
As shown in Table 1, the α-diversity indices of bacterial communities under different vegetation restoration models varied significantly (p < 0.05). Compared with CK, the Sob index, Shannon index, and phylogenetic diversity index (PD) increased by 35.29%, 3.50%, and 25.29%, respectively, for the bacterial community in MF. However, the vegetation type had no significant influence on the Sobs and Shannon indices of the fungal community. Compared with CK, PD of the fungal community in BF decreased by 29.5%.
Table 1. Alpha (α)-diversity indices of bacterial and fungal communities in soil under different vegetation restoration models.
As shown in Figure 2, the vegetation restoration model had a significant influence on the microbial community structure. Axes 1 and 2 explained 22.2% and 12.9%, respectively, of the β-diversity variation in the bacterial community on the operational taxonomic units (out) level. The sample points of CK were segregated from those of BF and MF along axis 1; the sample points of BF and MF were closer to each other than to others (Figure 2A). The first two principal coordinates explained 20.9% of the β-diversity variation in the fungal community on the OTU level. Sample points of CF were significantly segregated from those of BF and MF along axis 1; the sample points of CK were segregated from those of BL along axis 2 (Figure 2B). These results explained the dissimilarity and similarity in microbial community diversity across the sample points under different treatments.
Figure 2. PCoA of bacterial (A) and fungal (B) β-diversity under different vegetation restoration models.
Among the soil bacteria, phyla Actinobacteria accounted for 33%–40%, Proteobacteria accounted for 21%–24%, Acidobacteria accounted for 16%–21%, and Chloroflexi accounted for 10%–13% of the total relative dominance (Figure 3A). Among the soil fungi, phyla Ascomycota accounted for 28%–72%, and Basidiomycota accounted for 3%–29% of the dominance (Figure 3B). Compared with CK, the relative abundance of Ascomycota increased significantly under any vegetation restoration model (p < 0.05), while the relative abundance of Basidiomycota was the lowest in BF. Among different treatments, Rhizobiales accounted for 9%–13%, Propionibacteriales accounted for 4%–7%, and Micrococcales accounted for 2%–6% of all bacteria. There was a significant difference in the relative abundance of these bacteria under different vegetation restoration models (Figure 4A). Among the fungi, Chaetothyriales accounted for 7%–31%, Hypocreales accounted for 9%–26%, and Mortierellales accounted for 7%–17% of the relative abundance. There was a significant difference in the relative abundance of these fungi under different vegetation restoration models (Figure 4B).
Figure 4. Relative abundances of top 15 bacterial (A) and fungal (B) order that showed significant differences among rehabilitation modes. Kruskal–Wallis H test was used to evaluate the significance of differences between the indicated groups. *p < 0.05, **p < 0.01, ***p < 0.001.
Clusters of Orthologous Groups (COG) analysis was conducted using PICRUSt2. The soil bacterial communities under different vegetation restoration models shared similar functional features (Figure 5A). Twenty-two bacterial functions and metabolic pathways were identified, including those for energy production and conversion, amino acid transport and metabolism, nucleotide transport and metabolism, carbohydrate transport and metabolism, and coenzyme transport and metabolism. However, the FUNGuild-based functional prediction indicated significant differences in the functions of soil fungal communities under different vegetation restoration models (Figure 5B). Syntrophic mycorrhizae were mainly found in CK, CF, and MF samples; pathographic plant pathogens were mainly found in BF samples. Though the abundance distribution was uneven, undefined saprophytic fungi were widespread across the treatments.
Figure 5. Variations in the composition of bacterial (A) and fungal (B) functional groups inferred by the PICRUSt2 and FUN Guild, respectively.
We further constructed the soil bacterial and fungal co-occurrence network under different vegetation restoration models (Figure 6). In all bacterial networks, the identifiable dominant ASVs were primarily assigned to Actinobacteria, Proteobacteria, and Acidobacteria (Figure 6A). Compared with CK, the soil bacterial networks under different vegetation restoration models were more complex and had more nodes and edges. The average path distance and the modularity were smaller, while the average connectivity and clustering coefficient was higher. Besides, most nodes in the soil bacterial networks under different vegetation restoration models were peripherals, indicating closer connections between bacterial species. In all fungal networks, the identifiable dominant ASV was assigned to Ascomycota (Figure 6B). Compared with CK, the fungal network of MF had more nodes and edges. In fungal communities, the interspecies relationship tended to be co-excluded (negative relationship, red line).
Figure 6. Co-occurrence network of bacteria (A) and fungi (B) under differences among rehabilitation types. Each node represents individual amplicon sequence variants (ASVs). The size of each node is proportional to the number of connections (that is, degrees). The nodes are colored at the phylum level, and an edge represents a Spearman correlation with a correlation coefficient of >0.6, a green edge represents a positive interaction, and a red edge represents a negative interaction.
Vegetation restoration had a significantly differential impact on module hubs in the bacterial networks. Chloroflexi only appeared in the bacterial networks of BL and CF, Actinobacteria and Proteobacteria appeared in the bacterial networks of CK, BL, and CF; Methylomirabilota and Armatimonadota appeared in the bacterial networks of CK and CF; Proteobacteria was absent only in the bacterial network from BF; and Acidobacteria was the module hub in all networks. Besides, Burkholderiales, Sphingomonadales, and Rhizobiales, orders Proteobacteria, Vicinamibacterales, and Acidobacteria, were also module hubs. The bacterial networks from BL, CF, and MF had connected nodes, but those from CK and BF did not (Figure 7A). Compared with CK, the fungal network of MF had more nodes and edges. ASV204 assigned to Ascomycota was the module hub of the fungal network from BF. ASV83 assigned to Mortierellomycota was the module hub of the fungal network from MF. The fungal networks from CF, BF, and MF had connected nodes (Figure 7B), all of which were assigned to the Ascomycota. However, these nodes could be assigned to different orders, including Thelebolales, Chaetothyriales, and Hypocreales. There were no connected nodes in the fungal network of CK and BL (Figure 7B; Table 2).
Figure 7. Z-P plot showing the distribution of amplicon sequence variants (ASVs) based on their topological roles. Each color represents a kind of vegetation type. The topological role of each ASV was determined according to the scatter plot of within module connectivity (Zi) and among-module connectivity (Pi). (A) Z-P plot of bacterial community MENs; (B) Z-P plot of fungal community molecular ecological networks (MENs).
Table 2. Soil bacterial and fungal community network parameters in different vegetation restoration types.
The calculated values of βNTI and RCBray were further compared. For soil bacteria, |βNTI| < 2 (Figure 8A) indicated that the phylogenetic differences were controlled by the stochastic process. The contribution rate to bacterial community assembly was 100.0% for CK, CF, BF, and MF, respectively. For all bacterial communities, |RCbray| < 0.95 indicated that the bacterial community assembly was not governed, but weak selection, weak dispersal, speciation, and drift acted jointly (Figure 8C). For soil fungal communities from CK, BL, CF, and BF, |βNTI| < 2 indicated that the fungal community assembly was governed by the stochastic process (Figure 8B). Meanwhile, |RCbray| < 0.95 for fungal communities indicated that the community was assembled under the joint action of weak selection, weak dispersal, speciation, and drift, while the community assembly was not governed (Figure 8D). However, βNTI > 2 for the fungal community from MF indicated that the deterministic process governed the soil fungal community assembly in MF. Meanwhile, the contribution rate of the stochastic process was 42.86% (Figure 8B). As shown in Figure 8, irrespective of the vegetation restoration model, the governing effect exerted by the stochastic process over the microbial community assembly far surpassed that over the fungal community.
Figure 8. (A,C) were the β-nearest taxon index (βNTI) and Bray-Curtis-based Raup-Crick (RCbray) box plots of bacterial communities under different vegetation reconstruction models. (B,D) present βNTI and RCbray box plots of fungal communities under different vegetation reconstruction models. The red numbers indicate the contribution rate of random processes to the construction of microbial communities.
Microbes are indispensable in regulating ecological processes and functions. Vegetation restoration in the mining area can relieve soil environmental stress and promote the development of microbial flora (Delgado-Baquerizo et al., 2018). We found that the abundance and diversity of microbial communities from CF and MF were significantly higher than those from CK, indicating that CF and MF were more conducive to microbial community diversity, consistent with the previous studies (Yang et al., 2020). Under different vegetation restoration models, the dominant soil bacterial phyla were consistently Actinobacteria, Proteobacteria, Acidobacteria, and Chloroflexi, congruent with those reported in other mining areas of the Loess Plateau (Guo et al., 2020; Jia et al., 2020; Shi et al., 2020). However, the dominant bacterial flora did not vary significantly on the phylum level across the vegetation restoration models, but the difference was significant at the level of order (Figure 4). One dominant phylum in the study area was oligotrophic Acidobacteria, indicating soil depletion in the mining area of the Loess Plateau (Thomson et al., 2015). Acidobacteria exist in various environments, accounting for about 20% of the total bacteria, and some even reach more than 50%. The bacteria of Acidobacteria and Proteobacteria in the soil are equivalent in number, and they play an important role in the process of soil material circulation and ecological environment construction (Lee et al., 2008). Naether et al. (2012) showed that there were significant differences in the community structure of Acidobacteria between forest and grassland soil. Soil pH, organic carbon, total nitrogen, C/N ratio, phosphorus, nitrate nitrogen, ammonium nitrogen, soil moisture, soil temperature, and soil respiration, were all confirmed to have effects on the composition of the Acidobacteria community structure. Firere et al. demonstrated that the relative abundance of Acidobacteria in forest soil was significantly positively correlated with mean precipitation and soil organic carbon, while negatively correlated with soil pH value. Different vegetation types could lead to different soil physicochemical environment. Another dominant phylum was eutrophic Proteobacteria, indicating the abundance of carbon-containing organic pollutants in the soil of the mining area, which supply unstable carbon substrates for such Proteobacteria (Kurm et al., 2017). PICRUSt2-based functional prediction showed that the vegetation restoration type had no significant impact on the functions of the microbial community, which agreed with those reported in other mining areas of the Loess Plateau (Jia et al., 2020; Zhao et al., 2021).
The dominant soil fungal phyla in the study area were Ascomycota and Basidiomycota. The relative abundance of Ascomycota was higher, and most species of this phylum were saprophytic bacteria, insensitive to environmental stress (Ventorino et al., 2016). The relative abundance of Basidiomycota was significantly higher in MF, while that in BF was lower. Basidiomycota are important decomposers. As widespread ectomycorrhizal fungi (Zhong et al., 2020), they can promote soil nutrient recycling in the mining area. Given the above, the soil in BF can be suitably inoculated with Basidiomycota species. Besides, the FUNGuild-based functional prediction showed that the fungal functions varied significantly across the vegetation restoration models. Vegetation restoration had a much greater impact on the abundance of functional fungal clusters than on functional bacterial clusters. Similar reports have been published previously (Peay et al., 2013; Bahram et al., 2020). The reason is mostly that plant pathogenic fungi and ectomycorrhizal fungi live in symbiosis with the root systems and thus have closer connections to vegetation than bacteria (Guo et al., 2020).
Compared with CK, the bacterial networks in BL, CF, and MF had more nodes and edges and hence a higher complexity. The bacterial network in BF had a significantly higher average clustering coefficient and modularity but a lower average path distance. This indicated that the bacterial network in MF was a self-contained “small-world” module. The bacterial clusters within the module were closely interconnected and shared many similarities (Ling et al., 2016). Generally speaking, bacterial clusters serving as module hubs and connectors in the topology structure were key clusters (Derocles et al., 2018), which played a crucial role in system stability. Module hubs in soil bacterial networks under different vegetation restoration models were all Proteobacteria and Acidobacteria, which were closely related to the adaptation of eutrophic Proteobacteria to the complex carbon environment of the mining area (Kurm et al., 2017). Acidobacteria are particularly adapted to the mining area (Banerjee et al., 2016). We found that Burkholderiales, Sphingomonadales, Rhizobiales, and Vicinamibacterales were module hubs of the bacterial networks. Ascomycetes are saprophytic fungi, and decomposing plant residues and degrading organic matter are their main functions. The main function of Basidiomycetes is to decompose lignified vegetation debris. The rich contents of CK and BF organic matter may affect the content of Ascomycetes. The high lignin content of shrubs and trees, coupled with moist soil, could provide proper living conditions for the growth of Basidiomycetes. The results of this study showed that saprophytic fungi accounted for the largest proportion of each fungal function, while MF and BF could improve the enrichment of soil saprophytic fungi. Previous studies have shown that symbiotic trophic fungi may play an important role in soil health and nutrient cycling (Jing et al., 2021). It is worth noting that the symbiotic trophic fungi of CF and MF are the most abundant, and they have been reported to have the ability of reducing the risk of soil pests and diseases and improving soil quality. Attempts can be made in the future to isolate and purify the key bacterial species along with their amplification and inoculation to facilitate soil-vegetation restoration of the mining areas.
Ascomycota were observed to be module hubs in all soil fungal networks under different vegetation restoration models, playing key roles in the fungal network. Co-excluded negative relationships were predominant among clusters in the fungal networks, accounting for 61%–81% of potential interactions. The negative relationship was attributed to competition and homogenization (Faust and Raes, 2012), which enhanced the stability of the ecological network under the mining disturbance (de Vries et al., 2018). Compared with CK, vegetation restoration reduced the average clustering coefficients of the fungal networks. The external disturbance would not influence the entire fungal community within a short period, indicating increased resistance. Mortierellales, Thelebolales, Chaetothyriales, and Hypocreales were key clusters in the fungal networks. Species of these orders were isolated and purified and would be amplified and inoculated to the root systems of plants used for restoration. In the future, we will step up the exploration into relevant functional microbes to enhance the understanding of the mechanism of ecological restoration in the mining area.
Deterministic and stochastic processes jointly regulated microbial community assembly (Zhou and Ning, 2017; Mori et al., 2018), though the relative importance of each varied across the environments (Tian et al., 2017). In the current study, |βNTI| < 2 for all soil bacterial communities indicated that the stochastic process governed bacterial community assembly. Moreover, |RCbray| < 0.95 for the soil bacterial communities indicated that the assembly process was not governed (Zhou and Ning, 2017). Vegetation restoration in the mining area only constituted a local disturbance, which was quite different from the influence of large-scale spatial processes such as temperature and precipitation. Our result also demonstrates that the stochastic process usually occurs in small-scale spaces with environmental changes (Shi et al., 2018). Although the stochastic process is hard to predict (O’Malley, 2008), the stochastic factors influencing microbial community assembly are more or less predictable. Therefore, we can predict the probable process of microbial community assembly by exploring when stochastic factors take effect and when they do not (Fukami, 2015). Except for MF, |βNTI| < 2 for the fungal communities in CK, BL, BF, and CF indicated the governing role played by the stochastic process in fungal community assembly. Meanwhile, |RCbray| < 0.95 for all fungal communities indicated that the fungal community assembly was jointly governed by weak selection, weak dispersal, speciation, and drift. In addition to the lesser impact of selection, mutual cancellation or contrasting options might also exist, leading to a stochastic development model of soil microbial flora in the mining area (Zhou and Ning, 2017). For the fungal community in MF, βNTI>2 indicated that the deterministic process governed fungal community assembly in MF, which might be further attributed to heterogeneous selection (Zhou and Ning, 2017). The microbial community assembly process can become more predictable if we explore the temporal and conditional factors under which the microbial communities are more sensitive to the stochastic process (Fukami, 2015). On this basis, we can appropriately manipulate microbial community assembly to achieve the desired ecosystem functions, thereby assisting with ecological restoration and biodiversity protection and management in mining areas (Chase, 2010).
Ecosystem monitoring, as the key construction project, is always an important pathway to deal with environmental changes, especially in mining areas. This study has shown that different vegetation restoration types have significantly changed the soil microbial molecular ecological networks in the mining area. In relatively healthy mining areas, the microbial molecular ecological network was more complex and closely connected, and the interaction relationship is also more complicated. However, the links between soil microbial molecular ecological networks in the damaged mining areas tended to be single, while the connections between various nodes were less, as well as the network was simplified. Then this obvious regularity could be used to quantitatively and visually express the microscopic changes of the environment in the mining area, as well as monitor and evaluate the ecological succession law of the damaged mining area. Through comparing the changes of soil microbial molecular ecological network interaction between healthy mining areas and damaged mining areas, land reclamation and ecological restoration strategies could be reasonably formulated, while soil reconstruction work could be guided to a reasonable evolution direction. At the same time, the introduction of soil microbial molecular ecological network monitoring system will provide new ideas for improving the evaluation of mining ecosystem.
Studies have shown that different vegetation restoration types have significantly different effects on soil microbial community diversity. Compared with CK, BF and MF significantly changed the soil bacterial community structure, but did not change the bacterial community composition. BL, CF, and MF significantly altered the fungal community structure and increased the relative abundance of Ascomycota and decreased the relative abundance of Basidiomycota. Ascomycota and Basidiomycota are the dominant phyla in the fungal community. Different vegetation restoration types shaped different fungal functional groups, but there was no significant difference in bacterial functions. Vegetation restoration increased soil microbial community network and complexity. Compared with CK, the BL, CF, and MF bacterial networks were larger and more complex, and the MF fungal network was more complex and stable. Acidobacteria and Proteobacteria were the hubs and key taxa of the bacterial network module, while Ascomycota and Mortierella played key roles in BF and MF fungal community networks, respectively. The soil bacterial assembly process was dominated by stochastic processes and was hardly affected by the type of vegetation restoration. However, the MF fungal community assembly is dominated by deterministic processes, and the stochastic process controls the bacterial community assembly process much more than the fungal community. Clarifying the time and conditions when microbial communities are more sensitive to random processes will help to manipulate community assembly and achieve required ecological functions, thereby scientifically guiding ecological restoration, biodiversity conservation, and management in mining areas.
The datasets presented in this study can be found in online repositories. The names of the repository/repositories and accession number(s) can be found in the article/supplementary material.
YuC, YZ, and JM collected the samples. YaC, YZ, and YY performed the experiments. YuC performed the data analyses and wrote the manuscript. FC, JM, and YZ helped perform the analysis with constructive discussions. FC performed the supervision, project administration, and funding acquisition. All authors contributed to the article and approved the submitted version.
This work was supported by the National Natural Science Foundation of China (no. 51974313) and the key project of Jiangsu Key Laboratory of Coal-based Greenhouse Gas Control and Utilization (2020ZDZZ03). We gratefully acknowledge these programs for financial support.
We would like to thank LetPub (www.letpub.com) for its linguistic assistance during the preparation of this manuscript.
The authors declare that the research was conducted in the absence of any commercial or financial relationships that could be construed as a potential conflict of interest.
All claims expressed in this article are solely those of the authors and do not necessarily represent those of their affiliated organizations, or those of the publisher, the editors and the reviewers. Any product that may be evaluated in this article, or claim that may be made by its manufacturer, is not guaranteed or endorsed by the publisher.
Adams, R. I., Miletto, M., Taylor, J. W., and Bruns, T. D. (2013). Dispersal in microbes: fungi in indoor air are dominated by outdoor air and show dispersal limitation at short distances. ISME J. 7, 1262–1273. doi: 10.1038/ismej.2013.28
Bahram, M., Netherway, T., Hildebrand, F., Pritsch, K., Drenkhan, R., Loit, K., et al. (2020). Plant nutrient-acquisition strategies drive topsoil microbiome structure and function. New Phytol. 227, 1189–1199. doi: 10.1111/nph.16598
Baldrian, P. (2017). Forest microbiome: diversity, complexity and dynamics. FEMS Microbiol. Rev. 41, 109–130. doi: 10.1093/femsre/fuw040
Banerjee, S., Kirkby, C. A., Schmutter, D., Bissett, A., Kirkegaard, J. A., and Richardson, A. E. (2016). Network analysis reveals functional redundancy and keystone taxa amongst bacterial and fungal communities during organic matter decomposition in an arable soil. Soil Biol. Biochem. 97, 188–198. doi: 10.1016/j.soilbio.2016.03.017
Banerjee, S., Walder, F., Buchi, L., Meyer, M., Held, A. Y., Gattinger, A., et al. (2019). Agricultural intensification reduces microbial network complexity and the abundance of keystone taxa in roots. ISME J. 13, 1722–1736. doi: 10.1038/s41396-019-0383-2
Barberan, A., Bates, S. T., Casamayor, E. O., and Fierer, N. (2012). Using network analysis to explore co-occurrence patterns in soil microbial communities. ISME J. 6, 343–351. doi: 10.1038/ismej.2011.119
Bulgarelli, D., Rott, M., Schlaeppi, K., Ver Loren Van Themaat, E., Ahmadinejad, N., Assenza, F., et al. (2012). Revealing structure and assembly cues for Arabidopsis root-inhabiting bacterial microbiota. Nature 488, 91–95. doi: 10.1038/nature11336
Chase, J. M. (2010). Stochastic community assembly causes higher biodiversity in more productive environments. Science 328, 1388–1391. doi: 10.1126/science.1187820
Crouzeilles, R., Curran, M., Ferreira, M. S., Lindenmayer, D. B., Grelle, C. E., and Rey Benayas, J. M. (2016). A global meta-analysis on the ecological drivers of forest restoration success. Nat. Commun. 7, 11666. doi: 10.1038/ncomms11666
de Vries, F. T., Griffiths, R. I., Bailey, M., Craig, H., Girlanda, M., Gweon, H. S., et al. (2018). Soil bacterial networks are less stable under drought than fungal networks. Nat. Commun. 9, 3033. doi: 10.1038/s41467-018-05516-7
Delgado-Baquerizo, M., Oliverio, A. M., Brewer, T. E., Benavent-Gonzalez, A., Eldridge, D. J., Bardgett, R. D., et al. (2018). A global atlas of the dominant bacteria found in soil. Science 359, 320–325. doi: 10.1126/science.aap9516
Deng, Y., Jiang, Y. H., Yang, Y. F., He, Z. L., Luo, F., and Zhou, J. Z. (2012). Molecular ecological network analyses. BMC Bioinf. 13:113. doi: 10.1186/1471-2105-13-113
Deng, Y., Zhang, P., Qin, Y. J., Tu, Q. C., Yang, Y. F., He, Z. L., et al. (2016). Network succession reveals the importance of competition in response to emulsified vegetable oil amendment for uranium bioremediation. Environ. Microbiol. 18, 205–218. doi: 10.1111/1462-2920.12981
Derocles, S.A.P., Bohan, D.A., Dumbrell, A.J., Kitson, J.J.N., Massol, F., Pauvert, C., et al. (2018). Biomonitoring for the 21st century: integrating next-generation sequencing Into ecological network analysis. Adv. Ecol. Res. 58, 1–62. doi: 10.1016/bs.aecr.2017.12.001
Eviner, V. T. (2004). Plant traits that influence ecosystem processes vary independently among species. Ecology 85, 2215–2229. doi: 10.1890/03-0405
Faust, K., and Raes, J. (2012). Microbial interactions: from networks to models. Nat. Rev. Microbiol. 10, 538–550. doi: 10.1038/nrmicro2832
Festin, E. S., Tigabu, M., Chileshe, M. N., Syampungani, S., and Oden, P. C. (2019). Progresses in restoration of post-mining landscape in Africa. J. For. Res. 30, 381–396. doi: 10.1007/s11676-018-0621-x
Fukami, T. (2015). Historical contingency in community assembly integrating niches species pools and priority effects. Annu. Rev. Ecol. Evol. S 46, 1–23. doi: 10.1146/annurev-ecolsys-110411-160340
Gastauer, M., Souza Filho, P. W. M., Ramos, S. J., Caldeira, C. F., Silva, J. R., Siqueira, J. O., et al. (2019). Mine land rehabilitation in Brazil: goals and techniques in the context of legal requirements. Ambio 48, 74–88. doi: 10.1007/s13280-018-1053-8
Graham, E. B., Crump, A. R., Resch, C. T., Fansler, S., Arntzen, E., Kennedy, D. W., et al. (2017). Deterministic influences exceed dispersal effects on hydrologically-connected microbiomes. Environ. Microbiol. 19, 1552–1567. doi: 10.1111/1462-2920.13720
Guimera, R., and Amaral, L. A. N. (2005). Functional cartography of complex metabolic networks. Nature 433, 895–900. doi: 10.1038/nature03288
Guo, Y. N., Liu, X. H., Tsolmon, B., Chen, J., Wei, W., Lei, S. G., et al. (2020). The influence of transplanted trees on soil microbial diversity in coal mine subsidence areas in the Loess Plateau of China. Global Ecol. Conserv. 21:e00877. doi: 10.1016/j.gecco.2019.e00877
Jia, T., Guo, T., Yao, Y., Wang, R., and Chai, B. (2020). Seasonal microbial community characteristic and its driving factors in a copper tailings dam in the Chinese Loess Plateau. Front. Microbiol. 11, 1574. doi: 10.3389/fmicb.2020.01574
Jing, M., Gonzalez-Ollauri, A., Qi, Z., Dong, X., and Fu, C. (2021). Ecological network analysis to assess the restoration success of disturbed mine soil in Zoucheng, China. Land Degrad. Dev. 32, 5393–5411. doi: 10.1002/ldr.4116
Kurm, V., Van Der Putten, W. H., De Boer, W., Naus-Wiezer, S., and Hol, W. H. (2017). Low abundant soil bacteria can be metabolically versatile and fast growing. Ecology 98, 555–564. doi: 10.1002/ecy.1670
Lee, S., Ka, J., and Cho, J. (2008). Members of the phylumAcidobacteria are dominant and metabolicallyactive in rhizosphere soil. FEMS Microbiol. Lett. 285, 263–269. doi: 10.1111/j.1574-6968.2008.01232.x
Li, Y., Wen, H., Chen, L., and Yin, T. (2014). Succession of bacterial community structure and diversity in soil along a chronosequence of reclamation and re-vegetation on coal mine spoils in China. PLoS One 9:e115024. doi: 10.1371/journal.pone.0115024
Ling, N., Zhu, C., Xue, C., Chen, H., Duan, Y. H., Peng, C., et al. (2016). Insight into how organic amendments can shape the soil microbiome in long-term field experiments as revealed by network analysis. Soil Biol. Biochem. 99, 137–149. doi: 10.1016/j.soilbio.2016.05.005
Liu, Y., Chen, X. T., Liu, J. X., Liu, T. T., Cheng, J. M., Wei, G. H., et al. (2019). Temporal and spatial succession and dynamics of soil fungal communities in restored grassland on the Loess Plateau in China. Land Degrad. Dev. 30, 1273–1287. doi: 10.1002/ldr.3289
Mori, A. S., Isbell, F., and Seidl, R. (2018). Beta-diversity, community assembly, and ecosystem functioning. Trends Ecol. Evol. 33, 549–564. doi: 10.1016/j.tree.2018.04.012
Naether, A., Foesel, B. U., and Naegele, V. (2012). Environmental factors affect acidobacterial communities below the subgroup level in grassland and forest soils. Apply Environ. Microbiol. 78, 7398–7406. doi: 10.1128/AEM.01325-12
Nuccio, E. E., Anderson-Furgeson, J., Estera, K. Y., Pett-Ridge, J., De Valpine, P., Brodie, E. L., et al. (2016). Climate and edaphic controllers influence rhizosphere community assembly for a wild annual grass. Ecology 97, 1307–1318. doi: 10.1890/15-0882.1
O’malley, M. A. (2008). ‘Everything is everywhere: but the environment selects’: ubiquitous distribution and ecological determinism in microbial biogeography. Stud. Hist. Phil. Biol. Biomed. Sci. 39, 314–325. doi: 10.1016/j.shpsc.2008.06.005
Peay, K. G., Baraloto, C., and Fine, P. V. (2013). Strong coupling of plant and fungal community structure across western Amazonian rainforests. ISME J. 7, 1852–1861. doi: 10.1038/ismej.2013.66
Sharrar, A., Crits-Christoph, A., Raphal, M., Raphal, M., and Spencer, D. (2020). Bacterial secondary metabolite biosynthetic potential in soil varies with phylum, depth, and vegetation type. mBio 11, 416. doi: 10.1128/mBio.00416-20
Shi, Y., Delgado-Baquerizo, M., Li, Y., Yang, Y., Zhu, Y. G., Penuelas, J., et al. (2020). Abundance of kinless hubs within soil microbial networks are associated with high functional potential in agricultural ecosystems. Environ. Int. 142:105869. doi: 10.1016/j.envint.2020.105869
Shi, Y., Li, Y., Xiang, X., Sun, R., Yang, T., He, D., et al. (2018). Spatial scale affects the relative role of stochasticity versus determinism in soil bacterial communities in wheat fields across the North China plain. Microbiome 6, 27. doi: 10.1186/s40168-018-0409-4
Shi, S., Nuccio, E. E., Shi, Z. J., He, Z., Zhou, J., and Firestone, M. K. (2016). The interconnected rhizosphere: high network complexity dominates rhizosphere assemblages. Ecol. Lett. 19, 926–936. doi: 10.1111/ele.12630
Stegen, J. C., Lin, X., Fredrickson, J. K., Chen, X., Kennedy, D. W., Murray, C. J., et al. (2013). Quantifying community assembly processes and identifying features that impose them. ISME J. 7, 2069–2079. doi: 10.1038/ismej.2013.93
Thomson, B. C., Tisserant, E., Plassart, P., Uroz, S., Griffiths, R. I., Hannula, S. E., et al. (2015). Soil conditions and land use intensification effects on soil microbial communities across a range of European field sites. Soil Biol. Biochem. 88, 403–413. doi: 10.1016/j.soilbio.2015.06.012
Tian, J., Qiao, Y., Wu, B., Chen, H., Li, W., Jiang, N., et al. (2017). Ecological succession pattern of fungal Community in Soil along a retreating glacier. Front. Microbiol. 8, 1028. doi: 10.3389/fmicb.2017.01028
Ventorino, V., Parillo, R., Testa, A., Viscardi, S., Espresso, F., and Pepe, O. (2016). Chestnut green waste composting for sustainable forest management: microbiota dynamics and impact on plant disease control. J. Environ. Manag. 166, 168–177. doi: 10.1016/j.jenvman.2015.10.018
Wang, J., Wang, H., Cao, Y., Bai, Z., and Qin, Q. (2016). Effects of soil and topographic factors on vegetation restoration in opencast coal mine dumps located in a loess area. Sci. Rep. 6, 22058. doi: 10.1038/srep22058
Xu, M., Li, W., Wang, J., Zhu, Y., Feng, Y., Yang, G., et al. (2022). Soil ecoenzymatic stoichiometry reveals microbial phosphorus limitation after vegetation restoration on the Loess Plateau, China. Sci. Total Environ. 815:152918. doi: 10.1016/j.scitotenv.2022.152918
Xu, N., Tan, G. C., Wang, H. Y., and Gai, X. P. (2016). Effect of biochar additions to soil on nitrogen leaching, microbial biomass and bacterial community structure. Eur. J. Soil Biol. 74, 1–8. doi: 10.1016/j.ejsobi.2016.02.004
Yang, Y., Cheng, H., Liu, L. X., Dou, Y. X., and An, S. S. (2020). Comparison of soil microbial community between planted woodland and natural grass vegetation on the Loess Plateau. For. Ecol. Manag. 460, 117817. doi: 10.1016/j.foreco.2019.117817
Yang, Y., Dou, Y., and An, S. (2018). Testing association between soil bacterial diversity and soil carbon storage on the Loess Plateau. Sci. Total Environ. 626, 48–58. doi: 10.1016/j.scitotenv.2018.01.081
Zhang, C., Liu, G. B., Xue, S., and Wang, G. L. (2016). Soil bacterial community dynamics reflect changes in plant community and soil properties during the secondary succession of abandoned farmland in the Loess Plateau. Soil Biol. Biochem. 97, 40–49. doi: 10.1016/j.soilbio.2016.02.013
Zhang, C., Xue, S., Liu, G. B., and Song, Z. L. (2011). A comparison of soil qualities of different revegetation types in the Loess Plateau, China. Plant Soil 347, 163–178. doi: 10.1007/s11104-011-0836-5
Zhao, J., Ma, J., Yang, Y., Yu, H., Zhang, S., and Chen, F. (2021). Response of soil microbial community to vegetation reconstruction modes in mining areas of the Loess Plateau, China. Front. Microbiol. 12:714967. doi: 10.3389/fmicb.2021.714967
Zhong, Z. K., Li, W. J., Lu, X. Q., Gu, Y. Q., Wu, S. J., Shen, Z. Y., et al. (2020). Adaptive pathways of soil microorganisms to stoichiometric imbalances regulate microbial respiration following afforestation in the Loess Plateau, China. Soil Biol. Biochem. 151, 108048. doi: 10.1016/j.soilbio.2020.108048
Zhou, J., Deng, Y., Luo, F., He, Z., Tu, Q., and Zhi, X. (2010). Functional molecular ecological networks. MBio 1:169. doi: 10.1128/mBio.00169-10
Keywords: vegetation restoration, microbial community, ecological network, assembly process, key microbial taxa
Citation: Chang Y, Chen F, Zhu Y, You Y, Cheng Y and Ma J (2022) Influence of revegetation on soil microbial community and its assembly process in the open-pit mining area of the Loess Plateau, China. Front. Microbiol. 13:992816. doi: 10.3389/fmicb.2022.992816
Received: 13 July 2022; Accepted: 01 August 2022;
Published: 25 August 2022.
Edited by:
Yanfei Zhang, Tianjin Institute of Industrial Biotechnology (CAS), ChinaReviewed by:
Jinman Wang, China University of Geosciences, ChinaCopyright © 2022 Chang, Chen, Zhu, You, Cheng and Ma. This is an open-access article distributed under the terms of the Creative Commons Attribution License (CC BY). The use, distribution or reproduction in other forums is permitted, provided the original author(s) and the copyright owner(s) are credited and that the original publication in this journal is cited, in accordance with accepted academic practice. No use, distribution or reproduction is permitted which does not comply with these terms.
*Correspondence: Fu Chen, Y2hlbmZ1QGN1bXQuZWR1LmNu
Disclaimer: All claims expressed in this article are solely those of the authors and do not necessarily represent those of their affiliated organizations, or those of the publisher, the editors and the reviewers. Any product that may be evaluated in this article or claim that may be made by its manufacturer is not guaranteed or endorsed by the publisher.
Research integrity at Frontiers
Learn more about the work of our research integrity team to safeguard the quality of each article we publish.