- Department of Biology, York University, Toronto, ON, Canada
Bees and their microbes interact in complex networks in which bees form symbiotic relationships with their bacteria and fungi. Microbial composition and abundance affect bee health through nutrition, immunity, and fitness. In ever-expanding urban landscapes, land use development changes bee habitats and floral resource availability, thus altering the sources of microbes that wild bees need to establish their microbiome. Here, we implement metabarcoding of the bacterial 16S and fungal ITS regions to characterize the diversity and composition of the microbiome in 58 small carpenter bees, Ceratina calcarata, across urban land use gradients (study area 6,425 km2). By categorizing land use development, green space, precipitation, and temperature variables as indicators of habitat across the city, we found that land use variables can predict microbial diversity. Microbial composition was also found to vary across urban land use gradients, with certain microbes such as Acinetobacter and Apilactobacillus overrepresented in less urban locations and Penicillium more abundant in developed areas. Environmental features may also lead to differences in microbe interactions, as co-occurrences between bacteria and fungi varied across percent land use development, exemplified by the correlation between Methylobacterium and Sphingomonas being more prevalent in areas of higher urban development. Surrounding landscapes change the microbial landscape in wild bees and alter the relationships they have with their microbiome. As such, urban centres should consider the impact of growing cities on their pollinators’ health and protect wild bees from the effects of anthropogenic activities.
Introduction
Research on bee microbiomes uncovered their vital role in many aspects of bee health, including improving immunity (Engel et al., 2012; Mockler et al., 2018; Rubanov et al., 2019), nutrient utilization (Engel et al., 2012; Raymann and Moran, 2018), and reducing metalloid toxicity (Rothman et al., 2019). For example, the presence of non-pathogenic microbial symbionts in honey bees upregulates antimicrobial peptides in the bee and prepares the immune response against pathogenic microbes (Kwong et al., 2017a). The importance of microbes can also be implicated more broadly, with the sterilization of larval mason bee microbiomes negatively impacting bee fitness through declining growth rates, biomass, and survivorship (Dharampal et al., 2019). The gut microbiome has even been associated with different behavioural tasks in honey bees and memory retention in bumble bees (Jones et al., 2018; Li et al., 2021). With the common consensus that microbes engage in beneficial interactions with their bee hosts, research continues to examine the factors that influence the establishment and maintenance of the microbiome.
The core microbiome is described as the microbes that are consistently found within many individuals of a species (Turnbaugh and Gordon, 2009; Danko et al., 2021). Social and solitary bees acquire their microbiome in different ways, with honey and bumble bee microbiome composition directly influenced by social interactions with their colony members (Martinson et al., 2012; Tarpy et al., 2015; Kwong and Moran, 2016; Elijah Powell et al., 2018; Su et al., 2021), whereas less social wild bees inherit their microbiome from their surrounding environment and from their diet (McFrederick and Rehan, 2016; Dew et al., 2020; Voulgari-Kokota et al., 2020; Figueroa et al., 2021; Kapheim et al., 2021). Much work has been done on social bees such as Apis and Bombus to characterize and examine the health effects of an altered core microbiome (Martinson et al., 2011; Raymann and Moran, 2018; Rothman et al., 2019; Su et al., 2021). For solitary wild bees, this research is in its infancy; however, it is known that wild bees do not always maintain the same consistent core microbiome seen in social bees (McFrederick et al., 2012, 2014; Kwong and Moran, 2016; McFrederick and Rehan, 2016; Graystock et al., 2017). An example on how solitary wild bees can have differing core microbiomes can be found in C. calcarata, a wild bee displaying different core microbiomes from other bee species and across different regions (Graystock et al., 2017; Dew et al., 2020; Nguyen and Rehan, 2022; Shell and Rehan, 2022).
A range of variables, including developmental stage (McFrederick et al., 2014; Kapheim et al., 2021; Nguyen and Rehan, 2022), sociality of host species (Mohr and Tebbe, 2006; McFrederick et al., 2014; Graystock et al., 2017), climate (McFrederick and Rehan, 2019), geographical location (Almeida et al., 2022), and landscape features (Cohen et al., 2020), have been examined to determine their effects on the microbiome. Examining microbiomes across host developmental stages has allowed for closer examinations of the establishment of microbes, showing that diet is the main source of bacteria and fungi for developing solitary bees (Dew et al., 2020; Pozo et al., 2020; Kapheim et al., 2021; Nguyen and Rehan, 2022). Sociality can influence the bee microbiome by impacting how solitary and social bees interact with food resources, the environment, and other bees to transmit different microbes (Mohr and Tebbe, 2006). Across various bee species, environmental factors such as climate (McFrederick and Rehan, 2019), agriculture (Motta et al., 2018; Muñoz-Colmenero et al., 2020), natural habitat, floral resources, and wild bee diversity in the landscape can all shape microbe composition (Cohen et al., 2020; Shell and Rehan, 2022). In previous studies of Osmia lignaria, the presence of some microbes, such as Apilactobacillus sp., was associated with increased green spaces and an increased relative rarified ASV abundance in bees from less developed landscapes as opposed to urban and highly developed sites (Cohen et al., 2020). Thus, microbial members within the microbiome are subject to many different factors that can change their abundance, composition, and diversity.
The impact of a changing environment on bees and their microbiome needs to be studied as urbanization and anthropogenic activities continue to alter bee habitats in growing cities (Ritchie and Roser, 2018; Wilson and Jamieson, 2019; Ayers and Rehan, 2021; Prendergast et al., 2022). With more than half of the world currently living in urban areas and projections predicting this number to increase to two thirds of the global population living in a city centre by 2050 (Ritchie and Roser, 2018), the growth of large, developed areas is undeniable. Decreased availability of green space and the urban heat island effect tends to result in increased temperatures and reduced precipitation in these areas (Rinner and Ussain, 2011; Steensen et al., 2022). Urbanization affects the availability of green space, abundance and richness of floral resources, microclimate, and habitat quality for bees, changing the landscape features that can shape bee microbiomes (Goulson et al., 2015; Buchholz et al., 2020; Cohen et al., 2020, 2022; Ayers and Rehan, 2021; Danko et al., 2021). Floral abundances and garden sizes have a direct, positive effect on parasite and pathogen richness that is harmful to bumble bees, attributable to increased transmission from more resource provisioning (Cohen et al., 2022). Wild bumble bees have also been shown to harbour pesticides in both agricultural and urban landscapes (Botías et al., 2017), potentially jeopardizing microbial composition (Kakumanu et al., 2016; Rothman et al., 2020). Characterizing the microbiome of urban bees and how its composition and diversity varies across different landscapes offers an essential step towards understanding contributing factors to changes in bee health.
This study examines the small carpenter bee C. calcarata Robertson (Hymenoptera: Apidae). These subsocial bees nest within pithy stems, laying eggs on mass provisions that will provide brood the total nutrition required until they are fully grown (Michener, 2007; Rehan and Richards, 2010). Numerous studies have characterized diversity and composition of the microbiome and pollen provisions in C. calcarata (McFrederick and Rehan, 2016; Graystock et al., 2017; Dew et al., 2020; Nguyen and Rehan, 2022). In adult bees this core microbiome consists of 13 bacterial phylotypes, including Lactobacillus, Acinetobacter, Methylobacterium, Pseudomonas, and Gilliamella (Graystock et al., 2017; Shell and Rehan, 2022), several of which are common in other bee microbiomes as well (Vásquez et al., 2012; Voulgari-Kokota et al., 2019; Kapheim et al., 2021). The C. calcarata fungal microbiome includes members such as Alternaria, Ascosphaera and Penicillium (Nguyen and Rehan, 2022). However, despite various characterizations of this small carpenter bee bacterial and fungal microbiome, closer investigations into the specific factors driving differences in microbial composition and diversity, as well as the functional role of different microbial taxa on maintaining bee health, are fundamental.
The aim of this study is to determine whether the microbiome of adult C. calcarata differs across an urbanization gradient including local environmental features: percent land use development, percent green space, temperature, and precipitation. Using 16S and ITS metabarcoding, we examined the respective bacterial and fungal composition and diversity within 58 female small carpenter bees collected across a densely urban landscape, with different levels of urbanization. Here, we hypothesize that bees living under different environmental conditions across an urban land use gradient will result in varying microbial composition. We predict lower microbial diversity and the underrepresentation of beneficial microbes in more urban and developed areas with less available green space, increased temperatures, and reduced precipitation. This research aims to understand the differences in the microbiomes of wild bees living under different levels of urbanization.
Materials and methods
In June–July 2019 and 2020, 58 female individuals of C. calcarata Robertson (Hymenoptera: Apidae) were collected across 29 sites within Toronto, Canada (43.6532° N, 79.3832° W) (Figure 1). Between one and three bees were selected from each site and sites were chosen to cover a widespread area across the city. Ceratina calcarata is a native small carpenter bee commonly found in urban and rural contexts across eastern North America, including within the city of Toronto (Packer et al., 2007; City of Toronto, 2016; Shell and Rehan, 2016; Dew et al., 2020; Kelemen and Rehan, 2021). Nests established in the pithy stems of sumac, Rhus typhina, were opened with the lone adult female being removed from each collected nest, flash frozen in liquid nitrogen, and stored at-80°C until DNA extractions.
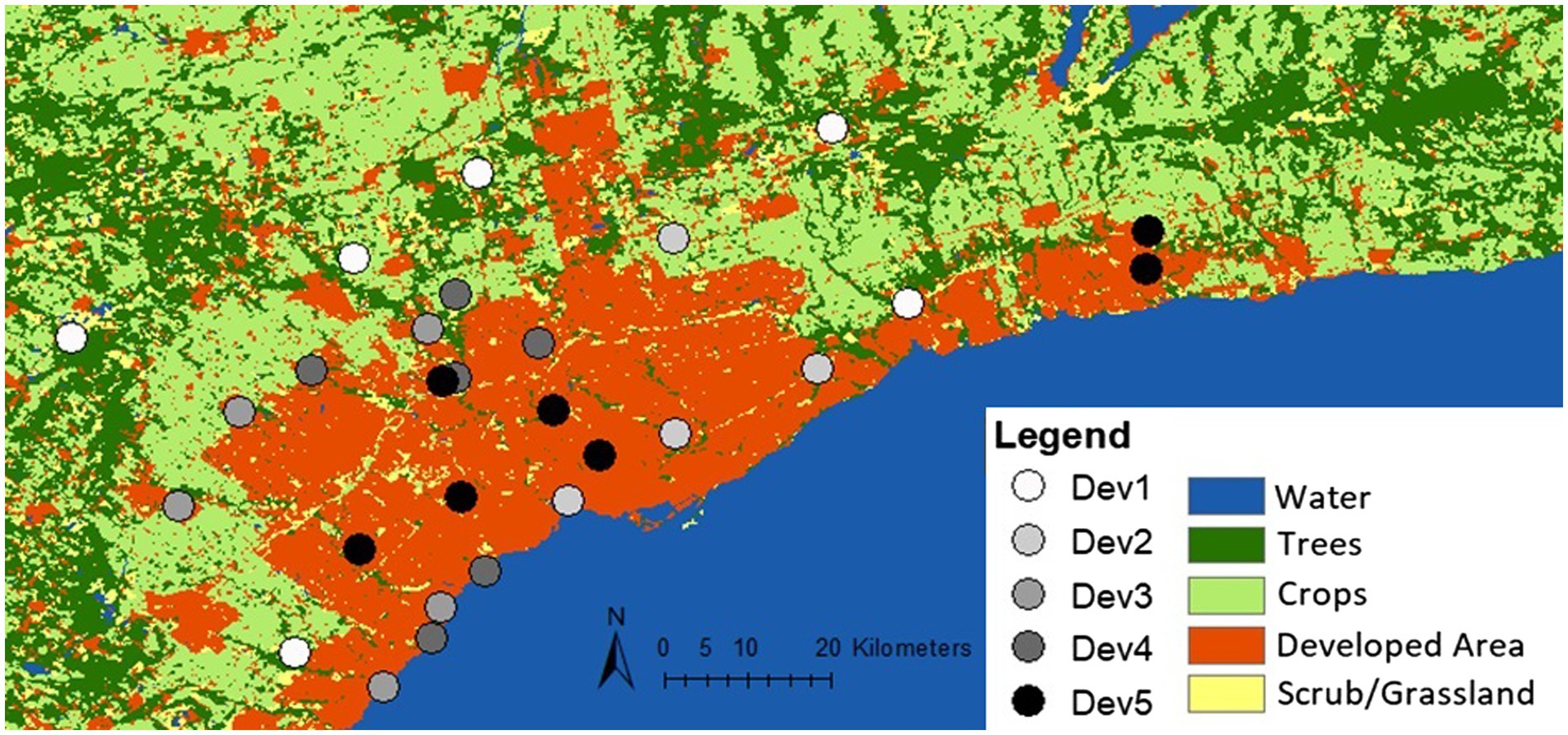
Figure 1. Development distribution and collection locations in and around Toronto, Canada using the Sentinel-2, 10 m land use time series from 2017 to 2021 by ESRI. Circles represent 29 collection sites. The trees class includes trees and flooded vegetation. Crops include human planted grass and crops below tree height. Scrub/grassland consists of bare ground and rangeland, or open areas with homogenous grasses. Developed areas are built areas with human made structures, roads, and impervious surfaces.
The collection map was created using the Sentinel-2 land use/land cover timeseries from 2017 to 2021 by Impact Observatory, Microsoft and Esri at a resolution of 10 m (Karra et al., 2021; Figure 1). Collection sites were characterized into five different levels of urban intensity using measurements of the developmental percent, percent green space, mean annual temperature, and annual precipitation (Supplementary Table S1). These categories were assigned by evenly dividing the range of values for each environmental variable into five categories ranging from 1 (very low) to 5 (very high). Landscape features of developmental percent and percent green space were calculated at each collection site using the Ontario Land Cover Compilation (OLCC) v.2.0 in ArcGIS as a percentage of landscape cover within a 500 m radius from the collection point (Land Information Ontario, 2019). Climate data, including mean annual temperature and annual precipitation, were calculated using the same process with WorldClim v.2.0 data at a resolution of 30 s (Fick and Hijmans, 2017). These features provide an overall characterization of urban land use gradients in the study region and were divided into the five categorical levels for later analyses (Supplementary Table S1).
DNA extractions were performed using the Omega-Biotek E.Z.N.A. Soil DNA kit, following the manufacturer’s protocol for 100–250 mg samples, with some modifications as described in Nguyen and Rehan (2022). This included the addition of 100 μg of 1xPBS, 30 μl of proteinase K, 5 μl of RNAse and manual crushing of the bees using a sterile pestle. DNA concentrations were checked using a QuBit HS DNA assay (Invitrogen) prior to submission to the Génome Québec Centre D’Expertise et de Services (Montreal, Canada), who conducted library preparation and sequencing. Illumina MiSeq amplicon sequencing with 300 bp paired-end reads was conducted using the 16S rRNA region for bacteria with the V5-V6 fragment (forward primer 799F-mod3 CMGGATTAGATACCCKGG and reverse primer modified 1115R AGGGTTGCGCTCGTTG) as in McFrederick and Rehan (2019) and the ITS region for fungi with the ITS1 fragment (forward primer ITS1F CTTGGTCATTTAGAGGAAGTAA and reverse primer ITS2 GCTGCGTTCTTCATCGATGC).
Qiime2 was then used to process reads for microbiome analysis (Bolyen et al., 2019). Demultiplexed sequences underwent sequence quality control using the DADA2 pipeline, which filters phiX reads, chimeric sequences, and joins paired ends (Callahan et al., 2016). Sequences were omitted when quality scores dropped below 30 and read lengths fell below 283 bases for forward reads and 260 bases for reverse reads. Qiime2 was also used to generate feature tables, representative sequences, and taxonomy tables (Price et al., 2010; McDonald et al., 2012; Katoh and Standley, 2013; Weiss et al., 2017; Bolyen et al., 2019). ASVs were tested against the SILVA 128 99% OTUs full length sequences classifier for 16S bacterial sequences and the UNITE 99% OTUs classifier for ITS sequences using the q2-feature-classifier and classify-sklearn pipeline (Pedregosa et al., 2011; Yilmaz et al., 2014; Bokulich et al., 2018; Abarenkov et al., 2021). The SILVA database with 99% sequence identity was used for its refinement and removal of duplicate sequences (Glöckner et al., 2017). Taxonomic classifications were then cross referenced against the NCBI nt database using BLAST, where classifications from the NCBI database were used to clarify and prioritized when there were any discrepancies within the two classifiers (Johnson et al., 2008, 2021).
Resultant amplicon sequence variants (ASVs) read counts and taxonomic classification tables for each ASV were imported into R (version 3.6.1) for further statistical analysis (R Core Team, 2019). ASVs of the genera Wolbachia and Sodalis were removed as they are common intracellular endosymbionts present due to mite contamination (Graystock et al., 2017). While one blank did not contain any reads, ASVs identified in the other two of blanks were reagent or human-sourced contaminants and either absent in all samples or had low read counts of less than 50 reads. Using the “phyloseq” package, reads from three blanks were proportionally removed (McMurdie and Holmes, 2013).
Alpha and beta diversity analyses, measured using the Shannon diversity index and Bray–Curtis dissimilarity respectively, were conducted using the “phyloseq” package (McMurdie and Holmes, 2013). The adonis function was used to conduct permutational multivariate analyses (PERMANOVA) that test whether microbial composition varies significantly in the different levels of urbanization (Oksanen et al., 2020). Assumptions required for the PERMANOVA test were validated using the betadisper function and significant results followed up with Tukey’s HSD test (Oksanen et al., 2020).
Bipartite networks were created using the “bipartite” package in R, examining associations between the top 18 bacterial and fungal taxa and land use development gradients (Dormann et al., 2008, 2009; Dormann, 2011). Statistics were calculated at the species level, examining the degree of connectance, effective number of interacting partners, Shannon diversity of interactions, and closeness centrality in a weighted network across five categories of land use development (Dormann et al., 2008). Redundancy analyses (RDA) were conducted using the rda function from the “vegan” package (Oksanen et al., 2020). Using the decostand function in “vegan,” the Hellinger transformation was applied to taxa abundances and the environmental variables were standardized prior to RDA analyses (Legendre and Gallagher, 2001). An ANOVA like permutation test was performed with the anova.cca function to determine the significance of which environmental features could model microbe abundance.
Similarity percentage (SIMPER) values were calculated within the PAST (version 4.07) program to identify taxa predominantly leading to differences in diversity (Hammer et al., 2001). Furthermore, correlation analyses using CoNet and SparCC were conducted to find co-occurring bacterial and fungal taxa amongst all the bees. CoNet was performed using the package “CoNetinR” and edge scores calculated with Spearman, Bray, Pearson, and Kullback–Leibler (Faust and Raes, 2016). The package “SpiecEasi” was used to conduct SparCC analyses with 100 bootstrap replicates (Friedman and Alm, 2012).
Results
Metabarcoding of the 58 adult C. calcarata resulted in an average of 31,394 reads, ranging from 19,860 to 43,593 paired-end reads per sample. The average quality of these reads was 34.5. A total of 192 bacterial and 367 fungal amplicon sequence variants (ASVs) with a mean sequence length of 317 bp were found and compared across 58 bee samples.
Diversity
Microbial community composition did not reveal differences across urban land use gradients through alpha diversity or due to sample collection date over the range of 2 years (Supplementary Figure S1). Sample collection year did not associate with any differences in the alpha diversity, beta diversity, or relative abundance of bacterial and fungal taxa. Shannon diversity index comparisons across each environmental variable revealed no overall significant differences in microbial alpha diversity among the five categorical levels of developmental percent, green space percent, temperature, or precipitation (Supplementary Figure S1).
Bray-Curtis dissimilarities revealed bacterial and fungal differences in beta diversity across three environmental variables including land use development, precipitation and temperature (Figure 2; Supplementary Figure S2). Land use development percent was associated with both bacterial and fungal beta diversity (PERMANOVA; bacteria, R2 = 0.10, df = 4, p = 0.017; fungi, R2 = 0.10, df = 4, p = 0.023; Figure 2). Figure 2A indicates that individuals from moderate to very high levels of development were similar in microbial composition and dissimilar to individuals from sites with very low to low levels of development. Samples from sites with very high development had more bacterial genera richness than sites with low development (Supplementary Table S2), corroborating that development positively associates with bacterial richness. However, green space percent was not a significant factor in determining differences in Bray-Curtis diversity (PERMANOVA; bacteria, R2 = 0.07, df = 4, p = 0.18; fungi, R2 = 0.09, df = 4, p = 0.056; Supplementary Figures S2A,B).
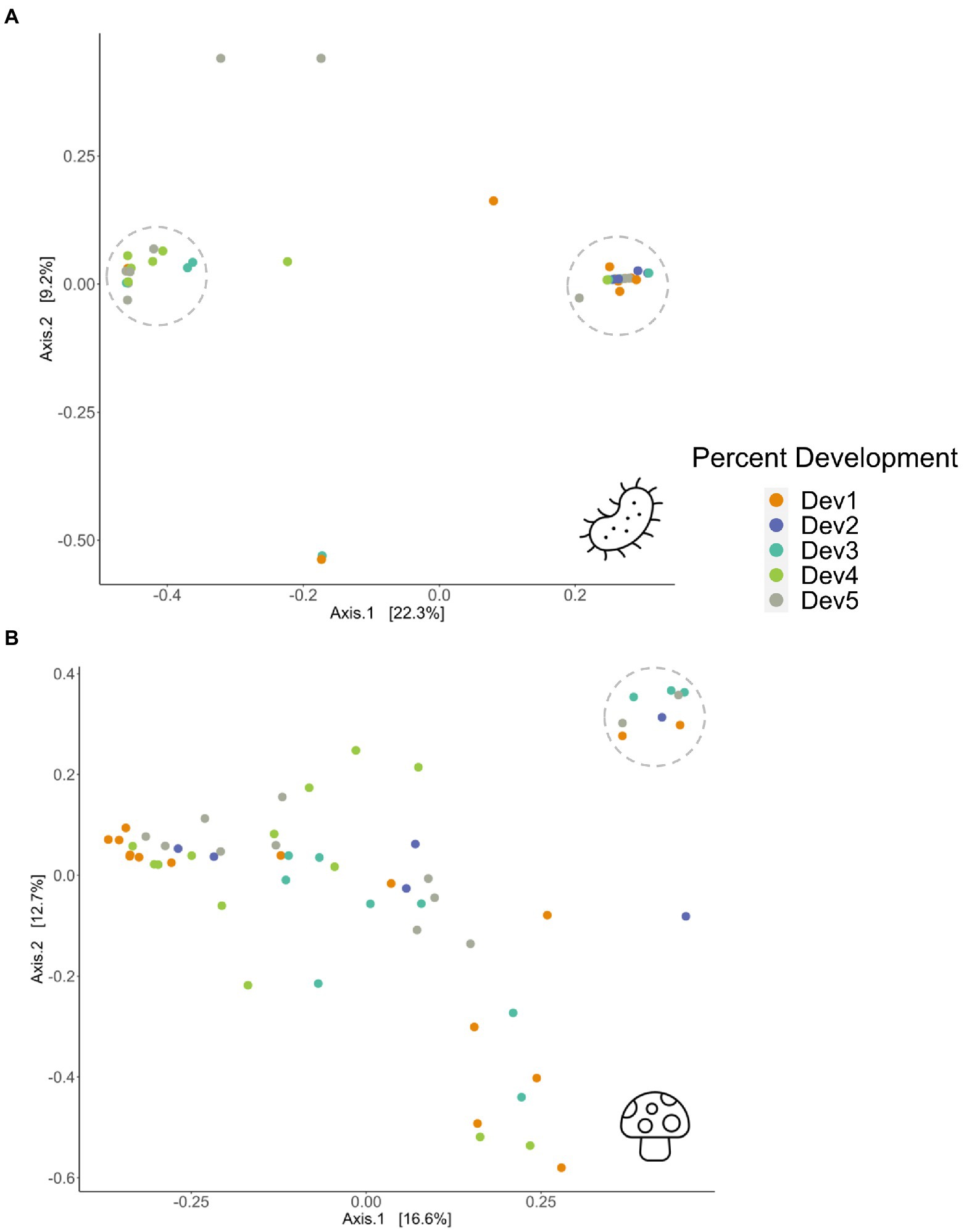
Figure 2. PCoA plots of Bray–Curtis dissimilarity matrices showing (A) bacterial (p = 0.017) and (B) fungal (p = 0.023) beta diversity in 58 adult Ceratina calcarata across five levels of percent development, ranging from Dev 1 (very low development) through Dev 5 (very high development). For exact development percentages, see Supplementary Table S1. Dotted circles represent clusters of individuals with similar beta diversity.
Temperature explained variations in fungal beta diversity (PERMANOVA; fungi, R2 = 0.09, df = 4, p = 0.021; Supplementary Figure S2D), while bacterial beta diversity did not pass the test for homogeneity of multivariate dispersions with temperature (betadisper; bacteria, F = 3.13, df = 4, p = 0.018; fungi, F = 0.33, df = 4, p = 0.855; Supplementary Figure S2C). As for precipitation gradients, fungal beta diversity differences were detected, while bacterial beta diversity differences were insignificant (PERMANOVA; bacteria, R2 = 0.08, df = 4, p = 0.181; fungi, R2 = 0.12, df = 4, p = 0.002; Supplementary Figures S2E,F). Clear clustering was less evident for the fungal PCoA, suggesting increased dissimilarity between individuals (Figure 2B). One group of fungal samples that were clustered, indicating similar beta diversity, tended to have moderate to high annual temperatures, and low to moderate annual precipitation (Supplementary Figures S2D,F). This was also consistent comparing the average number of genera across the environmental variables, which saw that the low to moderate development, high temperature, and low precipitation categories had the highest fungal genera richness (Supplementary Table S3). However, the individuals grouped closely on the PCoA were spread across different levels of land use development and green space, suggesting the environmental variables are not always correlated with each other. As temperature, green space, and precipitation were not significant environmental variables for bacteria, this comparative analyses between the environmental variables could not be performed for the bacterial PCoA.
An RDA was conducted and analyzed using an ANOVA with 999 permutations on all four environmental variables to determine which variables were associated with bacterial and fungal taxa (Figure 3). Development was significant (ANOVA; bacteria, F = 1.86, df = 1, p = 0.032; Figure 3A) in associations between urbanization level and bacterial taxa. Green space was significantly associated with both bacterial and fungal taxa (ANOVA; bacteria, F = 1.86, df = 1, p = 0.037; fungi, F = 2.45, df = 1, p = 0.002; Figures 3A,B). Precipitation was also key in the RDA analyses for fungal taxa (ANOVA; fungi, F = 1.86, df = 1, p = 0.012; Figure 3B) with variation in precipitation explaining variation in fungal taxa. In addition to the RDA with all environmental variables, forward selection modelling was performed to select the driving environment variables that could predict diversity. Bacterial taxa revealed a significant model (ANOVA; bacteria, F = 1.80, df = 1, p = 0.016, adjusted R-squared = 0.017) associated with development (ANOVA; bacteria, F = 1.86, df = 1, p = 0.036) and temperature (ANOVA; bacteria, F = 1.74, df = 1, p = 0.046). The fungal model resulted in a different significant model (ANOVA; F = 1.97, df = 1, p = 0.007, adjusted R-squared value of 0.17) involving only temperature (ANOVA, fungi, F = 1.97, df = 1, p = 0.005) predicting fungal taxa.
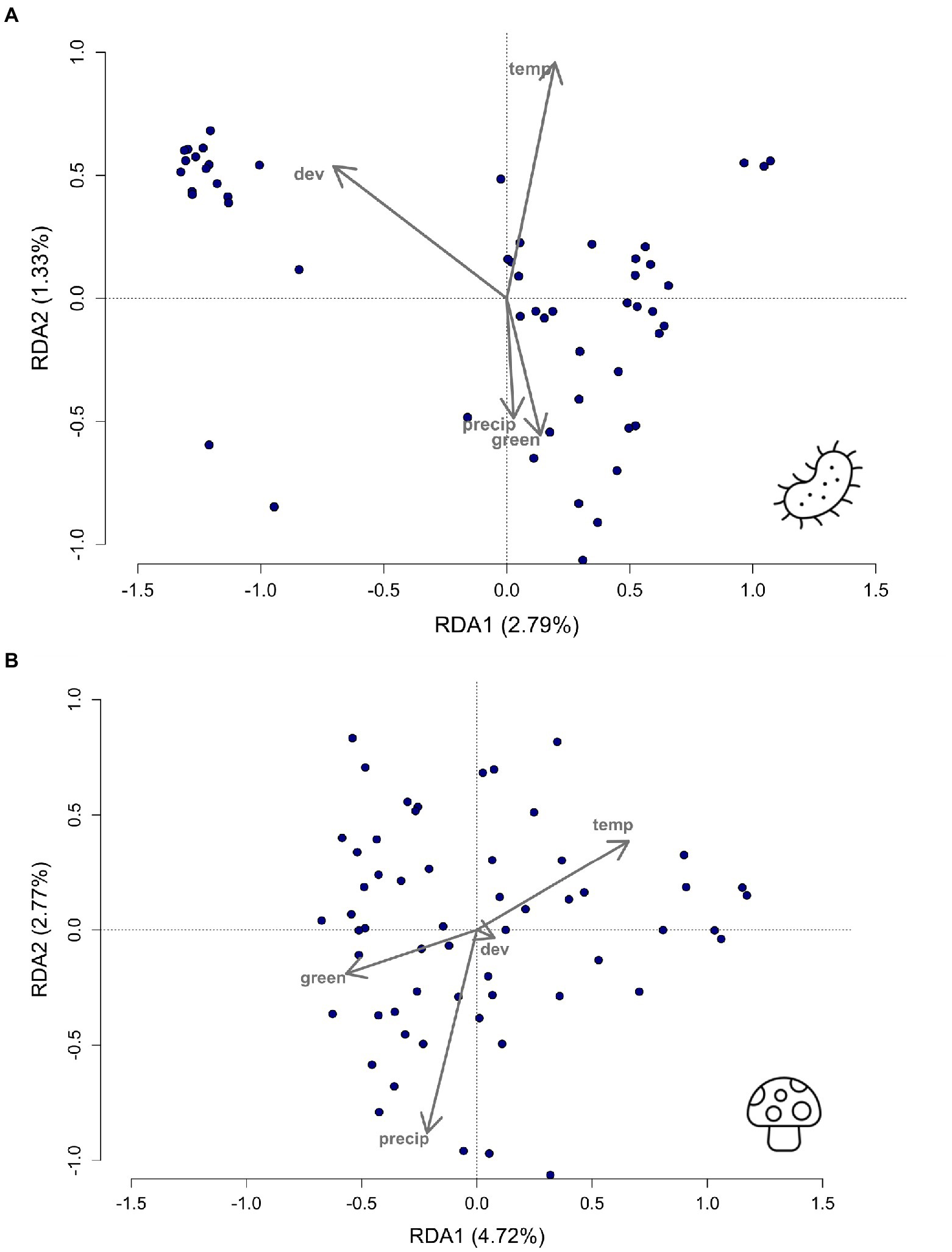
Figure 3. Redundancy analyses (RDA) plot showing whether (A) bacterial (p = 0.030) and (B) fungal (p = 0.001) taxa are associated with the environmental variables of dev = development, green = green space, temp = temperature, and precip = precipitation. Bacterial taxa are influenced by the development (p = 0.032) and green space (p = 0. 037) variables. Fungal taxa are driven by green space (p = 0.002) and precipitation (p = 0.012).
Taxonomy
Across the 58 samples, the bacterial genera Acinetobacter, Apilactobacillus, Nocardia, and Saccharibacter had the greatest summed relative abundances amongst all the bacterial genera (Supplementary Table S2). Particularly notable, Apilactobacillus had a relative abundance of over 50% of the total reads in 25 samples (Figure 4A). A low amount of bacterial diversity is noticed amongst the adults, as 26 samples contained reads from only one genus and 12 samples had two bacterial genera (Figure 4A; Supplementary Table S2). Overall, there was an average of 3.6 bacterial genera associated with each bee. In terms of fungi, Alternaria, Ascosphaera, and Penicillium had the greatest summed relative abundances and were common genera in the bee microbiome (Supplementary Table S3). Fungal genera richness was higher than bacterial, with an average of 6.5 genera per individual (Figure 4B; Supplementary Table S3).
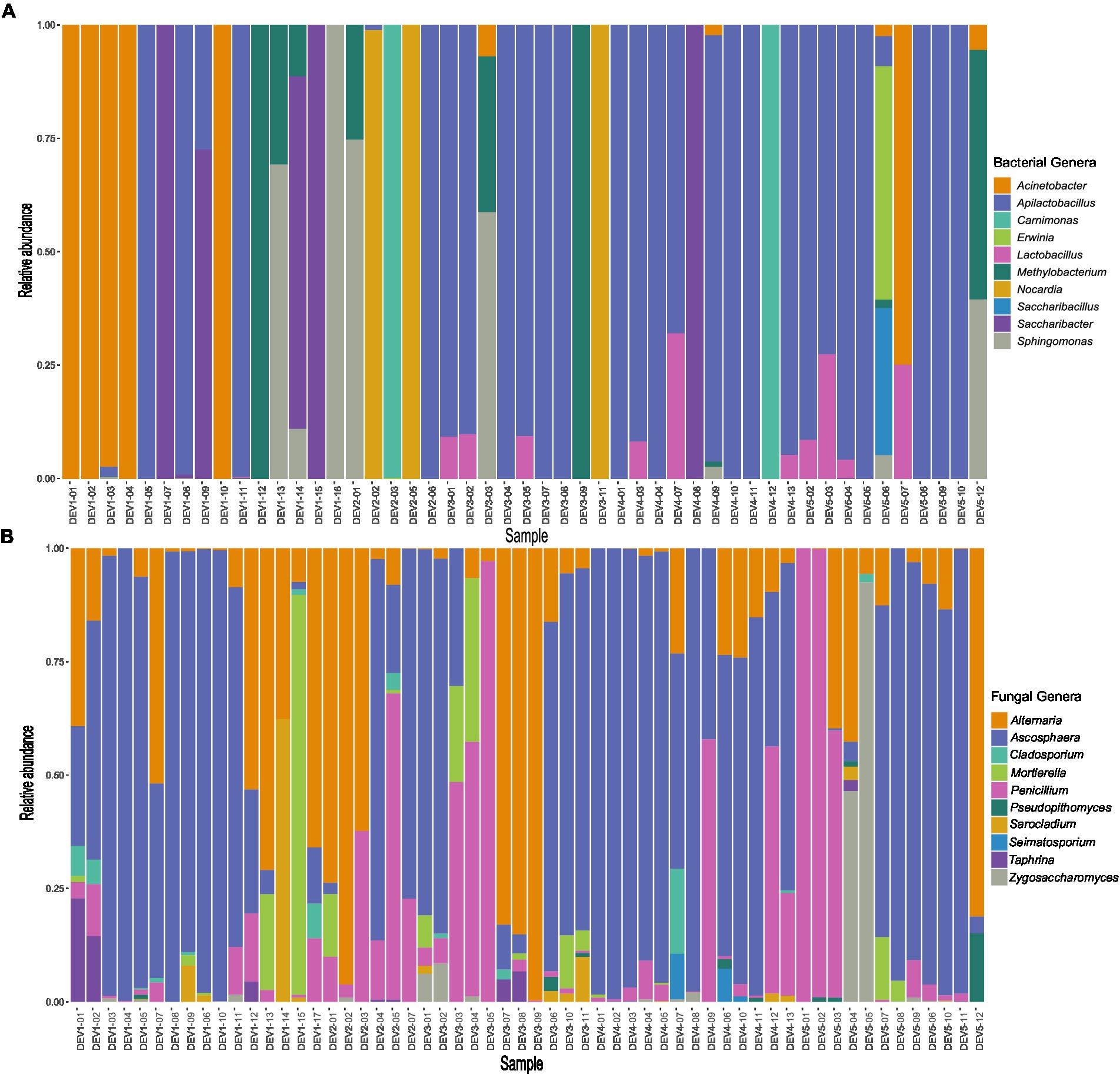
Figure 4. Top 10 (A) bacterial and (B) fungal genera found in 58 Ceratina calcarata from different levels of development across Toronto. The five categories range from Dev 1 (very low development) through Dev 5 (very high development).
Similarity percentage (SIMPER) analyses corroborated bacterial and fungal relative abundances were driven by environmental features (Supplementary Table S4). Some bacteria and fungi are typically overrepresented at either high levels of development or green space, suggesting patterns along an urbanization gradient (Supplementary Table S4). For example, Acinetobacter and Saccharibacter had high abundances in very low levels of development (Supplementary Table S4). On the contrary, Lactobacillus bacteria were found mostly in areas with moderate to high levels of development (Supplementary Table S4). Apilactobacillus was simultaneously overrepresented in areas with the highest amount of green space, least amount of development, and high levels of development (Supplementary Table S4). In terms of fungi, Ascosphaera was similarly abundant at both ends of the spectrum at low, high, and very high levels of development (Supplementary Table S4). Taphrina fungi were overrepresented in areas with very low levels of development, whereas the opposite was true for Zygosaccharomyces being overrepresented in areas of high development (Supplementary Table S4). Differences in taxa abundances were also apparent across varying precipitation and temperature gradients, with Alternaria being underrepresented with increased levels of precipitation and Apilactobacillus most abundant in environments with low annual temperature (Supplementary Table S4). In examining the clustered individuals on the fungal PCoA from sites with low precipitation and high temperatures (Supplementary Figures S2D,F), Alternaria was present in all these samples, with other common fungi including Mortierella and Ascosphaera. Thus, urbanization markedly characterizes disparate overrepresentations in bacteria and fungi.
To examine the uniqueness and connectedness of the microbiome across an urbanization gradient, bipartite networks were used to examine associations between different levels of development and microbial genera (Table 1; Supplementary Table S5; Supplementary Figure S3). The bacterial network resulted in overall lower levels of connectance (bacteria, 0.54; fungi, 0.82), links per species (bacteria, 2.13; fungi, 3.26), and Shannon diversity (bacteria, 1.56; fungi, 2.53) when compared to the fungal network. Bees from sites with the greatest percent development were found to have a higher degree of bacterial connectance, Shannon diversity of interactions, and effective partners, while also inversely showing low weighted closeness, when compared to areas with the least percent development (Table 1). Sites with low to moderate and very high percent development showed more abundant but less specialized relationships between bacteria and bees. This pattern held for fungi at a moderate level of land use development (Table 1). Thus, fungal networks maintained more consistency across the urban land use gradients. Networks revealed certain microbes like Apilactobacillus, Alternaria, Ascosphaera, and Penicillium had high degrees of connectance across all five development levels, whereas others had low degrees of connectance and were associated with an urbanization level. For example, Clostridium and Saccharibacillus were only found in very high levels of development and Enterobacter and Samsoniella were associated with very low levels of development.
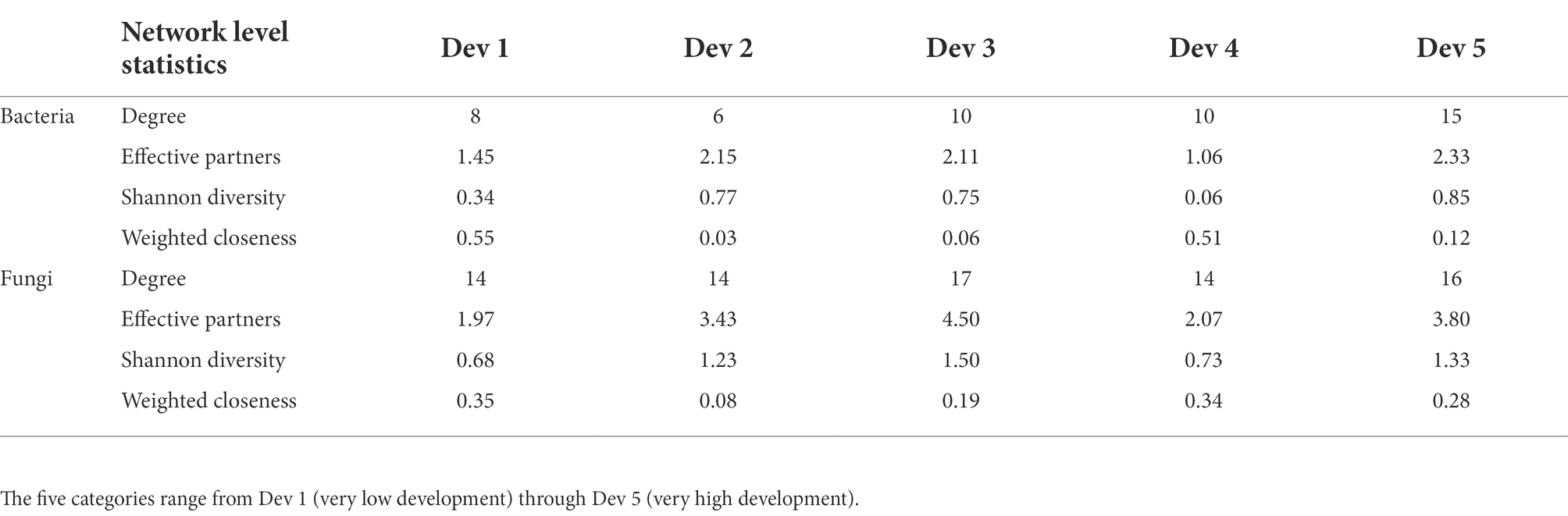
Table 1. Summary of bipartite network level statistics comparing the association between the top 18 bacterial and fungal taxa across five levels of development.
Bacterial and fungal co-occurrences
CoNet and SparCC analyses were used to determine if any bacterial and fungal associations were found among the top 10 bacterial and fungal taxa across all 58 samples (Table 2; Supplementary Tables S6, S7). Using all individuals, CoNet revealed 17 associations (Supplementary Table S6), while SparCC presented 28 total co-occurrences of positive and negative correlations (Supplementary Table S7). Interestingly, a positive association between bacteria-bacteria was found to be significant across both statistical analyses in Sphingomonas and Saccharibacillus (CoNet, correlation = 0.31, p = 0.019; SparCC, correlation = 0.53, p < 0.01; Supplementary Tables S6, S7). Additionally, separate analyses for each land use development level revealed patterns in co-occurrences. Through CoNet analyses, Metholybacterium was only found not correlated to Sphingomonas in the very low and moderate development levels, while Acinetobacter and Sphingomonas were associated only in the moderate to high development levels (Table 2). Associations between fungi-bacteria and fungi-fungi were absent in densely urban areas and only seen in sites with the lowest land use development, with the rest of the associations only existing between bacteria (Table 2).
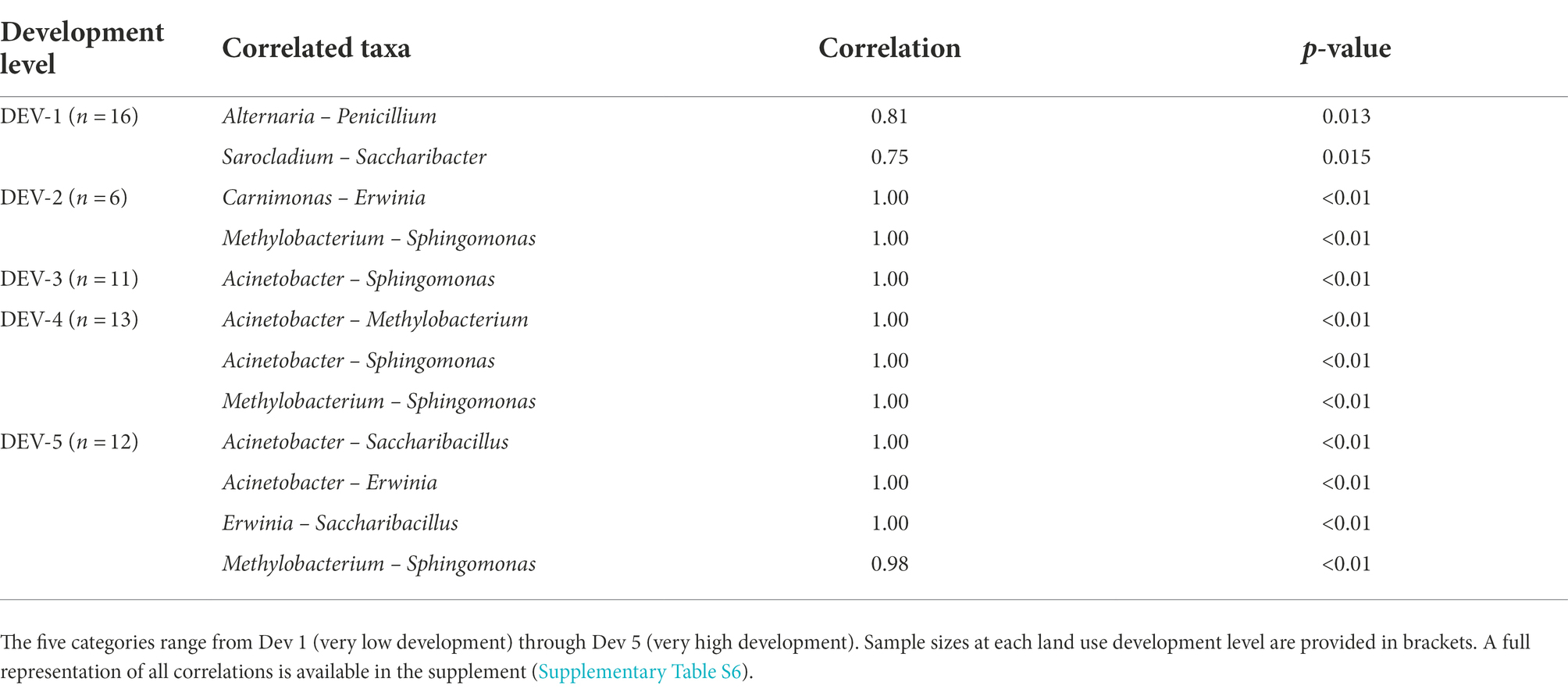
Table 2. CoNet correlations between bacteria-bacteria, fungi-fungi, and fungi-bacteria within the top 10 bacterial and fungal genera found in 58 Ceratina calcarata across five levels of land use development.
Discussion
This study examines the bacterial and fungal microbiome in 58 C. calcarata individuals across an urban land use gradient. Percent development, percent green space, annual temperature, and annual precipitation were examined to determine how environmental factors may drive differences in microbial diversity and composition. While alpha diversity did not differ across the city, beta diversity and redundancy analysis modelling could be predicted by percent development and temperature. Taxonomic comparisons also revealed some bacterial and fungal taxa were more commonly found in either very low or highly developed areas of the city, indicating differences in the microbiome between urban land use gradients. Different levels of land use development also result in varying degrees of connectance in networks and different co-occurrences between microbes.
Microbial diversity
Shannon’s diversity indices, a measure of alpha diversity, did not vary with environmental variables of development, green space, precipitation, or temperature and yielded low values of bacterial diversity, where more than half of the samples only contained one or two genera (Supplementary Figure S1). This aligns with a previous study of C. calcarata in Toronto, which found little change and overall low microbial alpha diversity as bees matured from brood to adults (Nguyen and Rehan, 2022). Similarly, a study comparing the stingless bee Tetragonula carbonaria microbiome between two different sites also showed alpha diversity remaining consistent, despite climatic and floral resource differences (Hall et al., 2021). Although studies with stingless bees have revealed the presence of environmental bacteria in the microbiome (Kwong et al., 2017b; Cerqueira et al., 2021), social bees often have a core microbiota and low diversity (Kwong and Moran, 2016). However, this is not representative of solitary wild bees, such as in a study with Osmia lignaria across different environmental contexts, which found that environmental factors drove differences in relative ASV abundances and alpha diversity (Cohen et al., 2020). Yet, as many factors affect microbiome alpha diversity it remains difficult to segregate how factors are affecting overall diversity in isolation and how a combination of environmental or situational variables co-occurring can affect alpha diversity.
Beta diversity, represented by Bray–Curtis dissimilarity matrices (Figure 2), was able to capture more of the microbial differences driven by urban land use gradients. Percent development was the most significant factor, with development associating with both bacterial and fungal Bray-Curtis dissimilarities, high development showing increased bacterial genera richness, and with development able to predict bacterial diversity through the redundancy modelling analyses (Figures 2, 3; Supplementary Table S2). Annual temperature was another considerable variable, yielding a significant ability to model differences in both bacterial and fungal microbiomes and associate with fungal beta diversity (Supplementary Figure S2F). Collectively, the interplay between these environmental characteristics may be dynamically changing microbial diversity, as features such as high temperature and low precipitation can act together to foster higher fungal genera richness and clustered Bray-Curtis dissimilarities, as seen in the circled individuals on the PCoA plots (Supplementary Table S3; Supplementary Figures S2D,F). However, these samples did not cluster with either land use development or green space, suggesting that the correlation between environmental variables is unclear (Figure 2; Supplementary Figure S2). Environmental features were expected to be a factor determining differences in beta diversity, as was initially seen in a study comparing two colonies of stingless bees at different locations (Hall et al., 2021), and in O. lignaria when dissimilarity matrices could be predicted by percent natural cover, number of trees and shrubs, bee species richness, and bare soil (Cohen et al., 2020). Similarly, McFrederick and Rehan (2019) found different species richness of fungi and bacteria when comparing subtropical, temperate and grassland zones across Australia, suggesting that climate shapes the C. australensis microbiome. Thus, environmental characteristics describing both land use and climate affect the microbial diversity of individual C. calcarata microbiomes.
Microbial composition
Apilactobacillus, Alternaria, Penicillium and Ascosphaera were the most prevalent and abundant bacterial and fungal genera found across the city (Supplementary Tables S2, S3). Apilactobacillus are common beneficial bee symbionts (Tlais et al., 2022) and were established as part of the core microbiome in C. calcarata in New Hampshire, a more rural landscape (McFrederick and Rehan, 2016; Graystock et al., 2017). In urban cities such as Toronto, Apilactobacillus was previously largely absent in adult C. calcarata (Nguyen and Rehan, 2022) and was found to be underrepresented at sites with moderate levels of land use development, overrepresented in sites with the most green space, and overrepresented at sites with lower annual temperatures in this study (Supplementary Table S4). Thus, urban bees reveal a different microbiome from those in rural contexts and of particular concern is the varying abundance of Apilactobacillus.
The fungal genus Ascosphaera contains both pathogenic and apathogenic fungi (Klinger et al., 2013), and the species A. major was common in C. calcarata (Supplementary Table S3). This species has caused chalkbrood-like diseases in Megachile centuncularis and Apis mellifera, but can also live relatively harmlessly as a facultative parasite within bee nests on pollen provisions and larval feces, including other wild bees such as Osmia bicornis (Holm and Skou, 1972; Bissett, 1988; Wynns et al., 2013). Therefore, the abundance of Ascosphaera may indicate a commensalism between C. calcarata and these bee specialist fungi. Future studies are needed to determine the fitness effects of Ascosphaera on this species.
Overrepresentations of certain bacterial and fungal taxa at sites of varying land use development may indicate such factors are affecting microbial composition. Areas with a low percentage of development were found to have a greater abundance of Acinetobacter, Ascosphaera, Saccharibacter, and Taphrina (Supplementary Table S4). Acinetobacter is a flower-associated species of bacteria also commonly associated with yeasts in nectar which can induce germination and pollen bursting that then benefits pollinators by way of improved nutrition from nectar (Christensen et al., 2021; Rering et al., 2021). Another flower-associated bacteria, Saccharibacter, is closely related to the bacteria Bombella apis which is known to protect developing honey bees from fungal pathogens and contains genetic loci involved with nutrition, microbial and host interactions, and immunity (Smith and Newton, 2020). Thus, the overrepresentation of beneficial microbes in areas with low land use development is promising for these pollinators. On the contrary, the two fungal genera found in high abundance in more rural areas, Ascosphaera and Taphrina, are facultative bee and plant pathogens, respectively, (Cissé et al., 2013; Wynns et al., 2013). However, these genera were also previously seen in immature C. calcarata and it is unclear if they pose any threat to this species (Nguyen and Rehan, 2022). Ascosphaera was also overrepresented at high and very high development levels (Supplementary Table S4; Figure 4B), suggesting this fungi may not be limited to rural areas.
The overrepresented genera present in sites with a high percentage of development, such as Lactobacillus, Penicillium, and Zygosaccharomyces (Supplementary Table S4), were not microbes that are known to be harmful to bee health. Lactobacillus spp., such as L. crispatus and L. intestinalis, have been seen in A. mellifera, Bombus terrestris, and O. bicornis (Mohr and Tebbe, 2006), and many studies have uncovered the important and beneficial relationship between Lactobacillus and bees (Rothman et al., 2019; Li et al., 2021). Penicillium molds are commonly found in Melipona scutellaris (Barbosa et al., 2018) and A. mellifera bee bread and is of importance as it produces enzymes involved in lipid, protein, and carbohydrate metabolism that can even protect bees against fungicides (Gilliam et al., 1989; Yoder et al., 2013). Zygosaccharomyces sp. are fungi that have been shown to provide steroid precursors crucial for the pupation of the stingless bee Scaptotrigona depilis (Paludo et al., 2018). Hence, while differing from areas of low land use development, bacteria and fungi found in urban bees may be supported by their own beneficial properties to their bee hosts. Regardless of urbanization, different overrepresentations of bacteria and fungi may serve varying, but equally beneficial, purposes. Areas with low development seem to harbour plant associated microbes that may be associated with natural plant availability, whereas high development sites contain microbes more associated with bee development and digestion.
Co-occurrences between microbes have been studied in bees and pollen to examine how microbial members interact and establish the microbiome (Russell et al., 2011; Graystock et al., 2017; Manirajan et al., 2018; Dew et al., 2020). This study found a strong positive relationship between Sphingomonas and Saccharibacillus when examining adults (Supplementary Tables S6, S7). While Saccharibacillus has been found in commercial bee pollen from Europe, little is known about its interactions and presence in bee microbiomes (Andrade et al., 2019). Bacteria of the genus Sphingomonas have been shown to be negatively correlated with Fusarium species that cause Fusarium Head Blight in maize crops (Cobo-Díaz et al., 2019). In C. calcarata, Sphingomonas co-occurred positively with the fungal genera Pantoea (Nguyen and Rehan, 2022), a genus prevalent in C. australensis (Shell and Rehan, 2022), A. mellifera (Wright et al., 2001), and stingless bees (Leonhardt and Kaltenpoth, 2014). Additionally, Sphingomonas is a dominant bacteria found in the nests of stingless bees Frieseomelitta varia, Melipona quadrifasciata, and Tetragonisca angustula (de Sousa, 2021) and is also found in A. mellifera (Anjum et al., 2018; Muñoz-Colmenero et al., 2020) and O. bicornis microbiomes (Mohr and Tebbe, 2006), thus this bacteria co-occurs naturally with wild bees. In regards to urban and agricultural bees, this bacteria may be particularly beneficial as it contains enzymes that degrade organochlorides in insecticides (Russell et al., 2011). Therefore, commonly occurring bacteria, such as Sphingomonas, may be playing an underappreciated role in the wild bee microbiome.
Microbe correlations can be examined considering land use development to determine if environmental factors may be affecting the stability of these associations. Methylobacterium was correlated with Sphingomonas in all but the low and moderate percent development sites (Table 2). Methylobacterium have shown beneficial relationships with plants and bacteria, sometimes even relying on growth factors produced by other microbes (Iguchi et al., 2015; Manirajan et al., 2018). The number of correlations present also increased with percent developed area, suggesting that urbanized areas may be associated with more positive co-occurrences between bacteria. This has been seen in urban soils, where environmental features altered microbial networks (Wang et al., 2018). Therefore, landscape features may be changing the way bacteria and fungi are supported, which can in turn affect the presence and abundance of these and other microbes. Further examination into the functional role of specific microbes as well as how they exist in symbioses is needed to explain how these networks are maintained.
Effects of a changing environment
Changes in microbial diversity and composition are of potential concern because bee-microbe symbioses play a key role maintaining bee health (Engel et al., 2012; Mockler et al., 2018; Dharampal et al., 2019, 2020; Rothman et al., 2019). While this study described several abundant bacterial and fungal genera dominating the microbiome, this is a large contrast to previous studies of C. calcarata that revealed 13 core bacterial phylotypes in bees and much more diversity (Graystock et al., 2017). The reason for bacteria showing decreased diversity compared to fungi, as seen in lower Shannon diversity measures, lower degrees of network connectance, and fewer effective partners (Table 2), remains unclear. This low bacterial diversity in adults was also found in a previous study of C. calcarata in Toronto, particularly when compared to developing brood, and suggests a persistent and concerning decrease in microbial diversity in urban landscapes (Nguyen and Rehan, 2022). Ongoing long-term and additional studies are needed to examine whether the few bacterial genera currently making up the microbiome are excluding other bacteria and/or whether bees in cities have generally less diverse microbiomes in this and other wild bee species.
As the urban land use gradients such as percent development and temperature reveal their effects on the bee microbiome, rapid urbanization becomes increasingly alarming. Urbanization and anthropogenic activities are a worsening problem driving declines in bee populations, altering bee community compositions, and negatively affecting certain bee species (Ritchie and Roser, 2018; Wilson and Jamieson, 2019; Ayers and Rehan, 2021; Prendergast et al., 2022). In addition, the presence of pesticides in urban areas may be driving declines in bee health and in bee microbiome structure, composition, and diversity (Kakumanu et al., 2016; Botías et al., 2017; Hotchkiss et al., 2022). Future work comparing a wider range of rural and agricultural landscapes across multiple regions will help determine how bee microbiomes change with land use. Additional studies examining pesticides present in bee habitats in urban and rural areas and how these accumulate in bees and their pollen provisions will also be important (Pisa et al., 2014; Kakumanu et al., 2016; Botías et al., 2017; Hotchkiss et al., 2022). As research continues to untangle the variables that work together to establish and maintain the wild bee microbiome, the ever-changing landscape in cities adds new considerations for possible environmental stressors.
In conclusion, this study examines the bacterial and fungal composition and diversity in adult C. calcarata across an urban land use gradient, revealing differences explained by percent land use development, green space, precipitation, and temperature. Individuals from low to moderate development levels tended to share similar bacterial composition within one cluster, while those from moderate to high development levels grouped separately. In examining fungal taxa, individuals showed greater dissimilarity in beta diversity, with climatic variables as possible drivers. The interplay of environmental factors across urbanization gradients act on microbial community composition, with overlapping characteristics such as annual temperature and annual precipitation coinciding with fungal beta diversity. Microbial composition in rural areas were dominated by genera such as Acinetobacter and Apilactobacillus, beneficial microbes that support bee health and may affect bee survival. Specific taxa varied across different levels of urbanization, which may be explained by co-occurrences between bacteria and fungi that varied amongst different land use development, and suggests that microbial relationships are dependent on changes in environment. These complex networks reveal that urban areas may exhibit a stronger degree of connectance in bacteria, while lower levels of urbanization foster greater connectance within the fungal microbiome. Overall, increased urbanization has led to a significant impact on microbial composition and diversity. As cities continue to expand and urbanization rises globally, it is increasingly important to understand how landscapes affect bee health through their microbiome.
Data availability statement
The datasets presented in this study can be found in online repositories. The names of the repository/repositories and accession number(s) can be found at: https://www.ncbi.nlm.nih.gov/ BioProject: PRJNA805022 BioSamples: SAMN25891990-SAMN25892379.
Author contributions
PN: sample preparation, DNA extraction, bioinformatics, and analyzed and visualized data. SR: conceived study, supervised, and provided funding. PN and SR: wrote and approved final manuscript. All authors contributed to the article and approved the submitted version.
Funding
This study was funded by the Weston Family Foundation Microbiome Initiative, NSERC Discovery Grant, Supplement and E.W.R. Steacie Fellowship to SR.
Acknowledgments
We thank members of the Rehan laboratory for helpful feedback on this manuscript with special thanks to Jesse Huisken, Evan Kelemen, and Mariam Shamekh for assistance with specimen collection and Génome Québec Centre D’Expertise et de Services for help with library preparation and sequencing.
Conflict of interest
The authors declare that the research was conducted in the absence of any commercial or financial relationships that could be construed as a potential conflict of interest.
Publisher’s note
All claims expressed in this article are solely those of the authors and do not necessarily represent those of their affiliated organizations, or those of the publisher, the editors and the reviewers. Any product that may be evaluated in this article, or claim that may be made by its manufacturer, is not guaranteed or endorsed by the publisher.
Supplementary material
The Supplementary material for this article can be found online at: https://www.frontiersin.org/articles/10.3389/fmicb.2022.992660/full#supplementary-material
References
Abarenkov, K., Zirk, A., Piirmann, T. P. R., Ivanov, F., Nilsson, R. H., and Kõljalg, U. (2021). UNITE QIIME release for fungi 2. UNITE Commun. doi: 10.15156/BIO/1264763
Almeida, E. L., Ribiere, C., Frei, W., Kenny, D., Coffey, M. F., and O’Toole, P. W. (2022). Geographical and seasonal analysis of the honeybee microbiome. Microb. Ecol. doi: 10.1007/S00248-022-01986-X/FIGURES/6
Andrade, V. D. M., Flores, J. L. H., López, M. A. R., Hernández, A. C., Gómez, S. R., Calvillo, R. P. M., et al. (2019). Evaluation of the presence of Paenibacillus larvae in commercial bee pollen using PCR amplification of the gene for tRNACys. Brazilian J. Microbiol. 50, 471–480. doi: 10.1007/s42770-019-00039-9
Anjum, S. I., Shah, A. H., Aurongzeb, M., Kori, J., Azim, M. K., Ansari, M. J., et al. (2018). Characterization of gut bacterial flora of Apis mellifera from north-West Pakistan. Saudi J. Biol. Sci. 25, 388–392. doi: 10.1016/J.SJBS.2017.05.008
Ayers, A. C., and Rehan, S. M. (2021). Supporting bees in cities: how bees are influenced by local and landscape features. Insects 12:128. doi: 10.3390/INSECTS12020128
Barbosa, R. N., Bezerra, J. D. P., Souza-Motta, C. M., Frisvad, J. C., Samson, R. A., Oliveira, N. T., et al. (2018). New Penicillium and Talaromyces species from honey, pollen and nests of stingless bees. Antonie van Leeuwenhoek. Int. J. Gen. Mol. Microbiol. 111, 1883–1912. doi: 10.1007/S10482-018-1081-1/FIGURES/13
Bissett, J. (1988). Contribution toward a monograph of the genus Ascosphaera. Can. J. Bot. 66, 2541–2560. doi: 10.1139/B88-346
Bokulich, N. A., Kaehler, B. D., Rideout, J. R., Dillon, M., Bolyen, E., Knight, R., et al. (2018). Optimizing taxonomic classification of marker-gene amplicon sequences with QIIME 2’s q2-feature-classifier plugin. Microbiome 6:90. doi: 10.1186/s40168-018-0470-z
Bolyen, E., Rideout, J. R., Dillon, M. R., Bokulich, N. A., Abnet, C. C., Al-Ghalith, G. A., et al. (2019). Reproducible, interactive, scalable and extensible microbiome data science using QIIME 2. Nat. Biotechnol. 37, 852–857. doi: 10.1038/s41587-019-0209-9
Botías, C., David, A., Hill, E. M., and Goulson, D. (2017). Quantifying exposure of wild bumblebees to mixtures of agrochemicals in agricultural and urban landscapes. Environ. Pollut. 222, 73–82. doi: 10.1016/J.ENVPOL.2017.01.001
Buchholz, S., Gathof, A. K., Grossmann, A. J., Kowarik, I., and Fischer, L. K. (2020). Wild bees in urban grasslands: urbanisation, functional diversity and species traits. Landsc. Urban Plan. 196:103731. doi: 10.1016/J.LANDURBPLAN.2019.103731
Callahan, B. J., McMurdie, P. J., Rosen, M. J., Han, A. W., Johnson, A. J. A., and Holmes, S. P. (2016). DADA2: high-resolution sample inference from Illumina amplicon data. Nat. Methods 13, 581–583. doi: 10.1038/nmeth.3869
Cerqueira, A. E. S., Hammer, T. J., Moran, N. A., Santana, W. C., Kasuya, M. C. M., and da Silva, C. C. (2021). Extinction of anciently associated gut bacterial symbionts in a clade of stingless bees. ISME J. 15, 2813–2816. doi: 10.1038/s41396-021-01000-1
Christensen, S. M., Munkres, I., and Vannette, R. L. (2021). Nectar bacteria stimulate pollen germination and bursting to enhance microbial fitness. Curr. Biol. 31:4380. doi: 10.1016/J.CUB.2021.07.016/ATTACHMENT/B0DC96EE-C50A-4145-B3F5-CED275B88A51/MMC1.PDF
Cissé, O. H., Almeida, J. M. G. C. F., Fonseca, Á., Kumar, A. A., Salojärvi, J., Overmyer, K., et al. (2013). Genome sequencing of the plant pathogen Taphrina deformans, the causal agent of peach leaf curl. MBio 4, e00055–e00013. doi: 10.1128/MBIO.00055-13/SUPPL_FILE/MBO002131496S2.DOCX
City of Toronto (2016). Bees of Toronto: A Guide to their Remarkable World Toronto: City of Toronto.
Cobo-Díaz, J. F., Baroncelli, R., Le Floch, G., and Picot, A. (2019). Combined metabarcoding and co-occurrence network analysis to profile the bacterial, fungal and fusarium communities and their interactions in maize stalks. Front. Microbiol. 10:261. doi: 10.3389/FMICB.2019.00261/BIBTEX
Cohen, H., McFrederick, Q. S., and Philpott, S. M. (2020). Environment shapes the microbiome of the blue orchard bee, Osmia lignaria. Microb. Ecol. 80, 897–907. doi: 10.1007/S00248-020-01549-Y/TABLES/2
Cohen, H., Ponisio, L. C., Russell, K. A., Philpott, S. M., and McFrederick, Q. S. (2022). Floral resources shape parasite and pathogen dynamics in bees facing urbanization. Mol. Ecol. 31, 2157–2171. doi: 10.1111/MEC.16374
Danko, D., Bezdan, D., Afshin, E. E., Ahsanuddin, S., Bhattacharya, C., Butler, D. J., et al. (2021). A global metagenomic map of urban microbiomes and antimicrobial resistance. Cells 184, 3376–3393.e17. doi: 10.1016/J.CELL.2021.05.002
de Sousa, L. P. (2021). Bacterial communities of indoor surface of stingless bee nests. PLoS One 16:e0252933. doi: 10.1371/JOURNAL.PONE.0252933
Dew, R. M., McFrederick, Q. S., and Rehan, S. M. (2020). Diverse diets with consistent core microbiome in wild bee pollen provisions. Insects 11:E499. doi: 10.3390/insects11080499
Dharampal, P. S., Carlson, C., Currie, C. R., and Steffan, S. A. (2019). Pollen-borne microbes shape bee fitness. Proc. R. Soc. B Biol. Sci. 286:20182894. doi: 10.1098/rspb.2018.2894
Dharampal, P. S., Diaz-Garcia, L., Haase, M. A. B., Zalapa, J., Currie, C. R., Hittinger, C. T., et al. (2020). Microbial diversity associated with the pollen stores of captive-bred bumble bee colonies. Insects 11:250. doi: 10.3390/INSECTS11040250
Dormann, C. F. (2011). How to be a specialist? Quantifying specialisation in pollination networks. Netw. Biol. 1, 1–20.
Dormann, C. F., Frueund, J., Bluethgen, N., and Gruber, B. (2009). Indices, graphs and null models: analyzing bipartite ecological networks. Open Ecol. J. 2, 7–24. doi: 10.2174/1874213000902010007
Dormann, C. F., Gruber, B., and Frueund, J. (2008). Introducing the bipartite package: analysing ecological networks. R News 8, 8–11.
Elijah Powell, J., Eiri, D., Moran, N. A., and Rangel, J. (2018). Modulation of the honey bee queen microbiota: effects of early social contact. PLoS One 13:e0200527. doi: 10.1371/JOURNAL.PONE.0200527
Engel, P., Martinson, V. G., and Moran, N. A. (2012). Functional diversity within the simple gut microbiota of the honey bee. PNAS 109, 11002–11007. doi: 10.1073/pnas.1202970109
Faust, K., and Raes, J. (2016). CoNet app: inference of biological association networks using Cytoscape. F1000Research 5.
Fick, S. E., and Hijmans, R. J. (2017). WorldClim 2: new 1-km spatial resolution climate surfaces for global land areas. Int. J. Climatol. 37, 4302–4315. doi: 10.1002/JOC.5086
Figueroa, L. L., Maccaro, J. J., Krichilsky, E., Yanega, D., and McFrederick, Q. S. (2021). Why did the bee eat the chicken? Symbiont gain, loss, and retention in the vulture bee microbiome. MBio 12, e02317–e02321. doi: 10.1128/MBIO.02317-21/SUPPL_FILE/MBIO.02317-21-ST006.XLSX
Friedman, J., and Alm, E. J. (2012). Inferring correlation networks from genomic survey data. PLoS Comput. Biol. 8:e1002687. doi: 10.1371/JOURNAL.PCBI.1002687
Gilliam, M., Prest, D. B., and Lorenz, B. J. (1989). Microbiology of pollen and bee bread: taxonomy and enzymology of molds. Apidology 20, 53–68. doi: 10.1051/apido:19890106
Glöckner, F. O., Yilmaz, P., Quast, C., Gerken, J., Beccati, A., Ciuprina, A., et al. (2017). 25 years of serving the community with ribosomal RNA gene reference databases and tools. J. Biotechnol. 261, 169–176. doi: 10.1016/J.JBIOTEC.2017.06.1198
Goulson, D., Nicholls, E., Botías, C., and Rotheray, E. L. (2015). Bee declines driven by combined stress from parasites, pesticides, and lack of flowers. Science 80:6229. doi: 10.1126/SCIENCE.1255957/SUPPL_FILE/PAP.PDF
Graystock, P., Rehan, S. M., and McFrederick, Q. S. (2017). Hunting for healthy microbiomes: determining the core microbiomes of Ceratina, Megalopta, and Apis bees and how they associate with microbes in bee collected pollen. Conserv. Genet. 18, 701–711. doi: 10.1007/s10592-017-0937-7
Hall, M. A., Brettell, L. E., Liu, H., Nacko, S., Spooner-Hart, R., Riegler, M., et al. (2021). Temporal changes in the microbiome of stingless bee foragers following colony relocation. FEMS Microbiol. Ecol. 97:236. doi: 10.1093/FEMSEC/FIAA236
Hammer, D. A. T., Ryan, P. D., Hammer, Ø., and Harper, D. A. T. (2001). Past: paleontological statistics software package for education and data analysis. Palaeontol. Electron. 4:178.
Holm, S. N., and Skou, J. P. (1972). Studies on trapping, nesting, and rearing of some Magachile species (hymenoptera, Megachilidae) and on their parasites in Denmark. Insect Syst. Evol. 3, 169–180. doi: 10.1163/187631272X00274
Hotchkiss, M. Z., Poulain, A. J., and Forrest, J. R. K. (2022). Pesticide-induced disturbances of bee gut microbiotas. FEMS Microbiol. Rev. 46, 1–22. doi: 10.1093/FEMSRE/FUAB056
Iguchi, H., Yurimoto, H., and Sakai, Y. (2015). Interactions of methylotrophs with plants and other heterotrophic bacteria. Microorganism 3, 137–151. doi: 10.3390/MICROORGANISMS3020137
Johnson, M. D., Fokar, M., Cox, R. D., and Barnes, M. A. (2021). Airborne environmental DNA metabarcoding detects more diversity, with less sampling effort, than a traditional plant community survey. BMC Ecol. Evol. 21, 218–215. doi: 10.1186/s12862-021-01947-x
Johnson, M., Zaretskaya, I., Raytselis, Y., Merezhuk, Y., McGinnis, S., and Madden, T. (2008). NCBI BLAST: a better web interface. Nucleic Acids Res. 36, W5–W9. doi: 10.1093/NAR/GKN201
Jones, J. C., Fruciano, C., Marchant, J., Hildebrand, F., Forslund, S., Bork, P., et al. (2018). The gut microbiome is associated with behavioural task in honey bees. Insect. Soc. 65, 419–429. doi: 10.1007/S00040-018-0624-9/FIGURES/4
Kakumanu, M. L., Reeves, A. M., Anderson, T. D., Rodrigues, R. R., and Williams, M. A. (2016). Honey bee gut microbiome is altered by in-hive pesticide exposures. Front. Microbiol. 7:1255. doi: 10.3389/fmicb.2016.01255
Kapheim, K. M., Johnson, M. M., and Jolley, M. (2021). Composition and acquisition of the microbiome in solitary, ground-nesting alkali bees. Sci. Rep. 11:2993. doi: 10.1038/s41598-021-82573-x
Karra, K., Kontgis, C., Statman-Weil, Z., Mazzariello, J. C., Mathis, M., and Brumby, S. P. (2021). Global land use/land cover with Sentinel 2 and deep learning. 2021 IEEE International Geoscience and Remote Sensing Symposium IGARSS. 2021, 4704–4707. doi: 10.1109/IGARSS47720.2021.9553499
Katoh, K., and Standley, D. M. (2013). MAFFT multiple sequence alignment software version 7: improvements in performance and usability. Mol. Biol. Evol. 30, 772–780. doi: 10.1093/molbev/mst010
Kelemen, E. P., and Rehan, S. M. (2021). Opposing pressures of climate and land-use change on a native bee. Glob. Chang. Biol. 27, 1017–1026. doi: 10.1111/GCB.15468
Klinger, E. G., James, R. R., Youssef, N. N., and Welker, D. L. (2013). A multi-gene phylogeny provides additional insight into the relationships between several Ascosphaera species. J. Invertebr. Pathol. 112, 41–48. doi: 10.1016/J.JIP.2012.10.011
Kwong, W. K., Mancenido, A., and Moran, N. A. (2017a). Immune system stimulation by the native gut microbiota of honey bees. R. Soc. Open Sci. 4:170003. doi: 10.1098/rsos.170003
Kwong, W. K., Medina, L. A., Koch, H., Sing, K. W., Soh, E. J. Y., Ascher, J. S., et al. (2017b). Dynamic microbiome evolution in ocial bees. Sci. Adv. 3:e1600513. doi: 10.1126/SCIADV.1600513
Kwong, W. K., and Moran, N. A. (2016). Gut microbial communities of social bees. Nat. Rev. Microbiol. 14, 374–384. doi: 10.1038/nrmicro.2016.43
Land Information Ontario (2019). Ontario Land Cover Compilation v.2.0. Available at: https://www.arcgis.com/home/item.html?id=7aa998fdf100434da27a41f1c637382c (Accessed December 6, 2021).
Legendre, P., and Gallagher, E. D. (2001). Ecologically meaningful transformations for ordination of species data. Oecologia 129, 271–280. doi: 10.1007/S004420100716
Leonhardt, S. D., and Kaltenpoth, M. (2014). Microbial communities of three sympatric Australian stingless bee species. PLoS One 9:e105718. Available at:. doi: 10.1371/journal.pone.0105718
Li, L., Solvi, C., Zhang, F., Qi, Z., Chittka, L., and Zhao, W. (2021). Gut microbiome drives individual memory variation in bumblebees. Nat. Commun. 12, 6588–6510. doi: 10.1038/s41467-021-26833-4
Manirajan, B. A., Maisinger, C., Ratering, S., Rusch, V., Schwiertz, A., Cardinale, M., et al. (2018). Diversity, specificity, co-occurrence and hub taxa of the bacterial–fungal pollen microbiome. FEMS Microbiol. Ecol. 94:112. doi: 10.1093/FEMSEC/FIY112
Martinson, V. G., Danforth, B. N., Minckley, R. L., Rueppell, O., Tingek, S., and Moran, N. A. (2011). A simple and distinctive microbiota associated with honey bees and bumble bees. Mol. Ecol. 20, 619–628. doi: 10.1111/J.1365-294X.2010.04959.X
Martinson, V. G., Moy, J., and Moran, N. A. (2012). Establishment of characteristic gut bacteria during development of the honeybee worker. Appl. Environ. Microbiol. 78, 2830–2840. doi: 10.1128/AEM.07810-11
McDonald, D., Clemente, J. C., Kuczynski, J., Rideout, J. R., Stombaugh, J., Wendel, D., et al. (2012). The biological observation matrix (BIOM) format or: how I learned to stop worrying and love the ome-ome. Gigascience 1:7. doi: 10.1186/2047-217X-1-7
McFrederick, Q. S., and Rehan, S. M. (2016). Characterization of pollen and bacterial community composition in brood provisions of a small carpenter bee. Mol. Ecol. 25, 2302–2311. doi: 10.1111/mec.13608
McFrederick, Q. S., and Rehan, S. M. (2019). Wild bee pollen usage and microbial communities co-vary across landscapes. Microb. Ecol. 77, 513–522. doi: 10.1007/s00248-018-1232-y
McFrederick, Q. S., Wcislo, W. T., Hout, M. C., and Mueller, U. G. (2014). Host species and developmental stage, but not host social structure, affects bacterial community structure in socially polymorphic bees. FEMS Microbiol. Ecol. 88, 398–406. doi: 10.1111/1574-6941.12302
McFrederick, Q. S., Wcislo, W. T., Taylor, D. R., Ishak, H. D., Dowd, S. E., and Mueller, U. G. (2012). Environment or kin: whence do bees obtain acidophilic bacteria? Mol. Ecol. 21, 1754–1768. doi: 10.1111/J.1365-294X.2012.05496.X
McMurdie, P. J., and Holmes, S. (2013). Phyloseq: an R package for reproducible interactive analysis and graphics of microbiome census data. PLoS One 8:e61217. doi: 10.1371/JOURNAL.PONE.0061217
Mockler, B. K., Kwong, W. K., Moran, N. A., and Koch, H. (2018). Microbiome structure influences infection by the parasite Crithidia bombi in bumble bees. Appl. Environ. Microbiol. 84:e02335-17. doi: 10.1128/AEM.02335-17
Mohr, K. I., and Tebbe, C. C. (2006). Diversity and phylotype consistency of bacteria in the guts of three bee species (Apoidea) at an oilseed rape field. Environ. Microbiol. 8, 258–272. doi: 10.1111/j.1462-2920.2005.00893.x
Motta, E. V. S., Raymann, K., and Moran, N. A. (2018). Glyphosate perturbs the gut microbiota of honey bees. Proc. Natl. Acad. Sci. U. S. A. 115, 10305–10310. doi: 10.1073/PNAS.1803880115/SUPPL_FILE/PNAS.1803880115.SAPP.PDF
Muñoz-Colmenero, M., Baroja-Careaga, I., Kovačić, M., Filipi, J., Puškadija, Z., Kezić, N., et al. (2020). Differences in honey bee bacterial diversity and composition in agricultural and pristine environments – a field study. Apidologie 51, 1018–1037. doi: 10.1007/s13592-020-00779-w
Nguyen, P. N., and Rehan, S. M. (2022). Developmental microbiome of the small carpenter bee Ceratina calcarata. Environ. DNA. 4, 808–819. doi: 10.1002/EDN3.291
Oksanen, J., Blanchet, F. G., Friendly, M., Kindt, R., Legendre, P., McGlinn, D., et al. (2020). Vegan: community ecology package. R package version 2, 5–7. Available at: https://cran.r-project.org/package=vegan (Accessed November 1, 2022).
Packer, L., Genaro, J. A., and Sheffield, C. S. (2007). The bee genera of eastern Canada. Can. J. Arthropod Identif. 3, 1–32. doi: 10.3752/cjai.2007.03
Paludo, C. R., Menezes, C., Silva-Junior, E. A., Vollet-Neto, A., Andrade-Dominguez, A., Pishchany, G., et al. (2018). Stingless bee larvae require fungal steroid to pupate. Sci. Rep. 8, 1–10. doi: 10.1038/s41598-018-19583-9
Pedregosa, F., Varoquaux, G., Gramfort, A., Michel, V., Thirion, B., Grisel, O., et al. (2011). Scikit-learn: machine learning in python. J. Mach. Learn. Res. 12, 2825–2830.
Pisa, L. W., Amaral-Rogers, V., Belzunces, L. P., Bonmatin, J. M., Downs, C. A., Goulson, D., et al. (2014). Effects of neonicotinoids and fipronil on non-target invertebrates. Environ. Sci. Pollut. Res. 22, 68–102. doi: 10.1007/S11356-014-3471-X/TABLES/7
Pozo, M. I., Van Kemenade, G., Van Oystaeyen, A., Aledón-Catalá, T., Benavente, A., Van Den Ende, W., et al. (2020). The impact of yeast presence in nectar on bumble bee behavior and fitness. Ecol. Monogr. 90:e01393. doi: 10.1002/ECM.1393
Prendergast, K. S., Dixon, K. W., and Bateman, P. W. (2022). A global review of determinants of native bee assemblages in urbanised landscapes. Insect Conserv. Divers. 15, 385–405. doi: 10.1111/ICAD.12569
Price, M. N., Dehal, P. S., and Arkin, A. P. (2010). FastTree 2--approximately maximum-likelihood trees for large alignments. PLoS One 5:e9490. doi: 10.1371/journal.pone.0009490
R Core Team (2019). R: a language and environment for statistical computing. Available at: https://www.r-project.org/.
Raymann, K., and Moran, N. A. (2018). The role of the gut microbiome in health and disease of adult honey bee workers. Curr. Opin. Insect Sci. 26, 97–104. doi: 10.1016/J.COIS.2018.02.012
Rehan, S. M., and Richards, M. H. (2010). Nesting biology and subsociality in Ceratina calcarata (hymenoptera: Apidae). Entomol. Soc. Canada 142, 65–74. doi: 10.4039/n09-056
Rering, C. C., Rudolph, A. B., and Beck, J. J. (2021). Pollen and yeast change nectar aroma and nutritional content alone and together, but honey bee foraging reflects only the avoidance of yeast. Environ. Microbiol. 23, 4141–4150. doi: 10.1111/1462-2920.15528
Rinner, C., and Ussain, M. (2011). Toronto’s urban heat island-exploring the relationship between land use and surface temperature. Remote Sens. 3, 1251–1265. doi: 10.3390/rs3061251
Rothman, J. A., Leger, L., Graystock, P., Russell, K., and McFrederick, Q. S. (2019). The bumble bee microbiome increases survival of bees exposed to selenate toxicity. Environ. Microbiol. 21, 3417–3429. doi: 10.1111/1462-2920.14641
Rothman, J. A., Russel, K. A., Leger, L., McFrederick, Q. S., and Graystock, P. (2020). The direct and indirect effects of environmental toxicants on the health of bumblees and their microbiomes. Proc. R. Soc. B 287:20200980. doi: 10.1098/rspb.2020.0980
Rubanov, A., Russell, K. A., Rothman, J. A., Nieh, J. C., and McFrederick, Q. S. (2019). Intensity of Nosema ceranae infection is associated with specific honey bee gut bacteria and weakly associated with gut microbiome structure. Sci. Rep. 9, 3820–3828. doi: 10.1038/s41598-019-40347-6
Russell, R. J., Scott, C., Jackson, C. J., Pandey, R., Pandey, G., Taylor, M. C., et al. (2011). The evolution of new enzyme function: lessons from xenobiotic metabolizing bacteria versus insecticide-resistant insects. Evol. Appl. 4, 225–248. doi: 10.1111/J.1752-4571.2010.00175.X
Shell, W. A., and Rehan, S. M. (2016). Recent and rapid diversification of the small carpenter bees in eastern North America. Biol. J. Linn. Soc. 117, 633–645. doi: 10.1111/BIJ.12692
Shell, W. A., and Rehan, S. M. (2022). Comparative metagenomics reveals expanded insights into intra-and interspecific variation among wild bee microbiomes. Commun. Biol. 5:603. doi: 10.1038/s42003-022-03535-1
Smith, E. A., and Newton, I. L. G. (2020). Genomic signatures of honey bee association in an acetic acid symbiont. Genome Biol. Evol. 12, 1882–1894. doi: 10.1093/GBE/EVAA183
Steensen, B. M., Marelle, L., Hodnebrog, Ø., and Myhre, G. (2022). Future urban heat island influence on precipitation. Clim. Dyn. 58, 3393–3403. doi: 10.1007/s00382-021-06105-z
Su, Q., Wang, Q., Mu, X., Chen, H., Meng, Y., Zhang, X., et al. (2021). Strain-level analysis reveals the vertical microbial transmission during the life cycle of bumblebee. Microbiome 9, 1–14. doi: 10.1186/S40168-021-01163-1/FIGURES/5
Tarpy, D. R., Mattila, H. R., and Newton, I. L. G. (2015). Development of the honey bee gut microbiome throughout the queen-rearing process. Appl. Environ. Microbiol. 81, 3182–3191. doi: 10.1128/AEM.00307-15
Tlais, A. Z. A., Polo, A., Filannino, P., Cantatore, V., Gobbetti, M., and Di Cagno, R. (2022). Biofilm formation as an extra gear for Apilactobacillus kunkeei to counter the threat of agrochemicals in honeybee crop. Microb. Biotechnol. 15, 2160–2175. doi: 10.1111/1751-7915.14051
Turnbaugh, P. J., and Gordon, J. I. (2009). The core gut microbiome, energy balance and obesity. J. Physiol. 587, 4153–4158. doi: 10.1113/JPHYSIOL.2009.174136
Vásquez, A., Forsgren, E., Fries, I., Paxton, R. J., Flaberg, E., Szekely, L., et al. (2012). Symbionts as major modulators of insect health: lactic acid bacteria and honeybees. PLoS One 7:e33188. doi: 10.1371/JOURNAL.PONE.0033188
Voulgari-Kokota, A., Grimmer, G., Steffan-Dewenter, I., and Keller, A. (2019). Bacterial community structure and succession in nests of two megachilid bee genera. FEMS Microbiol. Ecol. 95:218. doi: 10.1093/FEMSEC/FIY218
Voulgari-Kokota, A., Steffan-Dewenter, I., and Keller, A. (2020). Susceptibility of red mason bee larvae to bacterial threats due to microbiome exchange with imported pollen provisions. Insects 11:373. doi: 10.3390/insects11060373
Wang, H., Cheng, M., Dsouza, M., Weisenhorn, P., Zheng, T., and Gilbert, J. A. (2018). Soil bacterial diversity is associated with human population density in urban greenspaces. Environ. Sci. Technol. 52, 5115–5124. doi: 10.1021/ACS.EST.7B06417/ASSET/IMAGES/LARGE/ES-2017-06417U_0005.JPEG
Weiss, S., Xu, Z. Z., Peddada, S., Amir, A., Bittinger, K., Gonzalez, A., et al. (2017). Normalization and microbial differential abundance strategies depend upon data characteristics. Microbiome 5:27. doi: 10.1186/s40168-017-0237-y
Wilson, C. J., and Jamieson, M. A. (2019). The effects of urbanization on bee communities depends on floral resource availability and bee functional traits. PLoS One 14:e0225852. doi: 10.1371/JOURNAL.PONE.0225852
Wright, S. A. I., Zumoff, C. H., Schneider, L., and Beer, S. V. (2001). Pantoea agglomerans strain-EH318 produces two antibiotics that inhibit Erwinia amylovora in vitro. Appl. Environ. Microbiol. 67, 284–292. doi: 10.1128/AEM.67.1.284-292.2001
Wynns, A. A., Jensen, A. B., and Eilenberg, J. (2013). Ascosphaera callicarpa, a new species of bee-loving fungus, with a key to the genus for Europe. PLoS One 8:e73419. doi: 10.1371/JOURNAL.PONE.0073419
Yilmaz, P., Parfrey, L. W., Yarza, P., Gerken, J., Pruesse, E., Quast, C., et al. (2014). The SILVA and “all-species living tree project (LTP)” taxonomic frameworks. Nucleic Acids Res. 42, D643–D648. doi: 10.1093/nar/gkt1209
Yoder, J. A., Jajack, A. J., Rosselot, A. E., Smith, T. J., Yerke, M. C., and Sammataro, D. (2013). Fungicide contamination reduces beneficial fungi in bee bread based on an area-wide field study in honey bee, Apis mellifera, colonies. J. Toxicol. Environ. Health 76, 587–600. doi: 10.1080/15287394.2013.798846
Keywords: bacteria, fungi, urbanization, microbial diversity, land use, Apidae, Ceratina
Citation: Nguyen PN and Rehan SM (2022) The effects of urban land use gradients on wild bee microbiomes. Front. Microbiol. 13:992660. doi: 10.3389/fmicb.2022.992660
Edited by:
Hao Zheng, China Agricultural University, ChinaReviewed by:
Jo-anne Holley, University of Texas at Austin, United StatesAlan Emanuel Silva Cerqueira, Universidade Federal de Viçosa, Brazil
Copyright © 2022 Nguyen and Rehan. This is an open-access article distributed under the terms of the Creative Commons Attribution License (CC BY). The use, distribution or reproduction in other forums is permitted, provided the original author(s) and the copyright owner(s) are credited and that the original publication in this journal is cited, in accordance with accepted academic practice. No use, distribution or reproduction is permitted which does not comply with these terms.
*Correspondence: Sandra M. Rehan, c2FuZHJhLnJlaGFuQGdtYWlsLmNvbQ==