- 1Guangdong Provincial Key Laboratory of Zoonosis Prevention and Control, College of Veterinary Medicine, South China Agricultural University, Guangzhou, China
- 2Maoming Branch, Guangdong Laboratory for Lingnan Modern Agriculture, Guangzhou, China
- 3Key Laboratory of Animal Vaccine Development, Ministry of Agriculture and Rural Affairs, Guangzhou, China
- 4National Engineering Research Center for Breeding Swine Industry, South China Agricultural University, Guangzhou, China
- 5Guangdong Animal Health and Quarantine Office, Guangdong Animal Disease Prevention and Control Center, Guangzhou, China
Senecavirus A (SVA) is a member of the genus Senecavirus in the family Picornaviridae that infects pigs and shows symptoms similar to foot and mouth diseases and other vesicular diseases. It is difficult to prevent, thus, causing tremendous economic loss to the pig industry. However, the global transmission routes of SVA and its natural origins remain unclear. In this study, we processed representative SVA sequences from the GenBank database along with 10 newly isolated SVA strains from the field samples collected from our lab to explore the origins, population characteristics, and transmission patterns of SVA. The SVA strains were firstly systematically divided into eight clades including Clade I–VII and Clade Ancestor based on the maximum likelihood phylogenetic inference. Phylogeographic and phylodynamics analysis within the Bayesian statistical framework revealed that SVA originated in the United States in the 1980s and afterward spread to different countries and regions. Our analysis of viral transmission routes also revealed its historical spread from the United States and the risk of the global virus prevalence. Overall, our study provided a comprehensive assessment of the phylogenetic characteristics, origins, history, and geographical evolution of SVA on a global scale, unlocking insights into developing efficient disease management strategies.
Introduction
Senecavirus A (SVA), also named Seneca Valley virus (SVV), is a non-enveloped, single-stranded, positive-sense RNA virus, that is the only member of the genus Senecavirus under the family Picornaviridae. SVA has a linear RNA genome of approximately 7.3 kb enclosed in an icosahedral capsid and the diameter of its viral particle is around 30 nm (Hales et al., 2008; Vannucci et al., 2015; Adams et al., 2016). Similar to other picornaviruses, the genome of SVA encodes an individual open reading frame (ORF) and the single ORF encodes a polyprotein precursor with the representative “L + 4 + 3 + 4” pattern of the Picornaviridae. This polyprotein is subsequently cleaved into the L (leader protein), P1 (VP4, VP2, VP3, VP1), P2 (2A, 2B, 2C), and P3 (3A, 3B, 3C, 3D) proteins (Sun et al., 2016). Representative clinical symptoms in pigs caused by SVA include high mortality in neonatal piglets (40–80% in 0–3-day-old piglets and 0–30% in 4–7-day-old piglets), skin ulceration, and vesicular lesions especially on the feet, which are quite difficult to distinguish from other vesicular diseases including foot and mouth disease and swine vesicular stomatitis (Vannucci et al., 2015; Oliveira et al., 2017). Besides pigs, cows may also serve as natural hosts of SVA and asymptomatic SVA-positive cases are emerging, making it more difficult to control the spread of SVA (Zhang et al., 2019).
SVA (SVA-001) was first recognized as a cell culture contaminant in 2002 and the first full-length SVA genome was completed in 2008 (Hales et al., 2008; Guo et al., 2020). The identification of archived virus samples of pigs with diverse clinical manifestations revealed that SVA first emerged in 1988 in the United States (Wang et al., 2016). However, there was subsequent a series of SVA outbreaks that occurred within several months beginning in late 2014, which were estimated to have impacted 80% of all pig farms in Brazil (Leme et al., 2015, 2019a,2019b; Vannucci et al., 2015). Furthermore, over 200 cases of SVA were reported in the United States Midwest from 2015 to 2017 (Zhang et al., 2015; Leme et al., 2019a,b). Meanwhile, SVA was first reported in Guangdong Province in 2015 and following isolations from clinical samples, cases of SVA have been reported nationwide since then (Qian et al., 2016; Wu et al., 2017; Chen et al., 2018; Wang et al., 2018; Zhang et al., 2019). To date, over half of Chinese provinces, autonomous regions, and municipalities (P.A.M.s) have been reported with SVA isolation (Liu et al., 2020). However, information related to SVA epidemiology in recent years has remained limited.
A few phylogenetic analyses of SVA clustering based on locations were conducted previously, yet there is still a lack of a well-systematic assortment of SVA. Additionally, the global dispersal of SVA and its origins remain still unclear. The Bayesian systematic dynamics and systematic geographics are powerful approaches for the analysis of RNA virus evolution and transmission process (Drummond et al., 2005; Drummond and Suchard, 2008; Victoria et al., 2009; Shi et al., 2010; Jin et al., 2014). Thus, in this study, the ORF sequences of SVA strains from field specimens collected by our lab and those obtained from the National Center for Biotechnology Information (NCBI) GenBank database were compiled for the purpose of a systematic SVA classification. Furthermore, the origins, transmission routes, and phylodynamics of SVA worldwide were explored with the assistance of the Bayesian dynamic and phylogeographic frameworks, to provide a more comprehensive understanding of SVA epidemiology worldwide.
Materials and methods
Ethics statement
All experimental protocols processed in this study were established in accordance with the guidelines of the Institutional Animal Care and Use Committee (IACUC, SCAU-AEC-2022A010) and approved by the Animal Ethics Committee of South China Agricultural University.
Sample collection and sequencing
SVA isolation was carried out with BHK-21 cell line (stored in our lab) using Dulbecco‘s modified eagle medium (Gibco, #C11995-500CP) with 2% fetal bovine serum (Biological Industries, 04-001-1ACS) and 1% Penicillin-Streptomycin (New Cell and Molecular Biotech Co., Ltd. #C100C5) (Liu et al., 2019). Ten distinct SVA strains were successfully isolated from field samples, including from vesicular liquid and lymph nodes obtained from suspected SVA-positive pigs (Table 1). Samples were collected from 574 specimens collected from 2018 to 2021 and were processed for RNA extraction with RNAzol (Molecular Research Center, United States). Complementary DNA of SVA was acquired by reverse transcription (StarScript II MMLV, GeneStar, Beijing, China) and was amplified with polymerase chain reaction (PCR) with three pairs of self-designed primers (Table 2). The PCR amplicons were cloned into the empty pMD-18T vector followed by sequencing. All nucleotide acid and amino acid sequences were aligned, and the similarities were analyzed with MegAlign (DNAStar v7.0) (Table 3).
Preliminary phylogenetic analysis
All SVA sequences (n = 250) were obtained from the NCBI GenBank database (by December 2021) and field samples collected by our lab, and strains of 100% identity and also reported from a similar date/place of collection were excluded by CD-HIT (Li and Godzik, 2006). The ORF sequences were manually trimmed using MEGA 11 containing all indels/substitutions referred to several representative SVA isolates (including the initial SVV-001 (NC011349.1), Canadian strain CAN/07-503297/2007 (MN233023.1), Thailand strain G27_SV_2/2016/Thailand (MF416218.1), China strain GD-S1/2018 (MK802892.1), etc.). All sequences were aligned via MAFFT v7.313 using default parameters (Katoh et al., 2002). The maximum likelihood (ML) tree was applied to the single ORF sequences using IQ-TREE v1.6.12 with the best-fit nucleotide substitution model of GTR + F + I + G4 and 1,000 bootstrap replicates (Nguyen et al., 2015; Rambaut et al., 2020; Kim et al., 2021). MAFFT, IQ-TREE, and related tools were integrated within PhyloSuite (Zhang et al., 2020).
Time signal detection and evolutionary dynamics
To examine the time signals of the sequences after excluding recombinant strains using RDP4, Root-To-Tip (RTT) regression was employed using TempEst v1.5.3 (Martin et al., 2015; Rambaut et al., 2016). To eliminate the sample bias of global geographical analysis, we also excluded the strains from 2019 to 2021 since they were all extended from Chinese native clusters (Sun et al., 2019). Finally, the phylodynamic and phylogeographic analyses of the SVA sequences (n = 170) were conducted using BEAST v1.10.4 with the best nucleotide substitution model of GTR + I + G (Lanave et al., 1984; Zhang et al., 2020). The combination of best model performance was assessed through Marginal Likelihood Estimation using the path sampling/stepping-stone sampling (PS/SS) approaches in BEAUti (Table 4; Baele et al., 2012). The chain length (200,000,000) and log parameters (20,000) were set to assess the final effective sampling size (ESS) monitoring using Tracer v1.7.1 (Rambaut et al., 2018, 2020). The maximum clade credibility tree (MCC tree) was constructed through Tree Annotator v1.10.4 under the burn-in (10% of samples) followed by visualization, annotation, and elaboration using FigTree v1.4.4 (Rambaut, 2019).
Systematic Bayesian geographic reconstruction
Bayesian phylogeographic analyses of the sequences were conducted by assessing geographical information using BEAST v1.10.4 (Lemey et al., 2009; Sun et al., 2019). The Bayes Factor (BF) was calculated and visualized by comparing the posterior and prior probabilities that the individual rates were non-zero via spreaD3 v0.9.7.1 (Bielejec et al., 2016). The transmission routes for which BF is greater than 3 were considered to have well-supported diffusion rates, indicating well-supported migration routes between different geographical areas that constituted the SVA migration schematic diagram.
Results
Genomic and preliminary phylogenetic analysis
To obtain the full information and deepen our understanding of SVA, a total of 250 SVA sequences were collected from the NCBI GenBank database and field samples from our lab, and the ML phylogenetic tree was constructed after sequence alignment and pairwise comparison (Figure 1 and Table 1). Global phylogenetic analysis revealed that all representative SVA strains could be divided into eight clades: the Clade I–VII and the Clade Ancestor. The Clade Ancestor was the most initial SVA isolates and the Clade I–VII represented the circulating isolates in recent years. It could be found that Clade I–VII exhibited sequence identities in the same range (96.05–98.63%) while the Clade Ancestor displayed greater divergence (92.71–93.88%). Among Clade I–VII, Clade V showed greater divergence compared with other clades (Table 3). Furthermore, isolates from the United States were present in all clades except Clade II and IV. In China, SVA strains have been reported to have dispersed into six clades including Clade I–VI. In addition, SVA isolated from our lab were located in Clade II, V, and VI, indicating that SVA was diversified in China over recent years. Meanwhile, SVA reported from Brazil and Colombia were located in Clade III. SVA strains from Canada showed up in Clade V, and Clade V and IV SVA strains appeared in Thailand and Vietnam (Figure 1). Our findings suggested that each clade, from Clade I to VII, represented a constant genetic evolution and divergence.
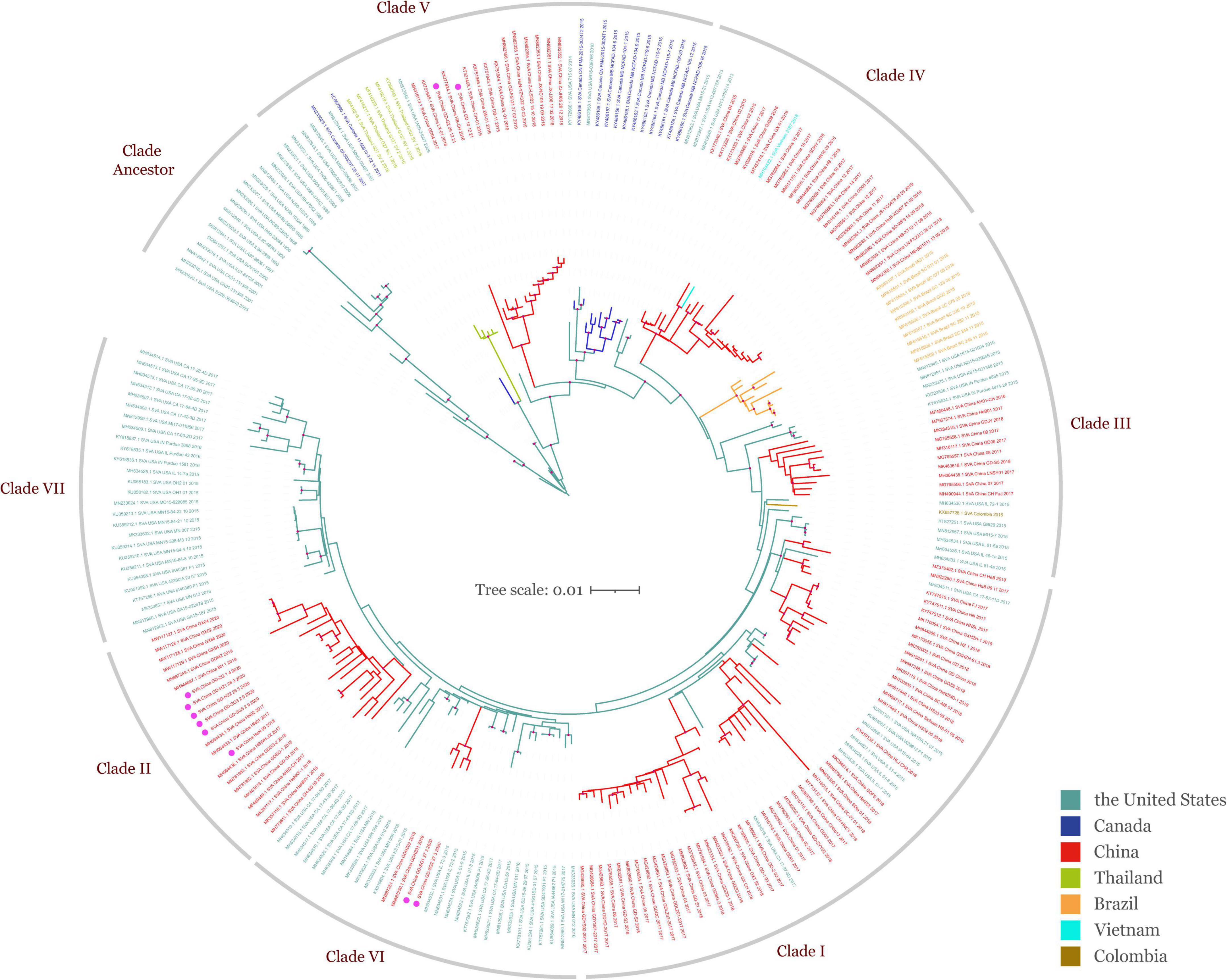
Figure 1. Global ML phylogeny of all publicly available SVA strains and SVA isolated in our lab based on the ORF gene. The GTR + F + I + G4 nucleotide substitution model was used for phylogenetic tree construction. All SVA strains (n = 250) from different countries are grouped by color and were clustered into eight clades including Clade Ancestor and Clade I–VII. The scale bar represents the number of substitutions per site along the branch in the tree topology. Light mauve dots on the tree topology indicate the bootstrap value of 100. Isolates obtained from field specimens are marked with magenta dots.
Evolutionary and population dynamics
The time signal was determined by the double verification of the RTT methods (Figure 2A). The RTT correlation coefficient was 0.9518 and the coefficient of determination (R2-value) was 0.9059, indicating that the time signal was of good quality. The uncorrelated lognormal relaxed molecular clock model and the Bayesian Skyline model were the most suitable models for this study after comparing different model combinations (Table 4). The topology of the MCC tree was relatively consistent with the ML tree. From the MCC tree, the United States was estimated to be the original reservoir of SVA. Furthermore, epidemic clusters of SVA developed in Thailand and Brazil developed (Figure 2B). Evolutionary analysis can provide a clear picture that the original emergence of SVA was September 1985 with the 95% highest probability density (HPD) from July 1983 to April 1987, which is around but earlier than the earliest described American case from the archived sample known of 1988. Furthermore, according to the MCC tree, the first presence of SVA in Canada, Thailand, and China was in around 2004, 2007, and 2010, respectively. The emerging time of the main clades circulating in China and the United States were as follows: Clade I: September 2012 [95% HPD (November 2011, June 2013)], Clade VI: February 2013 [95% HPD (June 2012, August 2013)], Clade VII: January 2014 [95% HPD (May 2014, September 2013)]. The average evolution rate of SVA was subsequently calculated and was inferred as 3.93 × 10–3 substitutions per nucleotide site per year (s/n/y) with the 95% HPD range from 3.27 × 10–3 to 4.68 × 10–3 s/n/y.
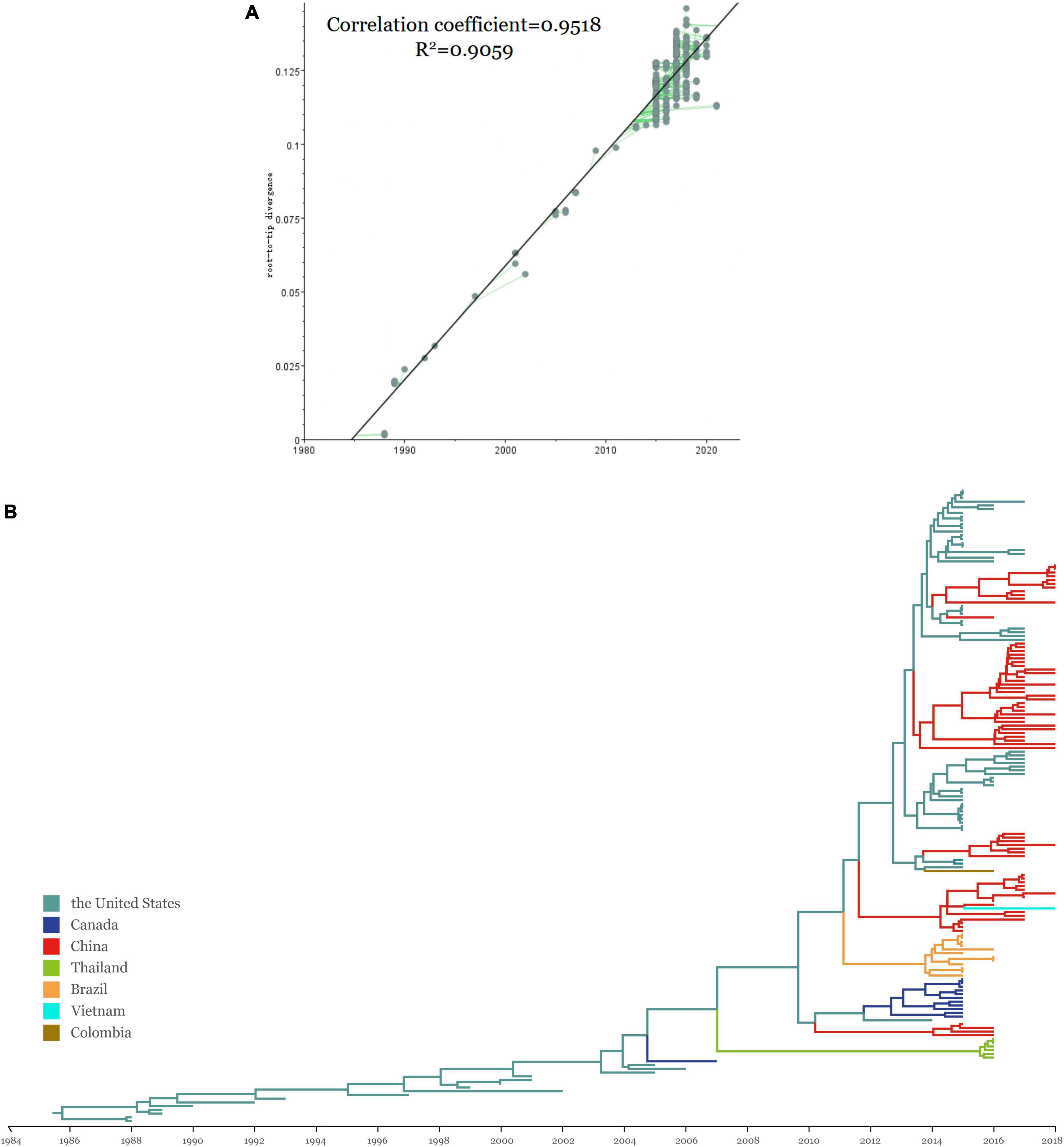
Figure 2. (A) Regression of the RTT genetic distance against the sampling time for evaluation of the molecular clock models using TempEst v1.5.3. Parameters including correlation coefficient and R2 are shown beside the linear regression. (B) The MCC tree of SVA strains using the ORF gene with BEAST v1.10.4 and FigTree v1.4.4. Colors represent different sampling locations, namely the United States (teal), China (red), Canada (blue), Colombia (tan) and Brazil (orange), Thailand (green), and Vietnam (purple).
Bayesian skyline plot coalescent model was processed to reconstruct the history of the effective population size and showed its temporal shifts (Figure 3; Drummond et al., 2005). Briefly, after a stably increasing period, the population size underwent two consequent rounds of spike periods since 2015. The effective population size showed an overall augmentation since the 1980s. Overall, the findings of sequence-based on the MCC tree were consistent with the results of epidemiological investigations into the timing of the initial emergence of SVA.
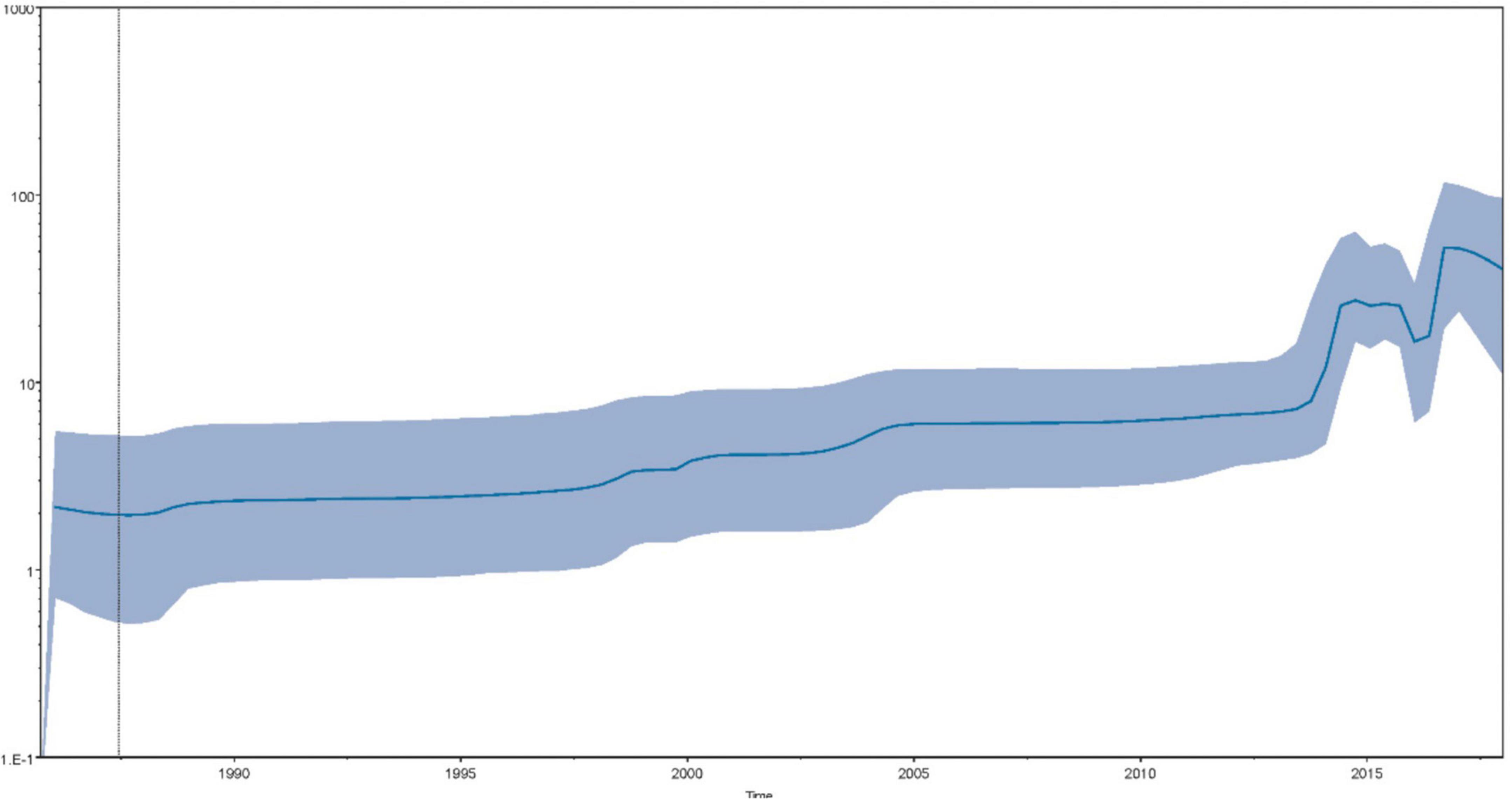
Figure 3. The Bayesian skyline plot depicts the temporal variation of the effective population size of SVA. The x-axis shows the time (year) and the y-axis indicates the product of the generation length in years and the effective population size. The dark blue solid line represents the median of the effective population size and the light blue shaded area indicates the 95% HPD.
Phylogeography and geographic circulation
The historical distribution of SVA was determined and visualized via global dynamism through maps. Conforming with the time points of the dispersal patterns, the United States was the origin of SVA, which then SVA was spread outwards to Canada in the early 2000s. Transmission to South America, China, and Southeast Asia then began in the 2010s. Since 2015, the United States and China have maintained a relatively large SVA population size, and they have also become the main SVA epidemic areas (Figure 4A).
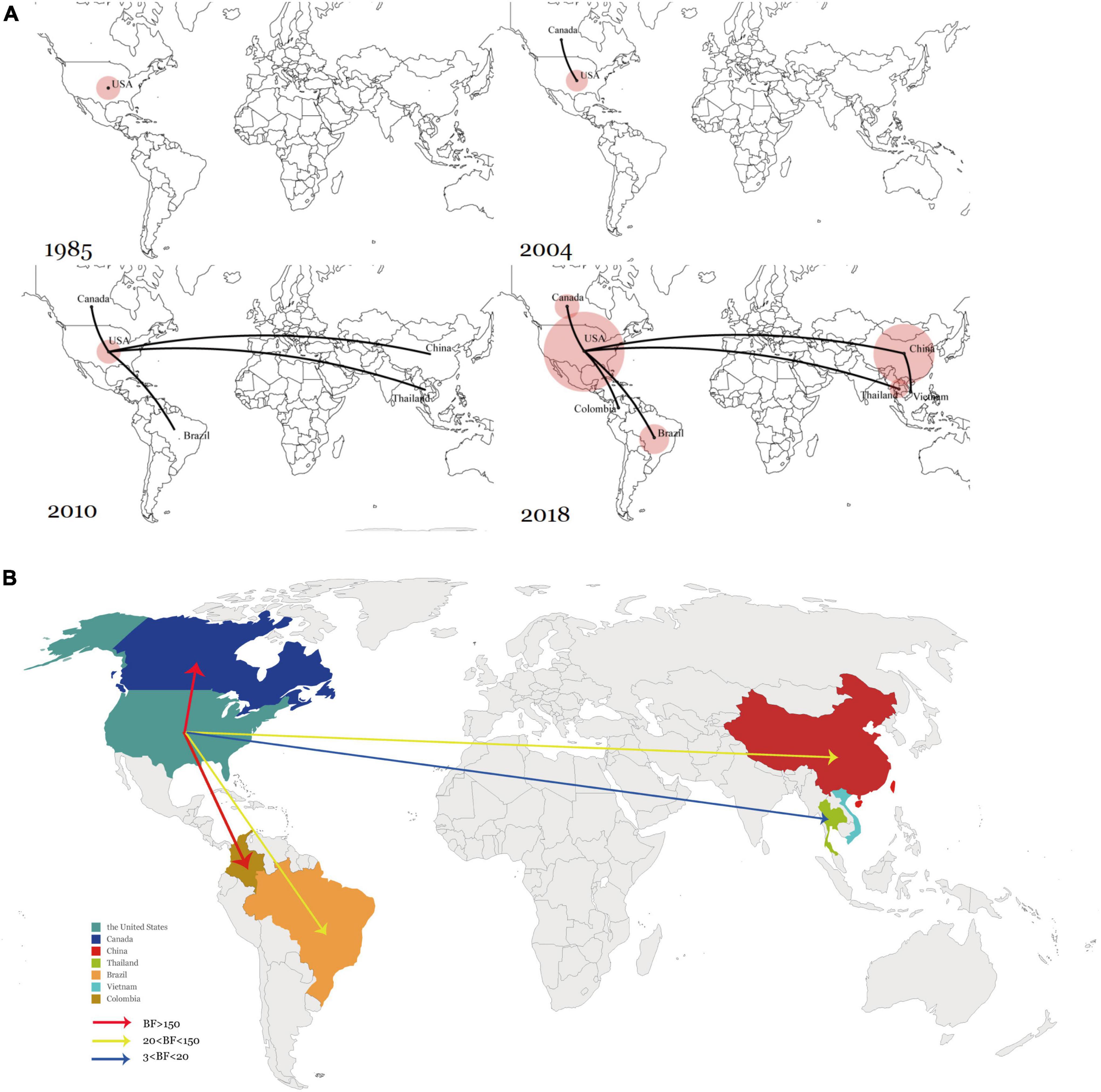
Figure 4. (A) Spatiotemporal dynamics of SVA among different regions. (B) Global transmission links of SVA tested by Bayes Factor for significant non-zero rates supported by the BSSVS. Line colors represent the relative strength by which the rates are supported: very strong (BF > 150, red) and strong (20 < BF < 150, yellow), and positive (BF > 3). Line directions indicate the direction of transmission.
To verify the inferred viral transmission routes, Bayesian Stochastic Search Variable Selection (BSSVS) was used to identify the most parsimonious descriptions of phylogenetic dispersal processes. The asymmetrical substitution model allowed for the BF value (> 3) determined among seven countries that had claimed SVA circulation. In summary, five transition events between discrete geographical locations were statistically well supported. Among the five transmission routes, SVA from the United States to Canada and Colombia were very strongly supported with a BF > 150 while the routes between the United States to Brazil and China exhibited 20 < BF < 150. In addition, transmission between the United States and Thailand was also statistically supported with 3 < BF < 20. Overall, the results showed that the United States had acted as the main source of SVA, and the transmission from the United States to Canada, Brazil, Colombia, China, and Thailand, was well supported by the BF values (Figure 4B).
Discussion
Several SVA epidemics have occurred since 2015, following the initial appearances of sporadic cases. Consequently, the global swine industries have suffered tremendous losses, while new viral clades have emerged (Leme et al., 2015; Vannucci et al., 2015; Qian et al., 2016; Wu et al., 2017; Saeng-Chuto et al., 2018; Wang et al., 2018). Genomic differences gradually emerged with the continuous spread and evolution of SVA. Previously constructed phylogenies of SVA have classified SVA based on the VP1 gene, and SVA has been reported to cluster in the US-like and Canadian-like clades by location of isolation (Chen et al., 2018; Zhang et al., 2021). Moreover, another earlier phylogenetic analysis indicated that the SVA strains isolated from South China could be clustered into five distinct groups based on the VP1, 3C, and 3D genes (Sun et al., 2018; Wang et al., 2019; Liu et al., 2020). In addition, a recent paper in 2021 mentioned SVA could be clustered into two groups containing two subgroups, respectively (Jiang et al., 2021). To clarify the phylogeny of SVA, we performed an ML tree construction based on the ORF gene in this study. The ML tree was constructed using a comprehensive dataset from GenBank and strains isolated from samples collected by our lab over the last 5 years. According to the topology of the ML tree, the representative SVA strains were divided into eight clades including the Clade Ancestor representing the very first SVA cases, and Clade I–VII representing the isolates that have been circulating since the mid-2010s. The initial sequencings of SVA archived samples and outbreaks were limited by their small magnitudes. However, a much larger population size and duration of SVA with distinct epidemiological patterns were detected due to the recent outbreaks and this virus has received more attention since the 2010s. Based on our field samples of SVA-positive, the strains of SVA currently circulating in China belong to Clade II, V, and VI. The co-occurrences of United States/Brazilian in Clade III and United States/Chinese strains in Clade I were also detected. These findings suggested that SVA has diversified among different countries, thereby increasing the difficulty of controlling SVA on a global scale. More importantly, this phylogeny study utilized global SVA strains in this study rather than limited data. As the first to systematically investigate all available SVA strains into distinct clades, this study could act as a good reference in future SVA epidemiological studies.
Bayesian phylodynamic models have rarely been used to facilitate disease prevention or to control pathogens in the pig industry (Alkhamis et al., 2016). In this study, the Bayesian algorithm system was processed to improve our understanding of the global history of SVA epidemics since the employment of the system in the RNA virus control such as COVID-19 pandemic management (Sun et al., 2019; Lemey et al., 2020; Li et al., 2020; Ades et al., 2021; Kline et al., 2021; Yiannoutsos et al., 2021). From the results of phylodynamic analysis, the average evolution rate of SVA was slightly lower than previously reported, which might be attributed to the different datasets analyzed (Xu et al., 2017). Meanwhile, the viral population dynamics can be seen from the Bayesian skyline plot. Since 2015, the population of SVA increased rapidly. In 2015, SVA outbreaks began being frequently reported worldwide, including in Canada, China, Brazil, Colombia, etc. The stable SVA population size suddenly increased again in 2017. Furthermore, as the prevalence and re-emergence of SVA have increased worldwide, the monitoring of SVA was being intensified, with larger volumes of samples being screened and the SVA diagnosis optimized. A large number of strains were isolated since then, which could be a reason why the size of the SVA population quickly increased (Hause et al., 2016; Joshi et al., 2016, 2020; Houston et al., 2019; Liu et al., 2020; Wang et al., 2020). Furthermore, the Bayesian systematic analysis used in this study can be employed as a real-time early monitoring system of SVA, thus allowing the pig industry and government agencies to make reasonable decisions in a timely manner.
The main countries that reported SVA was primarily reported from the United States and Canada in 2014. During this period, only one complete strain of the virus was detected in Canada, which may have affected the structural distribution of the early branches of the MCC tree to a certain extent (Leme et al., 2017). In addition, the population conditions were unclear prior to the mid-2010s, due to a lack of sampling and limited SVA surveillance. Consequently, SVA exhibited little genetic diversity by 2014. However, with its continuous spreading and evolution, SVA after 2014 was clustered into seven clades (Clade I–VII). This genetic diversity could further enhance viral diffusion and increase the risk of exposure of pigs with the emergence of high virulent SVA, even rendering spill-over events. From the phylogeography analysis, we can see that the United States was estimated to be the origin of SVA, and then it spread to Canada around 2004, and then around 2010, SVA was spread from the United States to China and Brazil, respectively, even in the absence of shared borders. Besides, all cases of SVA (except in Vietnam) were identified to be imported from the United States based on the well-supported BF values, which is consistent with the frequent pork trade between these countries and the United States. Moreover, SVA in Thailand and Brazil imported and had already developed respective native clusters in their own countries.
This study has several limitations. First, the data for some countries, like Vietnam and Colombia, were insufficient enough. BEAST framework utilizes efficient MCMC chain statistical methods to combine molecular phylogenetic relationship reconstruction with complex discrete trait evolution, divergence time, and demographic models for inferring the phylodynamics of the SVA population in this study. However, the certainty of the output may be affected by a series of factors including the integrity of the sequence information and the size of sequence data. Therefore, a more in-depth analysis of the true transmission links associated with these nations remains to be supported by more comprehensive data (Lemey et al., 2009). Also, SVA transmission at a province/state level in China and in the United States would be interesting to study where about one-third isolates lack the information of place of collection (Supplementary Table 1). Relevant work can be further dug with greater data volume and more comprehensive/accurate isolates information. Furthermore, we are also concerned about the sampling bias in this section of selecting SVA strains prior to 2019 for the elimination of the sample bias. To examine the possible novel introductions into China, we re-inspected the strains isolated and reported after 2019. All newly isolated SVA strains were extended from China clusters through evolutionary relation. Thus, novel introductions to China after 2019 were neglected.
In summary, this study revealed for the first time the introduction and global transmission of SVA. Moreover, by processing the phylodynamic and phylogeography in the framework of Bayesian statistics can we determine the origin of SVA was the United States and the introduction of SVA in most countries was from the United States, which has also acted as the disease epicenter. The effective population size, genetic diversity, and the risk of SVA spreading to various countries have elevated over time. Our study provides fresh insights into the global dispersal of SVA, and a better understanding requires more consideration of SVA epidemiological research.
Data availability statement
The datasets presented in this study can be found in online repositories. The names of the repository/repositories and accession number(s) can be found below: https://www.ncbi.nlm.nih.gov/genbank/, ON868368; https://www.ncbi.nlm.nih.gov/genbank/, ON868369; https://www.ncbi.nlm.nih.gov/genbank/, ON868370; https://www.ncbi.nlm.nih.gov/genbank/, ON868371; https://www.ncbi.nlm.nih.gov/genbank/, ON868372; https://www.ncbi.nlm.nih.gov/genbank/, ON868373; https://www.ncbi.nlm.nih.gov/genbank/, ON8683 74; https://www.ncbi.nlm.nih.gov/genbank/, ON868375; https://www.ncbi.nlm.nih.gov/genbank/, ON868376; and https://www.ncbi.nlm.nih.gov/genbank/, ON868377.
Ethics statement
The animal study was reviewed and approved by the Institutional Animal Care and Use Committee (IACUC, SCAU-AEC-2022A010).
Author contributions
HG and Y-JC designed the study and processed the data. HG wrote the manuscript. J-BX, Z-YX, JL, and S-JX helped, collected, and processed the data. HG, Y-JC, and Y-KS participated in the analysis and discussion. X-QX and Y-FZ helped with sample collection and diagnosis. Y-KS and G-HZ supervised and reviewed the manuscript. All authors read and approved the final manuscript.
Funding
This research was funded by the Start-up Research Project of Maoming Laboratory (2021TDQD002) and the China Agricultural Research System of MOF and MARA (cars-35).
Conflict of interest
The authors declare that the research was conducted in the absence of any commercial or financial relationships that could be construed as a potential conflict of interest.
Publisher’s note
All claims expressed in this article are solely those of the authors and do not necessarily represent those of their affiliated organizations, or those of the publisher, the editors and the reviewers. Any product that may be evaluated in this article, or claim that may be made by its manufacturer, is not guaranteed or endorsed by the publisher.
Supplementary material
The Supplementary Material for this article can be found online at: https://www.frontiersin.org/articles/10.3389/fmicb.2022.980862/full#supplementary-material
References
Adams, M. J., Lefkowitz, E. J., King, A. M., Harrach, B., Harrison, R. L., and Knowles, N. J. (2016). Ratification vote on taxonomic proposals to the International Committee on Taxonomy of Viruses (2016). Arch. Virol. 161, 2921–2949. doi: 10.1007/s00705-016-2977-6
Ades, A. E., Soriano-Arandes, A., Alarcon, A., Bonfante, F., Thorne, C., Peckham, C. S., et al. (2021). Vertical transmission of Zika virus and its outcomes: A Bayesian synthesis of prospective studies. Lancet Infect. Dis. 21, 537–545. doi: 10.1016/S1473-3099(20)30432-1
Alkhamis, M. A., Perez, A. M., Murtaugh, M. P., Wang, X., and Morrison, R. B. (2016). Applications of Bayesian Phylodynamic Methods in a Recent U.S. Porcine Reproductive and Respiratory Syndrome Virus Outbreak. Front. Microbiol. 7:67. doi: 10.3389/fmicb.2016.00067
Baele, G., Lemey, P., Bedford, T., Rambaut, A., Suchard, M. A., and Alekseyenko, A. V. (2012). Improving the accuracy of demographic and molecular clock model comparison while accommodating phylogenetic uncertainty. Mol. Biol. Evol. 29, 2157–2167. doi: 10.1093/molbev/mss084
Bielejec, F., Baele, G., Vrancken, B., Suchard, M. A., Rambaut, A., and Lemey, P. (2016). SpreaD3: Interactive Visualization of Spatiotemporal History and Trait Evolutionary Processes. Mol. Biol. Evol. 33, 2167–2169. doi: 10.1093/molbev/msw082
Chen, P., Yang, F., Cao, W., Liu, H., Zhang, K., Liu, X., et al. (2018). The Distribution of Different Clades of Seneca Valley Viruses in Guangdong Province, China. Virol. Sin. 33, 394–401. doi: 10.1007/s12250-018-0056-8
Drummond, A. J., and Suchard, M. A. (2008). Fully Bayesian tests of neutrality using genealogical summary statistics. BMC Genet. 9:68. doi: 10.1186/1471-2156-9-68
Drummond, A. J., Rambaut, A., Shapiro, B., and Pybus, O. G. (2005). Bayesian coalescent inference of past population dynamics from molecular sequences. Mol. Biol. Evol. 22, 1185–1192. doi: 10.1093/molbev/msi103
Guo, Z., Chen, X. X., Ruan, H., Qiao, S., Deng, R., and Zhang, G. (2020). Isolation of Three Novel Senecavirus A Strains and Recombination Analysis Among Senecaviruses in China. Front. Vet. Sci. 7:2. doi: 10.3389/fvets.2020.00002
Hales, L. M., Knowles, N. J., Reddy, P. S., Xu, L., Hay, C., and Hallenbeck, P. L. (2008). Complete genome sequence analysis of Seneca Valley virus-001, a novel oncolytic picornavirus. J. Gen. Virol. 89, 1265–1275. doi: 10.1099/vir.0.83570-0
Hause, B. M., Myers, O., Duff, J., and Hesse, R. A. (2016). Senecavirus A in Pigs. United States, 2015. Emerg. Infect. Dis. 22, 1323–1325. doi: 10.3201/eid2207.151591
Houston, E., Gimenez-Lirola, L. G., Magtoto, R., Mora-Diaz, J. C., Baum, D., and Pineyro, P. E. (2019). Seroprevalence of Senecavirus A in sows and grower-finisher pigs in major swine producing-states in the United States. Prev. Vet. Med. 165, 1–7. doi: 10.1016/j.prevetmed.2019.01.012
Jiang, J., Zha, Y., Liu, J., Xing, C., Mi, S., Yu, J., et al. (2021). Isolation and evolutionary analysis of Senecavirus A isolates from Guangdong province, China. Infect. Genet. Evol. 91:104819. doi: 10.1016/j.meegid.2021.104819
Jin, Y., Yu, D., Ren, H., Yin, Z., Huang, Z., Hu, M., et al. (2014). Phylogeography of Avian influenza A H9N2 in China. BMC Genomics 15:1110. doi: 10.1186/1471-2164-15-1110
Joshi, L. R., Mohr, K. A., Clement, T., Hain, K. S., Myers, B., Yaros, J., et al. (2016). Detection of the Emerging Picornavirus Senecavirus A in Pigs. Mice, and Houseflies. J. Clin. Microbiol. 54, 1536–1545. doi: 10.1128/JCM.03390-15
Joshi, L. R., Mohr, K. A., Gava, D., Kutish, G., Buysse, A. S., Vannucci, F. A., et al. (2020). Genetic diversity and evolution of the emerging picornavirus Senecavirus A. J. Gen. Virol. 101, 175–187. doi: 10.1099/jgv.0.001360
Katoh, K., Misawa, K., Kuma, K., and Miyata, T. (2002). MAFFT: A novel method for rapid multiple sequence alignment based on fast Fourier transform. Nucleic Acids Res. 30, 3059–3066. doi: 10.1093/nar/gkf436
Kim, J., Lee, K., Rupasinghe, R., Rezaei, S., Martinez-Lopez, B., and Liu, X. (2021). Applications of Machine Learning for the Classification of Porcine Reproductive and Respiratory Syndrome Virus Sublineages Using Amino Acid Scores of ORF5 Gene. Front. Vet. Sci. 8:683134. doi: 10.3389/fvets.2021.683134
Kline, D., Li, Z., Chu, Y., Wakefield, J., Miller, W. C., Norris Turner, A., et al. (2021). Estimating seroprevalence of SARS-CoV-2 in Ohio: A Bayesian multilevel poststratification approach with multiple diagnostic tests. Proc. Natl. Acad. Sci. U.S.A. 118:e2023947118. doi: 10.1073/pnas.2023947118
Lanave, C., Preparata, G., Saccone, C., and Serio, G. (1984). A new method for calculating evolutionary substitution rates. J. Mol. Evol. 20, 86–93. doi: 10.1007/BF02101990
Leme, R. A., Alfieri, A. F., and Alfieri, A. A. (2017). Update on Senecavirus Infection in Pigs. Viruses 9:170. doi: 10.3390/v9070170
Leme, R. A., Miyabe, F. M., Dall Agnol, A. M., Alfieri, A. F., and Alfieri, A. A. (2019a). A new wave of Seneca Valley virus outbreaks in Brazil. Transbound. Emerg. Dis. 66, 1101–1104. doi: 10.1111/tbed.13151
Leme, R. A., Miyabe, F. M., Dall Agnol, A. M., Alfieri, A. F., and Alfieri, A. A. (2019b). Seneca Valley virus RNA detection in pig feed and feed ingredients in Brazil. Transbound. Emerg. Dis. 66, 1449–1453. doi: 10.1111/tbed.13215
Leme, R. A., Zotti, E., Alcantara, B. K., Oliveira, M. V., Freitas, L. A., Alfieri, A. F., et al. (2015). Senecavirus A: An Emerging Vesicular Infection in Brazilian Pig Herds. Transbound. Emerg. Dis. 62, 603–611. doi: 10.1111/tbed.12430
Lemey, P., Hong, S. L., Hill, V., Baele, G., Poletto, C., Colizza, V., et al. (2020). Accommodating individual travel history and unsampled diversity in Bayesian phylogeographic inference of SARS-CoV-2. Nat. Commun. 11:5110. doi: 10.1038/s41467-020-18877-9
Lemey, P., Rambaut, A., Drummond, A. J., and Suchard, M. A. (2009). Bayesian phylogeography finds its roots. PLoS Comput. Biol. 5:e1000520. doi: 10.1371/journal.pcbi.1000520
Li, J., Li, Z., Cui, X., and Wu, C. (2020). Bayesian phylodynamic inference on the temporal evolution and global transmission of SARS-CoV-2. J. Infect. 81, 318–356. doi: 10.1016/j.jinf.2020.04.016
Li, W., and Godzik, A. (2006). Cd-hit. Bioinformatics 22, 1658–1659. doi: 10.1093/bioinformatics/btl158
Liu, C., Li, X., Liang, L., Li, J., and Cui, S. (2019). Isolation and phylogenetic analysis of an emerging Senecavirus A in China, 2017. Infect. Genet. Evol. 68, 77–83. doi: 10.1016/j.meegid.2018.12.009
Liu, F., Wang, Q., Huang, Y., Wang, N., and Shan, H. (2020). A 5-Year Review of Senecavirus A in China since Its Emergence in 2015. Front. Vet. Sci. 7:567792. doi: 10.3389/fvets.2020.567792
Martin, D. P., Murrell, B., Golden, M., Khoosal, A., and Muhire, B. (2015). RDP4: Detection and analysis of recombination patterns in virus genomes. Virus. Evol. 1:vev003. doi: 10.1093/ve/vev003
Nguyen, L. T., Schmidt, H. A., von Haeseler, A., and Minh, B. Q. (2015). IQ-TREE: A fast and effective stochastic algorithm for estimating maximum-likelihood phylogenies. Mol. Biol. Evol. 32, 268–274. doi: 10.1093/molbev/msu300
Oliveira, T. E. S., Michelazzo, M. M. Z., Fernandes, T., de Oliveira, A. G., Leme, R. A., Alfieri, A. F., et al. (2017). Histopathological, immunohistochemical, and ultrastructural evidence of spontaneous Senecavirus A-induced lesions at the choroid plexus of newborn piglets. Sci. Rep. 7:16555. doi: 10.1038/s41598-017-16407-0
Qian, S., Fan, W., Qian, P., Chen, H., and Li, X. (2016). Isolation and full-genome sequencing of Seneca Valley virus in piglets from China, 2016. Virol. J. 13:173. doi: 10.1186/s12985-016-0631-2
Rambaut, A. (2019). Figtree v1.4.4. Available online at: http://tree.bio.ed.ac.uk/software/figtree/
Rambaut, A., Drummond, A. J., Xie, D., Baele, G., and Suchard, M. A. (2018). Posterior Summarization in Bayesian Phylogenetics Using Tracer 1.7. Syst. Biol. 67, 901–904. doi: 10.1093/sysbio/syy032
Rambaut, A., Holmes, E. C., O’Toole, A., Hill, V., McCrone, J. T., Ruis, C., et al. (2020). nomenclature proposal for SARS-CoV-2 lineages to assist genomic epidemiology. Nat. Microbiol. 5, 1403–1407. doi: 10.1038/s41564-020-0770-5
Rambaut, A., Lam, T. T., Max Carvalho, L., and Pybus, O. G. (2016). Exploring the temporal structure of heterochronous sequences using TempEst (formerly Path-O-Gen). Virus Evol. 2:vew007. doi: 10.1093/ve/vew007
Saeng-Chuto, K., Rodtian, P., Temeeyasen, G., Wegner, M., and Nilubol, D. (2018). The first detection of Senecavirus A in pigs in Thailand, 2016. Transbound. Emerg. Dis. 65, 285–288. doi: 10.1111/tbed.12654
Shi, M., Lam, T. T., Hon, C. C., Murtaugh, M. P., Davies, P. R., Hui, R. K., et al. (2010). Phylogeny-based evolutionary, demographical, and geographical dissection of North American type 2 porcine reproductive and respiratory syndrome viruses. J. Virol. 84, 8700–8711. doi: 10.1128/JVI.02551-09
Sun, D., Chen, S., Cheng, A., and Wang, M. (2016). Roles of the Picornaviral 3C Proteinase in the Viral Life Cycle and Host Cells. Viruses 8:82. doi: 10.3390/v8030082
Sun, Y. K., Han, X. L., Wei, Y. F., Yu, Z. Q., Ji, C. H., Li, Q., et al. (2019). Phylogeography, phylodynamics and the recent outbreak of lineage 3 porcine reproductive and respiratory syndrome viruses in China. Transbound. Emerg. Dis. 66, 2152–2162. doi: 10.1111/tbed.13269
Sun, Y., Cheng, J., Wu, R. T., Wu, Z. X., Chen, J. W., Luo, Y., et al. (2018). Phylogenetic and Genome Analysis of 17 Novel Senecavirus A Isolates in Guangdong Province, 2017. Front. Vet. Sci. 5:314. doi: 10.3389/fvets.2018.00314
Vannucci, F. A., Linhares, D. C., Barcellos, D. E., Lam, H. C., Collins, J., and Marthaler, D. (2015). Identification and Complete Genome of Seneca Valley Virus in Vesicular Fluid and Sera of Pigs Affected with Idiopathic Vesicular Disease. Brazil. Transbound. Emerg. Dis. 62, 589–593. doi: 10.1111/tbed.12410
Victoria, M., Miagostovich, M. P., Ferreira, M. S., Vieira, C. B., Fioretti, J. M., Leite, J. P., et al. (2009). Bayesian coalescent inference reveals high evolutionary rates and expansion of Norovirus populations. Infect. Genet. Evol. 9, 927–932. doi: 10.1016/j.meegid.2009.06.014
Wang, L., Prarat, M., Hayes, J., and Zhang, Y. (2016). Detection and Genomic Characterization of Senecavirus A. Ohio, USA, 2015. Emerg. Infect. Dis. 22, 1321–1323. doi: 10.3201/eid2207.151897
Wang, M., Chen, L., Pan, S., Mou, C., Shi, K., and Chen, Z. (2019). Molecular evolution and characterization of novel Seneca Valley virus (SVV) strains in South China. Infect. Genet. Evol. 69, 1–7. doi: 10.1016/j.meegid.2019.01.004
Wang, Y., Das, A., Zheng, W., Porter, E., Xu, L., Noll, L., et al. (2020). Development and evaluation of multiplex real-time RT-PCR assays for the detection and differentiation of foot-and-mouth disease virus and Seneca Valley virus 1. Transbound. Emerg. Dis. 67, 604–616. doi: 10.1111/tbed.13373
Wang, Z., Zhang, X., Yan, R., Yang, P., Wu, Y., Yang, D., et al. (2018). Emergence of a novel recombinant Seneca Valley virus in Central China, 2018. Emerg. Microbes Infect. 7:180. doi: 10.1038/s41426-018-0183-1
Wu, Q., Zhao, X., Bai, Y., Sun, B., Xie, Q., and Ma, J. (2017). The First Identification and Complete Genome of Senecavirus A Affecting Pig with Idiopathic Vesicular Disease in China. Transbound. Emerg. Dis. 64, 1633–1640. doi: 10.1111/tbed.12557
Xu, W., Hole, K., Goolia, M., Pickering, B., Salo, T., Lung, O., et al. (2017). Genome wide analysis of the evolution of Senecavirus A from swine clinical material and assembly yard environmental samples. PLoS One 12:e0176964. doi: 10.1371/journal.pone.0176964
Yiannoutsos, C. T., Halverson, P. K., and Menachemi, N. (2021). Bayesian estimation of SARS-CoV-2 prevalence in Indiana by random testing. Proc. Natl. Acad. Sci. U.S.A. 118:e2013906118 doi: 10.1073/pnas.2013906118
Zhang, D., Gao, F., Jakovlic, I., Zou, H., Zhang, J., Li, W. X., et al. (2020). PhyloSuite: An integrated and scalable desktop platform for streamlined molecular sequence data management and evolutionary phylogenetics studies. Mol. Ecol. Resour. 20, 348–355. doi: 10.1111/1755-0998.13096
Zhang, J., Pineyro, P., Chen, Q., Zheng, Y., Li, G., Rademacher, C., et al. (2015). Full-Length Genome Sequences of Senecavirus A from Recent Idiopathic Vesicular Disease Outbreaks in U.S. Swine. Genome Announc. 3:e1270–e1215. doi: 10.1128/genomeA.01270-15
Zhang, J., Zhang, H., Sun, W., Jiao, C., Xiao, P., Han, J., et al. (2021). Genetic evolution and epidemiological analysis of Seneca Valley virus (SVV) in China. Virus Res. 291:198177. doi: 10.1016/j.virusres.2020.198177
Keywords: Senecavirus A (SVA), global dispersal, phylogeography, phylodynamics, phylogeny
Citation: Gao H, Chen Y-j, Xu X-q, Xu Z-y, Xu S-j, Xing J-b, Liu J, Zha Y-f, Sun Y-k and Zhang G-h (2022) Comprehensive phylogeographic and phylodynamic analyses of global Senecavirus A. Front. Microbiol. 13:980862. doi: 10.3389/fmicb.2022.980862
Received: 29 June 2022; Accepted: 15 August 2022;
Published: 29 September 2022.
Edited by:
Qin Zhao, Northwest A&F University, ChinaReviewed by:
Van Giap Nguyen, Vietnam National University of Agriculture, VietnamMoh A. Alkhamis, Kuwait University, Kuwait
Dachrit Nilubol, Chulalongkorn University, Thailand
Copyright © 2022 Gao, Chen, Xu, Xu, Xu, Xing, Liu, Zha, Sun and Zhang. This is an open-access article distributed under the terms of the Creative Commons Attribution License (CC BY). The use, distribution or reproduction in other forums is permitted, provided the original author(s) and the copyright owner(s) are credited and that the original publication in this journal is cited, in accordance with accepted academic practice. No use, distribution or reproduction is permitted which does not comply with these terms.
*Correspondence: Yan-kuo Sun, eWFua3Vvc3VuQHNjYXUuZWR1LmNu; Gui-hong Zhang, Z3VpaG9uZ3poQHNjYXUuZWR1LmNu
†These authors have contributed equally to this work