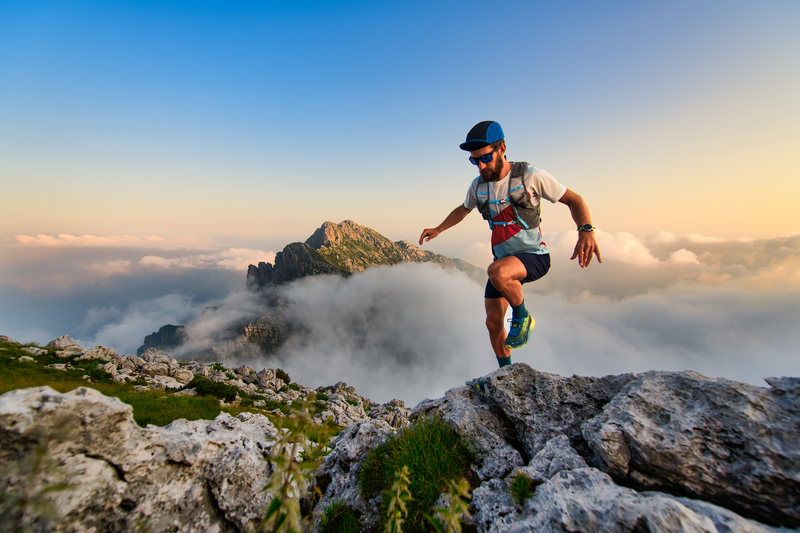
95% of researchers rate our articles as excellent or good
Learn more about the work of our research integrity team to safeguard the quality of each article we publish.
Find out more
ORIGINAL RESEARCH article
Front. Microbiol. , 11 November 2022
Sec. Microorganisms in Vertebrate Digestive Systems
Volume 13 - 2022 | https://doi.org/10.3389/fmicb.2022.980082
Objectives: The intestinal microbiota is essential in absorbing nutrients and defending against pathogens and is associated with various diseases, including obesity, type 2 diabetes, and hypertension. As an alternative medicine, Traditional Chinese Medicine (TCM) has long been used in disease treatment and healthcare, partly because it may mediate gut microbiota. However, the specific effects of TCM on the abundance and interactions of microbiota remain unknown. Moreover, using TCM ingredients and data detailing changes in the abundance of gut microorganisms, we developed bioinformatic methods that decipher the impact of TCM on microorganism interactions.
Methods: The dynamics of gut microorganisms affected by TCM treatments is explored using a mouse model, which provided the abundance of 70 microorganisms over time. The Granger causality analysis was used to measure microorganism interactions. Novel “serial connection” and “diverging connection” models were used to identify molecular mechanisms underlying the impact of TCM on gut microorganism interactions, based on microorganism proteins, TCM chemical ingredients, and KEGG reaction equations.
Results: Codonopsis pilosula (Dangshen), Cassia twig (Gui Zhi), Radices saussureae (Mu Xiang), and Sijunzi Decoction did not cause an increase in the abundance of harmful microorganisms. Most TCMs decreased the abundance of Bifidobacterium pseudolongum, suggesting a Bifidobacterium pseudolongum supplement should be used during TCM treatment. The Granger causality analysis indicated that TCM treatment changes more than half the interactions between the 70 microorganisms, and “serial connection” and “diverging connection” models suggested that changes in interactions may be related to the reaction number connecting species proteins and TCM ingredients. From a species diversity perspective, a TCM decoction is better than a single herb for healthcare. The Sijunzi Decoction only significantly increased the abundance of Bifidobacterium pseudolongum and did not cause a decrease in the abundance of other species but was found to improve the alpha diversity with the lowest replacement rate.
Conclusions: Because most of the nine TCMs are medicinal and edible plants, we expect the methods and results presented can be used to optimize and integrate microbiota and TCMs into healthcare processes. Moreover, as a control study, these results can be combined with future disease mouse models to link variations in species abundance with particular diseases.
The intestinal microbiota consists of 1013-1014 microorganisms, with a gene number 100 times that of the human genome (Gill et al., 2006). This microbiota acts as an important ecosystem (Sonnenburg et al., 2005) that plays essential biological roles in maintaining health by potentially protecting the host from pathogen infections (Sorbara and Pamer, 2019) and other diseases (Xu et al., 2015; Liu et al., 2017). Compared with an abundance per sample, a time series of microorganism abundance can characterize dynamic rather than static processes and examine inter-microorganism dependencies between adjacent time points (Mehta et al., 2018), and this scheme is used to study gut microorganisms in infants (Vatanen et al., 2016) and the effects of type 1 diabetes (T1D) (Kostic et al., 2015) and antibiotics on gut microbiota (Yassour et al., 2016).
Most human gut microbiome experiments must be carried out with animal models, mainly mouse models (Nguyen et al., 2015), for ethical and safety reasons. Germ-free (Schaedler et al., 1965), ASF (altered Schaedler flora) (Brand et al., 2015) and HFA (human flora-associated) (Hirayama et al., 1995) are often used in mouse model experiments. The mouse intestinal bacterial collection (miBC) database contains gut microbes from various mice (Lagkouvardos et al., 2016), and the mouse gut microbial biobank (mGMB) contains data covering 126 species represented by 244 strains (Liu et al., 2020). However, only a few projects have systematically constructed an abundance time series of mouse intestinal microorganisms, which limits our understanding of microorganism interactions.
As an important alternative medicine, Traditional Chinese Medicine (TCM) has a long utilization and treatment history over thousands of years and has contributed significantly to human disease prophylaxis and therapy (Qiu, 2007). TCM medicine for COVID-19 has received national clinical approval (Lyu et al., 2021). The World Health Organization has estimated that up to 80% of the global population living in developing countries relies on herbal medicines as a primary source of healthcare (World Health Organization, 2004). Even in developed countries in Europe and North America, herbal medicines are consumed in large quantities, and there is an increasing demand for these complementary and alternative medicines (Ekor, 2014). By studying ancient literature on TCM, Youyou Tu won the Nobel Prize for discovering artemisinin, a drug refined from a TCM, Artemisia apiacea, that can be used to treat malaria. Hence, investigating TCM ingredients as potential therapies is a worthwhile venture. TCM can also be used to treat intestinal diseases, perhaps representing the most important potential of TCM in medical therapy (Teschke et al., 2015; Bailly, 2021). Literature has studied the relationship between microbiota and TCM or its ingredients (Gong et al., 2020; Zheng et al., 2020); however, few papers have focused on the association of gut microbiota and TCM for intestinal diseases. In this report, we focus on nine effective TCMs for treating intestinal disease and explore their impact on typical mouse gut microorganisms. As a control, the results can be combined with future disease mouse models to identify variations in microorganism abundance associated with the disease. Moreover, most of the nine TCMs are both medicinal and edible plants; thus, the methods and results herein can be used to optimize and integrate microbiota and the nine TCMs into healthcare and intestinal disease treatments.
The interactions of microorganisms contribute to the stability of the microbiome. The strength of this interaction can be measured by the Granger causality approach, which identifies causality between different time series (Granger, 1969), can be used to construct sensorimotor cortical networks (Brovelli et al., 2004) and gene regulation networks (Finkle et al., 2018) and to connect species in complex ecosystems (Sugihara et al., 2012). A current review provides a comprehensive summary (Shojaie and Fox, 2022). In this paper, the null hypothesis for Granger causality tests is that the time series of one species' abundance (x2) does not cause the other species' time series (x1). We reject the null hypothesis when x2 does not cause x1 if p < 0.01 and accept the hypothesis when x2 causes x1 at the level of p < 0.01. For the python function “grangercausalitytests,” there are four kinds of tests for the null hypothesis: two tests are based on the F distribution, “params_ftest” and “ssr_ftest,” and two are based on the χ2 distribution, “ssr_chi2test” and “lrtest.”
To benefit healthcare and intestinal disease TCM treatment, we used the bio-statistic and Granger causality methods to explore the impact of TCM on gut microorganism abundance and interactions using nine TCMs and standard mouse gut microorganism sequencing data. Moreover, by novel serial and diverging connection models, we also deduced possible reaction pathways underlying the impact of the interactions.
The TCMs were obtained from Kangmei Pharmaceutical Industry Co., Ltd, with batch numbers (BN) as follows: Codonopsis pilosula (Dangshen, BN:17040212), Poria cocos (Fuling, BN:170809401), Rhizoma dioscoreae (Shan Yao, BN:170711221), Radices saussureae (Mu Xiang, BN:170501921), Rhizoma Zingiberis (Ganjiang, BN:170708491), Cassia twig (Gui Zhi, BN:170704361), Magnolia officinalis (Houpo, BN:170700141), White Atractylodes rhizome (Bai Zhu, BN: 170812601), Poria cocos (Fuling, BN:170809401), Liquiritiae glycyrrhizae (Gan Cao, BN:170808051), Astragalus mongholicus (Huangqi, BN:170800541), and Chinese angelica (Dang Gui, BN:170708441).
Initially, we weighed seven single dried Chinese herbs and two compounds used in intestinal treatment (Teschke et al., 2015; Bailly, 2021). Codonopsis pilosula may affect small intestinal propulsion movement (Li et al., 2004). Poria cocos can improve intestinal barrier function and intestinal microbiota (Yu and Liu, 2021). Rhizoma dioscoreae (Fu et al., 2008) and Radices saussureae (Tang et al., 2010) are medicines used to treat gastrointestinal disorders. Magnolia officinalis is a popular TCM with antibiotic and analgesic effects (Lu et al., 2004). Cassia twig is also an important alternative medicine that displays anti-inflammatory, antibacterial, antiviral, anti-allergic, and analgesic functions (Ye et al., 2022). The TCMs used herein have been verified by clinical investigation, are herbal slices without any secondary processing (granule, powder, and herbal leaven), and most of them, including Codonopsis pilosula (Dangshen), Poria cocos (Fuling), Rhizoma dioscoreae (Shan Yao), Rhizoma zingiberis (Ganjiang), Liquiritiae glycyrrhizae (Gan Cao), Astragalus mongholicus (Huangqi), and Chinese angelica (Dang Gui), are medicinal and edible plants. TCM samples listed in Tables 1, 2 were placed in round-bottom flasks and 10-fold the weight of pure water was added, and these samples were left to soak for 30 min. Samples were then heated in the round-bottom flasks and boiled for over an hour. After cooling, the flasks containing the TCM water extracts were filtered with six-layer gauze, and the nine water extracts were collected. We then added pure water (eight-fold in weight) to the nine remaining TCM residues, and after repeating the boiling, cooling, and filtering process, these nine water extracts were also stored. We combined the water extracts derived from the same TCMs and concentrated the nine water extracts to yield the crude drug concentrations listed in Tables 1, 2. Samples were stored at −20°C.
This study was performed with 198 male-specific pathogen-free (SPF) C57BL/6J mice aged 6 weeks. The mice were housed in the SPF animal breeding room of KMHD under License No. SYXK (Yue) 2019-0205, at a temperature of 23–25°C, moisture 54–57% and a 12-h light/dark cycle. This experimental study was approved by the Ethics Committee of KMHD under Approval No. IACUC-0210831-1.
After feeding for a week, 198 mice were randomly grouped into 11 groups, with each group consisting of 18 mice. Among the 11 groups, nine groups were treated with different TCMs by gastric infusion (Figure 1), and the other two groups were the blank control group (blank group) and the physiological saline treatment group (saline group). Mice were given free access to food and drinking water.
Figure 1. Experimental design and the microorganism relative abundance calculation for 70 species over five time points, where ASDSB represents the Dang Gui Bu Xue Decoction, and DFMD represents the Sijunzi Decoction.
Fecal collection process: after alcohol disinfection of each cage, three mice in each cage acted freely and defecated freely. Using sterile forceps, fresh feces were collected, placed into 2EP tubes, and quickly placed at −80°C. The fecal collection was performed before intragastric administration. The intragastric administration was suspended during the 3rd week (days 15 to 21) of the experiment.
High-quality genomic DNA was extracted from the mouse feces. The DNA that passed quality control was then used to construct a library using the TruSeq DNA HT Sample Prep Kit. Paired-end sequencing (2 × 150 bp) was carried out using the Illumina HiSeq X10 platform. After removing the host (mouse) and low-quality sequences, the relative abundance was calculated using MetaPhlAn 3.0 (Beghini et al., 2021) with default parameter values. Functional profiling was performed using HUMAnN3 (Beghini et al., 2021) in the UniRef90 mode. In Supplementary material 1, merged_abundance_table_species70.csv, we list the relative abundance of 70 species at 0, 3, 14, 21, and 28-day time points under the 11 different treatments, where the sum of each column is 100.
KEGG (Kyoto Encyclopedia of Genes and Genomes) (http://rest.kegg.jp/list/reaction) includes 11,075 reactions and represents a primary source to relate TCM with microorganisms. Chemical compositions and keywords of chemical reactions related to Cassia twig (Gui Zhi) and Sijunzi Decoction are listed in Supplementary material 2. Among the 70 microorganisms we annotated from mice gut microbiota, 28 microorganisms are present in KEGG (Supplementary Table 1 in Supplementary material 3), and their proteins and metabolites can also be obtained from KEGG. We retrieved reactions involving each TCM composition or microorganism metabolite from KEGG. Thus, a TCM or microorganism can be related to several hundred reactions. If a TCM and a microorganism share some reactions, they may naturally interact through these shared reactions. Similarly, the intersection of reactions between a TCM and two microorganisms may indicate where the TCM affects microorganism interactions.
Most microorganisms with increasing abundance following treatment with the nine TCMs have positive health functions for humans. There were 11 TCM-free samples on day 0. After N (0, 1) normalizing each column of merged_abundance_table_species70.csv, from the 11 TCM-free samples, we estimated the mean abundance values and variances for all 70 microorganisms. Then, we calculated the mean relative abundance for each microorganism species under each TCM treatment on days 3, 14, 21, and 28. If the mean is in the 95% confidence interval, treatment does not significantly change the abundance of the species at the p1 < 0.05 level according to the two-tail test. A mean located on the left side of the 95% confidence interval indicates that treatment significantly decreases the abundance of a species, whereas a mean on the right side of the confidence interval indicates that treatment increases the abundance of a species, with p2 < 0.025 using the one-tail test.
Regarding setting p2 < 0.005 with the one-tail test, no microorganisms treated with the nine TCMs showed a decrease; however, setting p2 < 0.05 with the one-tail test revealed that Dang Gui Bu Xue Decoction, Cassia twig (Gui Zhi), and Sijunzi Decoction did not cause a significant decrease in the abundance of all microorganisms, whereas the other TCMs caused a reduction in the abundance of Bifidobacterium pseudolongum. This observation suggests that people taking TCM for healthcare should also take probiotics (including Bifidobacterium pseudolongum) to neutralize the mild adverse effects because this species is enriched with specific enzymes that degrade complex plant carbohydrates and host glycans (Xiao et al., 2021).
In Figure 2 and Supplementary Table 2 in Supplementary material 3, we show species whose abundance increased under the nine treatments with a p2 < 0.025 using the one-tail test. Many microorganisms have positive (or conditionally positive) benefits to human health, including Lactobacillus_johnsonii (Lim et al., 2017), Parasutterella_excrementihominis (Fart et al., 2020), Bacteroides_vulgatus (You et al., 2019), Parabacteroides_distasonis (Wang et al., 2019), Parabacteroides_goldsteinii (Wu et al., 2018), and Lachnospiraceae_bacterium_A4, which increase the short-chain fatty acids (SCFA) content in the caecum, Enterorhabdus_caecimuris (Fang et al., 2022), which aids the production of tryptophan metabolism in the gut, and Bacteroides_thetaiotaomicron (Delday et al., 2019) and Dubosiella_newyorkensis, which improve various health indicators of a fatty mouse. Lactobacillus_murinus (Perelmuter et al., 2008), Bacteroides_uniformis (Zhao et al., 2016), Adlercreutzia_equolifaciens (Zhu et al., 2015), and Bacteroides_intestinalis are helpful for treating type 2 diabetes mellitus.
Figure 2. Increase in species abundance by the 11 treatments, where p2 < 0.025 with the one-tail test.
A few microorganisms with adverse conditional functions toward human health were also identified to increase in abundance following TCM treatments (Figure 2, Supplementary Table 2 in Supplementary material 3). For example, Desulfovibrionaceae_bacterium, which produces H2S, can harm intestinal epithelial cells, and Clostridium_bolteae (Cai et al., 2020), Flavonifractor_plautii (Wang et al., 2021), and Bilophila_wadsworthia also produce H2S and are associated with appendicitis and some other local inflammation. Lachnospiraceae_bacterium_28_4 and Romboutsia_ilealis was also found and may contribute to the development of diabetes in obese mice (Kameyama and Itoh, 2014; Rodrigues et al., 2021).
Most species identified to increase in abundance when exposed to the nine TCMs were positive or conditionally positive. This observation indicates that the nine TCMs, which have similar functions according to TCM theory (Wang and Guo, 2015), i.e., tonifying the spleen, regulating the flow of vital energy, and removing obstructions, have largely a consistent impact on gut microorganisms.
The LDA Effect Size (LEfSe) (Segata et al., 2011) is used to discover biomarkers that show a difference between two or more biological conditions or intervention groups. LEfSe is used to identify both statistical significance and biological relevance. LEfSe was applied to our data matrix (Supplementary material 1, merged_abundance_table_species70.csv), and the results are presented in Figure 3. Rhizoma dioscoreae (Shan Yao) was found to have five biomarkers: Bacteroides_caccae, Blautia_coccoides, Ruthenibacterium_lactatiformans, Clostridium_innocuum, and Lactobacillus_intestinalis. Rhizoma zingiberis (Ganjiang) was found to have Intestinimonas_butyriciproducens and Escherichia_coli as biomarkers. Codonopsis pilosula (Dangshen), Sijunzi, and Magnolia officinalis (Houpo) were found to have only one biomarker. Compared with Figure 2, the abundances of these biomarkers did not increase in their corresponding TCM treatment, except for Rhizoma zingiberis (Ganjiang), where the abundance of the two biomarkers increased.
Figure 3. LEfSe analysis identifies microorganism biomarkers that show distinctions among the nine TCM treatments.
Taking the python package statsmodels.tsa.stattools, importing the grangercausalitytests function and using gc_res = grangercausalitytests [DATA, (1)], the Granger causality p-values of two species were calculated based on their abundance time series included in variable DATA. We used the likelihood ratio test (“lrtest”), a χ2 distribution test, to calculate the p-values of the Granger causality. We found many possible causality relationships under the 11 treatments when p < 10−4. For example, we showed that the Granger causality network for mouse gut microorganisms treated by Sijunzi Decoction has a p < 10−4 (Figure 4). Supplementary Figures 1–10 of Supplementary material 4 show the Granger causality network for the other 10 networks. In summary, blank control, Dang Gui Bu Xue Decoction, Codonopsis pilosula (Dangshen), Poria cocos (Fuling), Rhizoma zingiberis (Ganjiang), Cassia twig (Gui Zhi), Magnolia officinalis (Houpo), Radices saussureae (Mu Xiang), saline control, Rhizoma dioscoreae (Shan Yao), and the Sijunzi Decoction have 372, 414, 426, 428, 467, 588, 443, 543, 431, 410 and 392 pairs of significantly correlated species, respectively.
Figure 4. Granger causality network for mouse gut microorganisms treated by the Sijunzi Decoction with p < 10−4.
Compared with the blank control group, the newly added causality pairs included 374, 383, 400, 454, 552, 423, 513, 410, 371, and 347 for the last 10 treatments, and 332, 329, 344, 359, 336, 352, 351, 351, 333, and 327 causality pairs vanished. In Figure 5, we also show the number of different causality pairs identified between 11 Granger causality networks with p < 10−4. Thus, correlations between the 70 species were found to change considerably by TCMs.
After setting lrtest to p < 0.00000001, a stricter cutoff, for blank control, Dang Gui Bu Xue Decoction, Codonopsis pilosula (Dangshen), Poria cocos (Fuling), Rhizoma zingiberis (Ganjiang), Cassia twig (Gui Zhi), Magnolia officinalis (Houpo), Radices saussureae (Mu Xiang), saline control, Rhizoma dioscoreae (Shan Yao), and Sijunzi Decoction, there remained 36, 30, 44, 45, 73, 22, 58, 61, 31, 64, and 45 pairs of significantly correlated species, respectively. Compared with the blank group, the number of newly added causality pairs was 29, 44, 45, 73, 22, 57, 61, 31, 63, and 45 for the last 10 treatments, and 35, 36, 36, 36, 36, 35, 36, 36, 35, and 36 causality pairs vanished, respectively. These results further confirmed that TCMs greatly changed the species correlations.
Moreover, the biomarkers shown in Figure 3 are not among the first 10 highest degree species in all Granger causality networks, including Figure 4 and Supplementary Figures 1–10 of Supplementary material 4.
A diversity index for a dataset is a quantity reflecting how many different species exist and how evenly individuals are distributed among those species. The diversity index increases with increasing species number and increasing evenness. Alpha diversity measures, in one sample, the number of different species and their different abundance, whereas beta diversity focuses on a group of samples and compares species compositions between different samples. For each sample of each treatment in our study, an alpha diversity index was calculated (Figure 6). Here, we found that for two decoction TCMs (Sijunzi Decoction and Dang Gui Bu Xue Decoction), the alpha diversity value increased slightly when comparing their start and end diversity values. In contrast, for the saline control, Poria cocos (Fuling) and Cassia twig (Gui Zhi), a decrease in the alpha diversity values were observed because their diversity at point “5” was significantly lower than that at point “1”. The remaining TCMs showed no significant change in their alpha diversity. From the perspective of species diversity, TCM decoctions were found to have a greater effect when compared with single herbs.
As shown in Supplementary material 5, we also calculated the Simpson and Invsimpson index, and found that three indexes were consistent and closely related, i.e., the Shannon index can describe the diversity of each sample.
Beta diversity measures differences between the species composition of samples, with larger beta diversity values indicating a larger replacement rate (Anderson et al., 2010). We calculated the beta diversity for the 11 treatments, with a beta diversity of 0.2222222 determined for the blank control group. The beta diversity of the Dang Gui Bu Xue Decoction treatment group was 0.2441315, whereas the value for the Codonopsis pilosula (Dangshen) treatment group was 0.2443439. A value of 0.2672811 was determined for Poria cocos (Fuling) treatment, 0.3333333 for Rhizoma zingiberis (Ganjiang) treatment, 0.2918660 for Cassia twig (Gui Zhi) treatment, 0.3302752 for Magnolia officinalis (Houpo) treatment, 0.3594470 for Radices saussureae (Mu Xiang) treatment, 0.2127660 for the saline control treatment, 0.4285714 for Rhizoma dioscoreae (Shan Yao) treatment and 0.1842105 for Sijunzi Decoction treatment. The Sijunzi Decoction, saline, and blank treatments have the lowest replacement rates, whereas Ganjiang, Houpo, Mu Xiang and Shan Yao have the highest replacement rates. Interestingly, the Sijunzi Decoction was found to only increase the abundance of Bifidobacterium_pseudolongum significantly, with no decrease in any other species abundance. Thus, this treatment improved the alpha diversity with the lowest replacement rate.
Two TCMs (Cassia twig and Sijunzi Decoction) and two microorganisms (Adlercreutzia equolifaciens and Bifidobacterium pseudolongum) were used as examples to clarify the novel mechanism exploration method as follows. First, the two microorganisms present very different correlation strengths under the two TCM treatments. Second, specific reactions involving the chemical compositions of the two TCMs and metabolisms of the two microorganisms were retrieved. Finally, by using novel serial and diverging connection reaction path models, contrast analysis was performed to deduce the possible mechanism of TCM that changes the microorganism correlation strength.
The interaction between Adlercreutzia equolifaciens and Bifidobacterium pseudolongum presents very different correlations: treatment with Cassia twig (Gui Zhi) gave a Granger causality lrtest p-value of 0.000064934, whereas treatment with the Sijunzi Decoction yielded a Granger causality lrtest p-value of 0.93943. We typically study three models to explore the relationship among the three objects (a TCM and two microorganisms): serial connection, diverging connection, and converging connection. Here, the “converging connection” is not applicable because microorganisms cannot have an impact on the TCM.
For convenience, in the following study, in Supplementary material 2 we have listed the chemical compositions of Cassia twig (Gui Zhi) and Sijunzi Decoction, respectively, and the metabolites of Adlercreutzia_equolifaciens and Bifidobacterium_pseudolongum are available from KEGG (data not shown due to size). “Serial connection” means three equations are involved in Adlercreutzia_equolifaciens → Bifidobacterium_pseudolongum → chemical composition of TCM, or Bifidobacterium_pseudolongum → Adlercreutzia_equolifaciens → chemical composition of TCM, where the TCM may increase the inter-species correlation by driving the chemical reaction based on Le Chatelier's principle/the equilibrium law.
For Cassia twig, taking the following as an example: {rn:R00237} acetyl-CoA + pyruvate ↔ (3S)-citramalyl-CoA (A. equolifaciens) → {rn:R02955} acetate + (3S)-citramalyl-CoA (A. equolifaciens) ↔ Acetyl-CoA (B. pseudolongum) + Citramalate → {rn:R10474} Acetyl-CoA (B. pseudolongum) + cinnamyl alcohol (Cassia twig) ↔ CoA + Cinnamyl acetate. Clearly, there are three equations involved in A. equolifaciens → B. pseudolongum → Cassia twig. Here, the metabolite “(3S)-citramalyl-CoA” of A. equolifaciens helps to produce the metabolite “acetyl-CoA” of B. pseudolongum, and the chemical composition of “cinnamyl alcohol” from Cassia twig can consume “acetyl-CoA” of B. pseudolongum. Hence, this serial connection may increase the correlation between A. equolifaciens and B. pseudolongum.
In Supplementary material, more information is provided on the novel serial connection models: in Supplementary material 6, we list 2,775 such serial connections: A. equolifaciens → B. pseudolongum → Cassia twig. In Supplementary material 7, 1,352 serial connections are listed: B. pseudolongum → A. equolifaciens → Cassia twig. In Supplementary material 8, 1,419 such serial connections are listed: A. equolifaciens → B. pseudolongum → Sijunzi Decoction, and in Supplementary material 9, we have 390 serial connections: B. pseudolongum → A. equolifaciens → Sijunzi Decoction. Clearly, for Adlercreutzia_equolifaciens and Bifidobacterium_pseudolongum, the number of serial connections driven by Cassia twig (2,775 + 1,352 = 4,127) is much larger than that driven by the Sijunzi Decoction (1,419 + 390 = 1,809). This observation may partially explain the stronger Granger causality of A. equolifaciens and B. pseudolongum under Cassia twig than that under the Sijunzi Decoction.
The “diverging connection” indicated that the compositions of TCM may react with both metabolites of A. equolifaciens and B. pseudolongum through the same or different reaction equations. As shown in Supplementary material 10, Cassia twig (Gui Zhi) connects with both A. equolifaciens and B. pseudolongum through 75 shared chemical reactions, whereas Cassia twig (Gui Zhi) has five specific equations with A. equolifaciens and 15 specific equations with B. pseudolongum. Data in Supplementary material 10 also shows that the Sijunzi Decoction connects with A. equolifaciens and B. pseudolongum through 11 shared chemical reactions, without any specific reactions. The more shared chemical reactions may explain, in part, the higher correlation between A. equolifaciens and B. pseudolongum under Cassia twig treatment than that found under Sijunzi Decoction treatment.
Mouse gut experiments and sequencing technology afforded a systematic analysis of the abundance of gut microorganisms over time with and without TCM treatments. The study also provided an opportunity to explore how TCM affects microorganism abundance and their inter-species correlations. Ingredient and reaction pathway analysis was used to explain the impact of TCM on gut microorganisms, and we focused our analysis on two TCMs, Cassia twig (Gui Zhi) and Sijunzi Decoction. Moreover, among the 70 microorganisms annotated in our mice model, only 28 microorganisms were annotated in KEGG. Given more detailed data, the novel serial and diverging connection models should provide additional insights into how TCM affects microorganism abundance and inter-species correlations.
The datasets presented in this study can be found in the Fecal Metagenomic sequencing reads from CNGB Nucleotide Sequence Archive under accession number CNP0002573.
The animal study was reviewed and approved by the Ethics Committee of KMHD on the use of animal subjects under Approval No. IACUC-0210831-1. Written informed consent was obtained from the owners for the participation of their animals in this study.
LH, XF, HY, and YZ designed the project. YC, XS, YD, QQ, and DZ conducted the experiments. DZ, YZ, and XB analyzed the data. DZ and XB developed the algorithm and software. YZ, DZ, and LH wrote and revised the paper. All authors contributed to the article and approved the submitted version.
This paper was supported by Science Technology and Innovation Committee of Shenzhen Municipality under Grant No. JCYJ20160331190123578 and the Natural Science Foundation of Hebei Province of China under Grant No. A2019208336.
We would like to thank Miaomiao Wang, Xiaochun Zhang, and Xifeng Lu for their support with experiments, and we also acknowledge Yajing Huang for interpreting the TCM clinical application. We thank Liwen Bianji (Edanz) (www.liwenbianji.cn) for editing the English text of a draft of this manuscript.
Authors YZ, DZ, XB, and XF were employed by Kangmeihuada GeneTech Co., Ltd.
The remaining authors declare that the research was conducted in the absence of any commercial or financial relationships that could be construed as a potential conflict of interest.
All claims expressed in this article are solely those of the authors and do not necessarily represent those of their affiliated organizations, or those of the publisher, the editors and the reviewers. Any product that may be evaluated in this article, or claim that may be made by its manufacturer, is not guaranteed or endorsed by the publisher.
The Supplementary Material for this article can be found online at: https://www.frontiersin.org/articles/10.3389/fmicb.2022.980082/full#supplementary-material
Anderson, M. J., Ellingsen, K. E., and Mcardle, B. H. (2010). Multivariate dispersion as a measure of beta diversity. Ecol. Lett. 9, 683–693. doi: 10.1111/j.1461-0248.2006.00926.x
Bailly, C. (2021). Atractylenolides, essential components of atractylodes-based traditional herbal medicines: antioxidant, anti-inflammatory and anticancer properties. Eur. J. Pharmacol. 891, 173735. doi: 10.1016/j.ejphar.2020.173735
Beghini, F., McIver, L. J., Blanco-Mìguez, A., Dubois, L., Asnicar, F., Maharjan, S., et al. (2021). Integrating taxonomic, functional, and strain-level profiling of diverse microbial communities with bioBakery 3. Elife 10, e65088. doi: 10.7554/eLife.65088
Brand, M. W., Wannemuehler, M. J., Phillips, G. J., Proctor, A., Overstreet, A. M., Jergens, A. E., et al. (2015). The altered schaedler flora: continued applications of a defined murine microbial community. ILAR J. 56, 169–178. doi: 10.1093/ilar/ilv012
Brovelli, A., Ding, M. Z., Ledberg, A., Chen, Y. H., Nakamura, R., Bressler, S. L., et al. (2004). Beta oscillations in a large-scale sensorimotor cortical network: directional influences revealed by Granger causality. Proc. Natl. Acad. Sci. U.S.A. 101, 9849–9854. doi: 10.1073/pnas.0308538101
Cai, J. T., Hu, J., Qin, C. J., Li, L. X., Shen, D. C., Tian, G. Z., et al. (2020). Chemical synthesis elucidates the key antigenic epitope of the autism-related bacterium Clostridium bolteae capsular octadecasaccharide. Angewandte Chem. 59, 20529–20537. doi: 10.1002/anie.202007209
Delday, M., Mulder, I., Logan, E. T., and Grant, G. (2019). Bacteroides thetaiotaomicron ameliorates colon inflammation in preclinical models of Crohn's disease. Inflamm. Bowel Dis. 25, 85–96. doi: 10.1093/ibd/izy281
Ekor, M. (2014). The growing use of herbal medicines: issues relating to adverse reactions and challenges in monitoring safety. Front. Pharmacol. 4, 177. doi: 10.3389/fphar.2013.00177
Fang, Z. F., Pan, T., Li, L. Z., Wang, H. C., Zhu, J. L., Zhang, H., et al. (2022). Bifidobacterium longum mediated tryptophan metabolism to improve atopic dermatitis via the gut-skin axis. Gut Microbes 14, 2044723. doi: 10.1080/19490976.2022.2044723
Fart, F., Rajan, S. K., Wall, R., Rangel, I., Ganda-Mall, J. P., Tingö, L., et al. (2020). Differences in gut microbiome composition between senior orienteering athletes and community-dwelling older adults. Nutrients 12, 2610. doi: 10.3390/nu12092610
Finkle, J. D., Wu, J. J., and Bagheri, N. (2018). Windowed Granger causal inference strategy improves discovery of gene regulatory networks. Proc. Natl. Acad. Sci. U.S.A. 115, 201710936. doi: 10.1073/pnas.1710936115
Fu, Z. Q., Cai, B. C., Bian, C. X., and Wang, M. Y. (2008). Comparison of the effect of polysaccharides from crude and processed Rhizoma Dioscoreae on gastrointestinal function in mice of splenic asthenia. Pharm. Clin. Res. 16, 181–183. doi: 10.13664/j.cnki.pcr.2008.03.007
Gill, S. R., Pop, M., DeBoy, R. T., Eckburg, P. B., Turnbaugh, P. J., Samuel, B. S., et al. (2006). Metagenomic analysis of the human distal gut microbiome. Science 312, 1355–1359. doi: 10.1126/science.1124234
Gong, X., Li, X., Bo, A., Shi, R. Y., Li, Q. Y., Lei, L. J., et al. (2020). The interactions between gut microbiota and bioactive ingredients of traditional Chinese medicines: a review. Pharmacol. Res. 157, 104824. doi: 10.1016/j.phrs.2020.104824
Granger, C. W. J. (1969). Investigating causal relations by econometric models and cross-spectral methods. Econometrica 424–438. doi: 10.2307/1912791
Hirayama, K., Miyaji, K., Kawamura, S., Itoh, K., Takahashi, E., Mitsuoka, T., et al. (1995). Development of intestinal flora of human-flora-associated (HFA) mice in the intestine of their offspring. Exp. Anim. 44, 219–222. doi: 10.1538/expanim.44.219
Kameyama, K., and Itoh, K. (2014). Intestinal colonization by a lachnospiraceae bacterium contributes to the development of diabetes in obese mice. Microbes Environ. 29, 427–430. doi: 10.1264/jsme2.ME14054
Kostic, A. D., Gevers, D., Siljander, H., Vatanen, T., Hyötyläinen, T., Hämäläinen, A. M., et al. (2015). The dynamics of the human infant gut microbiome in development and in progression toward type 1 diabetes. Cell Host Microbe. 17, 260–273. doi: 10.1016/j.chom.2015.01.001
Lagkouvardos, I., Pukall, R., Abt, B., Foesel, B. U., Meier-Kolthoff, J. P., Kumar, N., et al. (2016). The mouse intestinal bacterial collection (miBC) provides host-specific insight into cultured diversity and functional potential of the gut microbiota. Nat. Microbiol. 1, 16131. doi: 10.1038/nmicrobiol.2016.131
Li, C. R., Ning, L. X., and Su, S. J. (2004). Researches on the effect of magnetized codonopsis pilosula (Fra 4nch.) nannnf. Solution on rat's small intestinal propulsion movement. Biomagnetism 4, 9–11. doi: 10.13241/j.cnki.pmb.2004.04.00
Lim, S. M., Jang, H. M., Jeong, J. J., Han, M. J., and Kim, D. H. (2017). Lactobacillus johnsonii CJLJ103 attenuates colitis and memory impairment in mice by inhibiting gut microbiota lipopolysaccharide production and NF-κB activation. J. Funct. Foods 34, 359–368. doi: 10.1016/j.jff.2017.05.016
Liu, C., Zhou, N., Du, M. X., Sun, Y. T., Wang, K., Wang, Y. J., et al. (2020). The mouse gut microbial biobank expands the coverage of cultured bacteria. Nat. Commun. 11, 79. doi: 10.1038/s41467-019-13836-5
Liu, R., Hong, J., Xu, X., Feng, Q., Zhang, D., Gu, Y., et al. (2017). Gut microbiome and serum metabolome alterations in obesity and after weight-loss intervention. Nat. Med. 23, 859–868. doi: 10.1038/nm.4358
Lu, J. M., Chen, J., Liang, J. X., and Wang, Z. M. (2004). A comparative study of the antibiotic and analgesic effect of magnolia officinalis var biloba rehcoet wils dry hide before and after its diaphoretic processing. Inner Mongolia J. Tradit. Chin. Med. 1, 25–26. doi: 10.16040/j.cnki.cn15-1101.2004.01.026
Lyu, M., Fan, G. W., Xiao, G. X., Wang, T. Y., Xu, D., Gao, J., et al. (2021). Traditional Chinese medicine in COVID-19. Acta Pharm. Sin. B. 11, 3337–3363. doi: 10.1016/j.apsb.2021.09.008
Mehta, R. S., Abu-Ali, G. S., Drew, D. A., Lloyd-Price, J., Subramanian, A., Lochhead, P., et al. (2018). Stability of the human faecal microbiome in a cohort of adult men. Nat. Microbiol. 3, 347–355. doi: 10.1038/s41564-017-0096-0
Nguyen, T. L. A., Vieira-Silva, S., Liston, A., and Raes, J. (2015). How informative is the mouse for human gut microbiota research? Dis. Model. Mech. 8, 1–16. doi: 10.1242/dmm.017400
Perelmuter, K., Fraga, M., and Zunino, P. (2008). In vitro activity of potential probiotic Lactobacillus murinus isolated from the dog. J. Appl. Microbiol. 104, 1718–1725. doi: 10.1111/j.1365-2672.2007.03702.x
Rodrigues, R. R., Gurung, M., Li, Z. P., García-Jaramillo, M., Greer, R., Gaulke, C., et al. (2021). Transkingdom interactions between lactobacilli and hepatic mitochondria attenuate western diet-induced diabetes. Nat. Commun. 12, 101. doi: 10.1038/s41467-020-20313-x
Schaedler, R. W., Dubs, R., and Costello, R. (1965). Association of germfree mice with bacteria isolated from normal mice. J. Exp. Med. 122, 77–82. doi: 10.1084/jem.122.1.77
Segata, N., Izard, J., Walron, L., Gevers, D., Miropolsky, L., Garrett, W., et al. (2011). Metagenomic biomarker discovery and explanation. Genome Biol. 12, R60. doi: 10.1186/gb-2011-12-6-r60
Shojaie, A., and Fox, E. B. (2022). Granger causality: a review and recent advances. Ann. Rev. Stat. Applic. 9, 289–319. doi: 10.1146/annurev-statistics-040120-010930
Sonnenburg, J. L., Xu, J., Leip, D. D., Chen, C. H., Westover, B. P., Weatherford, J., et al. (2005). Glycan foraging in vivo by an intestine-adapted bacterial symbiont. Science 307, 1955–1959. doi: 10.1126/science.1109051
Sorbara, M. T., and Pamer, E. G. (2019). Interbacterial mechanisms of colonization resistance and the strategies pathogens use to overcome them. Mucosal Immunol. 12, 1–9. doi: 10.1038/s41385-018-0053-0
Sugihara, G., May, R., Ye, H., Hsieh, C. H., Deyle, E., Fogarty, M., et al. (2012). Detecting causality in complex ecosystems. Science 338, 496–500. doi: 10.1126/science.1227079
Tang, F., Noda, H., Yoshimura, M., Kubo, M., and Aeb, H. (2010). The effects of a chinese herbal medicine on gastrointestinal disorder with zinc abnormality. Jpn. J. Orient. Med. 45, 833–839. doi: 10.3937/kampomed.45.833
Teschke, R., Wolff, A., Frenzel, C., Eickhoff, A., and Schulze, J. (2015). Herbal traditional Chinese medicine and its evidence base in gastrointestinal disorders. World J. Gastroenterol. 21, 4466–4490. doi: 10.3748/wjg.v21.i15.4466
Vatanen, T., Kostic Aleksandar, D., d'Hennezel, E., Siljander, H., Franzosa Eric, A., Yassour, M., et al. (2016). Variation in microbiome LPS immunogenicity contributes to autoimmunity in humans. Cell 165, 842–853. doi: 10.1016/j.cell.2016.04.007
Wang, J. L., Ouyang, H., Xie, H. Y., Su, S., Jiao, S. L., Chen, Z. H., et al. (2021). Identification of endogenous bacteria RPB-32 of Radix pseudostellariae and its metabolites effect on intestinal microorganism community of mice. Microbiol. China. 48, 502–515. doi: 10.13344/j.microbiol.china.200168
Wang, K., Liao, M. F., Zhou, N., Bao, L., Ma, K., Zheng, Z. Y., et al. (2019). Parabacteroides distasonis modulates host metabolism and alleviates obesity and metabolic dysfunctions via production of succinate and secondary bile acids. Cell Rep. 26, 222–235. doi: 10.1016/j.celrep.2018.12.028
Wang, L., and Guo, J. (2015). Using experience of spleen and stomach theory and its clinical application. China J. Chin. Med. 30, 512–514. doi: 10.16368/j.issn.1674-8999.2015.04.176
World Health Organization (2004). WHO Guidelines on Safety Monitoring of Herbal Medicines in Pharmacovigilance Systems. Geneva: World Health Organization.
Wu, T. R., Lin, C. S., Chang, C. J., Lin, T. L., Martel, J., Ko, Y. F., et al. (2018). Gut commensal Parabacteroides goldsteinii plays a predominant role in the anti-obesity effects of polysaccharides isolated from Hirsutella sinensis. Gut 68, 248–262. doi: 10.1136/gutjnl-2017-315458
Xiao, Y., Zhao, J. X., Zhang, H., Zhai, Q. X., and Chen, W. (2021). Colonized niche, evolution and function signatures of Bifidobacterium pseudolongum within bifidobacterial genus. Foods 10, 2284. doi: 10.3390/foods10102284
Xu, J., Lian, F., Zhao, L., Zhao, Y., Chen, X., Zhang, X., et al. (2015). Structural modulation of gut microbiota during alleviation of type 2 diabetes with a Chinese herbal formula. ISME J. 9, 552–562. doi: 10.1038/ismej.2014.177
Yassour, M., Vatanen, T., Siljander, H., Hämäläinen, A. M., Härkönen, T., Ryhänen, S. J., et al. (2016). Natural history of the infant gut microbiome and impact of antibiotic treatment on bacterial strain diversity and stability. Sci. Transl. Med. 8, 343ra81. doi: 10.1126/scitranslmed.aad0917
Ye, T. L., Liu, X. N., and Shi, C. K. (2022). Research progress on pharmacological mechanism of Guizhi decoction. Drug Eval. Res. 45, 390–396. doi: 10.7501/j.issn.1674-6376.2022.02.027
You, J. S., Yong, J. H., Kim, G. H., Moon, S. M., Nam, K. T., Ryu, J. H., et al. (2019). Commensal-derived metabolites govern vibrio cholerae pathogenesis in host intestine. Microbiome 7, 132. doi: 10.1186/s40168-019-0746-y
Yu, J., and Liu, P. F. (2021). The effect of Poria cocos ethanol extract on the intestinal barrier function and intestinal microbiota in mice with breast cancer. J. Ethnopharmacol. 266, 113456. doi: 10.1016/j.jep.2020.113456
Zhao, S. Q., Liu, W., Shi, J., Wang, J. Q., Liu, R. X., Hong, J., et al. (2016). Bacteroides uniform is supplementation improves glucose homeostasis by alienating lipid accumulation and endo- plasmic reticulum stressin liver/muscle in chow-dietmice. J. Diagn. Concepts Pract. 15, 263–267. doi: 10.16150/j.1671-2870.2016.03.015
Zheng, Y., Gou, X., Zhang, L., Gao, H., Wei, Y., Yu, X., et al. (2020). Interactions between gut microbiota, host, and herbal medicines: a review of new insights into the pathogenesis and treatment of type 2 diabetes. Front. Cell. Infect. Microbiol. 10, 360. doi: 10.3389/fcimb.2020.00360
Keywords: bioinformatics, Granger causality, Traditional Chinese Medicine, intestinal microbiology, molecular mechanisms
Citation: Zhang Y, Zhang D, Bai X, Chen Y, Qiu Q, Shang X, Deng Y, Yang H, Fang X, Yang Z and Han L (2022) The impact of Traditional Chinese Medicine on mouse gut microbiota abundances and interactions based on Granger causality and pathway analysis. Front. Microbiol. 13:980082. doi: 10.3389/fmicb.2022.980082
Received: 14 July 2022; Accepted: 11 October 2022;
Published: 11 November 2022.
Edited by:
Haixia Chen, Tianjin University, ChinaReviewed by:
Chengping Wen, Zhejiang Chinese Medical University, ChinaCopyright © 2022 Zhang, Zhang, Bai, Chen, Qiu, Shang, Deng, Yang, Fang, Yang and Han. This is an open-access article distributed under the terms of the Creative Commons Attribution License (CC BY). The use, distribution or reproduction in other forums is permitted, provided the original author(s) and the copyright owner(s) are credited and that the original publication in this journal is cited, in accordance with accepted academic practice. No use, distribution or reproduction is permitted which does not comply with these terms.
*Correspondence: Yi Zhang, emhhcWkxOTcyQDE2My5jb20=; Lijuan Han, MTA5Mjc4MzI1QHFxLmNvbQ==; Zhimin Yang, eWFuZ3lvdmlwQDEyNi5jb20=
†These authors have contributed equally to this work
Disclaimer: All claims expressed in this article are solely those of the authors and do not necessarily represent those of their affiliated organizations, or those of the publisher, the editors and the reviewers. Any product that may be evaluated in this article or claim that may be made by its manufacturer is not guaranteed or endorsed by the publisher.
Research integrity at Frontiers
Learn more about the work of our research integrity team to safeguard the quality of each article we publish.