- Environment and Life Science Research Centre, Kuwait Institute for Scientific Research, Kuwait City, Kuwait
The airborne transmission of COVID-19 has drawn immense attention to bioaerosols. The topic is highly relevant in the indoor hospital environment where vulnerable patients are treated and healthcare workers are exposed to various pathogenic and non-pathogenic microbes. Knowledge of the microbial communities in such settings will enable precautionary measures to prevent any hospital-mediated outbreak and better assess occupational exposure of the healthcare workers. This study presents a baseline of the bacterial and fungal population of two major hospitals in Kuwait dealing with COVID patients, and in a non-hospital setting through targeted amplicon sequencing. The predominant bacteria of bioaerosols were Variovorax (9.44%), Parvibaculum (8.27%), Pseudonocardia (8.04%), Taonella (5.74%), Arthrospira (4.58%), Comamonas (3.84%), Methylibium (3.13%), Sphingobium (4.46%), Zoogloea (2.20%), and Sphingopyxis (2.56%). ESKAPEE pathogens, such as Pseudomonas, Acinetobacter, Staphylococcus, Enterococcus, and Escherichia, were also found in lower abundances. The fungi were represented by Wilcoxinia rehmii (64.38%), Aspergillus ruber (9.11%), Penicillium desertorum (3.89%), Leptobacillium leptobactrum (3.20%), Humicola grisea (2.99%), Ganoderma sichuanense (1.42%), Malassezia restricta (0.74%), Heterophoma sylvatica (0.49%), Fusarium proliferatum (0.46%), and Saccharomyces cerevisiae (0.23%). Some common and unique operational taxonomic units (OTUs) of bacteria and fungi were also recorded at each site; this inter-site variability shows that exhaled air can be a source of this variation. The alpha-diversity indices suggested variance in species richness and abundance in hospitals than in non-hospital sites. The community structure of bacteria varied spatially (ANOSIM r2 = 0.181–0.243; p < 0.05) between the hospital and non-hospital sites, whereas fungi were more or less homogenous. Key taxa specific to the hospitals were Defluvicoccales, fungi, Ganodermataceae, Heterophoma, and H. sylvatica compared to Actinobacteria, Leptobacillium, L. leptobacillium, and Cordycipitaceae at the non-hospital site (LefSe, FDR q ≤ 0.05). The hospital/non-hospital MD index > 1 indicated shifts in the microbial communities of indoor air in hospitals. These findings highlight the need for regular surveillance of indoor hospital environments to prevent future outbreaks.
Introduction
With the outbreak of COVID-19 and evidence of pandemic spread via air droplets, bioaerosol research has picked up momentum (Babin, 2020; Faridi et al., 2020; Lednicky et al., 2020; Li et al., 2020; Liu et al., 2020; Santarpia et al., 2020; Tan et al., 2020; Van Doremalen et al., 2020; Zhou et al., 2020; Habibi et al., 2021a,c, d, 2022a; Li, 2021; Tellier, 2022). A diverse microbial assemblage, including bacterial, fungal, and viral communities and pollens, is an integral part of bioaerosols (Prussin and Marr, 2015; Prussin et al., 2015; Habibi et al., 2022b). Studies have shown the prevalence of SARS-CoV-2 co-infection with non-coronavirus respiratory pathogens (Kim et al., 2020; Abbas et al., 2021; Habibi et al., 2021d; Karimzadeh et al., 2021). Hospitals being critical infrastructure for patient care, house patients with the compromised immune system and higher susceptibility to infection are at higher risk (Macintyre et al., 2014; Stockwell et al., 2019). Hospital-mediated outbreaks are not new (O’connor et al., 2015; Lax et al., 2017; Park et al., 2018; Weber et al., 2019; Mendes et al., 2022). More recently, hospitals were the hot spots for the novel SARS-CoV-2 spread through airborne transmission (Duverger et al., 2021; Løvestad et al., 2021; Paltansing et al., 2021), and reports of individuals involved in patient care getting infected and reinfected were not uncommon. Hence, characterization of the indoor aerosols is vital, as these microbes enter the human respiratory system leading to both chronic responses due to prolonged exposure and acute responses upon inhalation in case of compromised immune systems. Microbial inhalation may cause hypersensitive responses, asthma, allergic reactions, pulmonary infections, and toxicosis (Jiayu et al., 2019; Gevao et al., 2013, 2022).
However, the low concentration of microbial load in aerosol makes their identification an arduous task (Gregson et al., 2021; Uddin et al., 2022) that often does not get immediate attention. The microbial identification becomes further challenging due to matrix interference, physical damage, and crumbling due to the high-pressure collection of aerosol samples (Fennelly et al., 2015; Yao et al., 2021). Next-generation sequencing (NGS) has provided an effective solution for providing information on the bacterial, fungal, and viral communities in samples with high precision and accuracy (Chakrawarti et al., 2020; Habibi et al., 2021b). Next-generation sequencing has an advantage over the conventional culture-based microbial identification method that neglects the important indoor microorganisms that develop a viable but non-cultivable state (Muthuirulan and Sharma, 2017; Tong et al., 2017; Gao et al., 2018; He et al., 2020). The 16s amplicon sequencing method has been applied for bacterial community profiling from indoor aerosols (Chen et al., 2017; Chakrawarti et al., 2020; Behbehani et al., 2021a; Lee et al., 2021), particulate matter in the air (Habibi et al., 2021e), and dust (Al Salameen et al., 2020; Li et al., 2021), among other matrixes. The targeted sequences of the internal transcribed spacer (ITS) region have also been sequenced to gain information on the fungal communities within air samples (Okten and Asan, 2012; Al Salameen et al., 2020; Behbehani et al., 2021a,b; Lee et al., 2021; Salameen et al., 2021). Additionally, the 16S rRNA gene studies have extended our understanding of the functional contribution of individual community members (Shobo et al., 2020).
Besides the fact that the dispersal of microbial communities between humans and the surrounding environment through airborne release is a key medium to intercede outbreaks, the role of bioaerosols in the spread of pathogens remains largely unexplored in the region. Predominant viral communities were identified in the Kuwait’s hospital during the COVID-19 pandemic (Habibi et al., 2021b,e,g,2022a). Bacterial and fungal cells were also present, suggesting their roles in the spread of respiratory pathogens to other locations (Habibi et al., 2021a,c). This triggered an interest to map the bacterial and fungal genera through high-throughput molecular studies. The current study was aimed at an assessment of the indoor aerosol in two major hospitals (Mubarak Al Kabeer and Sheikh Jaber) in Kuwait involved in COVID-19 patient care and a non-hospital location. The characterization of bacterial communities was done using 16s rRNA gene and fungal communities using ITS region sequencing. The baseline microbial diversity in indoor air was compared between sites. The findings will improve the understanding of the bacterial and fungal populations present within the confined spaces in hospitals and aid to formulate strategies to protect healthcare personnel and uninfected people involved in patient care.
Materials and methods
Sampling site and aerosol collection
The indoor aerosol samples were collected between August and October 2020 amidst the height of COVID-19 lockdown after obtaining permission from the Ministry of Health, Kuwait. The aerosol samples were collected from Mubarak Al Kabeer (MKH) and Sheikh Jaber (SJH), involved in treating COVID-19 patients in Kuwait City and in a non-hospitalized setting at the Kuwait Institute for Scientific Research (KISR). Additional details are provided in Table 1. A custom-made sampling device was used to collect ambient air samples owing to the ongoing pandemic; aerosol was drawn at 30 L min–1 for 2 h collecting 3.6 m3 of aerosol (Habibi et al., 2021a). The collected bioaerosols were concurrently lysed while sampling to avoid SARS-CoV-2 dissemination. Trizol was used as a lysis and transport reagent to the laboratories to extract nucleic acid under BSL2 cabinets.
RNA extraction and cDNA conversion
The standard procedure of nucleic acid isolation from Trizol™ reagent (APB, Biosciences, Rockville, MD, United States) was followed to purify total RNA (Habibi et al., 2021d). The aerosols collected in Trizol™ were left at room temperature for 10 min, and the crude RNA was separated by adding 0.2 volumes of chloroform (Sigma Aldrich, WGK, Germany). The aqueous chloroform layer was transferred to 0.5 volumes of isopropanol (Merck, Darmstadt, Germany) to precipitate the RNA and subsequently washed with 70% ethanol (Merck, Rahway, NJ, United States) twice. The procedure is described in detail by Habibi et al. (2021a). The RNase-free water (Ambion, Austin, TX, United States) was used to dissolve the air-dried pellet. The high-sensitivity Qubit, HS ssRNA kit, was used to isolate RNA. The fluorometric estimation of isolated RNA was performed on a Qubit 4 Fluorometer (Thermo Scientific). The extracted total RNA was converted to complementary deoxyribonucleic acid (cDNA) using iScript™ Reverse Transcription Supermix (Bio-Rad, Darmstadt, Germany) for bacterial and fungal sequencing. The reaction mix was assembled by adding 18 μl of master mix to 2 μl of RNA and incubated for 5 min at 20°C (priming), 20 min at 46°C (revere transcription), and 1 min at 95°C (RT inactivation) (Li et al., 2017).
Targeted amplicon (16S rDNA/ITS) sequencing
The cDNA was used for PCR amplification by employing 16Sr RNA primers (515F-806R) targeting the V4 region of bacteria and 18S rRNA primers (528F-706R) targeting the internal transcribed spacer (ITS1 and ITS 2) region of fungi (Li et al., 2017). Phusion High-Fidelity PCR Master Mix (New England Biolabs) was used for carrying out all PCR reactions. The libraries were generated with NEBNext UltraTM DNA Library Prep Kit (New England BioLabs, France). Fluorometry (Qubit, Invitrogen, Santa Clara, CA, United States) and qPCR (QuantStudio 5 Real-Time PCR System, Applied Biosystems, Thermo Scientific, Paisley, United Kingdom) were used for library quantification and quality estimation. High-quality, purified libraries were sequenced on an Illumina HiSeq 2500 (San Diego, CA, United States) with 2 × 250 cycle chemistry. Paired-end reads were de-multiplexed, truncated (to remove the adapter and primer sequences), and merged using FLASH (V1.2.7) (Magoè and Salzberg, 2011). The raw tags thus generated were filtered to obtain high-quality clean tags employing QIIME (V1.7.0) (Caporaso et al., 2010). The de novo chimera removal method was implemented in UCHIME (version 11) (Edgar et al., 2011). Based on their sequence similarity, pre-processed reads from all samples were pooled and clustered into operational taxonomic units (OTUs). The sequence similarity was established using the Usparse v7.0.1090 program (similarity cutoff = 0.97) and queried against the SSUrRNA database of SILVA Database (Quast et al., 2012).
Bioinformatics and statistical analysis
Alpha- and beta-diversity indices were calculated with QIIME (Version 1.7.0) and displayed with R software (Version 2.15.3) (Caporaso et al., 2010). A pairwise comparison of alpha-diversity indices was made through the analysis of variance (ANOVA) method at a confidence interval of 95% (p ≤ 0.05). Cluster analysis was preceded by principal component analysis (PCA), which was applied to reduce the dimension of the original variables using the FactoMine R package and ggplot2 package in R software (Version 2.15.3). Analysis of similarity (ANOSIM) coefficients was calculated on weighted Unifrac distance. LEfSe analysis was conducted by LEfSe software (Segata et al., 2011). The p-value and q-value were calculated by the permutation test method and Benjamini and Hochberg false discovery rate method, respectively (White et al., 2009). The SPARCC correlation network analysis employed Kendall’s correlation test (threshold: 0.3, 100 permutations) (Watts et al., 2019). Functional annotation based on the 16S rRNA gene was done through Tax4Fun software on Silva taxonomies (Wemheuer et al., 2018). The microbial dysbiosis index (MD index) was calculated based on differentially abundant OTUs between H1, H2, and G2 (total abundance of OTUs increased in site 1) and the total abundance of OTUs decreased in site 2 (Soares et al., 2016).
Results
In the current investigation, the bacterial and fungal populations present within the indoor aerosols of two major hospitals in Kuwait were mapped. Targetted amplicon sequencing was performed to achieve this. High-quality libraries of 13 and 16 samples for bacteria and fungi were obtained. Libraries with compromised quality (n = 3; two from the H1 group and one from the G2 group) were omitted for further processing. Paired-end sequencing of the 16S rRNA and ITS regions generated approximately 21 million bacterial (639,858,259 bp) and 23 million fungal (646,493,926 bp) reads. More than 94% of bases had a Q score > 30 (Supplementary Tables 1, 2). The effective% ranged between 62–82% and 53–97% for bacterial and fungal sequences, respectively. Data rarefaction was performed to check the normality of sequenced reads. All the bacterial and fungal sequences plateaued at ca. 10,000 bp, and therefore no exclusions were made during data analysis (Supplementary Figures 1, 2). The filtered bacterial and fungal reads were processed to obtain tags that eventually yielded the OTUs (Supplementary Figures 3, 4). The data set was further explored for taxonomies, spatial variations, alpha–beta diversity analysis, network analysis, and functional prediction.
Taxonomic composition of bioaerosols
The baseline taxonomic composition of the bioaerosols was examined to identify the predominant forms. Considering the long list of taxa in the descending order of abundance, only the details of the first 10 taxa are presented in more detail. The complete classification of each OTU is provided in Supplementary Tables 3, 4.
Majority of the 16S sequences aligned with the (Figure 1A) phyla Proteobacteria (72.54%), followed by Actinobacteria (11.39%), Cyanobacteria (6.35%), Verrumicrobiota (4.19%), Firmicutes (2.19%), and Bacteroidota (2.09%). The classes of these phyla were represented by Alphaproteobacteria (38.66%) > Gammaproteobacteria (33.88%) > Actinobacteria (8.6%) > Cyanobacteriia (6.35%) > Verrumicrobiae (4.19%) > Thermoleophilia (2.75) > Bacteroidia (2.09%) > Bacilli (1.57%) > Clostridia (0.6%) and Phycisphaerae (0.33%). The classes were further classified into orders, such as Burkholderiales (26.44%), Sphingomonadales (8.48%), Parvibaculales (8.33%), Pseudonocardiales (6.48%), Ferrovibrionales (5.23%), Chthoniobacterales (4.16%), Defluviicoccales (3.84%), Reyranellales (3.75%), and Cyanobacteriales (3.56%). The most prevalent families were Commmonadaceae (20.59%), Sphingomonadacae (8.48%), Parvibaculaceae (8.33%), Pseudomonadaceae (6.48%), Terrimicrobiaceae (4.16%), Reyranellaceae (3.75%), Phormidiaceae (3.56%), Xanthobacteraceae (2.96%), Solirubrobacteraceae (2.68%), and Rhodocyclaceae (2.47%). At the genus level (Figure 1B), Variovorax (9.44%), Parvibaculum (8.27%), Pseudonocardia (8.04%), Taonella (5.74%), Arthrospira (4.58%), Comamonas (3.84%), Methylibium (3.13%), Sphingobium (4.46%), Zoogloea (2.20%), and Sphingopyxis (2.56%) were among the highly abundant ones. Besides these, the known pathogenic forms abbreviated as ESKAPEE (Enterococcus faecium, Staphylococcus aureus, Klebsiella pneumoniae, Acinetobacter baumannii, Pseudomonas aeruginosa, Enterobacter spp., and Escherichia coli) were also looked at. Among these, Pseudomonas (0.048), Staphylococcus (0.049), Acinetobacter (0.017), and Escherichia (0.002) were found in low abundance (Figure 1C).
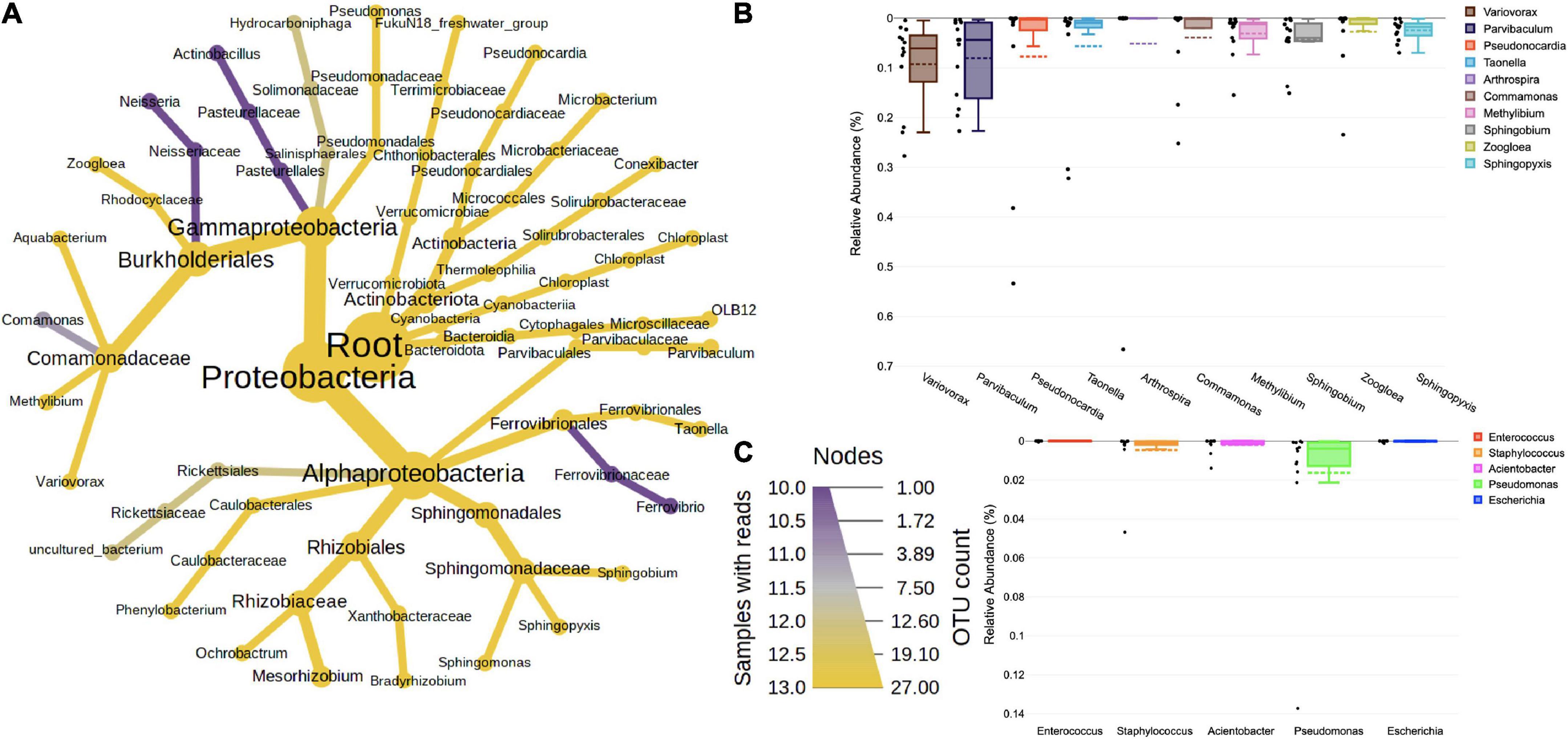
Figure 1. (A) Taxonomic composition of bacteria in bioaerosols. The Wilcoxon rank test (p < 0.05) was applied to median abundances of bacterial OTUs to create the differential abundance tree. The nodes represent the bacterial taxon. The size of the node denoted the OTU counts, and the color represents its presence in a number of samples. A key to the OTU count and sample prevalence is provided in the right-hand side corner. Box plots showing RA of (B) top 10 dominant bacterial genera and (C) ESKAPE pathogens. The RA are plotted on the Y-axis and the corresponding genera on the X-axis. Each box represents the inter-quartile range (25–75%), upper and lower whiskers (10–90%), and dashed lines are the mean RA%. Black dots represent the RA of the individual sample.
The ITS sequencing analysis provided information on diverse fungal taxa. The entire fungal classification up to species level was presented on a heated tree (Figure 2A). The fungal phyla Ascomycota (88%) was the most dominant in bioaerosols and was followed by Basidiomycota (2.72%). The leading classes of fungi were Pezizomycetes (63.98%), Eurotiomycetes (14.95%), Sordariomycetes (7.69%), Agaricomycetes (1.79%), Malasseziomycetes (0.94%), Dothideomycetes (0.78%), Saccharomycetes (0.38%), Letiomycetes (0.20%), Mucoromycetes (0.01%), and Mortierellomycetes (0.01%). Further classification at order level revealed the presence of Pezizales (63.98%), Eurotiales (14.83%), Hypocreales (4.07%), Sordariales (3.04%), Polyporales (1.65%), Malasseziales (0.94%), Pleosporales (0.55%), Saccharomycetales (0.38%), Glomerellales (0.37%), and Capnodiales (0.23%). Orders were distributed into families, namely Pyronemataceae (62.87%), Aspergillaceae (14.83%), Cordycipitaceae (2.85%), Chaetomiaceae (2.82%), Ganodermataceae (1.27%), Necteriaceae (1.17%), Malasseziaceae (0.94%), Didymellaceae (0.52%), Phanerochaetaceae (0.38%), and Glomerellaceae (0.31%). The predominant genera and their species were Wilcoxinia rehmii (64.38%), Aspergillus ruber (9.11%), Penicillium desertorum (3.89%), Leptobacillium leptobactrum (3.20%), Humicola grisea (2.99%), Ganoderma sichuanense (1.42%), Malassezia restricta (0.74%), Heterophoma sylvatica (0.49%), Fusarium proliferatum (0.46%), and Saccharomyces cerevisiae (0.23%) (Figure 2B). The species of Penicillium, Aspergillus, Fusarium, and Saccharomyces cause diseases in humans, plants, and animals.
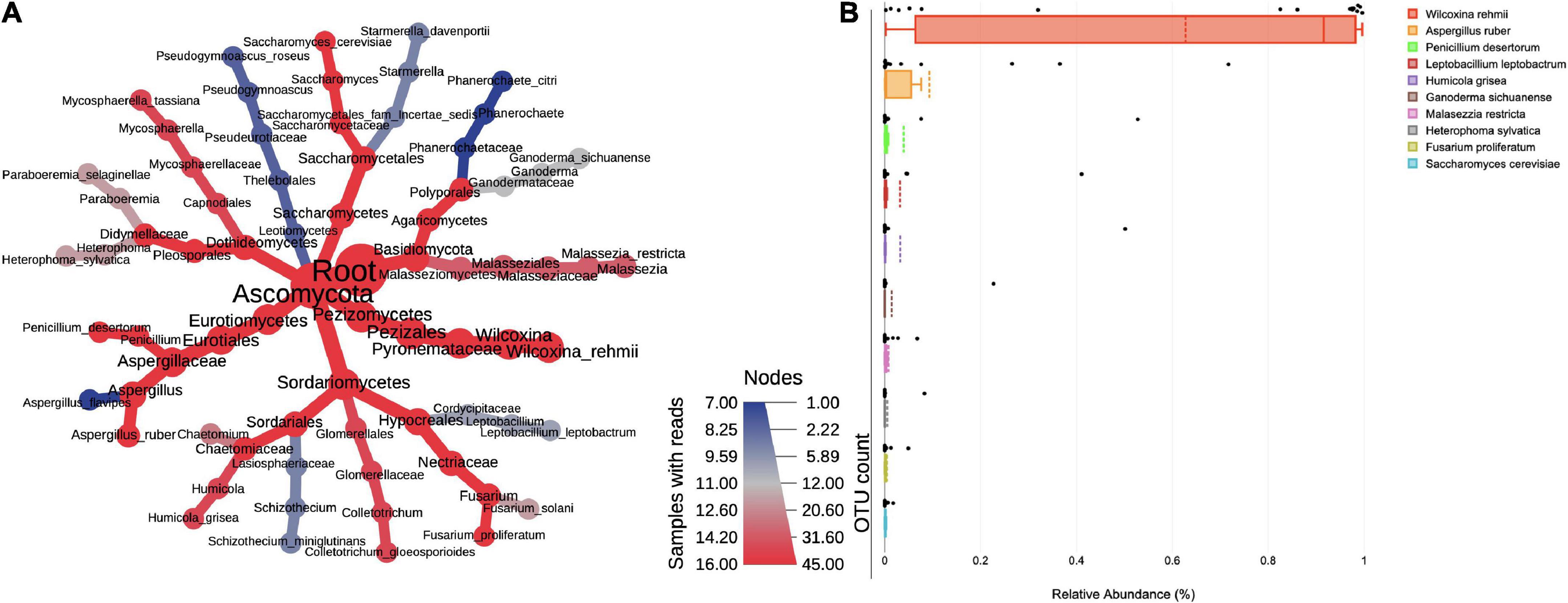
Figure 2. (A) Taxonomic composition of fungi in bioaerosols. The Wilcoxon rank test (p < 0.05) was applied to median abundances of fungal OTUs to create the differential abundance tree. The nodes represent the fungal taxon. The size of the node denoted the OTU counts and the color represents its presence in a number of samples. A key to the OTU count and sample prevalence is provided in the right-hand side corner. (B) Box plots showing RA of top 10 dominant fungal species. The RA values are plotted on the Y-axis and the corresponding genera on the X-axis. Each box represents the inter-quartile range (25–75%), upper and lower whiskers (10–90%), and dashed lines are the mean RA%. Black dots represent the RA of the individual sample.
Spatial variations in bacterial and fungal communities
The infrastructure and geographic location of each hospital differed, and the likelihood of microbes unique to each site was much expected. To prove this, a comparison of the RA of prevalent microbes in two hospitals (H1 and H2) and the non-hospital (G2) site was performed in the first place (Figure 3A). In bacteria, the phylum Proteobacteria was highest in abundance at H1, H2, and G2. Maximum RA (83.0%) was recorded at H1, followed by H2 and G2 (ca. 62.0%). The second in the list at H1 was phylum Verrumicrobiota (5.3%), followed by Actinobacteria (4.3%) > Bacteriodota (1.9%) > Cyanobacteria (1.3%) > Firmicutes (1.2%). H2 followed a similar trend to H1. The order of abundance at G2 differed with Actninobacterota (24.6%) > Firmicutes (3.9%) > Cyanobacteria (3.0%), Verrumicrobiota (2.4%). The fungal phyla varied in their RA, but their order of dominance was almost equal in all the sites. The RA of Ascomycota (Figure 3B) was strikingly higher at H1 and H2 (ca. 90%). At G2, it was 82.0%. The next phylum in the order of abundance at all the locations was Basidiomycota (H1: 4.65%, H2: 0.4%, and G2: 2.0%). This was followed by Mortierellomycota (<0.001%). Variations in RA at the lower taxonomic levels of class, order, family, genus, and species were also recorded. The description of bacterial genera and fungal species specific to each hospital is described in the following text.
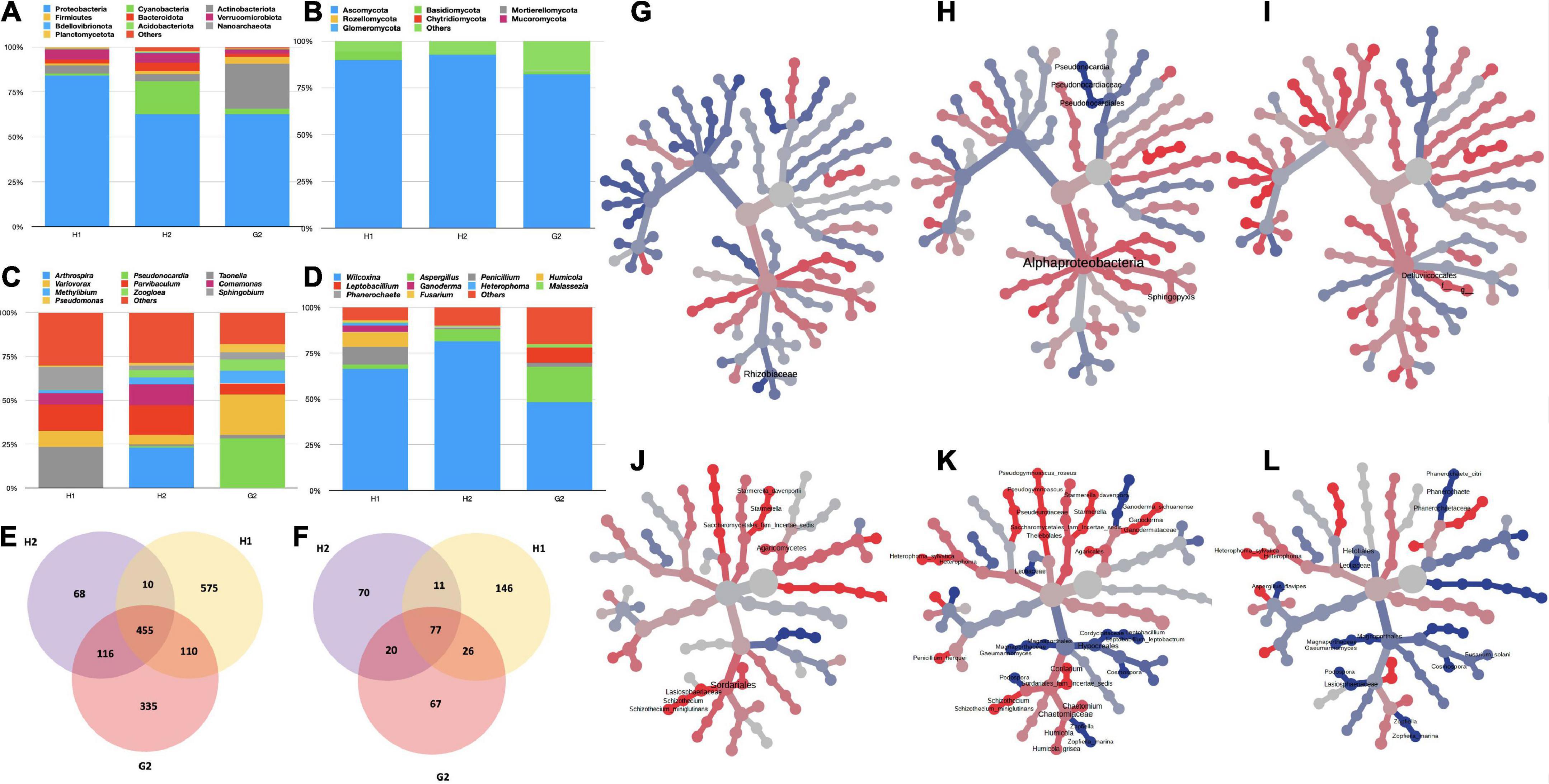
Figure 3. Variation in RA% of bacterial and fungal taxa in hospitalized (H1 and H2), and non-hospitalized (G2) settings. (A) Variations in bacterial phyla; (B) variations in fungal phyla; (C) variations in bacterial genera; and (D) variations in fungal genera. For all the bar plots, the groups are plotted on the x-axis, and relative abundances (RA) are shown on the y-axis. The color key to each taxon is presented on the top of each bar graph. OTU Clustering analysis: (E) Common and unique bacterial OTUs; (F) common and unique fungal OTUs. Differential abundance analysis (G) between bacterial taxa of H1 and H2; (H) between bacterial taxa of H1 and G2; (I) between bacterial taxa of H2 and G2; (J) between fungal taxa of H1 and H2; (K) between fungal taxa of H1 and G2; and (L) between fungal taxa of H2 and G2. The Wilcoxon rank test (p < 0.05) was applied to median abundances of OTUs to create the differential abundance tree.
Among the bacterial genera (Figure 3C), at H1 Taonella (15.4%) was maximum, followed by Parvibaculum (9.6%), Sphingobium (8.5%), Variovorax (5.9%), Comamonas (4.3%), Methylibium (1.2%), Pseudomonas (0.43%), Zoogloea (0.16%), Pseudonocardia (0.11%), and Arthrospira (0.0%). At H2, Arthrospira (13.9%) was the highest in abundance with Parvibaculum (10.3%) at second place, followed by Comamonas (7.1%), Variovorax (3.2%), Methylibium (2.2%), Zoogloea (2.5%), Sphingobium (1.5%), and Pseudomonas (1.0%). Unlike the hospital locations, at G2, Pseudonocardia (19.1%) was highest, followed by Variovorax (15.6%), Methylibium (4.8%), Parvibaculum (4.2%), Pseudomonas (2.7%), Sphingobium (0.27%), and Taonella (1.2%) in the order of their RA. The fungal genera also varied in RA at all three sites (Figure 3D). The genus Wilcoxinia was highest at all three sites (H1: 64.3%, H2: 81.0%, and G2: 47.5%). The second-most abundant fungal genera at H1 were Penicillium (9.4%), followed by Humicola (7.6%), Ganoderma (3.65), and Heterophoma (1.2%). At H2, Aspergillus was the second-highest level and was followed by Penicillium. The G2 had Aspergillus (19.0%) and Leptobacillium (8.1%) in second and third places, respectively.
It was important to see the variations in the abundance of ESKAPEE genera, as potential outbreaks caused by some of their species may cause serious consequences. The RA of ESKAPE genera also varied according to the sampling location. Enterococcus was only detected at H1. Acinetobacter exhibited a maximum RA of 2.1% at G2 and 0.2% at H1 and H2. The genus Pseudomonas (15.8%) was maximum at G2. Its abundance at H1 and H2 was 1.7 and 3.7%, respectively. Staphylococcus was almost comparable at H1 and H2, whereas its abundance was recorded as 5.3% at G2 (Table 2). Genus Escherichia was recorded both at H1 and H2; however, at G2 it was negligible. Fungal species of W. rehmii were recorded at H1 (66.4%), H2 (82%), and G2 (48.8%). A noticeable abundance of A. ruber (18%) was recorded at G2 and H2 (6.7%). At H1, P. desertorum (8.9%) was the next in abundance after W. rehmii (Table 2).
Additional analyses were performed to compare the inter-site variability among the bacterial and fungal taxa through OTU clustering. The common and unique features were recorded at each sampling site. We discovered 455 common OTUs (bacterial) in the aerosols and 575, 68, and 335 OTUs unique to H1, H2, and G2, respectively (Figure 3E). There was 10 OTUs common between H1 and H2, 110 were common between H1 and G2, and 116 were common between H2 and G2. In the case of fungi, 146, 67, and 70 OTUs were unique to H1, H2, and G2 respectively. Seventy-seven were common between all the sites, with 11 common between H1 and H2, 26 common between H1 and G2, and 20 common between G2 and H2 (Figure 3F).
Pairwise comparison of differential abundance through Wilcoxson’s sum test (p > 0.05) also returned significantly variant taxa among the three sites. In the bacterial category, the family Rhizobiaceae differed between H1 and H2 (Figure 3G). Similarly, class Alphaproteobacteria, order Pseudonocardiales, family Pseudonocardiaceae, and genera Pseudonocardia and Sphingopyxis differed between H1 and G2 (Figure 3H). The single-order Defluvicoccales varied significantly between H1 and G2 (Figure 3I). The fungal taxa exhibited more variations between the three sites (Figures 3J–L). A total of 8, 35, and 17 taxa were significantly differentially abundant among H1-H2, H1-G2, and H2-G2 groups, respectively.
Intra-site variability
The RA of bacterial genera varied within the sub-locations of H1, H2, and G2 (Supplementary Table 5). 16S rRNA results were obtained for four locations within H1 and H2, and five in G2. The top 10 genera were compared for variations in their RA within each site (Figure 4A). At H1, Taonella was most abundant in MKE (area near the main entrance) and MKP (reception of the main pharmacy). The RA of Sphingobium was also high when compared to other genera found at MKE and MKP. The genus Parvibaculum was maximum in abundance at MKPC (pediatric causality ward) and MKCW (COVID-19 isolation ward). The second-most abundant genera in the same places were Variovorax. At H2, genus Parvibaculum was highest at SJL1 (cytology laboratory), SJL2 (virology laboratory), SJCW (COVID ward), and SJCO (COVID-observation ward). The next dominant genus at all these sub-locations in H2 was Variovorax. In the case of G2, Variovorax was more commonly found in KGR1 (main reception), KGR2 (reception near the canteen), KFF1 (corridor on the first floor near the lift), KFF2 (corridor near the staircase and electrical unit), and KL1 (the area inside the COVID research laboratory). Actinobacillus was high at KFF1. In general, it was observed that the G2 bacterial genera exhibited more variations when compared to H1 and H2. This could be attributed to the similar air-conditioning, ventilation system, and sanitization followed in the hospitals when compared to G2.
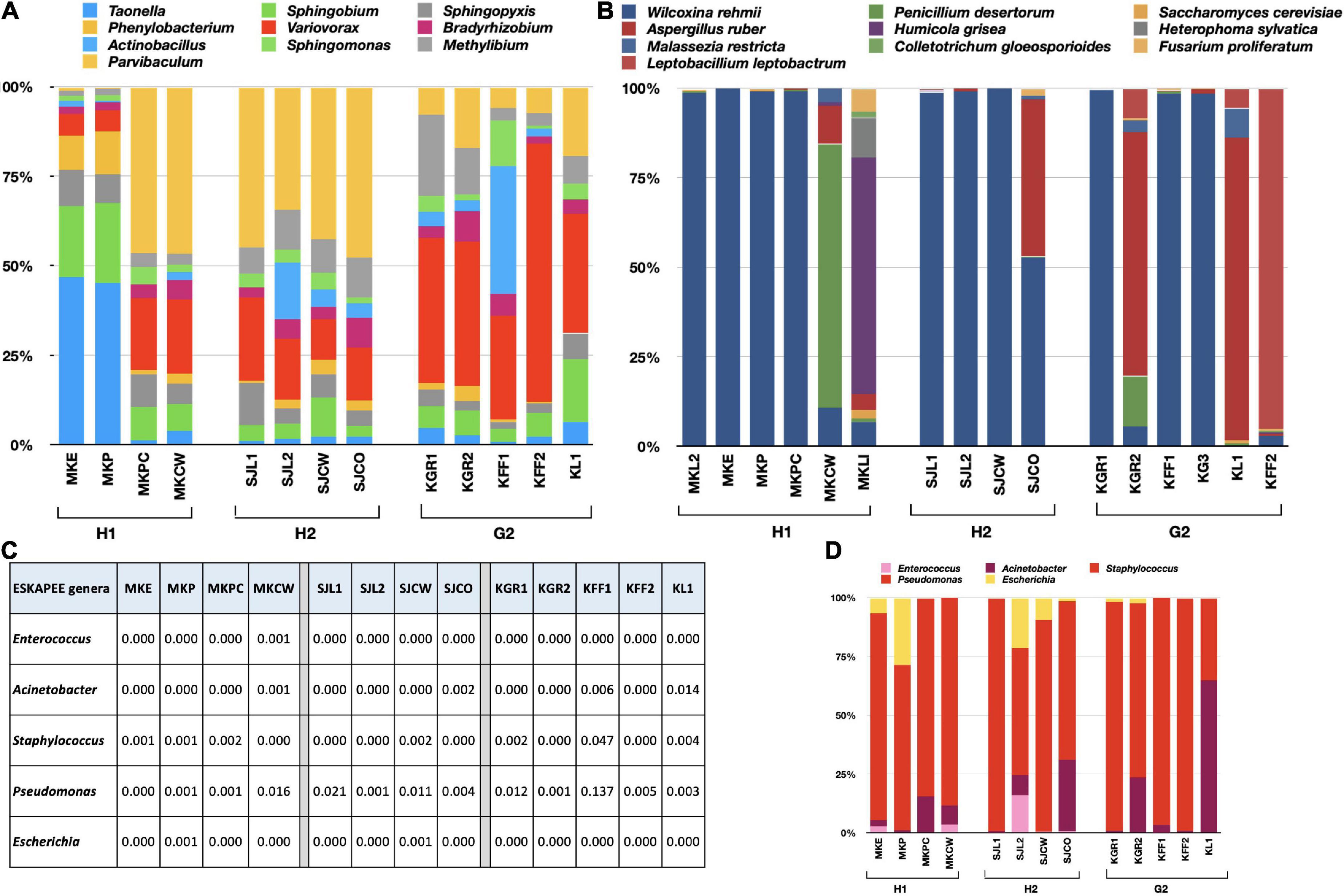
Figure 4. Bar charts showing the variations of RA within each sub-location of H1, H2, and G2. (A) Bacterial genera, (B) fungal species, (C) RA of ESKAPEE genera, and (D) bar chart showing variations in RA of ESKAPEE genera. The RA is plotted on the y-axis and the corresponding taxa on the x-axis.
The ITS sequences of six sub-locations of H1 and G2, and four sub-locations of H2 also exhibited variations in RA of fungal species (Supplementary Table 6). W. rehmii dominated at MKL2, MKE, MKP, and MKPC, whereas P. desertorum and H. grisea were overwhelmingly higher at MKCW and MKL1 (Figure 4B). The remaining species exhibited very low abundances. At H2, except at SJCO (maximum prevalence of A. ruber), again W. rehmii was highest at SJL1, SJL2, and SJCW. At G2, either W. rehmii (KGR1, KFF1, and KG3) or A. ruber (KGR2, KL1, and KFF2) were among the highly prevailing fungal species. These results were in congruence with the findings of the alpha-diversity analysis.
Variations in ESKAPEE genera were also looked at carefully, and similar patterns in RA abundances were recorded (Figure 4C). Enterococcus was sparingly present at MKCW and MKE, and was absent from all other locations of H1. At H2, its presence was only detected at SJL2. It was not detected at all at G2. In the case of Acinetobacter, exceptionally high abundances were recorded at KL1 (G2), whereas RA > 0.001 was recorded at other sub-locations of G2, H2, and H1. Staphylococcus was more at MKE, MKP, and MKPC within H1. Its abundance was comparable at MKCW, SJL1, SJL2, SJCO, SJCW, KGR1, KGR2, KFF1, KFF2, and KL1. Pseudomonas species were overwhelmingly high among the ESKAPEE genera. Its presence was recorded within all the sub-locations of H1, H2, and G2. On average, it was more at G2 (KGR1, KGR2, KFF1, KFF2, and KL1). Escherichia was only found at MKE and MKP within H1; SJL2, SJL2, SJCW, and SJCO within H2; and at KGR1 and KGR2 within G2 (Figure 4D). Pairwise comparisons through student’s t-test revealed p-values above 0.05, rendering the variations insignificant.
Alpha- and beta-diversity analysis
Each hospital had a unique infrastructure, most likely contributing to differences within and among the sites. To confirm this, the alpha- and beta-diversity analyses were performed. Intra-site diversity was assessed using alpha-diversity indices of observed species, ACE, Chao1, Shannon’s index, Simpsons, and Fisher index. Over 99% of all the samples had adequate biodiversity captured at each site (Supplementary Tables 7, 8). Data rarefaction was also performed with a plateau obtained at 10,000 sequences for bacteria (Supplementary Figure 3) and fungi (Supplementary Figure 4), indicating that sufficient diversity was captured through sequencing. For bacterial species, the highest diversity was at G2, followed by H2 and H1 (Figure 5A). Chao1 and ACE were comparable at G2 and H1 with slightly lower values at H2 (Figures 5B,C). The Shannon and Simpson were highest at H2, with the second-highest numbers at H1 and H2 (Figures 5D,E).
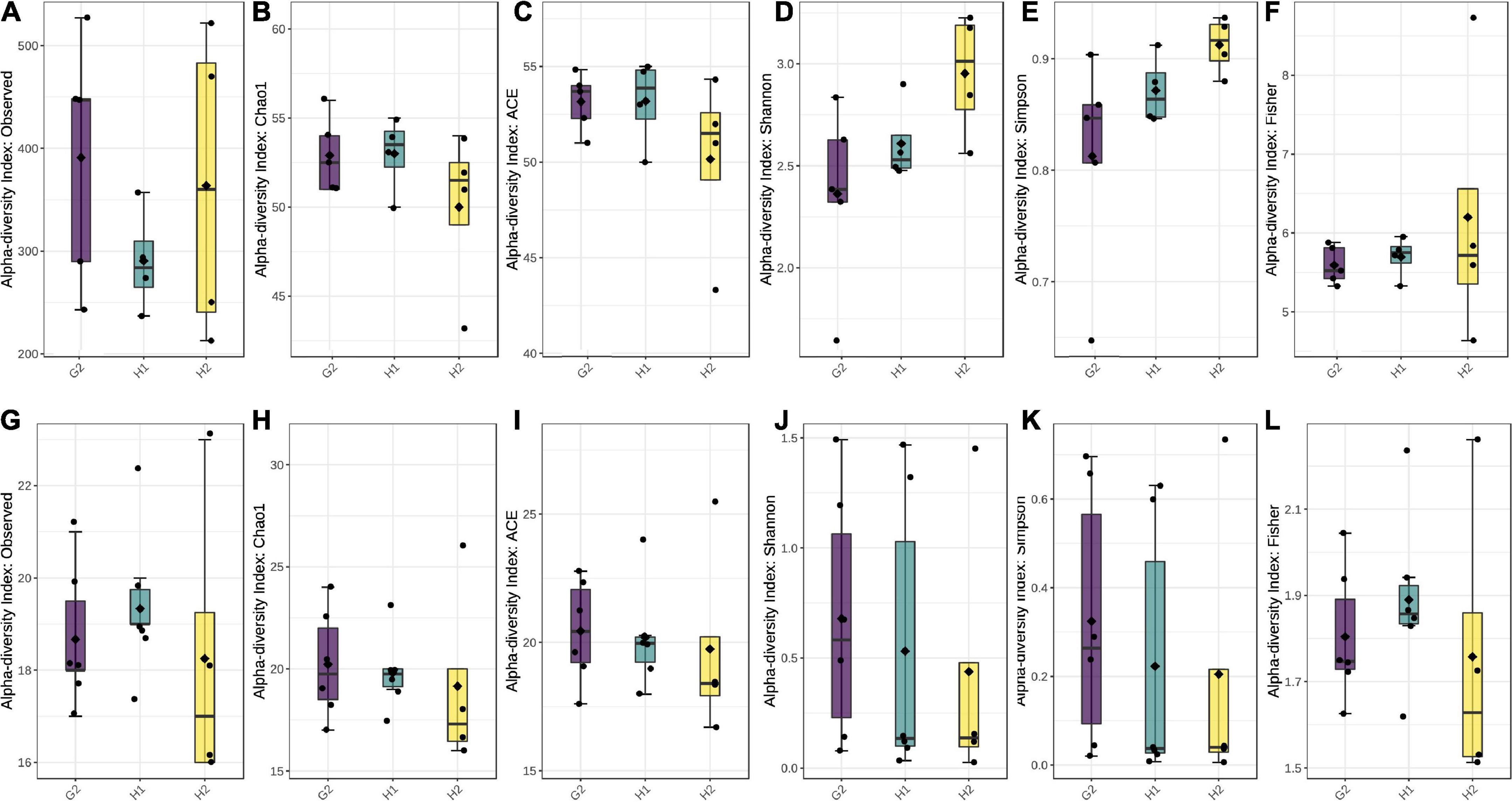
Figure 5. Box plots with alpha-diversity indices: (A–F) for bacterial species and (G–L) for fungal species. The boxes represent the range of alpha diversity within each location. Corresponding alpha-diversity indices (observed, Ace, Chao1, Shannon’s, Simpson, and Fisher) are plotted on Y-axis. All the comparisons were done employing Tukey’s and Wilcox’s tests at p ≤ 0.05.
Fisher values were almost comparable at all three locations (Figure 5F). A comparison of all the alpha-diversity indices by Tukey’s and Wilcox test revealed p-values > 0.05, indicating that within-site diversity was not significant. This could be attributed to the similar interior settings within each sampling location.
In the case of fungi, all the alpha-diversity indices, except for observed and Fisher, were highest at G2, followed by H1 and H2 (Figures 5G–L). The observed species were highest at G2 and lowest at H2. Likewise, Fischer indices were highest at H1 and second-highest at G2, followed by H2 (Figures 5J–L). This indicates both species richness and evenness of the fungal population to be higher in ambient air than in indoor aerosol. Among the indoor site, the non-hospital settings (G2) had comparatively higher fungal counts than hospital settings (G1). Unlike the bacterial population, the fungal alpha-diversity indices of observed, Chao1, and Fischer were significant at p < 0.05 compared with Tukey’s and Wilcox test.
The differences in community structure were analyzed through the beta-diversity analysis. The clustering of Bray-Curtis distances of bacterial communities distributed the samples into three overlapping groups. As evident from Figure 6A, the H1 and H2 communities lay close, whereas the G2 was slightly apart. Permutational multivariate analysis of variance (Adonis) was performed on UniFrac; distances between H1-H2-G2 (indoor aerosols of hospitalized and non-hospitalized settings) showed the difference in the community to be moderate but significantly diverse (r2 = 0.117; p = 0.046) (Figure 6C). The PCA analysis showed the fungal communities at H1 and H2 to be closer but distantly apart from G2 (Figure 6B). The Adonis coefficients were 0.069 and demonstrated insignificant p-values (p = 0.263) (Figure 6D). Pairwise Unifrac distances between samples are provided in Supplementary Figures 5, 6.
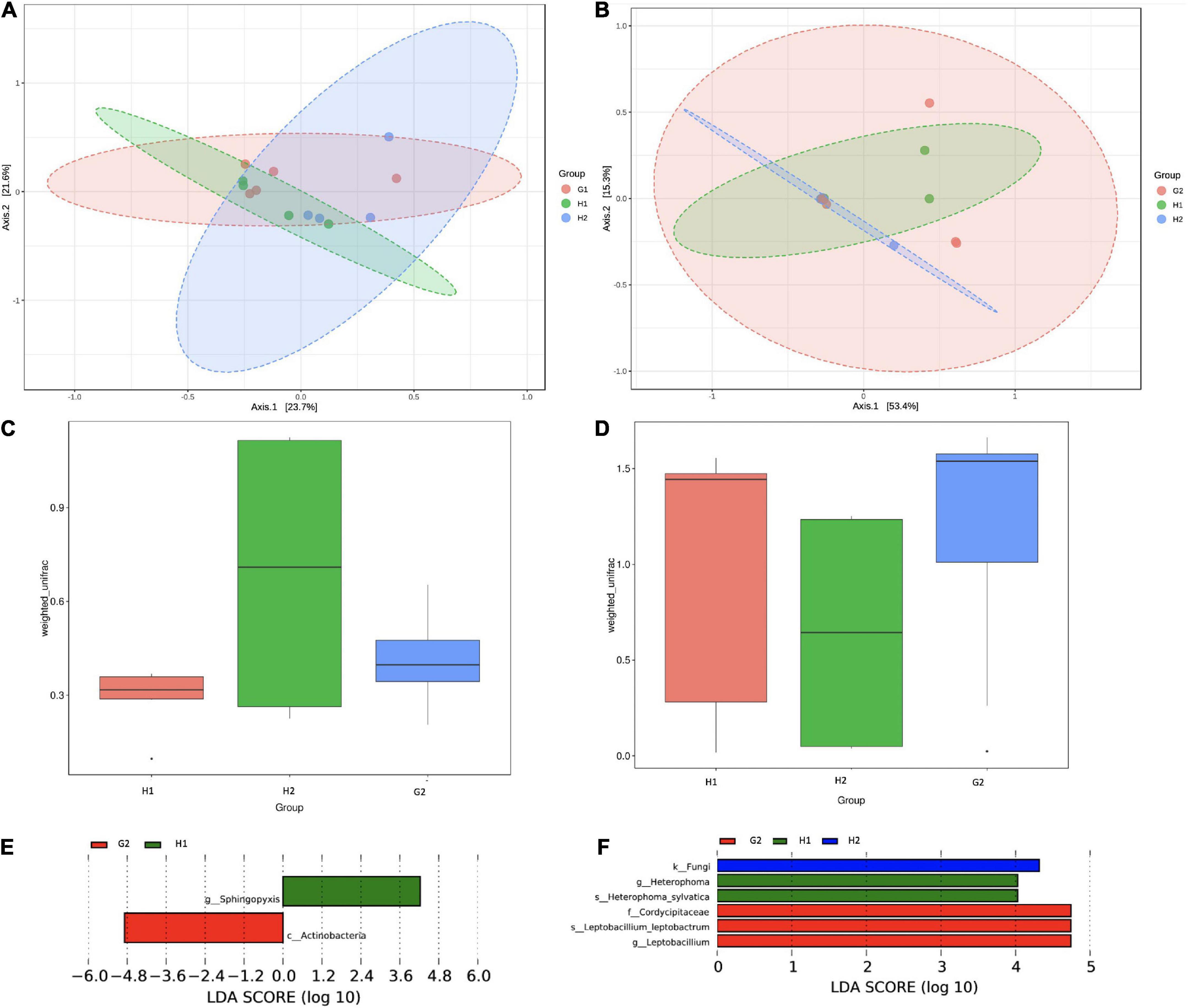
Figure 6. Beta-diversity analysis of microbial communities of indoor aerosols of hospitalized and non-hospitalized settings in Kuwait. (A) PCA plot of bacterial communities, (B) PCA plot of fungal communities, (C) ANOSIM analysis of bacterial community, (D) ANOSIM analysis of fungal communities, (E) LDA analysis of bacterial taxa, and (F) LDA analysis of fungal taxa. LDA scores are plotted on the x-axis on a logarithmic scale. The corresponding taxa are shown on the Y-axis. The LEfSe algorithm applying the non-parametric factorial Kruskal–Wallis (KW) sum-rank at an FDR (q) ≤ 0.05 followed by the linear discriminant analysis (LDA) was employed to select significantly differential taxa.
The linear discriminant analysis was performed to identify key indicator taxa between H1-H2-G2. The LefSe algorithm identified two bacterial taxa to be significantly abundant (FDR q ≤ 0.05) at H1 (order Defluvicoccales) and G2 (class Actinobacteria) (Figure 6E). Within the fungal communities, the differentially abundant taxa were kingdom fungi at H2, genus Heterophoma, and species H. sylvatica at H1, and family Cordycipitaceae, genus Leptobacillium, and species L. leptobactrum at G2 (Figure 6F). The observations were in partial agreement with the differential abundance testing.
Network analysis and functional prediction
The Kendall correlation among the top 100 genera was investigated. The network analysis (100 permutations; p < 0.05) returned complex lattices showing positive and negative relationships. The interactions were more complex among bacterial genera (Figure 7A) represented by 399 edges (an average of 5.55 edges per node). Of these, 52 were negative and 347 were positive. Interestingly, three of the ESKAPE genera were part of the network. Pseudomonas was positively correlated with seven genera (Zoogloea: 0.409, p = 0.033; Castellaniella: 0.390, p = 0.042; Cutibacterium: 0.561, p = 0.003; Hydrogenophaga: 0.478, p = 0.013; Nitrospira: 0.382, p = 0.047; Paracoccus: 0.409, p = 0.033; and Parapusillimonas: 0.386, p = 0.046) and negatively with three genera (Sediminibacterium: 0.516, p = –0.007; Microbacterium: 0.418, p = –0.0381; and Sphingobium: 0.4286, p = –0.026). Similarly, Acinetobacter was positively correlated with Delftia (0.421; p = 0.029) and negatively with Ferrovibrio (−0.402; p = 0.040) as well as Sphingobium (−0.466; p = 0.015). The genus Staphylococcus correlated positively with Allorhizobium (0.409; p = 0.033), Curvibacter (0.466; p = 0.015), and Sphingomonas (0.504; p = 0.008). It interacted negatively with a marine bacterium (−0.428, p = 0.026). To see the shifts in microbial communities toward diseased states in the indoor environments of hospitals, the microbial dysbiosis (MD) index was estimated. The H1/H2, H1/G2, and H2/G2 MD index was −0.6522, −0.2148, and 0.9305, respectively.
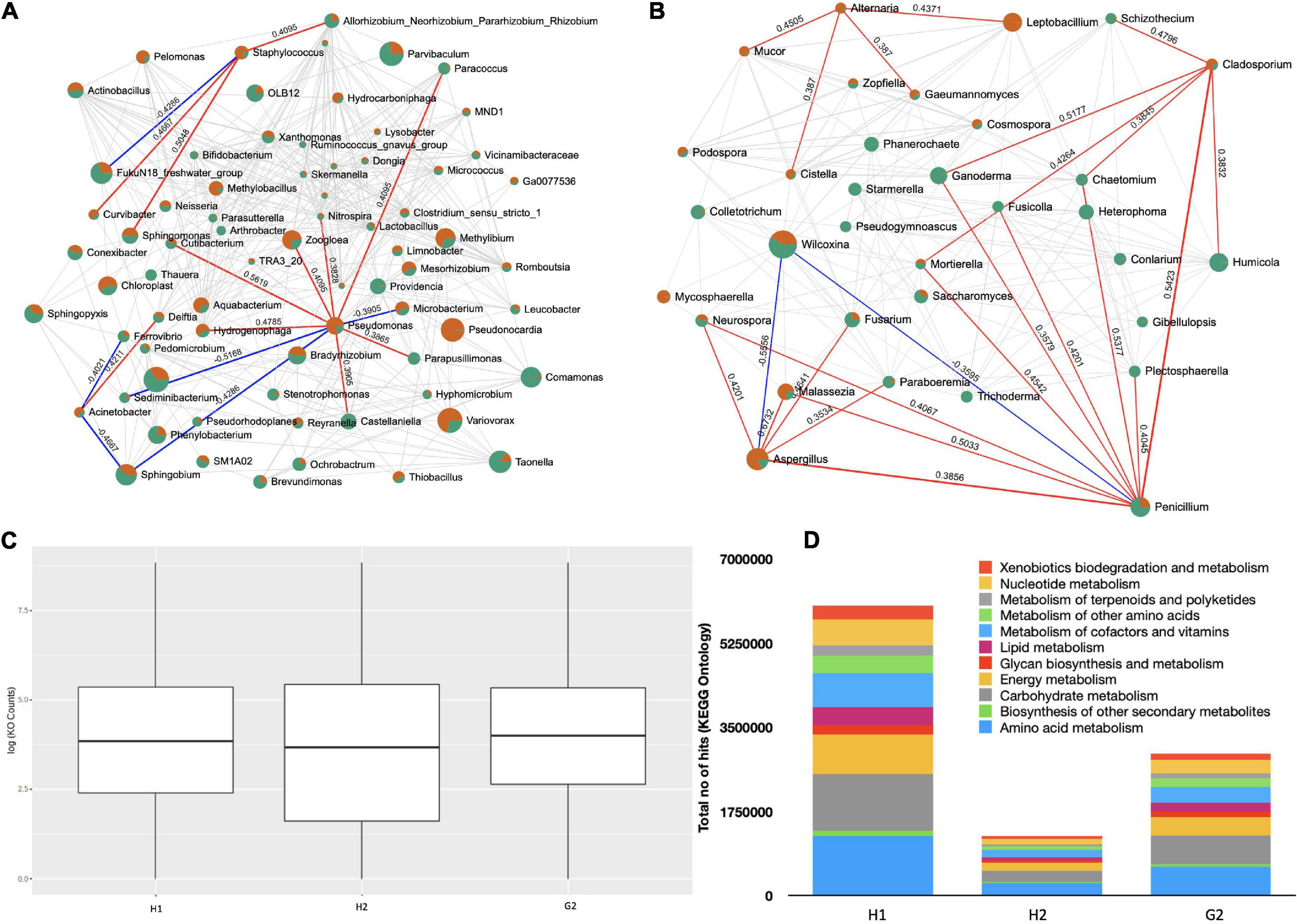
Figure 7. Network analysis and functional prediction: (A) Network lattice of bacterial genera, (B) network lattice of fungal species, (C) functional prediction of bacterial 16s rRNA genes, and (D) major KEGG pathways in bacterial genera.
The fungal genera were divided into 187 correlations (5.55 edges per genera) (Figure 7B). In total, 155 were positive and 32 negative. Many of the fungal forms were pathogenic; however, we only looked at the correlations that were most common, such as Alternaria, Aspergillus, Penicillium, and Fusarium. Alternaria interacted positively with Cistella (0.387, p = 0.046), Gaeumannomyces (0.387, p = 0.046), Leptobacillium (0.437, p = 0.018), and Mucor (0.450, p = 0.019). Aspergillus made positive associations with Fusarium (0.464, p = 0.007), Malassezia (0.673, p = 0.000), Neurospora (0.420, p = 0.016), Paraboeremia (0.353, p = 0.043), and Penicillium (0.385, p = 0.025). Penicillium was in positive correlation with Plectosphaerella (0.405, p = 0.029), Aspergillus (0.385, p = 0.025), Chaetomium (0.537, p = 0.001), Cladosporium (0.542, p = 0.002), Fusicolla (0.420, p = 0.024), Malassezia (0.503, p = 0.035), Mortierella (0.454, p = 0.010), Neurospora (0.406, p = 0.020), and Ganoderma (0.357, p = 0.044). Fusarium exhibited positive correlations with Gaeumannomyces (0.506, p = 0.007), Malassezia (0.426, p = 0.013), Mortierella (0.371, p = 0.036), Mucor (0.482, p = 0.009), Mycosphaerella (0.406, p = 0.018), Paraboeremia (0.553, p = 0.001), Phanerochaete (0.637, p = 0.000), Podospora (0.490, p = 0.009), Cosmpospora (0.522, p = 0.005), Aspergillus (0.464, p = 0.007), Cistella (0.539, p = 0.004), and Colletotrichum (0.411, p = 0.017). There were more opportunistic invaders in the fungal network when compared to bacteria and therefore comparatively higher H1/H2, H1/G2, and H2/G2 microbial dysbiosis (MD) index of −1.227, 3.062, and 2.34 were recorded, respectively.
A functional profile of bacterial communities was generated using the Tax4Fun to understand their metabolic contribution to the aerosol ecosystem. The OTUs mapped against 6,134 Kyoto Encyclopedia of Genes and Genomes (KEGG) terms. The log 10 counts of KEGG ontologies were at comparable levels at H1, H2, and G2 (Figure 7C). The KEGG pathways were part of 11 major metabolic pathways as shown in Figure 7D. The majority of them belonged to amino acid metabolism, biosynthesis of secondary metabolites, carbohydrate, energy, glucan, and lipid metabolisms, to name a few. Further details are provided in Supplementary Table 9. The absolute counts of KO terms of these functions were in the order of H1 > H2 > G2.
Discussion
Nosocomial aerosols are carriers of diverse microbial communities, including bacteria, fungi, and viruses. Inhalation of these microbes poses a 2.5 times higher risk to the hospital staff and frequent hospital visitors (Macintyre et al., 2012). Hospital-mediated outbreaks have resulted in severe infections (Yang et al., 2013; O’connor et al., 2015; Park et al., 2018; Weber et al., 2019; Løvestad et al., 2021; Paltansing et al., 2021), making the bioaerosol assessment in indoor hospital environment highly desirable. Earlier studies reporting both the bacteria and fungi simultaneously in the built environments of hospitals have relied on the traditional culture-dependent methods (Sarıca et al., 2002; Ekhaise et al., 2010; Okten and Asan, 2012; Sudharsanam et al., 2012; Park et al., 2013). The NGS approach for microbial community characterization has been a method of choice in the last few years owing to its fast, accurate, and precise estimation (King et al., 2016; Tong et al., 2017; Gao et al., 2018; Comar et al., 2019; Chakrawarti et al., 2020).
In this study, the bacterial and fungal communities were identified using the NGS approach at the two hospitals (H1 and H2) and non-hospital settings (G2). The community composition analysis of hospital indoor aerosols revealed phyla Proteobacteria was dominant among bacteria and Ascomycota among the fungi. Similar trends are reported elsewhere; the highest abundances of Proteobacteria were recorded in aerosols collected from the Delhi University Health Center, India (Chakrawarti et al., 2020), and urban hospitals in China (Gao et al., 2018). Ascomycota was found in four departments of a hospital in Beijing (Tong et al., 2017). Exhaled air seems to be a significant source of these communities within the indoor aerosols of hospitals (Prussin and Marr, 2015). This observation is further supported by a study conducted at the University of Chicago Medical Center that reports microbiota on surfaces within a patient room resembled the patient microbial community (Lax et al., 2017). Several other studies reported taxa from indoor air commonly found in human orifices and respiratory tract (Charlson et al., 2011; Nakatsuji et al., 2013; Madi et al., 2018; Habibi et al., 2021b). A study very well-demonstrated the microbiome of a hospital became dominated by a human skin-associated microbe as soon as it became operational (Lax et al., 2017).
Several studies have revealed the presence of different bacterial genera. For instance, a longitudinal metagenomic analysis of hospital air identified Stenotrophomonas as an opportunistic invader (King et al., 2016). In a university health center in Delhi, Psychrobacter, Arthrobacter, and the ESKAPE (Enterococcus faecium, Staphylococcus aureus, Klebsiella pneumoniae, Acinetobacter baumannii, Pseudomonas aeruginosa, and Enterobacter spp.) pathogens were recorded (Chakrawarti et al., 2020). Acinetobacter, Enterobacter, Pseudomonas, and Staphylococcus were the common genera identified in four healthcare institutes in Taiwan (Chen et al., 2017). Unlike the above studies, we recorded the presence of Variovorax, Parvibaculum, Pseudonocardia, Taonella, Methylibium, Sphingobium, Arthrospira, Comamonas, Sphingopyxis, and Zoogloea. Five of the ESKAPEE with very low RA pathogens were also discovered along with these predominant forms. Due to differences in sample methodologies, laboratory protocols, bacterial primer selection, sequencing methods, and data analysis pipelines, cross-study comparisons of bacterial and fungal genera are unequivocal. Similarly, the fungi observed were Wilcoxinia, Penicillium, Aspergillus, Humicola, Ganoderma, Heterophoma, Leptobacillus, Malassezzia, Saccharomyces, and Fusarium. Among these, Penicillium and Aspergillus were also found in the clinical microbiology laboratory of a university teaching hospital (Nagano et al., 2009). High-throughput sequencing revealed the prevalence of Aspergillus in the Respiratory Intensive Care Unit, Intensive Care Unit, Emergency Room, and Outpatient Department of a general hospital in Beijing (Tong et al., 2017).
From a health perspective, species identification is recommended as the bacterial and fungal species interact with the host to establish an antagonist, symbiotic, or synergistic relationship. However, due to the technical limitation of the 16S rRNA gene sequencing, we cannot go for the species-level classification for bacterial forms. A shotgun metagenomic approach will be followed in the future to gain knowledge of the bacterial species. Nevertheless, the presence of ESKAPEE genera (RA < 0.01) in our samples cannot be ignored. Analogous to our findings, unclassified species of Pseudomonas, Acinetobacter, and Staphylococcus were reported in extremely low percentage in aerosols of university healthcare center in Delhi (Chakrawarti et al., 2020). The concern also exuberates from the presence of some opportunistic pathogenic fungi, such as Aspergillus ruber (Lewis et al., 1994) (Guo et al., 2021), Colletrotrichum gloeosporiodes (Lin et al., 2015), Malassezia restricta (Houhamdi-Hammou et al., 2021), Fusarium proliferatum (Seyfarth et al., 2008), and Penicilium desertorum (Durmaz et al., 2021) in indoor hospital air. Besides these, the species of Wilcoxinia rehmii (Fenn et al., 2011), Ganoderma sichuaense (Xinsheng et al., 1995), and Humicola grisea (Andrioli et al., 2008) are plant pathogens and mycotoxin releasing forms. These might be considered major role players in causing allergic responses upon inhalation. It is, therefore, prudent to further investigate the infectivity dose of the pathogenic microbes upon inhalation.
Variations in alpha-diversity indices of bacterial and fungal communities were observed, although pairwise comparisons were non-significant. A small sample size most likely contributes to a higher p-value in our case. A substantial proportion of other genera with very low RA probably explains the variations in species richness and evenness (Lax et al., 2017) in the present samples. We would also like to mention that a reasonable number of unique OTUs were recorded at each sampling site (Rampelotto et al., 2019). Sogin et al. (2006) termed these features with low abundance as “rare biosphere” and proposed them to be representing some hidden functions. Also, the species abundance and richness were higher in the ambient air samples than in the indoor samples of hospital and non-hospital settings. This is attributed to the strict cleanliness and disinfection procedures followed at the Kuwaiti hospital consequent of the ongoing SARS-CoV-2 pandemic (Habibi et al., 2021d). A recent review published that about 80% of studies reported a decrease in hospital-associated infectious microbes upon following intensive healthcare environmental hygiene (Peters et al., 2022). The LEfSe analysis revealed the key indicator taxa as Defluvicoccales, Heterophoma, and H. sylvatica in hospitals when compared to Actinobacteria, Leptobacillium, L. leptobacillium, and Cordycipitaceae at G2 (KISR), all with very low RA.
We supposed the microbial community structure to widely differ between the hospital and non-hospital sites. We recorded a moderately diverse community structure of the bacterial population (ANOSIM r2 = 0.181–0.243; p < 0.05), whereas the fungal population was more or less homogenous at all the sampling locations. The small difference in bacterial community is due to the change in their RA (Jiayu et al., 2019). In congruence with our study, the assessment of bioaerosol particles from hospital wards and operating theaters in Tehran revealed slightly higher diversity in bacterial communities when compared to the fungal population (Bolookat et al., 2018). Moderately diverse bacterial communities were also observed in the indoor atmosphere of a Brazilian hospital (Rampelotto et al., 2019). Contrary to this, high fungal diversity was recorded in the indoor environments of Beijing hospital (Tong et al., 2017). Community structure is defined by the socio-economic status, human footfall, and the general architecture of the built environment (Kembel et al., 2012). All the buildings in Kuwait are centrally air-conditioned with controlled ventilation (ANON, 2008). This also accounts for the non-significant intra-site variations observed in H1, H2, and G2. Besides these, the non-hospital indoor site is an institute that is actively involved in COVID-19 research during the entire sampling period (Habibi et al., 2021a,d,g,2022b), and is accompanied by frequent visits by the staff members to these hospitals for sample collection, COVID-19 checkups, vaccination campaigns, etc. Hence, a more or less similar community structure between both the indoor sites is well-justified.
The community structure of bioaerosols plays an important role in public health, as a single pathogen cannot determine whether a diseased condition will be established (Jiayu et al., 2019). The network analysis revealed their involvement with each other and strengthens our belief that it is a complete microbial consortium that plays a role in dysbiosis. Rampelotto et al. (2019) suggested the positive correlations to be synergistic, while the negative correlations manifested antagonist interaction. The MD index > 2.0 suggested enrichment of fungal communities in hospital aerosols, respectively (Soares et al., 2016). In addition to this, we also found the association of these microbes (Bacteria) with several metabolic pathways. In partial agreement with our results, the functional profiling identified the metabolic pathways, such as citrate cycle (TCA), signal transduction mechanisms, bisphenol degradation, tyrosine metabolism, transcription factors, and antibiotic resistance in bacteria present on the environmental surfaces of a public hospital in South Africa (Shobo et al., 2020). Significant involvement of pathways in human diseases was also recorded by the same group. The biodegradation and metabolism of xenobiotics were one of the dominant pathways recorded in the present samples. The occurrence of antibiotic resistance genes in the microbes residing in the hospital environments is known (He et al., 2020; Li et al., 2021; Zhou et al., 2021), creating further need to understand the air resistome in the hospital indoors.
Conclusion
The 16s RNA and ITS amplicon sequencing successfully identified the bacterial and fungal communities of bioaerosols from hospitals in Kuwait. The microbial community consisted of pathogenic genera, although at lower abundances. These microbial populations varied spatially and were enriched in the hospital environments. The bacterial population was involved in a variety of metabolic pathways, including xenobiotic degradation. The baseline information gained from this study suggests a need to design a strategy for regular monitoring of indoor bioaerosols in a hospital-built environment as an early warning system for any pathogenic outbreak. It will be interesting to consider AMRs in these bioaerosols in future studies.
Data availability statement
The datasets presented in this study can be found in online repositories. The names of the repository/repositories and accession number(s) can be found below: NCBI SRA BioProject, accession numbers: PRJNA796511 (SRR17577942 to SRR17577943) and PRJNA796566 (SRR17579028 442 to SRR17579046).
Author contributions
NH and SU: conceptualization and reviewing and editing. NH, SU, and FA: methodology. NH: software, data curation, original draft preparation, and visualization. NH, SU, and MB: validation. FZ, AS, FA, and NR: formal analysis. SU: investigation. SU and MB: resources. FA and SU: supervision. MB: project administration. NH and MB: funding acquisition. All authors have read and agreed to the published version of the manuscript.
Funding
This research was funded by grants from the Kuwait Foundation for Advancement of Research (KFAS) PN20-43BO-01 and PR18-14SE-01.
Acknowledgments
The authors are thankful to Kuwait Institute for Scientific Research for supporting projects FB157C and FB160C.
Conflict of interest
The authors declare that the research was conducted in the absence of any commercial or financial relationships that could be construed as a potential conflict of interest.
Publisher’s note
All claims expressed in this article are solely those of the authors and do not necessarily represent those of their affiliated organizations, or those of the publisher, the editors and the reviewers. Any product that may be evaluated in this article, or claim that may be made by its manufacturer, is not guaranteed or endorsed by the publisher.
Supplementary material
The Supplementary Material for this article can be found online at: https://www.frontiersin.org/articles/10.3389/fmicb.2022.955913/full#supplementary-material
References
Abbas, M., Zhu, N. J., Mookerjee, S., Bolt, F., Otter, J. A., Holmes, A. H., et al. (2021). Hospital-onset COVID-19 infection surveillance systems: a systematic review. J. Hosp. Infect. 115, 44–50. doi: 10.1016/j.jhin.2021.05.016
Al Salameen, F., Habibi, N., Uddin, S., Al Mataqi, K., Kumar, V., Al Doaij, B., et al. (2020). Spatio-temporal variations in bacterial and fungal community associated with dust aerosol in Kuwait. PLoS One 15:e0241283.
Andrioli, W., Jorge, J., and Bastos, J. (2008). Phenolic metabolites from Humicola grisea var. thermoidea. Planta Med. 74:73.
ANON (2008). Guidelines for general ward design. Kuwait: Task Force Group for Designs and Constructions of Health Care Facilities, Infection Control Directorate, Ministry of Health.
Babin, S. (2020). Use of weather variables in SARS-CoV-2 transmission studies. Int. J. Infect. Dis. 100, 333–336.
Behbehani, M., Carvalho, F. P., Uddin, S., and Habibi, N. (2021a). Enhanced polonium concentrations in aerosols from the gulf oil producing region and the role of microorganisms. Int. J. Environ. Res. Public Health 18:13309. doi: 10.3390/ijerph182413309
Behbehani, M., Uddin, S., Habibi, N., Al Salameen, F., Sajid, S., Abdulrazzack, N., et al. (2021b). 210Po in Ultrafine Aerosol Particles and its Likelihood to Mutate the Microbial Community. Kuwait: Kuwait Institute for Scientific Research.
Bolookat, F., Hassanvand, M. S., Faridi, S., Hadei, M., Rahmatinia, M., and Alimohammadi, M. (2018). Assessment of bioaerosol particle characteristics at different hospital wards and operating theaters: a case study in Tehran. MethodsX 5, 1588–1596. doi: 10.1016/j.mex.2018.11.021
Caporaso, J. G., Kuczynski, J., Stombaugh, J., Bittinger, K., Bushman, F. D., Costello, E. K., et al. (2010). QIIME allows analysis of high-throughput community sequencing data. Nat. Methods 7, 335–336.
Chakrawarti, M. K., Singh, M., Yadav, V. P., and Mukhopadhyay, K. (2020). Temporal dynamics of air bacterial communities in a university health centre using illumina miseq sequencing. Aerosol Air Qual. Res. 20, 966–980.
Charlson, E. S., Bittinger, K., Haas, A. R., Fitzgerald, A. S., Frank, I., Yadav, A., et al. (2011). Topographical continuity of bacterial populations in the healthy human respiratory tract. Am. J. Respir. Crit. Care Med. 184, 957–963.
Chen, C.-H., Lin, Y.-L., Chen, K.-H., Chen, W.-P., Chen, Z.-F., Kuo, H.-Y., et al. (2017). Bacterial diversity among four healthcare-associated institutes in Taiwan. Sci. Rep. 7, 1–11. doi: 10.1038/s41598-017-08679-3
Comar, M., D’accolti, M., Cason, C., Soffritti, I., Campisciano, G., Lanzoni, L., et al. (2019). Introduction of NGS in environmental surveillance for healthcare-associated infection control. Microorganisms 7:708.
Durmaz, R., Durmaz, B., Arı, O., Abdulmajed, O., Çelik, S., and Kalcıoðlu, M. T. (2021). Mycobiome in the middle ear cavity with and without otitis media with effusion. Turk. Arch. Otorhinolaryngol. 59:261. doi: 10.4274/tao.2021.2021-10-4
Duverger, C., Souyri, V., Monteil, C., Fournier, S., Espinasse, F., Gramer, M., et al. (2021). Controlling healthcare-associated transmission of SARS-CoV-2 Variant of Concern 202012/01 in a large hospital network. J. Hosp. Infect. 114, 182–184. doi: 10.1016/j.jhin.2021.04.031
Edgar, R. C., Haas, B. J., Clemente, J. C., Quince, C., and Knight, R. (2011). UCHIME improves sensitivity and speed of chimera detection. Bioinformatics 27, 2194–2200. doi: 10.1093/bioinformatics/btr381
Ekhaise, F., Isitor, E., Idehen, O., and Emoghene, A. (2010). Airborne microflora in the atmosphere of an hospital environment of University of Benin Teaching Hospital (UBTH). Benin City, Nigeria. World J. Agric. Sci. 6, 166–170.
Faridi, S., Niazi, S., Sadeghi, K., Naddafi, K., Yavarian, J., Shamsipour, M., et al. (2020). A field indoor air measurement of SARS-CoV-2 in the patient rooms of the largest hospital in Iran. Sci. Total Environ. 725:138401. doi: 10.1016/j.scitotenv.2020.138401
Fenn, M., Allen, E., and Geiser, L. (2011). “Mediterranean California, Chapter 13,” in Assessment of Nitrogen Deposition Effects and Empirical Critical Loads of Nitrogen for Ecoregions of the United States. Gen. Tech. Rep. NRS-80, Vol. 80, eds L. H. Pardo, M. J. Robin-Abbott, and C. T. Driscoll (Newtown Square, PA: US Department of Agriculture, Forest Service, Northern Research Station), 143–169.
Fennelly, K. P., Tribby, M. D., Wu, C.-Y., Heil, G. L., Radonovich, L. J., Loeb, J. C., et al. (2015). Collection and measurement of aerosols of viable influenza virus in liquid media in an Andersen cascade impactor. Virus Adapt. Treat. 7, 1–9.
Gao, X.-L., Shao, M.-F., Wang, Q., Wang, L.-T., Fang, W.-Y., Ouyang, F., et al. (2018). Airborne microbial communities in the atmospheric environment of urban hospitals in China. J. Hazard. Mater. 349, 10–17. doi: 10.1016/j.jhazmat.2018.01.043
Gevao, B., Al-Ghadban, A. N., Bahloul, M., Uddin, S., and Zafar, J. (2013). Phthalates in indoor dust in Kuwait: Implications for non-dietary human exposure. Indoor Air 23, 126–133. doi: 10.1111/ina.12001
Gevao, B., Uddin, S., Al-Bahloul, M., and Al-Mutairi, A. (2022). Persistent organic pollutants on human and sheep hair and comparison with POPs in indoor and outdoor air. J. Environ. Expo. Assess. 1:5. doi: 10.20517/jeea.2021.06
Gregson, F. K., Sheikh, S., Archer, J., Symons, H. E., Walker, J. S., Haddrell, A. E., et al. (2021). Analytical challenges when sampling and characterising exhaled aerosol. Aerosol Sci. Technol. 56, 160–175. doi: 10.1007/s00216-022-03997-6
Guo, L.-N., Yu, S.-Y., Wang, Y., Liu, Y.-L., Yuan, Y., Duan, S.-M., et al. (2021). Species distribution and antifungal susceptibilities of clinical isolates of Penicillium and Talaromyces species in China. Int. J. Antimicrob. Agents 58:106349. doi: 10.1016/j.ijantimicag.2021.106349
Habibi, N., Behbehani, M., Uddin, S., Al Salamin, F., Shajan, A., and Zakir, F. (2021a). Environmental Resilience and Transformation in Times of COVID-19, eds A. L. Ramanathan, S. Chidambaram, M. P. Jonathan, F. Munoz-Arriola, M. V. Prasanna, and P. Kumar (Amsterdam: Elsevier), 194–199.
Habibi, N., Uddin, S., Al-Salameen, F., Al-Amad, S., Kumar, V., and Otaibi, M. (2021c). Identification and Characterization of Novel Corona and Associated Respiratory Viruses in Aerosols. Kuwait: Kuwait Institute for Scientific Research.
Habibi, N., Uddin, S., Al-Salameen, F., Al-Amad, S., Kumar, V., Al-Otaibi, M., et al. (2021d). SARS-CoV-2, other respiratory viruses and bacteria in aerosols: report from Kuwait’s hospitals. Indoor Air 31, 1815–1825. doi: 10.1111/ina.12871
Habibi, N., Mustafa, A. S., and Khan, M. W. (2021b). Composition of nasal bacterial community and its seasonal variation in health care workers stationed in a clinical research laboratory. PLoS One 16:e0260314. doi: 10.1371/journal.pone.0260314
Habibi, N., Uddin, S., Salameen, F. A., Behbehani, M., Shirshikhar, F., Razzack, N. A., et al. (2021e). Collection of bacterial community associated with size fractionated aerosols from Kuwait. Data 6:123. doi: 10.1371/journal.pone.0241283
Habibi, N., Uddin, S., Behbehani, M., Abdul Razzack, N., Hussain, F. Z., and Shajan, A. (2022a). SARS-CoV-2 in hospital air as revealed by comprehensive respiratory viral panel sequencing. Infect. Prev. Pract. 4:100199. doi: 10.1016/j.infpip.2021.100199
Habibi, N., Uddin, S., Fowler, S. W., and Behbehani, M. (2022b). Microplastics in the atmosphere: A review. J. Environ. Expo. Assess. 1:6. doi: 10.20517/jeea.2021.07
He, P., Wu, Y., Huang, W., Wu, X., Lv, J., Liu, P., et al. (2020). Characteristics of and variation in airborne ARGs among urban hospitals and adjacent urban and suburban communities: a metagenomic approach. Environ. Int. 139:105625. doi: 10.1016/j.envint.2020.105625
Houhamdi-Hammou, L., Benito, Y., Boibieux, A., Dupont, D., Delahaye, F., Thivolet-Bejui, F., et al. (2021). Malassezia restricta: an underdiagnosed causative agent of blood culture-negative infective endocarditis. Clin. Infect. Dis. 73, 1223–1230. doi: 10.1093/cid/ciab377
Jiayu, C., Qiaoqiao, R., Feilong, C., Chen, L., Jiguo, W., Zhendong, W., et al. (2019). Microbiology community structure in bioaerosols and the respiratory diseases. J. Environ. Sci. Public Health 3, 347–357. doi: 10.1016/j.scitotenv.2016.11.145
Karimzadeh, S., Bhopal, R., and Nguyen Tien, H. (2021). Review of infective dose, routes of transmission and outcome of COVID-19 caused by the SARS-COV-2: Comparison with other respiratory viruses. Epidemiol. Infect. 149:e96. doi: 10.1017/s0950268821000790
Kembel, S. W., Jones, E., Kline, J., Northcutt, D., Stenson, J., Womack, A. M., et al. (2012). Architectural design influences the diversity and structure of the built environment microbiome. ISME J. 6, 1469–1479.
Kim, D., Quinn, J., Pinsky, B., Shah, N. H., and Brown, I. (2020). Rates of co-infection between SARS-CoV-2 and other respiratory pathogens. JAMA 323, 2085–2086.
King, P., Pham, L. K., Waltz, S., Sphar, D., Yamamoto, R. T., Conrad, D., et al. (2016). Correction: longitudinal metagenomic analysis of hospital air identifies clinically relevant microbes. PLoS One 11:e0169376. doi: 10.1371/journal.pone.0169376
Lax, S., Sangwan, N., Smith, D., Larsen, P., Handley, K. M., Richardson, M., et al. (2017). Bacterial colonization and succession in a newly opened hospital. Sci. Transl. Med. 9:eaah6500. doi: 10.1126/scitranslmed.aah6500
Lednicky, J. A., Lauzardo, M., Fan, Z. H., Jutla, A., Tilly, T. B., Gangwar, M., et al. (2020). Viable SARS-CoV-2 in the air of a hospital room with COVID-19 patients. Int. J. Infect. Dis. 100, 476–482.
Lee, B. G., Yang, J. I., Kim, E., Geum, S. W., Park, J. H., and Yeo, M. K. (2021). Investigation of bacterial and fungal communities in indoor and outdoor air of elementary school classrooms by 16S rRNA gene and ITS region sequencing. Indoor Air 31, 1553–1562. doi: 10.1111/ina.12825
Li, R., Tun, H. M., Jahan, M., Zhang, Z., Kumar, A., Fernando, W. D., et al. (2017). Comparison of DNA-, PMA-, and RNA-based 16S rRNA Illumina sequencing for detection of live bacteria in water. Sci. Rep. 7, 1–11.
Li, X., Wu, Z., Dang, C., Zhang, M., Zhao, B., Cheng, Z., et al. (2021). A metagenomic-based method to study hospital air dust resistome. Chem. Eng. J. 406:126854. doi: 10.1016/j.cej.2020.126854
Li, Y. (2021). Hypothesis: SARS-CoV-2 transmission is predominated by the short-range airborne route and exacerbated by poor ventilation. Indoor Air 31, 921–925. doi: 10.1111/ina.12837
Li, Y. H., Fan, Y. Z., Jiang, L., and Wang, H. B. (2020). Aerosol and environmental surface monitoring for SARS-CoV-2 RNA in a designated hospital for severe COVID-19 patients. Epidemiol. Infect. 148, e154–e154. doi: 10.1017/S0950268820001570
Lin, L.-Y., Yang, C.-C., Wan, J.-Y., Chang, T. C., and Lee, J. Y.-Y. (2015). Cutaneous infection caused by plant pathogen Colletotrichum gloeosporioides. JAMA Dermatol. 151, 1383–1384. doi: 10.1001/jamadermatol.2015.2102
Liu, Y., Ning, Z., Chen, Y., Guo, M., Liu, Y., Gali, N. K., et al. (2020). Aerodynamic analysis of SARS-CoV-2 in two Wuhan hospitals. Nature 582, 557–560. doi: 10.1038/s41586-020-2271-3
Løvestad, A. H., Jørgensen, S. B., Handal, N., Ambur, O. H., and Aamot, H. V. (2021). Investigation of intra-hospital SARS-CoV-2 transmission using nanopore whole-genome sequencing. J. Hosp. Infect. 111, 107–116. doi: 10.1016/j.jhin.2021.02.022
Macintyre, C., Seale, H., Yang, P., Zhang, Y., Shi, W., Almatroudi, A., et al. (2014). Quantifying the risk of respiratory infection in healthcare workers performing high-risk procedures. Epidemiol. Infect. 142, 1802–1808.
Macintyre, R., Dwyer, D., Seale, H., Quanyi, W., Yi, Z., Yang, P., et al. (2012). High risk procedures and respiratory infections in hospital health care workers–quantifying the risk. Int. J. Infect. Dis. 16:e379.
Madi, N., Al-Nakib, W., Mustafa, A. S., and Habibi, N. (2018). Metagenomic analysis of viral diversity in respiratory samples from patients with respiratory tract infections in Kuwait. J. Med. Virol. 90, 412–420. doi: 10.1002/jmv.24984
Magoè, T., and Salzberg, S. L. (2011). FLASH: fast length adjustment of short reads to improve genome assemblies. Bioinformatics 27, 2957–2963. doi: 10.1093/bioinformatics/btr507
Mendes, G., Ramalho, J. F., Duarte, A., Pedrosa, A., Silva, A. C., Méndez, L., et al. (2022). First outbreak of NDM-1-producing Klebsiella pneumoniae ST11 in a portuguese hospital centre during the COVID-19 pandemic. Microorganisms 10:251. doi: 10.3390/microorganisms10020251
Muthuirulan, P., and Sharma, P. (2017). NGS: empowering infectious disease research beyond reality. Drug Target Rev. 4, 45–50.
Nagano, Y., Walker, J., Loughrey, A., Millar, C., Goldsmith, C., Rooney, P., et al. (2009). Identification of airborne bacterial and fungal species in the clinical microbiology laboratory of a university teaching hospital employing ribosomal DNA (rDNA) PCR and gene sequencing techniques. Int. J. Environ. Health Res. 19, 187–199. doi: 10.1080/09603120802474229
Nakatsuji, T., Chiang, H.-I., Jiang, S. B., Nagarajan, H., Zengler, K., and Gallo, R. L. (2013). The microbiome extends to subepidermal compartments of normal skin. Nat. Commun. 4, 1–8. doi: 10.1038/ncomms2441
O’connor, C., Powell, J., Finnegan, C., O’gorman, A., Barrett, S., Hopkins, K., et al. (2015). Incidence, management and outcomes of the first cfr-mediated linezolid-resistant Staphylococcus epidermidis outbreak in a tertiary referral centre in the Republic of Ireland. J. Hosp. Infect. 90, 316–321. doi: 10.1016/j.jhin.2014.12.013
Okten, S., and Asan, A. (2012). Airborne fungi and bacteria in indoor and outdoor environment of the pediatric unit of edirne government hospital. Environ. Monit. Assess. 184, 1739–1751. doi: 10.1007/s10661-011-2075-x
Paltansing, S., Sikkema, R., De Man, S., Koopmans, M., Munnink, B. O., and De Man, P. (2021). Transmission of SARS-CoV-2 among healthcare workers and patients in a teaching hospital in the Netherlands confirmed by whole-genome sequencing. J. Hosp. Infect. 110, 178–183. doi: 10.1016/j.jhin.2021.02.005
Park, D.-U., Yeom, J.-K., Lee, W. J., and Lee, K.-M. (2013). Assessment of the levels of airborne bacteria, gram-negative bacteria, and fungi in hospital lobbies. Int. J. Environ. Res. Public Health 10, 541–555. doi: 10.3390/ijerph10020541
Park, J.-S., Lee, E.-H., Park, N.-R., and Choi, Y. H. (2018). Mental health of nurses working at a government-designated hospital during a MERS-CoV outbreak: a cross-sectional study. Arch. Psychiatr. Nurs. 32, 2–6. doi: 10.1016/j.apnu.2017.09.006
Peters, A., Schmid, M. N., Parneix, P., Lebowitz, D., De Kraker, M., Sauser, J., et al. (2022). Impact of environmental hygiene interventions on healthcare-associated infections and patient colonization: a systematic review. Antimicrob. Resist. Infect. Control 11, 1–25.
Prussin, A. J., and Marr, L. C. (2015). Sources of airborne microorganisms in the built environment. Microbiome 3, 1–10.
Prussin, A. J., Ii, E. B. G., and Marr, L. C. (2015). Total virus. Environ. Sci. Technol. Lett. 2:84.
Quast, C., Pruesse, E., Yilmaz, P., Gerken, J., Schweer, T., Yarza, P., et al. (2012). The SILVA ribosomal RNA gene database project: improved data processing and web-based tools. Nucleic Acids Res. 41, D590–D596. doi: 10.1093/nar/gks1219
Rampelotto, P. H., Sereia, A. F., De Oliveira, L. F. V., and Margis, R. (2019). Exploring the hospital microbiome by high-resolution 16S rRNA profiling. Int. J. Mol. Sci. 20:3099. doi: 10.3390/ijms20123099
Salameen, F. A., Habibi, N., Uddin, S., Mataqi, K. A., Doaij, B. A., Amad, S. A., et al. (2021). Characterization and Identification of Microorganisms Associated with Airborne Dust. Kuwait: Figshare.
Santarpia, J. L., Rivera, D. N., Herrera, V., Morwitzer, M. J., Creager, H., Santarpia, G. W., et al. (2020). Aerosol and surface transmission potential of SARS-CoV-2. medRxiv [Preprint]. doi: 10.1101/2020.03.23.20039446
Sarıca, S., Asan, A., Otkun, M. T., and Ture, M. (2002). Monitoring indoor airborne fungi and bacteria in the different areas of Trakya University Hospital. Edirne, Turkey. Indoor Built Environ. 11, 285–292.
Segata, N., Izard, J., Waldron, L., Gevers, D., Miropolsky, L., Garrett, W. S., et al. (2011). Metagenomic biomarker discovery and explanation. Genome Biol. 12, 1–18.
Seyfarth, F., Ziemer, M., Sayer, H. G., Burmester, A., Erhard, M., Welker, M., et al. (2008). The use of ITS DNA sequence analysis and MALDI-TOF mass spectrometry in diagnosing an infection with Fusarium proliferatum. Exp. Dermatol. 17, 965–971. doi: 10.1111/j.1600-0625.2008.00726.x
Shobo, C. O., Alisoltani, A., Abia, A. L. K., Mtshali, P. S., Ismail, A., Zishiri, O., et al. (2020). Bacterial diversity and functional profile of microbial populations on surfaces in public hospital environments in South Africa: a high throughput metagenomic analysis. Sci. Total Envir. 719:137360. doi: 10.1016/j.scitotenv.2020.137360
Soares, R. C., Camargo-Penna, P. H., De Moraes, V., De Vecchi, R., Clavaud, C., Breton, L., et al. (2016). Dysbiotic bacterial and fungal communities not restricted to clinically affected skin sites in dandruff. Front. Cell. Infect. Microbiol. 6:157. doi: 10.3389/fcimb.2016.00157
Sogin, M. L., Morrison, H. G., Huber, J. A., Welch, D. M., Huse, S. M., Neal, P. R., et al. (2006). Microbial diversity in the deep sea and the underexplored “rare biosphere”. Proc. Natl. Acad. Sci. U.S.A. 103, 12115–12120.
Stockwell, R. E., Ballard, E. L., O’rourke, P., Knibbs, L. D., Morawska, L., and Bell, S. C. (2019). Indoor hospital air and the impact of ventilation on bioaerosols: a systematic review. J. Hosp. Infect. 103, 175–184. doi: 10.1016/j.jhin.2019.06.016
Sudharsanam, S., Swaminathan, S., Ramalingam, A., Thangavel, G., Annamalai, R., Steinberg, R., et al. (2012). Characterization of indoor bioaerosols from a hospital ward in a tropical setting. Afr. Health Sci. 12, 217–225. doi: 10.4314/ahs.v12i2.22
Tan, L., Ma, B., Lai, X., Han, L., Cao, P., Zhang, J., et al. (2020). Air and surface contamination by SARS-CoV-2 virus in a tertiary hospital in Wuhan. China. Int. J. Infect. Dis. 99, 3–7. doi: 10.1016/j.ijid.2020.07.027
Tong, X., Xu, H., Zou, L., Cai, M., Xu, X., Zhao, Z., et al. (2017). High diversity of airborne fungi in the hospital environment as revealed by meta-sequencing-based microbiome analysis. Sci. Rep. 7, 1–8. doi: 10.1038/srep39606
Uddin, S., Fowler, S. W., Habibi, N., Sajid, S., Dupont, S., and Behbehani, M. (2022). A preliminary assessment of size-fractionated microplastics in indoor aerosol-Kuwait’s baseline. Toxics 10:71. doi: 10.3390/toxics10020071
Van Doremalen, N., Bushmaker, T., Morris, D. H., Holbrook, M. G., Gamble, A., Williamson, B. N., et al. (2020). Aerosol and surface stability of SARS-CoV-2 as compared with SARS-CoV-1. N. Engl. J. Med. 382, 1564–1567.
Watts, S. C., Ritchie, S. C., Inouye, M., and Holt, K. E. (2019). FastSpar: rapid and scalable correlation estimation for compositional data. Bioinformatics 35, 1064–1066. doi: 10.1093/bioinformatics/bty734
Weber, R. E., Pietsch, M., Frühauf, A., Pfeifer, Y., Martin, M., Luft, D., et al. (2019). IS26-mediated transfer of blaNDM–1 as the main route of resistance transmission during a polyclonal. Multispecies Outbreak in a German Hospital. Front. Microbiol. 10:2817. doi: 10.3389/fmicb.2019.02817
Wemheuer, F., Taylor, J. A., Daniel, R., Johnston, E., Meinicke, P., Thomas, T., et al. (2018). Tax4Fun2: a R-based tool for the rapid prediction of habitat-specific functional profiles and functional redundancy based on 16S rRNA gene marker gene sequences. BioRxiv [Preprint]. doi: 10.1101/490037
White, J. R., Nagarajan, N., and Pop, M. (2009). Statistical methods for detecting differentially abundant features in clinical metagenomic samples. PLoS Comput. Biol. 5:e1000352. doi: 10.1371/journal.pcbi.1000352
Xinsheng, H., Manyc, Y., and Tao, Z. (1995). Study on the resources of the genus ganoderma in sichuan. J. Mianyang Coll. Econ. Technol. 1, 1–5.
Yang, J., Ye, L., Guo, L., Zhao, Q., Chen, R., Luo, Y., et al. (2013). A nosocomial outbreak of KPC-2-producing Klebsiella pneumoniae in a Chinese hospital: dissemination of ST11 and emergence of ST37, ST392 and ST395. Clin. Microbiol. Infect. 19, E509–E515. doi: 10.1111/1469-0691.12275
Yao, L., Zhu, W., Shi, J., Xu, T., Qu, G., Zhou, W., et al. (2021). Detection of coronavirus in environmental surveillance and risk monitoring for pandemic control. Chem. Soc. Rev. 50, 3656–3676.
Zhou, M.-Y., Xie, X.-L., Peng, Y.-G., Wu, M.-J., Deng, X.-Z., Wu, Y., et al. (2020). From SARS to COVID-19: what we have learned about children infected with COVID-19. Int. J. Infect. Dis. 96, 710–714.
Keywords: bioaerosol, bacteria, fungi, virus, exhaled air
Citation: Habibi N, Uddin S, Behbehani M, Al Salameen F, Razzack NA, Zakir F, Shajan A and Alam F (2022) Bacterial and fungal communities in indoor aerosols from two Kuwaiti hospitals. Front. Microbiol. 13:955913. doi: 10.3389/fmicb.2022.955913
Received: 29 May 2022; Accepted: 04 July 2022;
Published: 28 July 2022.
Edited by:
Samina Mehnaz, Forman Christian College, PakistanReviewed by:
Emrah Nikerel, Yeditepe University, TurkeyCheng Yan, China University of Geosciences Wuhan, China
Copyright © 2022 Habibi, Uddin, Behbehani, Al Salameen, Razzack, Zakir, Shajan and Alam. This is an open-access article distributed under the terms of the Creative Commons Attribution License (CC BY). The use, distribution or reproduction in other forums is permitted, provided the original author(s) and the copyright owner(s) are credited and that the original publication in this journal is cited, in accordance with accepted academic practice. No use, distribution or reproduction is permitted which does not comply with these terms.
*Correspondence: Saif Uddin, sdin@kisr.edu.kw