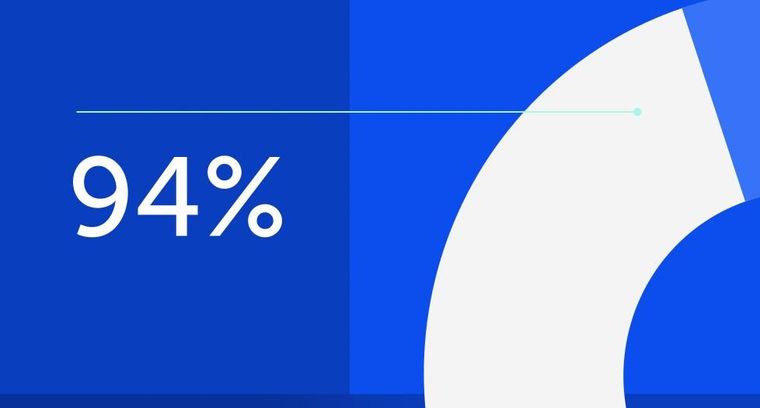
94% of researchers rate our articles as excellent or good
Learn more about the work of our research integrity team to safeguard the quality of each article we publish.
Find out more
ORIGINAL RESEARCH article
Front. Microbiol., 07 July 2022
Sec. Microbial Symbioses
Volume 13 - 2022 | https://doi.org/10.3389/fmicb.2022.953234
This article is part of the Research TopicCommunity series in the wildlife gut microbiome and its implication for conservation biology, volume IIView all 21 articles
Convergent evolution is an important sector of evolutionary biology. High-altitude environments are one of the extreme environments for animals, especially in the Qinghai Tibet Plateau, driving the inquiry of whether, under broader phylogeny, high-altitude factors drive the convergent evolution of Artiodactyla and Perissodactyla gut microbiomes. Therefore, we profiled the gut microbiome of Artiodactyla and Perissodactyla at high and low altitudes using 16S rRNA gene sequencing. According to cluster analyses, the gut microbiome compositions of high-altitude Artiodactyla and Perissodactyla were not grouped together and were far from those of low-altitude Artiodactyla and Perissodactyla. The Wilcoxon’s test in high-altitude ungulates showed significantly higher Sobs and Shannon indices than in low-altitude ungulates. At the phylum level, Firmicutes and Patescibacteria were significantly enriched in the gut microbiomes of high-altitude ungulates, which also displayed a higher Firmicutes/Bacteroidetes value than low-altitude ungulates. At the family level, Ruminococcaceae, Christensenellaceae, and Saccharimonadaceae were significantly enriched in the gut microbiomes of high-altitude ungulates. Our results also indicated that the OH and FH groups shared two significantly enriched genera, Christensenellaceae_R_7_group and Candidatus_Saccharimonas. These findings indicated that a high altitude cannot surpass the order level to drive the convergent evolution of ungulate gut microbiome composition but can drive the convergent evolution of alpha diversity and indicator microbiota in the gut microbiome of ungulates. Overall, this study provides a novel perspective for understanding the adaptation of ungulates to high-altitude environments.
The gut microbiome can provide energy, in the form of short-chain fatty acids, to its host and maintain the gut homeostatic balance (Gonçalves et al., 2018; Markowiak-Kopeć and Śliżewska, 2020). Many factors affect gut microbiome composition and function, including diet (Huang et al., 2022), phylogeny (Wang et al., 2019; Gregor et al., 2022), and environment (Gacesa et al., 2022; Wang et al., 2022). When a factor surpasses the other influencing factors, the gut microbiome converges to adapt toward this dominant factor. Giant panda (Ailuropoda melanoleuca) and red panda (Ailurus fulgens) are typical models for convergent evolution studies. Huang et al. (2021) found that their bamboo diet, rather than host phylogeny, is the dominant driver of gut microbiome convergence within these species. Surprisingly, giant pandas and bamboo-eating insects share strikingly similar gut microbiota (Yao et al., 2021). Owing to their diets, whales have similar gut microbiome compositions to that of carnivores (Wu et al., 2022). Similarly, the gut microbiomes of many myrmecophagous species, such as anteaters, aardvarks, and aardwolves, are clustered in the same group, although they belong to different orders (Delsuc et al., 2014).
In addition to animals with specialized diets, species with closer phylogenetic relationships or similar behavior have similar gut microbiome compositions (Gregor et al., 2022). Amato et al. (2019) found that host phylogeny outweighs the dietary niche in structuring primate gut microbiomes. The phylogeny of water bird species and their gut microbiome hierarchical tree evinced phylosymbiosis (Laviad-Shitrit et al., 2019). Flight behavior drives the convergent evolution of the gut microbiome in bats and birds, where Proteobacteria are the dominant bacterial species (Song et al., 2020).
Many scholars have focused on the environmental impacts to the gastrointestinal microbiome of different species, especially in high-altitude environments. For example, based on a principal component analysis plot of high-altitude rumen, such as yak and Tibetan sheep, microbiome compositions are clustered, with significantly enriched VFA-yielding pathways (Zhang et al., 2016). The Firmicutes/Bacteroidetes ratio in high-altitude blue sheep and European mouflon is higher than in low-altitude blue sheep and European mouflon, and principal component analysis and non-metric multidimensional scaling (NMDS) plots have shown that the gut microbiomes of blue sheep and European mouflon at high-altitude are more similar than those of the same species at low altitude (Sun et al., 2019). Ma et al. (2019) found that Tibetan antelopes (Pantholops hodgsonii), Tibetan sheep (Ovis aries), and Tibetan wild ass (Equus kiang) had similar gut microbiome compositions, and Tibetan antelopes and sheep were annotated to carbohydrate and energy metabolism. In these studies, significantly enriched microbiota and metabolic pathways were related to animal energy acquisition. High altitudes involve extreme environmental conditions, such as low oxygen (Wang et al., 2021), thus, driving the convergent evolution of gastrointestinal microbiome compositions and functions, which can produce more energy to maintain life activities in harsh plateau environments.
Ungulates are widely distributed, easy to domesticate and breed, and can adapt to a variety of environments (Mas-Coma et al., 2009). Some studies have shown that the feeding niche overlap of high-altitude (Qinghai-Tibet Plateau) ungulates is low in such high-altitude areas (Harris and Miller, 1995; Shrestha et al., 2005; Cao et al., 2009; Shi et al., 2016). There are abundant wild ungulates on the Tibetan Plateau, including Artiodactyla, such as yak (Bos grunniens), Tibetan antelopes (P. hodgsonii), and blue sheep (Pseudois nayaur), and Perissodactyla, such as Tibetan wild ass (E. kiang). Ungulates represent an excellent model for studying the convergent evolution of high-altitude gut microbiomes. Many scholars have conducted in-depth research on the convergent evolution of ungulates in different altitude environments based on microbiomics; however, in these studies, the species phylogeny is closely related at the family or genus level. Further, several of these studies have significant limitations; for example, Ma et al. (2019) did not compare the gut microbiome of E. kiang at different altitudes and performed cluster analyses between groups. Under broader phylogeny, high altitudes drive the convergent evolution of ungulate gut microbiomes; however, this issue remains unclear. Based on these previous studies, we hypothesized that high altitudes drive the convergent evolution of ungulate gut microbiome diversity patterns, core microbiota, composition, and indicator species at each classification level. Therefore, we used the 16S rRNA gene to compare the gut microbiomes of high- and low-altitude ungulates. We aim to reveal the how influential altitude and phylogeny are to ungulate microbiome compositions at different altitudes.
Samples of wild and captive ungulates were collected from Golmud (Qinghai Province, China) and Jinan (Shandong Province, China; Jinan wildlife and Jinan zoos), respectively. We collected samples from different wild ungulates and observed distinct individuals, immediately collecting excrement-core samples. Three observation points (point 1, B. grunniens and E. kiang; point 2, P. nayaur; and point 3, P. hodgsonii) along a straight-line distance of more than 100 km were considered. B. grunniens mainly feed on sedges in summer and graminoids in winter; E. kiang primarily consumes forbs in summer and browse in winter, while P. hodgsonii and P. nayaur primarily feed on graminoids and sedges in summer, respectively (Harris and Miller, 1995; Shi et al., 2016). Shi et al. (2016) observed that dietary overlaps were generally low among P. hodgsonii, B. grunniens, and E. kiang based on their Pianka’s indices. Captive ungulates ranged from 3 to 8 years old, were healthy, and had not received any medications within 3 months of sampling. The B. grunniens, P. nayaur, and E. kiang from the two zoos were fed approximately the same diets, consisting of cotton grass, alfalfa, carrots, and pellet feed. Information of all samples is detailed in Supplementary Tables S1, S2. All wild and captive samples were placed in sterile tubes and stored at −20°C during transit to Qufu Normal University, China, where all samples were frozen at −80°C until sequencing. This methodology followed the ethical standards of the Qufu Normal University Animal Care and Use Committee (Permit Number: 2022-020).
Bacterial DNA was extracted from the samples with HiPure Stool DNA Mini Kits (Magen, Guangzhou, China) following the manufacturer’s protocols. We used a Qubit 2.0 fluorometer (Thermo Fisher Scientific, Waltham, MA, United States of America) to measure the DNA to ensure concentrations were higher than 20 ng/μl. The V3–V4 hypervariable regions of bacterial 16S rRNA genes were amplified using PCR primers (forward primer: CTACGGGNGGCWGCAG; reverse primer: GACTACHVGGGTATCTAATCC; Wu et al., 2016). We performed PCR amplification in a 50 μl reaction mixture containing 1.5 μl of 2.5 mM dNTPs, 10 μl of 5 × Q5 Reaction Buffer, 10 μl of 5 × Q5 High GC Enhancer, 0.2 μl of Q5 High-Fidelity DNA Polymerase, 1.5 μl of the forward and reverse primers, and 25.3 μl of DNA. The PCR conditions were as follows: pre-denaturation at 95°C for 5 min, with 30 cycles of 1 min at 95°C for denaturation, 30 cycles of 1 min at 60°C for annealing, 30 cycles of 1 min at 72°C for elongation, and a final elongation at 72°C for 5 min. A TruSeq® DNA PCR-Free Sample Preparation Kit (Illumina, San Diego, CA, United States of America) was used to generate the DNA libraries, and the DNA library quality was quantified using a Qubit 2.0 Fluorometer (Thermo Fisher Scientific, Waltham, MA, United States of America) and FEMTO Pulse system (Agilent Technologies, Santa Clara, CA, United States of America). The DNA library was sequenced on an Illumina HiSeq platform (PE 250, United States of America) with a 250 bp.
The pair-ended reads were combined with raw tags using FLASH (v. 1.2.7; Magoč and Salzberg, 2011), and chimeric sequences were eliminated from them using the UCHIME algorithm (Edgar et al., 2011) to obtain effective tags. Based on a 16S rRNA sequence similarity at or greater than 97%, we performed UPARSE (v. 9.2.64; Edgar, 2013) to cluster the effective tags into operational taxonomic units (OTUs), and used the Mothur (Schloss et al., 2009) and SILVA databases (v 138.1; Quast et al., 2012) to annotate species with a threshold of 0.8–1. Alpha diversity [that is, the observed number of OTUs (Sobs), Chao1, Simpson, Shannon, and Good’s coverages] and beta diversity (that is, the Bray-Curtis distance) were calculated using the QIIME package (v. 1.9.1; Caporaso et al., 2010). Rarefaction curve and species accumulation boxplot analyses were performed with the Tutools platform (http://www.cloudtutu.com), a free online data analysis website. Principal coordinate analysis (PCoA), non-metric multidimensional scaling (NMDS), and the unweighted pair group method with arithmetic mean (UPGMA) were plotted using the ggplot2 package in R software (v 2.15.3). We used the Vega package in R software (v. 2.15.3) to perform Wilcoxon’s test (p < 0.05) and analysis of similarities, and used LDA Effect Size software (LDA score > 4, p < 0.05; White et al., 2009) to detect bacteria with significant differences between the groups.
We obtained 4,374,777 effective tags from 41 samples with an average of 106,702 effective tags per sample. With a 97% similarity criterion threshold, sample HA-9 showed the highest number of OTUs among all samples (2,560 OTUs), whereas sample HT-1 showed the lowest number of OTUs (1,161 OTUs; Supplementary Table S3, Sobs). Other alpha diversity indices are listed in Supplementary Table S3 (including Chao1, Simpson, Shannon, and Good’s coverages). The species accumulation boxplot and 41 rarefaction curves approached a plateau, suggesting that the number of samples and sequencing depth were sufficient for experimental analyses (Figure 1). Furthermore, the Good’s coverage index of each sample was higher than 95%, which also showed that the bacterial communities in the samples effectively represented those in the ungulate gut.
Figure 1. Species accumulation boxplot (A) and rarefaction curves (B). In the species accumulation boxplot, the abscissa is the sample size, and the ordinate is the observed species. In the rarefaction curves, the abscissa is the number of sequencing samples randomly chosen from the sample, and the ordinate is the number of OTUs (Sobs).
Since food composition differed between wild and captive ungulates, we added the published data of blue sheep (Group B) and European Mouflon (Group M); (samples were collected from the Xining wildlife zoo) into the cluster analyses to eliminate dietary effects. The ungulates of the Xining wildlife zoo were fed approximately the same diets as those of the Jinan wild zoo and Jinan zoo [Sun et al., 2019; Supplementary Table S4, with previously published sample information (Sun et al., 2019)]. Based on the relative abundance at the phylum level and the Bray–Curtis distance, we selected the top 10 phyla to generate a UPGMA tree (Figure 2). Artiodactyla species (groups HT, HB, HY, M, B, LB, and LY) were grouped together, and those from Perissodactyla (groups HA and LA) were clustered into another clade. The Artiodactyla and Perissodactyla clades diverged at high and low altitudes. PCoA (Figure 3) and NMDS analysis (stress = 0.051, Figure 4) also showed that the gut microbiome compositions of Artiodactyla differed from those of Perissodactyla, and the gut microbiome compositions of high-altitude Artiodactyla (groups HT, HB, HY, M, and B) and Perissodactyla (group HA) differed greatly from those of low-altitude Artiodactyla (groups LB and LY) and Perissodactyla (group LA), respectively. These results showed that high altitudes could not surpass the order level to drive the convergent evolution of ungulate gut microbiome composition. Next, we eliminated dietary composition effects between wild and captive ungulates. Thus, we observed that the similarity of the gut microbiome composition corresponding to the HT, HB, HY, M, and B groups was higher than that corresponding to the LB, LY, and LA groups. This implied that the main influencing factors were altitude and phylogeny, and not diet. Therefore, based on these two influencing factors, we classified high-altitude Artiodactyla (groups HT, HB, and HY), high-altitude Perissodactyla (group HA), low-altitude Artiodactyla (groups LB and LY), and low-altitude Perissodactyla (group LA) as groups the FH, OH, FL, and OL groups, respectively.
Figure 2. Cluster analysis with the Bray–Curtis distance. The unweighted pair group method with arithmetic mean (UPGMA) tree is generate based on the top 10 phyla.
Figure 3. Principal coordinates analysis (PCoA) of gut microbiome composition. Each symbol represents the gut microbiome of a group. HA, high-altitude Tibetan wild ass; HB, high-altitude blue sheep; HT, high-altitude Tibetan antelope; HY, high-altitude yak; LA, low-altitude Tibetan wild ass; LB, low-altitude blue sheep; LY, low-altitude yak. B, blue sheep from Xining wildlife zoo, M, European Mouflon from Xining wildlife zoo.
Figure 4. Non-metric multidimensional scaling (NMDS) of gut microbiome composition. Each symbol represents the gut microbiome of a group. HA, high-altitude Tibetan wild ass; HB, high-altitude blue sheep; HT, high-altitude Tibetan antelope; HY, high-altitude yak; LA, low-altitude Tibetan wild ass; LB, low-altitude blue sheep; LY low-altitude yak. B, blue sheep from Xining wildlife zoo, M, European Mouflon from Xining wildlife zoo.
The analysis of similarities (ANOSIM) demonstrated that the gut microbiome compositions of the OH and OL groups (R = 0.9695, p = 0.001) and the FH and FL groups (R = 0.7028, p = 0.001) showed significantly different unweighted uniFrac distances. This observation indicated that the division of these groups was reasonable.
Overall, 25 phyla, 49 classes, 99 orders, 170 families, 366 genera, and 136 species were detected in the bacterial microbiome communities of the Perissodactyla and Artiodactyla samples.
At the phylum level (top five abundance phyla), Firmicutes (F) and Bacteroidetes (B) dominated in groups such as FH (F, 53.77%; B, 20.57%), FL (F, 45.48%; B, 18.92%), OH (F, 43.71%; B, 31.35%), and OL (F, 38.98%; B, 41.5%). Other major phyla were Actinobacteria (7.99%), Euryarchaeota (5.27%), and Verrucomicrobia (4.13%) in the FH group; Proteobacteria (30.46%), Actinobacteria (2.41%), and Spirochaetes (0.72%) in the FL group; Kiritimatiellaeota (6.79%), Spirochaetes (5.62%), and Patescibacteria (3.68%) in the OH group; and Spirochaetes (6.71%), Proteobacteria (5.39%), and Kiritimatiellaeota (3.28%) in the OL group (Figure 5A).
Figure 5. Gut microbiome composition of ungulates at the phylum (A), family (B), and genus (C) levels. FH, high-altitude Artiodactyla (including groups HB, HT, and HY); FL, low-altitude Artiodactyla (including groups LB and LY); OH, high-altitude Perissodactyla (group HA); OL, low-altitude Perissodactyla (group LA).
At the family level, the top 10 families accounted for more than 59.26% of the four groups (FH: 69.19%; FL: 82.74%; OH: 59.26%; and OL: 66.72%). Among these 10 families, Ruminococcaceae was the most abundant in groups FH (29.98%) and OH (19.38%). Moraxellaceae (27.49%) was the most abundant in the FL group, and F082 (16.81%) was the most abundant in the OL group (Figure 5B). Additionally, at the genus level, Ruminococcaceae_UCG-005 (9.44%) was the most abundant genus in the FH group, Acinetobacter (27.39%) showed predominance in the FL group, while Rikenellaceae_RC9_gut_group showed predominance in the OH (7.63%) and OL (7.70%) groups (Figure 5C).
Wilcoxon’s test of Sobs (FH group vs. FL group, p = 0.003; OH group vs. OL group, p = 0.03) and Shannon (FH group vs. FL group, p = 0.001; OH group vs. OL group, p = 0.008) indices are shown in the boxplot (Figure 6). The gut microbiome diversity and richness of groups FH and OH were significantly higher than those of the low-altitude groups (FL and OL).
Figure 6. Wilcoxon’s test of alpha-diversity (Sobs and Shannon index) of gut microbiome between groups. (A) Sob index of group FH vs. group FL; (B) Sob index of group OH vs. group OL; (C) Shannon index of group FH vs. group FL; (D) Shannon index of group OH vs. group OL. FH, high-altitude Artiodactyla (including groups HB, HT, and HY); FL, low-altitude Artiodactyla (including groups LB and LY); OH, high-altitude Perissodactyla (group HA); OL, low-altitude Perissodactyla (group LA).
We used LDA Effect Size analysis (LDA score > 4, p < 0.05) to identify the indicator microbiota (biomarker) between groups (groups FH and FL and groups OH and OL). At the phylum level, Firmicutes and Patescibacteria were significantly enriched in the FH and FL groups. Verrucomicrobia and Euryarchaeota were significantly enriched in the FH group. Meanwhile, Kiritimatiellaeota were more abundant in the OH group than in the OL group, Proteobacteria were significantly enriched in groups FL and OL, and Bacteroidetes were more abundant in the OL group than in the OH group (Figure 7).
Figure 7. LEfSe (LDA Effect Size) analysis in ungulates between different altitudes. (A) Group FH (high-altitude Artiodactyla, including groups HB, HT, and HY) vs. group FL (low-altitude Artiodactyla, including groups LB and LY); (B) Group OH (high-altitude Perissodactyla, group HA) vs. group OL (low-altitude Perissodactyla, group LA).
At the family level, Ruminococcaceae, Christensenellaceae, and Saccharimonadaceae were significantly enriched in groups FH and OH; Akkermansiaceae, Lachnospiraceae, FamilyXIII, and Methanobacteriaceae were significantly enriched in the FH group. Moraxellaceae was significantly enriched in the FL and OL groups; Planococcaceae and Enterobacteriaceae were more abundant in the FL group than in the FH group; and F082 was more abundant in the OL group than in the OH group (Figure 7). At the genus level, Christensenellaceae_R_7_group and Candidatus_Saccharimonas were significantly enriched in the FH and OH groups. However, Ruminococcaceae_UCG-013, Ruminococcaceae_UCG-005, Methanobrevibacter, and Akkermansia were more abundant in the FH group than in the FL group. Our results also indicated that Escherichia_Shiella, Acinetobacter, Solibacillus, and Planococcus were more abundant in the FL group than in the FH group, and Acinetobacter was more abundant in the OL group than in the OH group (Figure 7).
Wilcoxon’s test was used to analyze the Firmicutes/Bacteroidetes (F/B) values between the groups. Supplementary Figure S1 shows that the F/B values of the high-altitude groups (FH and OH) were higher than those of the low-altitude groups (FL and OL; group FH vs. group FL, p > 0.05) and that of the OH group was significantly higher than that of the OL group (p < 0.01).
The gut microbiome is important for the ability of the host to adapt to various factors, including temperature, niche, and diet (Bo et al., 2019; Greene et al., 2020), which can provide energy and nutrients and maintain gut homeostasis in the host (Cani and Delzenne, 2007; Chung and Kasper, 2010; Wu et al., 2017). Many scholars have studied the adaptation mechanism of animals to high altitudes through the gut microbiome (Mazel, 2019; Xu et al., 2020), especially ungulates (Zhang et al., 2016, 2020; Sun et al., 2019). However, phylogeny has had little effect on their gut microbiome compositions in previous studies, because the phylogeny of ungulates was similar at the family, subfamily, and genus levels. Therefore, under broader phylogeny, this research explored the factors driving the convergent evolution of the gut microbiome of ungulates at different altitudes.
Using 16S rRNA high-throughput sequencing, we described the gut microbiome compositions of ungulates at different altitudes. At the phylum level, Firmicutes and Bacteroidetes were the dominant phyla in all samples (Figure 5). These results were consistent with other gut microbiomes of ungulates, such as the E. kiang (Gao et al., 2020), B. grunniens (Guo et al., 2021), P. hodgsonii (Bai et al., 2018), and P. nayaur (Sun et al., 2019).
According to the UPGMA tree, we found that groups HA, HB, HT, and HY were not clustered in the clade, while Artiodactyla (groups HB, HT, HY, LY, M, B, and LB) were clustered together. Ungulates with close phylogenetic relationships had similar gut microbiome compositions. These results revealed that high altitudes could not surpass the order level to drive the convergent evolution of the relative abundance composition of the ungulate. Phylogeny is one of the main factors affecting the gut microbiome composition of species (Benson et al., 2010; Zhu et al., 2021); for example, a study of 59 neotropical bird species found that the phylogeny factor was most frequently significant and explained most of the variation (Hird et al., 2015). In different niches, non-primates with close phylogenetic relationships possess similar gut microbiome compositions (Amato et al., 2019). Furthermore, the gut microbiome composition of Artiodactyla and Perissodactyla differed at high or low altitudes, and groups HB, HT, HY, M, and B clustered together through PCoA and NMDS plots. This showed that high altitudes drove the convergent evolution of ungulate gut microbiome composition at the order level. Notwithstanding, altitude is the main factor that affects the relative microbiome composition of ungulates. This is because, even though the diets of corresponding to the Xining wildlife zoo groups (the M and B groups) and the FL group were similar in composition, they did not group together.
High-altitude groups (OH and FH) were significantly higher than the low-altitude groups (FL and OL) in the Wilcoxon’s test of Sobs and Shannon. Higher gut bacterial diversity and richness generally correlate with a healthy and stable host gut microbiome (Petersen and Round, 2014; Waite and Taylor, 2014). High altitudes drove the gut microbiome of Artiodactyla and Perissodactyla to produce the same pattern of alpha diversity.
In this study, the OH and FH groups harbored large proportions of Firmicutes, which can decompose fibers and cellulose to provide volatile fatty acids to the host (Allison et al., 1962; Dai et al., 2015). Additionally, the F/B values of the high-altitude groups showed an increasing trend compared with that of the low-altitude groups. These high F/B values indicate that the host has a large body size and fat (Magne et al., 2020; Wolf et al., 2021). Many researchers have found that the F/B value of high-altitude ungulate gastrointestinal tract microbiomes was significantly higher than that of low-altitude ungulates (Ma et al., 2019; Sun et al., 2019). A high F/B ratio is beneficial for high-altitude ungulate energy harvesting (Zhang et al., 2016; Lan et al., 2017). The relative abundances of Patescibacteria in the OH and FH groups were higher than those in the OL and FL groups. Nitrate reductase (EC 1.7.99.4) positively predicts nitrite reductase (NO-forming, EC 1.7.2.1) and nitric-oxide reductase (cytochrome c, EC 1.7.2.5) in Patescibacteria, which has a synergistic effect on denitrification (Yang et al., 2022). Denitrification bacteria can reduce methane gas production in cow rumen liquids (Megga et al., 2017), as methane is a rumen fermentation by-product from methanogenic archaea, which leads to energy loss (Morgavi et al., 2010). Patescibacteria might reduce the energy loss of ungulates in high-altitude environments.
The relative abundance of Verrucomicrobia and Euryarchaeota was significantly higher in the FH group than in the OH group. Verrucomicrobia exists in the gut mucosa of healthy individuals and has anti-inflammatory effects (Fujio-Vejar et al., 2017) while maintaining glucose homeostasis in the host microbiome (Lindenberg et al., 2019). Euryarchaeota can promote carbohydrate fermentation to produce short-chain fatty acids, which play an important role in the gut ecosystem (Chaudhary et al., 2018). Kiritimatiellaeota is the major microbiota in horse (Edwards et al., 2020) and wild gelada (Baniel et al., 2021) hindguts and exists in the rumen of sheep (Wang et al., 2017) and cattle (Ribeiro et al., 2017). We speculate that Kiritimatiellaeota plays an important role in the digestion of herbivorous species, which requires further functional verification.
Ruminococcaceae, Christensenellaceae, and Saccharimonadaceae were characterized by a significant increase in the OH and FH groups. Ruminococcaceae and Christensenellaceae were the top 10 families in groups OH and FH. Ruminococcaceae is related to the decomposition of cellulose and starch (Andersen-Ranberg et al., 2018) and can produce acetic and formic acids (Ze et al., 2012). Christensenellaceae is an efficient sugar-fermenting family that can decompose glucose into acetic and butyric acids and can decompose cellulose (Morotomi et al., 2012). Meanwhile, Saccharimonadaceae is associated with immune response (Zhao et al., 2020). FamilyXIII is associated with the maintenance of gut health (Zhao et al., 2020), and Lachnospiraceae contained several cellulolytic/fibrolytic genera, and its abundance increased in the FH group, which is conducive to obtaining energy and maintaining homeostasis for the host in extreme environments (Biddle et al., 2013).
The OH and FH groups shared two significantly enriched genera, Christensenellaceae_R_7_group and Candidatus_Saccharimonas. The function of Christensenellaceae_R_7_group is the same as that of Christensenellaceae (Morotomi et al., 2012). Additionally, Candidatus_Saccharimonas species are positively correlated with propionic acid and butyric acid levels (Xia et al., 2022) and play an important role in maintaining gut functions (Xie et al., 2021). The relative abundance of the genus Akkermansia was greater in the FH group than in the other groups. Previous studies have shown that Akkermansia can consolidate the intestinal barrier and regulate immune functions (Zhao et al., 2022). Thus, the significantly enriched genera can help the high-altitude ungulates cope in extreme environments.
Based on the above results, the significantly enriched microbiota in high-altitude ungulates play an important role in disintegrating dietary fiber and cellulose, gaining energy, and maintaining gut homeostasis. High-altitude factors cannot surpass phylogeny to drive the convergent evolution of gut microbiome composition in ungulates, and high-altitude factors drive convergent evolution of alpha diversity and indicator microbiota in the gut microbiome of Artiodactyla and Perissodactyla at high altitudes. However, our understanding of the enriched microbiota and species level is still limited considering the limitations of 16S rRNA sequencing technology. Thus, in future studies, it would be necessary to employ metagenomics and metabolomics comprehensively to clarify the composition and function of enriched microbiota, especially at the species level, in ungulates at different altitudes.
The datasets presented in this study can be found in online repositories. The names of the repository/repositories and accession number(s) can be found at: https://www.ncbi.nlm.nih.gov/, PRJNA837737.
The animal study was reviewed and approved by Qufu Normal University Animal Care and Use Committee (Permit Number: 2022-020).
XWa: conceptualization, methodology, data curation, software, and writing-original draft preparation. XWu: methodology and writing—review and editing. YS: writing—review and editing. YG and YL: sample provision. QW: conceptualization and resources. XM: visualization. YD, SZ, and ZZ: conceptualization. GS: data curation. LL and BL: investigation. HZ: conceptualization and writing—review and editing. All authors contributed to the article and approved the submitted version.
This work was supported by the National Natural Science Foundation of China (32070405, 31872242, and 32001228).
The authors declare that the research was conducted in the absence of any commercial or financial relationships that could be construed as a potential conflict of interest.
All claims expressed in this article are solely those of the authors and do not necessarily represent those of their affiliated organizations, or those of the publisher, the editors and the reviewers. Any product that may be evaluated in this article, or claim that may be made by its manufacturer, is not guaranteed or endorsed by the publisher.
We thank the staff of the Butt Field Studio, Jinan Wildlife Zoo, and Jinan Zoo for helping with sample collection.
The Supplementary Material for this article can be found online at: https://www.frontiersin.org/articles/10.3389/fmicb. 2022.953234/full#supplementary-material
Allison, M. J., Bryant, M., Katz, I., and Keeney, M. (1962). Metabolic function of branched-chain volatile fatty acids, growth factors for ruminococci II: biosynthesis of higher branched-chain fatty acids and aldehydes. J. Bacteriol. 83, 1084–1093. doi: 10.1128/jb.83.5.1084-1093.1962
Amato, K. R., Sanders, J. G., Song, S. J., Nute, M., Metcalf, J. L., Thompson, L. R., et al. (2019). Evolutionary trends in host physiology outweigh dietary niche in structuring primate gut microbiomes. ISME J. 13, 576–587. doi: 10.1038/s41396-018-0175-0
Andersen-Ranberg, E. U., Barnes, C. J., Rasmussen, L., Salgado-Flores, A., Grøndahl, C., Mosbacher, J. B., et al. (2018). A comparative study on the Faecal bacterial community and potential zoonotic Bacteria of muskoxen (Ovibos moschatus) in Northeast Greenland, Northwest Greenland and Norway. Microorganisms 6:76. doi: 10.3390/microorganisms6030076
Bai, X., Lu, S., Yang, J., Jin, D., Pu, J., Díaz Moyá, S., et al. (2018). Precise fecal microbiome of the herbivorous Tibetan antelope inhabiting high-altitude alpine plateau. Front. Microbiol. 9:2321. doi: 10.3389/fmicb.2018.02321
Baniel, A., Amato, K. R., Beehner, J. C., Bergman, T. J., Mercer, A., Perlman, R. F., et al. (2021). Seasonal shifts in the gut microbiome indicate plastic responses to diet in wild geladas. Microbiome 9, 1–20. doi: 10.1186/s40168-020-00977-9
Benson, A. K., Kelly, S. A., Legge, R., Ma, F., Low, S. J., Kim, J., et al. (2010). Individuality in gut microbiota composition is a complex polygenic trait shaped by multiple environmental and host genetic factors. Proc. Natl. Acad. Sci. 107, 18933–18938. doi: 10.1073/pnas.1007028107
Biddle, A., Stewart, L., Blanchard, J., and Leschine, S. (2013). Untangling the genetic basis of fibrolytic specialization by Lachnospiraceae and Ruminococcaceae in diverse gut communities. Diversity 5, 627–640. doi: 10.3390/d5030627
Bo, T.-B., Zhang, X.-Y., Wen, J., Deng, K., Qin, X.-W., and Wang, D.-H. (2019). The microbiota–gut–brain interaction in regulating host metabolic adaptation to cold in male Brandt’s voles (Lasiopodomys brandtii). ISME J. 13, 3037–3053. doi: 10.1038/s41396-019-0492-y
Cani, P. D., and Delzenne, N. M. (2007). Gut microflora as a target for energy and metabolic homeostasis. Curr. Opin. Clin. Nutr. Metab. Care 10, 729–734. doi: 10.1097/MCO.0b013e3282efdebb
Cao, Y. F., Zhang, T. Z., Lian, X. M., Cui, Q. H., Deng, D. D., and Jian-Ping, S. U. J. S. J. O. Z. (2009). Diet overlap among selected ungulates in Kekexili region, Qinghai Province. Sichuan Journal of Zoology 28, 49–54.
Caporaso, J. G., Kuczynski, J., Stombaugh, J., Bittinger, K., Bushman, F. D., Costello, E. K., et al. (2010). QIIME allows analysis of high-throughput community sequencing data. Nat. Methods 7, 335–336. doi: 10.1038/nmeth.f.303
Chaudhary, P. P., Conway, P. L., and Schlundt, J. (2018). Methanogens in humans: potentially beneficial or harmful for health. Appl. Microbiol. Biotechnol. 102, 3095–3104. doi: 10.1007/s00253-018-8871-2
Chung, H., and Kasper, D. L. (2010). Microbiota-stimulated immune mechanisms to maintain gut homeostasis. Curr. Opin. Immunol. 22, 455–460. doi: 10.1016/j.coi.2010.06.008
Dai, X., Tian, Y., Li, J., Su, X., Wang, X., Zhao, S., et al. (2015). Metatranscriptomic analyses of plant cell wall polysaccharide degradation by microorganisms in the cow rumen. Appl. Environ. Microbiol. 81, 1375–1386. doi: 10.1128/AEM.03682-14
Delsuc, F., Metcalf, J. L., Wegener Parfrey, L., Song, S. J., González, A., and Knight, R. (2014). Convergence of gut microbiomes in myrmecophagous mammals. Mol. Ecol. 23, 1301–1317. doi: 10.1111/mec.12501
Edgar, R. C. (2013). UPARSE: highly accurate OTU sequences from microbial amplicon reads. Nat. Methods 10, 996–998. doi: 10.1038/nmeth.2604
Edgar, R. C., Haas, B. J., Clemente, J. C., Quince, C., and Knight, R. (2011). UCHIME improves sensitivity and speed of chimera detection. Bioinformatics 27, 2194–2200. doi: 10.1093/bioinformatics/btr381
Edwards, J., Shetty, S., Van Den Berg, P., Burden, F., Van Doorn, D., Pellikaan, W., et al. (2020). Multi-kingdom characterization of the core equine fecal microbiota based on multiple equine (sub) species. Animal Microbiome 2, 1–16. doi: 10.1186/s42523-020-0023-1
Fujio-Vejar, S., Vasquez, Y., Morales, P., Magne, F., Vera-Wolf, P., Ugalde, J. A., et al. (2017). The gut microbiota of healthy Chilean subjects reveals a high abundance of the phylum verrucomicrobia. Front. Microbiol. 8:1221. doi: 10.3389/fmicb.2017.01221
Gacesa, R., Kurilshikov, A., Vich Vila, A., Sinha, T., Klaassen, M., Bolte, L., et al. (2022). Environmental factors shaping the gut microbiome in a Dutch population. Nature 604, 732–739. doi: 10.1038/s41586-022-04567-7
Gao, H., Chi, X., Li, G., Qin, W., Song, P., Jiang, F., et al. (2020). Gut microbial diversity and stabilizing functions enhance the plateau adaptability of Tibetan wild ass (Equus kiang). MicrobiologyOpen 9, 1150–1161. doi: 10.1002/mbo3.1025
Gonçalves, P., Araújo, J. R., and Di Santo, J. P. (2018). A cross-talk between microbiota-derived short-chain fatty acids and the host mucosal immune system regulates intestinal homeostasis and inflammatory bowel disease. Inflamm. Bowel Dis. 24, 558–572. doi: 10.1093/ibd/izx029
Greene, L. K., Williams, C. V., Junge, R. E., Mahefarisoa, K. L., Rajaonarivelo, T., Rakotondrainibe, H., et al. (2020). A role for gut microbiota in host niche differentiation. ISME J. 14, 1675–1687. doi: 10.1038/s41396-020-0640-4
Gregor, R., Probst, M., Eyal, S., Aksenov, A., Sasson, G., Horovitz, I., et al. (2022). Mammalian gut metabolomes mirror microbiome composition and host phylogeny. ISME J. 16, 1262–1274. doi: 10.1038/s41396-021-01152-0
Guo, N., Wu, Q., Shi, F., Niu, J., Zhang, T., Degen, A. A., et al. (2021). Seasonal dynamics of diet–gut microbiota interaction in adaptation of yaks to life at high altitude. npj Biofilms Microbiomes 7, 1–11. doi: 10.1038/s41522-021-00207-6
Harris, R., and Miller, D. (1995). Overlap in summer Habitats and Diets of Tibetan Plateau Ungulates. Mammalia 59, 197–212. doi: 10.1515/mamm.1995.59.2.197
Hird, S. M., Sánchez, C., Carstens, B. C., and Brumfield, R. T. (2015). Comparative gut microbiota of 59 neotropical bird species. Front. Microbiol. 6:1403. doi: 10.3389/fmicb.2015.01403
Huang, G., Wang, X., Hu, Y., Wu, Q., Nie, Y., Dong, J., et al. (2021). Diet drives convergent evolution of gut microbiomes in bamboo-eating species. Sci. China Life Sci. 64, 88–95. doi: 10.1007/s11427-020-1750-7
Huang, G., Wang, L., Li, J., Hou, R., Wang, M., Wang, Z., et al. (2022). Seasonal shift of the gut microbiome synchronizes host peripheral circadian rhythm for physiological adaptation to a low-fat diet in the giant panda. Cell Rep. 38:110203. doi: 10.1016/j.celrep.2021.110203
Lan, D., Ji, W., Lin, B., Chen, Y., Huang, C., Xiong, X., et al. (2017). Correlations between gut microbiota community structures of Tibetans and geography. Sci. Rep. 7, 1–9. doi: 10.1038/s41598-017-17194-4
Laviad-Shitrit, S., Izhaki, I., Lalzar, M., and Halpern, M. (2019). Comparative analysis of intestine microbiota of four wild waterbird species. Front. Microbiol. 10:1911. doi: 10.3389/fmicb.2019.01911
Lindenberg, F., Krych, L., Fielden, J., Kot, W., Frøkiær, H., Van Galen, G., et al. (2019). Expression of immune regulatory genes correlate with the abundance of specific Clostridiales and Verrucomicrobia species in the equine ileum and cecum. Sci. Rep. 9:12674. doi: 10.1038/s41598-019-49081-5
Ma, Y., Ma, S., Chang, L., Wang, H., Ga, Q., Ma, L., et al. (2019). Gut microbiota adaptation to high altitude in indigenous animals. Biochem. Biophys. Res. Commun. 516, 120–126. doi: 10.1016/j.bbrc.2019.05.085
Magne, F., Gotteland, M., Gauthier, L., Zazueta, A., Pesoa, S., Navarrete, P., et al. (2020). The firmicutes/bacteroidetes ratio: a relevant marker of gut dysbiosis in obese patients? Nutrients 12:1474. doi: 10.3390/nu12051474
Magoč, T., and Salzberg, S. L. (2011). FLASH: fast length adjustment of short reads to improve genome assemblies. Bioinformatics 27, 2957–2963. doi: 10.1093/bioinformatics/btr507
Markowiak-Kopeć, P., and Śliżewska, K. (2020). The effect of probiotics on the production of short-chain fatty acids by human intestinal microbiome. Nutrients 12:1107. doi: 10.3390/nu12041107
Mas-Coma, S., Valero, M. A., and Bargues, M. D. (2009). Fasciola, lymnaeids and human fascioliasis, with a global overview on disease transmission, epidemiology, evolutionary genetics, molecular epidemiology and control. Adv. Parasitol. 69, 41–146. doi: 10.1016/S0065-308X(09)69002-3
Mazel, F. (2019). “Living the High Life: Could Gut Microbiota Matter for Adaptation to High Altitude? ”. Hoboken, NJ: Wiley Online Library.
Megga, R. P., Farah, M. Z., and Irawan, S. (2017). Bakteri Denitrifikasi Inaktif Sebagai Suplemen Untuk Mengurangi Gas Metana dari Cairan Rumen Sapi. Jurnal Ilmiah Aplikasi Isotop dan Radiasi 13, 69–78.
Morgavi, D., Forano, E., Martin, C., and Newbold, C. J. (2010). Microbial ecosystem and methanogenesis in ruminants. Animal 4, 1024–1036. doi: 10.1017/S1751731110000546
Morotomi, M., Nagai, F., and Watanabe, Y. (2012). Description of Christensenella minuta gen. Nov., sp. nov., isolated from human faeces, which forms a distinct branch in the order Clostridiales, and proposal of Christensenellaceae fam. nov. Int. J. Syst. Evol. Microbiol. 62, 144–149. doi: 10.1099/ijs.0.026989-0
Petersen, C., and Round, J. L. (2014). Defining dysbiosis and its influence on host immunity and disease. Cell. Microbiol. 16, 1024–1033. doi: 10.1111/cmi.12308
Quast, C., Pruesse, E., Yilmaz, P., Gerken, J., Schweer, T., Yarza, P., et al. (2012). The SILVA ribosomal RNA gene database project: improved data processing and web-based tools. Nucleic Acids Res. 41, D590–D596. doi: 10.1093/nar/gks1219
Ribeiro, G. O., Oss, D. B., He, Z., Gruninger, R. J., Elekwachi, C., Forster, R. J., et al. (2017). Repeated inoculation of cattle rumen with bison rumen contents alters the rumen microbiome and improves nitrogen digestibility in cattle. Sci. Rep. 7, 1–16. doi: 10.1038/s41598-017-01269-3
Schloss, P. D., Westcott, S. L., Ryabin, T., Hall, J. R., Hartmann, M., Hollister, E. B., et al. (2009). Introducing mothur: open-source, platform-independent, community-supported software for describing and comparing microbial communities. Appl. Environ. Microbiol. 75, 7537–7541. doi: 10.1128/AEM.01541-09
Shi, J., Lu, F., Li, X., Zhang, Z., Su, X., Dong, S., et al. (2016). Dietary overlap and co-existence of sympatric wild yak, Tibetan wild ass and Tibetan antelope in Arjin Shan National Nature Reserve, Xinjiang Province, China. Wildl. Res. 43, 323–331. doi: 10.1071/WR16045
Shrestha, R., Wegge, P., and Koirala, R. a. J. J. O. Z. (2005). Summer diets of wild and domestic ungulates in Nepal Himalaya. J. Zool. 266, 111–119. doi: 10.1017/S0952836905006527
Song, S. J., Sanders, J. G., Delsuc, F., Metcalf, J., Amato, K., Taylor, M. W., et al. (2020). Comparative analyses of vertebrate gut microbiomes reveal convergence between birds and bats. MBio 11, e02901–e02919. doi: 10.1128/mBio.02901-19
Sun, G., Zhang, H., Wei, Q., Zhao, C., Yang, X., Wu, X., et al. (2019). Comparative analyses of fecal microbiota in European Mouflon (Ovis orientalis musimon) and Blue Sheep (Pseudois nayaur) Living at Low or High Altitudes. Front. Microbiol. 10:1735. doi: 10.3389/fmicb.2019.01735
Waite, D. W., and Taylor, M. W. (2014). Characterizing the avian gut microbiota: membership, driving influences, and potential function. Front. Microbiol. 5:223. doi: 10.3389/fmicb.2014.00223
Wang, X., Chena, Y., Shanga, Y., Wua, X., Weia, Q., Chenb, J., et al. (2019). Comparison of the gut microbiome in red deer (Cervus elaphus) and fallow deer (Dama dama) by high-throughput sequencing of the V3–V4 region of the 16S rRNA gene. ScienceAsia 45, 515–524. doi: 10.2306/scienceasia1513-1874.2019.45.515
Wang, J., Fan, H., Han, Y., Zhao, J., and Zhou, Z. (2017). Characterization of the microbial communities along the gastrointestinal tract of sheep by 454 pyrosequencing analysis. Asian Australas. J. Anim. Sci. 30, 100–110. doi: 10.5713/ajas.16.0166
Wang, X., Shang, Y., Wei, Q., Wu, X., Dou, H., Zhang, H., et al. (2022). Comparative analyses of the gut microbiome of two fox species, the red fox (Vulpes Vulpes) and Corsac fox (Vulpes Corsac), that occupy different ecological niches. Microb. Ecol. 83, 753–765. doi: 10.1007/s00248-021-01806-8
Wang, X., Zhou, S., Wu, X., Wei, Q., Shang, Y., Sun, G., et al. (2021). High-altitude adaptation in vertebrates as revealed by mitochondrial genome analyses. Ecol. Evol. 11, 15077–15084. doi: 10.1002/ece3.8189
White, J. R., Nagarajan, N., and Pop, M. (2009). Statistical methods for detecting differentially abundant features in clinical metagenomic samples. PLoS Comput. Biol. 5:e1000352. doi: 10.1371/journal.pcbi.1000352
Wolf, J. F., Kriss, K. D., Macaulay, K. M., Munro, K., Patterson, B. R., and Shafer, A. B. (2021). Gut microbiome composition predicts summer core range size in two divergent ungulates. FEMS Microbiol. Ecol. 97:fiab048. doi: 10.1093/femsec/fiab048
Wu, X., Wei, Q., Wang, X., Shang, Y., and Zhang, H. (2022). Evolutionary and dietary relationships of wild mammals based on the gut microbiome. Gene 808:145999. doi: 10.1016/j.gene.2021.145999
Wu, X., Zhang, H., Chen, J., Shang, S., Wei, Q., Yan, J., et al. (2016). Comparison of the fecal microbiota of dholes high-throughput Illumina sequencing of the V3–V4 region of the 16S rRNA gene. Appl. Microbiol. Biotechnol. 100, 3577–3586. doi: 10.1007/s00253-015-7257-y
Wu, X., Zhang, H., Chen, J., Shang, S., Yan, J., Chen, Y., et al. (2017). Analysis and comparison of the wolf microbiome under different environmental factors using three different data of next generation sequencing. Sci. Rep. 7, 1–11. doi: 10.1038/s41598-017-11770-4
Xia, J., Lv, L., Liu, B., Wang, S., Zhang, S., Wu, Z., et al. (2022). Akkermansia muciniphila Ameliorates Acetaminophen-Induced Liver Injury by Regulating Gut Microbial Composition and Metabolism. Microbiol. Spectr. 10, e01596–e01521. doi: 10.1128/spectrum.01596-21
Xie, Y., Sun, J., Hu, C., Ruan, B., and Zhu, B. J. F. I. M. (2021). Oral microbiota is associated with immune recovery in human immunodeficiency virus-infected individuals. Front. Microbiol. 12:794746. doi: 10.3389/fmicb.2021.794746
Xu, L. L., Chen, H., Zhang, M., Zhu, W., Chang, Q., Lu, G., et al. (2020). Changes in the community structure of the symbiotic microbes of wild amphibians from the eastern edge of the Tibetan plateau. Microbiology 9:e1004. doi: 10.1002/mbo3.1004
Yang, X., Yuan, J., Guo, W., Tang, X., and Zhang, S. (2022). The enzymes-based intermediary model explains the influence mechanism of different aeration strategies on nitrogen removal in a sequencing batch biofilm reactor treating simulated aquaculture wastewater. J. Clean. Prod. 356:131835. doi: 10.1016/j.jclepro.2022.131835
Yao, R., Dai, Q., Wu, T., Yang, Z., Chen, H., Liu, G., et al. (2021). Fly-over phylogeny across invertebrate to vertebrate: The giant panda and insects share a highly similar gut microbiota. Comput. Struct. Biotechnol. J. 19, 4676–4683. doi: 10.1016/j.csbj.2021.08.025
Ze, X., Duncan, S. H., Louis, P., and Flint, H. J. (2012). Ruminococcus bromii is a keystone species for the degradation of resistant starch in the human colon. ISME J. 6, 1535–1543. doi: 10.1038/ismej.2012.4
Zhang, H., Chen, Y., Wu, X., Shang, S., Chen, J., Yan, J., et al. (2020). Comparison of the gut microbiota in the Tibetan wild ass (Equus kiang) collected from high and low altitude. Pak. J. Zool. 52:2281. doi: 10.17582/journal.pjz/20180522070505
Zhang, Z., Xu, D., Wang, L., Hao, J., Wang, J., Zhou, X., et al. (2016). Convergent evolution of rumen microbiomes in high-altitude mammals. Curr. Biol. 26, 1873–1879. doi: 10.1016/j.cub.2016.05.012
Zhao, Y., Yan, Y., Zhou, W., Chen, D., Huang, K., Yu, S., et al. (2020). Effects of polysaccharides from bee collected pollen of Chinese wolfberry on immune response and gut microbiota composition in cyclophosphamide-treated mice. J. Funct. Foods 72:104057. doi: 10.1016/j.jff.2020.104057
Zhao, Q., Yu, J., Hao, Y., Zhou, H., Hu, Y., Zhang, C., et al. (2022). Akkermansia muciniphila plays critical roles in host health. Crit. Rev. Microbiol. 1–19. doi: 10.1080/1040841X.2022.2037506
Keywords: gut microbiome, ungulates, phylogeny, high-altitude, convergent evolution
Citation: Wang X, Wu X, Shang Y, Gao Y, Li Y, Wei Q, Dong Y, Mei X, Zhou S, Sun G, Liu L, Lige B, Zhang Z and Zhang H (2022) High-Altitude Drives the Convergent Evolution of Alpha Diversity and Indicator Microbiota in the Gut Microbiomes of Ungulates. Front. Microbiol. 13:953234. doi: 10.3389/fmicb.2022.953234
Received: 26 May 2022; Accepted: 20 June 2022;
Published: 07 July 2022.
Edited by:
Lifeng Zhu, Nanjing Normal University, ChinaReviewed by:
Feng Jiang, Northwest Institute of Plateau Biology (CAS), ChinaCopyright © 2022 Wang, Wu, Shang, Gao, Li, Wei, Dong, Mei, Zhou, Sun, Liu, Lige, Zhang and Zhang. This is an open-access article distributed under the terms of the Creative Commons Attribution License (CC BY). The use, distribution or reproduction in other forums is permitted, provided the original author(s) and the copyright owner(s) are credited and that the original publication in this journal is cited, in accordance with accepted academic practice. No use, distribution or reproduction is permitted which does not comply with these terms.
*Correspondence: Honghai Zhang, emhhbmdob25naGFpNjdAMTI2LmNvbQ==
†These authors have contributed equally to this work
Disclaimer: All claims expressed in this article are solely those of the authors and do not necessarily represent those of their affiliated organizations, or those of the publisher, the editors and the reviewers. Any product that may be evaluated in this article or claim that may be made by its manufacturer is not guaranteed or endorsed by the publisher.
Research integrity at Frontiers
Learn more about the work of our research integrity team to safeguard the quality of each article we publish.