- 1State Key Laboratory of Mining Response and Disaster Prevention and Control in Deep Coal Mines, Anhui University of Science and Technology, Huainan, China
- 2School of Safety Science and Engineering, Anhui University of Science and Technology, Huainan, China
- 3Joint National-Local Engineering Research Centre for Safe and Precise Coal Mining, Anhui University of Science and Technology, Huainan, China
Microorganisms are the core drivers of biogeochemistry processes in petroleum reservoirs and have been widely used to enhance petroleum recovery. However, systematic information about the microbial communities related to the C-N-S cycle in petroleum reservoirs under different pH conditions remains poorly understood. In this study, 16S rRNA gene data from 133 petroleum samples were collected, and 756 C-N-S related genera were detected. The Chao1 richness and Shannon diversity indices for the C-N-S-related microbial communities showed significant differences among different pH conditions and at the lowest levels in acidic conditions with pH values of 4.5–6.5. In addition, pH was the most important factor influencing the C-N-S related microbial communities and contributed to 17.95% of the variation in the methanogenesis community. A total of 55 functional genera were influenced by pH, which accounted for 42.08% of the C-N-S related genera. Among them, the genera Pseudomonas and Arcobacter were the highest and were concentrated in acidic conditions with pH values of 4.5–6.5. In parallel, 56 predicted C-N-S related genes were examined, and pH affected 16 of these genes, including putative chitinase, mcrA, mtrB, cysH, narGHIVYZ, nirK, nirB, nifA, sat, aprAB, and dsrAB. Furthermore, the co-occurrence networks of the C-N-S related microbial communities distinctly varied among the different pH conditions. The acidic environment exhibited the lowest complex network with the lowest keystone taxa number, and Escherichia-Shigella was the only keystone group that existed in all three networks. In summary, this study strengthened our knowledge regarding the C-N-S related microbial communities in petroleum reservoirs under different pH conditions, which is of great significance for understanding the microbial ecology and geochemical cycle of petroleum reservoirs.
Introduction
Microorganisms are the core drivers of the biogeochemistry of important elements (especially C, N, and S) in petroleum reservoirs and have received extensive attention for their ability to enhance the recovery of energy materials such as petroleum (Brown, 2010; Nazina et al., 2019; Zhou et al., 2020). The distribution characteristics of microbial populations in global petroleum reservoirs have been extensively reported in the past decade (Aurepatipan et al., 2018; Zhou et al., 2019, 2020; de Sousa Pires et al., 2021). In addition, a variety of C-N-S related functional microorganisms, including sulfate reducers, fermentative bacteria, acidogens, and methanogens (Magot et al., 2000; Li et al., 2017b; Santos et al., 2020), have been widely detected in petroleum reservoirs. All of these functional taxa are inseparable from the exploitation and development of petroleum reservoirs. However, there is still a lack of a systematic description of these groups in petroleum reservoirs, which is crucial for understanding the C, N and S cycles in petroleum reservoirs.
Petroleum reservoirs are often considered extreme environments because of their physico-chemical properties, such as extremely variable pH and salinity, and are almost completely anaerobic (Varjani and Gnansounou, 2017). Under petroleum reservoir anaerobic environments, the coexistence and/or competition of different functional microorganisms could directly affect the quality of petroleum and the efficiency of petroleum recovery. In particular, the current microbial enhanced oil recovery (MEOR) technology is widely used to extract residual petroleum from low-productivity reservoirs by converting hydrocarbons through microbial fermentation and methanogenesis (Meslé et al., 2013; Quraishi et al., 2021). Previous studies have found that petroleum hydrocarbons can be degraded by a variety of microorganisms following a quasi-step-by-step biodegradation process (Meslé et al., 2013). Petroleum hydrocarbons are first degraded into single molecules and oligomers by petroleum hydrocarbon-degrading bacteria and fermentative bacteria, and then, some intermediate products are generated by different acidifying bacteria, acetic acid-producing bacteria and hydrogen-producing bacteria. The products from the degradation and fermentation of petroleum hydrocarbons could provide basic growth substrates for the hydrogenotrophic and acetate methanogenesis archaea (Magot et al., 2000). In addition, homotrophic oxidation pathways coupled to hydrogenotrophic methanogenesis are prevalent in many cases (Liang et al., 2015; Li et al., 2016). Because of the synergistic cooperation of C-N-S-related communities, including methanogens, petroleum hydrocarbon-degrading bacteria and fermentative bacteria, the petroleum degradation process has thermodynamic advantages under anaerobic conditions (Mbadinga et al., 2011). However, pH is an important factor that leads to changes in these functional groups (Zagrodnik and Łaniecki, 2017; Li et al., 2021).
The pH change in petroleum is closely related to sulfate-reducing bacteria (SRB). SRBs are of particular interest because they are associated with petroleum bioacidification and biocorrosion of metals used in the petroleum industry, which consequently result in huge financial losses (Varjani and Gnansounou, 2017; Narenkumar et al., 2019). Therefore, the implementation of MEOR technology often requires the addition of nitrate to stimulate the activity of nitrate-reducing bacteria (NRB) and inhibit the growth of SRB. It could be used to reduce sulfide generation caused by the sulfate reduction process, which could control the deterioration of petroleum quality and corrosion of engineering materials (Gao et al., 2013). Therefore, understanding petroleum C-N-S related microbes is beneficial to apply microbial knowledge to the actual production in the petroleum industry.
The co-occurrence relation of C-N-S-related functional groups is also important for community functional stability. For example, SRB in anaerobic environments are able to use sulfate as electron acceptors to provide biosulfur by the assimilation pathway for the production of amino acids and proteins (Mansilla et al., 2000; Tikariha and Purohit, 2019) and can also excrete H2S through the dissimilation pathway (Santos et al., 2020). Such dissimilation processes can still provide H+ for methanogens and are beneficial to support their carbon resource utilization (Muyzer and Stams, 2008; Tang et al., 2009). These mutual relationships among microorganisms, especially symbiosis, competition, predation, etc., can be reflected by the microbial co-occurrence network (Mougi and Kondoh, 2012; Qian and Akçay, 2020). Closely related microbes would allow them to occupy favorable ecological niches, and communities would become more stable against habitat change (i.e., pH changes). In general, keystone groups are often considered to have irreplaceable roles in microbial community structure and function (Ma Q. et al., 2020; Zhou et al., 2022) due to their unique topological properties, and they are also the core members of linking modules and networks (Coux et al., 2016; Xue et al., 2018). Keystone groups can be estimated based on the topological roles of nodes in networks, which are concatenated to microbial communities and provide new insights into the architecture in microbial co-occurrence networks (Banerjee et al., 2018; Wang et al., 2020). However, little is known about the co-occurrence networks of C-N-S-related functional groups in petroleum reservoirs and the keystone driver taxa under different pH conditions.
In this study, 16S rRNA data of petroleum samples from the NCBI database were extracted and used to reanalyze the C-N-S-related microbial composition. This study aims to (1) describe the C-N-S-related microbial communities and functional genes in petroleum reservoirs under different pH conditions and (2) explore the co-occurrence network of C-N-S-related microbial communities and keystone taxa in petroleum reservoirs.
Materials and methods
Datasets
Until February 2022, literature retrieval was conducted through the Web of Science database, and the published papers on “petroleum” and “microbial communities” were retrieved (Lenchi et al., 2013; Gao P. et al., 2015; Gao et al., 2016; Mand et al., 2016; Shelton et al., 2016; Li et al., 2017a; Aurepatipan et al., 2018; Nazina et al., 2019; Phetcharat et al., 2019; Zhou et al., 2019, 2020; Santos et al., 2020; de Sousa Pires et al., 2021). Samples of petroleum or produced water that are closely related to petroleum reservoirs were manually selected. The fastQ files according to the accession numbers of the 16S rRNA gene from petroleum samples were downloaded. These 16S rRNA gene data from 133 petroleum samples were collected for meta-analysis. The detailed sample information is shown in Supplementary Table 1. To analyze the changes in the C-N-S-related microbial communities under different pH conditions, the petroleum samples were divided into three groups based on their pH values, namely, an acidic group with pH 4.5–6.5 (4.5 ≤ pH ≤ 6.5) (n = 31), a neutral group with pH 6.5–7.5 (6.5 < pH < 7.5) (n = 57), and an alkaline group with pH 7.5–9.0 (7.5 ≤ pH < 9.0) (n = 45).
Bioinformatics analysis
For microbial community (bacteria and archaea) analysis, the reads from 16S genes were merged, and the raw sequences were quality filtered using the QIIME pipeline. The chimeric sequences were identified by the “identify_chimeric_seqs.py” command and removed with the “filter_fasta.py” command according to the UCHIME algorithm. The selection and taxonomic assignment of operational taxonomic units (OTUs) were performed based on the SILVA reference data (version 128) at 97% similarity. Reads that did not align to the anticipated region of the reference alignment were removed as chimeras by the UCHIME algorithm. Reads that were classified as “chloroplast,” “mitochondria,” or “unassigned” were removed.
The predictive functional abundance was predicted by PICRUSt2 (Phylogenetic Investigation of Communities by Reconstruction of Unobserved States) with “picrust2_pipeline.py”1 (Douglas et al., 2020), which performed 4 key steps, including sequence placement, hidden-state prediction of genomes, metagenome prediction and pathway-level predictions. In addition, the additional output file Predicted Enzyme Commission (EC) number copy numbers were used to screen the C-N-S-related microbial genera. These C-N-S-related microbial genera included carbon degradation (petroleum degradation) genera, methanogenesis genera, CH4 oxidation genera, N2 fixation genera, ammoxidation genera, denitrification genera, dissimilatory nitrate reduction to ammonium (DNRA) genera and sulfur reduction genera.
Data analysis
To avoid differences in amplified fragments among different samples, the analysis was performed at the genus level. The Shannon diversity and Chao1 richness for the C-N-S-related and each single C/N/S-related microbial communities were determined according to the relative abundance of genera. In addition, Bray-Curtis dissimilarities were calculated based on the relative abundance matrix of the C-N-S-related and each single C/N/S-related microbial genera in the Vegan package of R v 4.1.2. Non-metric multidimensional scaling (NMDS) was applied based on the Bray-Curtis dissimilarities by Vegan’s metaMDS function. Variation partitioning analysis (VPA) was used to determine the effects of chemical properties on the structure of the C-N-S-related microbial communities by Vegan’s varpart function.
Co-occurrence networks for the C-N-S-related microbial genera were constructed by the SparCC method with a significance of P < 0.05 and correlation coefficient | R| > 0.40 on the integrated Network Analysis Pipeline (iNAP)2 (Feng et al., 2022). For each pH condition, the genera detected in more than 10% of samples were included for Spearman correlation analysis. The network properties were assessed by the “Global Network Properties and Individual Nodes’ Centralit” module. The within-module connectivity (Zi) and among-module connectivity (Pi) values were calculated by the “Module separation and module hubs” module. Based on the Zi and Pi values, the functional genera in co-occurrence work were classified into four topological roles: module hubs (Zi ≥ 2.5, Pi < 0.62), network hubs (Zi ≥ 2.5, Pi ≥ 0.62), connectors (Zi < 2.5, Pi ≥ 0.62) and peripherals (Zi < 2.5, Pi < 0.62) (Olesen et al., 2007). Among them, module hubs, network hubs and connectors have been considered microbial keystone taxa (Banerjee et al., 2016).
Results
The diversity of C-N-S-related microbial communities
Based on the predicted EC number for each OTU in the petroleum microbial communities, a total of 756 C-N-S-related functional genera were detected, including 176 carbon degradation genera, 28 methanogenesis genera, 31 CH4 oxidation genera, 206 N2 fixation genera, 29 ammoxidation genera, 447 denitrification genera, 447 DNRA genera and 122 sulfur reduction genera (Supplementary Table 2). Among these 756 functional genera, 375 participated in multiple element cycles (Supplementary Table 2). For example, 26 known methanogens can fix N2. The Chao1 richness and Shannon diversity indices for the total C-N-S-related microbial communities ranged from 5 to 257 and 0.012 to 5.33, respectively, which showed lower numbers at pH 4.5–6.5 (Chao1 36.39 ± 8.21 and Shannon 1.73 ± 0.24, respectively, Figures 1A,B).
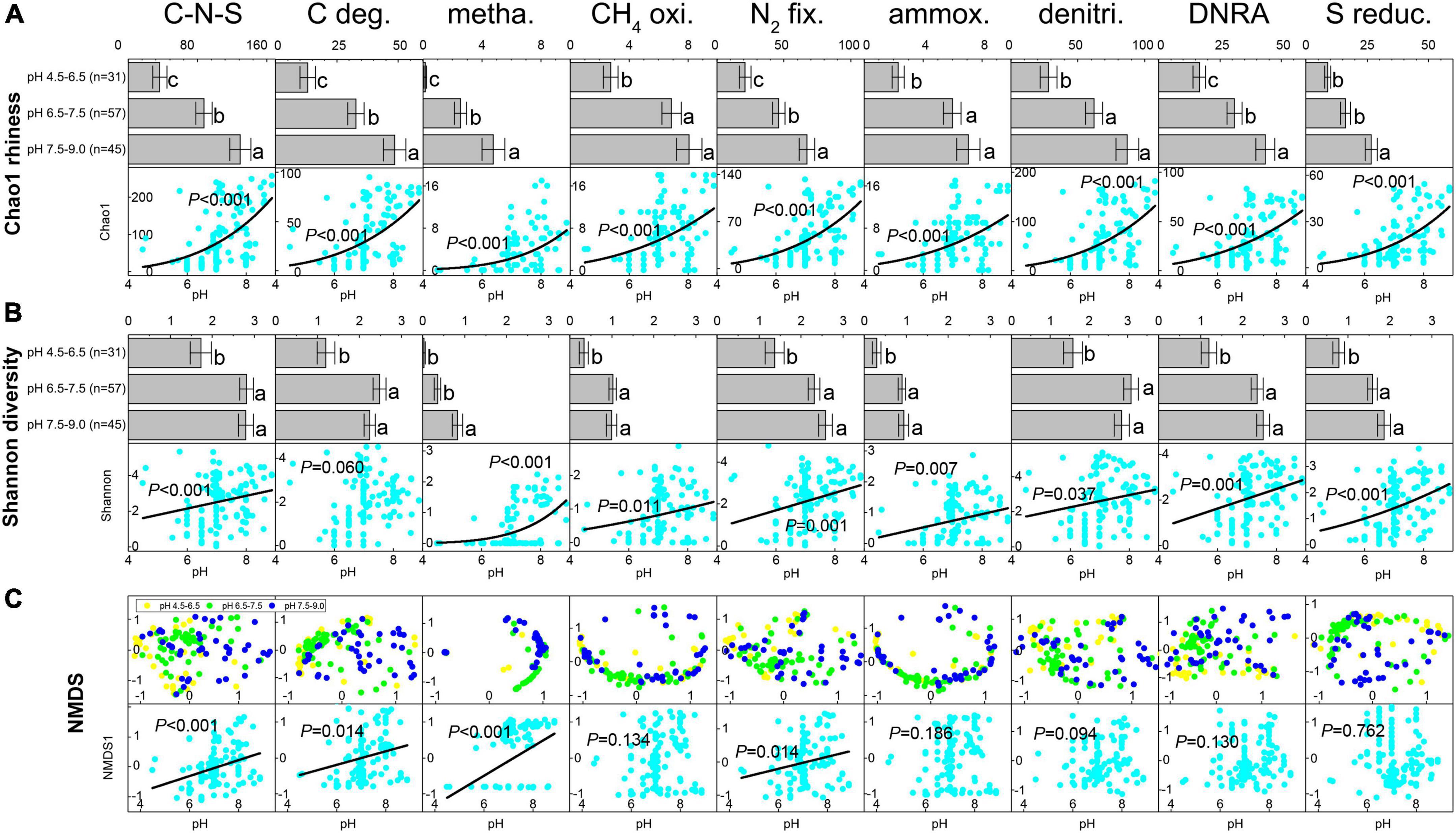
Figure 1. The shifts in Chao1 index (A) and Shannon index (B) for C-N-S-related microbial communities among different pH conditions. Difference letters indicated significant difference at p < 0.05. (C) Ordering of the composition of C-N-S-related microbial communities by non-metric multidimensional scaling (NMDS) using the Bray-Curtis distance index and the relationships between pH value and the first axes NMDS1 for the communities of C-N-S-related microbial communities. C-N-S, the total C-N-S-related microbial communities; C deg., carbon degradation; metha., methanogenesis; CH4 oxi., CH4 oxidation; N2 fix., N2 fixation; ammox., ammoxidation; denitri., denitrification; DNRA, dissimilatory nitrate reduction to ammonium; S reduc., sulfur reduction.
Among the relative abundance of the eight microbial groups, the relative abundance of N2 fixation and denitrification taxa was highest, followed by DNRA taxa, sulfur reduction taxa and carbon degradation taxa (Supplementary Table 3). The relative abundance of methanogenesis and ammoxidation taxa was the lowest. In addition, samples under acidic conditions with pH values of 4.5–6.5 exhibited the lowest relative number in methanogenesis but showed the highest relative abundance in CH4 oxidation, ammoxidation, denitrification, DNRA and sulfur reduction (Supplementary Table 3). In addition, the Chao1 richness and Shannon diversity indices among the eight microbial groups showed the lowest levels under acidic conditions with pH values of 4.5–6.5 (Figures 1A,B). The Chao1 richness and Shannon diversity indices in the majority of the eight microbial groups (except Shannon diversity in carbon degradation) increased with increasing pH (P < 0.05, Figures 1A,B).
The ordering of samples by NMDS (Figure 1C) showed that the total C-N-S-related microbial structures among different pH values shifted by a small amount along the first axis (NMDS1). Herein, the first axes for the communities of C-N-S, methanogenesis, carbon degradation and N2 fixation were significantly correlated with pH (Figure 1C). VPA also showed that pH rather than salinity, the essential metal iron (Ca2+ and Mg2+) and electron acceptors (NO32– and SO42–) were the most important factors influencing the C-N-S-related microbial communities (Figure 2A). The contribution of pH to the variation partitioning of the methanogenesis community was the highest (17.95%), followed by the communities of N2 fixation and carbon degradation (Figure 2B).
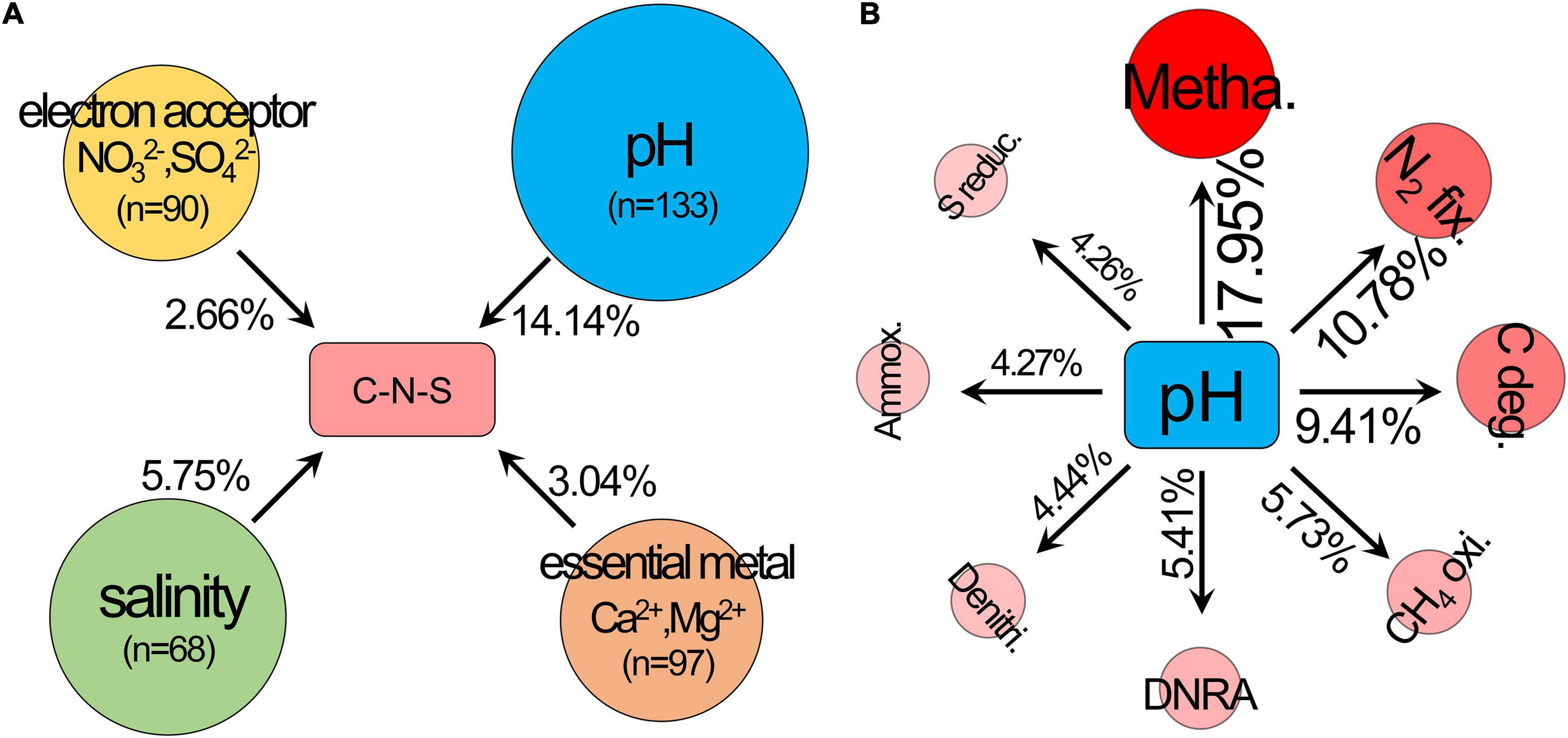
Figure 2. Variation partitioning analysis (VPA) was used to determine (A) the effects of pH, salinity, essential metal iron (Ca2+ and Mg2+) and electron acceptor (NO32– and SO42–) on the structure of the C-N-S-related microbial communities, (B) the effects of pH on the microbial community structures of carbon degradation, methanogenesis, CH4 oxidation, N2 fixation, ammoxidation, denitrification, dissimilatory nitrate reduction to ammonium (DNRA) and sulfur reduction. C deg., carbon degradation; metha., methanogenesis; CH4 oxi., CH4 oxidation; N2 fix., N2 fixation; ammox., ammoxidation; denitri., denitrification; DNRA, dissimilatory nitrate reduction to ammonium; S reduc., sulfur reduction.
The main C-N-S-related microbial genera and genes affected by pH
Among the 756 functional genera, 55 were influenced by pH (ANOVA, P < 0.05). These 55 functional genera (Supplementary Table 4) accounted for 30.20% of the total sequence reads and accounted for 42.08% of the C-N-S-related sequences. Among them, the top 20 genera (Figure 3) accounted for 29.53% of the total sequence reads, 18 of which had multiple functions. The genera Pseudomonas and Arcobacter were dominant and were mainly concentrated in acidic conditions with pH values of 4.5–6.5. The genus Pseudomonas, with most C-N-S functions except methanogenesis, showed the highest relative abundance (9.53 ± 1.72%) among the 55 functional genera, followed by Arcobacter (8.84 ± 2.12%). Some functional genera, including Methanolobus, Thermococcus, Sphaerochaeta, Desulfotignum, Achromobacter, Lactococcus, and Streptococcus, were concentrated in alkaline conditions with pH values of 7.5–9.0 (Figure 3). In addition, other genera were widely distributed in non-acidic environments with pH > 6.5. Among these genera, Methanothermococcus, Methanolobus and Thermococcus were dominant methanogenic groups and accounted for 5.22 ± 1.45%, 1.19 ± 0.55%, and 0.38 ± 0.13%, respectively.
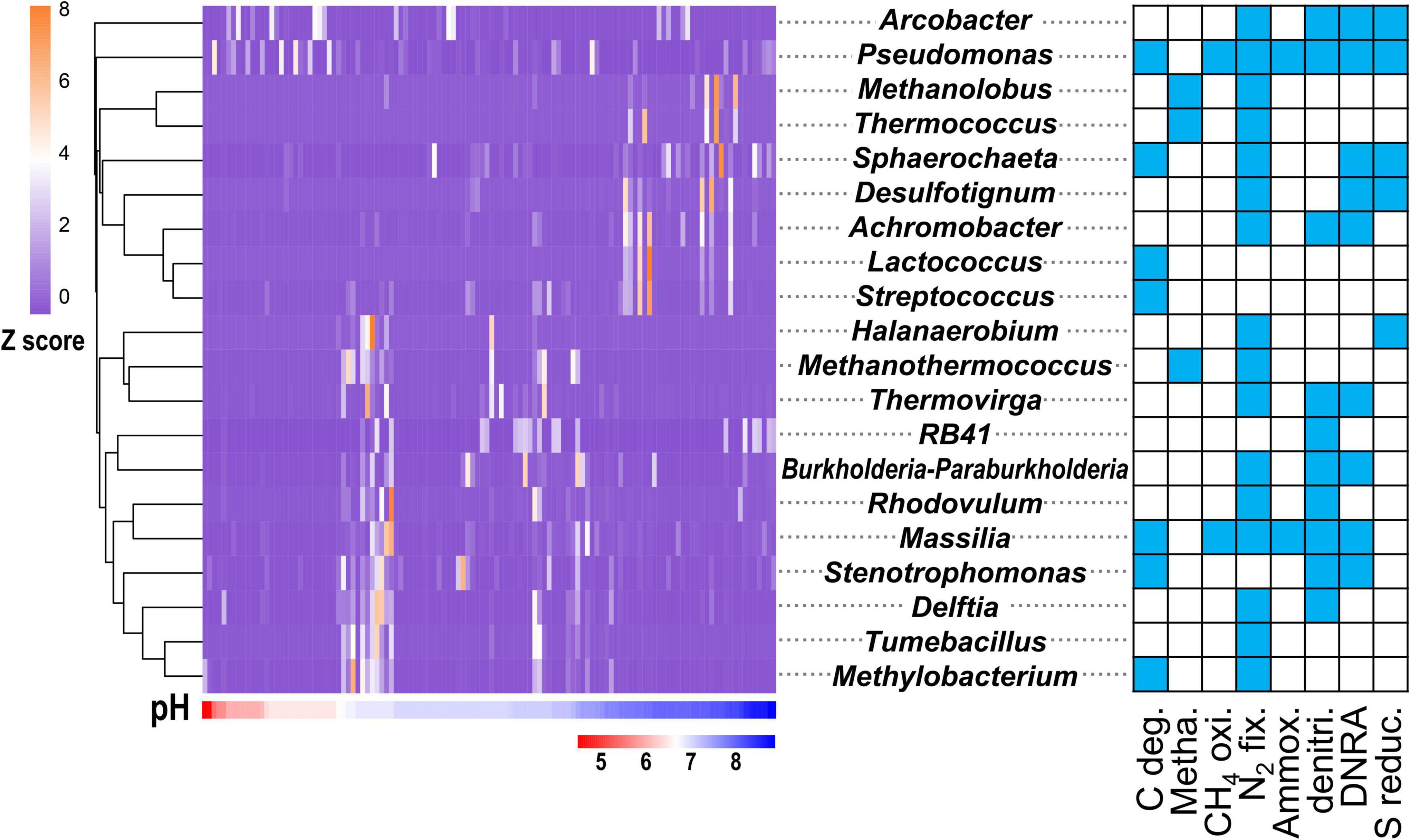
Figure 3. Relative abundance (exhibited by Z values) of top 20 genera affected by pH in the C-N-S-related microbial communities. The blue in the square indicates the corresponding function. The relative abundances of these genera are shown in Supplementary Table 4.
The relative abundance of 56 predicted C-N-S-related functional genes is shown in Figure 4. Among them, the cysH gene was the most abundant (4.98 × 10–4 ± 0.19 × 10–4). In addition, 16 genes were influenced by pH. Herein, putative chitinase (K03791, carbon degradation), mcrA (K00399, methanogenesis) and mtrB (K04480, methanogenesis) and cysH (K00390, sulfur reduction) genes in the neutral condition with pH at 6.5–7.5 were the highest in relative abundance among the samples. Genes E3.2.1.89 (K01224, carbon degradation), narGHIVYZ (K00370, K00371, K00374, denitrification), nirK (K00368, denitrification) and nirB (K00362, DNRA) in the acidic condition with pH at 4.5–6.5 were the highest in the relative abundances among the samples. The genes nifA (K03385, DNRA), sat (K00958, sulfur reduction), aprAB (K16950, K16951, sulfur reduction), and dsrAB (K11180, K11181, sulfur reduction) under alkaline conditions with pH values of 7.5–9.0 showed the highest relative abundance among the samples.
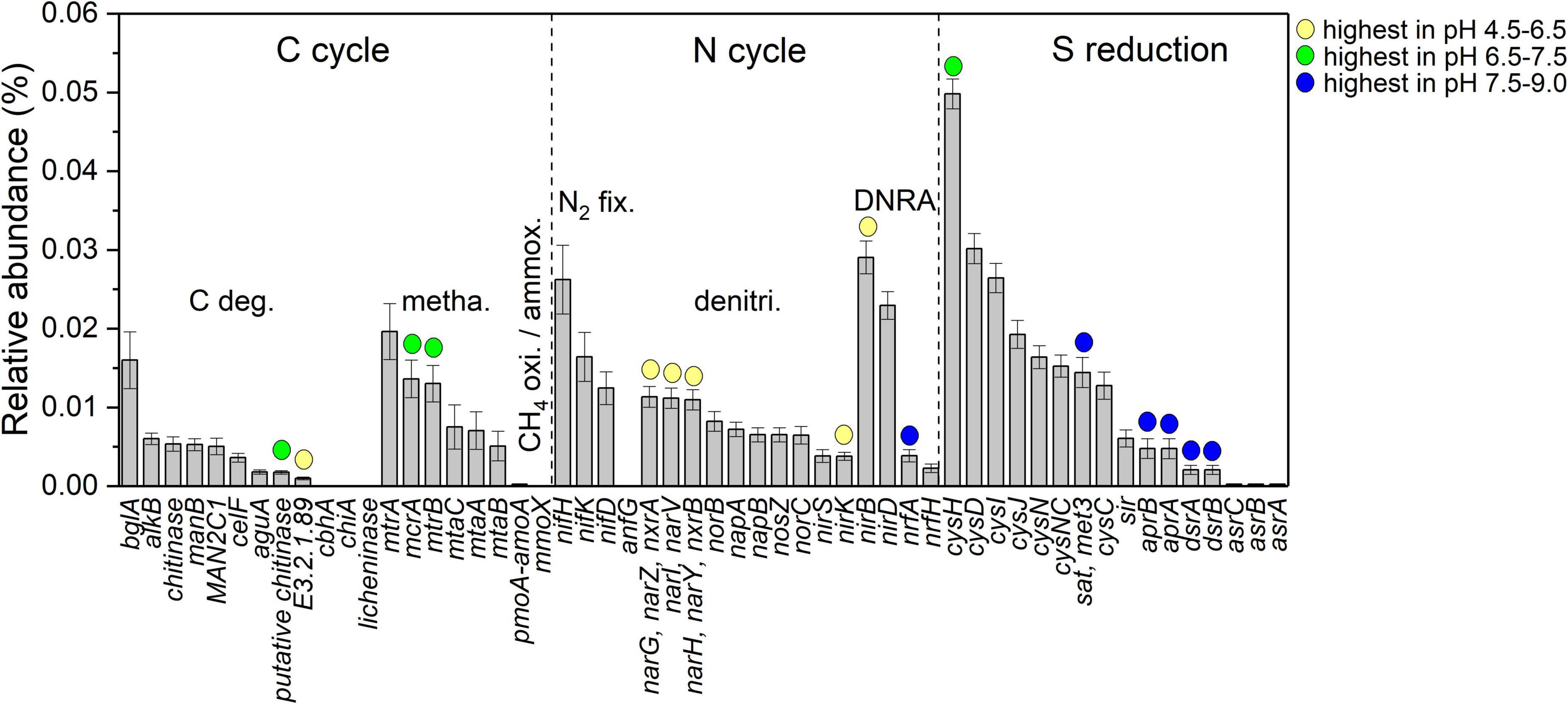
Figure 4. Comparison of different functional gene relative abundance among different pH conditions; yellow, green and blue circles indicate that the genes have the highest relative abundance in acidic condition with pH at 4.5–6.5, neutral condition with pH at 6.5–7.5 and alkaline condition with pH at 7.5–9.0, respectively (ANOVA with Tukey test, P < 0.05).
Co-occurrence networks of the C-N-S-related microbial communities among different pH conditions
The C-N-S-related co-occurrence networks among different pH conditions were constructed (Figure 5A) based on the 756 C-N-S-related functional genera. These three co-occurrence networks possessed scale-free topological properties, with R2 values of power-law ranging from 0.881 to 0.912 (Supplementary Table 5), which exhibited the non-randomized network structures. The multiple topological properties of the three C-N-S-related co-occurrence networks showed that the co-occurrence patterns shifted greatly among different pH conditions. The number of nodes increased from 56 to 342 with increasing pH, and the total links increased from 95 to 7577 with increasing pH (Figure 5B and Supplementary Table 5). In addition, microbial co-occurrence networks were also constructed (Supplementary Figure 1A). The relative abundances of C-N-S-related nodes to the total nodes decreased from 58.45 to 38.75% with increasing pH, and the relative abundances of the C-N-S-related links to the total links decreased from 80.61 to 63.48% with increasing pH (Supplementary Figure 1B).
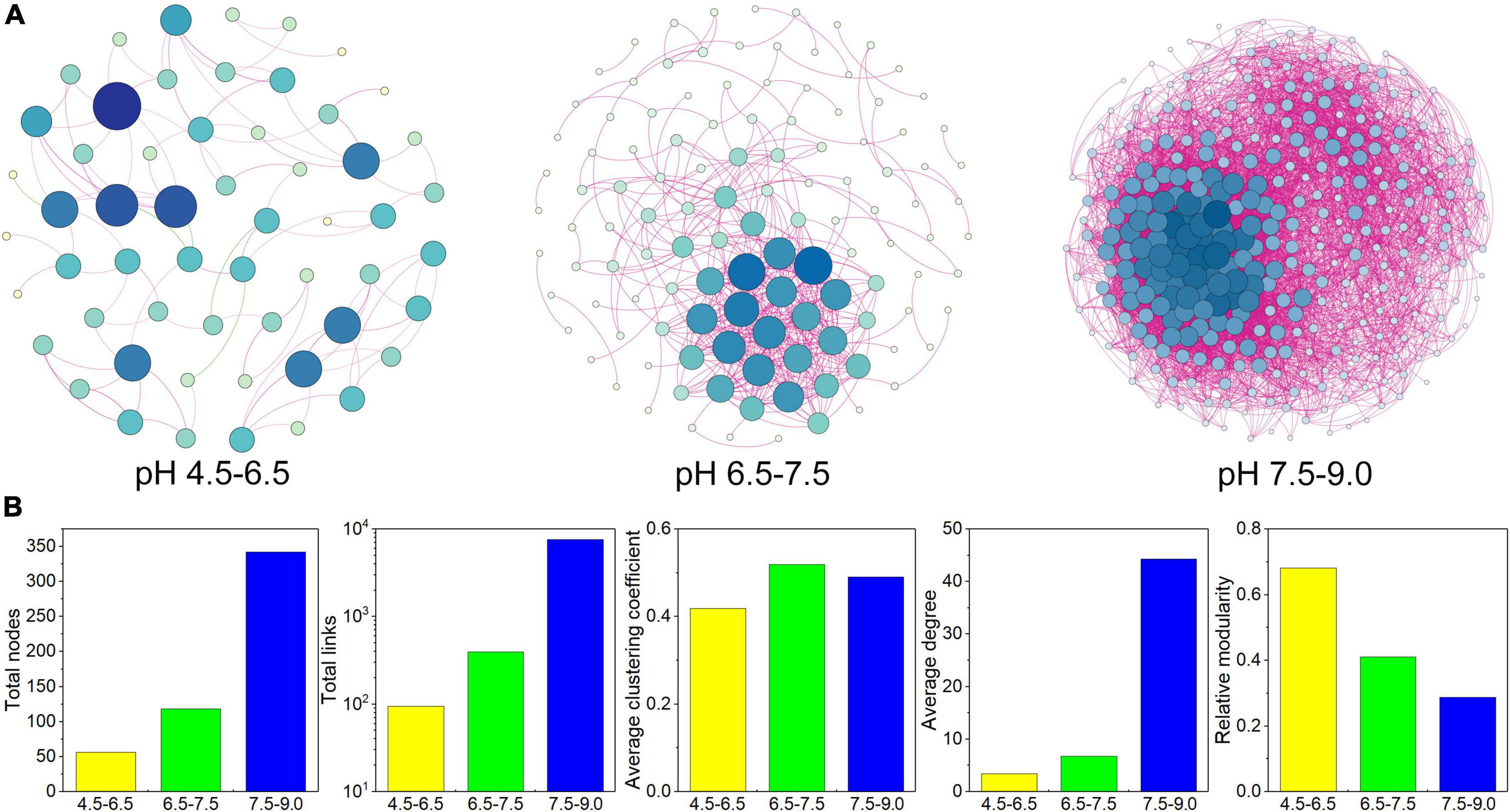
Figure 5. Co-occurrence network patterns of the C-N-S-related microbial communities among different pH conditions. (A) Visualization of constructed co-occurrence microbial networks. (B) Topological properties of the C-N-S-related microbial co-occurrence networks among different pH conditions.
In addition, the average clustering coefficient, average degree and connectedness of the C-N-S-related co-occurrence networks were the lowest in the acidic condition with pH at 4.5–6.5 (Figure 5B). Networks could be separated into 6, 22, and 4 modules under different pH conditions (Supplementary Table 5). In these modules, the number of nodes in the large modules (with at least 10 nodes) was 48.21% in the acidic condition with pH 4.5–6.5 and 100.00% in the alkaline condition with pH 7.5–9.0. Overall, these properties suggested that the C-N-S-related microbial communities under acidic conditions with pH values of 4.5–6.5 showed the lowest complex network.
A total of 0, 2, and 4 module hubs and a total of 4, 25, and 21 connectors were identified from the three networks in different pH conditions, respectively (Figure 6). Among them, Escherichia-Shigella was the connector that existed in all three networks. In addition, Sulfuricurvum was the connector detected in the networks of samples in acidic conditions with pH values of 4.5–6.5 and neutral conditions with pH values of 6.5–7.5. Several connectors between the networks of samples in the neutral condition with pH 6.5–7.5 and the alkaline condition with pH 7.5–9.0 were the same, including Burkholderia-Paraburkholderia, Candidatus Desulforudis, Candidatus Endomicrobium, Candidatus Nitrososphaera, Candidatus Solibacter, Corynebacterium 1, Prevotella 1, Ruminiclostridium 1, Ruminococcus 1, and Spirochaeta 2. All of these connectors, merely Arcobacter (connector in the acidic condition with pH at 4.5–6.5), Burkholderia-Paraburkholderia and Spirochaeta 2, were affected by pH (Supplementary Table 4).
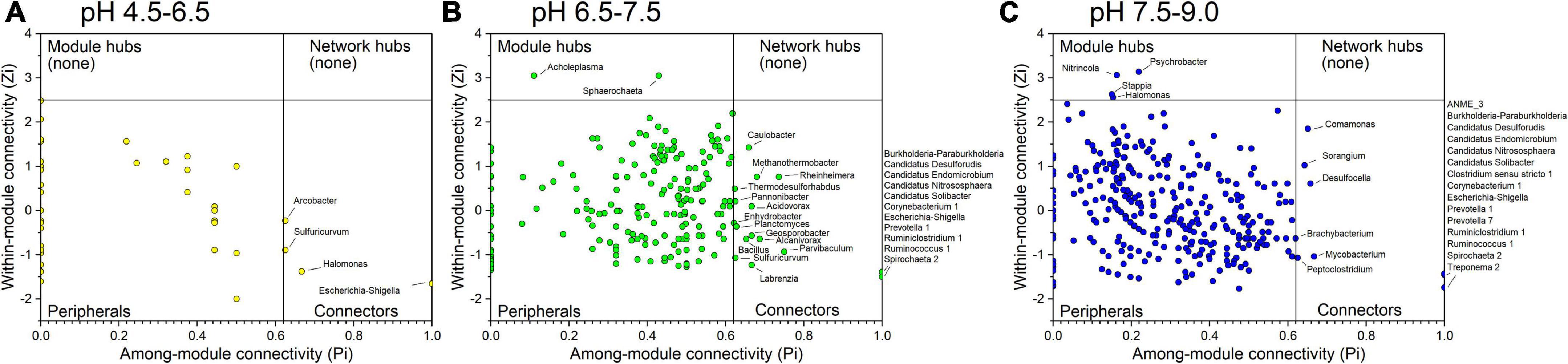
Figure 6. Identification of keystone taxa among different pH conditions based on their topological roles in networks. (A) acidic condition with pH at 4.5–6.5; (B) neutral condition with pH at 6.5–7.5; (C) alkaline condition with pH at 7.5–9.0. Module hubs are identified as Zi ≥ 2.5, Pi < 0.62, connectors are identified as Zi < 2.5, Pi ≥ 0.62, network hubs are identified as Zi ≥ 2.5, Pi ≥ 0.62.
Discussion
This study predicted 8567 genes in petroleum microbial data, and the groups with these 56 C-N-S-related genes accounted for 70.38 ± 20.88% of the total microbial communities. Among them, nitrogen- and sulfur-related microbes accounted for a relatively high proportion of the total microbial communities in this study, indicating that most groups participated in the nitrogen and sulfur biogeochemical cycles of petroleum. These results demonstrated the important role of these petroleum microorganisms in the petroleum C-N-S cycle, and the stable microecology in petroleum reservoirs was inseparable from the synergy of multiple functional microorganisms. In addition, these groups are mainly related to biodegradation, methanogenesis, and sulfate reduction (Zhou et al., 2019; Santos et al., 2020; de Sousa Pires et al., 2021), which are key hubs to realize practical production.
The main factor influencing the C-N-S-related microbial community was pH in the reported petroleum samples. The microbial diversity at the genus level changed greatly among different pH conditions and showed the trend that the microbial diversity indices increased with increasing pH (Figure 1). The impact of pH on microorganisms widely exists in various anaerobic environments, including lake sedimentary environments (Banda et al., 2021) and wetlands (Kang et al., 2021). The petroleum reservoirs are anaerobic, and pH can change the anaerobic fermentation process, including anaerobic degradation of organic carbon and methanogenesis (Zhou et al., 2021; Lv et al., 2022). In addition, pH might have a greater effect on microbial groups related to methanogenesis, carbon degradation and N2 fixation. Methanogenesis is an important anaerobic respiration process in anaerobic environments, but the methanogenesis community had the lowest abundance and biodiversity among the C-N-S-related microbial communities (Figure 1 and Supplementary Table 2). These phylotypes had lower abundance and narrower tolerance to environmental conditions among these eight functional groups. Carbon degradation and N2 fixation can provide available carbon and nitrogen for petroleum microorganisms. In particular, most of the pH-regulated genera were closely related to carbon degradation and nitrogen fixation (Figure 3). Microbial growth is influenced by many factors, the most important of which is the availability of nutrients. Therefore, the availability of nutrients and competition for them determines microbial community assembly (Dubinkina et al., 2019). Although petroleum is mainly composed of carbon elements, the lack of available nutrients could also (especially available C and N) limit microbial activity (Ali Khan et al., 2017). In addition, nutrient stimulation has been considered to improve microbial activities and further stimulate oil emulsification and gas production (Gao et al., 2014). Therefore, the response of these groups to pH is mainly to control energy metabolism and nutrient metabolism in petroleum microhabitats, thereby maintaining the stability of microorganisms.
The main anaerobic respiration modes possessed differences under different pH conditions, such as sulfate reduction under alkaline conditions with pH 7.5–9.0, methanogenesis under neutral conditions with pH 6.5–7.5, and denitrification under acidic conditions with pH 4.5–6.5. This result indicated the difference in the main electron transport modes in petroleum microhabitats (Wartell et al., 2021), and as a result, electron acceptors were also important factors in regulating petroleum microorganisms (Figure 2). In the field of petroleum microorganisms, sulfate reduction is also a biochemical process that has attracted much attention. For example, SRB containing dsr genes are capable of sulfate reduction with iron as an electron donor, resulting in electrical microbially influenced corrosion (EMIC) (Santos et al., 2020). In addition, these microorganisms were capable of producing H2S through sulfate dissimilatory reduction. The accumulation of H2S can lead to acidification of the oil, which can damage the characteristics and quality of the oil, thereby posing an explosion threat and a threat to human health (Varjani and Gnansounou, 2017). This study found that the sulfur reduction genes here were mainly dominated by the cys genes, not the dsr genes encoding sulfate dissimilatory reduction (Figure 4), indicating that sulfate in petroleum reservoirs was mainly utilized by microbial communities through the assimilatory sulfate reduction pathway. Several Cys enzymes were used to synthesize sulfites and convert sulfates into sulfides, and the existence of sulfate utilization enhanced the bacterial ability to produce amino acids, such as cysteine and methionine (Tikariha and Purohit, 2019). This process provided biosulfur for the microbial communities in petroleum reservoirs.
This study showed C-N-S-related microbial co-occurrence networks in petroleum reservoirs with various patterns among different pH conditions, which were mainly determined by microbial keystone groups that survived under different pH conditions. The unique biological interactions of keystone taxa maintain the stability of functional microbial networks (Coux et al., 2016). Many studies have generally focused on the predominant microbial populations, while specific microorganisms, such as keystone taxa, could also play unique and important roles in community structure and function (Ma B. et al., 2020; Zhou et al., 2022). For example, Pseudomonas affected by pH showed a higher relative abundance and was dominant and mainly concentrated in acidic environments but did not exhibit keystone taxa in the microbial co-occurrence network. Pseudomonas is a bacterial genus that has been reported to be ubiquitous in microbial communities of petroleum reservoirs (Gao P. K. et al., 2015; Zhou et al., 2020). It is precisely because of the existence of multiple functions of this group that it has different metabolic potentials, allowing it to persist and grow in a wide range of petroleum reserve environments and to utilize a variety of carbon compounds under special environmental conditions. Their lifestyle may be opportunotrophic, as described by Singer et al. (2011). In addition, Vick et al. (2019) observed two Pseudomonas species with markedly different metabolic and ecological lifestyles, reflecting the broad metabolic and lifestyle diversity within such taxa, from parasitic to mutually beneficial (Polz et al., 2006) and free-living lifestyles. Among the keystone taxa, only Escherichia-Shigella was the key connector that existed in all three networks, which showed that the keystone taxa maintaining the C-N-S microbial network changed dramatically with shifting pH. Escherichia-Shigella did not change in relative abundance under different pH conditions, which has not received widespread attention in the field of petroleum microorganisms, but it is considered to play an important role in the degradation of petroleum hydrocarbons (Cui et al., 2020). In addition, most of the keystone taxa were the same in non-acidic environments (pH > 6.5) but were different from those in acidic conditions. It was suggested that acidity leads to the reduction of keystone taxa and may eventually lead to the collapse of modules and networks (Coux et al., 2016; Liu et al., 2022). The genus Sulfuricurvum coexists in neutral and acidic conditions (Figure 6). It may be an important keystone taxon involved in nitrogen and sulfur metabolism in petroleum reservoirs. They grow best in pH 7.0 and low-intensity salt medium and use sulfide, elemental sulfur, thiosulfate and hydrogen as electron donors and nitrate as an electron acceptor under anaerobic conditions (Kodama and Watanabe, 2004).
Conclusion
This study comprehensively demonstrated the C-N-S-related microbial communities and functional genes in petroleum reservoirs under different pH conditions. Nitrogen- and sulfur-related microbes accounted for a relatively high proportion of the total microbial communities in petroleum reservoirs. In addition, the pH was the main factor influencing the C-N-S-related microbial diversities and microbial groups participating in methanogenesis, carbon degradation and N2 fixation. In addition, C-N-S-related microbial co-occurrence networks in petroleum reservoirs showed various patterns with different microbial keystone groups among different pH conditions. The interrelationship of these C-N-S-related microbial communities ultimately affects the microhabitat in petroleum reservoirs and has important implications for the decomposition of organic matter in petroleum reservoirs and the geochemical cycle.
Data availability statement
The original contributions presented in this study are included in the article/Supplementary material, further inquiries can be directed to the corresponding author/s.
Author contributions
YL, YZ, and SX conducted the bulk of the data analysis for the study and co-wrote the manuscript. YL and SX provided the funding for the study and were involved in the conceptualization of the study, as well as assisting in writing of the manuscript. All authors read and approved the final manuscript.
Funding
This study was funded by the Key Research and Development Projects in Anhui Province (202004a07020054) and the Independent Research Fund of the State Key Laboratory of Mining Response and Disaster Prevention and Control in Deep Coal Mines at Anhui University of Science and Technology (SKLMRDPC20ZZ08).
Conflict of interest
The authors declare that the research was conducted in the absence of any commercial or financial relationships that could be construed as a potential conflict of interest.
Publisher’s note
All claims expressed in this article are solely those of the authors and do not necessarily represent those of their affiliated organizations, or those of the publisher, the editors and the reviewers. Any product that may be evaluated in this article, or claim that may be made by its manufacturer, is not guaranteed or endorsed by the publisher.
Supplementary material
The Supplementary Material for this article can be found online at: https://www.frontiersin.org/articles/10.3389/fmicb.2022.952285/full#supplementary-material
Footnotes
References
Ali Khan, A. H., Tanveer, S., Alia, S., Anees, M., Sultan, A., Iqbal, M., et al. (2017). Role of nutrients in bacterial biosurfactant production and effect of biosurfactant production on petroleum hydrocarbon biodegradation. Ecol. Eng. 104, 158–164. doi: 10.1016/j.ecoleng.2017.04.023
Aurepatipan, N., Champreda, V., Kanokratana, P., Chitov, T., and Bovonsombut, S. (2018). Assessment of bacterial communities and activities of thermotolerant enzymes produced by bacteria indigenous to oil-bearing sandstone cores for potential application in Enhanced Oil Recovery. J. Pet. Sci. Eng. 163, 295–302. doi: 10.1016/j.petrol.2017.12.077
Banda, J. F., Zhang, Q., Ma, L., Pei, L., Du, Z., Hao, C., et al. (2021). Both pH and salinity shape the microbial communities of the lakes in Badain Jaran Desert, NW China. Sci. Total Environ. 791:148108. doi: 10.1016/j.scitotenv.2021.148108
Banerjee, S., Baah-Acheamfour, M., Carlyle, C. N., Bissett, A., Richardson, A. E., Siddique, T., et al. (2016). Determinants of bacterial communities in Canadian agroforestry systems. Environ. Microbiol. 18, 1805–1816. doi: 10.1111/1462-2920.12986
Banerjee, S., Schlaeppi, K., and Van Der Heijden, M. G. A. (2018). Keystone taxa as drivers of microbiome structure and functioning. Nat. Rev. Microbiol. 16, 567–576. doi: 10.1038/s41579-018-0024-1
Brown, L. R. (2010). Microbial enhanced oil recovery (MEOR). Curr. Opin. Microbiol. 13, 316–320. doi: 10.1016/j.mib.2010.01.011
Coux, C., Rader, R., Bartomeus, I., and Tylianakis, J. M. (2016). Linking species functional roles to their network roles. Ecol. Lett. 19, 762–770. doi: 10.1111/ele.12612
Cui, J.-Q., He, Q.-S., Liu, M.-H., Sun, M.-B., and Wen, J.-P. (2020). Comparative study on different remediation strategies applied in petroleum-contaminated soils. Int. J. Environ. Res. Public Health 17:1606. doi: 10.3390/ijerph17051606
de Sousa Pires, A., Dias, G. M., Chagas, De Oliveira Mariano, D., Akamine, R. N., Cruz De Albuquerque, A. C., et al. (2021). Molecular diversity and abundance of the microbial community associated to an offshore oil field on the southeast of Brazil. Int. Biodeterior. Biodegradation 160:105215. doi: 10.1016/j.ibiod.2021.105215
Douglas, G. M., Maffei, V. J., Zaneveld, J. R., Yurgel, S. N., Brown, J. R., Taylor, C. M., et al. (2020). PICRUSt2 for prediction of metagenome functions. Nat. Biotechnol. 38, 685–688. doi: 10.1038/s41587-020-0548-6
Dubinkina, V., Fridman, Y., Pandey, P. P., and Maslov, S. (2019). Multistability and regime shifts in microbial communities explained by competition for essential nutrients. Elife 8:e49720. doi: 10.7554/eLife.49720
Feng, K., Peng, X., Zhang, Z., Gu, S., He, Q., Shen, W., et al. (2022). iNAP: An integrated network analysis pipeline for microbiome studies. iMeta 1:e13. doi: 10.1002/imt2.13
Gao, P., Li, G., Dai, X., Dai, L., Wang, H., Zhao, L., et al. (2013). Nutrients and oxygen alter reservoir biochemical characters and enhance oil recovery during biostimulation. World J. Microbiol. Biotechnol. 29, 2045–2054. doi: 10.1007/s11274-013-1367-4
Gao, P., Tian, H., Li, G., Sun, H., and Ma, T. (2015). Microbial diversity and abundance in the Xinjiang Luliang long-term water-flooding petroleum reservoir. MicrobiologyOpen 4, 332–342. doi: 10.1002/mbo3.241
Gao, P. K., Li, G. Q., Tian, H. M., Wang, Y. S., Sun, H. W., and Ma, T. (2015). Differences in microbial community composition between injection and production water samples of water flooding petroleum reservoirs. Biogeosciences 12, 3403–3414. doi: 10.5194/bg-12-3403-2015
Gao, P., Tian, H., Wang, Y., Li, Y., Li, Y., Xie, J., et al. (2016). Spatial isolation and environmental factors drive distinct bacterial and archaeal communities in different types of petroleum reservoirs in China. Sci. Rep. 6:20174. doi: 10.1038/srep20174
Gao, P.-K., Li, G.-Q., Zhao, L.-X., Dai, X.-C., Tian, H.-M., Dai, L.-B., et al. (2014). Dynamic processes of indigenous microorganisms from a low-temperature petroleum reservoir during nutrient stimulation. J. Biosci. Bioeng. 117, 215–221. doi: 10.1016/j.jbiosc.2013.07.009
Kang, E., Li, Y., Zhang, X., Yan, Z., Wu, H., Li, M., et al. (2021). Soil pH and nutrients shape the vertical distribution of microbial communities in an alpine wetland. Sci. Total Environ. 774:145780. doi: 10.1016/j.scitotenv.2021.145780
Kodama, Y., and Watanabe, K. (2004). Sulfuricurvum kujiense gen. nov., sp. nov., a facultatively anaerobic, chemolithoautotrophic, sulfur-oxidizing bacterium isolated from an underground crude-oil storage cavity. Int. J. Syst. Evol. Microbiol. 54, 2297–2300. doi: 10.1099/ijs.0.63243-0
Lenchi, N., Inceoðlu, O., Kebbouche-Gana, S., Gana, M. L., Llirós, M., Servais, P., et al. (2013). Diversity of microbial communities in production and injection waters of Algerian oilfields revealed by 16S rRNA gene amplicon 454 pyrosequencing. PLoS One 8:e66588. doi: 10.1371/journal.pone.0066588
Li, C.-Y., Zhang, D., Li, X.-X., Mbadinga, S. M., Yang, S.-Z., Liu, J.-F., et al. (2016). The biofilm property and its correlationship with high-molecular-weight polyacrylamide degradation in a water injection pipeline of Daqing oilfield. J. Hazard. Mater. 304, 388–399. doi: 10.1016/j.jhazmat.2015.10.067
Li, L., Li, Z., Song, K., Gu, Y., Gao, X., and Zhao, X. (2021). Short-chain fatty acids resource recovery potential from algal sludge via anaerobic fermentation under various pH values. Chemosphere 275:129954. doi: 10.1016/j.chemosphere.2021.129954
Li, X.-X., Mbadinga, S. M., Liu, J.-F., Zhou, L., Yang, S.-Z., Gu, J.-D., et al. (2017b). Microbiota and their affiliation with physiochemical characteristics of different subsurface petroleum reservoirs. Int. Biodeterior. Biodegradation 120, 170–185. doi: 10.1016/j.ibiod.2017.02.005
Li, X.-X., Liu, J.-F., Zhou, L., Mbadinga, S. M., Yang, S.-Z., Gu, J.-D., et al. (2017a). Diversity and composition of sulfate-reducing microbial communities based on genomic DNA and RNA transcription in production water of high temperature and corrosive oil reservoir. Front. Microbiol. 8:1011. doi: 10.3389/fmicb.2017.01011
Liang, B., Wang, L.-Y., Mbadinga, S. M., Liu, J.-F., Yang, S.-Z., Gu, J.-D., et al. (2015). Anaerolineaceae and Methanosaeta turned to be the dominant microorganisms in alkanes-dependent methanogenic culture after long-term of incubation. AMB Express 5:37. doi: 10.1186/s13568-015-0117-4
Liu, S., Yu, H., Yu, Y., Huang, J., Zhou, Z., Zeng, J., et al. (2022). Ecological stability of microbial communities in Lake Donghu regulated by keystone taxa. Ecol. Indic. 136:108695. doi: 10.1016/j.ecolind.2022.108695
Lv, N., Cai, G., Pan, X., Li, Y., Wang, R., Li, J., et al. (2022). pH and hydraulic retention time regulation for anaerobic fermentation: Focus on volatile fatty acids production/distribution, microbial community succession and interactive correlation. Bioresour. Technol. 347:126310. doi: 10.1016/j.biortech.2021.126310
Ma, B., Wang, Y., Ye, S., Liu, S., Stirling, E., Gilbert, J. A., et al. (2020). Earth microbial co-occurrence network reveals interconnection pattern across microbiomes. Microbiome 8:82. doi: 10.1186/s40168-020-00857-2
Ma, Q., Liu, S., Li, S., Hu, J., Tang, M., and Sun, Y. (2020). Removal of malodorant skatole by two enriched microbial consortia: Performance, dynamic, function prediction and bacteria isolation. Sci. Total Environ. 725:138416. doi: 10.1016/j.scitotenv.2020.138416
Magot, M., Ollivier, B., and Patel, B. K. C. (2000). Microbiology of petroleum reservoirs. Antonie Van Leeuwenhoek 77, 103–116. doi: 10.1023/A:1002434330514
Mand, J., Park, H. S., Okoro, C., Lomans, B. P., Smith, S., Chiejina, L., et al. (2016). Microbial methane production associated with carbon steel corrosion in a Nigerian oil field. Front. Microbiol. 6:1538. doi: 10.3389/fmicb.2015.01538
Mansilla, M. C., Albanesi, D., and Mendoza, D. D. (2000). Transcriptional control of the sulfur-regulated cysH operon, containing genes involved inL-cysteine biosynthesis in Bacillus subtilis. J. Bacteriol. 182, 5885–5892. doi: 10.1128/JB.182.20.5885-5892.2000
Mbadinga, S. M., Wang, L.-Y., Zhou, L., Liu, J.-F., Gu, J.-D., and Mu, B.-Z. (2011). Microbial communities involved in anaerobic degradation of alkanes. Int. Biodeterior. Biodegradation 65, 1–13. doi: 10.1016/j.ibiod.2010.11.009
Meslé, M., Dromart, G., and Oger, P. (2013). Microbial methanogenesis in subsurface oil and coal. Res. Microbiol. 164, 959–972. doi: 10.1016/j.resmic.2013.07.004
Mougi, A., and Kondoh, M. (2012). Diversity of interaction types and ecological community stability. Science 337, 349–351. doi: 10.1126/science.1220529
Muyzer, G., and Stams, A. J. M. (2008). The ecology and biotechnology of sulphate-reducing bacteria. Nat. Rev. Microbiol. 6, 441–454. doi: 10.1038/nrmicro1892
Narenkumar, J., Alsalhi, M. S., Arul Prakash, A., Abilaji, S., Devanesan, S., Rajasekar, A., et al. (2019). Impact and role of bacterial communities on biocorrosion of metals used in the processing industry. ACS Omega 4, 21353–21360. doi: 10.1021/acsomega.9b02954
Nazina, T., Sokolova, D., Grouzdev, D., Semenova, E., Babich, T., Bidzhieva, S., et al. (2019). The potential application of microorganisms for sustainable petroleum recovery from heavy oil reservoirs. Sustainability 12, 1–23. doi: 10.3390/su12010015
Olesen, J. M., Bascompte, J., Dupont, Y. L., and Jordano, P. (2007). The modularity of pollination networks. Proc. Natl. Acad. Sci. U.S.A. 104, 19891–19896. doi: 10.1073/pnas.0706375104
Phetcharat, T., Dawkrajai, P., Chitov, T., Wongpornchai, P., Saenton, S., Mhuantong, W., et al. (2019). Effect of inorganic nutrients on bacterial community composition in oil-bearing sandstones from the subsurface strata of an onshore oil reservoir and its potential use in microbial enhanced oil recovery. PLoS One 13:e0198050. doi: 10.1371/journal.pone.0198050
Polz, M., Hunt, D., Preheim, S., and Weinreich, D. (2006). Patterns and mechanisms of genetic and phenotypic differentiation in marine microbes. Philos. Trans. R. Soc. B 361, 2009–2021. doi: 10.1098/rstb.2006.1928
Qian, J. J., and Akçay, E. (2020). The balance of interaction types determines the assembly and stability of ecological communities. Nat. Ecol. Evol. 4, 356–365. doi: 10.1038/s41559-020-1121-x
Quraishi, M., Bhatia, S. K., Pandit, S., Gupta, P. K., Rangarajan, V., Lahiri, D., et al. (2021). Exploiting microbes in the petroleum field: Analyzing the credibility of microbial enhanced oil recovery (MEOR). Energies 14:4684. doi: 10.3390/en14154684
Santos, J. C. D., Lopes, D. R. G., Da Silva, J. D., De Oliveira, M. D., Dias, R. S., Lima, H. S., et al. (2020). Diversity of sulfate-reducing prokaryotes in petroleum production water and oil samples. Int. Biodeterior. Biodegradation 151:104966. doi: 10.1016/j.ibiod.2020.104966
Shelton, J. L., Akob, D. M., Mcintosh, J. C., Fierer, N., Spear, J. R., Warwick, P. D., et al. (2016). Environmental drivers of differences in microbial community structure in Crude oil reservoirs across a methanogenic gradient. Front. Microbiol. 7:1535. doi: 10.3389/fmicb.2016.01535
Singer, E., Webb, E. A., Nelson, W. C., Heidelberg, J. F., Ivanova, N., Pati, A., et al. (2011). Genomic potential of Marinobacter aquaeolei, a biogeochemical “Opportunitroph”. Appl. Environ. Microbiol. 77, 2763–2771. doi: 10.1128/AEM.01866-10
Tang, K., Baskaran, V., and Nemati, M. (2009). Bacteria of the sulphur cycle: An overview of microbiology, biokinetics and their role in petroleum and mining industries. Biochem. Eng. J. 44, 73–94. doi: 10.1016/j.bej.2008.12.011
Tikariha, H., and Purohit, H. J. (2019). Assembling a genome for novel nitrogen-fixing bacteria with capabilities for utilization of aromatic hydrocarbons. Genomics 111, 1824–1830. doi: 10.1016/j.ygeno.2018.12.005
Varjani, S. J., and Gnansounou, E. (2017). Microbial dynamics in petroleum oilfields and their relationship with physiological properties of petroleum oil reservoirs. Bioresour. Technol. 245, 1258–1265. doi: 10.1016/j.biortech.2017.08.028
Vick, S. H. W., Greenfield, P., Tetu, S. G., Midgley, D. J., and Paulsen, I. T. (2019). Genomic and phenotypic insights point to diverse ecological strategies by facultative anaerobes obtained from subsurface coal seams. Sci. Rep. 9:16186. doi: 10.1038/s41598-019-52846-7
Wang, B., Zheng, X., Zhang, H., Xiao, F., He, Z., and Yan, Q. (2020). Keystone taxa of water microbiome respond to environmental quality and predict water contamination. Environ. Res. 187:109666. doi: 10.1016/j.envres.2020.109666
Wartell, B., Boufadel, M., and Rodriguez-Freire, L. (2021). An effort to understand and improve the anaerobic biodegradation of petroleum hydrocarbons: A literature review. Int. Biodeterior. Biodegradation 157:105156 doi: 10.1016/j.ibiod.2020.105156
Xue, Y., Chen, H., Yang, J. R., Liu, M., Huang, B., and Yang, J. (2018). Distinct patterns and processes of abundant and rare eukaryotic plankton communities following a reservoir cyanobacterial bloom. ISME J. 12, 2263–2277. doi: 10.1038/s41396-018-0159-0
Zagrodnik, R., and Łaniecki, M. (2017). The effect of pH on cooperation between dark- and photo-fermentative bacteria in a co-culture process for hydrogen production from starch. Int. J. Hydrog. Energy 42, 2878–2888. doi: 10.1016/j.ijhydene.2016.12.150
Zhou, J., Theroux, S. M., Bueno De Mesquita, C. P., Hartman, W. H., Tian, Y., and Tringe, S. G. (2022). Microbial drivers of methane emissions from unrestored industrial salt ponds. ISME J. 16, 284–295. doi: 10.1038/s41396-021-01067-w
Zhou, L., Lu, Y.-W., Wang, D.-W., Zhang, S.-L., Tang, E.-G., Qi, Z.-Z., et al. (2020). Microbial community composition and diversity in production water of a high-temperature offshore oil reservoir assessed by DNA- and RNA-based analyses. Int. Biodeterior. Biodegradation 151:104970. doi: 10.1016/j.ibiod.2020.104970
Zhou, X., Lu, Y., Huang, L., Zhang, Q., Wang, X., and Zhu, J. (2021). Effect of pH on volatile fatty acid production and the microbial community during anaerobic digestion of Chinese cabbage waste. Bioresour. Technol. 336:125338. doi: 10.1016/j.biortech.2021.125338
Zhou, Z., Liang, B., Wang, L. Y., Liu, J. F., Mu, B. Z., Shim, H., et al. (2019). Identifying the core bacterial microbiome of hydrocarbon degradation and a shift of dominant methanogenesis pathways in the oil and aqueous phases of petroleum reservoirs of different temperatures from China. Biogeosciences 16, 4229–4241. doi: 10.5194/bg-16-4229-2019
Keywords: petroleum acidification, functional communities, degradation, co-occurrence network, keystone taxa
Citation: Li Y, Zhang Y and Xue S (2022) pH mediated assemblage of carbon, nitrogen, and sulfur related microbial communities in petroleum reservoirs. Front. Microbiol. 13:952285. doi: 10.3389/fmicb.2022.952285
Received: 24 May 2022; Accepted: 26 August 2022;
Published: 14 September 2022.
Edited by:
Katrin Wendt-Potthoff, Helmholtz Association of German Research Centres (HZ), GermanyReviewed by:
Huimei Tian, Shandong Agricultural University, ChinaYang Yang, Nanjing Agricultural University, China
Bruna Dellagnezze, State University of Campinas, Brazil
Kelly Johanna Hidalgo Martinez, State University of Campinas, Brazil
Copyright © 2022 Li, Zhang and Xue. This is an open-access article distributed under the terms of the Creative Commons Attribution License (CC BY). The use, distribution or reproduction in other forums is permitted, provided the original author(s) and the copyright owner(s) are credited and that the original publication in this journal is cited, in accordance with accepted academic practice. No use, distribution or reproduction is permitted which does not comply with these terms.
*Correspondence: Yang Li, bGl5YW5nMjAxMzAxMDRAMTYzLmNvbQ==; bGl5YW5nX2F1c3RAMTYzLmNvbQ==; orcid.org/0000-0002-8946-3962