- 1Department of Stomatology, The First Affiliated Hospital of Bengbu Medical College, Bengbu, China
- 2Department of Stomatology, Bengbu Medical College, Bengbu, China
- 3Department of Biochemistry and Molecular Biology, Bengbu Medical College, Bengbu, China
- 4Department of Histology and Embryology, Bengbu Medical College, Bengbu, China
- 5Department of Epidemiology and Health Statistics, Bengbu Medical College, Bengbu, China
- 6Anhui Key Laboratory of Infection and Immunity, Bengbu Medical College, Bengbu, China
Streptococcus mutans (S. mutans) is one of the primary pathogens responsible for dental caries. Streptococcus gordonii (S. gordonii) is one of the early colonizers of dental plaque and can compete with S. mutans for growth. In the present analysis, we explored key target genes against S. gordonii in S. mutans using 80 S. mutans clinical isolates with varying capabilities against S. gordonii. A principal coordinate analysis revealed significant genetic diversity differences between antagonistic and non-antagonistic groups. Genomic comparisons revealed 33 and 61 genes that were, respectively, positively and negatively correlated with S. mutans against S. gordonii, with RNA-sequencing (RNA-seq) highlighting 11 and 43 genes that were, respectively, upregulated and downregulated in the antagonistic group. Through a combination of these results and antiSMASH analysis, we selected 16 genes for qRT-PCR validation in which the expression levels of SMU_137 (malate dehydrogenase, mleS), SMU_138 (malate permease, mleP), SMU_139 (oxalate decarboxylase, oxdC), and SMU_140 (glutathione reductase) were consistent with RNA-seq results. SMU_1315c-1317c (SMU_1315c transport-related gene) and SMU_1908c-1909c were, respectively, downregulated and upregulated in the antagonistic group. The expression patterns of adjacent genes were closely related, with correlation coefficient values greater than 0.9. These data reveal new targets (SMU_137–140, SMU_1315c-1317c, and SMU_1908c-1909c) for investigating the critical gene clusters against S. gordonii in S. mutans clinical isolates.
Introduction
Dental caries is a highly prevalent infectious bacterial disease, ranking first in prevalence among 328 diseases worldwide in 2016 (Vos et al., 2017). Dental caries can adversely impact the quality of life of affected patients, resulting in complications including pain and, in severe cases, tooth loss (Aldosari et al., 2021; Amarasena et al., 2021; Santos et al., 2021). Streptococcus mutans (S. mutans) is an essential cariogenic bacterium that metabolizes carbohydrates within the oral cavity and thereby lowers the local pH at the tooth surface (Loesche, 1986; OmerOglou et al., 2022). When this pH value drops to 5.5 or below, it can result in tooth surface demineralization and dental caries development (He et al., 2022).
Streptococcus gordonii (S. gordonii) is a Gram-positive bacterium included among some of the initial colonizers of the dental plaque biofilm (Pearce et al., 1995). The metabolic production of hydrogen peroxide produced by S. gordonii can inhibit the growth of S. mutans, and the production of alkaline ammonia can mitigate the localized acidity on the tooth surface, thereby helping to suppress cariogenesis (Liu and Burne, 2009; Cheng et al., 2020; Liu et al., 2022). Studies also showed that the detection rate of S. mutans in dental plaque is positively correlated with dental caries, while the detection rate of S. gordonii is negatively associated with dental caries (Saraithong et al., 2015; Tao et al., 2015). The activity of S. mutans to antagonize S. gordonii and colonize the tooth surface is thus a prerequisite for its cariogenic activity.
S. gordonii, Streptococcus sanguinis and other pioneer bacteria are effectively suppressed by mutacin IV, a bacteriocin released by S. mutans (Merritt et al., 2016). The comC gene encodes competence-stimulating peptide (CSP). ComC is processed and secreted by an ABC transporter nlmTE (SMU_286/SMU_287, previously designated comAB) to generate a 21-residue peptide (CSP-21; Hale et al., 2005). The SepM is a 346 amino acid cell wall anchor protein encoded by the SMU_518 consisting of transmembrane, PDZ, and C-terminal regions (amino acids 10–26, 131–195, and 233–314, respectively; Hossain and Biswas, 2012a; Biswas et al., 2016; Bikash et al., 2018). The CSP-21 can be cleaved to the 18-amino acid CSP-18 by SepM such that it can then interact with the ComDE two-component regulatory system, resulting in the histidine kinase membrane-bound ComD receptor autophosphorylates, and the phosphate is transferred to the cognate cytoplasmic response regulator ComE; once phosphorylated ComE binds to the promoter region of the genes that encode mutacin IV (nlmA and nlmB), thereby upregulating this bacteriocin (Hossain and Biswas, 2012a).
In addition to the genes associated with the production of mutacin IV, it is unknown if other genes play a crucial role in the virulence of S. mutans against S. gordonii. The significant promoting role of S. mutans in the onset of dental caries, the role of S. gordonii in inhibiting the growth of S. mutans and buffering the local pH value of tooth surface demonstrated that the antagonism of S. mutans against the growth of S. gordonii is of significance for the prevention and treatment of dental caries. In this study, we evaluated the effect of 80 S. mutans clinical isolates on S. gordonii antagonism and divided them into antagonisticand non-antagonistic groups, and analyzed whether there were differences between the two groups in the genome through phylogenetic tree and principal component analyses. On this basis, we speculated that differentially distributed genes and differentially expressed genes between the two groups may play an important role in the growth of S. mutans against S. gordonii. We then carried out the following analysis: (1) we found the core genes and differentially distributed genes (DDGs) between the two groups and analyzed the function of these genes; (2) mutacin is a secondary metabolite produced by S. mutans and plays an important role in bacterial interactions. We searched for genes involved in secondary metabolites in S. mutans through antiSMASH bioinformatics analysis; (3) we searched for differentially expressed genes by RNA sequencing of isolates between the antagonistic group and the non-antagonistic group; (4) we analyzed the common genes shared in 1–3 and these genes were the key candidates participated in the regulation of the growth of S. mutans against S. gordonii; (5) we used qRT-PCR to validate the role of these key genes in S. mutans antagonizing S. gordonii using S. mutans clinical isolates.
Materials and methods
Origin of Streptococcus mutans clinical isolates
80 S. mutans isolates used in this study were preserved in our laboratory. In our previous study, we reported the source, including dental caries status and the number of kindergartens in these samples (Figures 1, 2; Liu et al., 2020). Briefly, 62 (SMB1-SMB62) and 18 (SMB63-SMB80) isolates were collected from children with caries and caries-free, respectively. These children were from three kindergartens in Bengbu city, Anhui Province, China. The First Affiliated Hospital of Bengbu Medical College provided ethical approval ([2017] KY011) for the present study.
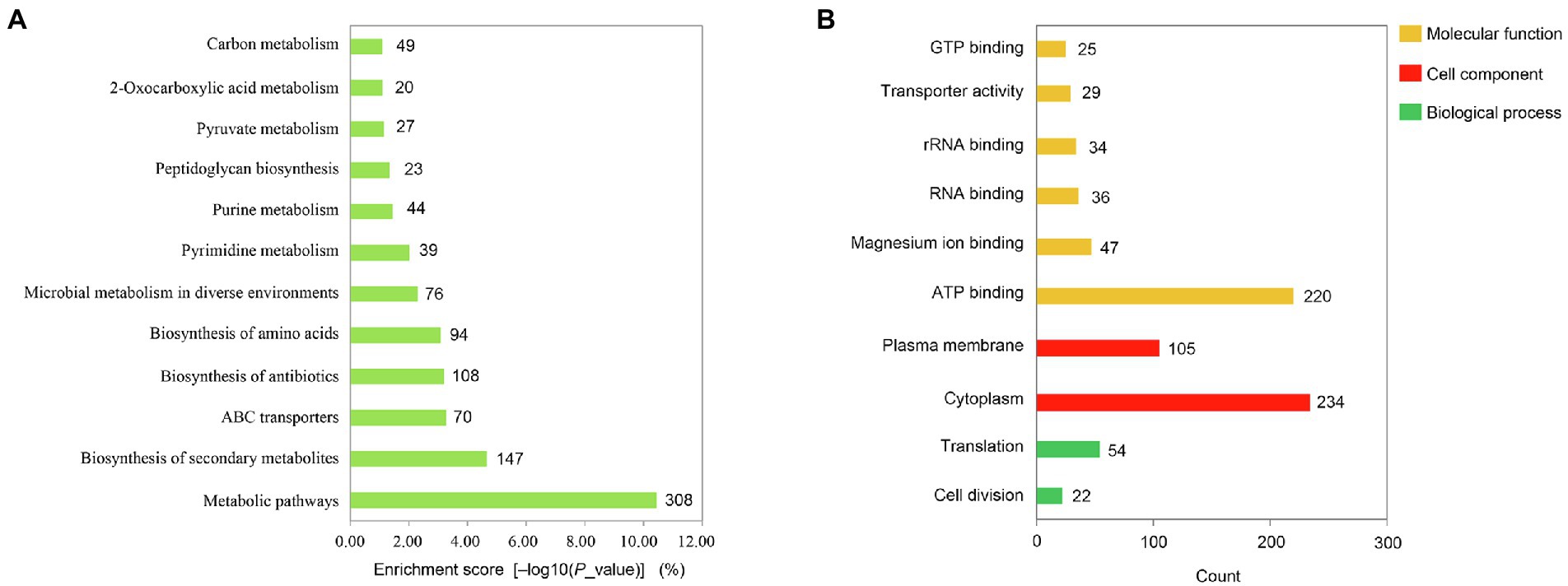
Figure 1. Core gene functional analysis. (A) KEGG pathways enriched for core genes. The pathways were sorted based on the enrichment score. (B) GO terms enriched for core genes. Each part’s GO terms (molecular function, cell component, or biological process) were ordered by the gene number proportion.
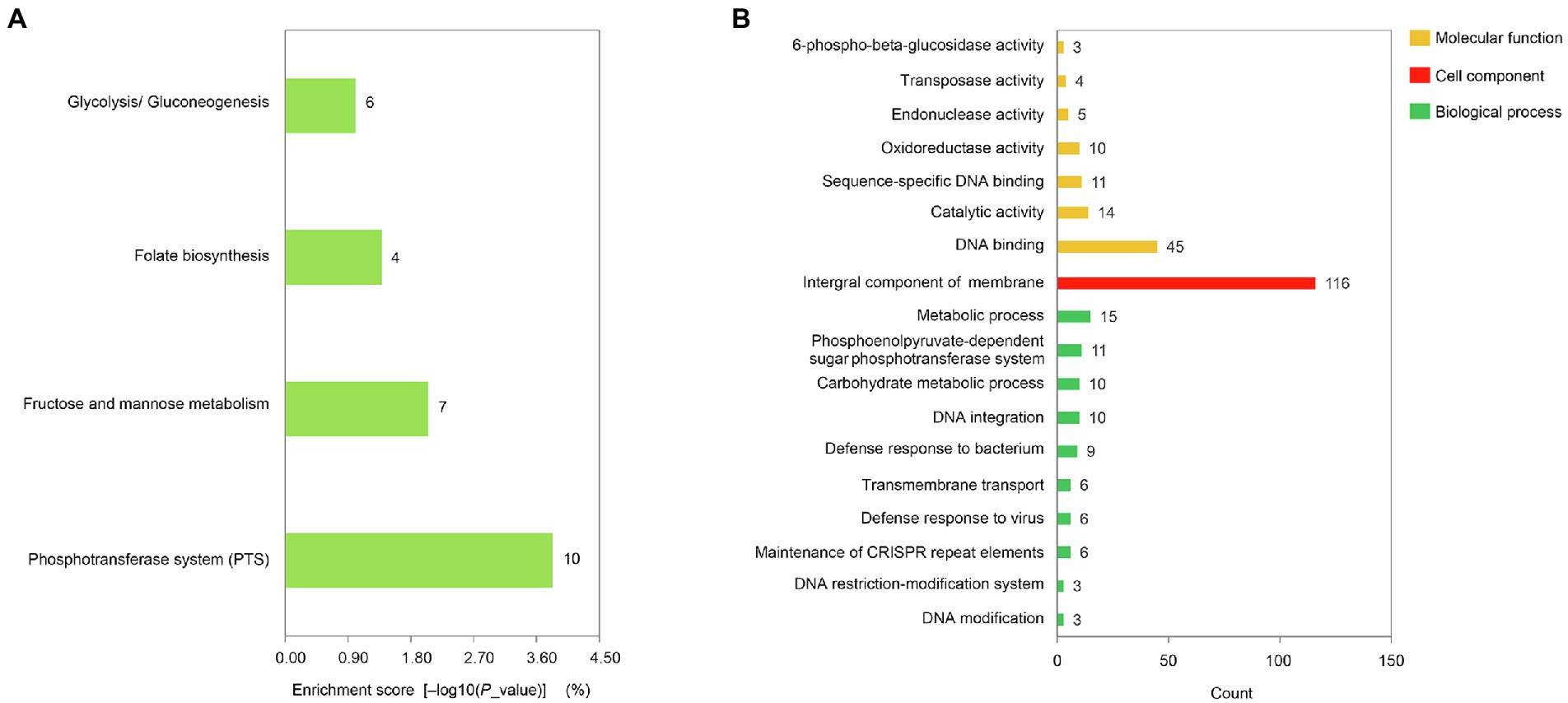
Figure 2. Functional analysis of non-core genes. (A) KEGG pathways enriched for non-core genes. (B) GO terms enriched for non-core genes. The categories were sorted in the same order as shown in Figure 1.
Average nucleotide identity analysis
Average nucleotide identity (ANI) values for 80 S. mutans clinical isolates, S. mutans UA159, and 40 other species downloaded from National Center for Biotechnology Information (NCBI; 38 Streptococci sp., Fusobacterium periodonticum, and Neisseria sicca; Table 1) were calculated as reported previously by Goris et al. (2007). Briefly, genomic sequences from one ‘query’ genome in the genomic pair being compared were cut into 1,020 nucleotide (nt) fragments that were then searched against the whole genome sequence of the other ‘reference’ genome within the pair using BLAST 2.2.26. The mean identity of all BLASTN matches exhibiting >30% overall sequence identity over an alignable region of at least 70% of the sequence length was calculated as the ANI between the query and reference genomes.
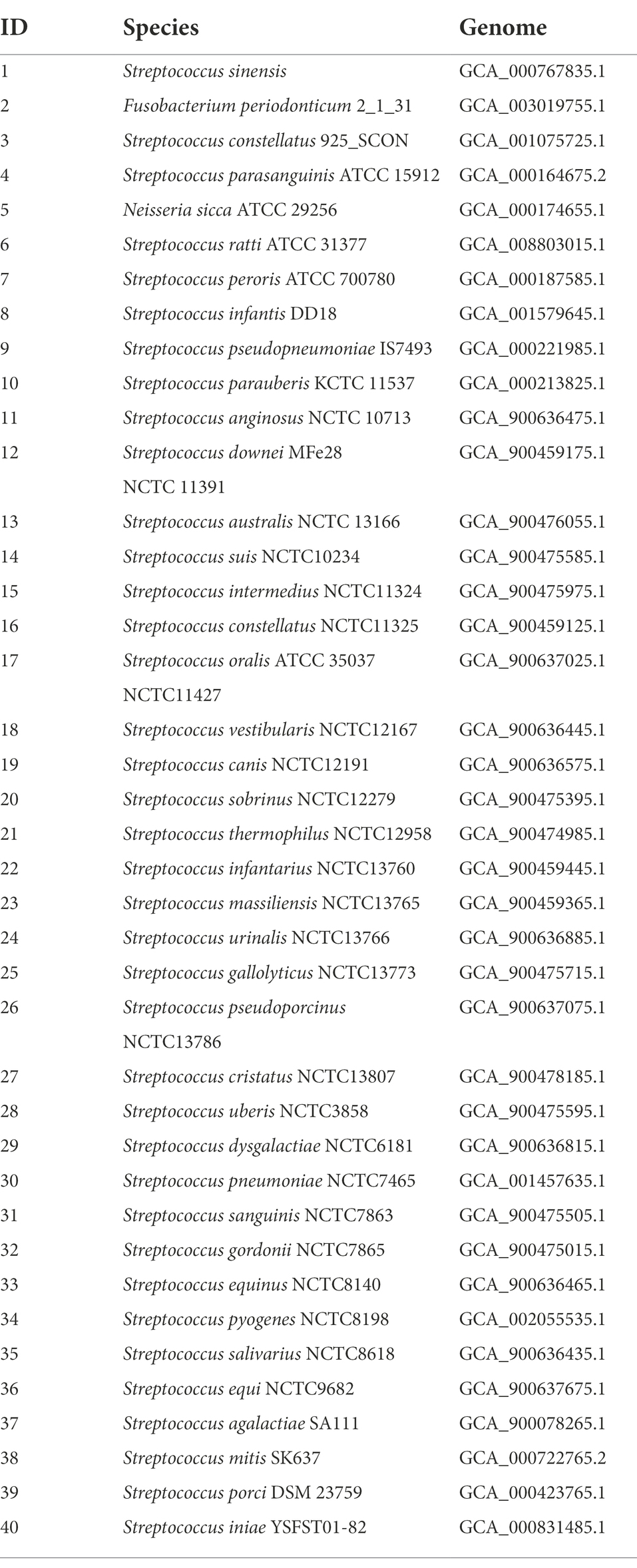
Table 1. The genome data for the 40 additional species included in average nucleotide identity analyses.
The activity of Streptococcus mutans against Streptococcus gordonii
We used a bacteriocin assay to evaluate the activity of S. mutans against S. gordonii (Huang et al., 2018; Liu et al., 2019). The positive reference strain was S. mutans UA159.Each isolate was incubated overnight in brain heart infusion (BHI) broth at 37°C under 5% CO2. Ten microliters of each S. mutans clinical isolate with an OD600 of 0.3 were added to BHI agar. We inoculated equal amounts of S. gordonii (ATCC 10558) adjacent to the S. mutans sample after 12 h of incubation. The agar plate medium was then left to culture for another 12 h. Finally, S gordonii clearing zones represent S. mutans’s activity against S. gordonii. Three biological replicates are used in the investigation. According to the activity of S. mutans against S. gordonii, we divided the 80 S. mutans into the antagonistic group and the non-antagonistic group.
Genetic diversity estimation
Genomic distances among the genomes of these isolates were determined using MASH1 (Ondov et al., 2016, 2019). Analysis (PCoA) was conducted using the R “vegan::pcoa” package. Wilcoxon rank-sum tests were used to analyze genome similarity. The Hasegawa-Kishino-Yano model was used for phylogenetic tree construction, with 1,000 bootstrap replicates based on the core genome-based multilocus sequence typing (cgMLST) data for S. mutans (Liu et al., 2020). The Phylogenetic trees were annotated using the Interactive Tree Of Life (iTOL 2; Letunic and Bork, 2021).
Core gene and differentially distributed gene analyses
Whole genome sequencing and assembly were performed as detailed in our previous study (Liu et al., 2020). The protein sequences of multiple samples were clustered using Cd-hit 4.6.1. Genes were identified with default minimum amino acid sequence identity and coverage thresholds (50 and 70%, respectively) compared to the S. mutans UA159 reference genome (Kovac et al., 2016). Genes detected in all 80 of these samples were identified as core genes, with all other genes being denoted as non-core genes. Differentially distributed genes (DDGs) are defined as non-core genes with different distribution frequencies (p < 0.05) across antagonistic and non-antagonistic groups.
Pathway annotation
KEGG pathways and GO terms associated with core and non-core genes were identified using the Database for Annotation, Visualization and Integrated Discovery (DAVID) gene annotation tool3 (Huang et al., 2007; Huang da et al., 2009).
Secondary metabolite biosynthetic gene clusters analysis
AntiSMASH is a widely used tool for detecting and characterizing biosynthetic gene clusters in microorganisms (Blin et al., 2021). We used the antiSMASH bacterial version4 to identify secondary metabolite biosynthetic gene clusters in S. mutans.
RNA-sequencing
Bacteria were cultured overnight in BHI broth, after which total bacterial RNA was extracted with the RNeasy Mini Kit (Qiagen, German) following the treatment of these bacterial suspensions with lysozyme for 40 min at 37°C. Random hexamer primers were used for reverse transcription-mediated first-strain cDNA synthesis, after which second-strand cDNA synthesis was performed. An A-Tailing Mix and RNA Index Adapters were then incubated with samples to facilitate end repair and sample indexing. The cDNA fragments were then amplified via PCR and purified using Ampure XP Beads. The resultant double-stranded PCR products were then denatured via heating and circularized with the splint oligo sequence to produce the final single-stranded circular DNA (ssCirDNA) library, which was amplified using phi29 to yield a DNA nanoball (DNB) with >200 copies per molecule. DNBs were then loaded into a patterned nanoarray, and paired-end 100 base reads were generated using the BGIseq500 platform (BGI Genomics, Shenzhen, China). Sample preparation was performed in triplicate.
Differential gene expression analysis
Sequencing data were filtered using SOAPnuke (v1.5.2), removing those reads that contained adapter sequences, exhibited a low-quality base ratio (base quality ≤5) of >20%, and showed an unknown base (‘N’ base) ratio > 5% (Li et al., 2008). The remaining clean reads were stored in the FASTQ format. Clean reads were aligned to the S. mutans UA159 reference genome using Bowtie2 2.2.5 (Langmead and Salzberg, 2012), then RSEM 1.2.12 was used to calculate gene expression levels (Li and Dewey, 2011). Differential expression analyses were conducted with DESeq2 1.4.5, using the following criteria for differential expression: adjusted value of p (padj) < 0.05 and fold change ≥2 or ≤ 0.5 (Love et al., 2014).
Comprehensive analysis of genes overlapping in core genes, DDG, antiSMASH, and RNA-seq results
Genes shared among different sets of results were compared using the interactive Venny 2.1 Venn diagram tool,5 with genes being compared within group A (core genes, antiSMASH, and RNA-seq) and group B (DDG, antiSMASH, and RNA-seq), separately.
qRT-PCR Validation
A PrimeScriptTM RT Reagent Kit (Perfect Real Time; Takara) was used to prepare cDNA from RNA samples. All qRT-PCR primers were designed using Primer3web (v 4.1.0), and were based upon known sequences for all genes other than SMU_1915, SMU_1317c, and SMU_1908c (Table 2). All RT-PCR reactions were conducted in a 20 μl volume containing 10 μl of 2 × TB Green Premix Ex TaqII (Takara), 0.8 μl each of the forward and reverse primers (0.4 μm), 1 μl of cDNA (300 μg/ml), 0.4 μl of ROX Reference Dye, and 7 μl of RNase-free H2O. Thermocycler settings were as follows: 95°C for 30 s; 40 cycles of 95°C for 5 s; and 58°C for 34 s. A melt curve was generated at the end of each reaction with continuous fluorescence monitoring. Relative gene expression was assessed via the 2−△△Ct method (Livak and Schmittgen, 2001). All qRT-PCR analyses were conducted in triplicate.
Statistical analysis
Data were analyzed using SPSS 20.0. The relationship between the activity of the isolates to antagonize S. gordonii and caries status was tested by Pearson chi-squared. Differentially distributed genes were identified via Pearson chi-squared tests with a theoretical frequency (TF) ≥ 5 (≤ 20% cell), while Fisher’s exact test was used in other cases. The Shapiro–Wilk test was used to establish whether qRT-PCR data were parametric, with t-tests and Mann–Whitney U-tests, respectively, being used to evaluate differentially expressed genes in parametric and nonparametric datasets. Correlations were analyzed using Spearman’s rank correlation coefficients. p < 0.05 was the threshold of significance.
Results
Estimation of average nucleotide identity and genetic diversity
For the 80 clinical S. mutans isolates included in the present study, the minimum ANI value for these isolates in a BLAST comparison with S. mutans UA159 was 98.76%. In contrast, the maximum ANI value for 40 other species, including 38 Streptococci, F. periodonticum, and N. sicca was 78.78% compared to S. mutans UA159. Of these 80 clinical isolates, 25 were capable of inhibiting S. gordonii growth (antagonistic group) while the remaining 55 were not (non-antagonistic group; Figure 3).
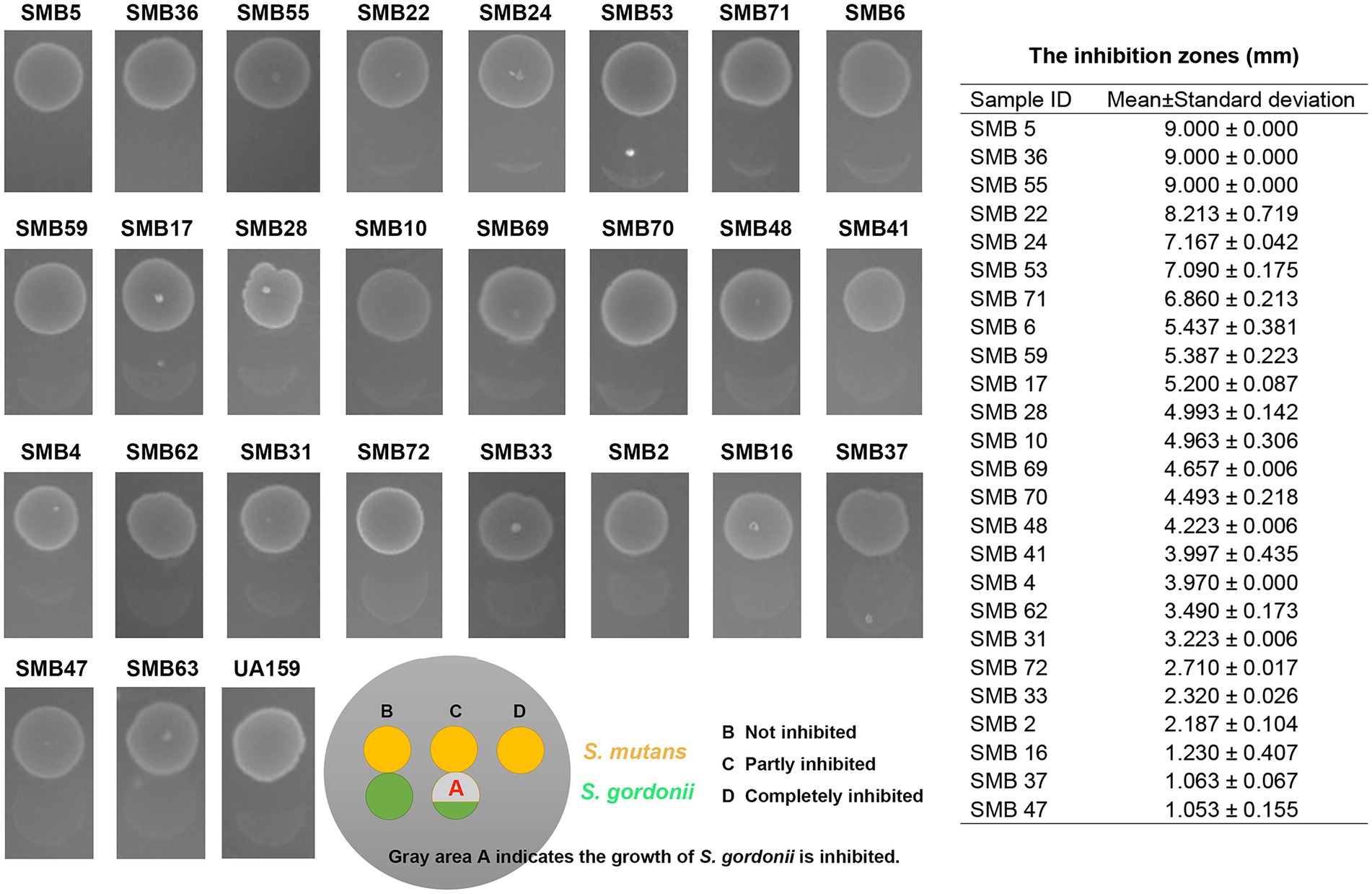
Figure 3. Bacteriocin assay. We used bacteriocin assay to detect the activity of Streptococcus mutans against Streptococcus gordonii in all samples. Here, we showed the results of isolates in antagonistic group, and displayed the result of SMB63 in the non-antagonistic group. SMB55 and SMB63 were the samples used in the RNA sequencing. The strain UA159 has been chosen as a positive control. The size of inhibition zones of the 25 S. mutans clinical isolates in the antagonistic group were also measured.
In the antagonistic group, 21 samples were from the caries population, and 4 samples were from the caries-free population. In the non-antagonistic group, 41 samples were from the caries population and 14 samples were from the caries-free population. The sample metadata including antagonist and caries classification are listed in Supplementary Table 1. The ability of S. mutans to inhibit S. gordonii growth in the 80 isolates has no significant relation to dental caries (p = 0.348). Therefore, the presence of dental caries does not affect the outcome. Phylogenetic tree analysis showed that although not all the isolates in the antagonistic group cluster together, the isolates between the two groups still showed different cluster distribution. Not all the isolates in the antagonistic or the antagonistic group clustered together, this may be due to differences in other virulence phenotypes of these isolates (Figure 4). In order to determine whether there are differences between the two groups, we further used a Principal Coordinate Analysis (PCoA). The Wilcoxon rank-sum test of variance confirmed a significant association between genome similarity and antagonism to S. gordonii (p < 0.001; Figure 5). Dissimilarity values derived from the PCoA revealed that the genomes of these isolates clustered following their effectiveness against S. gordonii.
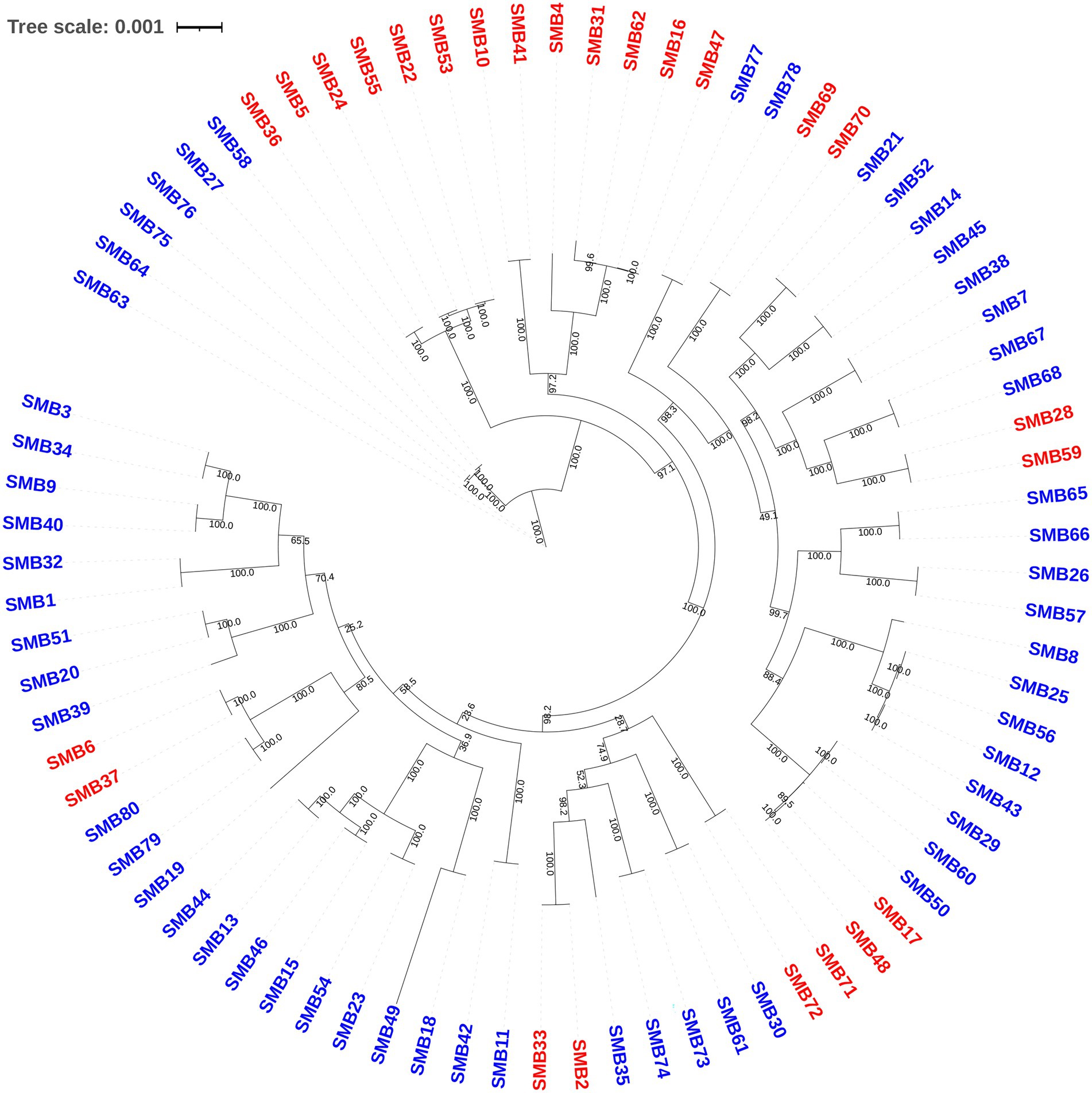
Figure 4. Phylogenetic tree analysis of S. mutans isolates from the mutacin IV and mutacin IV-free groups. A phylogenetic tree was constructed according to the cgMLST scheme and assembled using the HKY model. Numbers on lines denote bootstrap values determined for 1,000 replicates. Isolates in the mutacin IV group are marked with red color.
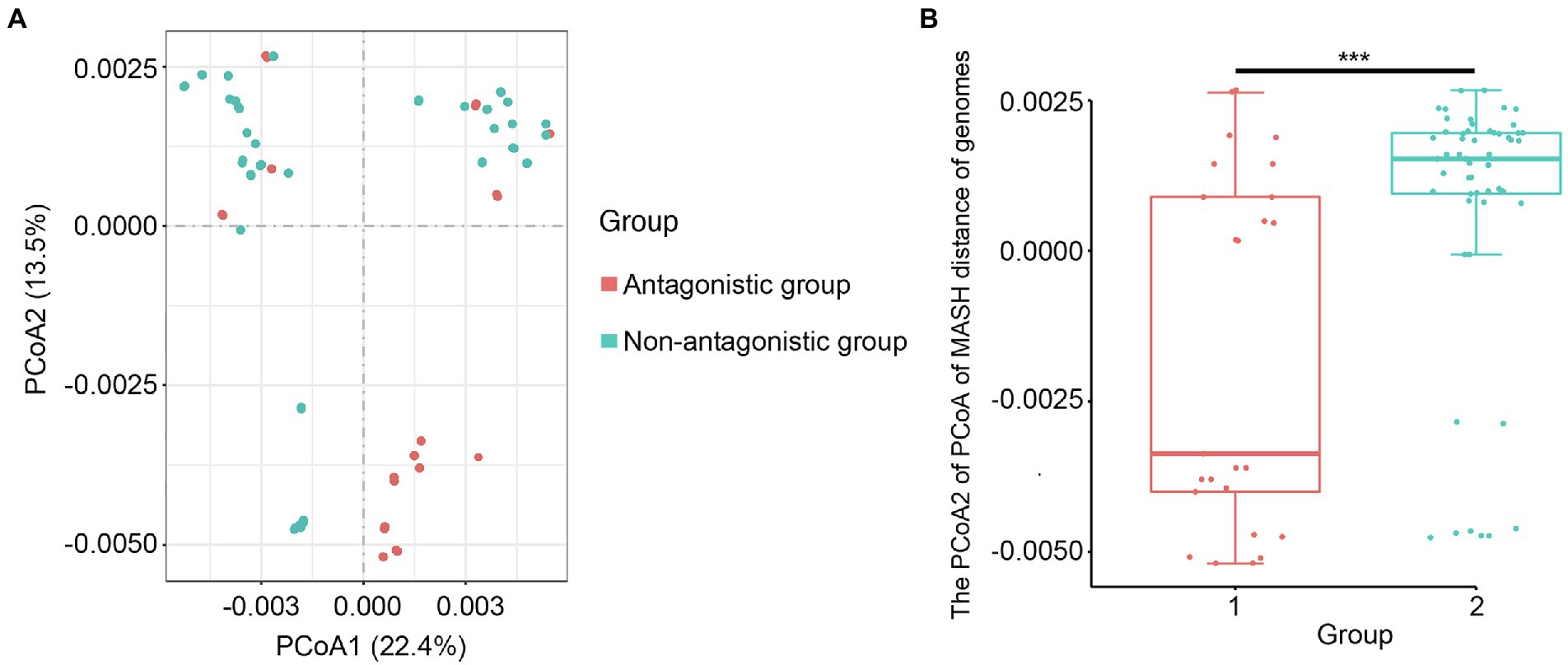
Figure 5. Principal Coordinate Analysis of S. mutans strains in the antagonistic and non-antagonistic groups. (A) Principal Coordinate Analysis (PCoA) of the genomes of 80 S. mutans clinical isolates from the antagonistic group and non-antagonistic group. (B) Principal component axis 2 of PCoA in (A) versus the group. *** represents a value of p < 0.001.
Core genes and associated pathways
In total, 1893 genes were mapped to the S. mutans UA159 genome in these 80 isolates, 1,432 of which were shared by all isolated strains. The reference strain was S. mutans UA159 (ATCC 700610). Gene ontology (GO) term and Kyoto Encyclopedia of Genes and Genomes (KEGG) pathway enrichment analyses were conducted using the Database for Annotation, Visualization and Integrated Discovery (DAVID) platform as a preliminary approach to exploring the functional roles of these core genes. KEGG analysis showed that 1,005 of these genes were enriched in 12 KEGG pathways, including the metabolic, biosynthesis of secondary metabolites, ABC transporters, biosynthesis of antibiotics, biosynthesis of amino acids, microbial metabolism in diverse environments, pyrimidine metabolism, purine metabolism, peptidoglycan biosynthesis, pyruvate metabolism, 2-Oxocarboxylic acid metabolism, and carbon metabolism pathways in S. mutans (Figure 1A). Moreover, 806 of these genes were associated with 10 GO terms including cell division, translation, plasma membrane, cytoplasm, ATP binding, magnesium ion binding, RNA binding, rRNA binding, transporter activity, and GTP binding functions (Figure 1B).
Non-core genes and associated pathways
Only 21 of the remaining 461 non-core genes were found to be related to 4 pathways, including the phosphotransferase system (PTS), fructose and mannose metabolism, folate biosynthesis, and glycolysis (or gluconeogenesis) pathways according to KEGG analysis (Figure 2A). Moreover, 73, 116, and 86 genes were associated with 10, biological processes, 1 cellular component, and 7 molecular function GO terms, respectively (Figure 2B).
Differentially distributed genes
There were 94 differentially distributed genes (DDGs) between the antagonistic and non-antagonistic groups, which include 33 and 61 genes that were positively and negatively associated with the activity of S. mutans against S. gordonii, respectively (p < 0.05). The data including the distribution and comparison of core genes and non-core genes in 80 samples are listed in Supplementary Table 2.
Secondary metabolite biosynthetic gene clusters
An antiSMASH analysis revealed 7 secondary metabolite biosynthetic gene clusters in S. mutans consisting of 169 genes, including core biosynthetic genes, additional biosynthetic genes, transport-related genes, regulatory genes, and other genes (Figure 6).
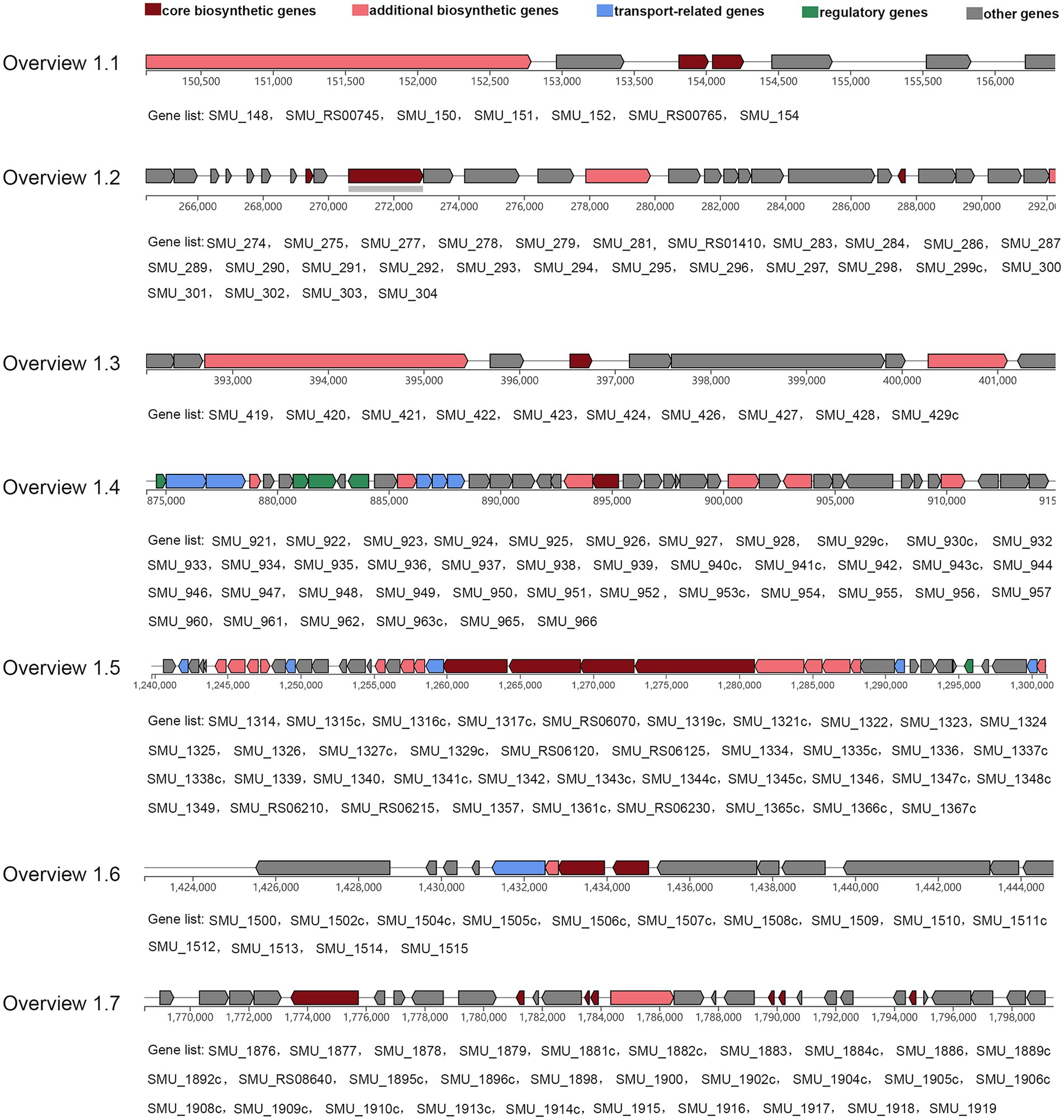
Figure 6. Seven clusters predicted by antiSMASH. The gene cluster contains five types of genes, including core biosynthetic genes, additional biosynthetic genes, transport-related genes, regulatory genes, and other genes. All genes involved in the corresponding cluster are attached.
Transcriptomic analyses of differentially expressed genes and associated pathway
As a prominent isolate for the antagonistic group, SMB55 wasselected randomly from three isolates (SMB5, SMB36, and SMB55) that could entirely inhibit the growth of S. gordonii. SMB63 was chosen randomly from 55 non-antagonistic samples to serve as the non-antagonistic group’s representative strain. Comparing the transcriptomes of isolates that fully inhibit S. gordonii growth and isolates that do not inhibit S. gordonii growth will allow us to better screen the target genes involved in regulating S. mutans anti-S. gordonii activity. Consequently, RNA sequencing (RNA-seq) was conducted to explore differential gene expression between the antagonistic and non-antagonistic groups using the underlined isolates with three repetitions. In total, 54 genes were differentially expressed between these groups (padj < 0.05 and Fold-change ≥2.00 or ≤ 0.5 with a minimum of 100 average reads in either group), of which 11 (Table 3) and 43 (Table 4) were, respectively, upregulated and downregulated in the antagonistic group relative to the non-antagonistic group. The expression levels of the detected genes (with a minimum of 100 average reads in either group) are listed in Supplementary Table 3. According to KEGG pathway analysis, these differentially expressed genes are primarily involved in the biosynthesis of secondary metabolites (Supplementary Table 4).
Comprehensive analysis of the common genes across the core gene/DDG, antiSMASH, RNA-seq, and KEGG pathway analyses
Of the 169 genes obtained through antiSMASH analyses, 95 belonged to the core gene set identified above. Of the remaining 74 genes, 5 (SMU_1315c, SMU_1316c, SMU_1317c, SMU_1348c, and SMU_1884c) were negatively correlated with the activity of S. mutans against S. gordonii, while 6 (SMU_151, SMU_925, SMU_1895c, SMU_1902c, SMU_1908c, and SMU_1909c) were positively correlated with this effect. RNA-seq analyses revealed 4 downregulated genes were mapped to the DDGs (SMU_137-SMU_140). In total, 7 genes were shared across the core gene, antiSMASH, and RNA-seq analyses. When RNA-seq and DDG results were correlated, we found that SMU_137-SMU_140 were downregulated in the antagonistic group in RNA-seq results and negatively associated with the activity of S. mutans against S. gordonii. Figure 7 illustrates the complex relationship described above.
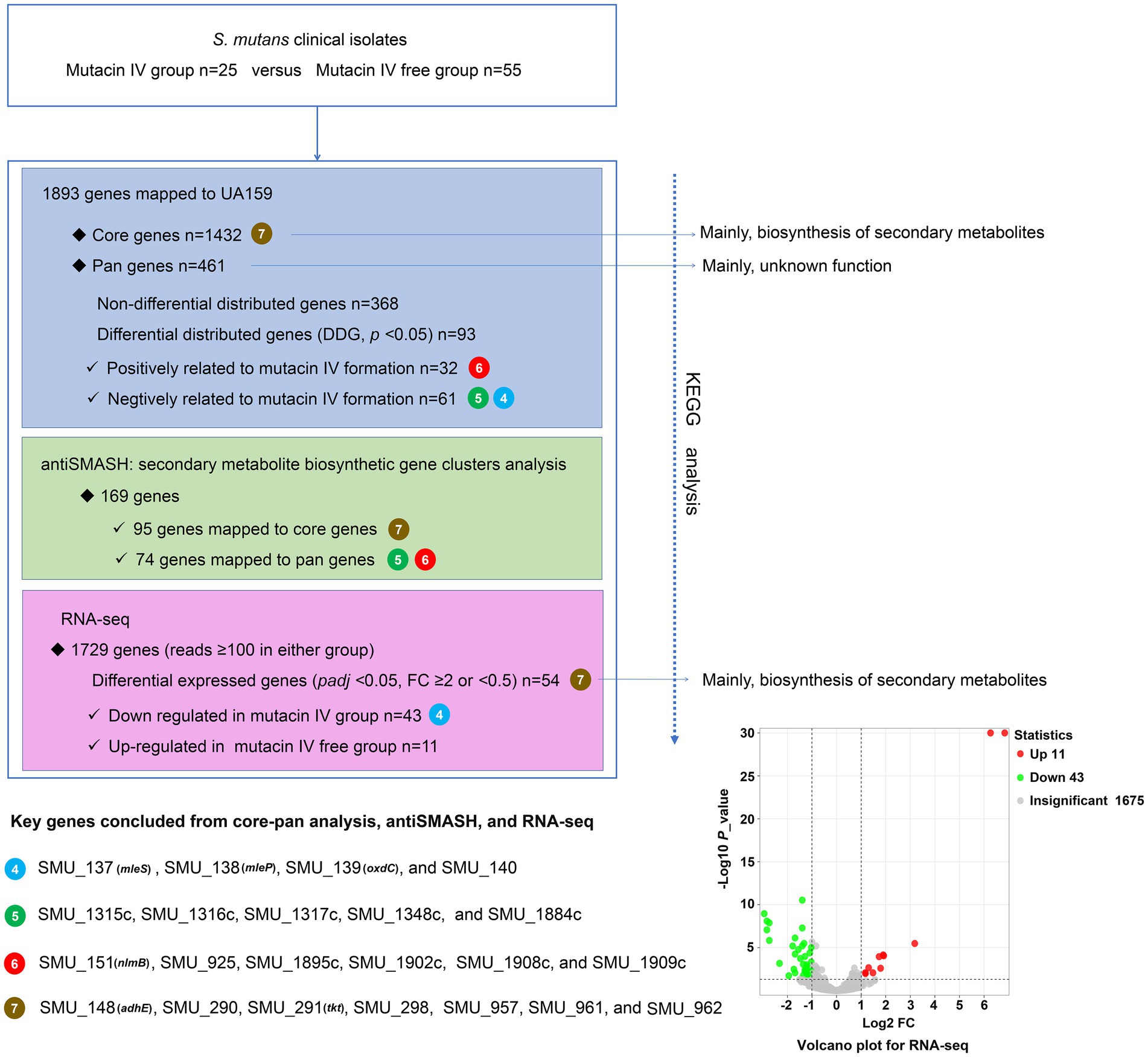
Figure 7. Overview of genomic comparison, antiSMASH, RNA-seq, and KEGG pathway analyses. The circle represents the shared genes discovered through various investigations, and the number within the circle shows the number of shared genes.
Expression levels validation of mRNAs based on qRT-PCR
We chose qRT-PCR to verify the results of RNA-seq, including more clinical samples, because the obtained results from these two samples (SMB55 and SMB63) could not adequately represent the transcriptome comparison results of the two groups (the antagonistic and the non-antagonistic groups). Overall, sixteen genes (SMU_137, SMU_138, SMU_139, SMU_140, SMU_141, SMU_150, SMU_151, SMU_518, SMU_1915, SMU_1916, SMU_1917, SMU_1315c, SMU_1316c, SMU_1317c, SMU_1908c, SMU_1909c) were selected for qRT-PCR-based validation performed using 35 clinical isolates, including 25 from the antagonistic group and 10 that were randomly selected from the non-antagonistic group. SMU_137, SMU_138, SMU_139, SMU_140, SMU_1315c, SMU_1316c, and SMU_1317c were downregulated in the antagonistic group, whereas SMU_1908c and SMU_1909c were upregulated in this group (Figure 8). The average SMU_150 and SMU_151 expression levels were higher in the antagonistic group than in the non-antagonistic group, but the difference was insignificant. Adjacent genes exhibited closely related expression levels (Figure 9). For example, SMU_137, SMU_138, SMU_139, and SMU_140 exhibited strongly positively correlated expression patterns, with correlation coefficient values greater than 0.9. Similarly, SMU_1908c and SMU_1909c were strongly positively correlated (r = 0.974), as were SMU_1315c and SMU_1316c (r = 0.991).
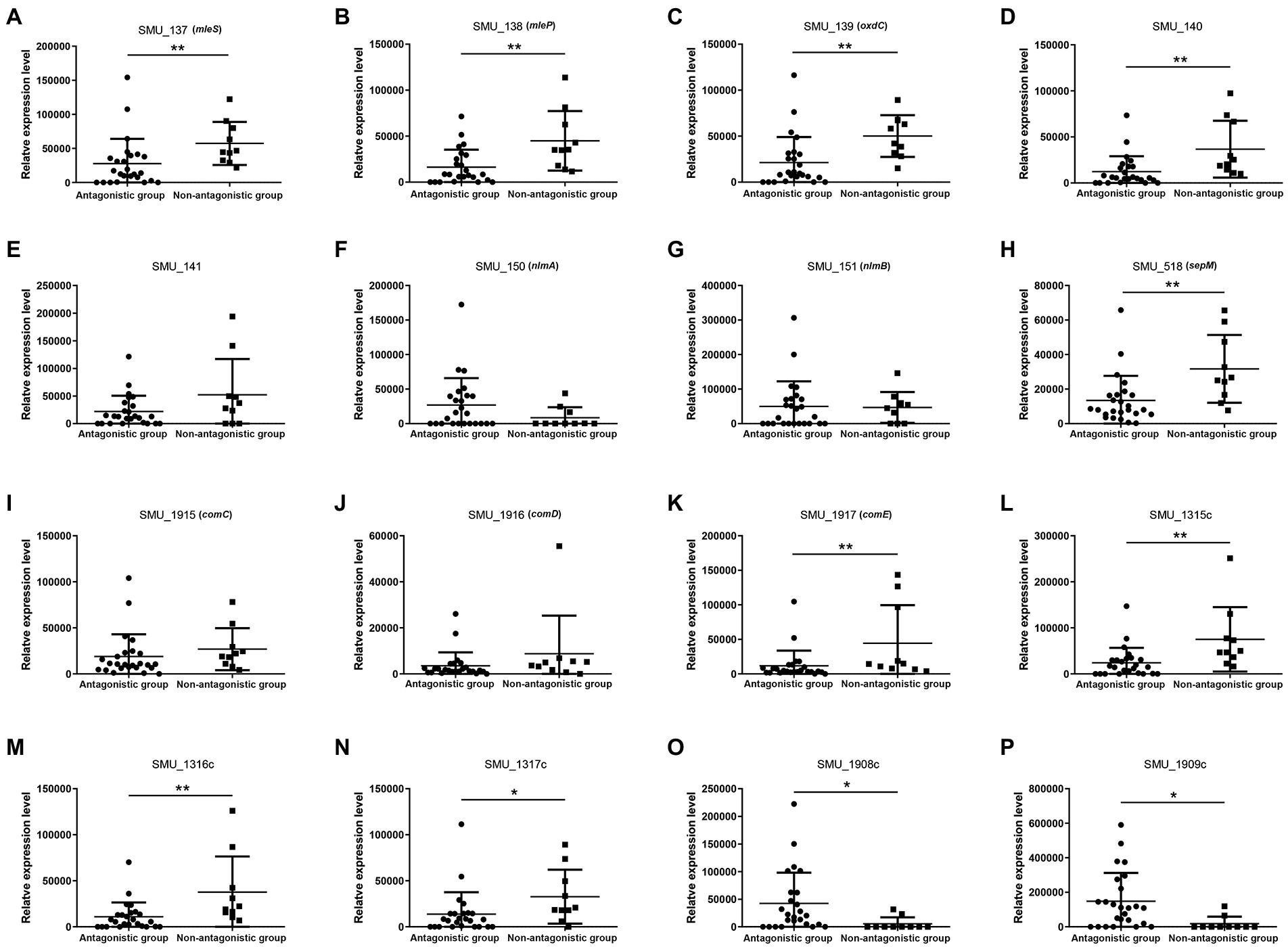
Figure 8. Gene expression levels for 16 selected genes (SMU_137–141, SMU_150, SMU_151, SMU_518, SMU_1915–1917, SMU_1315c-SMU_1317c, SMU_1908c, and SMU_1909c) as measured by qRT-PCR in the antagonistic group and non-antagonistic group. * represents value of p < 0.05, ** represents value of p < 0.01. The expression level of SMU_137 in SMB16 was defined as 1. The experiment on 35 strains was conducted in three technical replicates. The CT values of the qRT-PCR is listed in Supplementary Table 5.
Discussion
The current study investigated potential key genes involved in regulating the activity of S. mutans that inhibit the growth of S. gordonii using 80 S. mutans clinical isolates isolated from supragingival dental plaques of children with early childhood caries and caries-free, using a combination of comparative genome analysis, RNA-seq, antiSMASH, and qRT-PCR between antagonistic and non-antagonistic groups.
PCoA analysis of the antagonistic and non-antagonistic groups revealed significant variations in their genetic features. By comparing the genomes of samples from the two groups, we may identify critical target genes for S. mutans to inhibit S. gordonii growth. There were 1,432 core genes and 94 DDGs discovered in this study. The number of core genes identified here is approximately 30% higher than the number of core genes (1083) identified by Meng et al. (2017). The following two factors could explain this: (1) 80 S. mutans clinical isolates used in this study were collected from children living in Bengbu, China, while the 183 S. mutans isolates used in that study included 11 genomes assembled by authors and 172 previously published genomes. These isolates were obtained from Brazil, Iceland, South Africa, Turkey, the USA, and the rest. Our earlier investigation demonstrated that the significant allele heterogeneity of S. mutans among different countries is apparent (Liu et al., 2020). Because these strains originate from diverse regions, the isolation difference may be substantial, and their core gene count would be much lower than in this study and (2) The serotype c was identified in 80 S. mutans clinical isolates used in this study, but the serotypes of the isolates in that study were diverse. For example, S. mutans LJ23 and S. mutans UA159 are serotype k and serotype c, respectively, which may also affect the acquisition of core genes. According to KEGG pathway analysis, core genes are primarily involved in thesecondary metabolite biosynthesis, and the function of most DDGs isunidentified and needs to be explored. We believe DDGs are essential in the competition between S. mutans and S. gordonii.
Bacteriocins produced by lactic acid bacteria (LAB) are primarily active against other gram-positive bacteria that are closely related (Zheng and Sonomoto, 2018). Streptococcus mutans, a lactic acid bacterium, can produce mutacin IV against various streptococcal species growth including S. gordonii (Kreth et al., 2008). The nlmT (SMU_286), nlmE (SMU_287), sepM (SMU_518), comC (SMU_1915), comD (SMU_1916), and comE (SMU_1917) genes have previously been reported to be positive regulators of mutacin IV production, with nlmA (SMU_150) and nlmB (SMU_151) encoding mutacin IV (Hale et al., 2005; van der Ploeg, 2005; Hossain and Biswas, 2012a). SMU_152, topologically linked to nlmAB operon, acts as an immunity protein giving protection against mutacin IV in S. gordonii (Hossain and Biswas, 2012b). The present study identified nlmT, nlmE, sepM, comC, and comE as core genes. All but one isolate from the non-antagonistic group expressed comD in this study. The overall distribution frequency of nlmA and SMU_152 across both groups was 56%. The distribution frequency of nlmB in the antagonistic group was significantly higher than that in the non-antagonistic group (56% vs. 20%). Additionally, except for sepM, there was no statistically significant variation in the expression levels of nlmT, nlmE, nlmA, nlmB, comC, comD, and comE between the two groups according to RNA-seq analysis. These results suggest that, in addition to the mutacin IV -related genes, other key genes are involved in S. mutans anti-S. gordonii antagonism. These findings highlight the significance of our current research, which is focused on identifying candidate targets against S. gordonii in S. mutans clinical isolates.
The antiSMASH is an effective tool used extensively to screen bacterial biosynthetic gene clusters (Chakraborty, 2022). A previous study showed that nlmTE encoding of the ABC transport system is required to export nonlantibiotic mutacins in S. mutans (Hale et al., 2005). The antiSMASH also predicted 11 genes (SMU_922, SMU_923, SMU_934, SMU_935, SMU_936, SMU_1315c, SMU_1325, SMU_1338c, SMU_1348c, SMU_1366c, SMU_1506c) belonged to transport-related genes. To fully explore genes that may play a role in regulating the activity of S. mutans against S. gordonii, we combined results from core gene, DDG, RNA-seq, and antiSMASH analyses to select 16 genes for qRT-PCR-based validation in 35 S. mutans isolates. These validation results revealed that SMU_137 (malate dehydrogenase, mleS), SMU_138 (malate permease, mleP), SMU_139 (oxalate decarboxylase, oxdC), and SMU_140 were downregulated in isolates from the antagonistic group, consistent with RNA-seq results. Per the DDG analysis, SMU_1315c, SMU_1316c, and SMU_1317c were negatively associated with the activity of S. mutans against S. gordonii, whereas SMU_151, SMU_1908c, and SMU_1909c were positively related to this function. Consistently, our qRT-PCR results indicated that SMU_1315c, SMU_1316c, and SMU_1317c were downregulated in the antagonistic group, while SMU_1908c and SMU_1909c were upregulated in this group. Malolactic fermentation (MLF) has been identified as a primary mechanism whereby S. mutans and other oral streptococci induce alkalinity (Sheng and Marquis, 2007), and this pathway plays a central role in protecting S. mutans against acidic damage, oxidative stress, and starvation (Sheng and Marquis, 2007). MLF is important in protecting S. mutans against acid damage and oxidative and starvation damage (Sheng et al., 2010). SMU_137, SMU_138, and SMU_139 are the main genes associated with MLF in S. mutans (Lemme et al., 2010). However, the relationship between these genes and the activity of S. mutans against S. gordonii remains unknown. Our data suggest that SMU_137–140, SMU_1315c-1317c, and SMU_1908c-1909c may play key roles in regulating this function, and SMU_1315c may be the transport-related gene. As we further found that adjacent genes exhibit closely related patterns of expression, these data maysuggest synergistic roles for these gene clusters. The cooperative regulation relationship between genes in gene clusters has also been reported before. SMU_150 and SMU_151, for instance, are mutacin IV co-coding genes that shared a single operon (Qi et al., 2001). S. mutans produces mutacin IV, a bacteriocin that inhibits S. gordonii growth. SMU_150 and SMU_151 work together to control mutacin IV production. SMU_1915-SMU_1917, particularly SMU_1916 and SMU_1917, are positively correlated with mutacin IV production (Hossain and Biswas, 2012a). Phosphorylation of SMU_1916 causes phosphorylation of SMU_1917, which then interacts directly with the promoters of SMU_ 150 and SMU_ 151, promoting mutacin IV synthesis. Figure 9 shows the expression connection of these neighboring. Nevertheless, the mechanism by which these target gene clusters govern S. mutansand inhibit S. gordonii has not been revealed. Future investigation is required, including gene knockdown and post-transcriptional regulation.
Conclusion
Streptococcus mutans is an important cariogenic bacterium that causes dental caries, whereas S. gordonii is a non-cariogenic pioneer bacterium that colonizes the tooth surface and inhibits the growth of S. mutans. Identifying S. mutans’s essential genes against S. gordonii has substantial therapeutic implications for preventing and treating of dental caries. The findings of our study demonstrate that genome comparison help determine potential target genes in regulating the interaction between S. mutans and S. gordonii; SMU_137–140, SMU_1315c-1317c, and SMU_1908c-1909c were identified as 3 potential candidate gene clusters controlling this activity, with SMU_1315c possibly being a transport-related gene; adjacent genes in the gene cluster may synergistically regulate the activity of S. mutans against S. gordonii growth. These findings point toward a new direction for studying S. mutans’ cariogenic mechanism through the prism of bacterial interaction.
Data availability statement
The datasets presented in this study can be found in online repositories. The names of the repository/repositories and accession number(s) can be found at: https://www.ncbi.nlm.nih.gov/, PRJNA804356.
Ethics statement
The studies involving human participants were reviewed and approved by The First Affiliated Hospital of Bengbu Medical College provided ethical approval ([2017] KY011) for the present study. Written informed consent to participate in this study was provided by the participants’ legal guardian/next of kin.
Author contributions
SL and KZ contributed to conception and design of the study. SL, YS, YL, and LX analyzed the database. QZ, QW, and GZ assisted in data analysis. SL and FH performed the statistical analysis. SL wrote the first draft of the manuscript. YS wrote sections of the manuscript. All authors contributed to the article and approved the submitted version.
Funding
This work was supported by the National Natural Science Foundation of China (Grant no. 32000386), the First Affiliated Hospital of Bengbu Medical College Science Fund for Outstanding Young Scholars (Grant no. 2019byyfyyq07), and the University Synergy Innovation Program of Anhui Province, China (Grant no. GXXT-2021-056).
Conflict of interest
The authors declare that the research was conducted in the absence of any commercial or financial relationships that could be construed as a potential conflict of interest.
Publisher’s note
All claims expressed in this article are solely those of the authors and do not necessarily represent those of their affiliated organizations, or those of the publisher, the editors and the reviewers. Any product that may be evaluated in this article, or claim that may be made by its manufacturer, is not guaranteed or endorsed by the publisher.
Supplementary material
The Supplementary Material for this article can be found online at: https://www.frontiersin.org/articles/10.3389/fmicb.2022.945108/full#supplementary-material
Footnotes
1. ^https://github.com/marbl/Mash
3. ^https://david.ncifcrf.gov/
References
Aldosari, M., Mendes, S. D. R., Aldosari, A., Aldosari, A., and de Abreu, M. (2021). Factors associated with oral pain and oral health-related productivity loss in the USA, National Health and nutrition examination surveys (NHANES), 2015-2018. PLoS One 16:e0258268. doi: 10.1371/journal.pone.0258268
Amarasena, N., Chrisopoulos, S., Jamieson, L. M., and Luzzi, L. (2021). Oral health of Australian adults: distribution and time trends of dental caries, periodontal disease and tooth loss. Int. J. Environ. Res. Public Health 18:1539. doi: 10.3390/ijerph182111539
Bikash, C. R., Hamry, S. R., and Tal-Gan, Y. (2018). Structure-activity relationships of the competence stimulating peptide in Streptococcus mutans reveal motifs critical for membrane protease SepM recognition and ComD receptor activation. ACS Infect Dis 4, 1385–1394. doi: 10.1021/acsinfecdis.8b00115
Biswas, S., Cao, L., Kim, A., and Biswas, I. (2016). SepM, a streptococcal protease involved in quorum sensing, Displays Strict Substrate Specificity. J Bacteriol 198, 436–447. doi: 10.1128/JB.00708-15
Blin, K., Shaw, S., Kloosterman, A. M., Charlop-Powers, Z., van Wezel, G. P., Medema, M. H., et al. (2021). antiSMASH 6.0: improving cluster detection and comparison capabilities. Nucleic Acids Res. 49, W29–W35. doi: 10.1093/nar/gkab335
Chakraborty, P. (2022). Gene cluster from plant to microbes: their role in genome architecture, organism's development, specialized metabolism and drug discovery. Biochimie 193, 1–15. doi: 10.1016/j.biochi.2021.12.001
Cheng, X., Redanz, S., Treerat, P., Qin, H., Choi, D., Zhou, X., et al. (2020). Magnesium-dependent promotion of H2O2 production increases ecological competitiveness of Oral commensal streptococci. J. Dent. Res. 99, 847–854. doi: 10.1177/0022034520912181
Goris, J., Konstantinidis, K. T., Klappenbach, J. A., Coenye, T., Vandamme, P., and Tiedje, J. M. (2007). DNA-DNA hybridization values and their relationship to whole-genome sequence similarities. Int. J. Syst. Evol. Microbiol. 57, 81–91. doi: 10.1099/ijs.0.64483-0
Hale, J. D., Heng, N. C., Jack, R. W., and Tagg, J. R. (2005). Identification of nlmTE, the locus encoding the ABC transport system required for export of nonlantibiotic mutacins in Streptococcus mutans. J. Bacteriol. 187, 5036–5039. doi: 10.1128/JB.187.14.5036-5039.2005
He, J., Yang, J., Li, M., Li, Y., Pang, Y., Deng, J., et al. (2022). Polyzwitterion manipulates Remineralization and Antibiofilm functions against dental demineralization. ACS Nano 16, 3119–3134. doi: 10.1021/acsnano.1c10812
Hossain, M. S., and Biswas, I. (2012a). An extracelluar protease, SepM, generates functional competence-stimulating peptide in Streptococcus mutans UA159. J. Bacteriol. 194, 5886–5896. doi: 10.1128/JB.01381-12
Hossain, M. S., and Biswas, I. (2012b). SMU.152 acts as an immunity protein for mutacin IV. J. Bacteriol. 194, 3486–3494. doi: 10.1128/JB.00194-12
Huang, X., Browngardt, C. M., Jiang, M., Ahn, S. J., Burne, R. A., and Nascimento, M. M. (2018). Diversity in antagonistic interactions between commensal Oral streptococci and Streptococcus mutans. Caries Res. 52, 88–101. doi: 10.1159/000479091
Huang da, W., Sherman, B. T., and Lempicki, R. A. (2009). Bioinformatics enrichment tools: paths toward the comprehensive functional analysis of large gene lists. Nucleic Acids Res. 37, 1–13. doi: 10.1093/nar/gkn923
Huang, D. W., Sherman, B. T., Tan, Q., Kir, J., Liu, D., Bryant, D., et al. (2007). DAVID bioinformatics resources: expanded annotation database and novel algorithms to better extract biology from large gene lists. Nucleic Acids Res. 35, W169–W175. doi: 10.1093/nar/gkm415
Kovac, J., Miller, R. A., Carroll, L. M., Kent, D. J., Jian, J., Beno, S. M., et al. (2016). Production of hemolysin BL by Bacillus cereus group isolates of dairy origin is associated with whole-genome phylogenetic clade. BMC Genomics 17:581. doi: 10.1186/s12864-016-2883-z
Kreth, J., Zhang, Y., and Herzberg, M. C. (2008). Streptococcal antagonism in oral biofilms: streptococcus sanguinis and Streptococcus gordonii interference with Streptococcus mutans. J. Bacteriol. 190, 4632–4640. doi: 10.1128/JB.00276-08
Langmead, B., and Salzberg, S. L. (2012). Fast gapped-read alignment with bowtie 2. Nat. Methods 9, 357–359. doi: 10.1038/nmeth.1923
Lemme, A., Sztajer, H., and Wagner-Dobler, I. (2010). Characterization of mleR, a positive regulator of malolactic fermentation and part of the acid tolerance response in Streptococcus mutans. BMC Microbiol. 10:58. doi: 10.1186/1471-2180-10-58
Letunic, I., and Bork, P. (2021). Interactive tree Of life (iTOL) v5: an online tool for phylogenetic tree display and annotation. Nucleic Acids Res. 49, W293–W296. doi: 10.1093/nar/gkab301
Li, B., and Dewey, C. N. (2011). RSEM: accurate transcript quantification from RNA-Seq data with or without a reference genome. BMC Bioinformatics 12:323. doi: 10.1186/1471-2105-12-323
Li, R., Li, Y., Kristiansen, K., and Wang, J. (2008). SOAP: short oligonucleotide alignment program. Bioinformatics 24, 713–714. doi: 10.1093/bioinformatics/btn025
Liu, Y., and Burne, R. A. (2009). Multiple two-component systems modulate alkali generation in Streptococcus gordonii in response to environmental stresses. J. Bacteriol. 191, 7353–7362. doi: 10.1128/JB.01053-09
Liu, S., Li, H., Guo, Z., Guan, J., Sun, Y., and Zhang, K. (2019). Insight into the effect of small RNA srn225147 on Mutacin IV in Streptococcus mutans. Indian J. Microbiol. 59, 445–450. doi: 10.1007/s12088-019-00820-2
Liu, S., Li, X., Guo, Z., Liu, H., Sun, Y., Liu, Y., et al. (2020). A Core genome multilocus sequence typing scheme for Streptococcus mutans. mSphere 5:20. doi: 10.1128/mSphere.00348-20
Liu, Y., Liu, S., Zhi, Q., Zhuang, P., Zhang, R., Zhang, Z., et al. (2022). Arginine-induced metabolomic perturbation in Streptococcus mutans. J. Oral Microbiol. 14:2015166. doi: 10.1080/20002297.2021.2015166
Livak, K. J., and Schmittgen, T. D. (2001). Analysis of relative gene expression data using real-time quantitative PCR and the 2(-Delta Delta C(T)) method. Methods 25, 402–408. doi: 10.1006/meth.2001.1262
Loesche, W. J. (1986). Role of Streptococcus mutans in human dental decay. Microbiol. Rev. 50, 353–380. doi: 10.1128/mr.50.4.353-380.1986
Love, M. I., Huber, W., and Anders, S. (2014). Moderated estimation of fold change and dispersion for RNA-seq data with DESeq2. Genome Biol. 15:550. doi: 10.1186/s13059-014-0550-8
Meng, P., Lu, C., Zhang, Q., Lin, J., and Chen, F. (2017). Exploring the genomic diversity and cariogenic differences of Streptococcus mutans strains Through Pan-genome and comparative genome analysis. Curr. Microbiol. 74, 1200–1209. doi: 10.1007/s00284-017-1305-z
Merritt, J., Senpuku, H., and Kreth, J. (2016). Let there be bioluminescence: development of a biophotonic imaging platform for in situ analyses of oral biofilms in animal models. Environ. Microbiol. 18, 174–190. doi: 10.1111/1462-2920.12953
OmerOglou, E., Karaca, B., Kibar, H., Haliscelik, O., and Kiran, F. (2022). The role of microbiota-derived postbiotic mediators on biofilm formation and quorum sensing-mediated virulence of Streptococcus mutans: A perspective on preventing dental caries. Microb. Pathog. 164:105390. doi: 10.1016/j.micpath.2022.105390
Ondov, B. D., Starrett, G. J., Sappington, A., Kostic, A., Koren, S., Buck, C. B., et al. (2019). Mash screen: high-throughput sequence containment estimation for genome discovery. Genome Biol. 20:232. doi: 10.1186/s13059-019-1841-x
Ondov, B. D., Treangen, T. J., Melsted, P., Mallonee, A. B., Bergman, N. H., Koren, S., et al. (2016). Mash: fast genome and metagenome distance estimation using MinHash. Genome Biol. 17:132. doi: 10.1186/s13059-016-0997-x
Pearce, C., Bowden, G. H., Evans, M., Fitzsimmons, S. P., Johnson, J., Sheridan, M. J., et al. (1995). Identification of pioneer viridans streptococci in the oral cavity of human neonates. J. Med. Microbiol. 42, 67–72. doi: 10.1099/00222615-42-1-67
Qi, F., Chen, P., and Caufield, P. W. (2001). The group I strain of Streptococcus mutans, UA140, produces both the lantibiotic mutacin I and a nonlantibiotic bacteriocin, mutacin IV. Appl. Environ. Microbiol. 67, 15–21. doi: 10.1128/AEM.67.1.15-21.2001
Santos, P. S., Barasuol, J. C., Moccelini, B. S., Magno, M. B., Bolan, M., Martins-Junior, P. A., et al. (2021). Prevalence of toothache and associated factors in children and adolescents: a systematic review and meta-analysis. Clin. Oral Investig. 26, 1105–1119. doi: 10.1007/s00784-021-04255-2
Saraithong, P., Pattanaporn, K., Chen, Z., Khongkhunthian, S., Laohapensang, P., Chhun, N., et al. (2015). Streptococcus mutans and Streptococcus sobrinus colonization and caries experience in 3-and 5-year-old Thai children. Clin. Oral Investig. 19, 1955–1964. doi: 10.1007/s00784-015-1437-0
Sheng, J., Baldeck, J. D., Nguyen, P. T., Quivey, R. G. Jr., and Marquis, R. E. (2010). Alkali production associated with malolactic fermentation by oral streptococci and protection against acid, oxidative, or starvation damage. Can. J. Microbiol. 56, 539–547. doi: 10.1139/w10-039
Sheng, J., and Marquis, R. E. (2007). Malolactic fermentation by Streptococcus mutans. FEMS Microbiol. Lett. 272, 196–201. doi: 10.1111/j.1574-6968.2007.00744.x
Tao, Y., Zhou, Y., Ouyang, Y., and Lin, H. C. (2015). Association of oral streptococci community dynamics with severe early childhood caries as assessed by PCR-denaturing gradient gel electrophoresis targeting the rnpB gene. J. Med. Microbiol. 64, 936–945. doi: 10.1099/jmm.0.000093
van der Ploeg, J. R. (2005). Regulation of bacteriocin production in Streptococcus mutans by the quorum-sensing system required for development of genetic competence. J. Bacteriol. 187, 3980–3989. doi: 10.1128/JB.187.12.3980-3989.2005
Vos, T., Abajobir, A. A., Abate, K. H., Abbafati, C., Abbas, K. M., Abd-Allah, F., et al. (2017). Global, regional, and national incidence, prevalence, and years lived with disability for 328 diseases and injuries for 195 countries, 1990-2016: a systematic analysis for the global burden of disease study 2016. Lancet 390, 1211–1259. doi: 10.1016/S0140-6736(17)32154-2
Keywords: genetic analysis, clinical isolates, dental caries, Streptococcus mutans, Streptococcus gordonii
Citation: Liu S, Sun Y, Liu Y, Hu F, Xu L, Zheng Q, Wang Q, Zeng G and Zhang K (2022) Genomic and phenotypic characterization of Streptococcus mutans isolates suggests key gene clusters in regulating its interaction with Streptococcus gordonii. Front. Microbiol. 13:945108. doi: 10.3389/fmicb.2022.945108
Edited by:
Nicolas Nalpas, University of Tübingen, GermanyReviewed by:
Sadaf Hasan, New York University, United StatesSahana Vasudevan, SASTRA University, India
Copyright © 2022 Liu, Sun, Liu, Hu, Xu, Zheng, Wang, Zeng and Zhang. This is an open-access article distributed under the terms of the Creative Commons Attribution License (CC BY). The use, distribution or reproduction in other forums is permitted, provided the original author(s) and the copyright owner(s) are credited and that the original publication in this journal is cited, in accordance with accepted academic practice. No use, distribution or reproduction is permitted which does not comply with these terms.
*Correspondence: Kai Zhang, emhhbmdrYWkyOTc4OEAxNjMuY29t