- 1Ruminant Nutrition and Physiology Laboratory, College of Animal Science and Technology, Shandong Agricultural University, Tai’an, China
- 2Beijing Enhalor International Tech Co., Ltd., Beijing, China
- 3College of Life Sciences, Shandong Agricultural University, Tai’an, China
The effect of heat stress on ruminants is an important issue. In recent years, the growth of the Chinese dairy industry has rapidly increased, generating RMB 468,738 million revenue in 2021. A decreased milk yield is the most recognized impact of heat stress on dairy cows and results in significant economic loss to dairy producers. Heat stress also lowers immunity and antioxidant capacity and changes the bacterial composition and metabolites of the rumen. The purpose of this study was to investigate the effect of addition Saccharomyces cerevisiae culture on heat-stressed cows. The impact of S. cerevisiae culture on microbiota composition, functional profiles, and metabolomics was assessed in heat-stressed cows. A total of 45 Holstein cows in mid-lactation were selected and randomly divided into three groups (15 cows per group). Groups D-C, D-A, and D-B were fed with the basal diet, the basal diet + first S. cerevisiae culture 100 g/day, and the basal diet + second S. cerevisiae culture 30 g/day, respectively. The trial lasted 60 days. There was an increased abundance of the Phylum Firmicutes in the rumen of heat-stressed dairy cows fed with S. cerevisiae, of which four genera had significantly higher abundance, Ruminococcus_gauvreauii_group, Butyrivibrio_2, Moryella, and Ruminiclostridium_6. At the functional level, ten pathways differed significantly between the three groups (P < 0.05), with an increase in fatty acid biosynthesis, fatty acid metabolism, PPAR signaling pathway, ferroptosis, and biotin metabolism in the treatment groups. More differential metabolites were found in the D-C and D-A groups than in the D-C and D-B groups. These results indicate that S. cerevisiae cultures can influence the health status of heat-stressed cows by modulating rumen microbial composition, function, and metabolites, thereby improving rumen cellulolytic capacity. This study can provide or offer suggestions or recommendations for the development and utilization of feed additives.
Introduction
According to the United Nations Intergovernmental Panel on Climate Change (IPCC) report, the burning of fossil fuels and deforestation (Gray, 2007; Jianbin, 2013) accelerate the pace of global warming, which will be a big challenge for the dairy industry in China (Zhang et al., 2020), impacting the success of its dairy industry. Shandong Province in East China has a temperate monsoon climate with high summer temperatures, and cows in this region are severely affected by heat stress. This has led to a decrease in milk production and feed intake and an increase in the incidence of intramammary bacterial infection (IMI) (West, 2003; Rakib et al., 2020). Heat stress also lowers immunity and antioxidant capacity (Tao et al., 2012; Du et al., 2022) and changes the bacterial composition and metabolites of the rumen (Zhao et al., 2019). This is detrimental to the health of cows and causes serious losses to the dairy industry.
The gastrointestinal tract of cows is a complex ecosystem that has a strong impact on health (Zeineldin et al., 2018a). The rumen, the first stomach of ruminants, has a complex and diverse microbiota that can digest cellulose and provide nutrients to the organism (Bickhart and Weimer, 2018). The rumen microbiota can also stimulate and improve immune responses (Zhang et al., 2021). Recent studies have shown that the negative effects of heat stress on cows can be ameliorated by adding probiotics or other additives to the feed (Liu et al., 2014; Ye et al., 2016; Shah et al., 2020; Li et al., 2021a). These studies have shown varying degrees of improvement in milk production, immunity, and antioxidant capacity, as well as changes in the composition of the rumen microbiota and metabolites following the addition of these products. This may be due to the critical role played by host-rumen-microbiota interactions, and these additives alter the composition of the rumen flora and its microbial metabolites, thereby improving the health of the cow. The same argument is made in a review by Zeineldin et al. (2018b).
Our previous studies showed that Saccharomyces cerevisiae cultures could improve the performance, antioxidant capacity, and immunity of heat-stressed cows and reduce corporate losses (Du et al., 2022). Previous studies on heat-stressed cows have centered on rumen microbial diversity and metabolomics, and this experiment instead focused on rumen microbial diversity, metagenomics, and metabolomics. The objective of this study was to determine the effect of the addition of S. cerevisiae cultures on rumen microbial composition, rumen microorganisms’ function, and rumen metabolites.
Materials and methods
Animals, diets, and trial design
This study was conducted at the cattle farm of Xianghe Dairy Co., in Zaozhuang City, Shandong Province. A total of 45 Holstein cows in mid-lactation with a similar milk yield (29.4 ± 3.7), parity (1.8 ± 0.6), days in lactation (158 ± 14), and health were selected and divided into three groups of 15 cows each in a randomized complete block design. The control group (D-C) was fed with a basal diet, while the treatment groups were fed with a basal diet + the first S. cerevisiae culture at a rate of 100 g/day (D-A) or a basal diet + the second S. cerevisiae culture at a rate of 30 g/day (D-B). Both S. cerevisiae cultures were provided by Beijing Enhalor International Tech Co., Ltd. Cows in the treatment groups were fed with S. cerevisiae culture separately during the morning feeding. The trial lasted for 60 days, with 10-day and 50-day adaptation and formal trial periods, respectively. The average temperature of the dairy barn during the formal trial period was a minimum of 26.9°C. Cows were under heat stress at all times during the trial.
Rumen fluid sampling
Two hours after feeding the cows in the morning of day 50 of the formal trial period, the rumen fluid from 45 cows was sampled using a portable rumen fluid sampling tube. Rumen fluid (100 ml) was collected per cow, filtered through four layers of gauze, packed into 2 ml freezing tubes, and preserved in liquid nitrogen.
DNA extraction and sequencing
DNA was extracted from ruminal fluid samples using the HiPure Stool DNA Kits (Magen, Guangzhou, China) according to the manufacturer’s protocols. NanoDrop2000 was used to determine the purity of the extracted DNA. The 16S rDNA V3-V4 region was amplified by PCR using the following primers: 341F, 5′-CCTACGGGNGGCWGCAG-3′, and 806R 5′-GGACTACHVGGGTATCTAAT-3′. Amplicons were extracted from 2% agarose gels, purified using the AxyPrep DNA Gel Extraction Kit (Axygen Biosciences, Union City, CA, United States) according to the manufacturer’s instructions, and quantified using an ABI StepOnePlus Real-Time PCR System (Life Technologies, Foster City, CA, United States). Purified amplicons were pooled in equimolar ratios and paired-end sequenced on an Illumina platform (PE250) according to standard protocols.
Bioinformatics analysis
Raw reads were further filtered using FASTP to obtain high-quality clean reads (Chen et al., 2018a) (version 0.18.0). Paired-end clean reads were merged as raw tags using FLASH (Magoč and Salzberg, 2011) (version 1.2.11) with a minimum overlap of 10 bp and mismatch error rates of 2%. Noisy raw tag sequences were filtered under specific filtering conditions (Bokulich et al., 2013) (QIIME version 1.9.1) to obtain high-quality clean tags. The filtering conditions are as follows: (1) break raw tags from the first low-quality base site where the number of bases in the continuous low-quality value (the default quality threshold is ≤3) reaches the set length (the default length is 3 bp); (2) then, filter tags whose continuous high-quality base length is less than 75% of the tag length. The clean tags were clustered into operational taxonomic units (OTUs) with h ≥ 97% similarity using the UPARSE (Edgar, 2013) (version 9.2.64) pipeline. All chimeric tags were removed using the UCHIME algorithm (Edgar et al., 2011), and effective tags were obtained for further analysis.
The representative OTU sequences were classified into organisms using a naive Bayesian model with the RDP classifier (Wang et al., 2007) (version 2.2) based on the SILVA database (version 132), with a confidence threshold value of 0.8. Chao1, ACE, Shannon, and Simpson were calculated using QIIME (Caporaso et al., 2010) (version 1.9.1) and plotted histograms using the omicsmart online tool.1 Principal coordinate analysis (PCoA) of unweighted-unifrac distances was generated using the R Vegan package (Oksanen et al., 2012) (version 2.5.3) and plotted using the R ggplot2 package (Wickham, 2011) (version 2.2.1). The stacked bar plot of the phylum and genus composition was visualized using the R ggplot2 package (version 2.2.1). The GraphPad Prism version 8.0.2 software was used to draw horizontal bar charts.
DNA extraction, metagenomic sequencing, and metagenomic data processing
Genomic DNA was extracted using HiPure Bacterial DNA Kits (Magen, Guangzhou, China) according to the manufacturer’s instructions. DNA quality was detected using Qubit (Thermo Fisher Scientific, Waltham, MA, United States) and Nanodrop (Thermo Fisher Scientific, Waltham, MA, United States). Qualified genomic DNA was first fragmented by sonication to a size of 350 bp and then end-repaired, A-tailed, and adaptor-ligated using the NEBNext® MLtra™ DNA Library Prep Kit for Illumina (NEB, Ipswich, MA, United States) according to the preparation protocol. DNA fragments with a 300–400 bp length were enriched by PCR, PCR products were purified using the AMPure XP system (Beckman Coulter, Brea, CA, United States), and libraries were analyzed for size distribution using the 2100 Bioanalyzer (Agilent, Santa Clara, CA, United States). Genome sequencing was performed on the Illumina NovaSeq 6000 sequencer using pair-end technology (PE 150).
Raw data from the Illumina platform were filtered using FASTP (version 0.18.0) (Chen et al., 2018b) by removing reads with any of the following: (1) ≥10% unidentified nucleotides (N), (2) ≥50% bases having Phred quality scores ≤20, or (3) aligned to the barcode adapter. After filtering, clean reads of each sample were assembled individually using MEGAHIT (version 1.1.2) (Li et al., 2014). Genes were predicted based on the final assembly contigs (>500 bp) using MetaGeneMark (version 3.38) (Zhu et al., 2010). The predicted genes ≥300 bp in length from all samples were pooled and combined based on ≥95% identity and 90% read coverage using CD-HIT (version 4.6) (Fu et al., 2012) to reduce the number of redundant genes for the downstream assembly step. The reads were realigned to the predicted gene using Bowtie (version 2.2.5) (Langmead and Salzberg, 2012) to count the read numbers. The final gene catalog was obtained from non-redundant genes with gene reads > 2. Quantitative analysis of genes was conducted using Bowtie (version 2.2.5) and PathoScope (version 2.0.7). The unigenes were annotated using DIAMOND (version 0.9.24) (Buchfink et al., 2015) by aligning them with the Kyoto Encyclopedia of Genes and Genomes (KEGG). The histogram of the difference was drawn using the omicshare online tool (see text footnote 1). The Kruskal-Wallis test was adopted. Meanwhile, Tukey’s HSD test was used to understand where the differences originated.
Analysis of the rumen metabolome
The samples (1 mL) were freeze-dried and resuspended in 100 μL prechilled 80% methanol by well vortex, incubated on ice for 5 min, and centrifuged at 15,000 g at 4°C for 15 min. The supernatants were diluted to a final concentration containing 53% methanol using LCMS-grade water. The samples were subsequently transferred to fresh Eppendorf tubes and centrifuged at 15,000 g at 4°C for 15 min. Finally, the supernatants were injected into the LCMS/MS system for analysis.
UHPLC-MS/MS analyses were performed using a Vanquish UHPLC system (Thermo Fisher, Germany) coupled with an Orbitrap Q ExactiveTM HF-X mass spectrometer (Thermo Fisher, Germany) from Gene Denovo Co., Ltd. (Guangzhou, China). Samples were injected onto a Hypersil GOLD column (100 × 2.1 mm, 1.9 μm) using a 17-min linear gradient at a flow rate of 0.2 ml/min. The eluents for the positive polarity mode were eluent A (0.1% FA in Water) and eluent B (Methanol), and the eluents for the negative polarity mode were eluent A (5 mM ammonium acetate, pH 9.0) and eluent B (Methanol). The solvent gradient was set up as follows: 2% B, 1.5 min; 2–100% B, 12.0 min; 100% B, 14.0 min; 100–2% B, 14.1 min; 2% B, 17 min. A Q ExactiveTM HF-X mass spectrometer was operated in the positive/negative polarity mode with a spray voltage of 3.2 kV, capillary temperature of 320°C, sheath gas flow rate of 40 arb, and aux gas flow rate of 10 arb.
The raw data files generated by UHPLC-MS/MS were processed using the Compound Discoverer 3.1 (CD3.1, Thermo Fisher) to perform the peak alignment, peak picking, and quantitation of each metabolite. The main parameters included are as follows: retention time tolerance, 0.2 min; actual mass tolerance, 5 ppm; signal intensity tolerance, 30%; signal/noise ratio, 3; minimum intensity, 100,000. The peak intensities were normalized to the total spectral intensity. The normalized data were used to predict the molecular formula based on additive ions, molecular ion peaks, and fragment ions. The peaks were then matched with the mzCloud,2 mz Vaultand Mass List database, to obtain accurate qualitative and relative quantitative results. Statistical analyses were performed using the statistical software, namely, R (R version R-3.4.3), Python (Python 2.7.6 version), using CentOS to run this software (CentOS release 6.6). When data were not normally distributed, normal transformations were attempted using the MaxQuant (MaxQuant version 1.6.17.0). The abundance of differential metabolites in the same group was normalized using the z-score, and the VIP score of OPLS-DA was used to draw the graph. The omicsmart online tool was used to draw differential KEGG enrichment bubble map and VIP score.3
Results
16S rDNA sequencing analysis
16S rDNA in the rumen fluid sample was filtered to obtain clean reads (758,403 ± 3,568 reads in the D-C group, 772,109 ± 4,155 reads in the D-A group, and 760,383 ± 3,892 reads in the D-B group). Our study also obtained 25,312 OTUs.
Bacterial diversity analysis
Alpha diversity indices, including Chao1, ACE, Shannon, and Simpson, showed that microbial community diversity in the rumen fluid samples was higher in the D-A group than in the other two groups (Figures 1A–D); however, this difference was not significant (P > 0.05). Beta diversity showed that there was no significant separation between the three groups of microbiota, indicating that there were no significant differences between them (Figure 2). There was also no significant separation at the OTU level using the ANOSIM test with R2 = –0.0095 (P = 0.549) (Supplementary Figure 1).
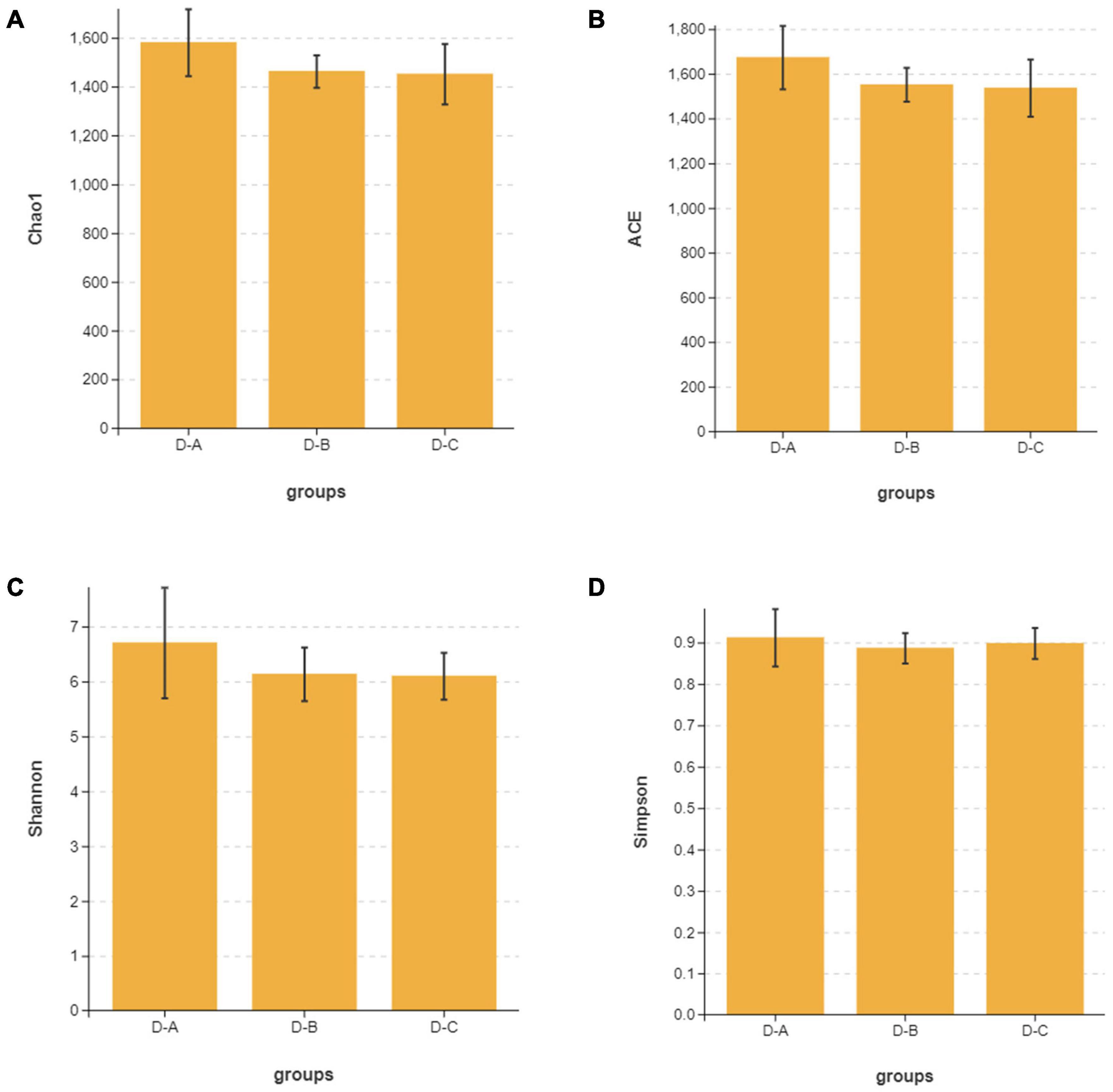
Figure 1. The (A) Chao1, (B) ACE, (C) Shannon, and (D) Simpson alpha diversity indices for the rumen microbiota.
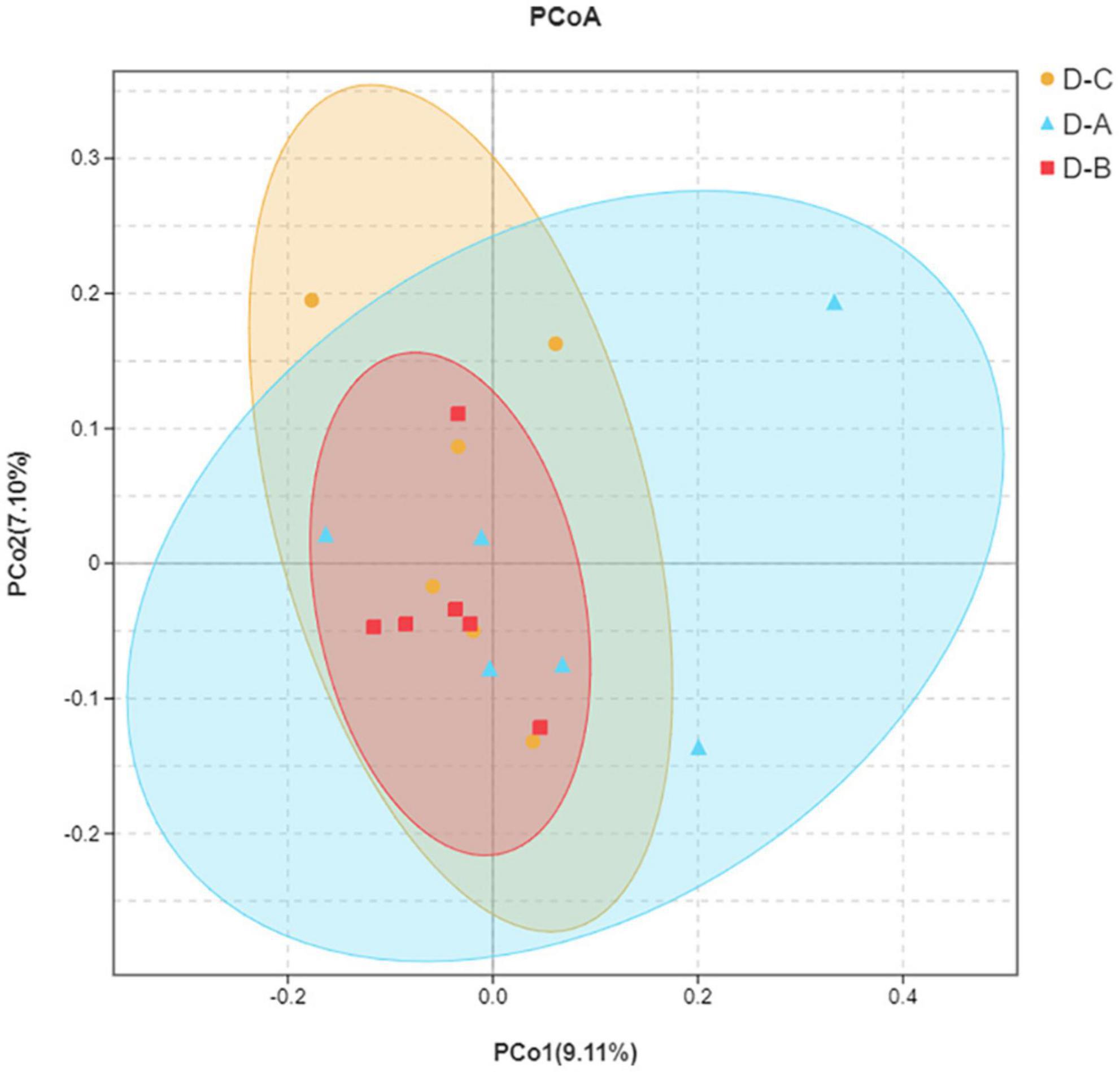
Figure 2. Principal coordinate analysis (PCoA) plot. The closer distance between the sample points indicates a higher similarity between the bacteria.
Rumen microbial composition at the phylum and genus levels
Figure 3 shows the top ten most abundant microbial species in the three groups. Of these phyla, Bacteroidetes, Proteobacteria, and Firmicutes had the highest abundances of 39.8 ± 2.02, 29.6 ± 3.7, and 25.9 ± 1.7%, respectively. The amount of Firmicutes was higher in the D-A group than in the other two groups, while the abundance of the Proteobacteria was lower in the D-A group, but these differences were not statistically significant (P > 0.05). The dominant genera were Succinivibrionaceae_UCG-001 (28.9 ± 3.78%), Prevotella_7 (18.7 ± 1.95%), Prevotella_1 (11.01 ± 0.95%), Ruminococcaceae_UCG-014 (3.31 ± 0.36%), Succiniclasticum (3.13 ± 0.81%), Dialister (2.36 ± 0.35%), Rikenellaceae_RC9_gut_group (1.47 ± 0.21%), Candidatus_Saccharimonas (1.16 ± 0.14%), Shuttleworthia (1.049 ± 0.087%), and Ruminococcus_1 (1.00 ± 0.12%). Four genera, namely, Ruminococcus_gauvreauii_group, Butyrivibrio_2, Moryella, and Ruminiclostridium_6, were significantly different (P < 0.05) after filtering species whose abundance was < 0.1% in all samples. Among the three genera, namely, Ruminococcus_gauvreauii_group, Butyrivibrio_2, and Moryella, the highest abundance was found in the D-A group. In the D-B group, Ruminiclostridium_6 is the highest abundance (Figure 4).
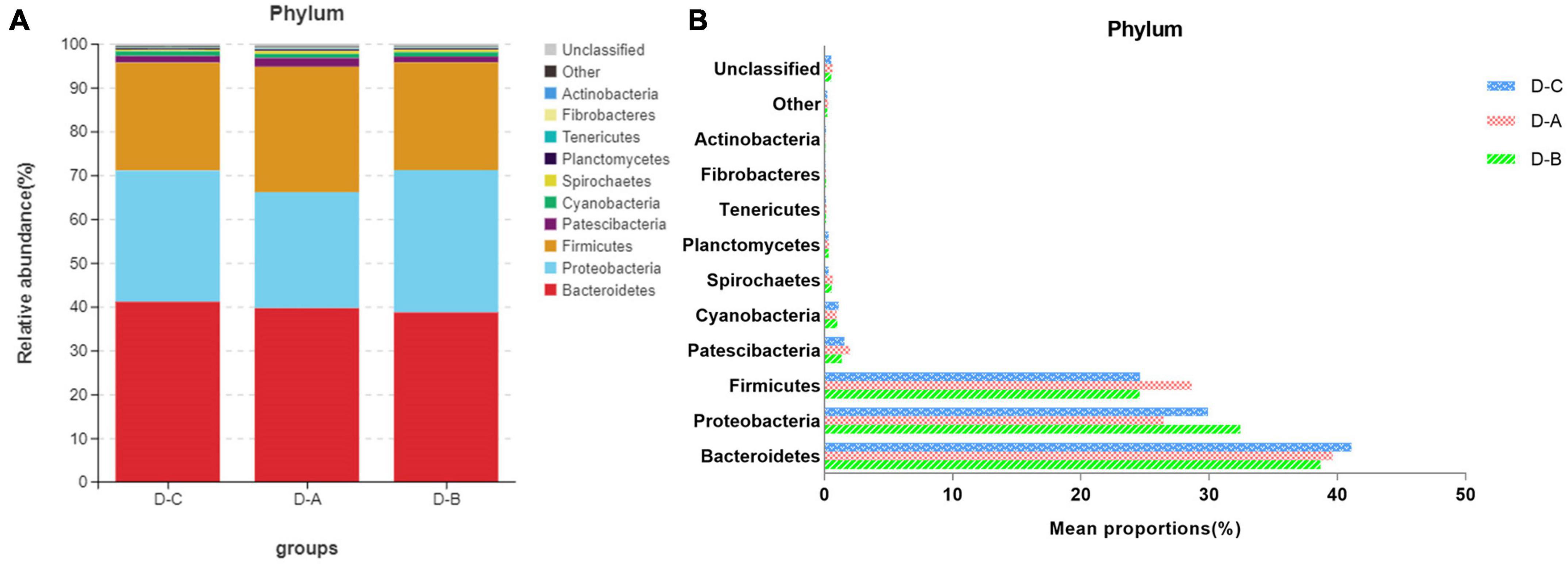
Figure 3. Relative rumen microbiota abundance at the (A,B) phylum level in the D-C, D-A, and D-B groups.
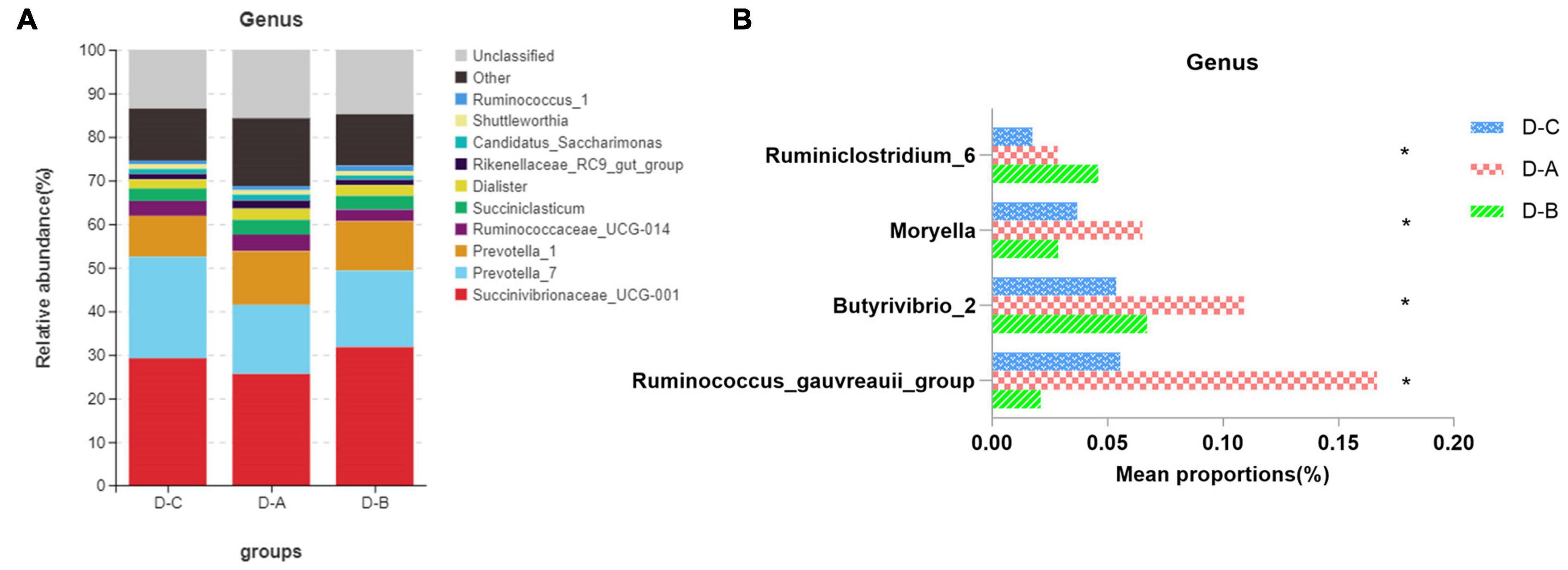
Figure 4. Relative rumen microbiota abundance at the (A,B) genus level in the D-C, D-A, and D-B groups. *P < 0.05.
Macrogenome and functional profiles of rumen microbiota
A total of 650,894,398 ± 4,988,354 clean reads were sequenced using the Illumina platform after quality control filtering. The sequences were then assembled to obtain 4,417,583 contigs. After gene annotation of the contigs and obtaining non-redundant gene sets, unigenes were compared with the KEGG database and 408 tertiary pathways were assessed. Of these, ten pathways differed significantly among the three phyla (P < 0.05), including five metabolic pathways, two cellular processing pathways, two organismal systems, and one genetic information processing pathway (Figure 5).
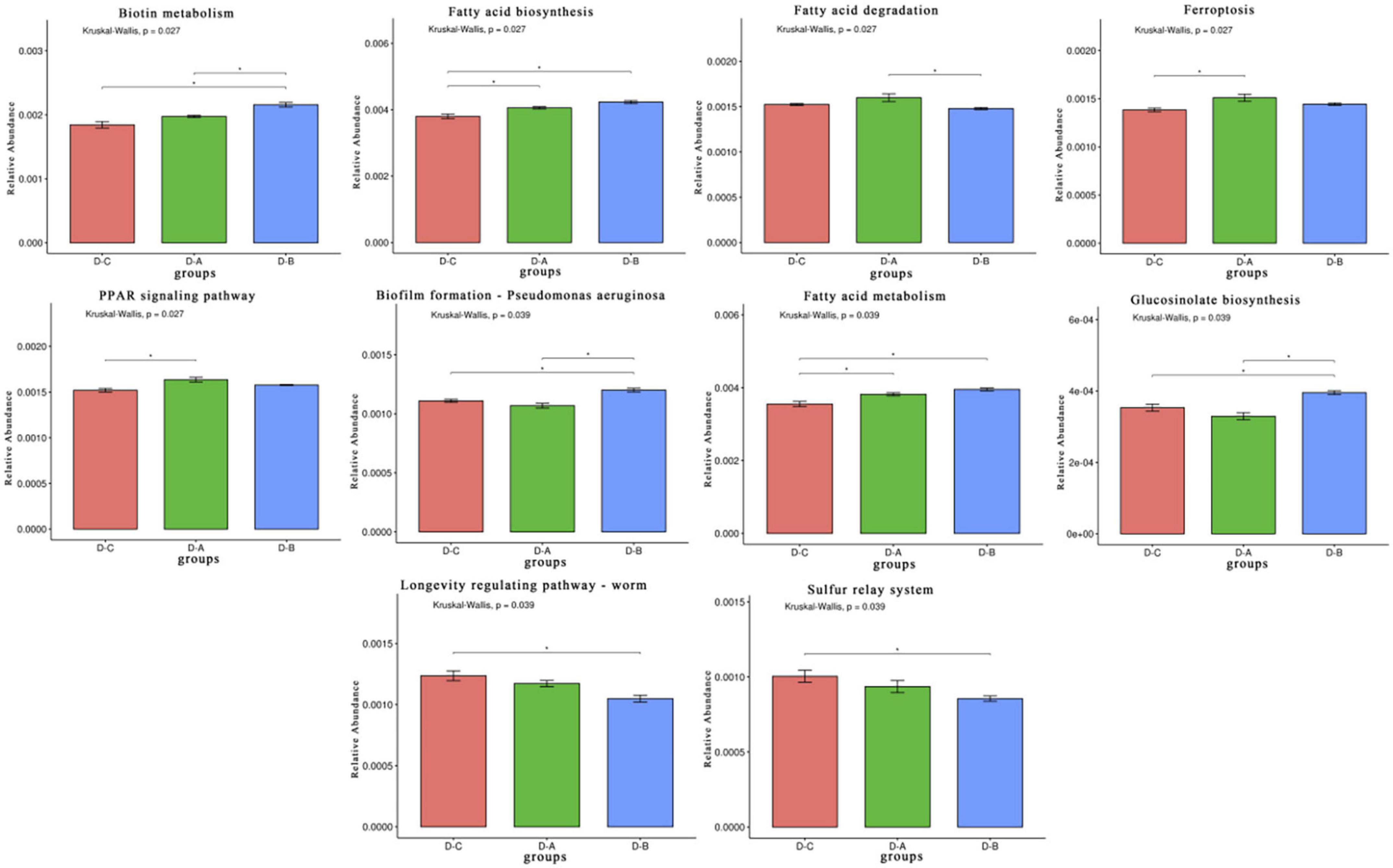
Figure 5. Rumen microbiota functional profiles. Differential Kyoto Encyclopedia of Genes and Genomes (KEGG) pathways were selected. *P < 0.05.
Metabolomics of the rumen fluid
To further assess the differences in rumen metabolites, the rumen fluid metabolomics were determined. We compared the D-C group with the D-A and D-B groups and identified 41 differential metabolites in the pos mode of the D-C vs. D-A group, 43 in the neg mode, 12 in the D-C vs. D-B pos mode, and 13 in the neg mode (P < 0.05, VIP > 1) (Figure 6). According to the differential metabolites, 50 enriched pathways were identified in the pos pattern of the D-C vs. D-A groups. The taste transduction pathway was significantly enriched, including upregulation of the four metabolites, D-phenylalanine, maltose, acetylcholine, and serotonin in the D-A group. In the neg model, 52 enriched pathways were identified, of which four, pentose phosphate, primary bile acid biosynthesis, α-linolenic acid metabolism, and carbon metabolism, were significantly enriched. In the pentose phosphate pathway, gluconic acid and D-glucono-1,5-lactone were upregulated in the D-A group and in the primary bile acid biosynthesis pathway, taurine and glycocholic acid were upregulated, sugarcane deoxycholic acid was downregulated in the D-A group and in the α-linolenic acid metabolism pathway, jasmonic acid was upregulated in the D-A group and in carbon metabolism, and gluconic acid and D-glucono-1,5-lactone were upregulated in the D-A group. In the D-C vs. D-B group, 21 enrichment pathways were found in the pos mode, of which one metabolic pathway, porphyrin, and chlorophyll metabolism were upregulated, and biliverdin and fecal biliverdin were downregulated in the D-B group. Twenty-five enriched pathways were found in the neg model, but none were significantly enriched (Figure 7).
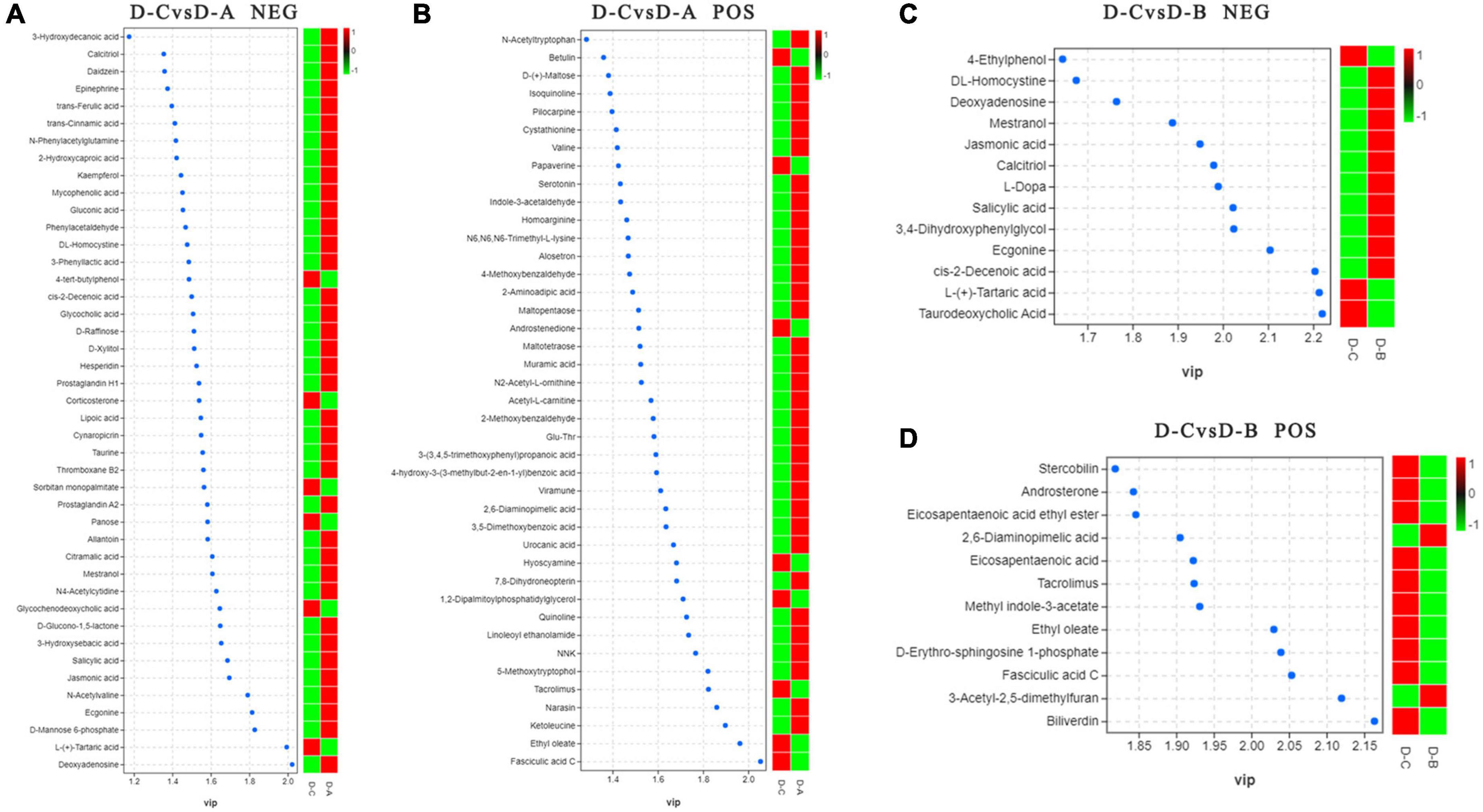
Figure 6. Distinguishing metabolites in the rumen fluid. (A) Differential metabolites between D-C and D-A in the neg mode, (B) differential metabolites between D-C and D-A in the pos mode, (C) differential metabolites between D-C and D-B in the neg mode, and (D) differential metabolites between D-C and D-B in the pos mode. VIP > 1, P < 0.05.
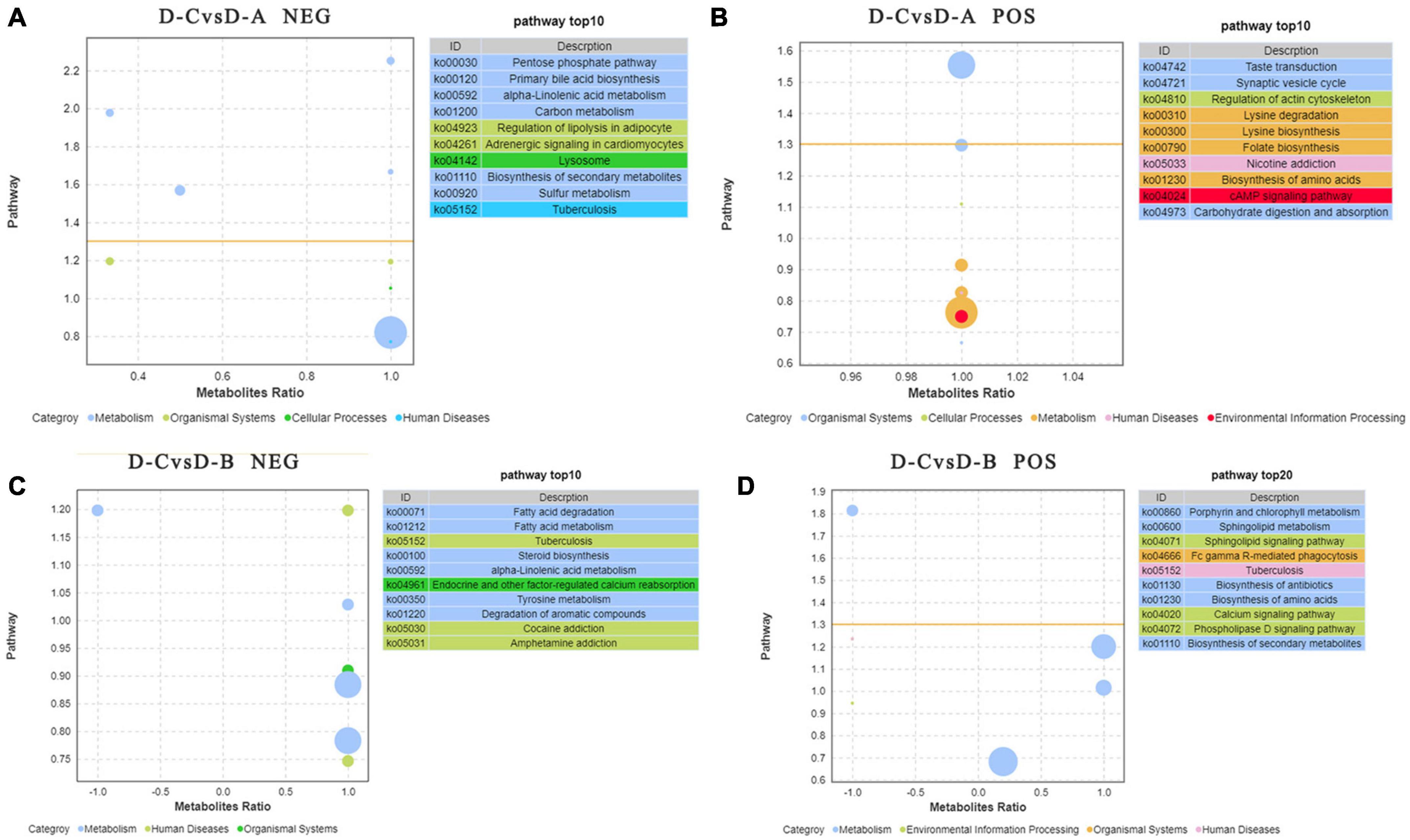
Figure 7. Pathways with significant enrichment in differential metabolites were selected, and bubble maps were drawn using information about upregulation and downregulation. The X-axis represents the difference between the number of upregulated and downregulated differential metabolites as a percentage of total differential metabolites. The yellow line represents the threshold of P = 0.05. The Y-axis represents –log10 (P-value). Different colors represent different categories. (A) Enrichment difference bubble chart of metabolites between D-C and D-A in the neg mode, (B) enrichment difference bubble chart of metabolites between D-C and D-A in the pos mode, (C) enrichment difference bubble chart of metabolites between D-C and D-B in the neg mode, and (D) enrichment difference bubble chart of metabolites between D-C and D-B in the pos mode.
Discussion
To understand the mechanism by which S. cerevisiae cultures alleviate heat stress in dairy cows, the experiment investigated the effects of S. cerevisiae cultures on the microbial composition, metagenome, and metabolome of the rumen.
Previous studies indicate that the species diversity and abundance of rumen microorganisms play a critical role in maintaining host physiology (Xu et al., 2021). While Chao1 and ACE indices are primarily used to measure species richness, the Simpson and Shannon indices combine the richness and evenness of a species. Our study found that rumen bacterial abundance and diversity were higher in cows that were fed with the first yeast culture than in the other two groups, although these findings were not statistically significant. This may be due to the increased diversity and abundance of rumen bacteria after the addition of S. cerevisiae culture. The environment within the rumen can change to some extent and may affect the bacterial species involved (Ghazanfar et al., 2017). Our results showed that Bacteroidetes, Proteobacteria, and Firmicutes were the dominant phyla in the rumen of all three groups of dairy cows, which supports the findings of prior studies (Xue et al., 2019). The abundance of Firmicutes was highest in the D-A group, while the abundance of Proteobacteria was lowest in the D-A group; however, these differences were not significant. Firmicutes are associated with the degradation of structural polysaccharides (Zened et al., 2013), which may impact the breakdown of cellulose in the rumen. Zou et al. (2019) found that yaks with lower feed conversion efficiency also have a lower relative abundance of Firmicutes in the rumen. In contrast, Proteobacteria is associated with increased disease risk and an elevated abundance of these bacteria correlates with gut inflammation. Ruminococcus and Firmicutes are involved in degrading fiber from cellulose-rich feeds (Evans et al., 2011), while Butyrivibrio is one of the few genera that can utilize xylan and pectin and degrade hemicellulose, hydrogenate fatty acids, and protein (Bryant and Small, 1956; Orpin et al., 1985; Paillard et al., 2007). Moryella, a member of the Lachnospira family of Firmicutes, can produce short-chain fatty acids through fermentation of dietary polysaccharides (Laffin et al., 2019). Short-chain fatty acids are important substrates for regulating gastrointestinal tract epithelial cells and are able to modulate the inflammatory response (Guo et al., 2020). Our study found a significant increase in the abundance of several genera involved in cellulose catabolism in the D-A group, some of which were also able to produce volatile fatty acids, which decreased the abundance of Proteobacteria. These findings indicated that the addition of S. cerevisiae culture increased cellulose degradation and reduced inflammation in heat-stressed cows.
The trial assessed the mechanism by which S. cerevisiae cultures affect the rumen of heat-stressed cows by metagenome and found ten pathways that differed significantly between the three groups. Fatty acid biosynthesis, fatty acid metabolism, peroxisome proliferator-activated receptor (PPAR) signaling, and ferroptosis and biotin metabolism were all enriched in the treatment groups. Yeast is known as a natural source of B vitamins. According to let’s eat right to keep fit by Adelle Davis, yeast is one of the four foods that contain complete vitamin B. S. cerevisiae culture is a product obtained from S. cerevisiae by fermentation and concentration, it is also rich in high levels of vitamin B, and studies indicate that vitamin B compounds can increase milk production in dairy cows (Chen et al., 2011). Biotin, a water-soluble B vitamin, is an essential nutrient in the rumen of dairy cows and a required cofactor for five carboxylases. These biotin-dependent carboxylases participate in several metabolic pathways, including fatty acid synthesis and fatty acid oxidation, and biotin or carboxylase deficiency can lead to metabolic dysfunction. Saika et al. (2019) and Lauridsen (2020) found that fatty acids are involved in intestinal mucosal barrier function and oxidative stress and inflammatory responses (Saika et al., 2019; Lauridsen, 2020). By activating fatty acid receptors, they can promote anti-inflammatory and neuronal responses and regulate immune homeostasis (Kimura et al., 2019). The PPAR is also activated by fatty acids and involved in regulating lipid metabolism and inflammation. In addition, PPAR signaling can inactivate NF-κB, promote antioxidant enzyme expression, and reduce the concentration of reactive oxygen species, thus improving antioxidant capacity (Korbecki et al., 2019). Cellulolytic bacteria in the rumen require biotin for growth, and Abel et al. (2001) showed that biotin improves fiber fermentation in the rumen. Thus, we speculated that the addition of S. cerevisiae culture increased B vitamin levels in the rumen, which increased biotin metabolism and production, elevated fatty acid synthesis, activated PPAR signaling, and improved the immune response and antioxidant capacity of the cows. The increase in biotin also elevated the abundance of cellulolytic Firmicutes in the rumen and improved the digestibility of the feed.
Ferroptosis is an iron-dependent type of programed cell death caused by massive lipid peroxidation-mediated membrane damage. This process is distinct from apoptosis, cellular necrosis, and cellular autophagy (Stockwell et al., 2017; Tang et al., 2019). In most cases, ferroptosis causes an inflammatory response in the body that induces cell death and is harmful to the organism. However, there is evidence that ferroptosis is a double-edged sword and may protect cells from inflammation under certain conditions (Sun et al., 2020). Two groups of cows fed with S. cerevisiae cultures in our study were enriched in the ferroptosis pathway, with significant differences between the D-A and control groups, which may be related to increased fatty acid metabolism.
The taste transduction pathway in mammals is not only capable of producing taste but is also expressed in gastrointestinal tract tissues so may play an important role in resisting microbial infections, regulating nutrient absorption, and maintaining internal homeostasis (Yangyu et al., 2017). The receptors for sweetness, freshness, and bitterness are all G protein-coupled, and Minic et al. (2005) found a high degree of homology between the yeast pheromone signaling pathway and mammalian G protein-coupled receptors. G protein-coupled receptors are also expressed in S. cerevisiae, which may explain why metabolites are enriched in the taste transduction pathway. The primary breakdown products of cellulose are sucrose and maltose, and according to the sequencing results, the increased abundance of cellulolytic bacteria is associated with elevated maltose levels. G protein-coupled receptors respond to maltose stimulation (Zhao et al., 2003) and release acetylcholine. These results suggest that S. cerevisiae cultures may enrich the ruminal taste transduction pathways involved in maintaining internal homeostasis.
The pentose phosphate pathway is the primary pathway for oxidative glucose catabolism in animals and microorganisms and regulates cellular energy supply and cellular respiration, improving metabolic efficiency (Li et al., 2021b). Ruminococcus metabolizes pentose via the pentose phosphate pathway, so the increased abundance of Ruminococcus in this study may account for the upregulation of metabolites associated with the pentose phosphate pathway in the D-A group. The pentose phosphate pathway produces NADPH, which is essential for antioxidant capacity (Patra and Hay, 2014), acting as a hydrogen donor for fatty acid synthesis, participating in fatty acid synthesis, and providing energy to the body (Ohlrogge and Jaworski, 1997). Taurine is a non-essential amino acid that cannot function as a direct energy source (Chesney, 1985) but can provide protection against oxidative stress by increasing superoxide dismutase and glutathione peroxidase activity (Nonaka et al., 2001). Significant enrichment of the pentose phosphate pathway and increased taurine content after the addition of S. cerevisiae cultures improved the antioxidant capacity of heat-stressed cows. Glycochenodeoxycholic acid is a hydrophobic bile salt that accumulates in the liver during cholestasis and can induce apoptosis in hepatocytes at certain concentrations. In the D-A group, glycochenodeoxycholic acid production was downregulated, which may have relieved stress on the liver and ensured the health of the organism.
Conclusion
This trial studied the effects of S. cerevisiae culture-supplemented diets of heat-stressed cows on rumen microbial composition, metagenomics, and metabolomics and identified a strong relationship between supplementation and vitamin B. As shown in Figure 8, yeast cultures are rich in vitamin B. Supplementation with S. cerevisiae culture enriched the rumen in biotin, a water-soluble vitamin B. Biotin can act as a carboxylase cofactor, participating in the synthesis and metabolism of fatty acids, which in turn can activate PPAR and improve the antioxidant capacity and immunity of the organism. In addition, most cellulolytic bacteria require vitamin B for growth, which may account for the increased abundance of cellulolytic bacteria such as Ruminococcus and Butyrivibrio. These bacteria stimulate cellulose catabolism, upregulate metabolites in the pentose phosphate pathway, increase primary bile acid biosynthesis and taste transduction pathway signaling, and positively impact the health of cows. Our findings indicate that S. cerevisiae cultures enriched with vitamin B provide protection against heat stress and should be considered as an anti-heat stress feed additive in production.
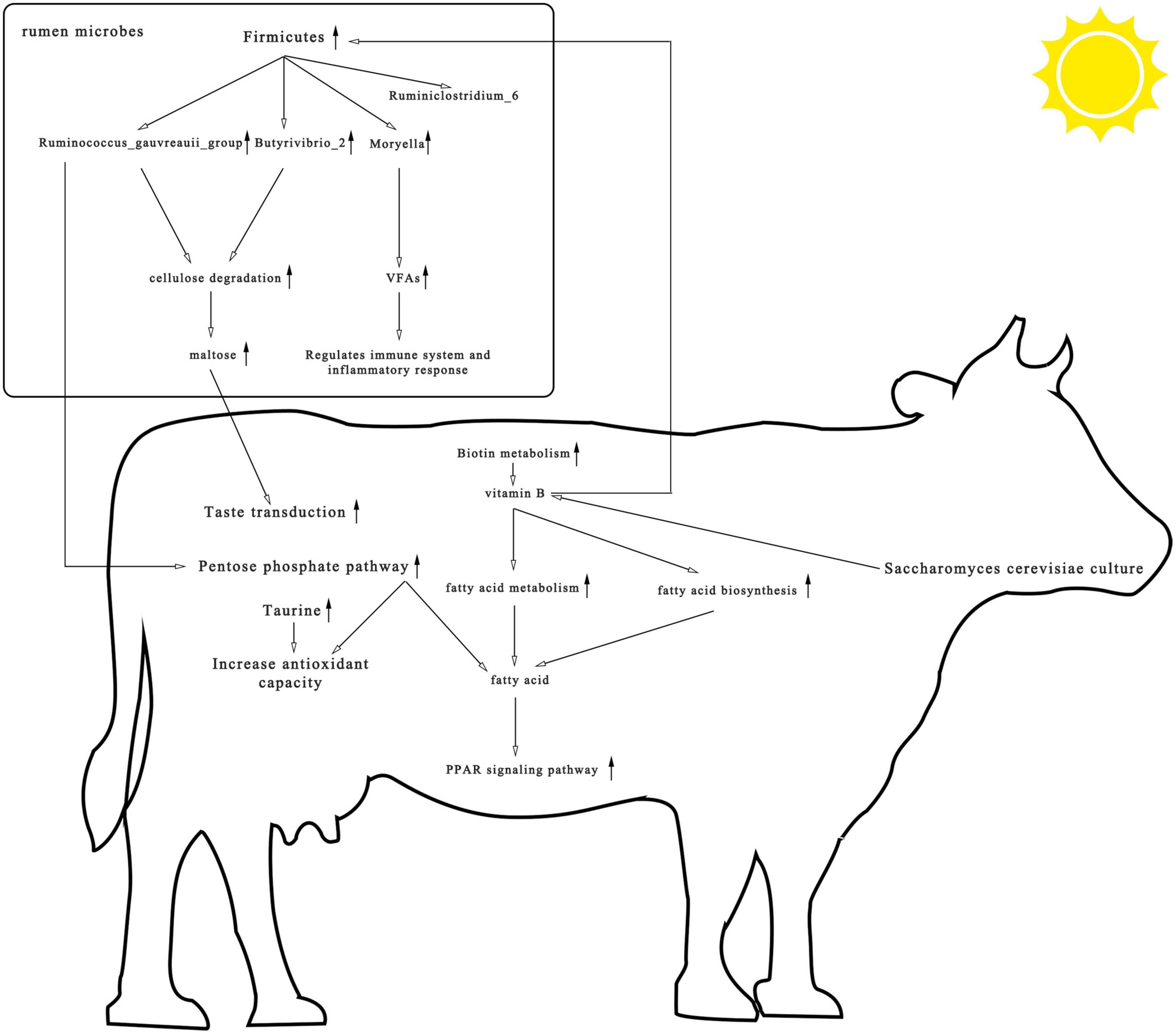
Figure 8. Consolidation of rumen microbial composition, pathways, and differential metabolite results found among heat-stressed cows fed with Saccharomyces cerevisiae cultures. The upward arrow represents the increase in rumen microbial composition, pathways, and differential metabolite after the addition of S. cerevisiae cultures.
Data availability statement
The datasets presented in this study can be found in online repositories. The names of the repository/repositories and accession number(s) can be found at: https://www.ncbi.nlm.nih.gov/, PRJNA834564.
Ethics statement
The animal study was reviewed and approved by Ethical Commission of Shandong Agricultural University.
Author contributions
DD, PC, CW, and ZH conceived the project and designed the protocol. DD and WJ performed the experiments. DD, LF, and YZ wrote the manuscript. All authors read and approved the final manuscript.
Funding
This research was funded by the National Natural Science Foundation of China, grant number: 32172760. It was also funded by the study on efficient feeding and Meat Quality Improvement Technology of Beef Cattle, grant number: 2020LZGC014-05.
Conflict of interest
PC was employed by Beijing Enhalor International Tech Co., Ltd.
The remaining authors declare that the research was conducted in the absence of any commercial or financial relationships that could be construed as a potential conflict of interest.
Publisher’s note
All claims expressed in this article are solely those of the authors and do not necessarily represent those of their affiliated organizations, or those of the publisher, the editors and the reviewers. Any product that may be evaluated in this article, or claim that may be made by its manufacturer, is not guaranteed or endorsed by the publisher.
Acknowledgments
We thank Beijing Enhalor International Tech Co., Ltd. (www.enhalor.com) for providing us with products. We also thank Shandong Xianghe Dairy Co., Ltd. for providing the dairy cows.
Supplementary material
The Supplementary Material for this article can be found online at: https://www.frontiersin.org/articles/10.3389/fmicb.2022.935004/full#supplementary-material
Footnotes
References
Abel, H., Immig, I., Gomez, C. C., and Steinberg, W. (2001). Research note: effect of increasing dietary concentrate levels on microbial biotin metabolism in the artificial rumen simulation system (RUSITEC). Arch. Tierernahr. 55, 371–376. doi: 10.1080/17450390109386203
Bickhart, D. M., and Weimer, P. J. (2018). Symposium review: host–rumen microbe interactions may be leveraged to improve the productivity of dairy cows. J. Dairy Sci. 101, 7680–7689. doi: 10.3168/jds.2017-13328
Bokulich, N. A., Subramanian, S., Faith, J. J., Gevers, D., Gordon, J. I., Knight, R., et al. (2013). Quality-filtering vastly improves diversity estimates from Illumina amplicon sequencing. Nat. Methods 10, 57–59. doi: 10.1038/nmeth.2276
Bryant, M. P., and Small, N. (1956). THE ANAEROBIC MONOTRICHOUS BUTYRIC ACID-PRODUCING CURVED ROD-SHAPED BACTERIA OF THE RUMEN. J. Bacteriol. 72, 16–21. doi: 10.1128/jb.72.1.16-21.1956
Buchfink, B., Xie, C., and Huson, D. H. (2015). Fast and sensitive protein alignment using DIAMOND. Nat. Methods 12, 59–60. doi: 10.1038/nmeth.3176
Caporaso, J. G., Kuczynski, J., Stombaugh, J., Bittinger, K., Bushman, F. D., Costello, E. K., et al. (2010). QIIME allows analysis of high-throughput community sequencing data. Nat. Methods 7, 335–336. doi: 10.1038/nmeth.f.303
Chen, B., Wang, C., Wang, Y. M., and Liu, J. X. (2011). Effect of biotin on milk performance of dairy cattle: a meta-analysis1. J. Dairy Sci. 94, 3537–3546. doi: 10.3168/jds.2010-3764
Chen, S., Zhou, Y., Chen, Y., and Gu, J. (2018b). fastp: an ultra-fast all-in-one FASTQ preprocessor. bioRxiv [Preprint]. doi: 10.1101/274100
Chen, S., Zhou, Y., Chen, Y., and Gu, J. (2018a). fastp: an ultra-fast all-in-one FASTQ preprocessor. Bioinformatics 34, i884–i890. doi: 10.1093/bioinformatics/bty560
Du, D., Feng, L., Chen, P., Jiang, W., Zhang, Y., Liu, W., et al. (2022). Effects of saccharomyces cerevisiae cultures on performance and immune performance of dairy cows during heat stress. Front. Veterinary Sci. 9:851184. doi: 10.3389/fvets.2022.851184
Edgar, R. C. (2013). UPARSE: highly accurate OTU sequences from microbial amplicon reads. Nat. Methods 10, 996–998. doi: 10.1038/nmeth.2604
Edgar, R. C., Haas, B. J., Clemente, J. C., Quince, C., and Knight, R. (2011). UCHIME improves sensitivity and speed of chimera detection. Bioinformatics 27, 2194–2200. doi: 10.1093/bioinformatics/btr381
Evans, N. J., Brown, J. M., Murray, R. D., Getty, B., Birtles, R. J., Hart, C. A., et al. (2011). Characterization of novel bovine gastrointestinal tract Treponema isolates and comparison with bovine digital dermatitis treponemes. Appl. Environ. Microbiol. 77, 138–147. doi: 10.1128/AEM.00993-10
Fu, L., Niu, B., Zhu, Z., Wu, S., and Li, W. (2012). CD-HIT: accelerated for clustering the next-generation sequencing data. Bioinformatics 28, 3150–3152. doi: 10.1093/bioinformatics/bts565
Ghazanfar, S., Ahmed, I., Khalid, N., and Imran, M. (2017). “Probiotic yeast: mode of action and its effects on ruminant nutrition,” in Yeast, eds A. Morata and I. Loira (London: IntechOpen).
Gray, V. (2007). Climate change 2007: the physical science basis summary for policymakers. Energy Environ. 18, 433–440. doi: 10.1260/095830507781076194
Guo, H., Chou, W.-C., Lai, Y., Liang, K., Tam, J., Brickey, J., et al. (2020). Multi-omics analyses of radiation survivors identify radioprotective microbes and metabolites. Science 370:eaay9097. doi: 10.1126/science.aay9097
Jianbin, W. S. L. Y. Z. Z. W. X. H. (2013). Global mean temperature has risen by 1°C since the industrialization. Adv. Clim. Change Res. 9, 383–385. doi: 10.3969/j.issn.1673-1719.2013.05.012
Kimura, I., Ichimura, A., Ohue-Kitano, R., and Igarashi, M. (2019). Free fatty acid receptors in health and disease. Physiologic. Rev. 100, 171–210. doi: 10.1152/physrev.00041.2018
Korbecki, J., Bobiński, R., and Dutka, M. (2019). Self-regulation of the inflammatory response by peroxisome proliferator-activated receptors. Inflamm. Res. 68, 443–458. doi: 10.1007/s00011-019-01231-1
Laffin, M., Fedorak, R., Zalasky, A., Park, H., Gill, A., Agrawal, A., et al. (2019). A high-sugar diet rapidly enhances susceptibility to colitis via depletion of luminal short-chain fatty acids in mice. Sci. Rep. 9:12294. doi: 10.1038/s41598-019-48749-2
Langmead, B., and Salzberg, S. L. (2012). Fast gapped-read alignment with Bowtie 2. Nat. Methods 9, 357–359. doi: 10.1038/nmeth.1923
Lauridsen, C. (2020). Effects of dietary fatty acids on gut health and function of pigs pre- and post-weaning. J. Anim. Sci. 98:skaa086. doi: 10.1093/jas/skaa086
Li, D., Liu, C.-M., Luo, R., Sadakane, K., and Lam, T.-W. (2014). MEGAHIT: an ultra-fast single-node solution for large and complex metagenomics assembly via succinct de Bruijn graph. Quantitat. Biol. doi: 10.1093/bioinformatics/btv033
Li, Y., Fang, L., Xue, F., Mao, S., Xiong, B., Ma, Z., et al. (2021a). Effects of bamboo leaf extract on the production performance, rumen fermentation parameters, and rumen bacterial communities of heat-stressed dairy cows. Anim. Biosci. 34, 1784–1793. doi: 10.5713/ab.20.0527
Li, Y., Lv, M., Wang, J., Tian, Z., Yu, B., Wang, B., et al. (2021b). Dandelion (Taraxacum mongolicum Hand.-Mazz.) supplementation-enhanced rumen fermentation through the interaction between ruminal microbiome and metabolome. Microorganisms 9:83. doi: 10.3390/microorganisms9010083
Liu, D.-Y., He, S.-J., Liu, S.-Q., Tang, Y.-G., Jin, E.-H., Chen, H.-L., et al. (2014). Daidzein enhances immune function in late lactation cows under heat stress. Anim. Sci. J. 85, 85–89. doi: 10.1111/asj.12080
Magoč, T., and Salzberg, S. L. (2011). FLASH: fast length adjustment of short reads to improve genome assemblies. Bioinformatics 27, 2957–2963. doi: 10.1093/bioinformatics/btr507
Minic, J., Sautel, M., Salesse, R., and Pajot-Augy, E. (2005). Yeast system as a screening tool for pharmacological assessment of g protein coupled receptors. Curr. Med. Chem. 12, 961–969. doi: 10.2174/0929867053507261
Nonaka, H., Tsujino, T., Watari, Y., Emoto, N., and Yokoyama, M. (2001). Taurine prevents the decrease in expression and secretion of extracellular superoxide dismutase induced by homocysteine: amelioration of homocysteine-induced endoplasmic reticulum stress by taurine. Circulation 104, 1165–1170. doi: 10.1161/hc3601.093976
Ohlrogge, J. B., and Jaworski, J. G. (1997). REGULATION OF FATTY ACID SYNTHESIS. Ann. Rev. Plant Physiol. Plant Mol. Biol. 48, 109–136. doi: 10.1146/annurev.arplant.48.1.109
Oksanen, J., Blanchet, F. G., Kindt, R., Legendre, P., and Wagner, H. (2012). Vegan: Community Ecology Package. Available online at: http://CRAN.R-project.org/package=vegan (accessed May 1, 2022).
Orpin, C. G., Mathiesen, S. D., Greenwood, Y., and Blix, A. S. (1985). Seasonal changes in the ruminal microflora of the high-arctic Svalbard reindeer (Rangifer tarandus platyrhynchus). Appl. Environ. Microbiol. 50, 144–151. doi: 10.1128/aem.50.1.144-151.1985
Paillard, D., McKain, N., Rincon, M. T., Shingfield, K. J., Givens, D. I., and Wallace, R. J. (2007). Quantification of ruminal Clostridium proteoclasticum by real-time PCR using a molecular beacon approach. J. Appl. Microbiology 103, 1251–1261. doi: 10.1111/j.1365-2672.2007.03349.x
Patra, K. C., and Hay, N. (2014). The pentose phosphate pathway and cancer. Trends Biochem. Sci. 39, 347–354. doi: 10.1016/j.tibs.2014.06.005
Rakib, M. R. H., Zhou, M., Xu, S., Liu, Y., Asfandyar Khan, M., Han, B., et al. (2020). Effect of heat stress on udder health of dairy cows. J. Dairy Res. 87, 315–321. doi: 10.1017/S0022029920000886
Saika, A., Nagatake, T., and Kunisawa, J. (2019). Host- and microbe-dependent dietary lipid metabolism in the control of allergy, inflammation, and immunity. Front. Nutr. 6:36. doi: 10.3389/fnut.2019.00036
Shah, A. M., Ma, J., Wang, Z., Zou, H., Hu, R., and Peng, Q. (2020). Betaine supplementation improves the production performance, rumen fermentation, and antioxidant profile of dairy cows in heat stress. Animals (Basel) 10:634. doi: 10.3390/ani10040634
Stockwell, B. R., Friedmann Angeli, J. P., Bayir, H., Bush, A. I., Conrad, M., Dixon, S. J., et al. (2017). Ferroptosis: a regulated cell death nexus linking metabolism, redox biology, and disease. Cell 171, 273–285. doi: 10.1016/j.cell.2017.09.021
Sun, Y., Chen, P., Zhai, B., Zhang, M., Xiang, Y., Fang, J., et al. (2020). The emerging role of ferroptosis in inflammation. Biomed. Pharmacother. 127:110108. doi: 10.1016/j.biopha.2020.110108
Tang, D., Kang, R., Berghe, T. V., Vandenabeele, P., and Kroemer, G. (2019). The molecular machinery of regulated cell death. Cell Res. 29, 347–364. doi: 10.1038/s41422-019-0164-5
Tao, S., Monteiro, A. P. A., Thompson, I. M., Hayen, M. J., and Dahl, G. E. (2012). Effect of late-gestation maternal heat stress on growth and immune function of dairy calves. J. Dairy Sci. 95, 7128–7136. doi: 10.3168/jds.2012-5697
Wang, Q., Garrity, G. M., Tiedje, J. M., and Cole, J. R. (2007). Naïve bayesian classifier for rapid assignment of rRNA sequences into the new bacterial taxonomy. Appl. Environ. Microbiol. 73, 5261–5267. doi: 10.1128/AEM.00062-07
West, J. W. (2003). Effects of heat-stress on production in dairy cattle. J. Dairy Sci. 86, 2131–2144. doi: 10.3168/jds.S0022-0302(03)73803-X
Xu, C., Liu, W., Sun, B., Zhang, S., Zhang, S., Yang, Y., et al. (2021). Multi-omics analysis reveals a dependent relationship between rumen bacteria and diet of grass- and grain-fed yaks. Front. Microbiol. 12:642959. doi: 10.3389/fmicb.2021.642959
Xue, M. Y., Sun, H. Z., Wu, X. H., Guan, L. L., and Liu, J. X. (2019). Assessment of rumen bacteria in dairy cows with varied milk protein yield. J. Dairy Sci. 102, 5031–5041. doi: 10.3168/jds.2018-15974
Yangyu, L., Ranhui, X., Xin, Z., Jinzhi, H., and Xin, X. (2017). [Taste signal transduction and the role of taste receptors in the regulation of microbial infection]. Hua Xi Kou Qiang Yi Xue Za Zhi 35, 549–554. doi: 10.7518/hxkq.2017.05.020
Ye, G., Liu, J., Liu, Y., Chen, X., Liao, S. F., Huang, D., et al. (2016). Feeding glycerol-enriched yeast culture improves lactation performance, energy status, and hepatic gluconeogenic enzyme expression of dairy cows during the transition period1. J. Anim. Sci. 94, 2441–2450. doi: 10.2527/jas.2015-0021
Zeineldin, M., Aldridge, B., and Lowe, J. (2018a). Dysbiosis of the fecal microbiota in feedlot cattle with hemorrhagic diarrhea. Microb. Pathogen. 115, 123–130. doi: 10.1016/j.micpath.2017.12.059
Zeineldin, M., Barakat, R., Elolimy, A., Salem, A. Z. M., Elghandour, M. M. Y., and Monroy, J. C. (2018b). Synergetic action between the rumen microbiota and bovine health. Microb. Pathogen. 124, 106–115. doi: 10.1016/j.micpath.2018.08.038
Zened, A., Combes, S., Cauquil, L., Mariette, J., Klopp, C., Bouchez, O., et al. (2013). Microbial ecology of the rumen evaluated by 454 GS FLX pyrosequencing is affected by starch and oil supplementation of diets. FEMS Microbiol. Ecol. 83, 504–514. doi: 10.1111/1574-6941.12011
Zhang, G. W., Zeng, G., Iyakaremye, V., and You, Q. L. (2020). Regional changes in extreme heat events in China under stabilized 1.5 °C and 2.0 °C global warming. Adv. Clim. Change Res. 11, 198–209. doi: 10.1016/j.accre.2020.08.003
Zhang, Y., Choi, S. H., Nogoy, K. M., and Liang, S. (2021). Review: the development of the gastrointestinal tract microbiota and intervention in neonatal ruminants. Animal 15:100316. doi: 10.1016/j.animal.2021.100316
Zhao, G. Q., Zhang, Y., Hoon, M. A., Chandrashekar, J., Erlenbach, I., Ryba, N. J. P., et al. (2003). The receptors for mammalian sweet and umami taste. Cell 115, 255–266. doi: 10.1016/S0092-8674(03)00844-4
Zhao, S., Min, L., Zheng, N., and Wang, J. (2019). Effect of heat stress on bacterial composition and metabolism in the rumen of lactating dairy cows. Animals 9:925. doi: 10.3390/ani9110925
Zhu, W., Lomsadze, A., and Borodovsky, M. (2010). Ab initio gene identification in metagenomic sequences. Nucleic Acids Res. 38:e132. doi: 10.1093/nar/gkq275
Keywords: heat stress, dairy cows, Saccharomyces cerevisiae culture, metagenomics, metabolomics
Citation: Du D, Jiang W, Feng L, Zhang Y, Chen P, Wang C and Hu Z (2022) Effect of Saccharomyces cerevisiae culture mitigates heat stress-related dame in dairy cows by multi-omics. Front. Microbiol. 13:935004. doi: 10.3389/fmicb.2022.935004
Received: 03 May 2022; Accepted: 27 June 2022;
Published: 15 July 2022.
Edited by:
Xinqing Zhao, Shanghai Jiao Tong University, ChinaReviewed by:
Lifang Yan, Mississippi State University, United StatesYanliang Bi, Chinese Academy of Agricultural Sciences (CAAS), China
Yangchun Cao, Northwest A&F University, China
Copyright © 2022 Du, Jiang, Feng, Zhang, Chen, Wang and Hu. This is an open-access article distributed under the terms of the Creative Commons Attribution License (CC BY). The use, distribution or reproduction in other forums is permitted, provided the original author(s) and the copyright owner(s) are credited and that the original publication in this journal is cited, in accordance with accepted academic practice. No use, distribution or reproduction is permitted which does not comply with these terms.
*Correspondence: Chengqiang Wang, d2FuZ2NxQHNkYXUuZWR1LmNu; Zhiyong Hu, aHp5MjAwNDAxMTFAMTI2LmNvbQ==
†These authors have contributed equally to this work