- 1Department of Biology, College of Education (Majmaah), Majmaah University, Al-Majmaah, Saudi Arabia
- 2Department of Medical Biotechnology, College of Applied Medical Sciences, Qassim University, Buraydah, Saudi Arabia
- 3Immunology Unit, Pathology Department, College of Medicine, King Saud University, Riyadh, Saudi Arabia
Burkholderia cepacia is a Gram-negative nosocomial pathogen and is considered as a troublesome bacterium due to its resistance to many common antibiotics. There is no licensed vaccine available to prevent the pathogen infections, thus making the condition more alarming and warrant the search for novel therapeutic and prophylactic approaches. In order to identify protective antigens from pathogen proteome, substantial efforts are put forth to prioritized potential vaccine targets and antigens that can be easily evaluated experimentally. In this vaccine design investigation, it was found that B. cepacia completely sequenced proteomes available in NCBI genome database has a total of 28,966 core proteins. Out of total, 25,282 proteins were found redundant while 3,684 were non-redundant. Subcellular localization revealed that 18 proteins were extracellular, 31 were part of the outer membrane, 75 proteins were localized in the periplasm, and 23 were virulent proteins. Five proteins namely flagellar hook protein (FlgE), fimbria biogenesis outer membrane usher protein, Type IV pilus secretin (PilQ), cytochrome c4, flagellar hook basal body complex protein (FliE) were tested for positive for antigenic, non-toxic, and soluble epitopes during predication of B-cell derived T-cell epitopes. A vaccine peptide of 14 epitopes (joined together via GPGPG linkers) and cholera toxin B subunit (CTBS) adjuvant (joined to epitopes peptide via EAAAK linker) was constructed. Binding interaction of the modeled vaccine with MHC-I, MHC-II, and Toll-like receptor 4 (TLR-4) immune receptors was studied using molecular docking studies and further analyzed in molecular dynamics simulations that affirms strong intermolecular binding and stable dynamics. The maximum root mean square deviation (RMSD) score of complexes in the simulation time touches to 2 Å. Additionally, complexes binding free energies were determined that concluded robust interaction energies dominated by van der Waals. The total energy of each complex is < −190 kcal/mol. In summary, the designed vaccine showed promising protective immunity against B. cepacia and needs to be examined in experiments.
Introduction
The term “antibiotic resistance” refers to bacteria ability to withstand the action of antibiotics to which they had previously been susceptible (Tacconelli et al., 2018; Albekairi et al., 2022b). Bacteria have developed antibiotic resistance rapidly in the past 25 years, and the situation is very alarming now (Hasan and Al-Harmoosh, 2020). In addition, new resistant strains are surfacing faster and spreading more rapidly than ever before (Chokshi et al., 2019). During this process, new mutations progress in bacterial genomes and resistance genes are acquired through environmental exposure. The overuse and misuse of antibiotics is the main pressure that force bacterial to evolve and adapt its self to ever changing environmental conditions (Kulik et al., 2019; Albekairi et al., 2022a). The antibiotic resistance genes are disseminated primarily through extra chromosomal plasmids from one organism to another. The antimicrobial resistance is increasing to a frightening level, while on the other hand, new antibiotic development is very slow (MacLean and San Millan, 2019). Therefore, new therapeutic and prophylactic approaches are urgently required to address antibiotic resistance crisis.
Antibiotic resistant bacterial pathogen can be managed by developing safe vaccines (Jansen and Anderson, 2018; ud-din et al., 2022). Compared to antibiotics, vaccine resistance and associated allergic reactions are less. A vaccine is a biological preparation that induces protective adaptive immune responses against the pathogen antigen(s). Vaccines have a long success history in managing infectious organisms. The first smallpox vaccine was developed by Edward Jenner using the cowpox virus to provide protection against variola virus (Smith, 2011). There are many types of approaches that can be used to develop good vaccines. Louis Pasteur used the weekend form of the bacillus as a vaccine to treat anthrax (Opal, 2010). Sabin and Stalk developed a vaccine for polio using the Pasteur vaccine principles (Naz et al., 2015). The Bacillus Calmette Guerin (BCG) vaccine was developed against Mycobacterium tuberculosis (Bali et al., 2015). Despite of conventional vaccinology success, its application for unculturable pathogen and non-conserved antigens is limited. Fewer culture-based vaccines have been developed as a result. Traditional vaccine development is time-consuming and expensive (Rappuoli, 2001).
The Burkholderia cepacia complex (BCC) consists Gram-negative bacteria that produce catalase and do not ferment lactose (LiPuma, 2005). B. cepacia is a human pathogen that causes pneumonia most often in immunocompromised people with underlying lung diseases (such as cystic fibrosis) (Datta et al., 2020). The pathogen is described originally as a plant pathogen but become a major cause of infections especially hospitals. This pathogen is still poorly understood in terms of its virulence and ability to cause pathogenesis in humans (Goldmann and Klinger, 1986). It is intrinsically resistant to number of antibiotics such as aminoglycosides and colistin (Mahenthiralingam et al., 2005). Most patients who contract B. cepacia are merely colonized, but has the capacity to cause serious infections such as those caused by surgical and burn wounds, bacteremia, meningitis, pneumonia, peritonitis, and urinary tract infections. The organism can survive in a variety of solutions, disinfectants, medications, and even antiseptics (Rojas-Rojas et al., 2018). Moreover, no licensed vaccine is available in the market to prevent B. cepacian infections.
Recent advances in vaccine technology have led to computational reverse vaccinology, where antigenic surface proteins are determined from a genome dataset (Rappuoli, 2000; Suleman et al., 2021; Alharbi et al., 2022b). Vaccine for meningococcal serogroup B (4CMenB) was developed using reverse vaccinology (Serruto et al., 2012). Considering the importance of reverse vaccinology in identifying potential vaccine targets in pathogens genomic data, the method was used herein to highlight protein targets and antigenic epitopes that can be prioritized against the pathogen to assist experimentalists in vaccine development. Moreover, a multi-epitopes vaccine construct is designed to overcome limitations of simple peptide vaccine. Further, biophysics approaches are utilized to show binding and dynamics of the vaccine with immune receptors.
Methodology
The different steps used for vaccine construct design are highlighted in Figure 1.
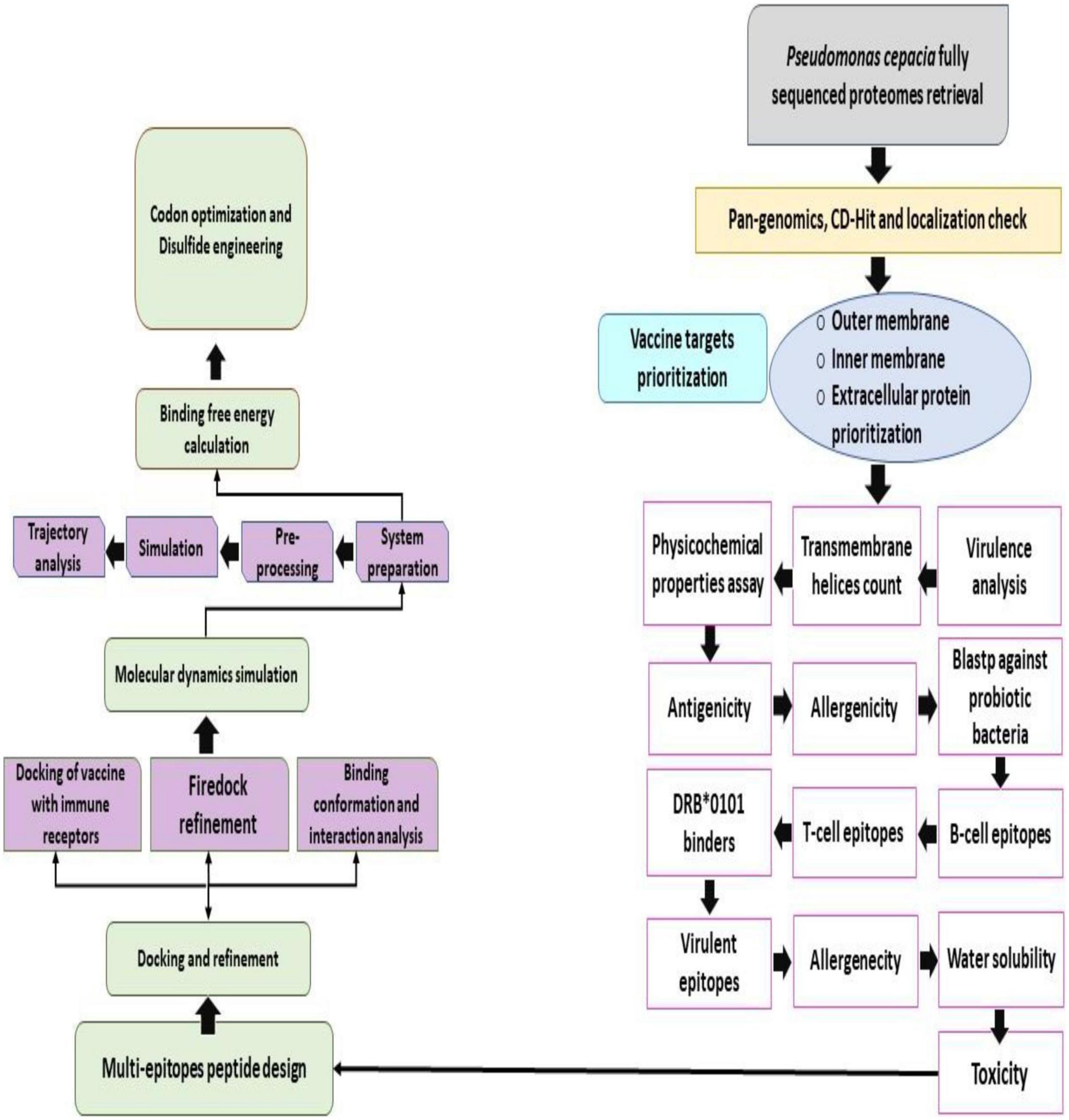
Figure 1. Work flow of the steps done in the study to design a multi-epitopes vaccine against B. cepacian.
Pan-Genomics and Subcellular Localization
The NCBI database was used to collect proteomic data of B. cepacian (Coordinators, 2017). Pan-proteome analysis was performed on the proteomes to determine pathogen core sequences. In order to perform the pan-proteome analysis, we used the Bacterial Pan Genome Analysis tool (BPGA) (Chaudhari et al., 2016). A CD-Hit clustering process was used to remove redundant proteins from the core sequence obtained through BPGA (Fu et al., 2012). We set the threshold at 0.5 and as such 50% similar sequences were discarded. PSORTb 3.0 subcellular localization prediction tool was used to predict the subcellular localization of selected non-redundant proteins (Yu et al., 2010). Only extracellular, outer membrane and periplasmic proteins were opted for further downward analysis. These proteins are exposed to the host immune systems, and have antigenic sequences capable of stimulating strong immune responses (Ahmad et al., 2019).
Selection of Vaccine Candidates
Afterward, several filters were applied on the selected outer membrane, extracellular and periplasmic proteins to perform selection of potential vaccine targets. Proteins that play a significant role in pathogenesis are prime targets for vaccine design (Ahmad et al., 2019; Alharbi et al., 2022a). A BLASTp search was conducted against the core virulent factor database (VFDB) (Liu et al., 2019) using output proteins from the subcellular localization step, and those with sequence identities of ≥ 30% and bit scores ≥ 100 were selected (Qamar et al., 2021). Afterward, the ProtParam tool was used to determine the physico-chemical properties of selected virulent proteins (ProtParam, 2017). It has been suggested that proteins having a molecular weight of ≤ 110 kDa serve as ideal candidates for vaccine development as they can be easily purified (Ismail et al., 2020). HMMTOP 2.0 (Tusnady and Simon, 2001) was used to predict the transmembrane helices of the proteins. The selection was made if a protein has 1or 0 transmembrane helices (Rizwan et al., 2017). As a next step, shortlisted proteins were BLASTp against a reference human proteome with the “taxonomic ID: 9606” and those with a sequence identity ≤ 30% were selected (Naz et al., 2015). All homologous proteins were discarded in order to avoid autoimmune reactions. A threshold of 0.4 was used by VaxiJen 2.0 to determine the antigenicity of proteins (Ong et al., 2021). The antigenic proteins are good vaccine targets because they are antibodies generator. After selecting antigenic proteins, it was necessary to determine whether they had adhesive properties because proteins that possess adhesive properties can trigger pathogenic pathways. A SPAAN server was used to test the adhesive properties of proteins (Sachdeva et al., 2005). AllerTOP2.0 was applied further to evaluate allergic proteins among the adhesive proteins (Dimitrov et al., 2013). The homology of non-allergic proteins to Lactobacillus rhamnosus, L. casei, and L. johnsonii was examined by BLASTp (Ahmad et al., 2019).
B-Cell and T-Cell Epitopes Prediction
Bepipred Linear Epitope Prediction 2.0 of Immune Epitope Database (IEDB) was used to predict linear B-cell epitopes at threshold of 0.5 (Vita et al., 2015; Jespersen et al., 2017). The resultant B-cell epitopes were utilized to predict common peptides showing strong binding to class I and class II alleles of the major histocompatibility complex (MHC) (Dhanda et al., 2019). This was one using IEDB T-cell epitopes prediction tools. Those with minimum percentile score were regarded as strong binders and subjected to MHCPred 2.0 analysis (Guan et al., 2003). In this anlaysis, only those epitopes with an IC50 value of ≤ 100 nM were selected as they show strong binding to the highly prevalent DRB*0101 allele. VaxiJen (Doytchinova and Flower, 2007), AllerTOP 2.0 (Dimitrov et al., 2013), and VirulentPred (Garg and Gupta, 2008) were again used to validate predicted epitopes antigenicity, allergenicity, and virulence, respectively. Innovagen, which is a peptide solubility calculator, was used to determine the solubility of peptides. Peptides that show good solubility in water were selected for further investigation. Using ToxinPred (Gupta et al., 2013), non-toxic epitopes were selected (Barh et al., 2013).
Chimeric Vaccine Design
The shortlisted epitopes were then used chimeric vaccine design. As single peptide vaccine has weak immunogenicity, this problem was overcome by fusing all epitopes with GPGPG linkers to stimulate strong immune responses that can effectively combat the pathogen (Malonis et al., 2019). A cholera toxin B (CTB) adjuvant was additionally added to epitopes peptide using EAAAK linker. SCRATCH protein predictor (Cheng et al., 2005) was used to predict the tertiary structure of the chimeric vaccine. The refinement of the structure was performed by Galaxy web refine tool (Heo et al., 2013).
Blind Docking Analysis
Blind docking between designed chimeric vaccine and immune cell receptors was PatchDock server (Schneidman-Duhovny et al., 2005), The TLR-4, MHC-I, or MHC-II pdb structures were retrieved from protein data bank (PDB) using 4 digit code of 4G8A, 1I1Y, and 1KG0, respectively. FireDock server was used for refinement of docked complexes generated through PatchDock (Mashiach et al., 2008). In each case, top complex that showed lowest global energy was considered and evaluated further. The binding between vaccine and receptors was examined using UCSF Chimera 1.13.1 (Pettersen et al., 2004).
Molecular Dynamics Simulation Assay
Molecular dynamics simulation was used to analyze the stability and dynamics of docked complexes (Karplus, 2002). AMBER20 was used for this purpose (Case et al., 2020). The systems were first subjected to energy minimization, followed by heating, equilibrium and production (Ahmad et al., 2019). The production run was conducted for 100 ns. The systems preparation was done using antechamber program (Wang et al., 2001). FF14SB force field was employed to parameterize the complexes (both receptors and vaccine molecules) (Case et al., 2014). In energy minimization, steepest descent and conjugate gradient algorithms were used. In temperature, the systems temperature was gradually increased to 310 K. During production, SHAKE algorithm was used to apply constrain on hydrogen bonds while Langevin algorithm was used to maintain temperature (Izaguirre et al., 2001; Kräutler et al., 2001). CPPTRAJ module of the AMBER was used to measure systems intermolecular docked stability (Roe and Cheatham, 2013). The XMGRACE was considered for plotting root mean square deviation (RMSD) and root mean square fluctuation (RMSF) graphs (Maiorov and Crippen, 1994; Turner, 2005; Qamar et al., 2021).
Estimation of Binding Free Energy
In order to calculate the solvation and gas free energies associated with the interactions of the vaccine with all three receptors, the MMPBSA.py module was employed (Miller et al., 2012). A total of 100 frames were picked from molecular dynamics simulation trajectories and used in calculation of binding free energy.
Codon Adaptation and Cloning
In order to achieve high expression of the vaccine, the vaccine sequence was converted into DNA sequences and then codon usage was adapted to the E. coli K-12 strain to attain high vaccine expression JCAT (Java Codon Adaptation Tool) server was used for this process (Grote et al., 2005). GC-content and codon adaptation index (CAI) was used to determine the expression level of the vaccine.
Disulfide Engineering
Introducing disulfide bonds to the vaccine structure was done to make the vaccine structure stabile. This also protect the vaccine enzyme degradable prone regions (Dombkowski et al., 2014). Disulfide engineering was carried out by Design 2.0 (Craig and Dombkowski, 2013). Only high energy residue pairs (> 0 kcal/mol) were mutated to cysteine.
Results
Retrieval of Burkholderia cepacia Proteomes
A total of 7 complete sequenced proteomes of B. cepacia were obtained from NCBI genome database. Only fully sequenced proteomes were considered to achieve consistency in the results. Information regarding each strain genome size and GC contents were also accessed as tabulated in Table 1.
Bacterial Pan-Genomics
Using BPGA, the core sequences shared by all the strains were extracted from the complete proteomes. Along with that, BPGA enabled the separation of strain-specific proteins as well as dispensable proteins. As core proteins are present in all of the sequenced strains, they are involved in core function of the pathogen and thus vital for pathogen survival, growth and functionality (Chaudhari et al., 2016). Considering this significance role of core proteins in the pathogen biology, they are termed as attractive vaccine targets. The core pan plot illustrating the number of gene families in each strain of the pathogen is given in Figure 2.
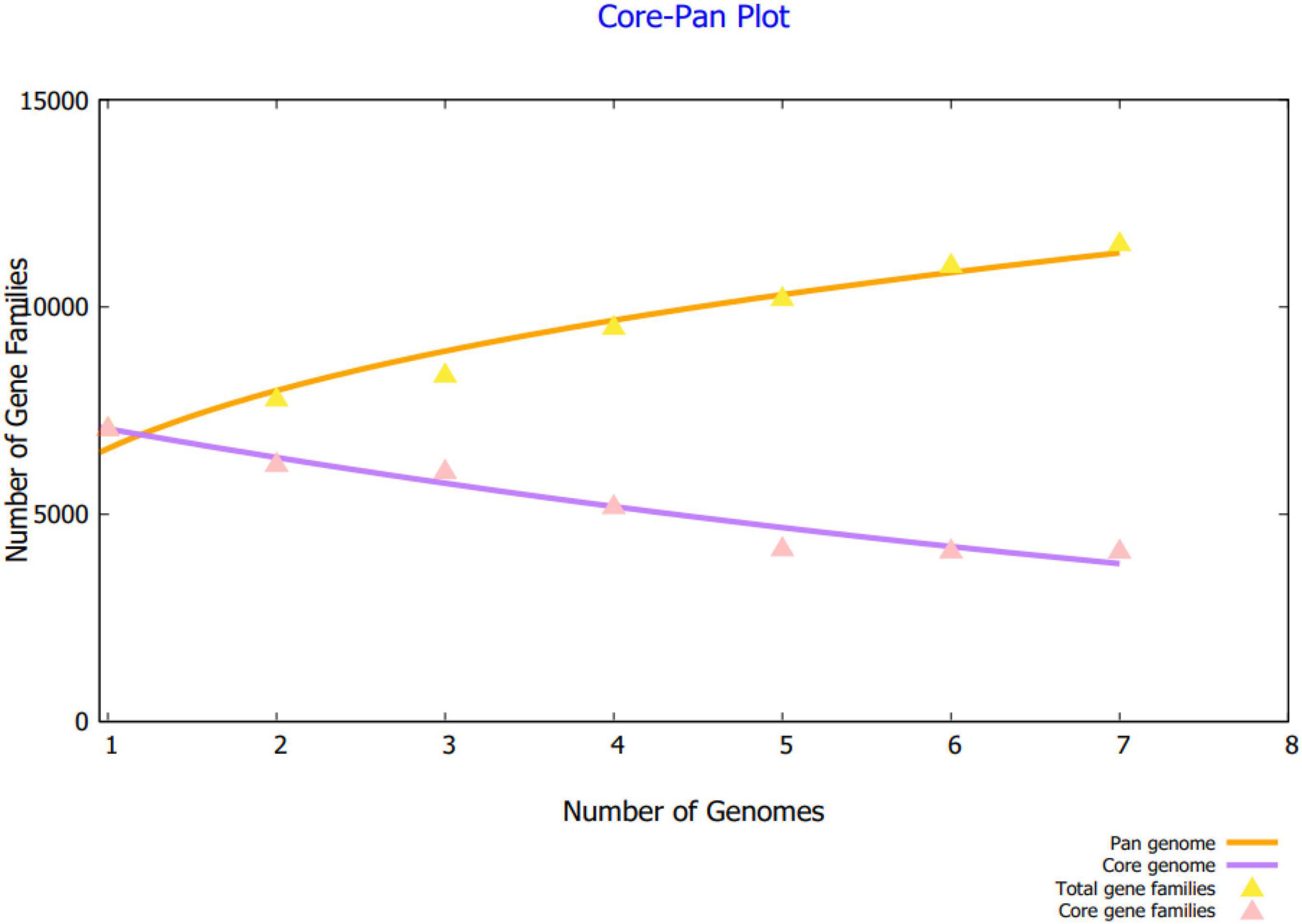
Figure 2. The plot of the core pan of seven B. cepacia strains. The number of gene families in respective pathogen strain is also provided.
CD-HIT Analysis
A CD-HIT analysis was used to separate redundant and non-redundant proteins in the core proteome. There were 28,966 total proteins in proteomic data. Approximately, 3,684 proteins were found non-redundant and 25,282 to be redundant as shown in Figure 3A. The redundant proteins are the products of gene duplication event and are present in more than one copy in the genomes/proteome. Thus, considering them in afterward analysis is less scientifically sound. On the other hand, non-redundant proteins are promising vaccine targets due to their prime role in pathogen essential pathways and functions (Sanober et al., 2017).
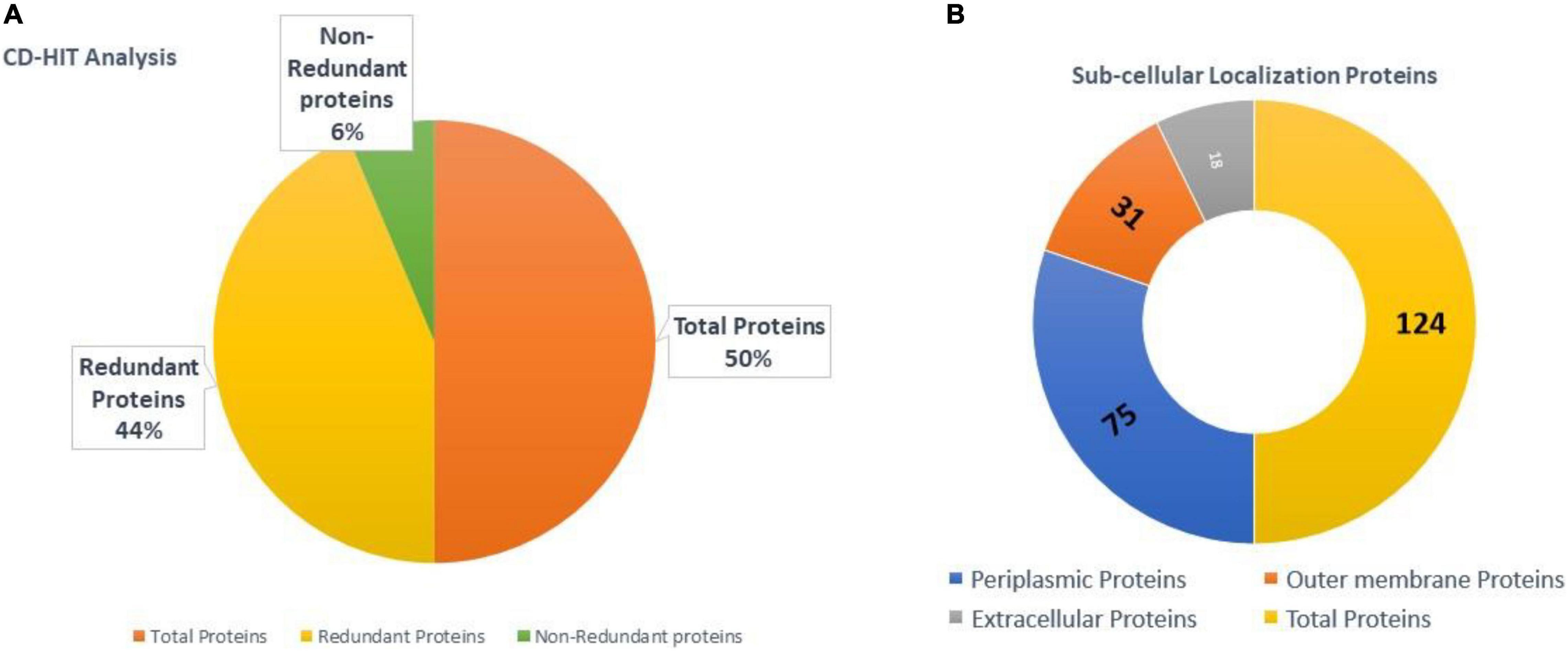
Figure 3. (A) Total, non-redundant proteins, and redundant proteins of B. cepacia. (B) Categorization of surface proteins obtained.
Subcellular Localization Analysis
A subcellular localization strategy was adopted in order to predict proteins part of the pathogen exoproteome and secretome. The surface exposed proteins play a vital role in pathogen ability to invade, adhere to, and multiply within host cells. Additionally, these proteins are disclosed to contain multiple antigenic regions capable of eliciting strong immune responses (Barh et al., 2013). Most proteins were located in cytoplasm, 18 were extracellular, 31 were outer membrane, 75 were in periplasm, and some were unknown. In total, 124 were surface-localized proteins as illustrated in Figure 3B.
Selection of Good Vaccine Targets
As part of the analysis, the virulence factor database (VFDB) was used to identify virulent proteins of B. cepacia. Virulent proteins trigger stronger immune responses and critical disease pathway initiation, making them good targets for vaccine development (Naz et al., 2015). The study found total of 19 virulent proteins as presented in Table 2. Further, estimation of transmembrane helices in the proteins revealed all 19 proteins to have either 0 or 1 transmembrane helices, which to its easy ability to be easily purified in experimental analysis. All of the proteins also unveiled to have less molecule weight than the threshold and thus are considered to be good targets because of their easy cloning and expression (Ahmad and Azam, 2018). The proteins are also non-allergic and adhesive in nature.
By using ProtParm tool, physico-chemical properties were evaluated for selected vaccine proteins. This analysis is helpful to investigate the vaccine targets experimentally. Only, 16 proteins were selected in this phase as they fulfill all the filters of physico-chemical stage. Different parameters such as aliphatic index, GRAVY, composition of amino acids, and instability index were estimated as given in Table 3. All proteins scored negatively on the GRAVY index, indicating their hydrophilic nature. The proteins were also found thermally stable and have accepted PI value.
Probiotics bacteria aid to inhibit the growth and survival of microbes that are harmful to the body. Vitamins such as biotin and vitamin K2 are produced by these organisms. In order to avoid accidental inhibition of probiotic bacteria, filtered proteins were used in BLASTp search was performed against multiple probiotic bacteria. By doing so, only 5 proteins were selected for further study and remaining proteins were discarded. The proteins also revealed non-homology to the human host and they are likely not to generate any autoimmune responses (Table 4).
Epitopes Prediction
In order to select B-cell epitopes of score greater than the cut-off, the proteins were first subjected to B-cell epitopes prediction. The predicted epitopes are shown in Table 5. Further analysis of B-cell epitopes was done to predict MHC-I and MHC-II epitopes. For prediction of MHC epitopes, reference alleles were used in T-cell prediction. T-cell epitopes with low percentile rank were considered good binders to MHC alleles. The MHC-Pred server was used further to determine which epitopes showed IC50 values for the DRB*0101 gene. This DRB belongs to the human leukocyte antigen II family and is widely distributed throughout the world. Hence, epitopes showing good binding to DRB*0101 can produce strong and specific immune responses (Naz et al., 2019). All predicted epitopes were also non-allergenic, antigenic, water soluble and non-toxic. The screened 14 soluble and non-toxic epitopes were used in the design of a multi-epitopes as shown in Figure 4.
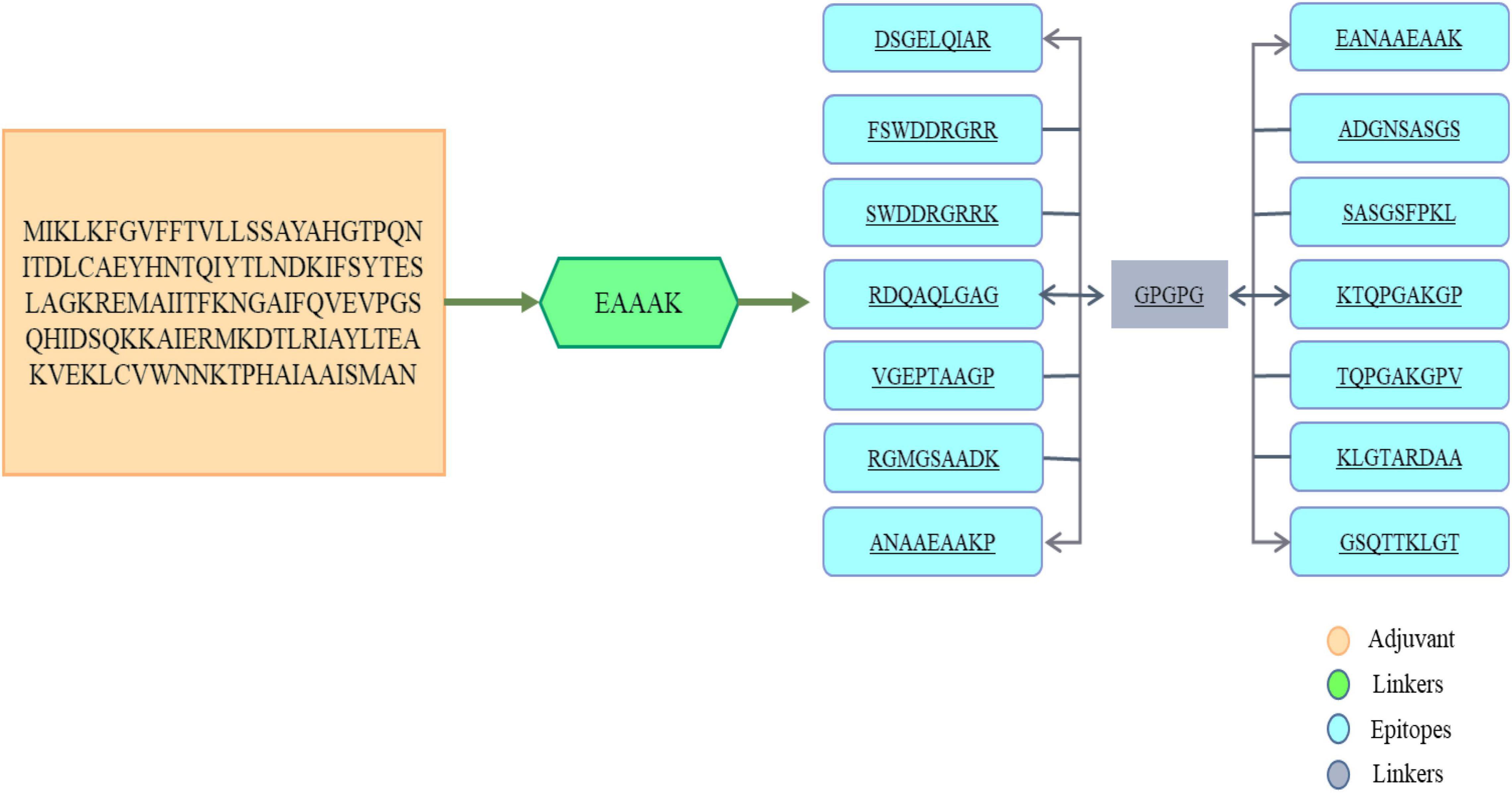
Figure 4. The primary amino acids sequence of vaccine. To link the antigenic B-cell derived T-cell epitopes, GPGPG linkers were used as shown in gray block. At the amino terminal of the multi-epitope’s peptide, an adjuvant (cholera toxin B subunit) was attached shown in orange box while the EAAAK linker is shown in green color box.
Chimeric Vaccine Construct
Peptide vaccines are poor immunogenic and therefore the predicted epitopes were joined to design a multi-epitope vaccine construct (Reche et al., 2014). The immunodominant epitopes were linked using GPGPG linkers and the resultant peptide is joined to an adjuvant cholera toxin B subunit via EAAAK linker. The said linkers are rigid in nature and keep the epitopes and adjuvant molecule separated and stop epitopes folder over each other (Ismail et al., 2020). This physical separation of epitopes allows the host immune simulation system to easily recognize and process the epitopes. The cholera toxin B subunit is safe and has stronger affinity for monosialotetrahexosylganglioside present on variety of antigen presenting cells, B-cells, and macrophages and thus facilitates the antigenic peptide access to the immune system (Baldauf et al., 2015). The multi-epitopes vaccine is thus producing more rigorous immune responses that are specific and accurate.
3D Vaccine Structure and Refinement
Figure 5 shows the tertiary structure of the chimeric vaccine that was modeled by ab initio method as no appropriate template was present to be the vaccine structure on homology principle. The vaccine was then subjected to loop modeling where loop regions were modeled into secondary structure elements. The loop model structure was then refined for structure errors and the most refined model was selected (Heo et al., 2013). Model 1 was opted due to its good galaxy energy of -4200.50, absence of poor rotamers, low clash score, and highest percentage of residues in Rama favored.
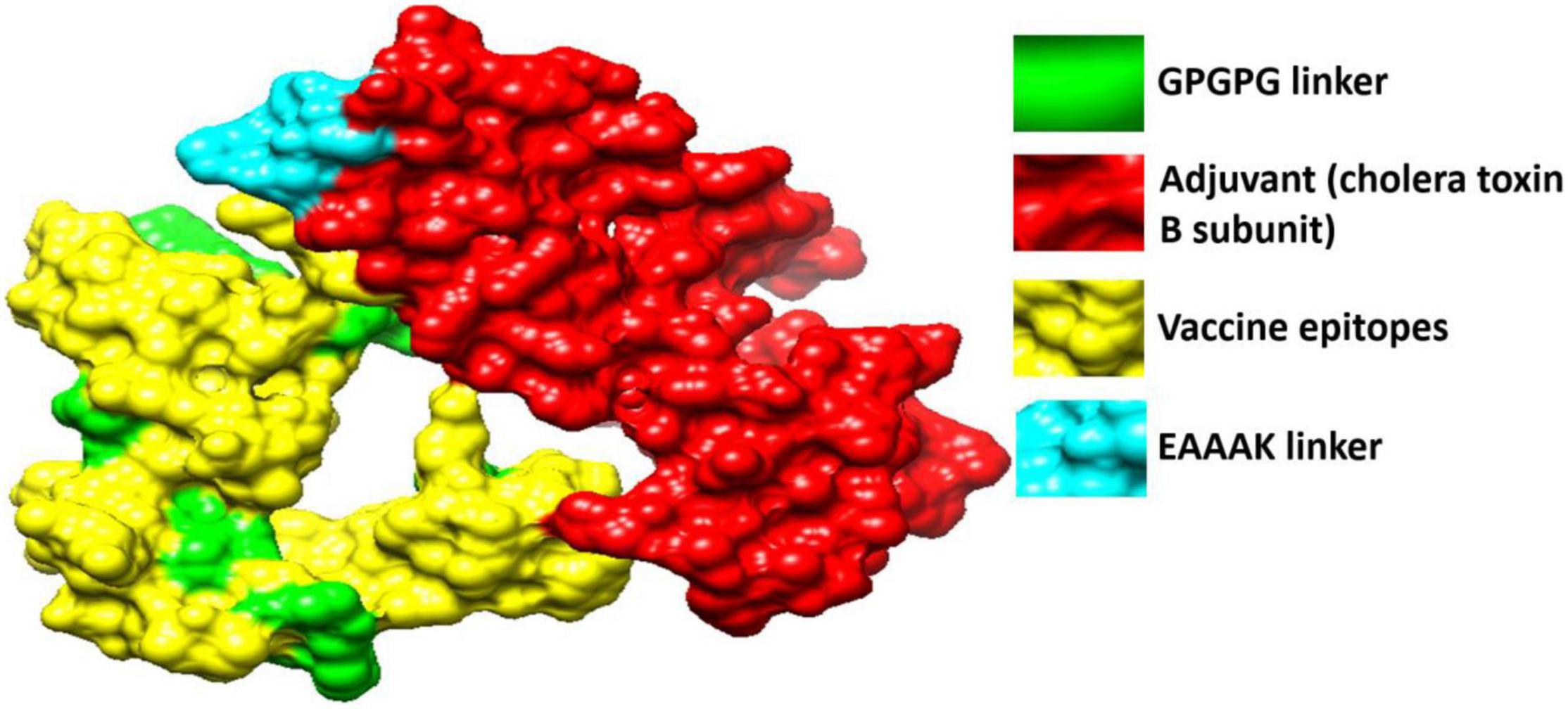
Figure 5. 3D structure of the designed vaccine. The red color represents the adjuvant (cholera toxin B subunit), yellow color is for vaccine epitopes, cyan blue color denotes EAAAK while the green color is for GPGPG linkers.
Molecular Docking
In case of MHC-I, solution 10 was regarded as the best representative intermolecular binding conformation as it has the lowest global binding energy (-12.94 kJ mol–1) which can be split into attractive van der Waals (-34.63 kJ mol–1), atomic contact energy (4.12 kJ mol–1), and hydrogen bond energy (-2.10 kJ mol–1). Solution 8 from MHC-II and solution 5 from TLR-4 has total global binding of -5.21 kJ mol–1 and -7 kJ mol–1, respectively. The FireDock refinement results for MHC-I and MHC-II, as well as TLR-4 are summarized in Supplementary Tables 1–3, respectively. The docked vaccine conformations with the different immune receptors used are given in Figure 6.
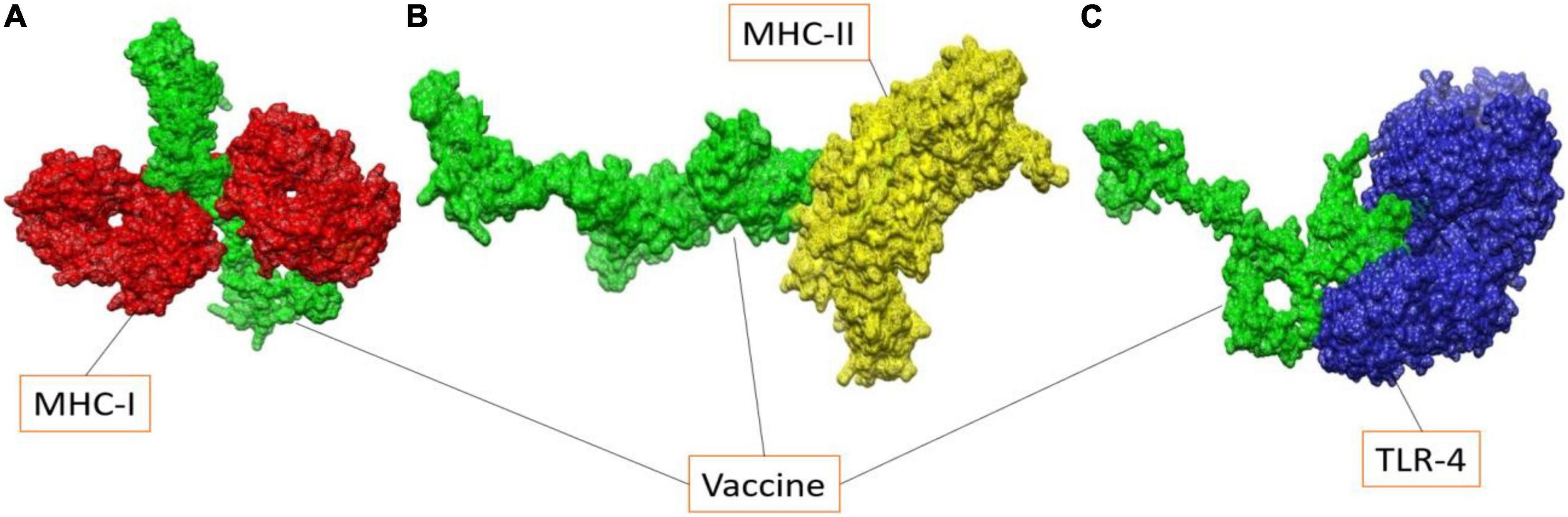
Figure 6. Illustration of docked vaccine to MHC-I molecule (A), MHC-II molecule (B) and TLR-4 molecule (C).
Proper immune response depends on the interaction between vaccines and host immune cells. Using the protein-peptide docking approach, these interactions between vaccine constructs and MHC-I were determined, and specific residue-wise interactions of MHC-I, MHC-II and TLR-4 were found in the UCSF chimera as shown in Table 6.
Molecular Dynamic Simulation
The dynamics of complexes were investigated in order to validate intermolecular conformational stability and interactions. The structure stability was determined through root mean square deviation (RMSD), which is used to investigate global structure changes in the superimposed snapshots (Figure 7A). It was found that the complexes are quite stable along the simulation time and no major structure deviations were noticed. The RMSD of the systems are within 3 angstrom range.
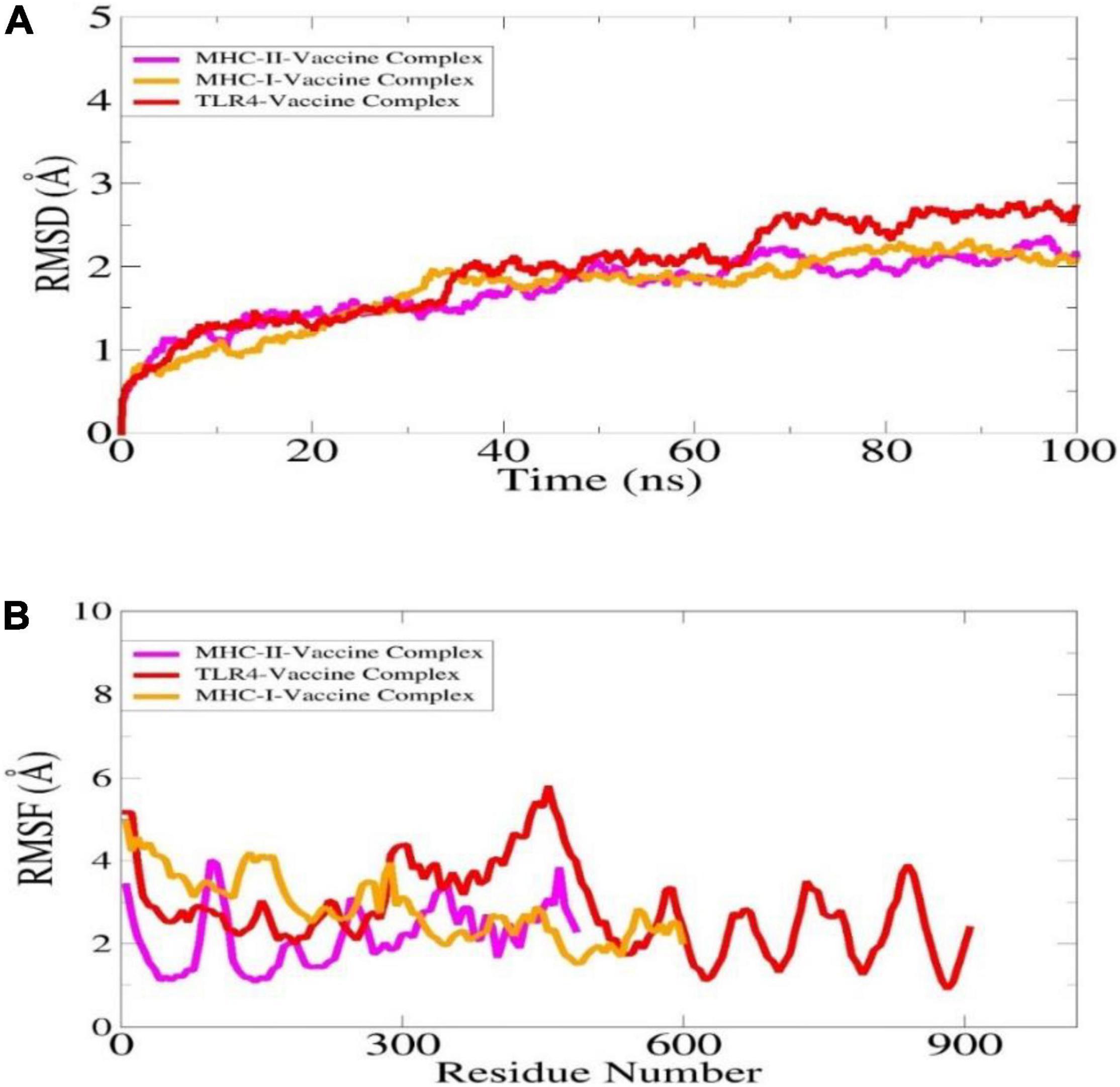
Figure 7. Statistical analysis of MD simulation trajectories is illustrated here: RMSD (A), RMSF (B).
Additionally, the complexes stability was evaluated through root mean square fluctuation (RMSF) (Figure 7B). Both ends of the complexes were found flexible while the complexes overall remained stable. The high flexibility of the ends is due to flexible loops which confer structure instability to the systems however, it does not affect overall vaccine binding to the receptors. The affirm that the vaccine binding to the immune receptors is strong, and the epitopes are exposed which further ensured that the epitopes might be easily recognized and processed by the host immune system cells. This further interprets the vaccine epitopes may generate stronger immune reactions.
Estimation of Binding Free Energies
Binding free energies calculation of the docked complexes were calculated using MM/PBSA and MM/GBSA approaches. In MM-GBSA the total binding free energies of TLR-4-vaccine complex, MHC-I-vaccine complex, and MHC-II-vaccine complex for MM-GBSA were -301 kcal/mol, -250 kcal/mol, and -197 kcal/mol, respectively as given in Table 7. Similarly in MM-PBSA, it has been found that TLR-4-vaccine complex has net binding free energy of -294.64 kcal/mol while MHC-I-vaccine has -245.86 kcal/mol and MHC-II-vaccine complex net binding energy of -192.99 kcal/mol. It was further revealed that both van der Waals and electrostatic energies are favorable in complexes formation.
Codon Optimization
During this process, the sequence of the model vaccine construct was first reverse translated into DNA sequence by using Java Codon Adaptation Tool (JCat) to calculate the codon adaptation index (CAI) and GC percentage rates of the cloned vaccine construct as the maximum level of expression in the E. coli expression system. The GC contents of the designed vaccine is 58.2% and CAI value is 1, which is considered ideal for high expression.
Disulfide Engineering
Disulfide engineering is a useful approach to mutate vaccine residues that are unstable from energy perspective and are pronce to host enzymatic degredation. It also enhance vaccine folded conformation stability by achieving low entropy (Dombkowski et al., 2014). Cysteine residues were added replacing unstable residues. The residues that were mutated have high unfavorable binding energy. The mutated residues can be seen in Figure 8 as yellow sticks. The following 9 residues pair: Ser16-Ala19, Ala17-Asn36, Ala31-His34, Lys44-Val71, Lys55-Gln70, Met58-Ala67, Gly211-Ala214, Ala217-Pro224, and Lys293-Asp229 were mutated to cystein in disulfide engineering.
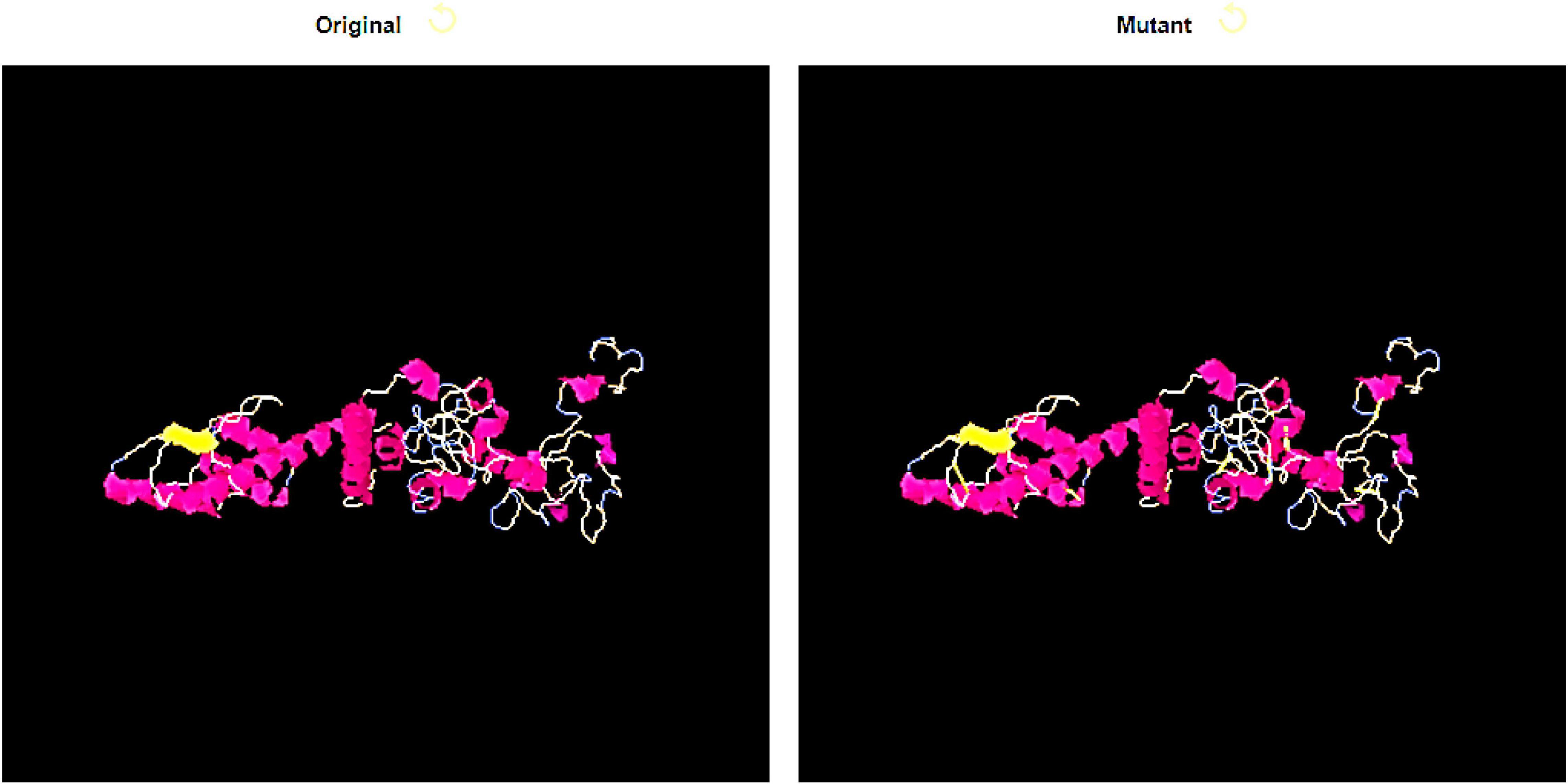
Figure 8. Disulfide engineering of the designed vaccine molecule. Both wild (left) and mutated structure (right) is shown. The yellow sticks in mutated structure are residues replaced with cysteine.
Discussion
Antibiotic resistance is a natural mechanism by which bacteria make itself adaptable to the changing environmental conditions. The bacterial resistance to antibiotics has reached to a very dangerous level and strains resistance to several and all commonly used antibiotics are now routinely reported across the globe (Hutchings et al., 2019). This not only length human mortality rate but also increase disease burden and pressured health care systems (Tacconelli et al., 2017; Hutchings et al., 2019). B. cepacia, which is a Gram-negative nosocomial human pathogen is responsible for several diseases in particular pneumonia most often in people who have immunodeficiencies or lung diseases (like cystic fibrosis) (Mahenthiralingam et al., 2005). The pathogen has become one of the most common causes of infections in hospitals. The bacteria resist many antibiotics such as cephalosporins, aminoglycosides, fluroquinolones, and trimoxazole. So far, no efficacious vaccine is present to prevent B. cepacian infections (Devanga Ragupathi and Veeraraghavan, 2019). None of the vaccine candidate evaluated experimentally has shown sterilizing immunity.
The introduction of fast sequencing technologies and as a result genomic data is added to databases enabled researchers to discover new vaccine candidates that were harder to detect in traditional vaccine development (Moriel et al., 2008; Naz et al., 2021). The identification of putative surface-associated antigens can be done using advanced bioinformatic approaches (Yero et al., 2021). It has been shown that reverse vaccinology is a safe, effective, and reliable method for identifying surface-associated proteins from the pathogen genome without the need to cultivate the microbes (Yero et al., 2021). An effective meningococcal vaccine has been developed using the reverse vaccinology method (Abdullah et al., 2021). A number of studies have indicated that pan-genomic reverse vaccinology (PGRV) is more effective compared to conventional reverse vaccinology because it screens highly conserved targets (Fatima et al., 2021; Ullah et al., 2021; Gul et al., 2022). Therefore, pan-genomics studies were integrated with reverse vaccinology and immunoinformatic to map potential B and T-cell epitopes in B. cepacian genome.
In contrast to simple peptide based vaccine, a multi-epitopes vaccine against antibiotic resistant B. cepacia is designed herein. Good vaccine targets were prioritized using several properties such as antigenicity, immunogenicity, non-homology to human host and probiotic bacteria, non-allergenicity, and localization on the pathogen surface. The properties listed above are based on literature and highly desirable for designing a chimeric vaccine (Qamar et al., 2020; Ismail et al., 2021). Further, immunoinformatic approaches were used for predicting B and T-cell epitopes and only safe and powerful antigens were shortlisted to be used in a chimeric vaccine design. The vaccine construct designed in this study comprises five proteins flagellar hook protein (FlgE), fimbria biogenesis outer membrane usher protein, Type IV pilus secretin (PilQ), cytochrome c4, flagellar hook basal body complex protein (FliE). A total of 14 epitopes have been predicted from selected proteins that share common sequences with ability to stimulate stronger immune responses. In addition to allergenicity and solubility analysis, we also examined toxicity and allergenicity of the epitopes. Cholera toxin B subunit adjuvant was further combined with predicted B-cell derived from T-cells epitopes peptide.
The binding ability of the designed multi-epitopes vaccine with different immune receptors was further examined using biophysics approaches. The findings reported that the designed vaccine showed robust interactions with the receptors and are engaged by several intermolecular interactions. The stable intermolecular dynamics validated the efficient representation of epitopes to the immune cells for processing of immune signaling pathways.
Conclusion
The study investigates the development of a multi-epitopes vaccine against B. cepacia. Several computer aided vaccine approaches are being explored, such as reverse vaccination, subtractive proteomics, immunoinformatic, and biophysical analysis. We predicted several vaccine targets; flagellar hook protein (FlgE), fimbria biogenesis outer membrane usher protein, type IV pilus secretin (PilQ), cytochrome c4, flagellar hook basal body complex protein (FliE). Total of 14 soluble and non-toxic epitopes were predicted from the above proteins and were used in the design of a multi-epitopes vaccine. The designed vaccine showed robust interactions with the host immune receptors and found to show stable conformation in simulation time. Thus, it can be concluded that the predicted epitopes and designed vaccine construct might provide protection against the pathogen.
Data Availability Statement
The original contributions presented in this study are included in the article/Supplementary Material, further inquiries can be directed to the corresponding author/s.
Author Contributions
NA and AA contributed to conception and design of the study. SA-S collected the data. NA performed the analysis. AA wrote the first draft of the manuscript. NA and SA-S wrote sections of the manuscript. All authors contributed to manuscript revision, read, and approved the submitted version.
Conflict of Interest
The authors declare that the research was conducted in the absence of any commercial or financial relationships that could be construed as a potential conflict of interest.
Publisher’s Note
All claims expressed in this article are solely those of the authors and do not necessarily represent those of their affiliated organizations, or those of the publisher, the editors and the reviewers. Any product that may be evaluated in this article, or claim that may be made by its manufacturer, is not guaranteed or endorsed by the publisher.
Acknowledgments
We would like to thank Deanship of Scientific Research at Majmaah University, Kingdom of Saudi Arabia, for supporting this work under Project Number: (R-2022-191).
Supplementary Material
The Supplementary Material for this article can be found online at: https://www.frontiersin.org/articles/10.3389/fmicb.2022.929400/full#supplementary-material
References
Abdullah, M., Kadivella, M., Sharma, R., Faisal, S. M., and Azam, S. (2021). Designing of multiepitope-based vaccine against Leptospirosis using Immuno-Informatics approaches. bioRxiv [Preprint]. doi: 10.1101/2021.02.22.431920
Ahmad, S., and Azam, S. S. (2018). A novel approach of virulome based reverse vaccinology for exploring and validating peptide-based vaccine candidates against the most troublesome nosocomial pathogen: Acinetobacter baumannii. J. Mol. Graph. Model. 83, 1–11. doi: 10.1016/j.jmgm.2018.04.020
Ahmad, S., Ranaghan, K. E., and Azam, S. S. (2019). Combating tigecycline resistant Acinetobacter baumannii: a leap forward towards multi-epitope based vaccine discovery. Eur. J. Pharm. Sci. 132, 1–17.
Albekairi, T. H., Alshammari, A., Alharbi, M., Alshammary, A. F., Tahir ul Qamar, M., Ullah, A., et al. (2022b). Designing of a Novel Multi-Antigenic Epitope-Based Vaccine against E. hormaechei: an Intergraded Reverse Vaccinology and Immunoinformatics Approach. Vaccines 10:665.
Albekairi, T. H., Alshammari, A., Alharbi, M., Alshammary, A. F., Tahir ul Qamar, M., Anwar, T., et al. (2022a). Design of a Multi-Epitope Vaccine against Tropheryma whipplei Using Immunoinformatics and Molecular Dynamics Simulation Techniques. Vaccines 10:691.
Alharbi, M., Alshammari, A., Alasmari, A. F., Alharbi, S., Tahir ul Qamar, M., Abbasi, S. W., et al. (2022b). Whole Proteome-Based Therapeutic Targets Annotation and Designing of Multi-Epitope-Based Vaccines against the Gram-Negative XDR-Alcaligenes faecalis Bacterium. Vaccines 10:462. doi: 10.3390/vaccines10030462
Alharbi, M., Alshammari, A., Alasmari, A. F., Alharbi, S. M., Tahir ul Qamar, M., Ullah, A., et al. (2022a). Designing of a Recombinant Multi-Epitopes Based Vaccine against Enterococcus mundtii Using Bioinformatics and Immunoinformatics Approaches. Int. J. Environ. Res. Public Health 19:3729. doi: 10.3390/ijerph19063729
Baldauf, K. J., Royal, J. M., Hamorsky, K. T., and Matoba, N. (2015). Cholera toxin B: one subunit with many pharmaceutical applications. Toxins 7, 974–996. doi: 10.3390/toxins7030974
Bali, P., Tousif, S., Das, G., and Van Kaer, L. (2015). Strategies to improve BCG vaccine efficacy. Immunotherapy 7, 945–948. doi: 10.2217/imt.15.60
Barh, D., Barve, N., Gupta, K., Chandra, S., Jain, N., Tiwari, S., et al. (2013). Exoproteome and secretome derived broad spectrum novel drug and vaccine candidates in Vibrio cholerae targeted by Piper betel derived compounds. PLoS One 8:e52773. doi: 10.1371/journal.pone.0052773
Case, D. A., Babin, V., Berryman, J. T., Betz, R. M., Cai, Q., Cerutti, D. S., et al. (2014). The FF14SB force field. Amber 14, 29–31.
Case, D. A., Belfon, K., Ben-Shalom, I., Brozell, S. R., Cerutti, D., Cheatham, T., et al. (2020). Amber 2020. Available online at: https://ambermd.org/doc12/Amber20.pdf
Chaudhari, N. M., Gupta, V. K., and Dutta, C. (2016). BPGA-an ultra-fast pan-genome analysis pipeline. Sci. Rep. 6:24373. doi: 10.1038/srep24373
Cheng, J., Randall, A. Z., Sweredoski, M. J., and Baldi, P. (2005). SCRATCH: a protein structure and structural feature prediction server. Nucleic Acids Res. 33, W72–W76. doi: 10.1093/nar/gki396
Chokshi, A., Sifri, Z., Cennimo, D., and Horng, H. (2019). Global contributors to antibiotic resistance. J. Glob. Infect. Dis. 11, 36–42.
Coordinators, N. R. (2017). Database resources of the national center for biotechnology information. Nucleic Acids Res. 45:D12.
Craig, D. B., and Dombkowski, A. A. (2013). Disulfide by Design 2.0: a web-based tool for disulfide engineering in proteins. BMC Bioinform. 14:346. doi: 10.1186/1471-2105-14-346
Datta, P., Gupta, M., Kumar, M. B., Gupta, V., and Chander, J. (2020). Burkholderia cepacia complex causing pneumonia in an immunocompetent non-cystic fibrosis patient: case report and review of literature. Infect. Disord. Drug Targets 20, 106–110. doi: 10.2174/1871526518666181022112857
Devanga Ragupathi, N. K., and Veeraraghavan, B. (2019). Accurate identification and epidemiological characterization of Burkholderia cepacia complex: an update. Ann. Clin. Microbiol. Antimicrob. 18:7. doi: 10.1186/s12941-019-0306-0
Dhanda, S. K., Mahajan, S., Paul, S., Yan, Z., Kim, H., Jespersen, M. C., et al. (2019). IEDB-AR: immune epitope database—analysis resource in 2019. Nucleic Acids Res. 47, W502–W506. doi: 10.1093/nar/gkz452
Dimitrov, I., Flower, D. R., and Doytchinova, I. (2013). AllerTOP-a server for in silico prediction of allergens. BMC Bioinform. 14:S4. doi: 10.1186/1471-2105-14-S6-S4
Dombkowski, A. A., Sultana, K. Z., and Craig, D. B. (2014). Protein disulfide engineering. FEBS Lett. 588, 206–212.
Doytchinova, I. A., and Flower, D. R. (2007). VaxiJen: a server for prediction of protective antigens, tumour antigens and subunit vaccines. BMC Bioinform. 8:4. doi: 10.1186/1471-2105-8-4
Fatima, I., Ahmad, S., Abbasi, S. W., Ashfaq, U. A., Shahid, F., ul Qamar, M. T., et al. (2021). Designing of a multi-epitopes-based peptide vaccine against rift valley fever virus and its validation through integrated computational approaches. Comput. Biol. Med. 141:105151. doi: 10.1016/j.compbiomed.2021.105151
Fu, L., Niu, B., Zhu, Z., Wu, S., and Li, W. (2012). CD-HIT: accelerated for clustering the next-generation sequencing data. Bioinformatics 28, 3150–3152. doi: 10.1093/bioinformatics/bts565
Garg, A., and Gupta, D. (2008). VirulentPred: a SVM based prediction method for virulent proteins in bacterial pathogens. BMC Bioinform. 9:62. doi: 10.1186/1471-2105-9-62
Goldmann, D. A., and Klinger, J. D. (1986). Pseudomonas cepacia: biology, mechanisms of virulence, epidemiology. J. Pediatr. 108, 806–812. doi: 10.1016/s0022-3476(86)80749-1
Grote, A., Hiller, K., Scheer, M., Münch, R., Nörtemann, B., Hempel, D. C., et al. (2005). JCat: a novel tool to adapt codon usage of a target gene to its potential expression host. Nucleic Acids Res. 33, W526–W531. doi: 10.1093/nar/gki376
Guan, P., Doytchinova, I. A., Zygouri, C., and Flower, D. R. (2003). MHCPred: a server for quantitative prediction of peptide–MHC binding. Nucleic Acids Res. 31, 3621–3624. doi: 10.1093/nar/gkg510
Gul, S., Ahmad, S., Ullah, A., Ismail, S., Khurram, M., Tahir ul Qamar, M., et al. (2022). Designing a Recombinant Vaccine against Providencia rettgeri Using Immunoinformatics Approach. Vaccines 10:189. doi: 10.3390/vaccines10020189
Gupta, S., Kapoor, P., Chaudhary, K., Gautam, A., Kumar, R., Consortium, O. S. D. D., et al. (2013). In silico approach for predicting toxicity of peptides and proteins. PLoS One 8:e73957. doi: 10.1371/journal.pone.0073957
Hasan, T. H., and Al-Harmoosh, R. A. (2020). Mechanisms of antibiotics resistance in bacteria. Sys. Rev. Pharm. 11, 817–823.
Heo, L., Park, H., and Seok, C. (2013). GalaxyRefine: protein structure refinement driven by side-chain repacking. Nucleic Acids Res. 41, W384–W388. doi: 10.1093/nar/gkt458
Hutchings, M., Truman, A., and Wilkinson, B. (2019). Antibiotics: past, present and future. Curr. Opin. Microbiol. 51, 72–80. doi: 10.1016/j.mib.2019.10.008
Ismail, S., Ahmad, S., and Azam, S. S. (2020). Vaccinomics to design a novel single chimeric subunit vaccine for broad-spectrum immunological applications targeting nosocomial Enterobacteriaceae pathogens. Eur. J. Pharm. Sci. 146:105258. doi: 10.1016/j.ejps.2020.105258
Ismail, S., Shahid, F., Khan, A., Bhatti, S., Ahmad, S., Naz, A., et al. (2021). Pan-Vaccinomics Approach Towards a Universal Vaccine Candidate Against WHO Priority Pathogens to Address Growing Global Antibiotic Resistance. Comput. Biol. Med. 136:104705. doi: 10.1016/j.compbiomed.2021.104705
Izaguirre, J. A., Catarello, D. P., Wozniak, J. M., and Skeel, R. D. (2001). Langevin stabilization of molecular dynamics. J. Chem. Phys. 114, 2090–2098. doi: 10.1063/1.2464095
Jansen, K. U., and Anderson, A. S. (2018). The role of vaccines in fighting antimicrobial resistance (AMR). Hum. Vaccin. Immunother. 14, 2142–2149. doi: 10.1080/21645515.2018.1476814
Jespersen, M. C., Peters, B., Nielsen, M., and Marcatili, P. (2017). BepiPred-2.0: improving sequence-based B-cell epitope prediction using conformational epitopes. Nucleic Acids Res. 45, W24–W29. doi: 10.1093/nar/gkx346
Kräutler, V., Van Gunsteren, W. F., and Hünenberger, P. H. (2001). A fast SHAKE algorithm to solve distance constraint equations for small molecules in molecular dynamics simulations. J. Comput. Chem. 22, 501–508.
Kulik, E. M., Thurnheer, T., Karygianni, L., Walter, C., Sculean, A., and Eick, S. (2019). Antibiotic susceptibility patterns of aggregatibacter actinomycetemcomitans and porphyromonas gingivalis strains from different decades. Antibiotics 8:253. doi: 10.3390/antibiotics8040253
LiPuma, J. J. (2005). Update on the Burkholderia cepacia complex. Curr. Opin. Pulm. Med. 11, 528–533.
Liu, B., Zheng, D., Jin, Q., Chen, L., and Yang, J. (2019). VFDB 2019: a comparative pathogenomic platform with an interactive web interface. Nucleic Acids Res. 47, D687–D692. doi: 10.1093/nar/gky1080
MacLean, R. C., and San Millan, A. (2019). The evolution of antibiotic resistance. Science 365, 1082–1083.
Mahenthiralingam, E., Urban, T. A., and Goldberg, J. B. (2005). The multifarious, multireplicon Burkholderia cepacia complex. Nat. Rev. Microbiol. 3, 144–156. doi: 10.1038/nrmicro1085
Maiorov, V. N., and Crippen, G. M. (1994). Significance of root-mean-square deviation in comparing three-dimensional structures of globular proteins. J. Mol. Biol. 235, 625–34. doi: 10.1006/jmbi.1994.1017
Malonis, R. J., Lai, J. R., and Vergnolle, O. (2019). Peptide-Based Vaccines: Current Progress and Future Challenges. Chem. Rev. 120, 3210–3229. doi: 10.1021/acs.chemrev.9b00472
Mashiach, E., Schneidman-Duhovny, D., Andrusier, N., Nussinov, R., and Wolfson, H. J. (2008). FireDock: a web server for fast interaction refinement in molecular docking. Nucleic Acids Res. 36, W229–W232. doi: 10.1093/nar/gkn186
Miller, B. R., McGee, T. D., Swails, J. M., Homeyer, N., Gohlke, H., and Roitberg, A. E. (2012). MMPBSA.py: An efficient program for end-state free energy calculations. J. Chem. Theory Comput. 8, 3314–3321. doi: 10.1021/ct300418h
Moriel, D. G., Scarselli, M., Serino, L., Mora, M., Rappuoli, R., and Masignani, V. (2008). Genome-based vaccine development: A short cut for the future. Hum. Vaccin. 4, 184–188.
Naz, A., Awan, F. M., Obaid, A., Muhammad, S. A., Paracha, R. Z., Ahmad, J., et al. (2015). Identification of putative vaccine candidates against Helicobacter pylori exploiting exoproteome and secretome: a reverse vaccinology based approach. Infect. Genet. Evol. 32, 280–291. doi: 10.1016/j.meegid.2015.03.027
Naz, K., Naz, A., Ashraf, S. T., Rizwan, M., Ahmad, J., Baumbach, J., et al. (2019). PanRV: Pangenome-reverse vaccinology approach for identifications of potential vaccine candidates in microbial pangenome. BMC Bioinform. 20:123. doi: 10.1186/s12859-019-2713-9
Naz, S., Ahmad, S., Abbasi, S. W., Ismail, S., Waseem, S., Ul Qamar, M. T., et al. (2021). Identification of immunodominant epitopes in allelic variants VK210 and VK247 of Plasmodium Vivax Circumsporozoite immunogen. Infect. Genet. Evol. 96:105120. doi: 10.1016/j.meegid.2021.105120
Ong, E., Cooke, M. F., Huffman, A., Xiang, Z., Wong, M. U., Wang, H., et al. (2021). Vaxign2: the second generation of the first Web-based vaccine design program using reverse vaccinology and machine learning. Nucleic Acids Res. 49, W671–W678. doi: 10.1093/nar/gkab279
Opal, S. M. (2010). “A brief history of microbiology and immunology,” in Vaccines: A Biography, ed. A. W. Artenstein (Berlin: Springer), 31–56.
Pettersen, E. F., Goddard, T. D., Huang, C. C., Couch, G. S., Greenblatt, D. M., Meng, E. C., et al. (2004). UCSF Chimera—a visualization system for exploratory research and analysis. J. Comput. Chem. 25, 1605–1612. doi: 10.1002/jcc.20084
ProtParam, E. (2017). ExPASy-ProtParam Tool. Available online at: https://web.expasy.org/protparam/
Qamar, M. T. U., Saba Ismail, S. A., Mirza, M. U., Abbasi, S. W., Ashfaq, U. A., and Chen, L.-L. (2021). Development of a Novel Multi-Epitope Vaccine Against Crimean-Congo Hemorrhagic Fever Virus: An Integrated Reverse Vaccinology, Vaccine Informatics and Biophysics Approach. Front. Immunol. 12:669812. doi: 10.3389/fimmu.2021.669812
Qamar, M. T. U., Shokat, Z., Muneer, I., Ashfaq, U. A., Javed, H., Anwar, F., et al. (2020). Multiepitope-based subunit vaccine design and evaluation against respiratory syncytial virus using reverse vaccinology approach. Vaccines 8:288. doi: 10.3390/vaccines8020288
Rappuoli, R. (2001). Reverse vaccinology, a genome-based approach to vaccine development. Vaccine 19, 2688–2691.
Reche, P. A., Fernandez-Caldas, E., Flower, D. R., Fridkis-Hareli, M., and Hoshino, Y. (2014). Peptide-based immunotherapeutics and vaccines. J. Immunol. Res. 2014:256784.
Rizwan, M., Naz, A., Ahmad, J., Naz, K., Obaid, A., Parveen, T., et al. (2017). VacSol: a high throughput in silico pipeline to predict potential therapeutic targets in prokaryotic pathogens using subtractive reverse vaccinology. BMC Bioinform. 18:106. doi: 10.1186/s12859-017-1540-0
Roe, D. R., and Cheatham, T. E. III (2013). PTRAJ and CPPTRAJ: software for processing and analysis of molecular dynamics trajectory data. J. Chem. Theory Comput. 9, 3084–3095. doi: 10.1021/ct400341p
Rojas-Rojas, F. U., López-Sánchez, D., Meza-Radilla, G., Mendez-Canarios, A., Ibarra, J. A., and Estrada-de Los Santos, P. (2018). The controversial Burkholderia cepacia complex, a group of plant growth promoting species and plant, animals and human pathogens. Rev. Argent. Microbiol. 51, 84–92. doi: 10.1016/j.ram.2018.01.002
Sachdeva, G., Kumar, K., Jain, P., and Ramachandran, S. (2005). SPAAN: a software program for prediction of adhesins and adhesin-like proteins using neural networks. Bioinformatics 21, 483–491. doi: 10.1093/bioinformatics/bti028
Sanober, G., Ahmad, S., and Azam, S. S. (2017). Identification of plausible drug targets by investigating the druggable genome of MDR Staphylococcus epidermidis. Gene. Rep. 7, 147–153.
Schneidman-Duhovny, D., Inbar, Y., Nussinov, R., and Wolfson, H. J. (2005). PatchDock and SymmDock: servers for rigid and symmetric docking. Nucleic Acids Res. 33, W363–W367. doi: 10.1093/nar/gki481
Serruto, D., Bottomley, M. J., Ram, S., Giuliani, M. M., and Rappuoli, R. (2012). The new multicomponent vaccine against meningococcal serogroup B, 4CMenB: immunological, functional and structural characterization of the antigens. Vaccine 30, B87–B97. doi: 10.1016/j.vaccine.2012.01.033
Smith, K. A. (2011). Edward Jenner and the small pox vaccine. Front. Immunol. 2:21. doi: 10.3389/fimmu.2011.00021
Suleman, M., ul Qamar, M. T., Rasool, S., Rasool, A., Albutti, A., Alsowayeh, N., et al. (2021). Immunoinformatics and Immunogenetics-Based Design of Immunogenic Peptides Vaccine against the Emerging Tick-Borne Encephalitis Virus (TBEV) and Its Validation through In Silico Cloning and Immune Simulation. Vaccines 9:1210. doi: 10.3390/vaccines9111210
Tacconelli, E., Carrara, E., Savoldi, A., Harbarth, S., Mendelson, M., Monnet, D. L., et al. (2018). Discovery, research, and development of new antibiotics: the WHO priority list of antibiotic-resistant bacteria and tuberculosis. Lancet Infect. Dis. 18, 318–327. doi: 10.1016/S1473-3099(17)30753-3
Tacconelli, E., Magrini, N., Kahlmeter, G., and Singh, N. (2017). Global priority list of antibiotic-resistant bacteria to guide research, discovery, and development of new antibiotics. World Heal. Organ. 27, 318–327.
Turner, P. J. (2005). XMGRACE, Version 5.1. 19. Beaverton, OR: Center for Coastal and Land-Margin Research, Oregon Graduate Institute of Science and Technology.
Tusnady, G. E., and Simon, I. (2001). The HMMTOP transmembrane topology prediction server. Bioinformatics 17, 849–850. doi: 10.1093/bioinformatics/17.9.849
ud-din, M., Albutti, A., Ullah, A., Ismail, S., Ahmad, S., Naz, A., et al. (2022). Vaccinomics to Design a Multi-Epitopes Vaccine for Acinetobacter baumannii. Int. J. Environ. Res. Public Health 19:5568.
Ullah, A., Ahmad, S., Ismail, S., Afsheen, Z., Khurram, M., Tahir ul Qamar, M., et al. (2021). Towards A Novel Multi-Epitopes Chimeric Vaccine for Simulating Strong Immune Responses and Protection against Morganella morganii. Int. J. Environ. Res. Public Health 18:10961. doi: 10.3390/ijerph182010961
Vita, R., Overton, J. A., Greenbaum, J. A., Ponomarenko, J., Clark, J. D., Cantrell, J. R., et al. (2015). The immune epitope database (IEDB) 3.0. Nucleic Acids Res. 43, D405–D412. doi: 10.1093/nar/gku938
Wang, J., Wang, W., Kollman, P. A., and Case, D. A. (2001). Antechamber: an accessory software package for molecular mechanical calculations. J. Am. Chem. Soc 222:U403.
Yero, D., Conchillo-Solé, O., and Daura, X. (2021). “Antigen Discovery in Bacterial Panproteomes,” in Vaccine Delivery Technology, eds B. A Feifer and A Hill (Berlin: Springer), 43–62. doi: 10.1007/978-1-0716-0795-4_5
Yu, N. Y., Wagner, J. R., Laird, M. R., Melli, G., Rey, S., Lo, R., et al. (2010). PSORTb 3.0: improved protein subcellular localization prediction with refined localization subcategories and predictive capabilities for all prokaryotes. Bioinformatics 26, 1608–1615. doi: 10.1093/bioinformatics/btq249
Keywords: pseudomonas cepacia, reverse vaccinology, immunoinformatic, molecular docking, MD simulations
Citation: Alsowayeh N, Albutti A and Al-Shouli ST (2022) Reverse Vaccinology and Immunoinformatic Assisted Designing of a Multi-Epitopes Based Vaccine Against Nosocomial Burkholderia cepacia. Front. Microbiol. 13:929400. doi: 10.3389/fmicb.2022.929400
Received: 26 April 2022; Accepted: 20 May 2022;
Published: 28 June 2022.
Edited by:
Som G. Nanjappa, University of Illinois at Urbana-Champaign, United StatesReviewed by:
Hafiz Ishfaq Ahmad, University of Veterinary and Animal Sciences, PakistanZihao Chen, University of Freiburg, Germany
Muhahammad Jamil Ahmad, Huazhong Agricultural University, China
Copyright © 2022 Alsowayeh, Albutti and Al-Shouli. This is an open-access article distributed under the terms of the Creative Commons Attribution License (CC BY). The use, distribution or reproduction in other forums is permitted, provided the original author(s) and the copyright owner(s) are credited and that the original publication in this journal is cited, in accordance with accepted academic practice. No use, distribution or reproduction is permitted which does not comply with these terms.
*Correspondence: Noorah Alsowayeh, bi5hbHNvd2F5ZWhAbXUuZWR1LnNh; Aqel Albutti, YXMuYWxidXR0aUBxdS5lZHUuc2E=
†These authors have contributed equally to this work