- 1Department of Crop Sciences, University of Illinois, Urbana, IL, United States
- 2Centro de Recursos Naturales Renovables de la Zona Semiárida, UNS-CONICET, Departamento de Agronomía, Universidad Nacional del Sur, Bahía Blanca, Argentina
- 3Department of Animal Sciences, University of Illinois, Urbana, IL, United States
Cover cropping (CC) is a promising in-field practice to mitigate soil health degradation and nitrogen (N) losses from excessive N fertilization. Soil N-cycling microbial communities are the fundamental drivers of these processes, but how they respond to CC under field conditions is poorly documented for typical agricultural systems. Our objective was to investigate this relationship for a long-term (36 years) corn [Zea mays L.] monocultures under three N fertilizer rates (N0, N202, and N269; kg N/ha), where a mixture of cereal rye [Secale cereale L.] and hairy vetch [Vicia villosa Roth.] was introduced for two consecutive years, using winter fallows as controls (BF). A 3 × 2 split-plot arrangement of N rates and CC treatments in a randomized complete block design with three replications was deployed. Soil chemical and physical properties and potential nitrification (PNR) and denitrification (PDR) rates were measured along with functional genes, including nifH, archaeal and bacterial amoA, nirK, nirS, and nosZ-I, sequenced in Illumina MiSeq system and quantified in high-throughput quantitative polymerase chain reaction (qPCR). The abundances of nifH, archaeal amoA, and nirS decreased with N fertilization (by 7.9, 4.8, and 38.9 times, respectively), and correlated positively with soil pH. Bacterial amoA increased by 2.4 times with CC within N269 and correlated positively with soil nitrate. CC increased the abundance of nirK by 1.5 times when fertilized. For both bacterial amoA and nirK, N202 and N269 did not differ from N0 within BF. Treatments had no significant effects on nosZ-I. The reported changes did not translate into differences in functionality as PNR and PDR did not respond to treatments. These results suggested that N fertilization disrupts the soil N-cycling communities of this system primarily through soil acidification and high nutrient availability. Two years of CC may not be enough to change the N-cycling communities that adapted to decades of disruption from N fertilization in corn monoculture. This is valuable primary information to understand the potentials and limitations of CC when introduced into long-term agricultural systems.
Introduction
As one of the most promising conservation practices, cover cropping (CC) bolsters the natural functions of an agricultural system through ecological intensification (Tittonell, 2014). This leads to various benefits like reducing soil erosion, returning organic matters to the soil, and weed suppression (Blanco-Canqui et al., 2015; Blesh et al., 2019; Reiss and Drinkwater, 2020). Many of such benefits contribute toward soil health, the soil’s capacity to support ecological services crucial for sustainable agriculture (Lehmann et al., 2020). Thus, CC has been widely proposed as a crucial strategy to improve the soil health of intensely managed systems that are vulnerable to soil degradation and nutrient pollution (IL-EPA et al., 2015). Simplified cropping systems concentrated on commercially important crops like corn [Zea mays L.] and soybean [Glycine max (L.) Merr.] dominate the crucial agricultural regions like the United States Midwest (Hatfield et al., 2018). As these systems, especially those based on corn, rely on frequent and heavy N fertilization, an estimated four Tg of inorganic N is applied each year in this region alone (USDA-ERS, 2020; Socolar et al., 2021). However, the low N use efficiency of these systems leads to excess soil N, which causes chemical imbalances that not only degrade the soil health but also become major sources of water pollution and greenhouse gas (GHG) emissions (Rengel, 2011; Fagodiya et al., 2017). Here, replacing bare fallows with CC can scavenge the excess N and release them slowly upon decomposition, thereby reducing the soil N in forms vulnerable to loss Indeed, past primary research and research syntheses have demonstrated that CC mixtures that include non-legumes generally reduce NO3– leaching (Thapa et al., 2018) and nitrous oxide (N2O), a potent GHG, and emissions (Basche et al., 2014; Muhammad et al., 2019).
The soil microbes are the fundamental drivers of soil processes that constitute ecological services. Likewise, the soil N-cycling is largely determined by the complex web of activities by the N-cycling microbial communities. Therefore, changes in the soil environment from management practices can perturb the N-cycling communities and their functionality (Behnke et al., 2022; Kim et al., 2022b). Indeed, soil chemical imbalances and N losses in vulnerable agricultural systems are symptoms of excessive N fertilization disturbing the soil microbial N-cycling (Hirsch and Mauchline, 2015; Li et al., 2022). Likewise, various effects of CC, including excess soil N removal, organic matter return, and root exudation may also modify the soil environment and lead to unintended responses from the N-cycling communities. For instance, compared to leaving the terminated CC residues on the surface, incorporating them into the soil can rather increase N2O emissions by accelerating the N mineralization by the decomposers (Muhammad et al., 2019). Also, Foltz et al. (2021) have shown that although cereal rye CC decreased field N2O emission from a corn-soybean rotation, it increased the N2O production potential under assay settings, suggesting that CC might increase emissions without proper nutrient management. Thus, evaluating the effectiveness of CC in mitigating the detriments of excessive N fertilization must include how the soil N-cycling communities respond to changes in the soil environment under CC.
Abundances of the functional genes that code N-cycling enzymes represent the population sizes of their respective N-cycling communities. Indeed, the abundances of N-cycling functional genes tend to highly correlate with their respective inorganic N products (Lourenço et al., 2022). For example, N2O emission correlates negatively with functional genes that code N2O reductase but does so positively with those of nitrifiers (You et al., 2022). The commonly used N-cycling functional genes include nifH (nitrogenase; N-fixation), ammonia-oxidizing archaea (AOA) and bacterial (AOB) amoA (ammonia monooxygenase; nitrification), nirK and nirS (nitrite reductase; denitrification), and nosZ-I (nitrous oxide reductase; denitrification; Hirsch and Mauchline, 2015). As N fertilizers directly interact with the microbes harboring these genes, many studies have reported their sensitivity to N fertilization. For example, an Illinois study on the bioenergy production system of Mollisols reported that N fertilization (112 kg N/ha) increased the abundances of AOB amoA, but decreased those of nirS and nifH (Kim et al., 2022a). Meanwhile, a meta-analysis by Ouyang et al. (2018) compiled the results from 47 primary studies on how N fertilization affects these genes. The authors suggested that N inputs can intensify microbial N-cycling. Furthermore, the responses of these genes were sensitive to cofactors like fertilizer forms (organic vs. inorganic) and cropping systems (monoculture vs. rotation). Like these cofactors, CC might also alter the interactions between these functional genes and N fertilization. While these functional genes provide crucial insights into specific N-cycling communities, they do not equate to overall functionality as population size may not necessarily translate to activity. Thus, they need to be complemented by more direct measures of functionality like potential nitrification (PNR) and denitrification (PDR) rates determined by enzyme assays. Indeed, reports from various systems show that functional genes do correlate with PNR and PDR (Xu et al., 2012; Chen et al., 2013; Li et al., 2022). For instance, a study in acidic soils under soybean-corn-corn rotation reported significant correlations between amoA genes and PNR (Xu et al., 2012). However, primary research that coupled functional genes and more direct measures functionality is scarce for N-cycling communities under CC, especially within typical Midwestern cropping systems.
A few past studies have characterized the N-cycling functional genes under CC. For example, Hu et al. (2021), on cotton monoculture on Tennessee Alfisols, compared bacterial functional genes among bare fallow, legume CC, and grass CC between two N rates (0 and 67 kg N/ha), and between tillage and no-till. The authors reported that AOB amoA increased in abundance with legume CC and more so with N input, while grass CC only increased AOB amoA when unfertilized. They also showed that nifH increased sequentially from bare fallow, legume CC, and grass CC. Yet, the N rates of this study are much lower than those typically simplified cropping systems. Another recent study by Castellano-Hinojosa et al. (2022) also reported that CC, especially a legume and non-legume mixture, generally increased the abundance of the N-cycling functional genes. Yet, their system was a citrus orchard, which is also different from the United States Midwestern cropping systems. Thus, currently available studies on this topic are done in systems dissimilar to the intensely managed and simplified cropping systems typical to the major agricultural regions like the United States Midwest. Therefore, there is a critical lack of primary information on how the N-cycling functional genes respond to introducing CC to such systems.
Three past reports exist on this study’s experimental site where Kim et al. (2022b) and Huang et al. (2019) each characterized its soil and the N-cycling functional genes, respectively, before CC; while Kim et al. (2022c) described the soil microbiota with high taxonomic resolution using genus-level bioindicators after introducing CC. Kim et al. (2022b) demonstrated that significant soil acidification, increase in NO3–, and nutrient depletion marked the long-term effects of heavy N inputs in corn monocultures over resilient Mollisols. Huang et al. (2019) found that N fertilization decreased the abundance of nifH while increasing that of AOB amoA. Kim et al. (2022c) observed more diversified N-cycling communities in unfertilized soils, including an increased abundance of an indicator of AOA. They also reported soil acidification as the major modulating factor for the soil microbes of this system. Meanwhile, CC increased the abundances of N-fixers and nirK denitrifiers, while decreasing those of N2O reducers (Kim et al., 2022c). These potential shifts in the N-cycling guilds after introducing CC may have translated into the abundance of the functional genes, and, ultimately, into soil microbial N-cycling.
Thus, we aimed to investigate whether CC can mitigate the disruptions in the soil microbial N-cycling after long-term N fertilization in typical simplified agricultural systems. In accordance with aforementioned past reports on this study’s site, we hypothesized that: (i) N fertilization would decrease the number of nifH and AOA amoA but increase that of AOB amoA; (ii) introducing CC will increase the abundances of nifH and nirK; and that (iii) these changes will translate to changes in PNR and PDR. Our objective was to characterize the soil N-cycling communities after introducing CC to a long-term corn monoculture of 36 years of consistent management with and without N fertilization, using six commonly used functional genes (nifH, AOA and AOB amoA, nirK, nirS, and nosZ-I). This study also determined the soil properties, and PNR and PDR to test whether these genes are consistent with the changes in the soil environment and functionality by treatments. This study will provide primary information on how CC affects the soil microbial N-cycling in a simplified cropping system under heavy N inputs. This will be valuable for future research and decision-makers to evaluate CC as a strategy to improve soil health and nutrient cycling.
Materials and Methods
Experimental Site Description and Management Practices
The field experimental site was established at the Northwestern Illinois Agricultural Research and Demonstration Center (40° 55′ 50″ N, 90° 43′ 38″ W) in 1981 to study the effects of N fertilization rates (Nrates) on corn yields of continuous corn and corn-soybean short rotations (Figure 1). The mean annual precipitation and temperature of this site are 914 mm and 10.6°C, respectively, Illinois State Water Survey (2010). The soil series is Muscatune silt loam (fine-silty, mixed, and mesic Aquic Argiudoll), dark-colored and very deep soils of moderate permeability, and low surface runoff potential that developed under prairie vegetation in a layer of 2–3 m thick loess over glacial till (Soil Survey Staff et al., 2020). The topography of this site is nearly flat (Soil Survey Staff et al., 2020). Kim et al. (2022b) described further information on this experimental site and field management up to 2018.
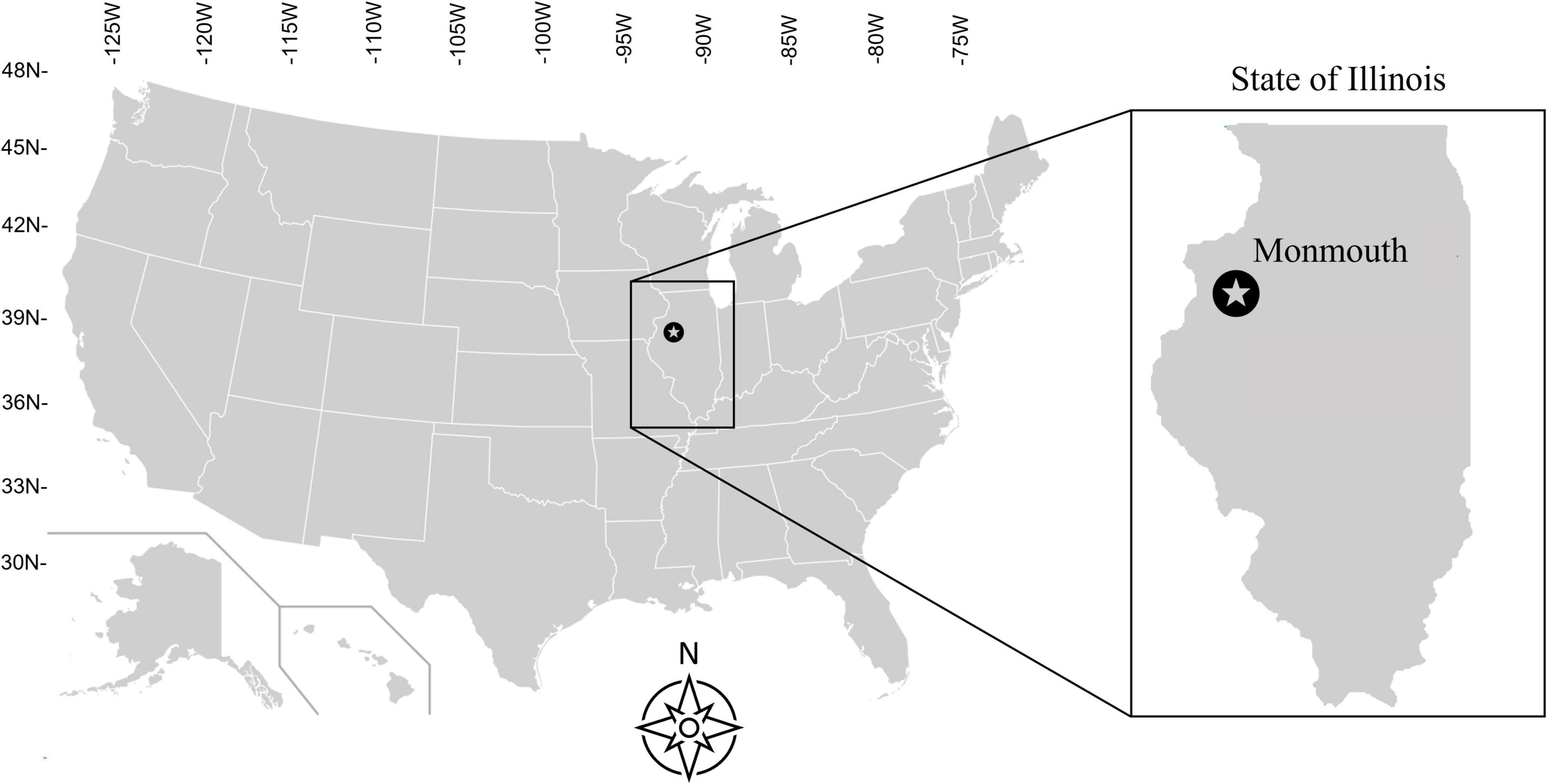
Figure 1. Location and coordinates of the experimental site within the United States and the state of Illinois.
This study started in 2018 and focused on introducing CC to the corn monoculture plots, spanning two CC growing seasons in 2018–2019 and 2019–2020. The experiment was arranged in a split-plot of Nrates (N0, N202, and N269; kg N/ha) and CC (cover crop, CC; bare fallow control, BF) in a randomized complete block design with three replicates. The main plot dimension was 18 m × 6 m, and the subplots were 18 m × 3 m. Fertilizer, weed, and pest management decisions were based on the best management practices as recommended by the Illinois Agronomy Handbook (Fernández and Hoeft, 2009). No P and K fertilizers or lime were applied during this experiment. Corn was harvested in mid-October with a plot combine (Almaco, Nevada, IA, United States). Following corn harvest, on October 3, 2018, and on October 19, 2019, a mixture of cereal rye [Secale cereale L.] and hairy vetch [Vicia villosa Roth.] was no-till drill-seeded at 84 kg seeds/ha (70% cereal rye, and 30% hairy vetch). Cover crop termination occurred in early May, both years following soil sampling, using glyphosate [N-(phosphonomethyl)glycine] (Roundup WeatheMAX®, Bayer AG, Leverkusen, Germany) at the rate of 1.89 kg ai/ha. Spring N fertilizer treatments were applied as incorporated urea ammonium nitrate (UAN, 28%) on May 15, 2019 and May 5, 2020, following CC suppression and before planting corn. Spring tillage using a rotary tiller (Dyna Drive Cultivator, EarthMaster, Alamo Group, Inc., Seguin, TX, United States) was conducted on June 3, 2019 and May 11, 2020. Corn cash crop was planted on June 3, 2019 and May 26, 2020, at 88,000 seeds/ha.
Soil and Cover Crop Biomass Sampling and Properties Measurement
Soil sampling occurred on April 26, 2019 and April 30, 2020. Within each experimental unit, three composited soil subsamples, each up to 500 g, were taken from random points at 0–10 cm depth with an Eijelkamp grass plot sampler (Royal Eijkelkamp Company, Giesbeek, Netherlands) for microbial DNA extraction and PNR and PDR rates. These soil samples were transported in coolers filled with ice from the experimental site and then stored at −20°C. Also, three 0–90 cm depth soil core subsamples were taken per experimental unit using a tractor-mounted soil sampler with soil sleeve inserts (Amity Tech, Fargo, ND, United States). These soil cores were divided into depths of 0–30, 30–60, and 60–90 cm, and transported to the lab to determine the water content (%) and bulk density (Bd; Mg/m3). Only the data from 0 to 30 cm is reported in this study. About 10 g of soil per subsample was oven-dried at 105°C to measure the gravimetric water content and obtain Bd using the core method (Blake and Hartge, 1986). About 4 g of each subsample was used to determine water soil aggregate stability (WAS,%) with a west sieving apparatus (Eijkelkamp, Giesbeek, Netherlands). The remaining was sent to a commercial laboratory (Brookside Laboratories, Inc., New Bremen, OH, United States) to determine additional soil properties using standard methods recommended for United States-North Central region1. Thus, soil organic matter (SOM,%) content was determined by loss-on-ignition at 360°C (Schulte and Hopkins, 1996). Soil available P was measured with Bray I extraction (Pbray, mg/kg), and the extractable elements, including sulfur, calcium, magnesium, sodium, and potassium (K, mg/kg), with the Mehlich 150 III method (Ziadi and Tran, 2008). The summation of these exchangeable cations was used to estimate the cation exchange capacity (CEC, cmolc/kg; Sumner and Miller, 1996).
The PNR and PDR were measured with the methods adapted from Drury et al. (2008) and Malique et al. (2019), respectively. For PNR, a mixture of 6 g of the soil subsample and 40 mL of working solution was activated for assay by shaking them for an hour in a 125 mL jar covered with parafilm with several holes, on a rotary shaker (Corning® LSE™ orbital shaker, Corning, NY, United States) at 180 RPM at room temperature. After assay activation, 5 mL of initial aliquot was taken and it was centrifuged for 10 min at 9,000 RPM (Sorvall® RC 5C Plus, Kendro Laboratory Products, Asheville, NC, United States) and the supernatant was transferred and stored frozen for further analysis. NO3– and NO2– were measured using SmartChem 200 (Westco Scientific Instruments, Inc., Danbury, CN, United States). For the dry mass of the soil used in this equation, the mass of 1 g of soil subsample was measured after drying it for 48 h in the DKN-810 Mechanical Convection Oven (Yamato Scientific America Inc., Santa Clara, CA, United States). For PDR, 10 g of soil and 20 mL of working solution were put in 125 mL jars with septa caps. The headspace was vacuumed and, then, refilled with 100 mL of N2 gas with a syringe; this process was repeated once. Then, 20 mL of acetylene was inserted into the jar to prevent N2O reduction, and a 15 mL initial gas sample was taken and replenished with 15 mL of N2 gas. The jar that was shaken by hand to initiate reaction was incubated in a shaker (Eberbach, Ann Arbor, MI, United States). Gas samples were taken three times in 30 min intervals, replenishing the jars with 15 mL N2 gas each time. The gas samples were analyzed in a gas chromatograph equipped with an electron capture detector and autosampler (Shimadzu GC-2014 and AOC 5000 Plus, Kyoto, Japan). The N2O concentrations were calculated with the equation used in Malique et al. (2019), but the PDR (ng N/g of dry soil hour) was calculated using the t = 0min N2O concentration instead of that of t = 30min.
Soil DNA Extraction and Real-Time Quantitative Polymerase Chain Reaction Analysis
Soil DNA was extracted from 0.25 g of each homogenized soil subsample, using PowerSoil® DNA isolation kits (MoBio Inc., Carlsbad, CA, United States) according to the manufacturer’s instruction, and stored at −20°C. A Nanodrop 1000 Spectrophotometer (Thermo Fisher Scientific, Waltham, MA, United States) was used to test the quantity and quality of the extracted DNA. The DNA samples were sequenced using primers for the bacterial V4 region 16S rRNA and archaeal 16S rRNA, fungal internal transcribed spacer (ITS) region, nitrogenase nifH, bacterial and archaeal ammonia monooxygenase amoA, nitrite reductase nirK and nirS, and nitrous oxide reductase nosZ-I. For this, the DNA samples were sent to W.M. Keck Center for Comparative and Functional Genomic lab at the University of Illinois Biotechnology Center (Urbana, IL, United States) for Illumina MiSeq paired-end System (2 × 250 bp; Illumina, Inc., San Diego, CA, United States). The maximum sample DNA concentration was set to 50 ng/μL. The primer sets used for amplification were 515F (GTGYCAGCMGCCGCGGTAA) and 806R (GGACTACVSGGGTWTCTAAT) for the bacterial 16S rRNA gene (Fierer et al., 2005); 349F (GTGCASCAGKCGMGAAW) and 806R (GGACTACVSGGGTATCTAAT) for the archaeal 16S rRNA gene (Colman et al., 2015); 3F (GCATCGATGAA GAACGCAGC) and 4R (TCCTCCGCTTATTGATATGC) for the fungal ITS region (Crawford et al., 2012); CS1-nifH-PolF-124-for (TGCGAYCCSAARGCBGACTC) and CSs-nifH-PolR-118-rev (ATSGCCATCATYTCRCCGGA) for nifH (Poly et al., 2001); CrenamoA23f (ATGGTCTGGCTW AGACG) and CrenamoA616r (GCCATCCATCTGTATG TCCA) for AOA amoA (Tourna et al., 2008); amoA-1F-10-for (GGGGTTTCTACTGGTGGT) and amoA-2R-10-rev (CCCCTCKGSAAAGCCTTCTTC) for AOB amoA (Rotthauwe et al., 1997); CS1-nirK876-209-for (ATYGGCGGVCAYG GCGA) and CS2-nirK1040-197-rev (GCCTCGATCAGRTTRT GGTT) for nirK (Henry et al., 2004); CS1-nirSCd3aF-211-for (AACGYSAAGGARACSGG) and CS2-nirSR3cd-199-rev (GASTTCGGRTGSGTCTTSAYGAA) for nirS (Kandeler et al., 2006); and CS1-nosZ1F-16-for (WCSYTGTTCMTCGACA GCCAG) and CS2-nosZ1R-15-rev (ATGTCGATCARCTGV KCRTTYTC) for nosZ-I (Henry et al., 2006).
The resulting pooled amplicons of template DNA from the Illumina MiSeq system from all samples were prepared into PCR mixtures by mixing 1 μL of the pooled amplicon, 25.8 μL of ddH2O, 10 μL of 5X GoTaq green reaction buffer (Promega Corp., Madison, WI, United States), 4 μL of dNTP at 2.5 mM, 5 μL of MgCl2 at 25 mM, 0.2 μL of GoTaq DNA polymerase (Promega Corp., Madison, WI, United States), and 2 μL of primers for each forward and reverse sequences for each of the nine target sequences described above. This procedure was repeated by replacing the pooled amplicon with water to make controls. The mixtures were amplified in PCR with BioRad T100 thermal cycler (Bio-Rad Laboratories, Hercules, CA, United States) with parameters of 95°C for 10 min, followed by 34 cycles of amplification (45 s at 95°C; 45 s at 58°C; 45 s at 72°C), and a final extension at 72°C for 10 min. The amplified mixtures were run in electrophoresis with 1.5% agarose gel containing GreenGlo™ Safe DNA dye (Denville Scientific, Inc. Metuchen, NJ, United States), so that each pure, amplified marker genes are contained in one of the wells. The thick bands in the gel visible under UV light at the respective molecular weights of each target gene were cut out. Then, DNA was extracted from the cut gels using Monarch® Genomic DNA Purification Kit (New England Biolabs, Ipswich, MA, United States), following the manufacturer’s instructions. The resulting DNA samples were again checked for quantity and quality using Nanodrop 1000 Spectrophotometer. Then, to make the standard curve, aliquots of each target gene were taken in unique amounts that equalized their estimated gene counts to 1.51 × 1010 copies for each of the nine marker genes. The aliquots were mixed and topped to 500 μL with 1× TE buffer. Then, the aliquots were diluted in 10-fold series and used 108 to 101 dilutions, so that the range of dilution encompasses the possible DNA copies per sample. Finally, quantitative polymerase chain reaction (qPCR) plates were prepared, including 70 μL of primers for each of the nine marker genes, 10 μL for each dilution of the standard curve for each plate, and 10 μL of aliquots for each sample. The prepared plates were run for high-throughput qPCR in a BioMark HD™ System (Fluidigm Corporation, South San Francisco, CA, United States) at the W.M. Keck Center for Comparative and Functional Genomics at the University of Illinois Biotechnology Center.
Statistical Analysis and Visualization
Linear mixed models were fitted using the GLIMMIX procedure in SAS software version 9.4 (SAS Institute, Cary, NC, United States) to determine the effects of Nrate, CC treatments (CC), and their interactions on the response variables: soil properties, the log10-transformed gene copy counts, and PNR and PDR (Littell et al., 2006). The Nrate, CC, and their interaction were considered fixed effects, while the blocks, years, and their interactions with the fixed effects were considered random terms in the analysis of variance (ANOVA). For any significant treatment effects on the response variables in ANOVA, their least-square means were separated by treatment levels, with the lines option and setting the probability of a type I error at α = 0.10. To assess the correlations among the soil properties, PNR, PDR, and the nine marker genes, their Spearman’s rank correlation coefficients were calculated using the R function cor with option method = “spearman.” Relationships with coefficients (Spearman’s rho, ρ) above |0.8| were considered “very strong,” those between |0.6–0.8| as “strong,” and those between |0.4–0.6| as “moderate,” using the ranges from Huang et al. (2019) and setting the Type I error rate at α = 0.05. Weak relationships between |0.2–0.4| were disregarded even if they were statistically significant, to make the analysis more selective. The Spearman correlation also included soil pH, NH4+, and NO3– as their correlations with the functional genes and PNR and PDR represent new information not reported by Kim et al. (2022c). The ggplot2 package in R version 4.1.0 was used to create the figures (Wickham, 2016; R Core Team, 2019).
Results
Responses of Soil Properties and Potential Nitrification Rate and Potential Denitrification Rate to N Rate and Cover Cropping Treatments
Table 1 shows the estimated treatment means, the standard errors of the mean (SEM), sample size (n), and the results of mean separation procedures for each level of Nrate, CC, and their interactions for selected soil properties (CEC, SOM, Pbray, K, Bd, and WAS) at 0–30-cm depth. Table 1 also shows the degrees of freedom (df) and probability values (p-value) associated with the ANOVA for each source of variation. The ANOVA and mean separation results for Nrate and CC main effects and their interaction effects on PNR and PDR are also included in Table 1. This study detected statistically significant Nrate main effects on CEC (p = 0.0098), Pbray (p = 0.0209), and K (p = 0.0005). The estimated means for CEC decreased sequentially with higher N rates. The mean Pbray and K decreased significantly with N fertilization, by 75 and 31%, respectively. Meanwhile, PNR and PDR did not have any statistically significant treatment effects. No statistically significant CC main effect nor Nrate × CC interaction effect was detected.
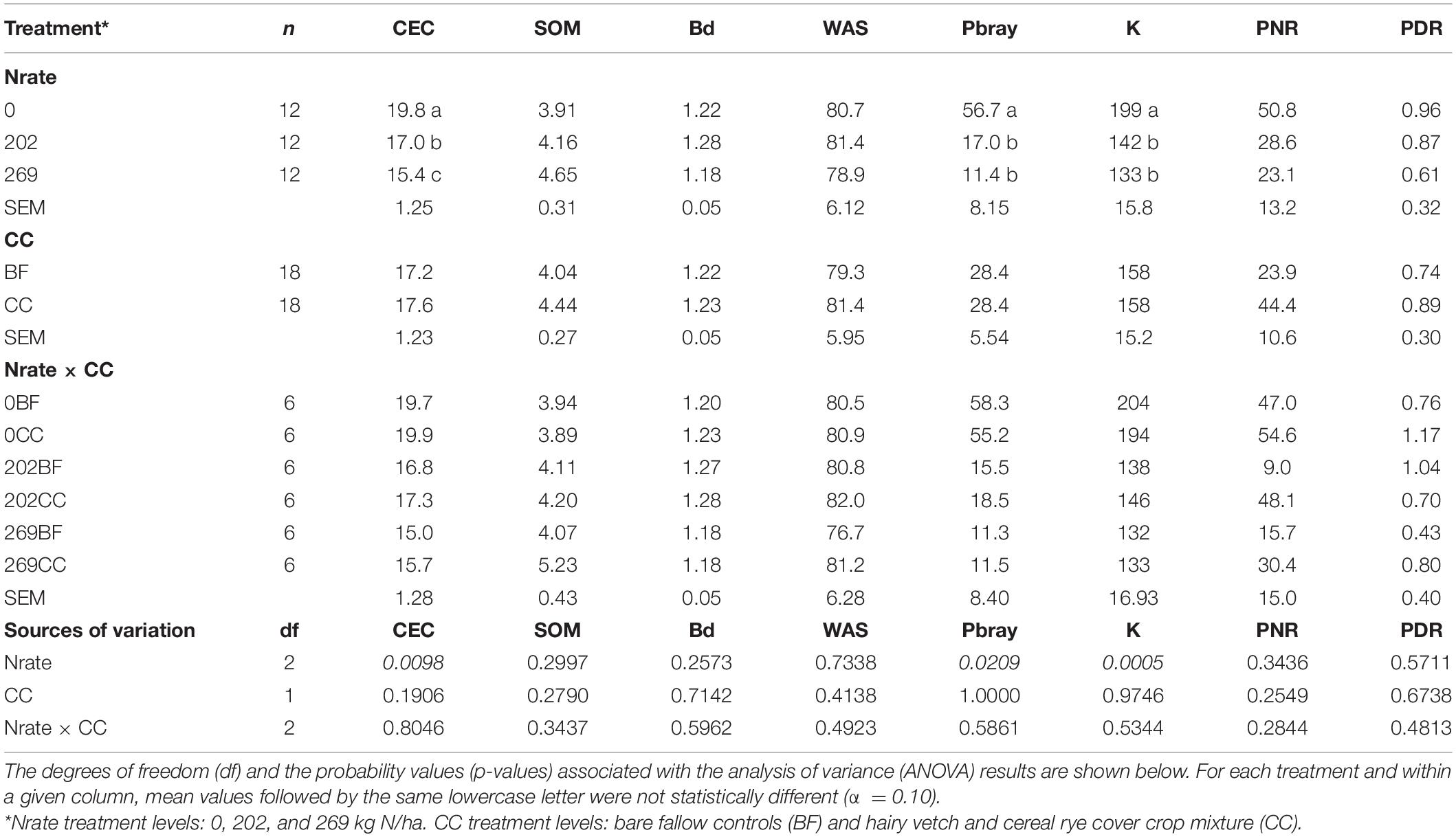
Table 1. Estimated treatment means, standard errors of the mean values (SEM), and sample size (n) of selected soil chemical properties, including cation exchange capacity (CEC, cmolc/kg), soil organic matter (SOM,%), bulk density (Bd, Mg/m3), water aggregate stability (WAS,%), available phosphorus (Pbray, mg/kg), and extractable potassium (K, mg/kg), along with the potential nitrification (PNR, mg N/kg dry soil day), and denitrification (PDR, ng N/g dry soil hr) rates determined under the N fertilization (Nrate) and cover cropping (CC) treatments, and their interactions.
Responses of N-Cycling Functional Genes to N Rate and Cover Cropping Treatments
The estimated means, the SEM, and the mean separation by treatment levels of Nrate, CC, and their interactions for each log10-transformed count of the bacterial and archaeal 16S rRNA, fungal ITS, and the six N-cycling genes are summarized in Table 2. The sample size (n), df, and p-values associated with the results of ANOVA for each source of variation are also shown in Table 2. Figures 2–4 each illustrate the treatment means and the SEM of these genes for the Nrate main effect, CC main effect, and their interaction effect, respectively. The number of bacterial 16S rRNA region copies ranged between 1.95 × 107 and 1.12 × 108 copies/μg DNA, with the average count of 5.89 × 107 copies/μg DNA. The number of archaeal 16S rRNA regions ranged between 2.04 × 105 and 1.73 × 106 copies/μg DNA, with the average count of 6.76 × 105 copies/μg DNA. Bacterial and archaeal 16S rRNA did not have any treatment effect as a significant source of variation. The fungal ITS region counts ranged between 1.05 × 107 and 1.70 × 108 copies/μg DNA and averaged at 4.90 × 107 copies/μg DNA. The fungal ITS region counts had a statistically significant (p = 0.0499) Nrate main effect, where the mean counts increased with N fertilization.
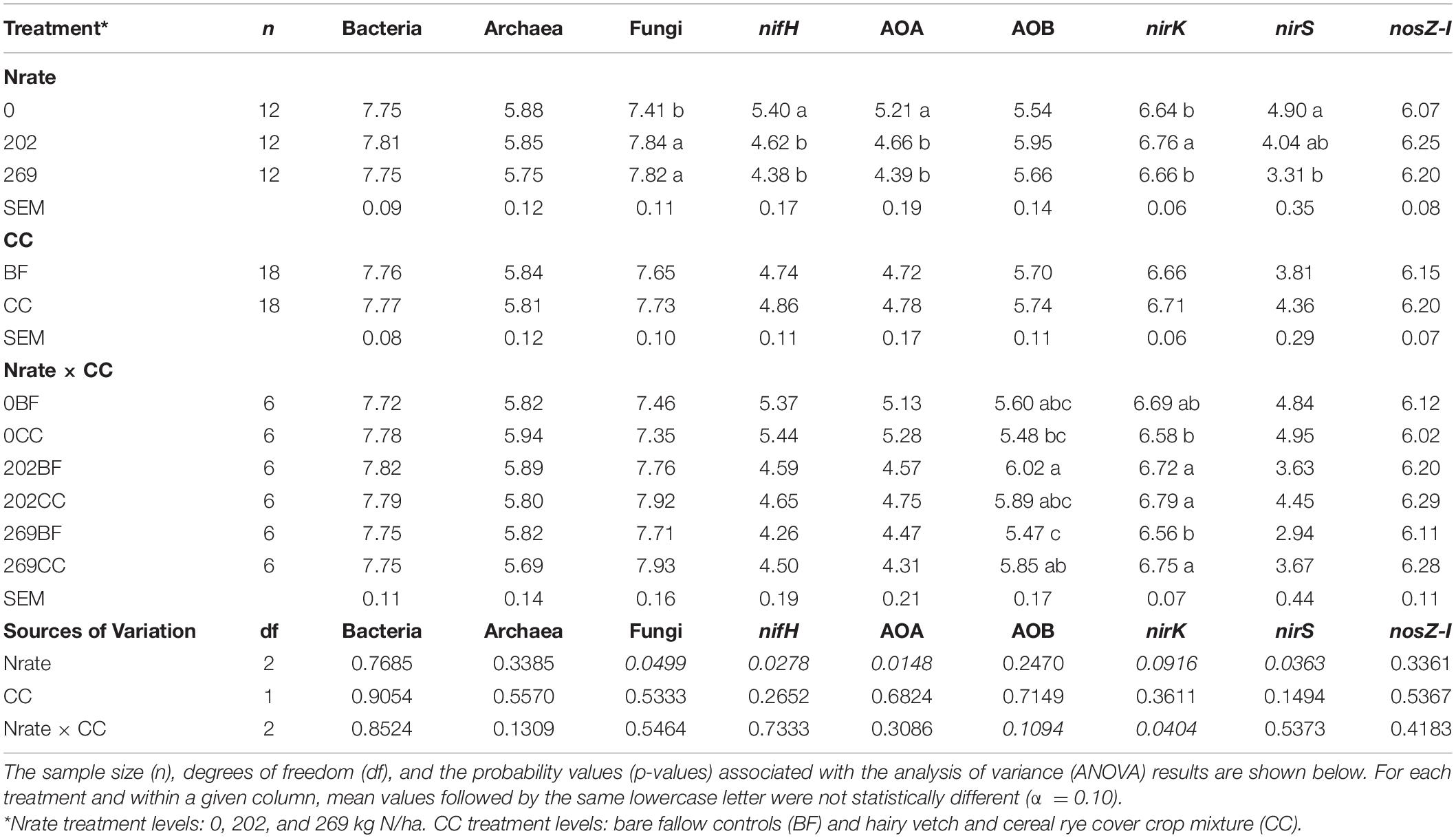
Table 2. Estimated treatment means and standard errors of the mean (SEM) of the log10-transformed copies (per μg DNA) of microbial marker genes, including bacterial (Bacteria) and archaeal (Archaea) 16S rRNA, fungal ITS region (Fungi), nifH, archaeal (AOA) and bacterial (AOB) amoA, nirK, nirS, and nosZ-I determined under N fertilization (Nrate), Cover crop (CC) treatments, and their interactions.
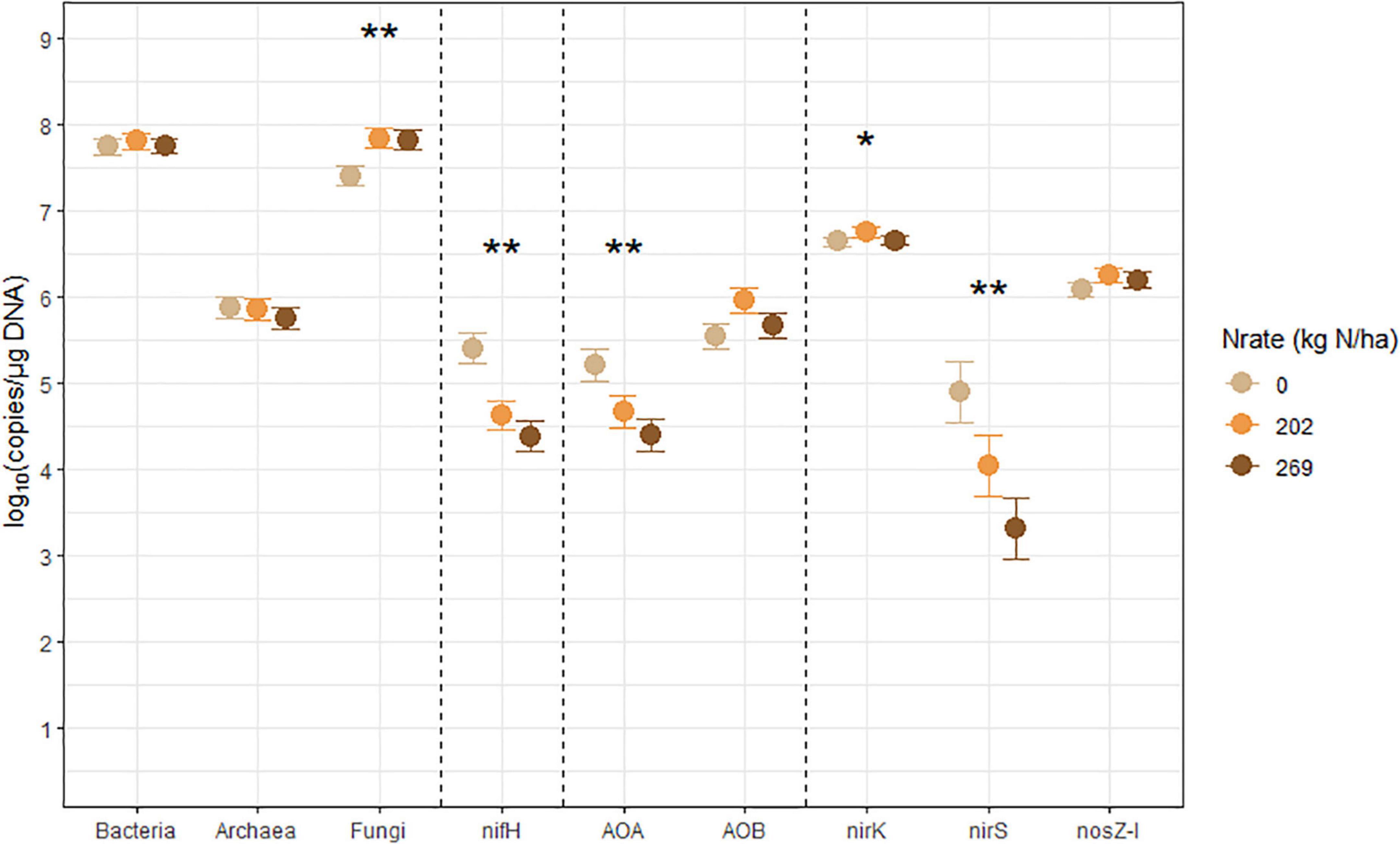
Figure 2. Treatment means of soil microbial marker genes (log10 copies/μg DNA), including bacterial (Bacteria) and archaeal (Archaea) 16S rRNA, fungal ITS region (Fungi), nifH, archaeal (AOA) and bacterial (AOB) amoA, nirK, nirS, and nosZ-I separated by N fertilization rate (Nrate), with their standard errors of the mean as whiskers. Asterisks indicate the level of significance associated with the probability value from analysis of variance of the factors (*p < 0.1, **p < 0.05). Nrate treatment levels were: 0, 202, and 269 kg N/ha.
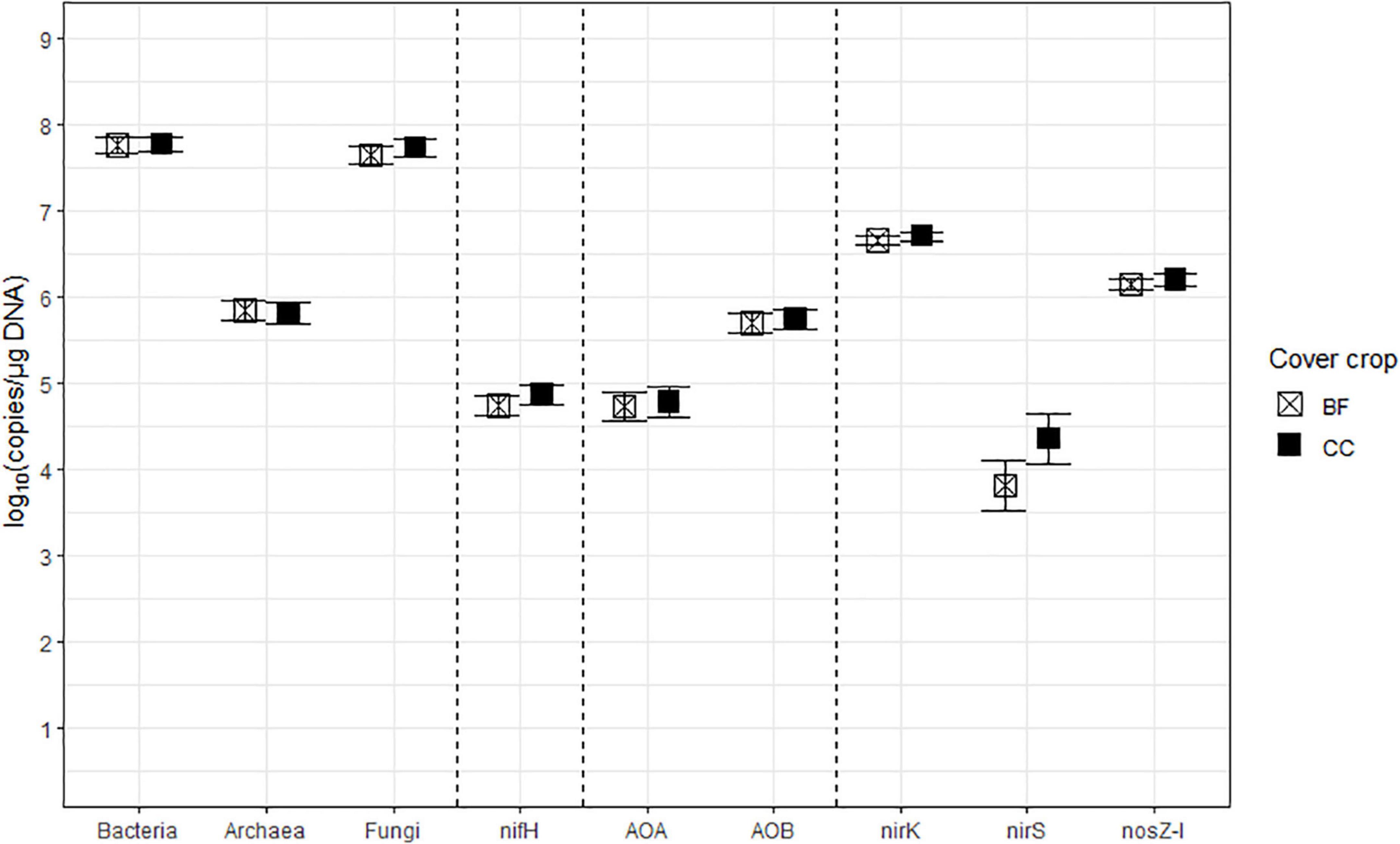
Figure 3. The treatment means of soil microbial marker genes in log10 (copies/μg DNA), including bacterial (Bacteria) and archaeal (Archaea) 16S rRNA, fungal ITS region (Fungi), nifH, archaeal (AOA) and bacterial (AOB) amoA, nirK, nirS, and nosZ-I separated by cover cropping (CC), with their standard errors of the mean as whiskers. The CC treatment levels were bare fallow control (BF) and hairy vetch and cereal rye cover crop mixture (CC).
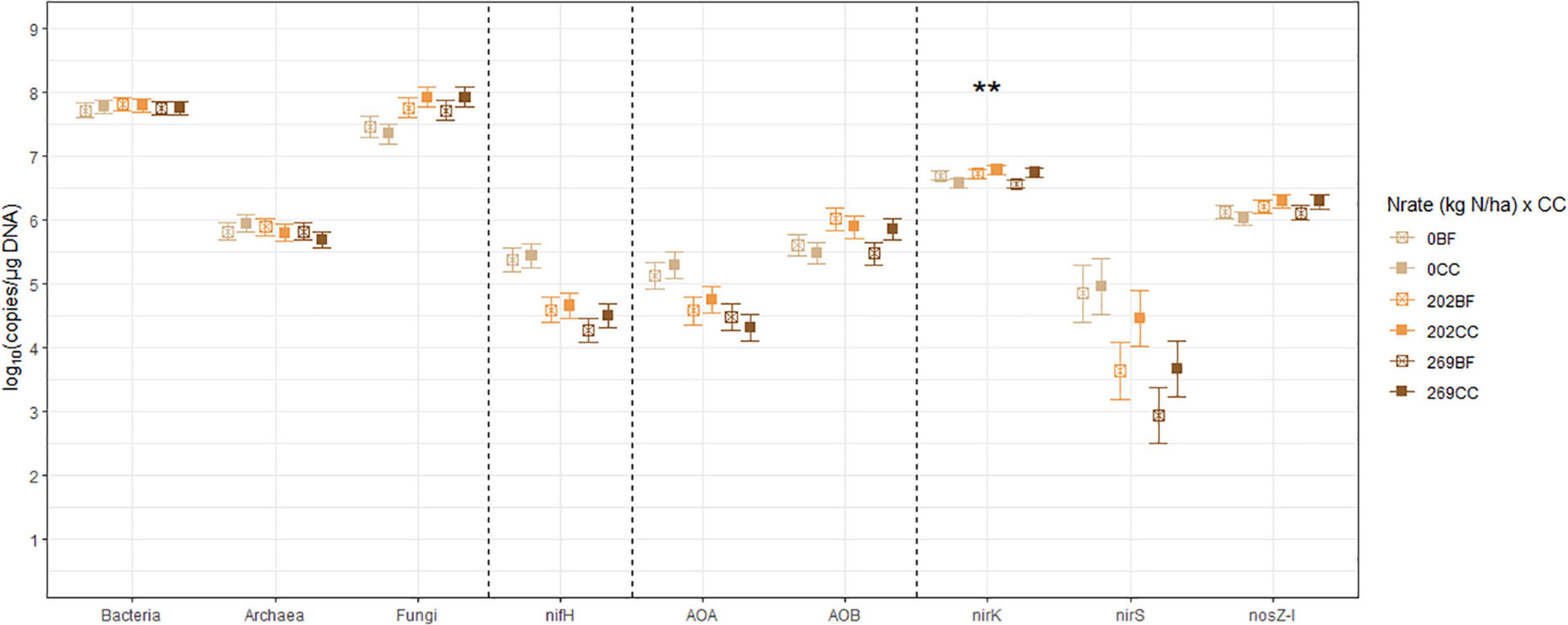
Figure 4. The treatment means of soil microbial marker genes in (log10 copies/μg DNA), including bacterial (Bacteria) and archaeal (Archaea) 16S rRNA, fungal ITS region (Fungi), nifH, archaeal (AOA) and bacterial (AOB) amoA, nirK, nirS, and nosZ-I separated by the interactions between N fertilization rate (Nrate) and cover cropping (CC), with their standard errors of the mean as whiskers. The asterisks indicate the probability value of the treatment effect from analysis of variance (**p < 0.05). Nrate treatment levels were: 0, 202, and 269 kg N/ha. CC treatment levels were bare fallow control (BF) and hairy vetch and cereal rye cover crop mixture (CC).
The nitrogenase-coding nifH gene counts were used to infer the abundance of the N-fixing community, and they ranged between 7.41 × 103 and 7.41 × 105 copies/μg DNA and averaged at 6.31 × 104 copies/μg DNA. This gene had a statistically significant (p = 0.0278) Nrate main effect, where its means decreased with N fertilization. The AOA and AOB amoA gene counts were each used as proxies for the abundances of AOA and AOB communities, respectively. The AOA amoA counts ranged between 7.08 × 103 and 6.46 × 105 copies/μg DNA and averaged at 5.62 × 104 copies/μg DNA. The bacterial amoA gene counts ranged between 3.98 × 104 and 3.54 × 106 copies/μg DNA, with an average of 5.25 × 105 copies/μg DNA. AOA amoA had a statistically significant (p = 0.0148) Nrate main effect and decreased with N fertilization. Statistically marginal (p = 0.1094) N rate and CC interaction effects were detected for AOB amoA. Within BF, the mean abundance was greater with N202 than N269, with N0 being intermediate, while the means did not differ by N rate within CC; its mean count increased with CC when fertilized at N269. The nirK and nirS genes that code NO2– reductase were both used as proxies for the NO2–-reducing denitrifier community. The nirK counts ranged between 2.51 × 106 and 1.15 × 107 copies/μg DNA, with an average of 4.79 × 106 copies/μg DNA. This gene had a statistically significant (p = 0.0404) Nrate and CC interaction effect. The mean counts of nirK increased with N fertilization within CC, and they were greater with N202 than N269 within BF, with N0 being intermediate. The nirS count ranged between 81 and 3.89 × 105 copies/μg DNA and had an average of 1.20 × 104 copies/μg DNA. It had a statistically significant (p = 0.0363) Nrate main effect, so that its mean counts decreased sequentially with higher N rates, with N202 being intermediate. Finally, the N2O reductase coding nosZ-I gene, used to represent the N2O-reducing community, ranged between 6.03 × 105 and 3.31 × 106 copies/μg DNA with an average of 1.48 × 106 copies/μg DNA. This gene did not have any statistically significant treatment effect.
Correlations Among Gene Counts, Soil Properties, and Potential Nitrification Rate and Potential Denitrification Rate
Supplementary Table 1 shows the Spearman’s rank correlation matrix with coefficients among bacterial and archaeal 16S rRNA, and fungal ITS regions, the six N-cycling functional genes, selected soil properties (CEC, pH, SOM, Bd, WAS, NH4+, NO3–, Pbray, and K), PNR, and PDR. This matrix is also visualized as a heatmap in Figure 5. We observed five very strong (|>0.8|), twelve strong (|0.6–0.8|), and 24 moderate (|0.4–0.6|) associations. The bacterial 16S rRNA gene counts had a very strong positive association with those of archaea, followed by moderate positive association with WAS and negative ones with Bd and K. Archaeal 16S rRNA associated moderately positively with archaeal amoA and WAS, and negatively with Bd. Fungal ITS region counts are associated strongly and positively with AOB amoA and nosZ-I, moderately positively with nirK, and moderately negatively with soil pH. The N-fixing nifH a had strong positive association with AOA amoA, nirS, pH, and Pbray, and moderately with CEC and K. AOA amoA had a very strong positive association with nirS, and moderate positive correlations with pH and Pbray. AOB amoA had strong positive associations with nirK and moderately positive associations with nosZ-I and NO3–. Denitrifying nirK gene counts are associated very strongly and positively with nosZ-I, while nirS is associated positively with pH strongly, and with CEC, Pbray, and K moderately. Besides the above-mentioned relationships, nosZ-I did not have further associations. Other than those already mentioned, CEC is very strongly and positively associated with pH and K, strongly with Pbray, and moderately with Bd and PNR. Soil pH is positively and strongly associated with K and moderately with Pbray and PNR, while having a moderate and negative association with NO3–. Meanwhile, SOM had a positive moderate association with NH4+. Bd had a very strong negative correlation with WAS. Pbray and K had a strong positive correlation, and K had a positive moderate association with PNR. In addition, there were 16 statistically significant (p < 0.05) but weak (p < |0.4|) correlations (Supplementary Table 1).
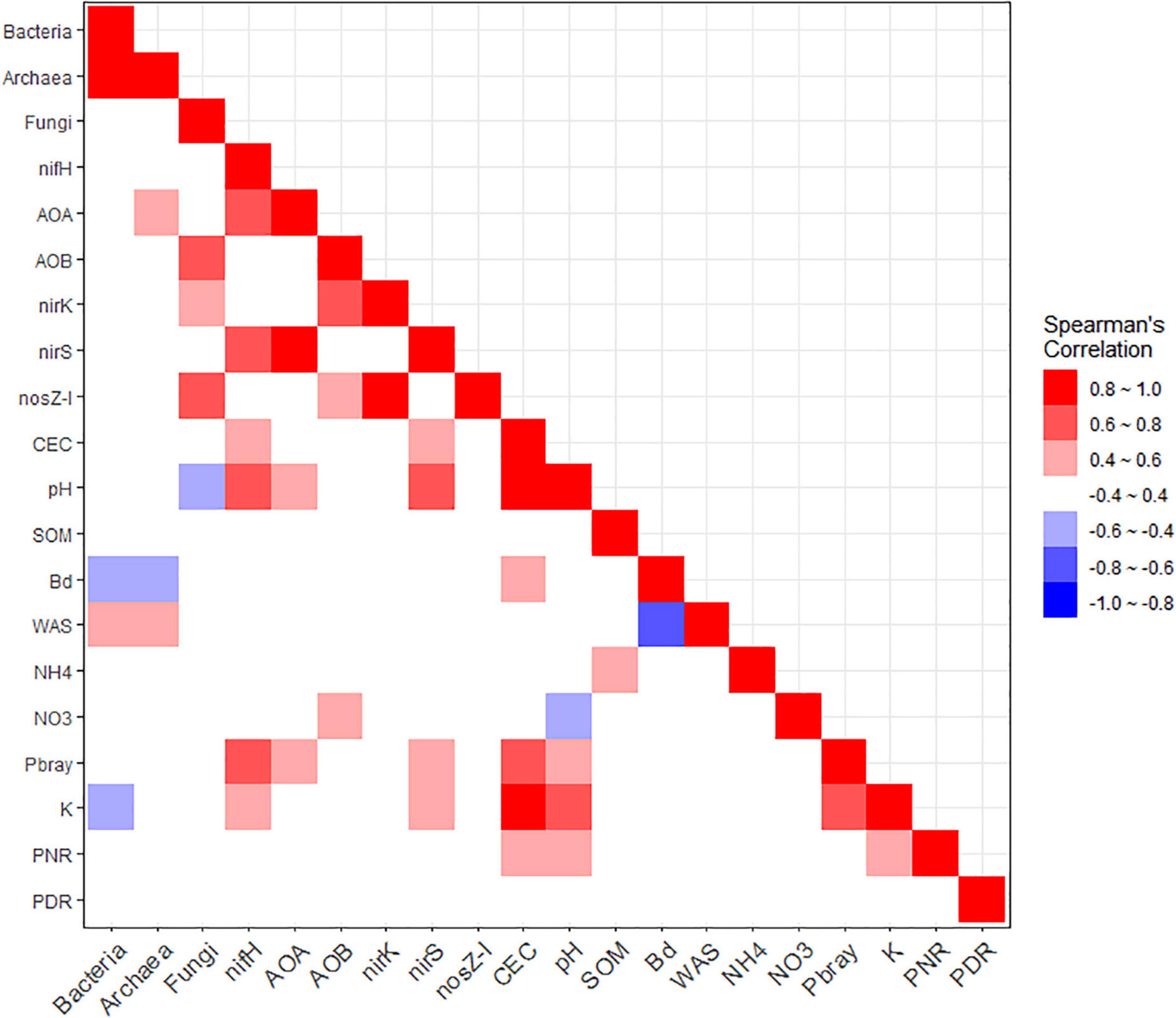
Figure 5. Heatmap depicting the matrix of Spearman’s rank correlation coefficients among the soil microbial marker genes, soil properties, and potential nitrification (PNR) and denitrification (PDR) rates. Marker genes included bacterial (Bacteria) and archaeal (Archaea) 16S rRNA, fungal ITS region (Fungi), nifH, archaeal (AOA) and bacterial (AOB) amoA, nirK, nirS, and nosZ-I. Soil properties included cation exchange capacity (CEC, cmolc/kg), pH, soil organic matter (SOM,%), bulk density (Bd, Mg/m3), water aggregate stability (WAS,%), ammonium (NH4, mg/kg), nitrate (NO3, mg/kg), available phosphorus (Pbray, mg/kg), and extractable potassium (K, mg/kg). The red and blue hues each indicate positive and negative associations, respectively. Higher color saturation indicates greater absolute values of Spearman’s rank correlation coefficients.
Discussion
This study observed that CC had little impact on the soil environment of an intensely managed and simplified cropping system; thus, the soil properties remained stable since Kim et al. (2022b) before CC introduction. Higher N rates decreased CEC, which Kim et al. (2022b) explained, was due to the increasing soil acidity from the nitrifying N fertilizers and increased crop root uptakes (Tang and Rengel, 2003), leading to loss of exchange sites within soil particles (Barak et al., 1997). N fertilization also significantly depleted the soil available P and K via increased crop uptake. Before CC, Kim et al. (2022b) reported a statistically significant N rate main effect on Bd, but this study could not detect this. These contrasting results may owe to the thicker layer of topsoil analyzed in this study (30 cm) than in the previous study (15 cm). Similar to Kim et al. (2022b), SOM and WAS did not differ significantly by N rate. Indeed, Necpálová et al. (2014) estimated that statistically detectable changes in SOM of resilient Mollisols like in this study would take several decades. As for CC, only Kim et al. (2022c) found that CC decreased NO3– in this experimental site. Similar results have been observed by Acuña and Villamil (2014), also in Illinois, where CC only affected NO3– among various soil properties. Before CC, Kim et al. (2022b) demonstrated soil acidification and high nutrient availability as the main results of long-term N fertilization and corn monoculture over fertile United States Midwestern soils. Overall, in addition to the innate resilience of the Mollisols of this region, this study suggests that these soils may have become resistant to conservation practices after long-term exposure to disruptive practices. Thus, desired improvements from deploying CC may take more time and effort. The marginal CC effects on N-cycling communities and their functionality in this study may have stemmed from this resistant soil environment.
Consistent with Huang et al. (2019), this study found a statistically significant N rate’s main effect on fungal ITS counts, while bacteria and archaea did not respond to treatments. In both studies, fungal ITS region counts increased with N fertilization. This result agrees with the fungal species richness that also increased with N input in this site (Kim et al., 2022c). The fungal community may have benefited from N fertilization due to the stoichiometric differences between fungi and bacteria. Because fungal biomass has a greater C:N ratio than bacteria, fungi might benefit from N fertilizers increasing the returns of corn residues high in C:N ratio (Strickland and Rousk, 2010). Furthermore, fungi generally tolerate soil acidity better than bacteria, which allows them to take advantage of the acidifying soils from prolonged N fertilization (Strickland and Rousk, 2010; Kim et al., 2022c). Indeed, the ITS counts correlated negatively with soil pH. Another study in Illinois Mollisols on the effects of crop rotation and tillage also showed similar results, where only fungal abundance responded to crop rotation, due to soil acidification from corn monoculture (Behnke et al., 2020). Meanwhile, the bacterial and archaeal 16S counts had a strong monotonic relationship and correlated negatively and positively with Bd and WAS, respectively. Indeed, less dense soil and more stable soil aggregates are associated with higher soil carbon (C; Trivedi et al., 2017), wherein the soil’s microbial growth depends on (Kamble and Bååth, 2018). These results suggest that bacterial and archaeal abundances might depend more on these soil’s physical properties and perhaps soil C, than on N fertilizers and CC.
Responses of the Soil N-Cycling Functional Genes
Detrimental Effect of N Fertilization on N-Fixing Community
As hypothesized, nifH decreased in abundance with N fertilization, consistent with the report before CC introduction (Huang et al., 2019). Similarly, a continuous wheat study of 22 years on the effects of N and P fertilization in Saskatchewan Mollisols also found that N fertilization decreased nifH and bacterial N-fixation (Li et al., 2020). Also, in a semi-arid grassland study in China, testing 3 years of N application (0, 25, 50, and 100 kg N/ha), the authors found that nifH initially increased in abundance at a low N rate but decreased sequentially as the N rate increased (Liao et al., 2021). High N availability from fertilizers mitigates the plant dependence on microbial N-fixers and disincentivizes their recruitment, which may explain why nifH decreased with higher N rates in this study (Liao et al., 2021). In addition, soil acidification is known to repress N-fixation (Bakari et al., 2020; Zhang et al., 2021), which also agrees with its strong positive association with soil pH. Therefore, soil acidification from excessive N fertilization could also be a factor. Kim et al. (2022c) reported that the genus Mesorhizobium, which includes nodule-forming N-fixers, increased in abundance with CC. Accordingly, this study hypothesized that this would reflect on the nifH counts. However, CC was not a significant source of variation for nifH. Indeed, Mesorhizobium also includes NO2– reducers (Okada et al., 2005). Perhaps, Kim et al. (2022c) detected this genus not as an indicator of N-fixers but of denitrifiers. There have been studies reporting either positive (Castellano-Hinojosa et al., 2022) or insignificant (Hu et al., 2021) CC effects on nifH. The authors explained that the non-legume CC in the mixture depletes soil N, thus, encouraging N-fixation to meet the legume’s N demand (Castellano-Hinojosa et al., 2022). If this also applies to corn monoculture, this study might have not detected a significant CC effect on nifH because heavy fertilization overrode the N depletion by non-legume CC.
Ammonia-Oxidizing Archaea and Ammonia-Oxidizing Bacteria Responded Differently to Treatments (amoA)
In this study, a higher N rate sequentially decreased the abundance of AOA amoA. This agreed with Kim et al. (2022c), who also found that N fertilization decreased the abundance of Nitrososphaera, a genus, including neutrophilic AOA. Furthermore, AOA amoA correlated positively with soil pH. These results implied that AOA responded negatively to soil acidification, to which they may have contributed as nitrifiers themselves (Bolan and Hedley, 2003). Sun et al. (2019) studied the relationships between various soil properties and nitrifier communities in long-term fertilized, slightly acidic to neutral Chinese Vertisols (Wei et al., 2018). Similar to this study, the authors reported that AOA correlated the most with soil pH and decreased with soil acidification. AOA may adapt and form communities of distinct compositions at different soil pH (Hirsch and Mauchline, 2015). Thus, responses of these distinct AOA communities to changes in the soil conditions may vary by the initial soil pH they adapted to. For example, AOA is better adapted to acidic soils than AOB (Zhang et al., 2012) and can respond positively to N inputs in this condition (Gubry-Rangin et al., 2010). Yet, another AOA community that is initially adapted to more neutral soils may respond negatively to N inputs, like on Sun et al. (2019). Therefore, this study further suggested that soil acidity may have a detrimental impact on the AOA community that adapted to the slightly acidic to neutral soils typical to the US Midwest region. Meanwhile, CC did not have a significant impact on AOA amoA. This also agrees with Kim et al. (2022c), who reported no AOA, as indicators, is associated with CC. Castellano-Hinojosa et al. (2022) observed that legume and non-legume CC mixture increased AOA amoA in the citrus orchard, but this may not be the case for corn monoculture. A greenhouse study on Argentinian Mollisols by Allegrini et al. (2021) compared the nitrifier community responses to different CC termination methods. They found that AOA amoA increased with mechanical CC termination compared to chemical (glyphosate) termination and control without CC, which they explained that glyphosate can hamper the growth of AOA (Allegrini et al., 2021). Perhaps, chemical CC termination masked CC effects on AOA in this study as well, which might be worthwhile investigating in a field study.
According to the findings of Huang et al. (2019), this study hypothesized that AOB amoA will increase in abundance with higher N rates. Instead, the mean separations between fertilized and unfertilized control within CC were not statistically significant for AOB amoA in this study. Moreover, under bare fallow, AOB amoA mean counts did not differ statistically and significantly between fertilized plots and the unfertilized control. Overall, these results suggested that the AOB of this system was not as sensitive as AOA to N fertilization and its subsequent soil acidification. Indeed, past reports showed that AOB responds more sensitively to nutrient availability than soil pH. The meta-analysis by Ouyang et al. (2018) showed that AOB amoA, consistently, had positive effect sizes with N fertilization regardless of soil pH, while AOA amoA had an insignificant effect size below pH 6. Sun et al. (2019) also reported that soil acidification rather increased AOB abundance, although at the cost of their diversity. A microcosm study by Hink et al. (2018) showed that while AOA preferred a low rate of N inputs from organic sources, AOB increased in abundance with high rate of inorganic N inputs, showing that AOB may better exploit the inorganic N from fertilizers than AOA. Sun et al. (2019) suggested that the AOB community may occupy broader niches than AOA because they have more ecophysiological diversity. Perhaps, this ecological versatility allows AOB to adapt to acidifying soils and maintain their abundance. Also, Sun et al. (2019) showed that AOB significantly correlated with soil C. Thus, labile C from CC as root exudates and residues could explain why AOB amoA was more abundant with CC compared to bare fallow when fertilized at the highest N rate (Wang et al., 2021).
Despite a significant decrease in AOA amoA with a higher N rate, PNR did not differ by treatments in this study. While reports on PNR from similar cropping systems are scarce, a study from Northeastern Chinese Alfisols under soybean-corn-corn rotation found that PNR decreased with higher N rates (Xu et al., 2012). Another study from Kentucky Alfisols with corn monoculture and grass CC without bare fallow control found that PNR increase with N fertilization (Liu et al., 2017). Thus, currently, there is no consensus on how N fertilization affects PNR. The N rate effect on PNR may have not been statistically significant because stable AOB population size may have masked the decreasing contributions from AOA. Indeed, AOB amoA was 9.33 times more abundant than AOA amoA on average. Also, while AOA amoA abundance differed up to 15.13 times by treatment levels, AOB amoA only did so by 3.55 times. Likewise, the topsoil NO3– level had a statistically significant positive correlation with AOB amoA, suggesting that AOB could have mainly driven the nitrification in this system. Meanwhile, there currently is no report on how PNR responds to CC in systems comparable to this study. While this study found no significant effects, further research will have to accumulate on this relationship.
Distinct Responses Between nirK- and nirS-Harboring Nitrite-Reducing Groups
In this study, nirK and nirS responded differently to the treatments. While N fertilization consistently decreased nirS, it slightly increased nirK within CC and had no difference from unfertilized control within bare fallow. These responses of nirK and nirS were analogous to AOB and AOA amoA, respectively, but the nirK differences by N rates within CC were statistically significant. Indeed, past reports agree with this study that N fertilization effects on nirK tend to range from insignificant to positive, while they are negative for nirS (Yin et al., 2014; Yang et al., 2017). For example, Yang et al. (2017) on a wheat-corn rotation system over alkaline soils of northern China reported that a higher N rate increased the abundances of nirK but decreased that of nirS. Yin et al. (2014) on soybean-corn-corn rotation observed that inorganic fertilizers decreased the abundances of nirS but had no impact on nirK. These genes may be responding differently to each other because the denitrifier guilds that each harbor adapt differently to soil acidification, similar to the amoA-nitrifiers discussed above. Indeed, this study observed that soil pH correlated strongly and positively with nirS, but not with nirK, which agreed with the past reports (Čuhel et al., 2010; Yang et al., 2017; Bowen et al., 2018). For example, Čuhel et al. (2010), on the effects of soil pH changes in pasture systems over Czech Mollisols, showed that nirS increased in abundance from acidic to alkaline soils, while nirK did not differ by soil acidity. Therefore, soil acidification from N fertilization in this study’s system may negatively impact the nirS-harboring denitrifiers. Also, Yang et al. (2017) showed that the abundance of nirS correlated significantly with the soil pH, while nirK did so with soil inorganic N levels. Thus, the nirK-harboring denitrifiers might be better adapted to N fertilizers, although this was only detected within CC in this study. Furthermore, fungi hold significant shares of the nirK community. For example, Xu et al. (2019) found that the fungal nirK-harboring community may contribute up to 50% of the N2O production during corn crops over black soils of Northeast China. Indeed, the present study observed statistically significant monotonic relationships among fungal ITS and nirK. As discussed earlier, fungi have better tolerance for soil acidity, which could have factored into nirK having less sensitivity to soil pH (Bowen et al., 2018).
This study hypothesized that CC would increase nirK but have no effect on nirS, based on the bioindicators of Kim et al. (2022c) that included nirK-harboring NO2–-reducers (Mesorhizobium and Luteimonas), but not nirS (Falk et al., 2010; Yin et al., 2014; Zhong et al., 2020). This hypothesis somewhat held true as nirS did not respond to CC, while nirK increased in abundance with CC under the context of N fertilization. However, past studies reported that CC tends to benefit both genes. The microcosm study by Wang et al. (2021) showed that ryegrass CC increased the abundances of nirK and nirS regardless of N rate (0 to 200 kg N/ha). They attributed these results to the labile C from CC root exudates and residues that generally promote the soil microbial community, including the denitrifiers. Similarly, Castellano-Hinojosa et al. (2022), on citrus orchards, found that CC increased the abundance of both genes. Still, their systems are very different from that of this study. Thus, the primary information on how nirK and nirS respond to CC within the typical corn-based cropping systems is critically lacking. In this study, nirS did have higher mean counts with CC than bare fallow control, but the CC main effect was still not statistically significant. Overall, this study found that nirK of this system may increase with CC when heavily fertilized, which also agreed with the bioindicators found by Kim et al. (2022c). However, whether nirS also responds positively to CC in this system will need further investigation.
Similar to nitrification, PDR did not have a statistically significant treatment effect, yet this result contrasts with past reports on PDR that increased with N fertilization and CC (Foltz et al., 2021; Li et al., 2022). Similar to amoA communities, nirK was much more abundant (nearly 400 times) than nirS, which decreased significantly with N fertilization. Also, nirK was more resistant to change than nirS, only differing up to 1.55 times in abundance by treatment levels compared to nirS that differed more than a hundred times. Maeda et al. (2017) reported that nirK-denitrifiers were more responsible for N2O emission in a compost pile despite their smaller number than nirS. Meanwhile, Liang et al. (2021) showed that denitrification was associated more with the nirS community because it was more responsive to climate factors and soil pH. Therefore, the lack of statistically significant responses from PDR could be reflecting both the dominant nirK contribution to PDR and sensitive changes in nirS communities to the treatments. Yet, this is beyond the insight that PDR can provide overall; moreover, nitrifier denitrification complicates this even more. Therefore, further investigation should determine how much each nitrifier and denitrifiers contributes to PDR, by using inhibitors of specific groups, for example.
N2O-Reducing Denitrifiers Unresponsive to N Fertilization and Cover Cropping (nosZ-I)
Consistent with past reports from this study’s site (Huang et al., 2019), the treatments had no statistically significant effect on nosZ-I. Conversely, Kim et al. (2022c) identified Gemmatirosa as a nosZ-harboring bioindicator that decreased with CC. Indeed, the denitrifiers in this genus belong to the nosZ-II clade (Xu et al., 2020), implying that nosZ-I and nosZ-II may respond differently to treatments in this system. This could be because these denitrifier groups each perform different soil functions and occupies distinct niches (Shan et al., 2021). Bowen et al. (2018) reported that the nosZ-II clade might be more sensitive to soil properties like pH than nosZ-I. Also, Wang et al. (2021) showed that the presence of ryegrass CC and high N rate increased the abundance of the nosZ-I clade, while nosZ-II did not respond to the treatments. Possibly, nosZ-II may have responded to N fertilizers and CC in this study, but this gene was not analyzed in this study. Therefore, future research should include nosZ-II for a more complete picture of how the N2O-reducers respond to CC in this system. Meanwhile, although high-rate N fertilization might not change the abundance of nosZ, soil acidification can interfere with the functionality of its product, N2O reductase, potentially exacerbating N2O emission (Qu et al., 2014). Thus, further investigation on the transcripts or enzyme activity of these genes might be necessary to determine how N2O reduction itself responds to CC in this system.
Conclusion
This study is the first to determine how the soil N-cycling microbial community responds to cover crops in highly fertile, yet intensely manage cropping systems that are common to critical agricultural regions like the United States Midwest. Overall, the results on functional genes, the measured PNR and denitrification rates, and soil properties were consistent with each other without unexplainable discrepancies. Excessive N fertilization disrupts the N-fixer, AOA, and nirS-denitrifier communities, likely due to their sensitivity to soil acidification and a disincentivized plant-symbiosis for the N-fixers. Conversely, AOB and denitrifiers harboring nirK and nosZ-I responded subtly to N fertilization, likely because they are less sensitive to soil acidity or prefer high soil nutrient availability. Therefore, adaptation to soil acidification and nutrient availability from excessive N fertilization might be the discerning factor for the N-cycling communities in this system. Moreover, as in the cases of AOA amoA and nirK, some functional genes mirrored the previously reported genus-level bioindicators of the same site that are also known to harbor the respective genes. Thus, this study further supported the high-taxonomic resolution bioindicators as useful predictors of the soil microbial functions, although further investigation and reproduction of these results should follow. Despite these changes in the N-cycling communities, the enzyme assays did not respond to treatments, showing that overall functionality could be more resilient to changes. Contrary to the initial hypothesis, CC had a limited impact on the soil properties, N-cycling communities, and their functionality. This suggested that the soil environment and its N-cycling communities became resistant to changes after decades-long adaptation to consistent disruptions from heavy N fertilization and corn monocultures. Therefore, short-term CC may not be enough to improve heavily disrupted N-cycling communities. Further research should expand on this study with long-term CC, different cropping systems, and more functional genes for a more complete understanding of cover cropping as a sustainable practice to mitigate soil degradation and nutrient loss.
Data Availability Statement
The original contributions presented in this study are included in the article/Supplementary Material, further inquiries can be directed to the corresponding author.
Author Contributions
MV and NK: conceptualization, formal analysis. NK, CR, MZ, MV, and SR-Z: methodology. MV, SR-Z, and CR: resources. MV, MZ, CR, and NK: data curation. NK: visualization, writing—original draft preparation. MV and MZ: writing—review and editing. MV: supervision, project administration, and funding acquisition. All authors have read and agreed to the published version of the manuscript.
Funding
This research was funded by awards ILLU-802-978 and AG 2018-67019-27807, both from the United States Department of Agriculture, USDA-NIFA.
Conflict of Interest
The authors declare that the research was conducted in the absence of any commercial or financial relationships that could be construed as a potential conflict of interest.
Publisher’s Note
All claims expressed in this article are solely those of the authors and do not necessarily represent those of their affiliated organizations, or those of the publisher, the editors and the reviewers. Any product that may be evaluated in this article, or claim that may be made by its manufacturer, is not guaranteed or endorsed by the publisher.
Acknowledgments
We acknowledge Alvaro Hernandez and Mark Band from the Roy Carver Biotechnology Center for Functional Genomics lab at the University of Illinois at Urbana-Champaign for their assistance in creating the amplicon libraries. We would like to thank Greg Steckel and Marty Johnson for their contribution to managing the experimental plots, and to Gevan Behnke for his assistance with soil sampling and overall lab management. We would also like to thank Marco Allegrini for sharing his expertise in soil microbiology.
Supplementary Material
The Supplementary Material for this article can be found online at: https://www.frontiersin.org/articles/10.3389/fmicb.2022.926592/full#supplementary-material
Supplementary Table 1 | Spearman rank correlation coefficients for bacterial and archaeal 16S rRNA, fungal ITS, nifH, AOA and AOB amoA, nirK, nirS, nosZ-I, estimated cation exchange capacity (CEC), pH, soil organic matter (SOM), bulk density (Bd), water aggregate stability (WAS), ammonium (NH4), nitrate (NO3), available phosphorus (Pbray), potassium (K), and potential nitrification (PNR) and denitrification (PDR) rates. Statistically significant coefficients (p < 0.05) are in bold. Cells are colored based on their coefficients, with red being positive and blue being negative, and darker the color the greater the value.
Footnotes
- ^ https://www.blinc.com/resources/testing-methods (accessed on 20 February 2022).
References
Acuña, J. C. M., and Villamil, M. B. (2014). Short-term effects of cover crops and compaction on soil properties and soybean production in Illinois. Agron. J. 106, 860–870. doi: 10.2134/agronj13.0370
Allegrini, M., Morales, M. E., Villamil, M. B., and Zabaloy, M. C. (2021). Ammonia oxidizing prokaryotes respond differently to fertilization and termination methods in common oat’s rhizosphere. Front. Microbiol. 2021:12. doi: 10.3389/fmicb.2021.746524
Bakari, R., Mungai, N., Thuita, M., and Masso, C. (2020). Impact of soil acidity and liming on soybean (Glycine max) nodulation and nitrogen fixation in Kenyan soils. Acta Agric. Scand. B Soil Plant Sci. 70, 667–678. doi: 10.1080/09064710.2020.1833976
Barak, P., Jobe, B. O., Krueger, A. R., Peterson, L. A., and Laird, D. A. (1997). Effects of long-term soil acidification due to nitrogen fertilizer inputs in Wisconsin. Plant Soil. 197, 61–69. doi: 10.1023/A:1004297607070
Basche, A. D., Miguez, F. E., Kaspar, T. C., and Castellano, M. J. (2014). Do cover crops increase or decrease nitrous oxide emissions? A meta-analysis. J. Soil Water Conserv. 69, 471–482. doi: 10.2489/jswc.69.6.471
Behnke, G. D., Kim, N., Riggins, C. W., Zabaloy, M. C., Rodriguez-Zas, S. L., and Villamil, M. B. (2022). A longitudinal study of the microbial basis of nitrous oxide emissions within a long-term agricultural experiment. Front. Agron. 2022:4. doi: 10.3389/fagro.2022.833338
Behnke, G. D., Zabaloy, M. C., Riggins, C. W., Rodríguez-Zas, S., Huang, L., and Villamil, M. B. (2020). Acidification in corn monocultures favor fungi, ammonia oxidizing bacteria, and nirK-denitrifier groups. Sci. Total Environ. 720:137514. doi: 10.1016/j.scitotenv.2020.137514
Blake, G. R., and Hartge, K. H. (1986). “Bulk Density,” in Methods of Soil Analysis: Part 1 Physical and Mineralogical Methods, 2 Edn, ed. A. Klute (Madison, WI: American Society of Agronomy), 363–375. doi: 10.2136/sssabookser5.1.2ed.c13
Blanco-Canqui, H., Shaver, T. M., Lindquist, J. L., Shapiro, C. A., Elmore, R. W., Francis, C. A., et al. (2015). Cover crops and ecosystem services: Insights from studies in temperate soils. Agron J. 107, 2449–2474. doi: 10.2134/agronj15.0086
Blesh, J., Vandusen, B. M., and Brainard, D. C. (2019). Managing ecosystem services with cover crop mixtures on organic farms. Agron J. 111, 826–840. doi: 10.2134/agronj2018.06.0365
Bolan, N. S., and Hedley, M. J. (2003). “Role of carbon, nitrogen, and sulfur cycles in soil acidification,” in Handbook of Soil Acidity, ed. Z. Rengel (Boca Raton, FL: CRC Press), 29–56. doi: 10.1016/j.scitotenv.2019.134418
Bowen, H., Maul, J. E., Poffenbarger, H., Mirsky, S., Cavigelli, M., and Yarwood, S. (2018). Spatial patterns of microbial denitrification genes change in response to poultry litter placement and cover crop species in an agricultural soil. Biol. Fertil. Soils. 54, 769–781. doi: 10.1007/s00374-018-1301-x
Castellano-Hinojosa, A., Martens-Habbena, W., Smyth, A. R., Kadyampakeni, D. M., and Strauss, S. L. (2022). Short-term effects of cover crops on soil properties and the abundance of N-cycling genes in citrus agroecosystems. Appl. Soil Ecol. 172:104341. doi: 10.1016/j.apsoil.2021.104341
Chen, Y., Xu, Z., Hu, H., Hu, Y., Hao, Z., Jiang, Y., et al. (2013). Responses of ammonia-oxidizing bacteria and archaea to nitrogen fertilization and precipitation increment in a typical temperate steppe in Inner Mongolia. Appl. Soil Ecol. 68, 36–45. doi: 10.1016/j.apsoil.2013.03.006
Colman, D. R., Thomas, R., Maas, K. R., and Takacs-Vesbach, C. D. (2015). Detection and analysis of elusive members of a novel and diverse archaeal community within a thermal spring streamer consortium. Extremophiles 19, 307–313. doi: 10.1007/s00792-014-0715-0
Crawford, J. W., Deacon, L., Grinev, D., Harris, J. A., Ritz, K., Singh, B. K., et al. (2012). Microbial diversity affects self-organization of the soil microbe system with consequences for function. J. R. Soc. Interface 9, 1302–1310. doi: 10.1098/rsif.2011.0679
Čuhel, J., Šimek, M., Laughlin, R. J., Bru, D., Chèneby, D., Watson, C. J., et al. (2010). Insights into the effect of soil pH on N2O and N2 emissions and denitrifier community size and activity. Appl. Environ. Microbiol. 76, 1870–1878. doi: 10.1128/AEM.02484-09
Drury, C., Hart, S. C., and Yang, X. (2008). Nitrification techniques for soils. Soil Sampl. Methods Anal. 2008, 495–513.
Fagodiya, R. K., Pathak, H., Kumar, A., Bhatia, A., and Jain, N. (2017). Global temperature change potential of nitrogen use in agriculture: a 50-year assessment. Sci. Rep. 7:44928. doi: 10.1038/srep44928
Falk, S., Liu, B., and Braker, G. (2010). Isolation, genetic and functional characterization of novel soil nirK-type denitrifiers. Syst. Appl. Microbiol. 33, 337–347. doi: 10.1016/j.syapm.2010.06.004
Fernández, F. G., and Hoeft, R. G. (2009). Managing soil pH and crop nutrients. Illin. Agron. Handbook 24, 91–112.
Fierer, N., Jackson, J. A., Vilgalys, R., and Jackson, R. B. (2005). Assessment of soil microbial community structure by use of taxon-specific quantitative PCR assays. Appl. Environ. Microbiol. 71, 4117–4120. doi: 10.1128/AEM.71.7.4117-4120.2005
Foltz, M. E., Kent, A. D., Koloutsou-Vakakis, S., and Zilles, J. L. (2021). Influence of rye cover cropping on denitrification potential and year-round field N2O emissions. Sci. Total Environ. 765:144295. doi: 10.1016/j.scitotenv.2020.144295
Gubry-Rangin, C., Nicol, G. W., and Prosser, J. I. (2010). Archaea rather than bacteria control nitrification in two agricultural acidic soils. FEMS Microbiol. Ecol. 74, 566–574. doi: 10.1111/j.1574-6941.2010.00971.x
Hatfield, J. L., Wright-Morton, L., and Hall, B. (2018). Vulnerability of grain crops and croplands in the Midwest to climatic variability and adaptation strategies. Clim. Change 146, 263–275. doi: 10.1007/s10584-017-1997-x
Henry, S., Baudoin, E., López-Gutiérrez, J. C., Martin-Laurent, F., Brauman, A., and Philippot, L. (2004). Quantification of denitrifying bacteria in soils by nirK gene targeted real-time PCR. J. Microbiol. Methods 59, 327–335. doi: 10.1016/j.mimet.2004.07.002
Henry, S., Bru, D., Stres, B., Hallet, S., and Philippot, L. (2006). Quantitative detection of the nosZ gene, encoding nitrous oxide reductase, and comparison of the abundances of 16S rRNA, narG, nirK, and nosZ genes in soils. Appl. Environ. Microbiol. 72, 5181–5189. doi: 10.1128/AEM.00231-06
Hink, L., Gubry-Rangin, C., Nicol, G. W., and Prosser, J. I. (2018). The consequences of niche and physiological differentiation of archaeal and bacterial ammonia oxidisers for nitrous oxide emissions. ISME J. 12, 1084–1093. doi: 10.1038/s41396-017-0025-5
Hirsch, P. R., and Mauchline, T. H. (2015). “The Importance of the Microbial N Cycle in Soil for Crop Plant Nutrition,” in Adv Appl Microbiol, eds S. Sariaslani and G. M. Gadd (Cambridge, MA: Academic Press), 45–71. doi: 10.1016/bs.aambs.2015.09.001
Hu, J., Jin, V. L., Konkel, J. Y. M., Schaeffer, S. M., Schneider, L. G., Debruyn, J. M., et al. (2021). Soil health management enhances microbial nitrogen cycling capacity and activity. mSphere 6, e1237–e1220. doi: 10.1128/mSphere.01237-20
Huang, L., Riggins, C. W., Rodríguez-Zas, S., Zabaloy, M. C., and Villamil, M. B. (2019). Long-term N fertilization imbalances potential N acquisition and transformations by soil microbes. Sci. Total Environ. 691, 562–571. doi: 10.1016/j.scitotenv.2019.07.154
IL-EPA, Il-Doa, and Extension, U. (2015). Illinois Nutrient Loss Reduction Strategy (INLRS). Springfield, IL: Il-Epa.
Illinois State Water Survey (2010). Illinois climate normals. Champaign, IL: Illinois State Water Survey.
Kamble, P. N., and Bååth, E. (2018). Carbon and nitrogen amendments lead to differential growth of bacterial and fungal communities in a high-pH soil. Pedosphere 28, 255–260. doi: 10.1016/S1002-0160(18)60014-1
Kandeler, E., Deiglmayr, K., Tscherko, D., Bru, D., and Philippot, L. (2006). Abundance of narG, nirS, nirK, and nosZ genes of denitrifying bacteria during primary successions of a glacier foreland. Appl. Environ. Microbiol. 72, 5957–5962. doi: 10.1128/AEM.00439-06
Kim, N., Behnke, G. D., and Villamil, M. B. (2022b). Characterization of Mollisols after long-term N fertilization at successive rates in continuous and rotated corn systems. Agronomy 12:625. doi: 10.3390/agronomy12030625
Kim, H., Lee, D. K., Voigt, T. B., Tian, G., and Yannarell, A. C. (2022a). Agricultural practices of perennial energy crops affect nitrogen cycling microbial communities. Appl. Soil Ecol. 172:104366. doi: 10.1016/j.apsoil.2021.104366
Kim, N., Riggins, C. W., Zabaloy, M. C., Allegrini, M., Rodríguez-Zas, S., and Villamil, M. B. (2022c). High-resolution indicators of soil microbial responses to N fertilization and cover cropping in corn monoculture. Agronomy 12:954. doi: 10.3390/agronomy12040954
Lehmann, J., Bossio, D. A., Kögel-Knabner, I., and Rillig, M. C. (2020). The concept and future prospects of soil health. Nat. Rev. Earth Env. 1, 544–553. doi: 10.1038/s43017-020-0080-8
Li, L., Yang, M., Li, J., Roland, B., Du, Z., and Wu, D. (2022). Potential denitrification activity response to long-term nitrogen fertilization - A global meta-analysis. J. Cleaner Product. 336:130451. doi: 10.1016/j.jclepro.2022.130451
Li, Y., Tremblay, J., Bainard, L. D., Cade-Menun, B., and Hamel, C. (2020). Long-term effects of nitrogen and phosphorus fertilization on soil microbial community structure and function under continuous wheat production. Environ. Microbiol. 22, 1066–1088. doi: 10.1111/1462-2920.14824
Liang, Y., Wu, C., Wei, X., Liu, Y., Chen, X., Qin, H., et al. (2021). Characterization of nirS- and nirK-containing communities and potential denitrification activity in paddy soil from eastern China. Agric. Ecosyst. Environ. 319:107561. doi: 10.1016/j.agee.2021.107561
Liao, L., Wang, X., Wang, J., Liu, G., and Zhang, C. (2021). Nitrogen fertilization increases fungal diversity and abundance of saprotrophs while reducing nitrogen fixation potential in a semiarid grassland. Plant Soil. 465, 515–532. doi: 10.1007/s11104-021-05012-w
Littell, R. C., Milliken, G. A., Stroup, W. W., Wolfinger, R. D., and Oliver, S. (2006). SAS for Mixed Models. Cary, NC: SAS Institute.
Liu, S., Coyne, M. S., and Grove, J. H. (2017). Long-term tillage and nitrogen fertilization: consequences for nitrifier density and activity. Appl. Soil Ecol. 120, 121–127. doi: 10.1016/j.apsoil.2017.07.034
Lourenço, K. S., Costa, O. Y. D. A., Cantarella, H., and Kuramae, E. E. (2022). Ammonia-oxidizing bacteria and fungal denitrifier diversity are associated with N2O production in tropical soils. Soil Biol. Biochem. 166:108563. doi: 10.1016/j.soilbio.2022.108563
Maeda, K., Toyoda, S., Philippot, L., Hattori, S., Nakajima, K., Ito, Y., et al. (2017). Relative contribution of nirK- and nirS- bacterial denitrifiers as well as fungal denitrifiers to nitrous oxide production from dairy manure compost. Environ. Sci. Technol. 51, 14083–14091. doi: 10.1021/acs.est.7b04017
Malique, F., Ke, P., Boettcher, J., Dannenmann, M., and Butterbach-Bahl, K. (2019). Plant and soil effects on denitrification potential in agricultural soils. Plant Soil. 439, 459–474. doi: 10.1007/s11104-019-04038-5
Muhammad, I., Sainju, U. M., Zhao, F., Khan, A., Ghimire, R., Fu, X., et al. (2019). Regulation of soil CO2 and N2O emissions by cover crops: a meta-analysis. Soil Till Res. 192, 103–112. doi: 10.1016/j.still.2019.04.020
Necpálová, M., Anex, R. P., Kravchenko, A. N., Abendroth, L. J., Del Grosso, S. J., Dick, W. A., et al. (2014). What does it take to detect a change in soil carbon stock? A regional comparison of minimum detectable difference and experiment duration in the north central United States. J. Soil Water Conserv. 69, 517–531. doi: 10.2489/jswc.69.6.517
Okada, N., Nomura, N., Nakajima-Kambe, T., and Uchiyama, H. (2005). Characterization of the aerobic denitrification in Mesorhizobium sp. Strain NH-14 in comparison with that in related rhizobia. Microbes Environ. 20, 208–215. doi: 10.1264/jsme2.20.208
Ouyang, Y., Evans, S. E., Friesen, M. L., and Tiemann, L. K. (2018). Effect of nitrogen fertilization on the abundance of nitrogen cycling genes in agricultural soils: a meta-analysis of field studies. Soil Biol. Biochem. 127, 71–78. doi: 10.1016/j.soilbio.2018.08.024
Poly, F., Monrozier, L. J., and Bally, R. (2001). Improvement in the RFLP procedure for studying the diversity of nifH genes in communities of nitrogen fixers in soil. Res. Microbiol. 152, 95–103. doi: 10.1016/s0923-2508(00)01172-4
Qu, Z., Wang, J., Almøy, T., and Bakken, L. R. (2014). Excessive use of nitrogen in Chinese agriculture results in high N2O/(N2O+N2) product ratio of denitrification, primarily due to acidification of the soils. Glob Change Biol. 20, 1685–1698. doi: 10.1111/gcb.12461
R Core Team (2019). R: A language and environment for statistical computing. Vienna: R Foundation for Statistical Computing.
Reiss, E. R., and Drinkwater, L. E. (2020). Ecosystem service delivery by cover crop mixtures and monocultures is context dependent. Agron J. 112, 4249–4263. doi: 10.1002/agj2.20287
Rengel, Z. (2011). “Soil pH, Soil Health and Climate Change,” in Soil Health and Climate Change, eds B. P. Singh, A. L. Cowie, and K. Y. Chan (Berlin: Springer Berlin Heidelberg), 69–85. doi: 10.1007/978-3-642-20256-8_4
Rotthauwe, J.-H., Witzel, K.-P., and Liesack, W. (1997). The ammonia monooxygenase structural gene amoA as a functional marker: molecular fine-scale analysis of natural ammonia-oxidizing populations. Appl. Environ. Microbiol. 63, 4704–4712. doi: 10.1128/aem.63.12.4704-4712.1997
Schulte, E., and Hopkins, B. (1996). Estimation of soil organic matter by weight loss-on-ignition. Soil Org. Matter: Analy. Interpret. 46, 21–31. doi: 10.1016/s0048-9697(02)00135-3
Shan, J., Sanford, R. A., Chee-Sanford, J., Ooi, S. K., Löffler, F. E., Konstantinidis, K. T., et al. (2021). Beyond denitrification: The role of microbial diversity in controlling nitrous oxide reduction and soil nitrous oxide emissions. Glob. Change Biol. 27, 2669–2683. doi: 10.1111/gcb.15545
Socolar, Y., Goldstein, B. R., De Valpine, P., and Bowles, T. M. (2021). Biophysical and policy factors predict simplified crop rotations in the US Midwest. Environ. Res. Lett. 16:054045.
Soil Survey Staff, NRCS, and USDA (2020). Web Soil Survey. Available online at: http://websoilsurvey.sc.egov.usda.gov/ (accessed date July-31- 2019)
Strickland, M. S., and Rousk, J. (2010). Considering fungal:bacterial dominance in soils – Methods, controls, and ecosystem implications. Soil Biol. Biochem. 42, 1385–1395. doi: 10.1016/j.soilbio.2010.05.007
Sumner, M., and Miller, W. (1996). “Cation Exchange Capacity and Exchange Coefficients,” in Methods of Soil Analysis Part 3—Chemical Methods, eds D. Spark, A. Page, P. Helmke, R. Loeppert, P. Soltanpour, M. Tabatabai, et al. (Madison, WI: Soil Science Society of America, American Society of Agronomy), 1201–1229. doi: 10.2136/sssabookser5.3.c40
Sun, R., Myrold, D. D., Wang, D., Guo, X., and Chu, H. (2019). AOA and AOB communities respond differently to changes of soil pH under long-term fertilization. Soil Ecol. Lett. 1, 126–135. doi: 10.1007/s42832-019-0016-8
Tang, C., and Rengel, Z. (2003). “Role of plant cation/anion uptake ratio in soil acidification,” in Handbook of Soil Acidity, ed. Z. Rengel. (New York, NY: CEC Press). doi: 10.1201/9780203912317.ch3
Thapa, R., Mirsky, S. B., and Tully, K. L. (2018). Cover crops reduce nitrate leaching in agroecosystems: a global meta-analysis. J. Environ. Qual. 47, 1400–1411. doi: 10.2134/jeq2018.03.0107
Tittonell, P. (2014). Ecological intensification of agriculture—sustainable by nature. Curr. Opin. Env. Sustainab. 8, 53–61. doi: 10.1016/j.cosust.2014.08.006
Tourna, M., Freitag, T. E., Nicol, G. W., and Prosser, J. I. (2008). Growth, activity and temperature responses of ammonia-oxidizing archaea and bacteria in soil microcosms. Environ Microbiol. 10, 1357–1364. doi: 10.1111/j.1462-2920.2007.01563.x
Trivedi, P., Delgado-Baquerizo, M., Jeffries, T. C., Trivedi, C., Anderson, I. C., Lai, K., et al. (2017). Soil aggregation and associated microbial communities modify the impact of agricultural management on carbon content. Environ. Microbiol. 19, 3070–3086. doi: 10.1111/1462-2920.13779
Wang, H., Beule, L., Zang, H., Pfeiffer, B., Ma, S., Karlovsky, P., et al. (2021). The potential of ryegrass as cover crop to reduce soil N2O emissions and increase the population size of denitrifying bacteria. Eur. J. Soil Sci. 72, 1447–1461.
Wei, C., Gao, W., Whalley, W. R., and Li, B. (2018). Shrinkage characteristics of lime concretion black soil as affected by biochar amendment. Pedosphere 28, 713–725. doi: 10.1016/S1002-0160(18)60041-4
Wickham, H. (2016). Ggplot2: Elegant Graphics for Data Analysis. New York, NY: Springer-Verlag New York. doi: 10.1007/978-3-319-24277-4
Xu, H., Sheng, R., Xing, X., Zhang, W., Hou, H., Liu, Y., et al. (2019). Characterization of fungal nirK-containing communities and N2O emission from fungal denitrification in arable soils. Front. Microbiol. 2019:10. doi: 10.3389/fmicb.2019.00117
Xu, X., Liu, Y., Singh, B. P., Yang, Q., Zhang, Q., Wang, H., et al. (2020). NosZ clade II rather than clade I determine in situ N2O emissions with different fertilizer types under simulated climate change and its legacy. Soil Biol. Biochem. 150:107974. doi: 10.1016/j.soilbio.2020.107974
Xu, Y.-G., Yu, W.-T., Ma, Q., and Zhou, H. (2012). Responses of bacterial and archaeal ammonia oxidisers of an acidic luvisols soil to different nitrogen fertilization rates after 9 years. Biol. Fertil. Soils. 48, 827–837. doi: 10.1007/s00374-012-0677-2
Yang, Y., Zhao, J., Jiang, Y., Hu, Y., Zhang, M., and Zeng, Z. (2017). Response of bacteria harboring nirS and nirK genes to different N fertilization rates in an alkaline northern Chinese soil. Eur. J. Soil Biol. 82, 1–9.
Yin, C., Fan, F., Song, A., Li, Z., Yu, W., and Liang, Y. (2014). Different denitrification potential of aquic brown soil in Northeast China under inorganic and organic fertilization accompanied by distinct changes of nirS- and nirK-denitrifying bacterial community. Eur. J. Soil Biol. 65, 47–56. doi: 10.1016/j.ejsobi.2014.09.003
You, L., Ros, G. H., Chen, Y., Yang, X., Cui, Z., Liu, X., et al. (2022). Global meta-analysis of terrestrial nitrous oxide emissions and associated functional genes under nitrogen addition. Soil Biol. Biochem. 165:108523. doi: 10.1016/j.soilbio.2021.108523
Zhang, L.-M., Hu, H.-W., Shen, J.-P., and He, J.-Z. (2012). Ammonia-oxidizing archaea have more important role than ammonia-oxidizing bacteria in ammonia oxidation of strongly acidic soils. ISME J. 6, 1032–1045. doi: 10.1038/ismej.2011.168
Zhang, M., Zhang, X., Zhang, L., Zeng, L., Liu, Y., Wang, X., et al. (2021). The stronger impact of inorganic nitrogen fertilization on soil bacterial community than organic fertilization in short-term condition. Geoderma 382:114752. doi: 10.1016/j.geoderma.2020.114752
Zhong, X.-Z., Zeng, Y., Wang, S.-P., Sun, Z.-Y., Tang, Y.-Q., and Kida, K. (2020). Insight into the microbiology of nitrogen cycle in the dairy manure composting process revealed by combining high-throughput sequencing and quantitative PCR. Bioresour. Technol. 301:122760. doi: 10.1016/j.biortech.2020.122760
Keywords: N cycle genes, nosZ, amoA, nifH, nirK, nirS, maize (Zea mays L.), N fertilization
Citation: Kim N, Riggins CW, Zabaloy MC, Rodriguez-Zas SL and Villamil MB (2022) Limited Impacts of Cover Cropping on Soil N-Cycling Microbial Communities of Long-Term Corn Monocultures. Front. Microbiol. 13:926592. doi: 10.3389/fmicb.2022.926592
Received: 22 April 2022; Accepted: 11 May 2022;
Published: 10 June 2022.
Edited by:
Manoj Kumar Solanki, University of Silesia in Katowice, PolandReviewed by:
Mukesh Kumar Malviya, Guangxi Academy of Agricultural Sciences, ChinaOrlando Borras-Hidalgo, Qilu University of Technology, China
Copyright © 2022 Kim, Riggins, Zabaloy, Rodriguez-Zas and Villamil. This is an open-access article distributed under the terms of the Creative Commons Attribution License (CC BY). The use, distribution or reproduction in other forums is permitted, provided the original author(s) and the copyright owner(s) are credited and that the original publication in this journal is cited, in accordance with accepted academic practice. No use, distribution or reproduction is permitted which does not comply with these terms.
*Correspondence: María B. Villamil, dmlsbGFtaWxAaWxsaW5vaXMuZWR1