- Department of Pediatrics, Peking University First Hospital, Beijing, China
Introduction: Small for gestational age (SGA) infants are at a higher risk of neurodevelopmental delay than infants appropriate for gestational age (AGA). Previous studies have confirmed that gut microbiota in early life influences subsequent neurodevelopment. However, few studies have reported corresponding data in SGA populations.
Objective: We aimed to evaluate the characteristics of the gut microbiota of term SGA infants and the associations between the gut microbiota in SGA infants and neurodevelopmental outcomes at 6 months of age.
Methods: Fecal samples were collected on days 1, 3, 5, and 7 from term SGA and AGA infants born between June 2020 and June 2021 at the Peking University First Hospital. 16S ribosomal deoxyribonucleic acid amplicon sequencing was used to analyze the fecal microbiota. We followed up for 6 months and used the Ages and Stages Questionnaires-3 (ASQ-3) to evaluate the neurodevelopmental outcomes among SGA infants.
Results: A total of 162 neonates were enrolled, with 41 SGA infants (25.3%) in the study group and 121 AGA infants (74.7%) in the control group. The gut microbial diversity in the SGA group was lower than that in the AGA group on days 1, 3, 5, and 7. Non-metric multidimensional scaling and analysis of similarities showed significant differences between the two groups. The SGA group had increased relative abundances of Ralstonia (3, 5, and 7 days) and Clostridium (3 and 7 days). The dominant microorganisms of the SGA group were Ralstonia on day 1, Escherichia_Shigella on days 3 and 7, and Clostridia on day 5. We found that the gut microbial diversity of SGA infants with poor communication scores was higher than that of SGA infants with good communication scores on day 3. Fine motor scores were negatively correlated with the relative abundance of Bacteroides_fragilis on day 1. A negative correlation was observed between gross motor scores and relative abundance of Clostridium_saccharobutylicum on day 7. Bacteroidota, Bacteroidia, Bacteroides, and Bacteroides_fragilis were the dominant microorganisms in the good communication score group on day 7. Communication scores were positively correlated with the relative abundance of Bacteroidota, Bacteroides, and Bacteroides_fragilis on day 7.
Conclusion: The gut microbial diversity of term SGA infants was significantly lower in the first week of life than that of term AGA infants. Certain pathogenic and conditional pathogenic bacteria, such as Escherichia_Shigella, Ralstonia and Clostridium increased or formed the dominant microbiota in SGA infants. Alpha diversity, Bacteroidota, Bacteroides, Bacteroides_fragilis, and Clostridium_saccharobutylicum found in SGA infants may be associated with neurodevelopmental outcomes at 6 months of age, indicating possible therapeutic targets for clinical intervention.
Introduction
Small for gestational age (SGA) infants, defined as having a birth weight less than the 10th percentile of the birth weight of the same sex at the same gestational age, comprise a heterogeneous group (Chen et al., 2017; McCowan et al., 2018; Chawla, 2019). The incidence of SGA in China is ∼ 6.5%, ranking fifth worldwide (Lee et al., 2013). The development of each SGA system is imperfect, and the incidence of neurodevelopmental delay is significantly higher than that in appropriate for gestational age (AGA) infants (Sharma et al., 2016; McCowan et al., 2018; Kesavan and Devaskar, 2019). At present, the mechanisms leading to neurodevelopmental delays are unclear.
In recent years, gut microbiota has become a research hotspot in the fields of biology and medicine. Researchers have realized that the gut microbiota plays an important role in digestion, immune response, nutrient absorption, growth, and metabolism. The gut microbiota is involved in the regulation of many diseases such as inflammatory bowel disease, metabolic syndrome, and diabetes (Adak and Khan, 2019; Dabke et al., 2019; Ma et al., 2019; Mentella et al., 2020). Studies have found that microbiota plays a significant role in early neurological development (Carlson et al., 2018; Cohen Kadosh et al., 2021; Seki et al., 2021).
The so-called “gut–brain axis” represents a two-way communication network between the gut microbiota and the brain. The gut–brain axis theory proposes that the gut microbiota participates in the regulation of brain development and maturation, thus impacting brain functions, including anxiety-like behavior, locomotor behavior, social cognition, learning, and working memory (Al-Asmakh et al., 2012; Cryan et al., 2019; Long-Smith et al., 2020; Saurman et al., 2020). Compared with mice with normal gut microbiota, germ-free mice showed more obvious short-term cognitive and working memory impairments, whereas probiotic treatment prevented memory impairment after an inflammatory response in mice (Gareau et al., 2011). Gut microbiota affects various normal psychological processes and phenomena, participating in the pathophysiology of several psychological and neurological diseases (Liang et al., 2018). It has been reported that the gut microbial composition is altered in children with autism (Finegold et al., 2010; Plaza-Díaz et al., 2019; Dan et al., 2020; Saurman et al., 2020; Wong et al., 2022) and adults with Parkinson’s disease (Vascellari et al., 2020; Hirayama and Ohno, 2021) and Alzheimer’s disease (Zhuang et al., 2018; Bostanciklioğlu, 2019). Many studies have shown that probiotics are effective against anxiety, depression, autism spectrum disorder (ASD), and obsessive-compulsive disorder, and can also improve cognitive function, learning, and memory ability (Wang et al., 2016; Eastwood et al., 2021; Kim et al., 2021; Alemohammad et al., 2022). The intake of probiotics may ameliorate neurodegenerative disorders, including Alzheimer’s disease, multiple sclerosis, Parkinson’s disease, and amyotrophic lateral sclerosis (Cheng et al., 2019; Roy Sarkar and Banerjee, 2019).
Research focusing on children has indicated associations between gut microbiota in the first year of life and subsequent early neurodevelopment (Carlson et al., 2018; Sordillo et al., 2019; Tamana et al., 2021). Researchers have found that the alpha diversity of the gut microbiota in 1-year-old children could predict cognitive function at 2 years of age (Carlson et al., 2018). A cohort study found strong evidence of positive associations between Bacteroidetes in late infancy and subsequent cognitive and language performance (Tamana et al., 2021). Another cohort study observed an association between the gut microbiome composition of infants aged 3–6 months and communication—personal and social—and fine motor skills at 3 years of age (Sordillo et al., 2019).
At present, there are many studies on the development and establishment of gut microbiota in healthy neonates. However, studies on the characteristics and evolution of the gut microbiota in SGA infants and their relationship with long-term neurodevelopmental outcomes remain scarce. Therefore, the objective of this study was to explore the characteristics of the gut microbiota of SGA infants during the first week of life using high-throughput sequencing technology. Additionally, this study aimed to further explore the potential relationship between gut microbiota and neurodevelopmental prognosis of SGA infants at 6 months of age. The discovery of the effects of specific microbiota on neural development would provide important insights into potential therapeutic targets for the clinical improvement of neurological development in SGA infants.
Subjects and methods
Subjects
Term SGA and AGA neonates hospitalized in the pediatric neonatal ward of Peking University First Hospital between June 2020 and June 2021 were recruited for this study.
Inclusion criteria
The following inclusion criteria were used: (a) neonates in the study group needed to meet the diagnostic criteria of SGA infants: newborns whose birthweight was less than the 10th percentile of the birth weight of the same sex at the same gestational age (1); (b) gestational age was defined as ≥ 37 and < 42 weeks; (c) neonates without asphyxia, neonatal hypoxic-ischemic encephalopathy, severe intracranial hemorrhage, cerebral infarction, cytomegalovirus infection, recurrent hypoglycemia, bilirubin encephalopathy, and genetic metabolic diseases were enrolled; (d) informed consent was provided by the legal guardian(s); and (e) neonates were only enrolled with the agreement of cooperation with the follow-up by the legal guardian(s).
Exclusion criteria
The following exclusion criteria were used: (1) critical clinical conditions, such as sepsis and multiple organ failure; (2) gastrointestinal malformation, abdominal distension, vomiting, diarrhea, bloody stool, necrotizing enterocolitis, and other gastrointestinal diseases within 1 week; and (3) the presence of diseases that might affect neurological development during the follow-up period, such as severe brain trauma, epilepsy, meningitis, and genetic metabolic diseases.
Methods
Data collection
Clinical data, including sex, gestational age, birth weight, mode of delivery, and antibiotic application within 1 week after birth, were collected. Feces produced on postnatal days 1, 3, 5, and 7 were collected. Fecal samples were stored in sterile freezing tubes (Haimen Morder Experimental Equipment Factory) at −20°C and subjected to microbiota analysis within 1 week.
Gut microbiota test and analysis
Microbiota sequencing
A biological information database was built using an Illumina TruSeq§ DNA PCR-Free Sample Preparation Kit. Quality was evaluated with the assistance of the Qubit@ 2.0 and Agilent Bioanalyzer 2100 system. High-throughput sequencing was performed using an Illumina NovaSeq 6000 platform.
Bioinformatics analysis
The effective data were obtained by filtering the original data. The sequences were then clustered into operational taxonomic units (OTUs) with 97% identity, and the OTUs sequences were compared with the silva138 database for species annotation to obtain the basic analysis results of the OTUs and taxonomic pedigree for each sample. Finally, the analysis of OTUs, including alpha and beta diversity, was completed according to species annotation.
• Alpha diversity analysis: The richness and diversity of microbiota can be indicated by alpha diversity, wherein Observed species, Chao1, abundance-based coverage estimator (ACE), Shannon, Simpson, and goods coverage are major evaluation indices of alpha diversity. Observed species represents the actual number of OTUs in the sample. The Chao1 and ACE indices use different calculation methods to estimate the number of OTUs in a sample; the higher the number of OTUs, the higher the diversity of the sample. The abundance and uniformity of the gut microbiota can be expressed using the Shannon and Simpson indices. If all the OTUs contained in the sample were the same, the diversity was the lowest; if they were different, the diversity was the highest. The larger the values of the Shannon and Simpson indices, the higher the diversity of the samples. Good coverage index indicates the sequencing depth; the higher the value, the better the sequencing. The closer the value is to 1, the closer the sequencing depth is to cover all bacteria in the test sample. Rarefaction and rank variance curves are common curves that describe the diversity of the samples in the group. The rarefaction curve directly reflects the rationality of the sequencing data and indirectly reflects the richness of species in the sample, whereas the rank variance curve intuitively reflects the richness and uniformity of species in the sample.
• Beta diversity analysis: Beta diversity analysis focuses on the differences in the microbial community composition of different samples, which is used for the analysis of differences between groups. Principal coordinate analysis (PCoA) and non-metric multidimensional scaling (NMDS) directly reflects the differences in community composition between groups based on the distance between samples. Each point in the figure represents a sample; points of the same color belong to the same group, and the distance between points represents the degree of difference. The distance is directly proportional to the difference between points. A stress score < 0.2 indicates that NMDS can accurately reflect the degree of difference between groups. Analysis of similarities (Anoism) is a non-parametric test used to test the significance of differences. An R-value > 0 indicates significant differences between groups, an R-value < 0 indicates that the differences within groups were greater than those between groups, and a P-value < 0.05 indicates statistical significance.
• Differential analysis of gut microbiota: (1) Differential relative abundance: We compared the differences in microbial distribution between groups according to the relative abundance of communities at different levels of phylum, class, order, family, genus, and species. In this study, we used the Metastat method to analyze microbial differences between the two groups at the phylum, family, and genus levels. (2) Differentially dominant microorganisms: We found microbial differences between groups using linear discriminant analysis effect size (LEfSe) analysis. The LEfSe calculation method not only has statistical significance, but also focuses on biological correlation by using linear discriminant analysis (LDA) to reduce the dimension and evaluate the impact of species with significant differences (i.e., LDA score). The default LDA score was 2, which can be increased according to the characteristics of the community distribution to obtain more accurate data.
Follow-up of study group
We followed up with the SGA infants until 6 months after birth and assessed their neurodevelopmental outcomes using the Age and Staging Questionnaire-3 (ASQ-3). The same neurodevelopmental professional evaluation doctor, proficient in ASQ-3 scoring criteria, conducted the evaluation. ASQ-3 mainly includes five parts, namely communication, gross motor, fine motor, problem-solving, and personal–social, with each part containing six specific assessment questions.
Statistical analysis
Statistical software (SPSS 25.0) was used to analyze the data. Measurement data consistent with a normal distribution are expressed as the mean ± standard deviation (x ± s). Student’s t-test was used to compare two groups, while analysis of variance (ANOVA) was used to compare three or more groups. The measurement data that were not in line with the normal distribution were expressed as median (IQR) or median (P25, P75). The Wilcoxon Mann–Whitney U test was used for the comparison between two groups, while the Kruskal–Wallis H test was used for the comparison of three groups and above. The enumeration data were expressed as the number of cases and percentages, and comparisons between groups were performed using the χ2 test. If the total number n was < 40 or at least one actual frequency, t < 1, Fisher’s exact test method was applied. For the correlation analysis of two quantitative datasets, Pearson correlation analysis was adopted if it conformed to the bivariate normal distribution; otherwise, Spearman correlation analysis was adopted. Statistical significance was set at P < 0.05.
Ethical approval
The study was approved by the Ethics Committee of the Peking University First Hospital. The legal guardians of each participant provided written informed consent.
Results
Clinical characteristics of neonates in the small for gestational age and appropriate for gestational age groups
A total of 41 SGA neonates were enrolled in the SGA group, including 19 males (46.3%) and 24 neonates (58.5%) delivered via cesarean section. A total of 121 AGA neonates were enrolled in the AGA group, with 75 males (62.0%) and 33 neonates (27.5%) delivered via cesarean section. All neonates were born at a gestational age of 37–42 weeks and fed a mixed feed (breast milk + formula). The clinical characteristics of the enrolled neonates are presented in Table 1. A total of 31 neonates (75.6%) in the SGA group and 112 neonates (92.6%) in the AGA group had aspiration pneumonia or increased non-specific inflammatory indices. The proportion of ampicillin users in the AGA group was significantly higher than that in the SGA group. The gestational age and birth weight of the neonates in the SGA group were significantly lower than those in the AGA group, and the proportion of cesarean sections was significantly higher in the SGA group. The following clinical characteristics differed significantly between the SGA and AGA groups: infants from twin pregnancies, premature rupture of membranes, hospital stay of neonates, chorioamnionitis, and maternal antibiotics. There were no significant differences in sex, Apgar scores at 1 and 5 min, region, siblings, mother’s pregnancy weight gain, pregnancy complications (diabetes or gestational hypertension), or pet ownership between the two groups. None of the enrolled neonates were infected with the novel coronavirus, and neither their mothers nor their family members showed the emergence of pandemic-related mental or personality disturbances.
Gut microbiota analysis of the small for gestational age and appropriate for gestational age groups
In this study, an average of 76,816 tags were measured per sample, and an average of 75,108 valid data points was obtained after quality control. The sequence was clustered into OTUs with 97% identity and 13,747 OTUs were obtained.
Sequencing depth and rationality
After obtaining all the OTUs, a rarefaction curve was drawn to evaluate whether the current sequencing depth of each sample could fully reflect the microbial diversity in the community samples. When the dilution curve tended to be flat (Supplementary Figure 1), the sequencing data gradually became reasonable. The coverage index of these samples fluctuated between 0.974 and 1, indicating that the sequencing depth was close to covering all bacterial communities in the tested samples.
Alpha and beta diversities
A comparison of the alpha diversity of fecal microbiota in the SGA group on different days in the first week of life revealed no statistical difference in the Chao1, ACE, Observed species, Simpson, and Shannon indices, indicating that there was no significant difference in the richness and diversity of gut microbiota within the SGA group at postnatal days 1, 3, 5, and 7 (Supplementary Table 1).
A comparison of alpha diversity of fecal microbiota between the SGA and AGA groups revealed that the SGA group’s gut microbial diversity was significantly lower than that of the AGA group in the Chao1, ACE, Observed species, Simpson, and Shannon indices on the first day (< 0.05). On days 3, 5, and 7, the Chao1, ACE, and Observed species indices of fecal microbiota of the SGA group remained significantly lower than the AGA group (P < 0.05), whereas there was no significant difference in the Simpson and Shannon indices (Supplementary Figure 2 and Supplementary Table 2).
PCoA showed significant differences in the gut microbiota on days 1, 3, 5, and 7 between the two groups. The R-value > 0, and statistical analysis between groups showed significant differences (P < 0.05) (Supplementary Figures 3A–H and Supplementary Table 3). The NMDS analysis (Supplementary Table 4) indicated that the gut microbiota of the two groups differed significantly on days 1, 3, 5, and 7 (stress score < 0.2) (Figure 1).
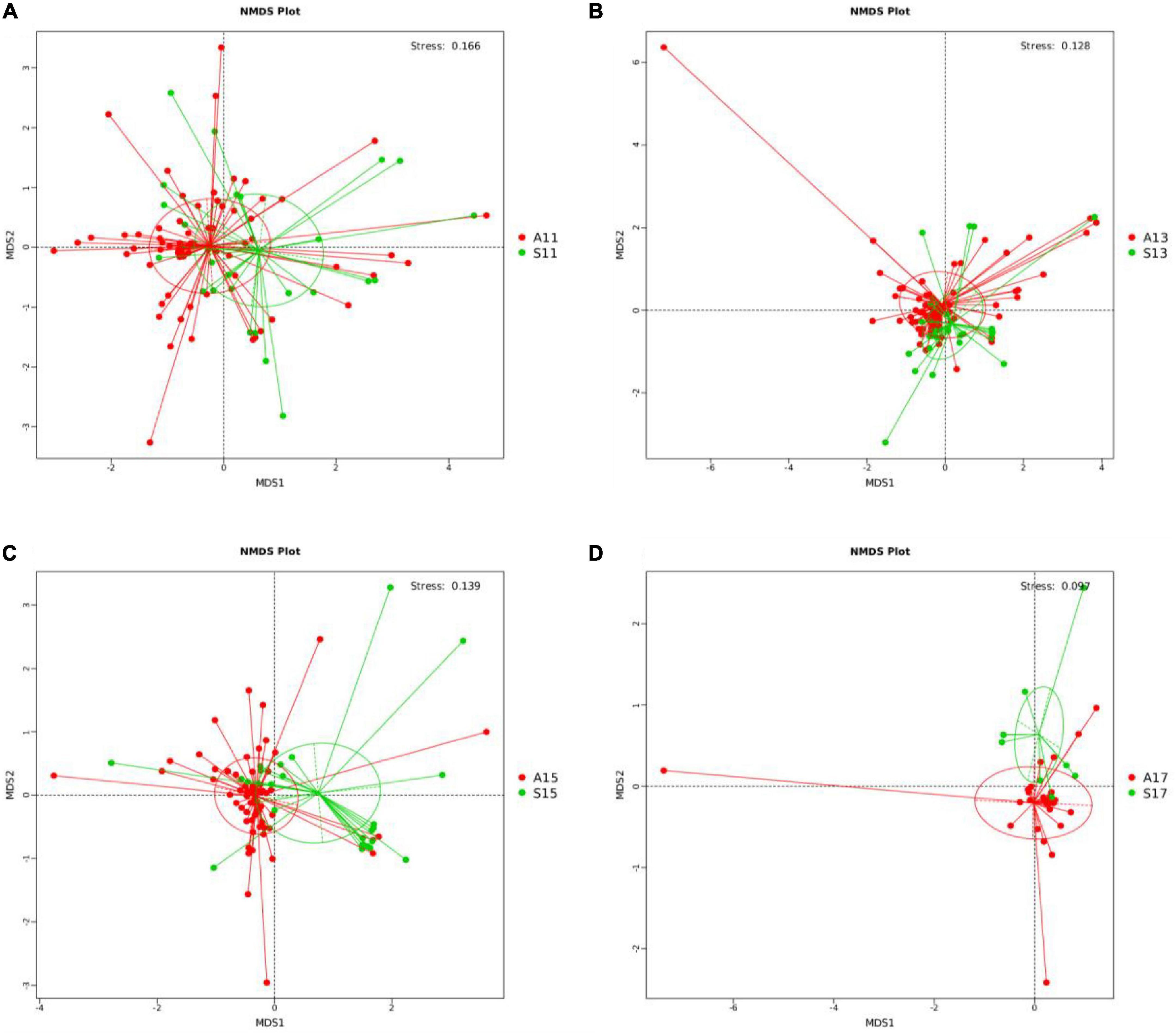
Figure 1. NMDS analysis between the SGA and AGA groups on days 1, 3, 5, and 7. (A) NMDS analysis on day 1. (B) NMDS analysis on day 3. (C) NMDS analysis on day 5. (D) NMDS analysis on day 7. NMDS, non-metric multi-dimensional scaling. The red points represent belonging to the AGA group, and the green points represent belonging to the SGA group. S11, gut microbiota of the SGA group on day 1; A11, gut microbiota of the AGA group on day 1; S13, gut microbiota of the SGA group on day 3; A13, gut microbiota of the AGA group on day 3; S15, gut microbiota of the SGA group on day 5; A15, gut microbiota of the AGA group on day 5; S17, gut microbiota of the SGA group on day 7; A17, gut microbiota of the AGA group on day 7.
Analysis of differential gut microbiota
Differential relative abundance
The main microbiota in the AGA group at the phylum level were Firmicutes, Proteobacteria, Actinobacteria, and Bacteroidetes (Supplementary Figures 4, 5); at the family level, Enterococcaceae, Streptococcaceae, Enterococcaceae, Staphylococcaceae, Vibrionaceae (Supplementary Figures 6, 7); and at the genus level, Enterococcus, Streptococcus, Escherichia-Shigella, Staphylococcus, and Vibrio (Supplementary Figures 8A, 9).
On day 1, the SGA group showed a decreased relative abundance of Cyanobacteria, Vibrionaceae, Parabacteroides, Lactiplantibacillus, Serratia, Citrobacter, and Cutibacterium. However, the relative abundance of Ileibacterium was higher in the SGA group than in the AGA group (Table 2, Figure 2, and Supplementary Figures 10, 11).
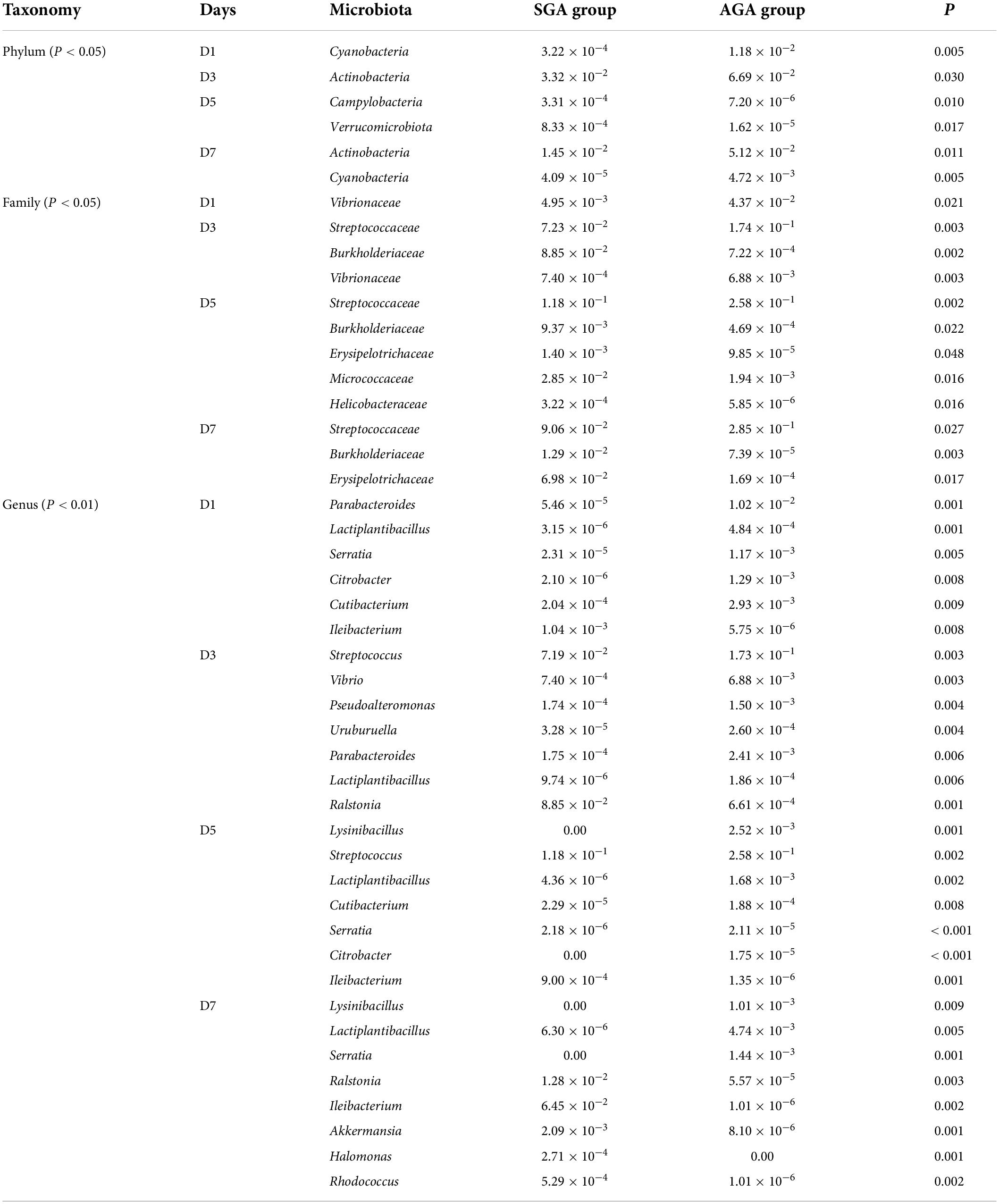
Table 2. Gut microbiota analysis of the SGA and AGA groups on days 1, 3, 5, and 7 at levels of phylum, family, and genus.
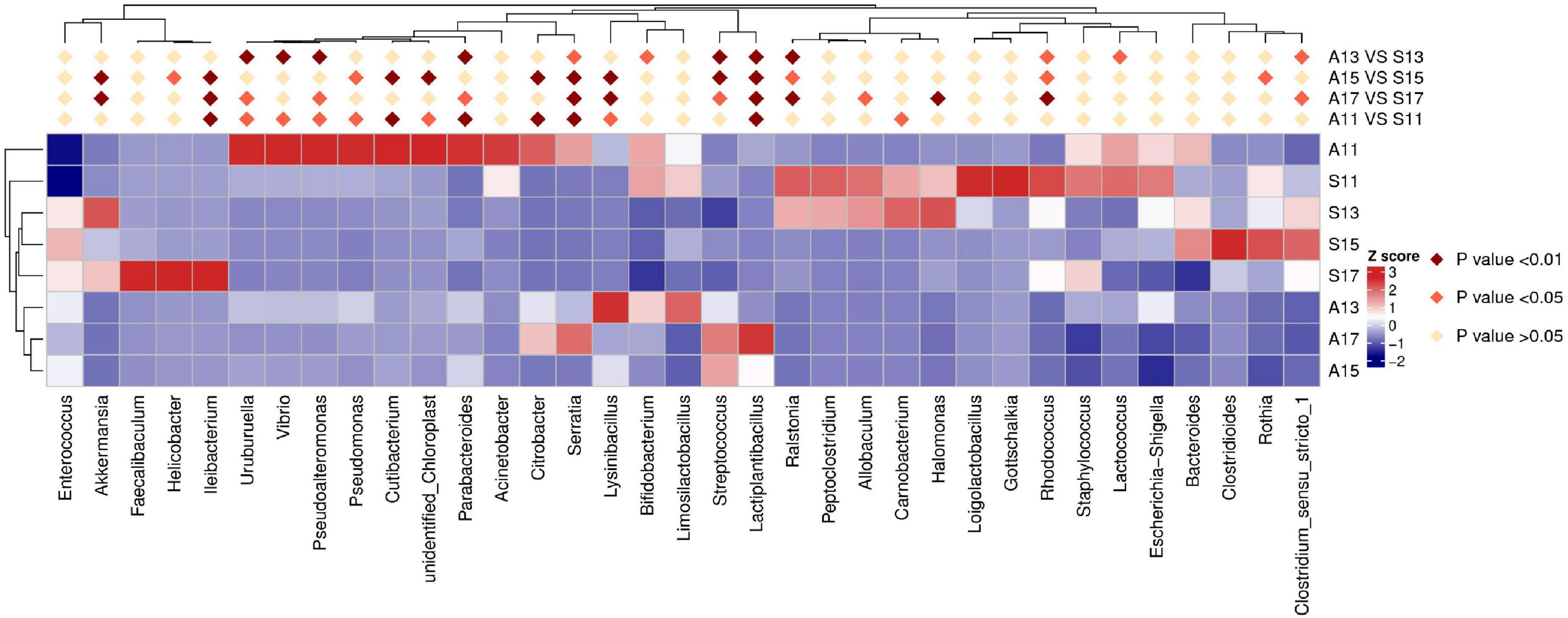
Figure 2. Results of heatmap analysis of species with significant differences between the SGA and AGA groups at the genus level. S11, gut microbiota of the SGA group on day 1; A11, gut microbiota of the AGA group on day 1; S13, gut microbiota of the SGA group on day 3; A13, gut microbiota of the AGA group on day 3; S15, gut microbiota of the SGA group on day 5; A15, gut microbiota of the AGA group on day 5; S17, gut microbiota of the SGA group on day 7; A17, gut microbiota of the AGA group on day 7.
On day 3, the SGA group showed decreased relative abundances of Actinobacteria, Streptococcaceae, Vibrionaceae, Streptococcus, Vibrio, Pseudoalteromonas, Uruburuella, Parabacteroides, and Lactiplantibacillus, whereas Burkholderiaceae, and Ralstonia were higher in the SGA group than in the AGA group (Table 2, Figure 2, and Supplementary Figures 10, 11).
On day 5, the SGA group showed decreased relative abundances of Streptococcaceae, Lysinibacillus, Streptococcus, Lactiplantibacillus, Cutibacterium, Serratia and Citrobacter, while those of Campylobacteria, Verrucomicrobiota, Burkholderiaceae, Erysipelotrichaceae, Micrococcaceae, Helicobacteraceae, Ileibacterium, and Akkermansia were higher in the SGA group than in the AGA group (Table 2, Figure 2, and Supplementary Figures 10, 11).
On day 7, the SGA group showed decreased relative abundances of Actinobacteria, Cyanobacteria, Streptococcaceae, Lysinibacillus, Lactiplantibacillus, and Serratia, whereas those of Burkholderiaceae, Erysipelotrichaceae, Ralstonia, Ileibacterium, Akkermansia, Halomonas, and Rhodococcus were higher in the SGA group than in the AGA group (Table 2, Figure 2, and Supplementary Figures 10, 11).
Differential dominant microorganisms
LEfSe was used to analyze differentially dominant microorganisms, and the LDA value was set to 4. On day 1, the dominant microorganisms in the SGA group were g-Ralstonia and s-Ralstonia_pickettii, while s-Streptococcus_sp_FDAARGOS_192, f-Vibrionaceae, and g-Vibrio were dominant in the AGA group. On day 3, the dominant microorganisms in the SGA group were s-Ralstonia_pickettii and g-Escherichia_Shigella, while f-Streptococcaceae, g-Streptococcus, and s-Streptococcus_sp_FDAARGOS_192 were dominant in the AGA group. On day 5, the dominant microorganisms in the SGA group were c-Clostridia, g-Rothia, s-Bacteroides_fragilis, and o-Clostridiales, while f-Streptococcaceae, g-Streptococcus, and s-Streptococcus_sp_FDAARGOS_192 were dominant in the AGA group. On day 7, the dominant microorganisms in the SGA group were s-Ileibacterium_valens, g-Ileibacterium, f-Enterobacteriaceae, g-Helicobacter, and g-Escherichia_Shigella, while s-Streptococcus_sp_FDAARGOS_192 were dominant in the AGA group (Figure 3 and Supplementary Figures 12A–D).
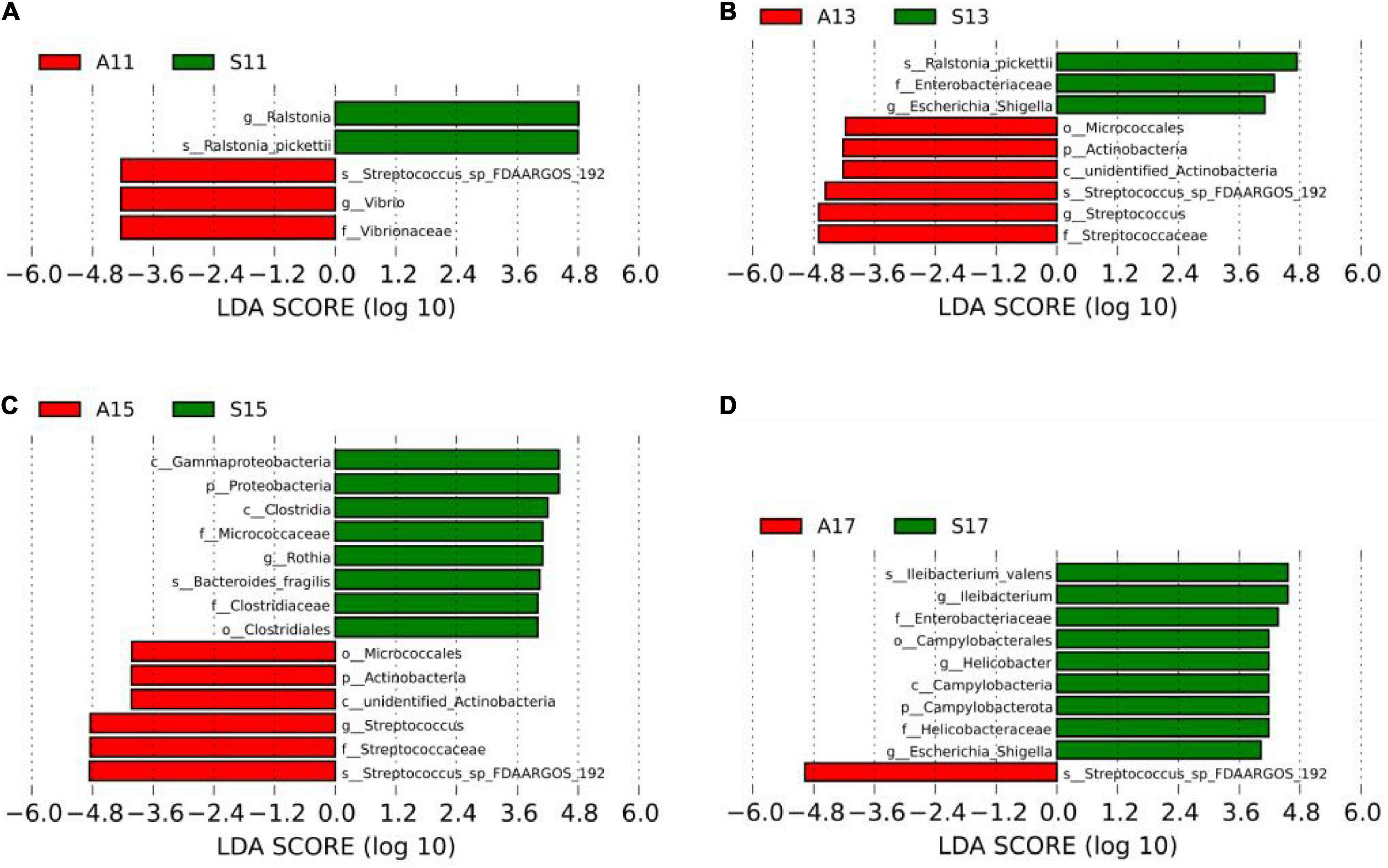
Figure 3. LEfSe comparison between the SGA and AGA groups. (A) LDA score histogram of differential microbiota of the two groups on day 1. (B) LDA score histogram of differential microbiota of the two groups on day 3. (C) LDA score histogram of differential microbiota of the two groups on day 5. (D) LDA score histogram of differential microbiota of the two groups on day 7. p_ represents phylum level, c _ represents class level, o_ represents order level, f_ represents family level, g_ represents genus level, and s_ represents species level. The length of the column represents the LDA score, and the greater the score, the greater the influence of the dominant microbiota. LDA, linear discriminant analysis; LEfSe, linear discriminant analysis effect size. S11, gut microbiota of the SGA group on day 1; A11, gut microbiota of the AGA group on day 1; S13, gut microbiota of the SGA group on day 3; A13, gut microbiota of the AGA group on day 3; S15, gut microbiota of the SGA group on day 5; A15, gut microbiota of the AGA group on day 5; S17, gut microbiota of the SGA group on day 7; A17, gut microbiota of the AGA group on day 7.
Correlation analysis between the top six microbiota at the genus and species levels in the small for gestational age group and ASQ-3 scores at 6 months of age
Neonates in the SGA group were followed up to 6 months of age, of which, 38 (92.7%) infants completed the follow-up and three infants were lost to follow-up (7.3%). The fine motor scores of ASQ-3 were negatively correlated with the relative abundance of s-Bacteroides_fragilis on day 1 (r = −0.412, P = 0.041). On day 7, the communication scores were positively correlated with the relative abundances of g-Bacteroides (r = 0.875, P = 0.004) and s-Bacteroides_fragilis (r = 0.886, P = 0.003), whereas a negative correlation was observed between gross motor scores and the relative abundance of s-Clostridium_saccharobutylicum (r = −0.736, P = 0.037; Table 3).
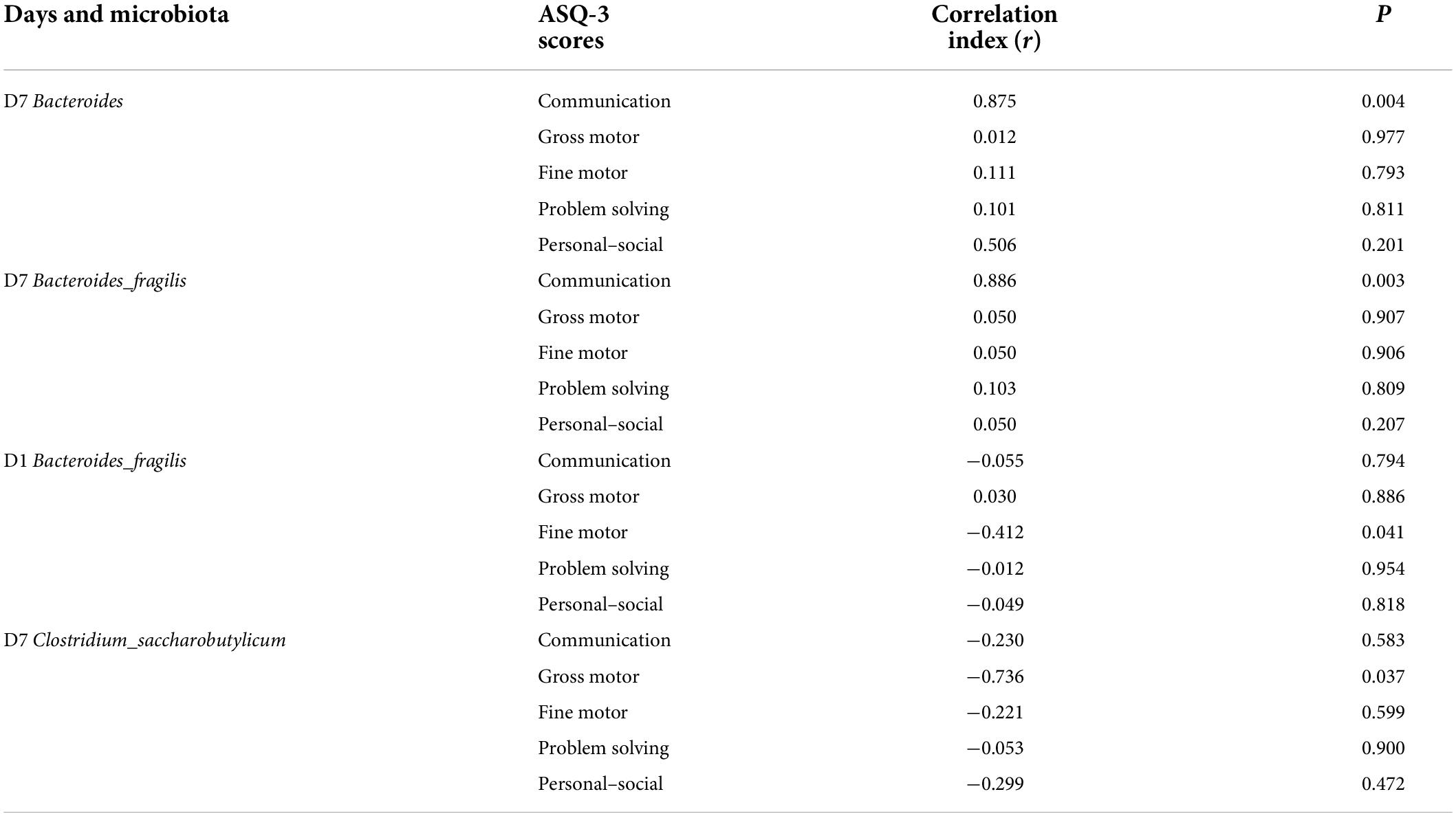
Table 3. Correlation analysis between the top six microbiota at genus and species level in the SGA group and ASQ-3 scores at 6 months postnatal age.
Analysis of gut microbiota of neonates in small for gestational age group with different neurological prognosis (communication)
We followed SGA infants until 6 months of age, and 38 of them completed the follow-up. A communication score ≥ 40 was considered normal. There were 31 (81.6%) infants with normal communication scores and 7 (18.4%) infants with poor communication scores in the SGA group. The two subgroups showed no significant differences in sex, gestational age, birth weight, mode of delivery, feeding pattern, or antibiotic application within 1 week after birth (Table 4).
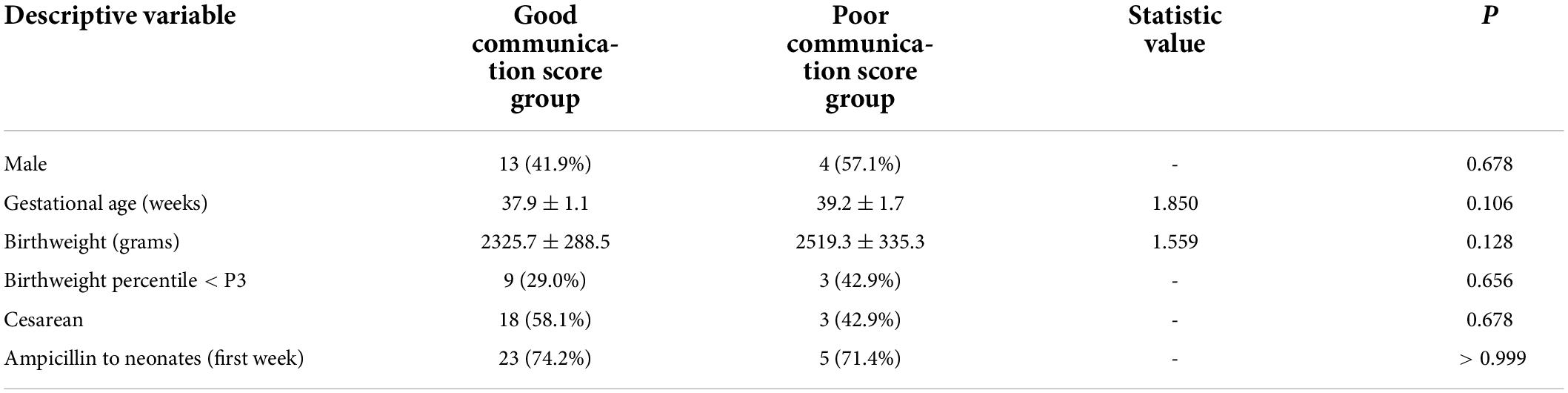
Table 4. Clinical characteristics of the good and poor communication score groups in the SGA population.
Alpha and beta diversities
A comparison of the alpha diversity of fecal microbiota between the good and poor communication score groups revealed no statistical difference in the Chao1, ACE, Observed species, Simpson, and Shannon indices on days 1, 5, and 7. On day 3, the gut microbial diversity of the poor communication score group was significantly higher than that of the good communication score group in the Chao1, ACE, and Observed species indices (P < 0.05), whereas there was no significant difference in the Simpson and Shannon indices (Figure 4 and Supplementary Table 5).
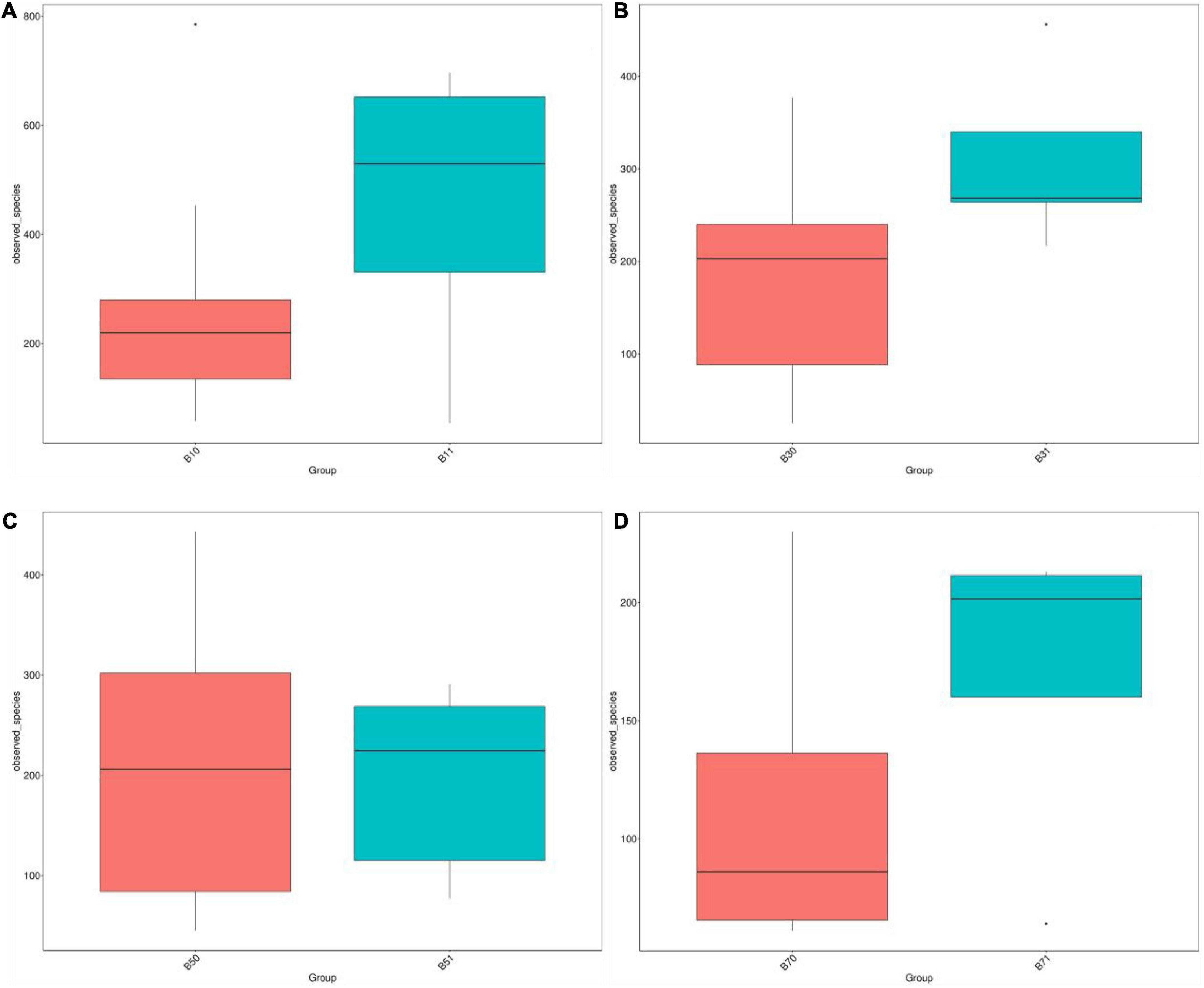
Figure 4. Distribution box plots of alpha diversity index between the good and poor communication score groups on days 1, 3, 5, and 7. (A) Distribution box plots of the alpha diversity index on day 1. (B) Distribution box plots of alpha diversity index on day 3. (C) Distribution box plots of alpha diversity index on day 5. (D) Distribution box plots of alpha diversity index on day 7. B11, gut microbiota of the poor communication score group on day 1; B10, gut microbiota of the good communication score group on day 1; B31, gut microbiota of the poor communication score group on day 3; B30, gut microbiota of the good communication score group on day 3; B51, gut microbiota of the poor communication score group on day 5; B50, gut microbiota of the good communication score group on day 5; B71, gut microbiota of the poor communication score group on day 7; B70, gut microbiota of the good communication score group on day 7.
PCoA and Anosim showed no significant differences in the gut microbiota on days 1 and 7 between the good and poor communication score groups; although the R-value was > 0, but the statistical analysis between the groups showed no significant difference (P > 0.05). There were no significant differences in gut microbiota on days 3 and 5 in the good and poor communication score groups; the R-value was < 0, but the statistical analysis within groups showed no significant difference (P > 0.05) (Supplementary Figures 13A–D and Supplementary Table 6). However, NMDS analysis indicated that the gut microbiota of the two groups differed significantly on days 1, 3, 5, and 7 (stress score < 0.2) (Supplementary Figures 14A–D).
Analysis of differential gut microbiota
Differential relative abundance
The main microbiota in the good communication score group included Firmicutes, Proteobacteria, Actinobacteria, and Bacteroidetes at the phylum level (Supplementary Figure 15); Enterococcaceae, Burkholderiaceae, Enterobacteriaceae, Streptococcaceae, and Staphylococcaceae at the family level (Supplementary Figure 16); Enterococcus, Streptococcus, Escherichia-Shigella, Staphylococcus, Bacteroides, and Ileibacterium at the genus level (Supplementary Figure 17); and Ralstonia_pickettii, Streptococcus_sp_FDAARGOS_192, Bacteroides_fragilis, Ileibacterium_valen, Rothia_mucilaginosa, and Clostridium_saccharobutylicum at the species level (Supplementary Figure 18). On day 1, the poor communication score group showed decreased relative abundances of Enterobacteriaceae, Streptococcaceae, and Streptococcus (Supplementary Table 7 and Supplementary Figures 19A–D). On day 3, the main microbiota between the good and poor communication score groups showed no significant differences in the phylum, family, genus, and species levels (Supplementary Table 7 and Supplementary Figures 20A–D). On day 5, the poor communication score group showed decreased relative abundances of Staphylococcaceae and Staphylococcus, whereas the relative abundance of Enterococcus was higher in the poor communication score group (Supplementary Table 7 and Supplementary Figures 21A–D). On day 7, the poor communication score group showed decreased relative abundances of Bacteroidota, Bacteroides, and Bacteroides_fragilis, whereas the relative abundance of Corynebacterium was higher in the poor communication score group (Supplementary Table 7 and Supplementary Figures 22A–D).
Differential dominant microorganisms
On day 1, the dominant microorganisms in the poor communication score group were f-Erysipelotrichaceae, f-Carnobacteriaceae, and g-Allobaculum. On day 3, they were p-Actinobacteria, c-unidentified Actinobacteria, and f-Peptostreptococcaceae. There were no dominant microorganisms in the poor communication score group on day 5. On day 7, the dominant microorganism in the poor communication score group was f-Peptostreptococcaceae. p-Bacteroidota, c-Bacteroidia, o-Bacteroidales, f-Bacteroidaceae, g-Bacteroides, and s_Bacteroides_fragilis formed the dominant microorganisms in the good communication score group on day 7 (LDA score > 3). There were no dominant microorganisms in the good communication score group on days 1, 3, and 5 (Figure 5 and Supplementary Figure 23).
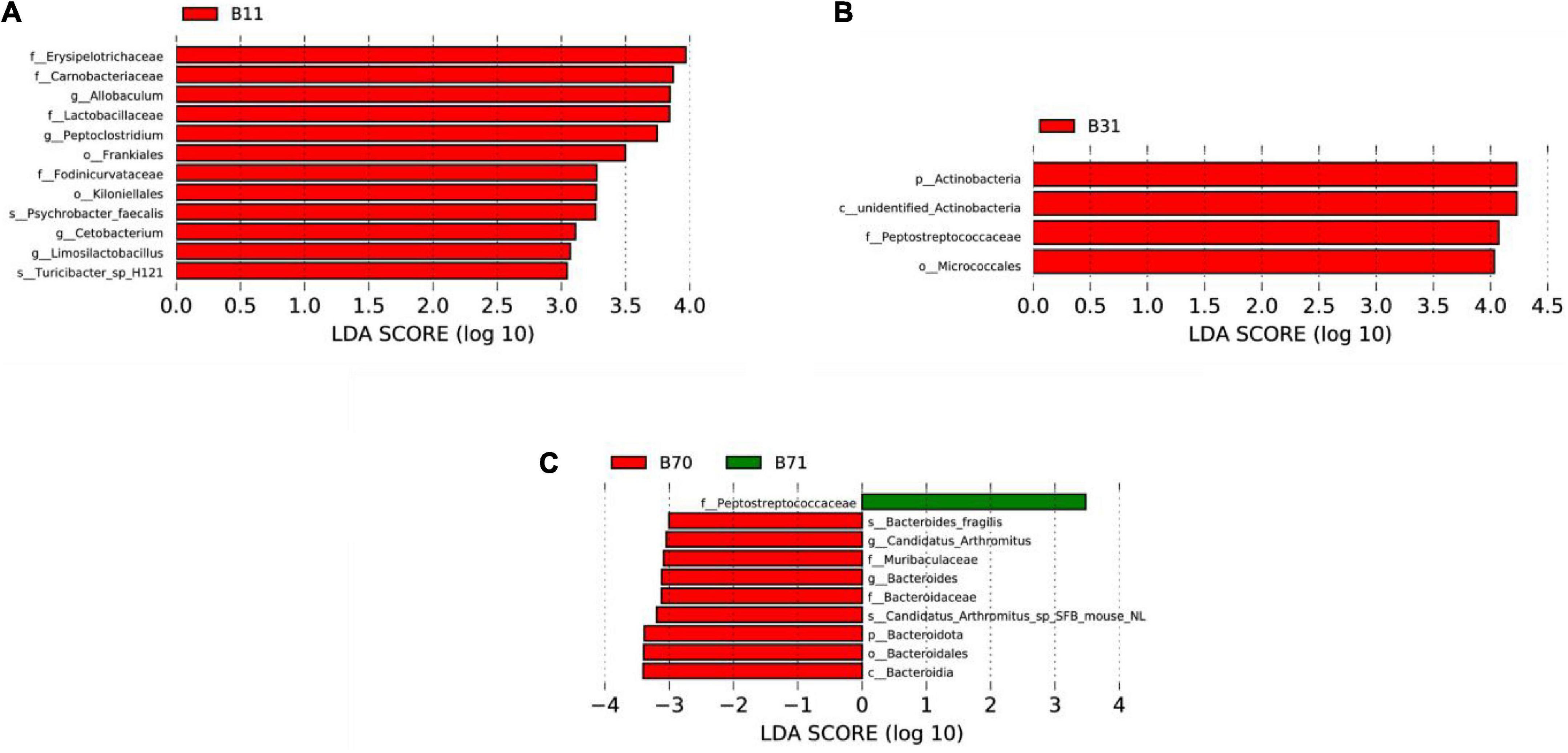
Figure 5. LEfSe comparison between the good and poor communication score groups. (A) LDA score histogram of differential microbiota of the two groups on day 1. (B) LDA score histogram of differential microbiota of the two groups on day 3. (C) LDA score histogram of differential microbiota of the two groups on day 7. p_ represents phylum level, c_ represents class level, o_ represents order level, f_ represents family level, g_ represents genus level, and s_ represents species level. B11, gut microbiota of the poor communication score group on day 1; B31, gut microbiota of the poor communication score group on day 3; B71, gut microbiota of the poor communication score group on day 7; B70, gut microbiota of the good communication score group on day 7.
Analysis of differential microbiota and communication scores at 6 months of age
We analyzed the correlation between differentially abundant microbiota and communication scores at 6 months postnatal, and found that p-Bacteroidota, g-Bacteroides, and s-Bacteroides_fragilis were positively correlated with communication scores, on day 7. Moreover, there was no correlation between the rest of the differentially abundant microbiota and communication scores (Supplementary Table 8).
Effect of delivery mode on gut microbiota of small for gestational age neonates and ASQ-3 scores
A total of 41 SGA neonates were enrolled, including 24 neonates (58.5%) delivered by cesarean section and 17 neonates (41.5%) through vaginal delivery. The two subgroups showed no significant differences in sex, gestational age, birth weight, feeding patterns, or antibiotic application within 1 week after birth (Supplementary Table 9).
Alpha and beta diversities
A comparison of alpha diversity of fecal microbiota between the cesarean birth and vaginal delivery groups revealed no statistical difference in the Chao1, ACE, Observed species, Simpson, and Shannon indices on days 1, 3, and 5 (Supplementary Table 10 and Supplementary Figure 24).
PCoA and Anosim showed no significant differences in gut microbiota between the cesarean birth and vaginal delivery groups; the R-value was > 0, but the statistical analysis between the groups showed no significant difference (P > 0.05; Supplementary Figures 25A–C, 26A–C and Supplementary Table 11). However, NMDS analysis indicated that the gut microbiota of the two groups differed significantly on days 1, 3, and 5 (stress score < 0.2; Supplementary Figures 27A–C).
Differential relative abundance and dominant microorganisms
Between the two groups, there were differences in the relative abundances of Campylobacterota, Bacteroidota, and Actinobacteria at the phylum level (Supplementary Figures 28A–C and Supplementary Table 12); Bacteroidaceae and Tannerellaceae at the family level (Supplementary Figures 29A–C and Supplementary Table 12); and Bacteroides, Eubacterium_hallii_group, and Ileibacterium at the genus level (Supplementary Figures 30A–C and Supplementary Table 12). On day 1, the dominant microorganisms in the vaginal delivery group were g-Ileibacterium and s-Ileibacterium_valens (Supplementary Figure 31A); f-Bacteroidaceae, g-Bacteroides, and s-Bacteroides_fragilis on day 3 (Supplementary Figure 31B); and p-Bacteroidota, c-Bacteroidia, o-Bacteroidales, f-Bacteroidaceae, g-Bacteroides, and s-Bacteroides_fragilis on day 5. The dominant microorganisms in the cesarean birth group were o-Staphylococcales, f-Staphylococcaceae, and g-Staphylococcus (Supplementary Figure 31C).
In summary, the gut microbiota with differences between the two delivery modes mainly included g-Bacteroides, g-Staphylococcus, and g-Ileibacterium, whereas the gut microbiota with differences between the SGA and AGA groups mainly included g-Escherichia_Shigella, g-Ralstonia, g-Clostridium, and g-Streptococcus.
Effect of delivery mode on ASQ-3 scores of small for gestational age infants at 6 months of age
The ASQ-3 scores of SGA neonates at 6 months of age were not associated with the delivery mode (Supplementary Table 13).
Effect of antibiotic application on gut microbiota of small for gestational age neonates and ASQ-3 scores
A total of 41 SGA neonates were enrolled, including 31 neonates (75.6%) treated with antibiotics and 10 neonates (24.4%) treated without antibiotics, according to their condition. The two subgroups showed no significant differences in sex, gestational age, birth weight, feeding patterns, or delivery mode (Supplementary Table 14).
Alpha and beta diversities
A comparison of the alpha diversity of fecal microbiota between the group with antibiotics and the group without antibiotics revealed no statistical difference in the Chao1, ACE, Observed species, Simpson, and Shannon indices on days 1, 3, and 5 (Supplementary Table 15 and Supplementary Figure 32).
The R- and P-values were > 0.05 on days 1 and 5, indicating that there was no statistical difference between the two groups on days 1 and 5. On day 3, R-value < 0 and P > 0.05 3, indicating that there was no statistical difference between the two groups (Supplementary Figures 33A–C, 34A–C and Supplementary Table 16). However, NMDS analysis indicated that the gut microbiota of the two groups differed significantly on days 1, 3, and 5 (stress score < 0.2; Supplementary Figures 35A–C).
Differential relative abundance and dominant microorganisms
Between the two groups, there was a difference in the relative abundance of Gemmatimonadota at the phylum level (Supplementary Figures 36A–C and Supplementary Table 17); Carnobacteriaceae, Enterococcaceae, Enterobacteriaceae, and Burkholderiaceae at the family level (Supplementary Figures 37A–C and Supplementary Table 17); Aminobacter, Georgenia, and Loigolactobacillus at the genus level (Supplementary Figures 38A–C and Supplementary Table 17). On day 1, the dominant microorganisms in the antibiotic-treated group were f-Micrococcaceae, g-Rothia, o-Micrococcales, and s-Rothia_mucilaginosa (Supplementary Figure 39A); s-Clostridium_saccharobutylicum, g-Staphylococcus, o-Staphylococcales, f-Staphylococcaceae, s-Clostridium_perfringens, s-Enterococcus_faecalis, s-Clostridioides_difficile, and g-Clostridioides on day 3 (Supplementary Figure 39B); and o-Lactobacillales, f-Enterococcaceae, g-Enterococcus, and c-Bacilli on day 5. The dominant microorganisms in the group without antibiotics were o-Enterobacterales, f-Enterobacteriaceae, g-Escherichia_Shigella, g-Clostridium_sensu_stricto_1, f-Peptostreptococcaceae, g-Clostridioides, and s-Clostridioides_difficile (Supplementary Figure 39C).
In summary, the gut microbiota with differences between the two groups with different applications of antibiotics mainly included g-Rothia, g-Clostridioides, g-Staphylococcus, g-Enterococcus, g-Escherichia_Shigella, and g-Clostridium; while the gut microbiota with differences between the SGA and AGA groups mainly included g-Escherichia_Shigella, g-Ralstonia, g-Clostridium, and g-Streptococcus.
Effect of application of antibiotics on ASQ-3 scores of small for gestational age infants at 6 months of age
The ASQ-3 scores of SGA neonates at 6 months of age were not associated with the use of antibiotics (Supplementary Table 18).
Discussion
SGA infants exhibit more significant long-term health issues, including a variety of major and subtle neurodevelopmental delays, than their appropriate gestational age counterparts (Sharma et al., 2016; McCowan et al., 2018; Kesavan and Devaskar, 2019). At present, the mechanisms underlying neurodevelopmental delay in SGA infants are unclear. A growing number of studies have indicated that the gut microbiota plays an important role in early neural development (Carlson et al., 2018; Cohen Kadosh et al., 2021; Seki et al., 2021). Colonization and maturation of the gut microbiota overlap with the critical period of early brain development, and an imbalance in gut microbiota during the early postnatal period may disrupt the developmental programming of the brain through the gut−brain axis, leading to brain injury and long-term neurodysplasia later in life (Al-Asmakh et al., 2012; Cryan et al., 2019; Seki et al., 2021). Therefore, it is of great significance to study the association between gut microbiota and neural development in SGA infants and to explore the impact of specific microbiota on neural development.
In our study, the alpha diversity of gut microbiota in the SGA group was significantly lower than that in the AGA group on days 1, 3, 5, and 7, consistent with the findings of a previous study (Zhang et al., 2019). At the phylum level, the main microbiota of the SGA group were Firmicutes, Proteobacteria, Actinobacteria, and Bacteroidota, similar to the results of previous studies (Martí et al., 2021; Zhang et al., 2021). With respect to the differential abundance of gut microbiota between the SGA and AGA groups, Actinobacteria (3, 7 days) and Cyanobacteria (1, 7 days) were significantly lower in the SGA group. Furthermore, another study found that pigs with intrauterine growth restriction had a lower relative abundance of Actinobacteria (Che et al., 2019), consistent with observations of our study. Cyanobacteria have been reported to exhibit good anti-inflammatory, antioxidant, cholesterol-lowering, and antimicrobial activities (Ferrazzano et al., 2020). Campylobacterota, considered to be associated with intestinal and extraintestinal infections (Fitzgerald, 2015; Same and Tamma, 2018), were more abundant on day 5 and were the dominant microbiota on day 7 in the SGA group.
At the genus level, the main microbiota of the SGA group included Enterococcus, Ralstonia, Staphylococcus, Streptococcus, Escherichia-Shigella, and Bacteroides, consistent with findings of previous research (Bäckhed et al., 2015; Gabriel et al., 2018; Zhang et al., 2021). Among the differential microbiota between the SGA and AGA groups, Ralstonia and Ralstonia_pickettii were the dominant microorganisms in the SGA group on day 1, and Ralstonia was higher in the SGA group on days 3, 5, and 7. Reports have shown that Ralstonia is related to the pathogenesis of nervous system diseases such as autism spectrum disorder (ASD) and Parkinson’s disease (PD), and its mechanism may involve neuroinflammation and immune activation (Keshavarzian et al., 2015; Ragusa et al., 2020). F-Enterobacteriaceae (7 days), g-Escherichia_Shigella (3, 7 days), and g-Helicobacter (7 days) were the dominant microorganisms in the SGA group. Escherichia_Shigella is well-known as a pathogenic enterobacterium; some strains of Helicobacter are recognized as important pathogens in gastrointestinal diseases, such as peptic ulcers and gastric cancer, and some strains are associated with bacteremia in immunocompromised and immunocompetent human hosts (On et al., 2002; Boltin et al., 2019). c-Clostridia, o-Clostridiales, and g-Rothia were the dominant microorganisms in the SGA group on day 5, and Clostridium_sensu_stricto_1 was higher in the SGA group on days 3 and 7, consistent with a previous study on the gut microbiota of neonates with asphyxia (Zhang et al., 2021). Researchers have found that the predominance of o-Clostridiales in infants is associated with poorer communication performance at 3 years of age (Sordillo et al., 2019). Clostridium_sensu_stricto_1 is associated with a variety of inflammatory genes and is considered an opportunistic pathogen associated with intestinal inflammation (Wang et al., 2018; Li et al., 2019; Wen et al., 2021). Studies have found that Rothia is an opportunistic pathogen associated with various infections in humans, and most reported Rothia infections have occurred in patients with pneumonia, endocarditis, peritonitis, and septicemia (Fatahi-Bafghi, 2021). Burkholderiaceae was higher in the SGA group on days 3, 5, and 7. An increased relative abundance of Burkholderiaceae was observed in the gut of rats with cognitive impairment (Rong et al., 2021), and Burkholderiaceae was elevated in the brain tissue of patients with Alzheimer’s disease (AD) (Alonso et al., 2018). The SGA group showed decreased relative abundances of Lactiplantibacillus (1, 3, 5, and 7 days), Streptococcus (3, 5, 7 days), Bifidobacterium, and Lactococcus (3 days). Some strains of these four genera are considered probiotics (Guo et al., 2019; Prete et al., 2020; Wright et al., 2020; Griffin et al., 2022) because they improve memory impairment and cognitive function by reducing inflammation and oxidative stress (Den et al., 2020; Sivamaruthi et al., 2020; Griffin et al., 2022). Ileibacterium was higher in the SGA group on days 1, 5, and 7, and it was the dominant microbiota on day 7. Ileibacterium is a gram-positive anaerobic bacterium related to metabolic health that can decompose polysaccharides (Wang et al., 2021). An increased relative abundance of Ileibacterium has been observed in animal models of intestinal microecological disorders (Xiao et al., 2021; Wu et al., 2022). Akkermansia was higher in the SGA group on days 5 and 7. Akkermansia is the predominant member of Verrucomicrobiota (Wagner and Horn, 2006), with elevated levels in patients with multiple sclerosis (MS) linked to lower disability, and Akkermansia isolated from these patients ameliorates experimental autoimmune encephalomyelitis (EAE), suggesting that increased Akkermansia in MS may be a compensatory beneficial response (Cox et al., 2021).
In summary, large differences were observed in the gut microbiota between the SGA and AGA groups in the first week of life, which can be summarized as follows: (1) compared with AGA infants, the alpha diversity of term SGA infants was significantly lower; (2) certain pathogenic and conditional pathogenic bacteria increased or formed the dominant microorganisms in SGA infants, such as Escherichia_Shigella, Ralstonia, and Clostridium.
After a 6 month follow-up, we found an association between higher alpha diversity on day 3 and communication problems in SGA infants. This finding was surprising because a more mature gut microbial community in infancy usually has a high level of biological diversity (Carlson et al., 2018; Coker et al., 2021), and decreased microbial diversity is related to adverse health outcomes in adults (Rinninella et al., 2019; Nikolova et al., 2021). However, the relationship between microbial diversity and health conditions in children is mixed (Abrahamsson et al., 2014; Kostic et al., 2015; Aatsinki et al., 2019). Our findings are consistent with recent evidence that increased microbial diversity in infancy is not necessarily beneficial for subsequent neurocognitive outcomes (Carlson et al., 2018; Gao et al., 2019; Loughman et al., 2020). One study showed that a higher alpha diversity of the gut microbiota in 1-year-old children was associated with lower overall composite, visual reception, and expressive language scores at the age of 2 years (Carlson et al., 2018). Their team found a correlation between higher levels of microbiome diversity in infancy and weaker thalamus-amygdala connectivity a year later (Gao et al., 2019), while the amygdala regulates emotion and controls learning and memory (McDonald and Mott, 2017). The same study also found positive associations between alpha diversity and functional connectivity between the supplementary motor area (SMA) and the inferior parietal lobule (IPL), while SMA-IPL connectivity at 1 year of age was negatively correlated with cognitive outcomes at 2 years of age (Gao et al., 2019).
With respect to the differential gut microbiota of SGA infants with different neurodevelopmental outcomes at 6 months, g-Allobaculum was the dominant microbiota in the SGA group with a poor communication score on day 1. Allobaculum may provide good anti-inflammatory functions by producing free long-chain fatty acids and short-chain fatty acids (SCFAs) which are related to glucose and lipid metabolism (Wu et al., 2020; Pujo et al., 2021). Therefore, we assumed that Allobaculum-dominant gut microbiome may be a compensatory beneficial response for SGA infants with poor communication scores. We found that p-Bacteroidota, g-Bacteroides, and s_Bacteroides_fragilis increased in abundance and were the dominant microorganisms in the good communication score group on day 7. In addition, we observed correlations between increased abundances of p-Bacteroidota, g-Bacteroides, and s-Bacteroides_fragilis on day 7 and improved communication scores at 6 months, consistent with findings of previous studies on the association between gut microbiota in late infancy and subsequent neurodevelopment (Carlson et al., 2018; Tamana et al., 2021). We found that the relative abundance of s-Bacteroides_fragilis on day 1 was negatively correlated with the fine motor scores, similar to observation of a previous study on the association between Bacteroides-dominant gut microbiota at 3–6 months and subsequent delayed fine motor skills (Sordillo et al., 2019). Bacteroides_fragilis is a gram-negative obligate anaerobe with two subtypes. Enterotoxigenic B. fragilis (ETBF), identified as a common opportunistic pathogen in clinical infections, mainly causes colitis and systemic inflammation with the stimulation of toxins or lipopolysaccharides. The second subtype, non-toxigenic B. fragilis (NTBF), has been suggested as a potential probiotic in recent studies because of its ability to produce immunomodulatory substances such as polysaccharide A (PSA) and SCFAs (Sun et al., 2019; Qu et al., 2022). Non-toxigenic B. fragilis was found to be capable of protecting mice from central nervous system demyelination; this protective mechanism depended on the production of IL-10 (Ochoa-Repáraz et al., 2010). In addition, it was found to improve communication, stereotyped movement, anxiety-like behavior, and sensorimotor behavior in ASD model mice, as well as reduce the increased expression of IL-6 (Hsiao et al., 2013). Decreased levels of intestinal Bacteroides are also characteristic of children diagnosed with ASD (Dan et al., 2020; Iglesias-Vázquez et al., 2020). Clostridium_saccharobutylicum has a strong ability to produce butyrate (Huang et al., 2018; Miguel et al., 2019), and butyrate, as a microbial metabolite, can indirectly affect host metabolism through the gut–brain axis (Stilling et al., 2016). It has been reported that butyrate can reduce the inflammatory response of microglia and the hippocampus, inhibit inflammatory activities, promote the production of BDNF, and repair injured nerves (Kundu et al., 2019). What puzzled us was the negative correlation between the relative abundance of s-Clostridium_saccharobutylicum on day 7 and the gross motor scores at 6 months postnatal. This finding was unexpected and it is unclear why there was such a connection. Thus, we believe further investigation is required to confirm these results.
Briefly, gut microbial characteristics associated with neurological prognosis in SGA infants can be summarized as follows: (1) higher alpha diversity on day 3 was associated with poor communication performance in SGA infants at 6 months of age; (2) Bacteroidota, Bacteroides, Bacteroides_fragilis, and Clostridium_saccharobutylicum may be related to the neurodevelopmental outcomes of SGA infants at 6 months of age.
The development of neonatal gut microbiota is affected by several factors (Bäckhed et al., 2015; Rutayisire et al., 2016; Kapourchali and Cresci, 2020; Vandenplas et al., 2020). Most early colonists in the gut of neonates are maternal, and the mode of delivery strongly affects the formation of the early gut microbiota in term infants (Bäckhed et al., 2015; Rutayisire et al., 2016; Kapourchali and Cresci, 2020; Coelho et al., 2021). Studies have shown that vaginally-delivered neonates have more abundant Bacteroides, Bifidobacterium, and Lactobacillus (Bäckhed et al., 2015; Rutayisire et al., 2016; Coelho et al., 2021), whereas neonates delivered by cesarean section (CS) are enriched in Staphylococcus, Streptococcus, and Clostridium (Coelho et al., 2021). In our study, there was no difference in the alpha diversity of SGA neonates born by vaginal delivery and CS on days 1, 3, and 5, while the alpha diversity of SGA neonates delivered by CS showed a decreasing trend on day 7. Some researchers also found lower microbial diversity in the gut of infants delivered by CS than in vaginally-delivered infants in the first week of life (Bäckhed et al., 2015; MacIntyre et al., 2015; Rutayisire et al., 2016; Shi et al., 2018). LEfSe showed that the dominant microorganisms in vaginally-delivered SGA neonates were g-Bacteroides and g-Ileibacterium. Bacteroides seem to increase in abundance in vaginally-delivered infants compared with CS-delivered infants (Gronlund et al., 1999; Kabeerdoss et al., 2013; Hesla et al., 2014; Jakobsson et al., 2014; Rutayisire et al., 2016; Coelho et al., 2021). In addition, Bifidobacterium and Lactobacillus found in the vaginal delivery group did not increase significantly, in contrast to other findings (Bäckhed et al., 2015; Rutayisire et al., 2016; Coelho et al., 2021). The dominant microbiota of the cesarean birth group was g-Staphylococcus on day 5, similar to findings of previous studies (Li et al., 2018; Wampach et al., 2018; Coelho et al., 2021). However, Streptococcus and Clostridium found in the cesarean birth group did not increase significantly, in contrast to previous findings (Coelho et al., 2021). Therefore, SGA and delivery mode may have different effects on the development of gut microbiota in term neonates.
Antibiotic therapy can greatly alter the diversity and composition of neonatal gut microbiota (Nobel et al., 2015; Gasparrini et al., 2016). Associations between antibiotic therapy and decreased microbial diversity, increased abundance of Firmicutes, and decreased abundance of Bacteroides and Bifidobacterium have been reported (Gasparrini et al., 2016; Ficara et al., 2020). In our study, despite no statistical difference between the alpha diversity of the group with antibiotics and the group without antibiotics, the alpha diversity of SGA neonates treated with antibiotics was lower in the first week of life, similar to previous findings (Gasparrini et al., 2016; Ficara et al., 2020). Hence, we assumed that the therapeutic duration of antibiotics was insufficient to significantly disrupt gut microbial diversity. We also found that the composition of neonatal gut microbiota was altered by antibiotic therapy. However, there was no difference in the relative abundance of Firmicutes, Bifidobacterium, and Bacteroides between the two groups, which differed from previous findings (Ficara et al., 2020).
This study is the first to analyze the characteristics and evolution of the gut microbiota of term SGA infants in the first week of life, and also the first to explore the potential association between specific microbiota and neural development in SGA infants. Alongside the novelty of this study, it is worth mentioning the following limitations. (1) This was a single-center study; there may be differences in the gut microbiota of SGA infants from other hospitals. The results need to be further confirmed by multicenter and large-exponent investigations. (2) We only studied the gut microbiota of term SGA infants within 1 week after birth and the neurological prognosis at 6 months of age; we will follow up with SGA infants and discuss the relationship between neonatal gut microbiota and neurological development by comprehensively considering various factors, such as education mode and society. (3) The ASQ-3 is a screening scale, and because of the prevalence of the novel coronavirus, it was difficult to use diagnostic scales such as the Bayley scale to evaluate the prognosis of neurodevelopment.
Conclusion
Compared to AGA infants, the gut microbial diversity of term SGA infants was significantly lower in the first week of life. Certain pathogenic and conditional pathogenic bacteria increased or formed the dominant microbiota in SGA infants, such as Escherichia_Shigella, Ralstonia, and Clostridium. This study suggests that there may be associations between alpha diversity, certain gut microbiota, and neurodevelopmental outcomes in SGA infants. The results showed that higher alpha diversity on day 3 was associated with poor communication performance in SGA infants at 6 months of age, and the gut microbiota factors affecting the prognosis of SGA infants included Bacteroidota, Bacteroides, Bacteroides_fragilis, and Clostridium_saccharobutylicum. SGA infants are at a higher risk for adverse neurodevelopmental outcomes; however, current methods for the clinical treatment of neurodevelopmental delay in SGA infants are limited. With further studies of the gut microbiota of SGA infants, we hope to provide further insights into the early treatment of SGA infants.
Data availability statement
The datasets presented in this study can be found in online repositories. The names of the repository/repositories and accession number(s) can be found in the article/Supplementary material.
Ethics statement
The studies involving human participants were reviewed and approved by the Ethics Committee of Peking University First Hospital. Written informed consent to participate in this study was provided by the participants’ legal guardian/next of kin.
Author contributions
All authors listed have made great contributions to this work and have read and agreed to the published version of the manuscript.
Funding
This work was supported by grants from the National Key Research and Development Program of China (2021YFC2700700) and Young Scientists Fund of the NSFC (82101806).
Acknowledgments
We would like to thank all the families who took part in this study.
Conflict of interest
The authors declare that the research was conducted in the absence of any commercial or financial relationships that could be construed as a potential conflict of interest.
Publisher’s note
All claims expressed in this article are solely those of the authors and do not necessarily represent those of their affiliated organizations, or those of the publisher, the editors and the reviewers. Any product that may be evaluated in this article, or claim that may be made by its manufacturer, is not guaranteed or endorsed by the publisher.
Supplementary material
The Supplementary Material for this article can be found online at: https://www.frontiersin.org/articles/10.3389/fmicb.2022.912968/full#supplementary-material
References
Aatsinki, A.-K., Lahti, L., Uusitupa, H.-M., Munukka, E., Keskitalo, A., Nolvi, S., et al. (2019). Gut microbiota composition is associated with temperament traits in infants. Brain Behav. Immun. 80, 849–858.
Abrahamsson, T. R., Jakobsson, H. E., Andersson, A. F., Björkstén, B., Engstrand, L., and Jenmalm, M. C. (2014). Low gut microbiota diversity in early infancy precedes asthma at school age. Clin. Exp. Allergy 44, 842–850. doi: 10.1111/cea.12253
Adak, A., and Khan, M. R. (2019). An insight into gut microbiota and its functionalities. Cell Mol. Life Sci. 76, 473–493. doi: 10.1007/s00018-018-2943-4
Al-Asmakh, M., Anuar, F., Zadjali, F., Rafter, J., and Pettersson, S. (2012). Gut microbial communities modulating brain development and function. Gut Microbes 3, 366–373. doi: 10.4161/gmic.21287
Alemohammad, S. M. A., Noori, S. M. R., Samarbafzadeh, E., and Noori, S. M. A. (2022). The role of the gut microbiota and nutrition on spatial learning and spatial memory: a mini review based on animal studies. Mol. Biol. Rep. 49, 1551–1563. doi: 10.1007/s11033-021-07078-2
Alonso, R., Pisa, D., Fernández-Fernández, A. M., and Carrasco, L. (2018). Infection of fungi and bacteria in brain tissue from elderly persons and patients with Alzheimer’s disease. Front. Aging Neurosci. 10:159. doi: 10.3389/fnagi.2018.00159
Bäckhed, F., Roswall, J., Peng, Y., Feng, Q., Jia, H., Kovatcheva-Datchary, P., et al. (2015). Dynamics and stabilization of the human gut microbiome during the first year of life. Cell Host Microbe 17, 690–703. doi: 10.1016/j.chom.2015.04.004
Boltin, D., Niv, Y., Schütte, K., and Schulz, C. (2019). Review: Helicobacter pylori and non-malignant upper gastrointestinal diseases. Helicobacter 24(Suppl.), e12637. doi: 10.1111/hel.12637
Bostanciklioğlu, M. (2019). The role of gut microbiota in pathogenesis of Alzheimer’s disease. J. Appl. Microbiol. 127, 954–967. doi: 10.1111/jam.14264
Carlson, A. L., Xia, K., Azcarate-Peril, M. A., Goldman, B. D., Ahn, M., Styner, M. A., et al. (2018). Infant gut microbiome associated with cognitive development. Biol. Psychiatry 83, 148–159. doi: 10.1016/j.biopsych.2017.06.021
Chawla, D. (2019). Small for gestation age neonates: unmet clinical care and research need. Indian J. Pediatr. 86, 572–573. doi: 10.1007/s12098-019-02986-5
Che, L., Zhou, Q., Liu, Y., Hu, L., Peng, X., Wu, C., et al. (2019). Flaxseed oil supplementation improves intestinal function and immunity, associated with altered intestinal microbiome and fatty acid profile in pigs with intrauterine growth retardation. Food Funct. 10, 8149–8160. doi: 10.1039/c9fo01877h
Chen, Y., Wu, L., Zou, L., Li, G., and Zhang, W. (2017). Update on the birth weight standard and its diagnostic value in small for gestational age(SGA) infants in China. J. Matern. Fetal Neonatal Med. 30, 801–807. doi: 10.1080/14767058.2016.1186636
Cheng, L. H., Liu, Y. W., Wu, C. C., Wang, S., and Tsai, Y. C. (2019). Psychobiotics in mental health, neurodegenerative and neurodevelopmental disorders. J. Food Drug Anal. 27, 632–648. doi: 10.1016/j.jfda.2019.01.002
Coelho, G. D. P., Ayres, L. F. A., Barreto, D. S., Henriques, B. D., Prado, M. R. M. C., and Passos, C. M. D. (2021). Acquisition of microbiota according to the type of birth: an integrative review. Rev. Lat. Am. Enfermagem. 29:e3446. doi: 10.1590/1518.8345.4466.3446
Cohen Kadosh, K., Muhardi, L., Parikh, P., Basso, M., Jan Mohamed, H. J., Prawitasari, T., et al. (2021). Nutritional support of neurodevelopment and cognitive function in infants and young children-an update and novel insights. Nutrients 13:199. doi: 10.3390/nu13010199
Coker, M. O., Laue, H. E., Hoen, A. G., Hilliard, M., Dade, E., Li, Z., et al. (2021). Infant feeding alters the longitudinal impact of birth mode on the development of the gut microbiota in the first year of life. Front. Microbiol. 12:642197. doi: 10.3389/fmicb.2021.642197
Cox, L. M., Maghzi, A. H., Liu, S., Tankou, S. K., Dhang, F. H., Willocq, V., et al. (2021). Gut microbiome in progressive multiple sclerosis. Ann. Neurol. 89, 1195–1211. doi: 10.1002/ana.26084
Cryan, J. F., O’Riordan, K. J., Cowan, C. S. M., Sandhu, K. V., Bastiaanssen, T. F. S., Boehme, M., et al. (2019). The microbiota-gut-brain axis. Physiol. Rev. 99, 1877–2013. doi: 10.1152/physrev.00018.2018
Dabke, K., Hendrick, G., and Devkota, S. (2019). The gut microbiome and metabolic syndrome. J. Clin. Invest. 129, 4050–4057. doi: 10.1172/JCI129194
Dan, Z., Mao, X., Liu, Q., Guo, M., Zhuang, Y., Liu, Z., et al. (2020). Altered gut microbial profile is associated with abnormal metabolism activity of Autism Spectrum Disorder. Gut Microbes 11, 1246–1267. doi: 10.1080/19490976.2020.1747329
Den, H., Dong, X., Chen, M., and Zou, Z. (2020). Efficacy of probiotics on cognition, and biomarkers of inflammation and oxidative stress in adults with Alzheimer’s disease or mild cognitive impairment - a meta-analysis of randomized controlled trials. Aging 12, 4010–4039. doi: 10.18632/aging.102810
Eastwood, J., Walton, G., Van Hemert, S., Williams, C., and Lamport, D. (2021). The effect of probiotics on cognitive function across the human lifespan: a systematic review. Neurosci. Biobehav. Rev. 128, 311–327. doi: 10.1016/j.neubiorev.2021.06.032
Fatahi-Bafghi, M. (2021). Characterization of the Rothia spp. and their role in human clinical infections. Infect. Genet. Evol. 93:104877. doi: 10.1016/j.meegid.2021.104877
Ferrazzano, G. F., Papa, C., Pollio, A., Ingenito, A., Sangianantoni, G., and Cantile, T. (2020). Cyanobacteria and microalgae as sources of functional foods to improve human general and oral health. Molecules 25:5164. doi: 10.3390/molecules25215164
Ficara, M., Pietrella, E., Spada, C., Della Casa Muttini, E., Lucaccioni, L., Iughetti, L., et al. (2020). Changes of intestinal microbiota in early life. J. Matern. Fetal Neonatal Med. 33, 1036–1043. doi: 10.1080/14767058.2018.1506760
Finegold, S. M., Dowd, S. E., Gontcharova, V., Liu, C., Henley, K. E., Wolcott, R. D., et al. (2010). Pyrosequencing study of fecal microflora of autistic and control children. Anaerobe 16, 444–453. doi: 10.1016/j.anaerobe.2010.06.008
Gabriel, I., Olejek, A., Stencel-Gabriel, K., and Wielgoś, M. (2018). The influence of maternal vaginal flora on the intestinal colonization in newborns and 3-month-old infants. J. Matern. Fetal Neonatal Med. 31, 1448–1453. doi: 10.1080/14767058.2017.1319352
Gao, W., Salzwedel, A. P., Carlson, A. L., Xia, K., Azcarate-Peril, M. A., Styner, M. A., et al. (2019). Gut microbiome and brain functional connectivity in infants-a preliminary study focusing on the amygdala. Psychopharmacology 236, 1641–1651. doi: 10.1007/s00213-018-5161-8
Gareau, M. G., Wine, E., Rodrigues, D. M., Cho, J. H., Whary, M. T., Philpott, D. J., et al. (2011). Bacterial infection causes stress-induced memory dysfunction in mice. Gut 60, 307–317.
Gasparrini, A. J., Crofts, T. S., Gibson, M. K., Tarr, P. I., Warner, B. B., and Dantas, G. (2016). Antibiotic perturbation of the preterm infant gut microbiome and resistome. Gut Microbes 7, 443–449. doi: 10.1080/19490976.2016.1218584
Griffin, S. M., Lehtinen, M. J., Meunier, J., Ceolin, L., Roman, F. J., and Patterson, E. (2022). Restorative effects of probiotics on memory impairment in sleep-deprived mice. Nutr. Neurosci. 3, 1–11. doi: 10.1080/1028415X.2022.2042915
Gronlund, M. M., Lehtonen, O. P., Eerola, E., and Kero, P. (1999). Fecal microflora in healthy infants born by different methods of delivery: permanent changes in intestinal flora after cesarean delivery. J. Pediatr. Gastroenterol. Nutr. 28, 19–25. doi: 10.1097/00005176-199901000-00007
Guo, Q., Goldenberg, J. Z., Humphrey, C., El Dib, R., and Johnston, B. C. (2019). Probiotics for the prevention of pediatric antibiotic-associated diarrhea. Cochrane Database Syst. Rev. 4:CD004827. doi: 10.1002/14651858.CD004827.pub5
Hesla, H. M., Stenius, F., Jaderlund, L., Nelson, R., Engstrand, L., Alm, J., et al. (2014). Impact of lifestyle on the gut microbiota of healthy infants and their mothers - the ALADDIN birth cohort. FEMS Microbiol. Ecol. 90, 791–801. doi: 10.1111/1574-6941.12434
Hirayama, M., and Ohno, K. (2021). Parkinson’s disease and gut microbiota. Ann. Nutr. Metab. 77(Suppl. 2), 28–35. doi: 10.1159/000518147
Hsiao, E. Y., McBride, S. W., Hsien, S., Sharon, G., Hyde, E. R., McCue, T., et al. (2013). Microbiota modulate behavioral and physiological abnormalities associated with neurodevelopmental disorders. Cell 155, 1451–1463. doi: 10.1016/j.cell.2013.11.024
Huang, C. N., Liebl, W., and Ehrenreich, A. (2018). Restriction-deficient mutants and marker-less genomic modification for metabolic engineering of the solvent producer Clostridium saccharobutylicum. Biotechnol. Biofuels 11:264. doi: 10.1186/s13068-018-1260-3
Iglesias-Vázquez, L., Van Ginkel Riba, G., Arija, V., and Canals, J. (2020). Composition of gut microbiota in children with autism spectrum disorder: a systematic review and meta-analysis. Nutrients 12:792. doi: 10.3390/nu12030792
Jakobsson, H. E., Abrahamsson, T. R., Jenmalm, M. C., Harris, K., Quince, C., Jernberg, C., et al. (2014). Decreased gut microbiota diversity, delayed Bacteroidetes colonisation and reduced Th1 responses in infants delivered by Caesarean section. Gut 63, 559–566. doi: 10.1136/gutjnl-2012-303249
Kabeerdoss, J., Ferdous, S., Balamurugan, R., Mechenro, J., Vidya, R., Santhanam, S., et al. (2013). Development of the gut microbiota in southern Indian infants from birth to 6 months: a molecular analysis. J. Nutr. Sci. 2:e18. doi: 10.1017/jns.2013.6
Kapourchali, F. R., and Cresci, G. A. M. (2020). Early-life gut microbiome-the importance of maternal and infant factors in its establishment. Nutr. Clin. Pract. 35, 386–405. doi: 10.1002/ncp.10490
Kesavan, K., and Devaskar, S. U. (2019). Intrauterine growth restriction: postnatal monitoring and outcomes. Pediatr. Clin. North Am. 66, 403–423. doi: 10.1016/j.pcl.2018.12.009
Keshavarzian, A., Green, S. J., Engen, P. A., Voigt, R. M., Naqib, A., Forsyth, C. B., et al. (2015). Colonic bacterial composition in Parkinson’s disease. Mov. Disord. 30, 1351–1360. doi: 10.1002/mds.26307
Kim, C. S., Cha, L., Sim, M., Jung, S., Chun, W. Y., Baik, H. W., et al. (2021). Probiotic supplementation improves cognitive function and mood with changes in gut microbiota in community-dwelling older adults: a randomized, double-blind, placebo-controlled, multicenter trial. J. Gerontol. A Biol. Sci. Med. Sci. 76, 32–40. doi: 10.1093/gerona/glaa090
Kostic, A. D., Gevers, D., Siljander, H., Vatanen, T., Hyötyläinen, T., Hämäläinen, A. M., et al. (2015). The dynamics of the human infant gut microbiome in development and in progression toward type 1 diabetes. Cell Host Microbe 17, 260–273. doi: 10.1016/j.chom.2015.01.001
Kundu, P., Lee, H. U., Garcia-Perez, I., Tay, E. X. Y., Kim, H., Faylon, L. E., et al. (2019). Neurogenesis and prolongevity signaling in young germ-free mice transplanted with the gut microbiota of old mice. Sci. Transl. Med. 11:eaau4760. doi: 10.1126/scitranslmed.aau4760
Lee, A. C., Katz, J., Blencowe, H., Cousens, S., Kozuki, N., Vogel, J. P., et al. (2013). National and regional estimates of term and preterm babies born small for gestational age in 138 low-income and middle-income countries in 2010. Lancet Glob. Health 1, e26–e36. doi: 10.1016/S2214-109X(13)70006-8
Li, H., Wang, J., Wu, L., Luo, J., Liang, X., Xiao, B., et al. (2018). The impacts of delivery mode on infant’s oral microflora. Sci. Rep. 8:11938. doi: 10.1038/s41598-018-30397-7
Li, Y., Han, H., Yin, J., He, X., Tang, Z., Li, T., et al. (2019). D- and l-Aspartate regulates growth performance, inflammation and intestinal microbial community in young pigs. Food Funct. 10, 1028–1037. doi: 10.1039/c8fo01410h
Liang, S., Wu, X., and Jin, F. (2018). Gut-brain psychology: rethinking psychology from the microbiota-gut-brain axis. Front. Integr. Neurosci. 12:33. doi: 10.3389/fnint.2018.00033
Long-Smith, C., O’Riordan, K. J., Clarke, G., Stanton, C., Dinan, T. G., and Cryan, J. F. (2020). Microbiota-gut-brain axis: new therapeutic opportunities. Annu. Rev. Pharmacol. Toxicol. 60, 477–502. doi: 10.1146/annurev-pharmtox-010919-023628
Loughman, A., Ponsonby, A. L., O’Hely, M., Symeonides, C., Collier, F., Tang, M. L. K., et al. (2020). Gut microbiota composition during infancy and subsequent behavioural outcomes. EBioMedicine 52:102640. doi: 10.1016/j.ebiom.2020.102640
Ma, Q., Li, Y., Li, P., Wang, M., Wang, J., Tang, Z., et al. (2019). Research progress in the relationship between type 2 diabetes mellitus and intestinal flora. Biomed. Pharmacother. 117:109138. doi: 10.1016/j.biopha.2019.109138
MacIntyre, D. A., Chandiramani, M., Lee, Y. S., Kindinger, L., Smith, A., Angelopoulos, N., et al. (2015). The vaginal microbiome during pregnancy and the postpartum period in a European population. Sci. Rep. 5:8988. doi: 10.1038/srep08988
Martí, M., Spreckels, J. E., Ranasinghe, P. D., Wejryd, E., Marchini, G., Sverremark-Ekström, E., et al. (2021). Effects of Lactobacillus reuteri supplementation on the gut microbiota in extremely preterm infants in a randomized placebo-controlled trial. Cell Rep. Med. 2:100206. doi: 10.1016/j.xcrm.2021.100206
McCowan, L. M., Figueras, F., and Anderson, N. H. (2018). Evidence-based national guidelines for the management of suspected fetal growth restriction: comparison, consensus, and controversy. Am. J. Obstet. Gynecol. 218, S855–S868. doi: 10.1016/j.ajog.2017.12.004
McDonald, A. J., and Mott, D. D. (2017). Functional neuroanatomy of amygdalohippocampal interconnections and their role in learning and memory. J. Neurosci. Res. 95, 797–820. doi: 10.1002/jnr.23709
Mentella, M. C., Scaldaferri, F., Pizzoferrato, M., Gasbarrini, A., and Miggiano, G. A. D. (2020). Nutrition, IBD and gut microbiota: a review. Nutrients 12:944. doi: 10.3390/nu12040944
Miguel, M., Lee, S. S., Mamuad, L., Choi, Y. J., Jeong, C. D., Son, A., et al. (2019). Enhancing butyrate production, ruminal fermentation and microbial population through supplementation with Clostridium saccharobutylicum. J. Microbiol. Biotechnol. 29, 1083–1095. doi: 10.4014/jmb.1905.05016
Nikolova, V. L., Hall, M. R. B., Hall, L. J., Cleare, A. J., Stone, J. M., and Young, A. H. (2021). Perturbations in gut microbiota composition in psychiatric disorders: a review and meta-analysis. JAMA Psychiatry 78, 1343–1354. doi: 10.1001/jamapsychiatry.2021.2573
Nobel, Y. R., Cox, L. M., Kirigin, F. F., Bokulich, N. A., Yamanishi, S., Teitler, I., et al. (2015). Metabolic and metagenomic outcomes from early-life pulsed antibiotic treatment. Nat. Commun. 6:7486. doi: 10.1038/ncomms8486
Ochoa-Repáraz, J., Mielcarz, D. W., Wang, Y., Begum-Haque, S., Dasgupta, S., Kasper, D. L., et al. (2010). A polysaccharide from the human commensal Bacteroides fragilis protects against CNS demyelinating disease. Mucosal Immunol. 3, 487–495. doi: 10.1038/mi.2010.29
On, S. L., Hynes, S., and Wadström, T. (2002). Extragastric Helicobacter species. Helicobacter 7(Suppl. 1), 63–67. doi: 10.1046/j.1523-5378.7.s1.2.x
Plaza-Díaz, J., Gómez-Fernández, A., Chueca, N., Torre-Aguilar, M. J., Gil, Á, Perez-Navero, J. L., et al. (2019). Autism spectrum disorder (ASD) with and without mental regression is associated with changes in the fecal microbiota. Nutrients 11:337. doi: 10.3390/nu11020337
Prete, R., Garcia-Gonzalez, N., Di Mattia, C. D., Corsetti, A., and Battista, N. (2020). Food-borne Lactiplantibacillus plantarum protect normal intestinal cells against inflammation by modulating reactive oxygen species and IL-23/IL-17 axis. Sci. Rep. 10:16340. doi: 10.1038/s41598-020-73201-1
Pujo, J., Petitfils, C., Le Faouder, P., Eeckhaut, V., Payros, G., Maurel, S., et al. (2021). Bacteria-derived long chain fatty acid exhibits anti-inflammatory properties in colitis. Gut 70, 1088–1097. doi: 10.1136/gutjnl-2020-321173
Qu, D., Sun, F., Feng, S., Yu, L., Tian, F., Zhang, H., et al. (2022). Protective effects of Bacteroides fragilis against lipopolysaccharide-induced systemic inflammation and their potential functional genes. Food Funct. 13, 1015–1025. doi: 10.1039/d1fo03073f
Ragusa, M., Santagati, M., Mirabella, F., Lauretta, G., Cirnigliaro, M., Brex, D., et al. (2020). Potential associations among alteration of salivary miRNAs, saliva microbiome structure, and cognitive impairments in autistic children. Int. J. Mol. Sci. 21:6203. doi: 10.3390/ijms21176203
Rinninella, E., Raoul, P., Cintoni, M., Franceschi, F., Miggiano, G. A. D., Gasbarrini, A., et al. (2019). What is the healthy gut microbiota composition? a changing ecosystem across age, environment, diet, and diseases. Microorganisms 7:14. doi: 10.3390/microorganisms7010014
Rong, W., Han, K., Zhao, Z., An, J., Li, Q., and Bi, K. (2021). The protective effect of Xanthoceras sorbifolia Bunge husks on cognitive disorder based on metabolomics and gut microbiota analysis. J. Ethnopharmacol. 279:113094. doi: 10.1016/j.jep.2020.113094
Roy Sarkar, S., and Banerjee, S. (2019). Gut microbiota in neurodegenerative disorders. J. Neuroimmunol. 328, 98–104. doi: 10.1016/j.jneuroim.2019.01.004
Rutayisire, E., Huang, K., Liu, Y., and Tao, F. (2016). The mode of delivery affects the diversity and colonization pattern of the gut microbiota during the first year of infants’ life: a systematic review. BMC Gastroenterol. 16:86. doi: 10.1186/s12876-016-0498-0
Same, R. G., and Tamma, P. D. (2018). Campylobacter infections in children. Pediatr. Rev. 39, 533–541. doi: 10.1542/pir.2017-0285
Saurman, V., Margolis, K. G., and Luna, R. A. (2020). Autism spectrum disorder as a brain-gut-microbiome axis disorder. Dig. Dis. Sci. 65, 818–828. doi: 10.1007/s10620-020-06133-5
Seki, D., Mayer, M., Hausmann, B., Pjevac, P., Giordano, V., Goeral, K., et al. (2021). Aberrant gut-microbiota-immune-brain axis development in premature neonates with brain damage. Cell Host Microbe 29, 1558.e4–1572.e4. doi: 10.1016/j.chom.2021.08.004
Sharma, D., Farahbakhsh, N., Shastri, S., and Sharma, P. (2016). Intrauterine growth restriction - part 2. J. Matern. Fetal Neonatal Med. 29, 4037–4048. doi: 10.3109/14767058.2016.1154525
Shi, Y. C., Guo, H., Chen, J., Sun, G., Ren, R. R., Guo, M. Z., et al. (2018). Initial meconium microbiome in Chinese neonates delivered naturally or by cesarean section. Sci. Rep. 8:3255. doi: 10.1038/s41598-018-21657-7
Sivamaruthi, B. S., Suganthy, N., Kesika, P., and Chaiyasut, C. (2020). The role of microbiome, dietary supplements, and probiotics in autism spectrum disorder. Int. J. Environ. Res. Public Health 17:2647. doi: 10.3390/ijerph17082647
Sordillo, J. E., Korrick, S., Laranjo, N., Carey, V., Weinstock, G. M., Gold, D. R., et al. (2019). Association of the infant gut microbiome with early childhood neurodevelopmental outcomes: an ancillary study to the VDAART randomized clinical trial. JAMA Netw Open 2:e190905. doi: 10.1001/jamanetworkopen.2019.0905
Stilling, R. M., van de Wouw, M., Clarke, G., Stanton, C., Dinan, T. G., and Cryan, J. F. (2016). The neuropharmacology of butyrate: the bread and butter of the microbiota-gut-brain axis? Neurochem. Int. 99, 110–132. doi: 10.1016/j.neuint.2016.06.011
Sun, F., Zhang, Q., Zhao, J., Zhang, H., Zhai, Q., and Chen, W. (2019). A potential species of next-generation probiotics? The dark and light sides of Bacteroides fragilis in health. Food Res. Int. 126:108590. doi: 10.1016/j.foodres.2019.108590
Tamana, S. K., Tun, H. M., Konya, T., Chari, R. S., Field, C. J., Guttman, D. S., et al. (2021). Bacteroides-dominant gut microbiome of late infancy is associated with enhanced neurodevelopment. Gut Microbes 13, 1–17. doi: 10.1080/19490976.2021.1930875
Vandenplas, Y., Carnielli, V. P., Ksiazyk, J., Luna, M. S., Migacheva, N., Mosselmans, J. M., et al. (2020). Factors affecting early-life intestinal microbiota development. Nutrition 78:110812. doi: 10.1016/j.nut.2020.110812
Vascellari, S., Palmas, V., Melis, M., Pisanu, S., Cusano, R., Uva, P., et al. (2020). Gut microbiota and metabolome alterations associated with Parkinson’s disease. mSystems 5:e00561-20. doi: 10.1128/mSystems.00561-20
Wagner, M., and Horn, M. (2006). The Planctomycetes, Verrucomicrobia, Chlamydiae and sister phyla comprise a superphylum with biotechnological and medical relevance. Curr. Opin. Biotechnol. 17, 241–249. doi: 10.1016/j.copbio.2006.05.005
Wampach, L., Heintz-Buschart, A., Fritz, J. V., Ramiro-Garcia, J., Habier, J., Herold, M., et al. (2018). Birth mode is associated with earliest strain-conferred gut microbiome functions and immunostimulatory potential. Nat. Commun. 9:5091. doi: 10.1038/s41467-018-07631-x
Wang, H., Lee, I. S., Braun, C., and Enck, P. (2016). Effect of probiotics on central nervous system functions in animals and humans: a systematic review. J. Neurogastroenterol. Motil. 22, 589–605. doi: 10.5056/jnm16018
Wang, J., Ji, H., Wang, S., Liu, H., Zhang, W., Zhang, D., et al. (2018). Probiotic Lactobacillus plantarum promotes intestinal barrier function by strengthening the epithelium and modulating gut microbiota. Front. Microbiol. 9:1953. doi: 10.3389/fmicb.2018.01953
Wang, Y., Ablimit, N., Zhang, Y., Li, J., Wang, X., Liu, J., et al. (2021). Novel β-mannanase/GLP-1 fusion peptide high effectively ameliorates obesity in a mouse model by modifying balance of gut microbiota. Int. J. Biol. Macromol. 191, 753–763. doi: 10.1016/j.ijbiomac.2021.09.150
Wen, X., Wang, H. G., Zhang, M. N., Zhang, M. H., Wang, H., and Yang, X. Z. (2021). Fecal microbiota transplantation ameliorates experimental colitis via gut microbiota and T-cell modulation. World J. Gastroenterol. 27, 2834–2849. doi: 10.3748/wjg.v27.i21.2834
Wong, O. W. H., Lam, A. M. W., Or, B. P. N., Mo, F. Y. M., Shea, C. K. S., Lai, K. Y. C., et al. (2022). Disentangling the relationship of gut microbiota, functional gastrointestinal disorders and autism: a case-control study on prepubertal Chinese boys. Sci. Rep. 12:10659. doi: 10.1038/s41598-022-14785-8
Wright, M. E., Yu, A. O., Marco, M. L., and Panigrahi, P. (2020). Genome sequence of Lactiplantibacillus plantarum ATCC 202195, a probiotic strain that reduces sepsis and other infections during early infancy. Microbiol. Resour. Announc. 9:e00741-20. doi: 10.1128/MRA.00741-20
Wu, M., Yang, S., Wang, S., Cao, Y., Zhao, R., Li, X., et al. (2020). Effect of berberine on atherosclerosis and gut microbiota modulation and their correlation in high-fat diet-Fed ApoE-/- mice. Front. Pharmacol. 11:223. doi: 10.3389/fphar.2020.00223
Wu, X., Chen, H., Gao, X., Gao, H., He, Q., Li, G., et al. (2022). Natural herbal remedy wumei decoction ameliorates intestinal mucosal inflammation by inhibiting Th1/Th17 cell differentiation and maintaining microbial homeostasis. Inflamm. Bowel Dis. [Epub ahead of print]. doi: 10.1093/ibd/izab348
Xiao, X., Cheng, Y., Fu, J., Lu, Z., Wang, F., Jin, M., et al. (2021). Gut immunity and microbiota dysbiosis are associated with altered bile acid metabolism in LPS-challenged piglets. Oxid. Med. Cell Longev. 2021:6634821. doi: 10.1155/2021/6634821
Zhang, W., Ma, C., Xie, P., Zhu, Q., Wang, X., Yin, Y., et al. (2019). Gut microbiota of newborn piglets with intrauterine growth restriction have lower diversity and different taxonomic abundances. J. Appl. Microbiol. 127, 354–369. doi: 10.1111/jam.14304
Zhang, X., Liu, L., Bai, W., Han, Y., and Hou, X. (2021). Evolution of intestinal microbiota of asphyxiated neonates within 1 week and its relationship with neural development at 6 months. Front. Pediatr. 9:690339. doi: 10.3389/fped.2021.690339
Keywords: gut microbiota, Bacteroides, SGA, neonates, neurodevelopment
Citation: Chen X, Yan Z, Liu L, Zhang R, Zhang X, Peng C, Geng Y, Zhou F, Han Y and Hou X (2022) Characteristics of gut microbiota of term small gestational age infants within 1 week and their relationship with neurodevelopment at 6 months. Front. Microbiol. 13:912968. doi: 10.3389/fmicb.2022.912968
Received: 05 April 2022; Accepted: 29 July 2022;
Published: 24 August 2022.
Edited by:
Ekaterina Avershina, Oslo University Hospital, NorwayReviewed by:
Francesca Ronchi, University of Bern, SwitzerlandShan Liang, Institute of Microbiology (CAS), China
Rakesh Singh, National Institute of Pharmaceutical Education and Research, India
Copyright © 2022 Chen, Yan, Liu, Zhang, Zhang, Peng, Geng, Zhou, Han and Hou. This is an open-access article distributed under the terms of the Creative Commons Attribution License (CC BY). The use, distribution or reproduction in other forums is permitted, provided the original author(s) and the copyright owner(s) are credited and that the original publication in this journal is cited, in accordance with accepted academic practice. No use, distribution or reproduction is permitted which does not comply with these terms.
*Correspondence: Ying Han, aGFueWluZzE1NjhAMTI2LmNvbQ==; Xinlin Hou, eGlubGluLmhvdUBwa3VmaC5jb20=
†These authors share first authorship