- 1Centre for Ecological Research, Institute of Evolution, Budapest, Hungary
- 2MTA-ELTE, Research Group of Theoretical Biology and Evolutionary Ecology, Eötvõs University, Budapest, Hungary
- 3Center for Evolutionary Hologenomics, GLOBE Institute, University of Copenhagen, Copenhagen, Denmark
- 4Department of Computational Biology, University of Lausanne, Lausanne, Switzerland
- 5Swiss Institute of Bioinformatics, Lausanne, Switzerland
Microbiomes provide key ecological functions to their host; however, most host-associated microbiomes are too complicated to allow a model of essential host–microbe–microbe interactions. The intestinal microbiota of salmonids may offer a solution since few dominating species often characterize it. Healthy fish coexist with a mutualistic Mycoplasma sp. species, while stress allows the spread of pathogenic strains, such as Aliivibrio sp. Even after a skin infection, the Mycoplasma does not recover; Aliivibrio sp. often remains the dominant species, or Mycoplasma–Aliivibrio coexistence was occasionally observed. We devised a model involving interactions among the host immune system, Mycoplasma sp. plus a toxin-producing pathogen. Our model embraces a complete microbiota community and is in harmony with experimental results that host–Mycoplasma mutualism prevents the spread of pathogens. Contrary, stress suppresses the host immune system allowing dominance of pathogens, and Mycoplasma does not recover after stress disappears.
Introduction
Almost every eukaryotic organism hosts an associated core microbial community providing key biological functions to the host (McFall-Ngai et al., 2013; Bosch and Miller, 2016; Müller et al., 2016). This has led influential thinkers to coin the term holobiont as describing the sum of a host and its commensal microbes (Margulis, 1990; Baedke et al., 2020). These host–microbiota systems range in complexity from one-to-one symbiotic associations between a host and a single microorganism, such as the bioluminescent Aliivibrio bacteria in light organs of bob-tail squids (Nyholm and McFall-Ngai, 2004), to intricate arrangements between a host and a dynamic community of microorganisms like vertebrates and their gut microbiota (Ley et al., 2008), or plants and their root microbiota (Sasse et al., 2018). The renewed realization that microbes play essential roles for the hosts has catalyzed an increased focus on the study of host–bacteria and bacteria–bacteria dynamics within a holobiont (Zilber-Rosenberg and Rosenberg, 2008; Bordenstein and Theis, 2015; Theis et al., 2016). In extension, the generation of knowledge potentially allowing active manipulation of holobionts has become a global strategic priority across life sciences (Małyska et al., 2019), including food production (Limborg et al., 2018).
One approach to better understand microbiome dynamics is ecological models that include a realistic parameter space for characterizing host–microbe interactions in the holobiont. Microbiomes of animal hosts are generally very complex (Gralka et al., 2020; Alberdi et al., 2021). So far, theoretical studies have achieved limited success in explaining empirical data of these complex systems. Even verification of more simplified feedback and dynamical models describing host–microbe interactions remains scarce (Abbott et al., 2021; Remien et al., 2021). The challenge becomes even more significant if we model how pathogenic microbes interact with the host and the host's commensal and mutualistic contingent of the host–microbiome dynamics (Coyte et al., 2015; Rúa and Umbanhowar, 2015; Jiang et al., 2020). Indeed, to adequately describe realistic host–microbiome dynamics, models must consider at least two key factors that have been ignored in attempts to model realistic holobiont systems reflecting empirical data. First, the host immune system needs to be included as it is known to control microbiome composition (Earley et al., 2018; Zheng et al., 2020). Second, microbial metabolites can act as toxins, common goods, or resources, further shaping the qualitative dynamics of the system (Scheuring and Yu, 2012; Rybicki et al., 2018; Kokou et al., 2019; Gralka et al., 2020). We address this challenge by studying a holobiont system containing relatively few microbial members while covering the complete microbiome community.
Recent investigations have revealed a general trend of low diversity among intestinal microbiota in teleosts compared to warm-blooded animals, including numerous studies from commercially important species such as Atlantic salmon (Salmo salar) and rainbow trout (Oncorhynchus mykiss) (Huang et al., 2020). Adult salmon are piscivorous and characterized by physiological adaptations necessary to cope with a strictly carnivorous diet. These adaptations may extend to an adaptive composition of its associated gut microbiota. Furthermore, several studies have revealed that the intestinal microbiota of salmonids is characterized by strikingly low diversity, with as little as one or two species dominating the microbial biomass (Holben et al., 2002; Llewellyn et al., 2016; Bozzi et al., 2021; Wang et al., 2021). Together, these observations suggest that salmon and related species are well-suited holobiont systems to study concrete biological interactions between a eukaryotic host and its commensal microbiota (Limborg et al., 2018; Nyholm et al., 2020; Alberdi et al., 2021).
Mycoplasma sp. has recently emerged as a core and often dominating member of the gut microbiome in some salmonid species. This novel Mycoplasma species have been reported at high-relative abundances in the gut of different salmonids species in numerous independent studies over the past 20 years (Holben et al., 2002; Zarkasi et al., 2014; Lowrey et al., 2015; Llewellyn et al., 2016; Dehler et al., 2017; Lyons et al., 2017; Brown et al., 2019; Rimoldi et al., 2019; Bozzi et al., 2021; Rasmussen et al., 2022a,b). Mycoplasma sp. abundance has been associated with enhanced health conditions (Bozzi et al., 2021), disese resilience (Rasmussen et al., 2022b), and improved growth performances (Rimoldi et al., 2019; Bozzi et al., 2021) of the salmonid host. More detailed studies using genome-resolved metagenomics further point toward a putative mutualistic relationship between Mycoplasma sp. and its salmonid hosts (Cheaib et al., 2021a; Rasmussen et al., 2021). For example, Mycoplasma sp. can provide the host with a suite of beneficial functions, such as arginine biosynthesis, ammonia detoxification, and degradation of long-chain polymers, which could improve the nutritional value of both chitin-rich diet and strict carnivory during the juvenile and adult life stages of salmon (Rasmussen et al., 2021).
Interestingly, the proposed beneficial role of Mycoplasma sp. is further supported by numerous observations where slower-growing or disease-susceptible salmonid cohorts have a reduced abundance of Mycoplasma sp. in concomitance with the increase of pathogenic/opportunistic strains (Table 1). These observations, together with the resolved Mycoplasma phylogeny (Rasmussen et al., 2021), suggest a mutualistic relationship, thus providing an excellent system to further model and understand adaptively important host–microbe and microbe–microbe interactions. Here, we build upon a previous case study to develop a simple mathematical model describing the dynamics of an observed change in Mycoplasma sp. abundance in a sick cohort of Atlantic salmon.
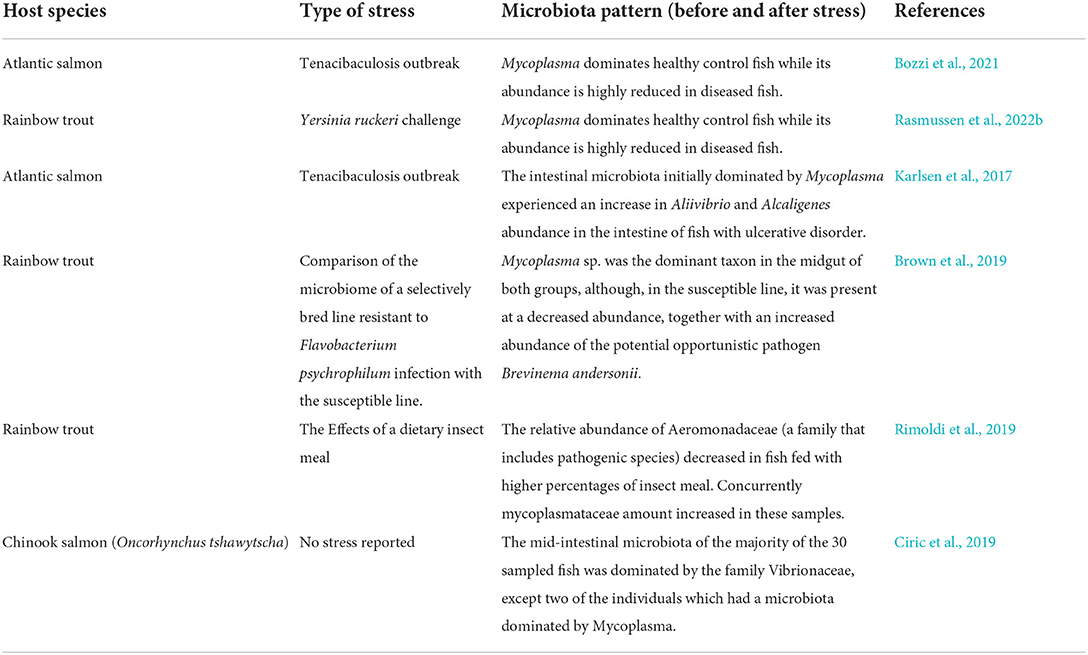
Table 1. A non-exhaustive list of relevant studies showing similar positive correlations with Mycoplasma sp. abundance and fish health.
The study of Bozzi et al. (2021) provides a valuable dataset to describe a model involving interactions among the host immune system, Mycoplasma sp. plus a toxin-producing pathogenic competitor as the two dominant gut microbes. Bozzi et al. (2021) assessed changes in the composition of the Atlantic salmon distal gut microbiota in the context of a bacterial skin infection caused by the pathogen Tenacibaculum dicentrarchi. The infected fish developed an ulcerative skin disease, which would eventually lead to the death of the fish. The researchers collected samples from the distal gut content and the distal gut mucosa tissue of both healthy and diseased salmon. The stressful event was resolved by a water disinfection treatment aimed at killing the skin pathogen. The sampling procedure was then repeated after treatment. The microbiome composition was investigated with 16S rRNA amplicon sequencing and described the relative abundance of dominating microbial species (Figure 1). Before treatment, most healthy fish had a Mycoplasma-dominated gut microbiome. The infection, even if it affects the outer skin of the host, allows the spread of an opportunistic and potentially pathogenic Aliivibrio strain, leading to its dominance in the gut of the sick fish. These observations were consistent with the gut content and mucosa tissue samples. After treatment of the infection, the Mycoplasma-dominated microbiomes of healthy fish do not recover, and Aliivibrio sp. remains the dominant species in most of the samples of the gut mucosa tissue. Instead, in the gut content, we observe the presence of some samples showing patterns of Mycoplasma–Aliivibrio coexistence (Figure 1).
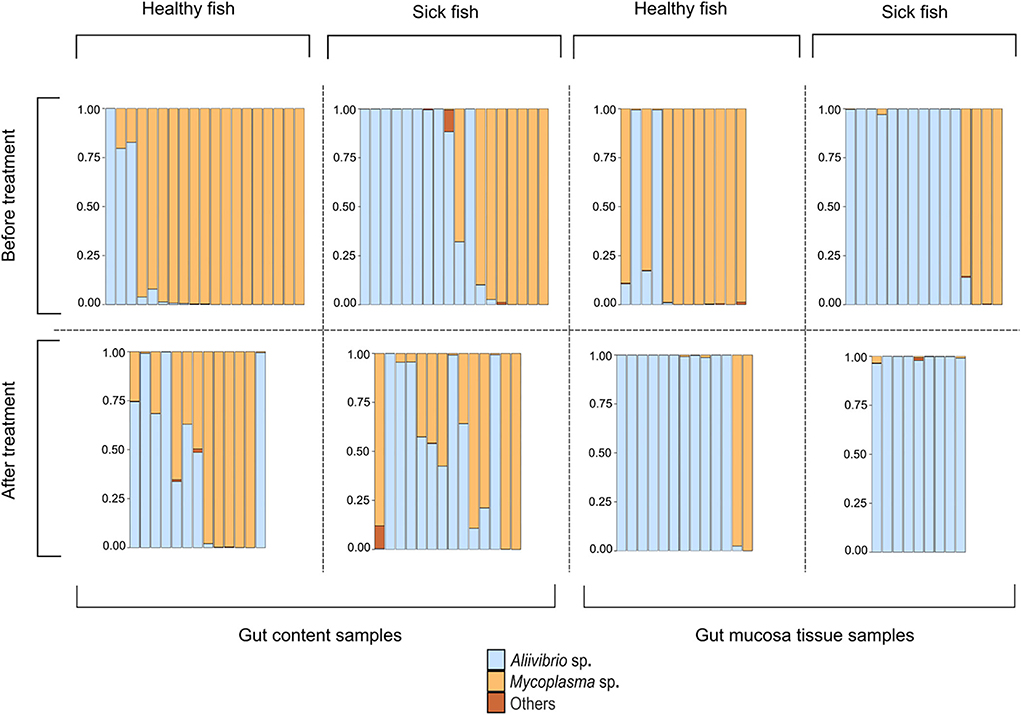
Figure 1. Intestinal microbiota composition for the two dominant bacterial species Mycoplasma sp. and Aliivibrio sp. for eight distinct cohorts of Atlantic salmon during a disease outbreak. Each bar represents one fish. The eight cohorts represent three relevant variables pertaining to the sampled tissue (gut content vs. gut mucosa), the health status during a Tenacibaculosis outbreak (Healthy vs. Sick), and whether fish were sampled before or after treatment against the Tenacibaculosis causing pathogen Tenacibaculum dicentrarchi (before vs. after treatment). The figure has been reformatted based on data from Bozzi et al. (2021).
In this study, we consider the study of Bozzi et al. (2021) as a case to model and understand the dynamics of a concrete host–microbe–microbe model exemplified by the Mycoplasma-dominated salmonid microbiomes in the context of a stressful event and the emergence of an opportunistic/pathogenic bacteria.
Based on the experimental observations described above, we define the following assumptions about the case study for building our model:
1. Salmon and Mycoplasma form a mutualistic relationship (Rasmussen et al., 2021), so we assume that the immune system of the host increases the carrying capacity of the Mycoplasma in the distal gut, and vice versa the presence of Mycoplasma activates the immune system either directly or indirectly by keeping the host in a healthier state (Cerf-Bensussan and Gaboriau-Routhiau, 2010; Pérez et al., 2010; Koch and Schmid-Hempel, 2011; Earley et al., 2018; Xiong et al., 2019).
2. Aliivibrio is a putative toxin-producing pathogen of salmonids. The assumption is based on the fact that the known Aliivibrio sp. generally infects its host with the help of a toxin by suppressing the immune system (Shinoda, 1999; Karlsen et al., 2014; Pérez-Reytor et al., 2018). We build our assumption on these studies to allow the pathogenic species to exert a negative impact on mutualistic bacteria in the model.
3. Mycoplasma colonizes the intestine of salmonids in the juvenile phase (Cheaib et al., 2021a; Rasmussen et al., 2022b) before the Aliivibrio can infect it. Alternatively, it can be the case that Aliivibrio infection in the juvenile phase is highly lethal for the host, which does not modify our argument below.
4. Mycoplasma and Aliivibrio compete in the distal intestine; that is, space and nutrients are common limiting factors of these two species. Additionally, Aliivibrio can also be toxic for Mycoplasma, which is considered in the model.
5. Infection or other stress factors elicit an acute immune response that will remove resources from other fish metabolic processes, including transcription of host genes usually involved in maintaining gut homeostasis in the host fish (Tort, 2011; Nardocci et al., 2014; Cámara-Ruiz et al., 2021).
Materials and methods
We consider a simple dynamical model to describe the dynamics of the host immune system, the mutualistic microbe, and the invading toxic producing bacterium. As we argued above, in the case of salmonid hosts, the resident microbiome is typically dominated by Mycoplasma. Still, after some stress, the microbiome is frequently replaced by an opportunistic pathogenic Aliivibrio species. We apply the common Lotka–Volterra competition model to describe the Mycoplasma–Aliivibrio competition in their common habitat. We define a model where the mutualistic Mycoplasma facilitates the immune system of the host; in return, the host's immune system selectively helps to maintain a higher density of Mycoplasma in the gut. Furthermore, we use a simple model for the pathogen-immune sub-system, where the Aliivibrio pathogen produces toxins that inhibit immune response while immune effectors try to eliminate pathogens (Rybicki et al., 2018). Figure 2 depicts the interactions between the microbes and host, and the corresponding dynamical system is the following:
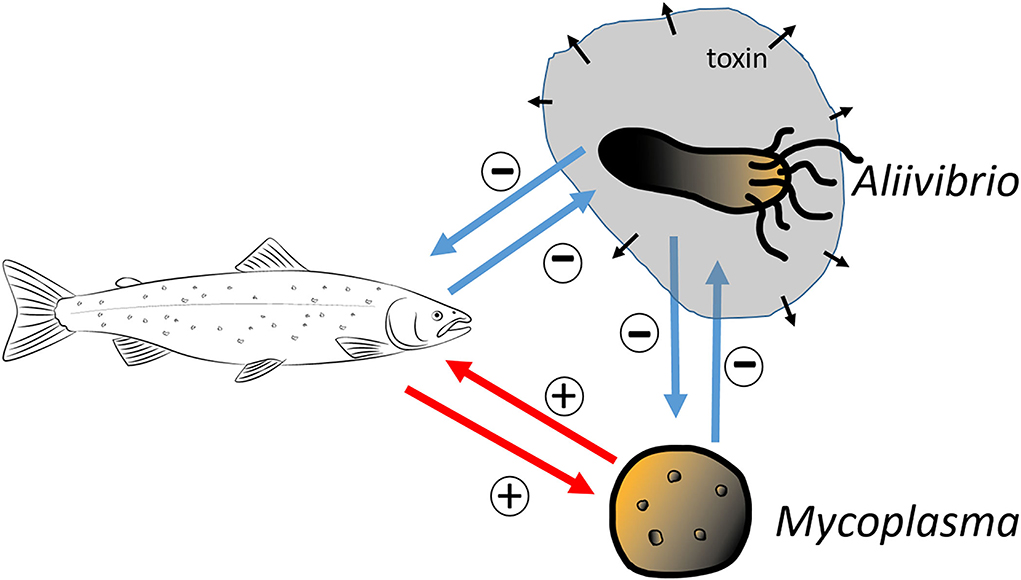
Figure 2. A conceptual schematic of the model. The salmon and Mycoplasma engaged in mutualistic interaction with each other (+ signs at the red arrows). Conversely, the toxin-producing Aliivibrio harms the salmon, which in turn defends itself via its immune response (– signs at the blue arrows). The Mycoplasma and Aliivibrio species of the model compete for the same niches and resources so that the expansion of one species is at the expense of the other species (– signs at the blue arrows).
where A and M are the concentration of Aliivibrio and Mycoplasma species in the gut of the host, T and I are the concentration of toxin and immune effectors, r and s are the growth rates of microbes and the effector cells, and k is the rate at which the immune effector eliminates the pathogen Aliivibrio. aMA and aAM are the intraspecific competition coefficients. s and m are the toxin production and decay rates, and e is the rate at which toxin (or any other mechanism by the pathogen) inactivates the active immune effectors. KM(I), I0(M) are increasing saturating functions of I and M, in harmony with the assumptions that Mycoplasma activates the immune system to reach a higher equilibrium proliferation level, while in return the immune system of the host enhances the carrying capacity of the Mycoplasma:
Parameters ε and δ determine the maximal effect of M and I on I0 and KM, while β and σ are the half-saturation constants of these functions. If the host can only tolerate Mycoplasma (that is immune system neither support nor harm it), then δ and probably ε are zero in the previous functions. In this case, only the direct competition of Mycoplasma with Aliivibrio has to be taken into account, a situation that we will also analyze later.
We might assume that the dynamics of I and T are much faster than the dynamics of microbes (s, m, r, e ≫ rA, rM), that is, = in Equations (1c,1d) (Rybicki et al., 2018). Consequently, the concentrations of immune effectors and toxins in steady-state are I*(A, M) = =
By substituting I*(A, M) and T* into (1a, 1b), we receive the following dynamical system for A and M:
where are variables, and other parameters are rescaled to . π is the relative immune efficiency, μ is the relative toxin efficiency, and aMA and aAM are the rescaled intraspecific competition coefficients, which are the key parameters of the model.
Results
We examine the condition that the invading Aliivibrio could not spread if Mycoplasma is the dominant microbial resident of the host. According to the experimental observations, we assume that Mycoplasma arrives earlier in the distal intestine [typically in the early juvenile phase (Llewellyn et al., 2016)] than Aliivibrio and dominates in this section of the intestine before the infection. Mycoplasma reaches its equilibrium density, the stable fixed point of (2b) when A = 0. It is easy to show that 1 < M* < 1+δ, the solution of , is the only stable fixed point of (2b). Aliivibrio could not invade the Mycoplasma dominated microbiome if in the case of A ≈ 0 and M = M*. This leads to the following relation:
which is satisfied if
where . However, there are two different cases even if relation (4) is valid. If
then Aliivibrio can never spread independently to its initial dose. However, if then Aliivibrio spreads if its initial concentration is above a critical level. So, there is a critical dose of the pathogen above which it can infect the host. Naturally, if (4) is not valid, then ; thus, Aliivibrio always spreads independently to its initial concentration.
Mycoplasma defends the host by having a direct competition with Aliivibrio, which is manifested in the parameter α*; however, it also benefits the host indirectly by facilitating its immune response, which is involved in the parameter ε*. Notably, the direct and the indirect effects both take a role in relations (4) and (5); that is, Mycoplasma not only prevents the rare Aliivibrio from spreading, but its presence increases the critical dose of Aliivibrio above which it can spread (see Figure 3).
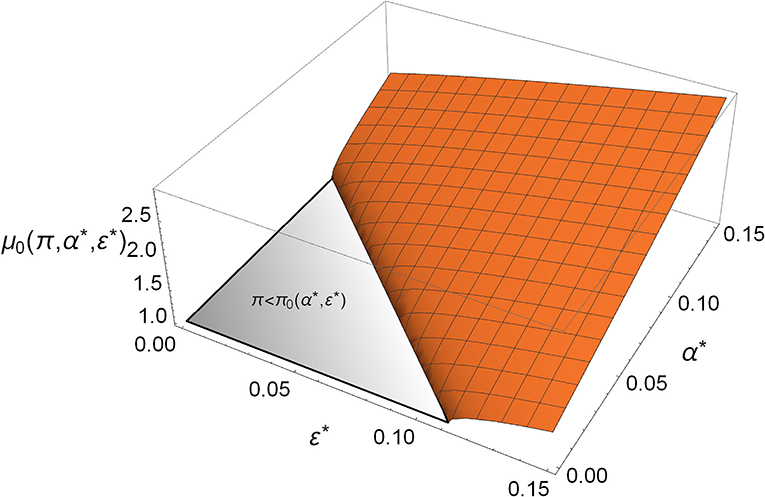
Figure 3. The critical toxin efficiency (μ0) in the function of direct competition (α*) and immune system facilitation (ε*). The gray triangle denotes the region where rare Aliivibrio always/spreads in the Mycoplasma-dominated microbiome []. The orange region covers the α*, ε* values where rare Aliivibrio cannot invade. If the actual which can be satisfied more easily when α*, ε* are bigger, then the host is defended from the invasion of even a high dose of Aliivibrio, (π = 0.9).
According to the experimental results, Aliivibrio cannot expand in healthy hosts where Mycoplasma dominates the microbial abundance in the distal intestine (Bozzi et al., 2021) (Figure 1). Hence, this means that relation (4) and probably (5) are valid in most cases in healthy fishes. Assume that infection on the skin or any other external stress suppresses the immune system or decreases the health condition of the host, which causes a less effective immune reaction. Thus I0, and consequently π decreases. It leads to a decrease in M*, and thus in α* and in ε*. Therefore, the right-hand side of (4) increases while the left-hand side decreases.
For the same reason, the right-hand side of (5) decreases too. Consequently, it can happen that π, the actual relative immune efficiency is no longer sufficiently high to prevent the spread of the pathogen. Alternatively, it can happen as well that the decrease of π and M* keeps the relation (4) valid, but μ, the relative toxin efficiency becomes higher than the threshold level in (5), thus because of the stress a higher dose of pathogens can spread in the host.
The results presented by Bozzi et al. (2021) also suggest that Mycoplasma generally cannot spread if Aliivibrio becomes the dominant microbe in the distal intestine (Figure 1). This means in our model that if M ≈ 0 when A = A* < 1 is at the equilibrium density. Substituting these values into (2b), we receive that guarantees that Mycoplasma invading in a low dose (rare invader) could not spread in an Aliivibrio dominated microbiota. Since A* < 1, therefore aMA > 1 is necessary to satisfy the previous relation. This means that the negative effect of Aliivibrio on Mycoplasma should be more intense than the negative effect of Aliivibrio on itself (since this constant is normalized to one in the model). This happens if the Aliivibrio species actively destroys the living conditions of Mycoplasma. Since most Aliivibrio strains produce toxin, it is conceivable that toxin harms Mycoplasma too, which mapped to the condition aMA > 1 in our model. Contrary, if the competing efficiency of Aliivibrio is not strong enough, that is if then rare Mycoplasma can spread to the Aliivibrio dominated state.
Collecting the possible invasion scenarios listed above, there are four qualitatively different competition situations if an invasion of Mycoplasma is not possible at lower π-s (higher A*) but possible at higher π-s (lower A*): (a) Aliivibrio is dominant over Mycoplasma, (b) neither rare invaders can spread, so the system is bistable, and (c) Aliivibrio can spread above a critical concentration while rare Mycoplasma can spread. The two species either are in stable coexistence or Mycoplasma is the winner of the competition, (d) Mycoplasma is dominant over Aliivibrio (Figure 4; Supplementary material).
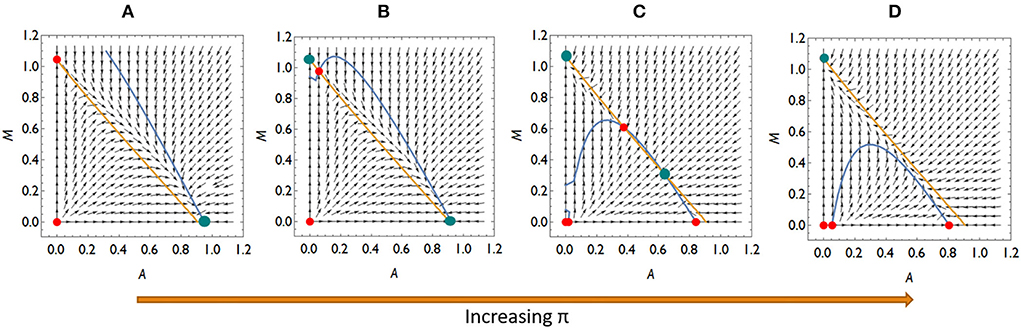
Figure 4. The qualitatively different dynamics of the Mycoplasma–Aliivibrio system when Aliivibrio deteriorate Mycoplasma living conditions. The nullclines of (A,B) are depicted (yellow dM/dt = 0, blue dA/dt = 0), so their intersections define the fixed points of the dynamics. Red points denote the unstable, while green points denote the stable fixed points of the system. At low relative immune efficiency (π) Aliivibrio dominates the dynamics (A). At intermediate π the system is bistable (B,C), while at high π Mycoplasma dominates the dynamics (D). Parameters: (A) π = 0.4, (B) π = 0.6, (C) π = 1.1, (d) π = 1.3, other parameters are the same for all subfigures: rA = rM = 1, aAM = 0.5 aMA = 1.1, ε = 0.1, σ = 0.5, δ = 0.1, β = 0.5, μ = 7.
Importantly, the microbial pattern experienced in Figure 1 can be explained by the behavior of our model. The stress decreases I0, thus it decreases π as well. This allows Aliivibrio to spread and dominate the total microbial abundance (Figure 4A). After the treatment, parameter I0 recovers leading to an increase of π again, but if this recovered π is not high enough, then Aliivibrio remains dominant (Figure 4B) or the two strains coexist after reinvasion of Mycoplasma (Figure 4C). The observation of different microbial states of the hosts after treatment (Figure 1) can be the consequence of different health states, such as the immune efficiency π, of the hosts which, as we have shown, can lead to different microbiome dynamics.
Let us also consider what dynamic cases are possible if Aliivibrio cannot significantly hamper the living conditions of Mycoplasma even though Aliivibrio's toxin suppresses the salmon host's immune system. Then aMA < 1, thus rare Mycoplasma can always replace the resident Aliivibrio population. There are typically three different dynamical scenarios in this case. There is a stable coexistence of species for weak relative immune efficiency (Figure 5A), while the system is bistable with a coexistence or a Mycoplasma only stable state for intermediate relative immune efficiency (Figure 5B). For high relative immune efficiency, Mycoplasma will be dominant as in the previous scenario (compare Figure 4D with Figure 5C). Under these conditions, stress does not lead to the displacement of Mycoplasma. However, the coexistence of the strains is the expected outcome, which is still compatible with the experimental results for some individuals (see Figure 1). However, assuming this dynamic situation, the Mycoplasma concentrations should increase after the stress is removed (after treatment). But this is not what we see in the experiment. Therefore, we can assume that once Aliivibrio reaches a particular concentration, it negatively impacts the living conditions of Mycoplasma, a scenario following our analyses above (Figures 4A,B) as the typical case.
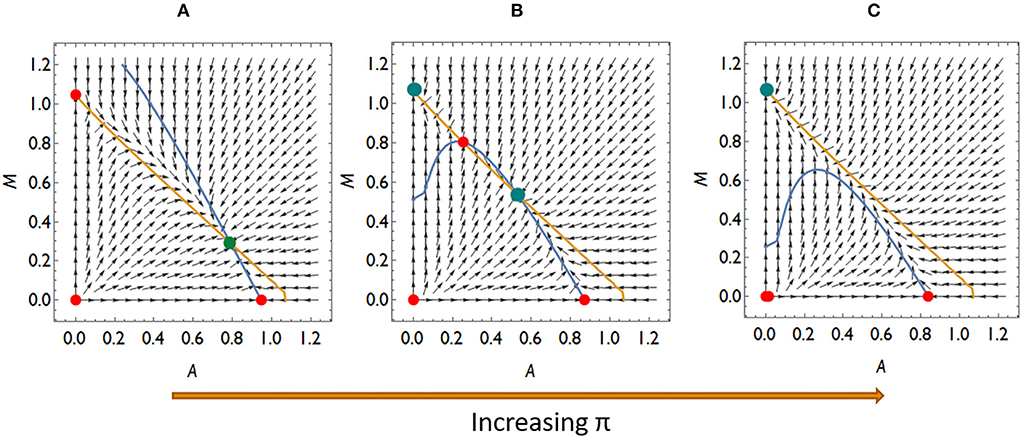
Figure 5. The qualitatively different dynamics of the Mycoplasma–Aliivibrio system when rare Mycoplasma always invades Aliivibrio. The nullclines of (1–2) are depicted (yellow dM/dt = 0, blue dA/dt = 0). Red dots denote the unstable fixed points, green ones denote the stable fixed points of the system. At low-relative immune efficiency (π), rare species invade and Mycoplasma are in coexistence with Aliivibrio (A). At intermediate π the system is bistable (B), while at high π Mycoplasma dominates the dynamics (C). Parameters: (A) π = 0.4, (B) π = 0.9, (C) π = 1.1, (D) other parameters are the same for all subfigures: rA = rM = 1, aAM = 0.5 aMA = 0.9, ε = 0.1, σ = 0.5, δ = 0.1, β = 0.5, μ = 7 .
The model parameters determine which of the above scenarios will play out. The dynamical parameters can be considered constant in a given host–microbiome system, except π, the relative efficiency of the immune system, which can decrease and increase because of stress and treatment. As π increases, we can move from an Aliivibrio-dominated stable microbiome to a Mycoplasma dominated state via bistable behavior, including the stable coexistence of Mycoplasma and Aliivibrio.
Since the coexistence of Mycoplasma and Aliivibrio after the stress is occasionally observed and the reinvasion of Mycoplasma after the treatment is experienced too, although it is not typical in the Atlantic salmon experiment (Figure 1), it is likely that Aliivibrio actively harms Mycoplasma (aMA > 1). However, according to the experimental results depicted in Figure 1, stress decreases π to a value where Aliivibrio becomes dominant (Figure 4A), and after treatment, it can increase only to allow a bistable state (Figures 5B,C) in most cases. Naturally, these observations do not exclude that the scenario presented in Figure 5 occurs in other salmonid-related Mycoplasma pathogen systems.
Mycoplasma does not facilitate the immune system, and the immune system does not increase Mycoplasma concentration
We consider here the case when Mycoplasma and the host conform to a mutualistic interaction or where the host tolerates Mycoplasma. However, the immune system is not facilitated by Mycoplasma, that is, ε = 0 in the model. These modifications did not lead to qualitative changes compared to the previous analysis. The difference is only quantitative, making Mycoplasma stable against invasion of Aliivibrio in a narrower parameter space [see Equations (4 and 5)]. Similarly, suppose the host immune system does not increase the carrying capacity of Mycoplasma directly; that is, when δ = 0, then M* = 1, which again does not change the previous derivations except that the invasion of Aliivibrio will be more likely [see Equations (4 and 5)].
The invader species (Aliivibrio) does not suppress the host immune system
To make a comprehensive analysis, we consider the situation when Aliivibrio does not harm the efficiency of the immune system directly. This means formally in the model that μ = 0. The consequence is that the dosage effect disappears in the system; that is, rare Aliivibrio simply cannot invade the resident Mycoplasma if Equation (4) is valid, and invades if this relationship does not hold. Since it is assumed that Aliivibrio does not produce a toxin, Aliivibrio does not deteriorate Mycoplasma habitat, that is aMA < 1 (intraspecific competition is more robust than interspecific) should be valid in the model. So, Mycoplasma invariably invades the Aliivibrio-dominated community (dM/dt > 0 if M ≈ 0 and A = A* < 1).
Two different dynamical outcomes are possible, either Mycoplasma dominates for stronger relative immune efficiency [π is bigger, Equation (4) is invalid], or the two competing strains are in coexistence [π is lower, Equation (4) is valid]. Consequently, stress never leads to Aliivibrio dominance which contradicts the experimental results presented in Figure 1.
Discussion
We present one of the first models able to describe a, albeit simple, complete intestinal microbiome community of a vertebrate host. Our model stands out from predecessors by considering realistic parameters of the host immune function, a mutualist microbe able to induce host immune reactions, and a toxin-producing pathogenic microbe. The dynamics explained by our model are in line with multiple empirical observations (Table 1).
Based on the experimental observations described, we assumed that salmon and Mycoplasma form a mutualistic relationship in a way that the immune system of the host increases the carrying capacity of Mycoplasma in the distal gut, and vice versa, the presence of Mycoplasma can boost the immune response of the host. Furthermore, we assume that Aliivibrio represents any toxin-producing intestinal pathogen of salmonids. Mycoplasma is believed to colonize the intestine of salmon in the juvenile phase before the Aliivibrio can infect it. Mycoplasma and Aliivibrio compete in the distal intestine, where Aliivibrio can be toxic for Mycoplasma, which is also considered in the model. The last assumption of the model is that infection or other stress factors elicit an acute immune response that will remove resources from other metabolic processes in the host fish.
Analyzing the mathematical model of the above system, we have shown that Mycoplasma helps to prevent the host from the Aliivibrio infection. If relative immune efficiency is high enough, Aliivibrio cannot invade (Figures 4D, 5C). Suppose the host is infected or stressed in any way that leads to an immuno-deprived state, or the Mycoplasma density reduces for any reason, then Aliivibrio can spread and replace Mycoplasma (Figures 4A,B). We have shown that if Aliivibrio becomes dominant in the distal intestine, then Mycoplasma cannot invade in low concentrations if the toxin harms Mycoplasma growth (Figure 4B). The system is bistable in a wide range of relative immune efficiency: depending on the parameters, the two stable states are: Mycoplasma only and Aliivibrio only (Figure 4B) or Mycoplasma only and coexistence of Mycoplasma and Aliivibrio (Figures 4C, 5B). The system flips from the Mycoplasma only state to the other one if the invader Aliivibrio concentration is high enough. Mycoplasma and the host immune system define that critical level of invasion. Together, this prevents the pathogen from spreading easily in a way that, besides the level of relative immune efficiency, the level of mutualism helps the competitive ability of Mycoplasma involved in the protection from the pathogen (Figure 3). We emphasize here that the behavior of the model explains the observations of a previous experiment (Figure 1). Furthermore, while Mycoplasma–Aliivibrio dominant microbiomes are widespread in salmonid hosts (Table 1), it is highly likely that the dynamics covered by our model are common in both these economically important and numerous related species. Our analysis points out that, due to the bistability of the system, the Aliivibrio dominant state can only be eliminated by introducing high doses of Mycoplasma. A possible solution would be to feed the individuals infected by Aliivibrio with the gut content (or shredded intestine) of healthy individuals carrying high intestinal biomass of Mycoplasma sp.
Since some assumptions of the model are based only on indirect observations, consequently we examined the robustness of the model to these assumptions. We have shown that the dynamical behavior does not change qualitatively if the immune system of the host and Mycoplasma do not help each other directly (ε = 0, δ = 0); however, the presence of mutual help (ε > 0, δ > 0) increases the range of conditions where the Mycoplasma dominated state is stable against invasion of Aliivibrio. Similarly, the dosage effect, the possibility of mutual invasion of Mycoplasma and Aliivibrio, and stable coexistence of them are possible even if Aliivibrio does not harm Mycoplasma effectively (Figures 4A,B). Contrary, in a model where Aliivibrio does not harm the immune system, the Allee effect (invasion only above a critical concentration of Aliivibrio) disappears. Naturally, outer stress suppressing the immune system still facilitates invasion of the pathogen, but successful invasion always leads to the coexistence of Mycoplasma and Aliivibrio, which is not compatible with the results of (Bozzi et al., 2021) (see Figure 1).
Naturally, there are simplifications of the study. First, it should be stated that the Mycoplasma component in our model represents a single dominant species following the observations listed in Table 1, whereas numerous distinct species of Mycoplasma may be associated with their fish hosts, including the skin tissue (Cheaib et al., 2021b). The model neglects the spatial constraints and heterogeneities present in the gut and the non-even distributions of the cells and materials by the finite speed of diffusion of these materials and cells. Based on previous studies, however, it is highly probable that we do not lose the essence of the dynamics with these simplifications (compare e.g., Scheuring and Yu, 2012) with (Boza et al., 2019). To make the model tractable, we consider only the dominant species of the community, and the immune system dynamics are highly simplified. While this is an excellent first step toward developing models that help us move from only studying host–microbe and microbe–microbe interactions to better understand host–microbe–microbe interactions, the effect of our simplifications needs to be further explored in the context of species with more complex gut microbiome communities.
In summary, our model robustly describes the patterns seen in the experiments and remains consistent with other experimental observations. Based on the model, it is expected that the unfavorable Aliivibrio dominated microbiome community after stress can, in most cases, only be restored to a favorable Mycoplasma dominated state by introducing a high dose of Mycoplasma. We propose to test this specific hypothesis and the broader relevance of our model in future experiments.
Data availability statement
The original contributions presented in the study are included in the article/Supplementary material, further inquiries can be directed to the corresponding author/s.
Author contributions
IS designed and analyzed the mathematical model. IS, JR, DB, and ML wrote the paper. IS and ML designed the project. DB crafted Figure 1. DB and JR performed the literature research for Table 1. All authors contributed to the article and approved the submitted version.
Funding
The research was funded by the Hungarian National Fund, NKFI (K128289) to IS, the Independent Research Fund Denmark (HappyFish, Grant No. 8022-00005B), the Danish National Research Foundation Grant No. DNRF143 to ML, and the Two European Union's Horizon 2020 Actions HoloFood (817729) to ML as well as FindingPheno (952914) to IS and ML.
Acknowledgments
We thank Dr. Harald Sveier for helping design the underlying trial and interpreting the empirical results.
Conflict of interest
The authors declare that the research was conducted in the absence of any commercial or financial relationships that could be construed as a potential conflict of interest.
Publisher's note
All claims expressed in this article are solely those of the authors and do not necessarily represent those of their affiliated organizations, or those of the publisher, the editors and the reviewers. Any product that may be evaluated in this article, or claim that may be made by its manufacturer, is not guaranteed or endorsed by the publisher.
Supplementary material
The Supplementary Material for this article can be found online at: https://www.frontiersin.org/articles/10.3389/fmicb.2022.912806/full#supplementary-material
References
Abbott, K. C., Eppinga, M. B., Umbanhowar, J., Baudena, M., and Bever, J. D. (2021). Microbiome influence on host community dynamics: conceptual integration of microbiome feedback with classical host-microbe theory. Ecol. Lett. 24, 2796–2811. doi: 10.1111/ele.13891
Alberdi, A., Andersen, S. B., Limborg, M. T., Dunn, R. R., and Gilbert, M. T. P. (2021). Disentangling host-microbiota complexity through hologenomics. Nat. Rev. Genetics. 21, 23. doi: 10.1038/s41576-021-00421-0
Baedke, J., Fábregas-Tejeda, A., and Nieves Delgado, A. (2020). The holobiont concept before Margulis. J. Experiment. Zool. Part B Mol. Develop. Evol. 334, 149–155. doi: 10.1002/jez.b.22931
Bordenstein, S. R., and Theis, K. R. (2015). Host biology in light of the microbiome: ten principles of holobionts and hologenomes. PLoS Biol. 13, e1002226. doi: 10.1371/journal.pbio.1002226
Bosch, T. C. G., and Miller, D. J. (2016). The Holobiont Imperative: Perspectives from Early Emerging Animals. New York, NY: Springer.
Boza, G., Worsley, S. F., Yu, D. W., and Scheuring, I. (2019). Efficient assembly and long-term stability of defensive microbiomes via private resources and community bistability. PLoS Computat. Biol. 15, e1007109. doi: 10.1371/journal.pcbi.1007109
Bozzi, D., Rasmussen, J. A., Carøe, C., Sveier, H., Nordøy, K., Gilbert, M. T. P., et al. (2021). Salmon gut microbiota correlates with disease infection status: potential for monitoring health in farmed animals. Anim. Microb. 3, 1–17. doi: 10.1186/s42523-021-00096-2
Brown, R. M., Wiens, G. D., and Salinas, I. (2019). Analysis of the gut and gill microbiome of resistant and susceptible lines of rainbow trout (Oncorhynchus mykiss). Fish Shellfish Immunol. 86, 497–506. doi: 10.1016/j.fsi.2018.11.079
Cámara-Ruiz, M., Cerezo, I. M., Guardiola, F. A., García-Beltrán, J. M., Balebona, M. C., Moriñigo, M. Á., et al. (2021). Alteration of the immune response and the microbiota of the skin during a natural infection by vibrio harveyi in european seabass (Dicentrarchus labrax). Microorganisms 9, 5. doi: 10.3390/microorganisms9050964
Cerf-Bensussan, N., and Gaboriau-Routhiau, V. (2010). The immune system and the gut microbiota: friends or foes? Nat. Rev. Immunol. 10, 735–744. doi: 10.1038/nri2850
Cheaib, B., Seghouani, H., and Llewellyn, M. (2021b). The yellow perch (Perca flavescens) microbiome revealed resistance to colonisation mostly associated with neutralism driven by rare taxa under cadmium disturbance. Anim. Microbiome 3, 3. doi: 10.1186/s42523-020-00063-3
Cheaib, B., Yang, P., Kazlauskaite, R., Lindsay, E., Heys, C., Dwyer, T., et al. (2021a). Genome erosion and evidence for an intracellular niche—exploring the biology of mycoplasmas in Atlantic salmon. Aquaculture 21, 736772. doi: 10.1016/j.aquaculture.2021.736772
Ciric, M., Waite, D., Draper, J., Jones, J., and Brian. (2019). Characterization of mid-intestinal microbiota of farmed Chinook salmon using 16S rRNA gene metabarcoding. Archiv. Biologic. Sci. 71, 577–587. doi: 10.2298/ABS190402040C
Coyte, K. Z., Schluter, J., and Foster, K. R. (2015). The ecology of the microbiome: networks, competition, and stability. Science 350, 663–666. doi: 10.1126/science.aad2602
Dehler, C. E., Secombes, C. J., and Martin, S. A. M. (2017). Environmental and physiological factors shape the gut microbiota of Atlantic salmon parr (Salmo salar L.). Aquaculture 467, 149–157. doi: 10.1016/j.aquaculture.2016.07.017
Earley, A. M., Graves, C. L., and Shiau, C. E. (2018). Critical role for a subset of intestinal macrophages in shaping gut microbiota in adult zebrafish. Cell Rep. 25, 424–436. doi: 10.1016/j.celrep.2018.09.025
Gralka, M., Szabo, R., Stocker, R., and Cordero, O. X. (2020). Trophic interactions and the drivers of microbial community assembly. Curr. Biol. CB 30, R1176–R1188. doi: 10.1016/j.cub.2020.08.007
Holben, W. E., Williams, P., Gilbert, M. A., Saarinen, M., Särkilahti, L. K., and Apajalahti, J. H. A. (2002). Phylogenetic analysis of intestinal microflora indicates a novel Mycoplasma phylotype in farmed and wild salmon. Microb. Ecol. 44, 175–185. doi: 10.1007/s00248-002-1011-6
Huang, Q., Sham, R. C., Deng, Y., Mao, Y., Wang, C., Zhang, T., et al. (2020). Diversity of gut microbiomes in marine fishes is shaped by host-related factors. Mol. Ecol. 20, 1699. doi: 10.1111/mec.15699
Jiang, J., Abbott, K. C., Baudena, M., Eppinga, M. B., Umbanhowar, J. A., and Bever, J. D. (2020). Pathogens and mutualists as joint drivers of host species coexistence and turnover: implications for plant competition and succession. Am. Natural. 195, 591–602. doi: 10.1086/707355
Karlsen, C., Ottem, K. F., Brevik, Ø. J., Davey, M., Sørum, H., and Winther-Larsen, H. C. (2017). The environmental and host-associated bacterial microbiota of Arctic seawater-farmed Atlantic salmon with ulcerative disorders. J. Fish Dis. 40, 1645–1663. doi: 10.1111/jfd.12632
Karlsen, C., Vanberg, C., Mikkelsen, H., and Sørum, H. (2014). Co-infection of Atlantic salmon (Salmo salar), by Moritella viscosa and Aliivibrio wodanis, development of disease and host colonization. Veterin. Microbiol. 171, 112–121. doi: 10.1016/j.vetmic.2014.03.011
Koch, H., and Schmid-Hempel, P. (2011). Socially transmitted gut microbiota protect bumble bees against an intestinal parasite. Proceed. Nat. Acad. Sci. USA 108, 19288–19292. doi: 10.1073/pnas.1110474108
Kokou, F., Sasson, G., Friedman, J., Eyal, S., Ovadia, O., Harpaz, S., et al. (2019). Core gut microbial communities are maintained by beneficial interactions and strain variability in fish. Nat. Microbiol. 4, 2456–2465. doi: 10.1038/s41564-019-0560-0
Ley, R. E., Lozupone, C. A., Hamady, M., Knight, R., and Gordon, J. I. (2008). Worlds within worlds: evolution of the vertebrate gut microbiota. Nat. Rev. Microbiol. 6, 776–788. doi: 10.1038/nrmicro1978
Limborg, M. T., Alberdi, A., Kodama, M., Roggenbuck, M., Kristiansen, K., and Gilbert, M. T. P. (2018). Applied Hologenomics: feasibility and Potential in Aquaculture. Trends Biotechnol. 36, 252–264. doi: 10.1016/j.tibtech.2017.12.006
Llewellyn, M. S., McGinnity, P., Dionne, M., Letourneau, J., Thonier, F., Carvalho, G. R., et al. (2016). The biogeography of the atlantic salmon (Salmo salar) gut microbiome. The ISME J. 10, 1280–1284. doi: 10.1038/ismej.2015.189
Lowrey, L., Woodhams, D. C., Tacchi, L., and Salinas, I. (2015). Topographical mapping of the rainbow trout (Oncorhynchus mykiss) microbiome reveals a diverse bacterial community with antifungal properties in the skin. Appl. Environ. Microbiol. 81, 6915–6925. doi: 10.1128/AEM.01826-15
Lyons, P. P., Turnbull, J. F., Dawson, K. A., and Crumlish, M. (2017). Phylogenetic and functional characterization of the distal intestinal microbiome of rainbow trout Oncorhynchus mykiss from both farm and aquarium settings. J. Appl. Microbiol. 122, 347–363. doi: 10.1111/jam.13347
Małyska, A., Markakis, M. N., Pereira, C. F., and Cornelissen, M. (2019). The microbiome: a life science opportunity for our society and our planet. Trends Biotechnol. 37, 1269–1272. doi: 10.1016/j.tibtech.2019.06.008
Margulis, L. (1990). Words as battle cries–symbiogenesis and the new field of endocytobiology. Bioscience 40, 673–677. doi: 10.2307/1311435
McFall-Ngai, M., Hadfield, M. G., Bosch, T. C. G., Carey, H. V., Domazet-Lošo, T., Douglas, A. E., et al. (2013). Animals in a bacterial world, a new imperative for the life sciences. Proceed. Nat. Acad. Sci. USA 110, 3229–3236. doi: 10.1073/pnas.1218525110
Müller, D. B., Vogel, C., Bai, Y., and Vorholt, J. A. (2016). The plant microbiota: systems-level insights and perspectives. Ann. Rev. Genetics 50, 211–234. doi: 10.1146/annurev-genet-120215-034952
Nardocci, G., Navarro, C., Cortés, P. P., Imarai, M., Montoya, M., Valenzuela, B., et al. (2014). Neuroendocrine mechanisms for immune system regulation during stress in fish. Fish Shellfish Immunol. 40, 531–538. doi: 10.1016/j.fsi.2014.08.001
Nyholm, L., Koziol, A., Marcos, S., Botnen, A. B., Aizpurua, O., Gopalakrishnan, S., et al. (2020). Holo-omics: integrated host-microbiota multi-omics for basic and applied biological research. iScience 23, 101414. doi: 10.1016/j.isci.2020.101414
Nyholm, S. V., and McFall-Ngai, M. J. (2004). The winnowing: establishing the squid-vibrio symbiosis. Nat. Rev. Microbiol. 2, 632–642. doi: 10.1038/nrmicro957
Pérez, T., Balcázar, J. L., Ruiz-Zarzuela, I., Halaihel, N., Vendrell, D., de Blas, I., et al. (2010). Host–microbiota interactions within the fish intestinal ecosystem. Mucosal Immunol. 3, 355–360. doi: 10.1038/mi.2010.12
Pérez-Reytor, D., Jaña, V., Pavez, L., Navarrete, P., and García, K. (2018). Accessory toxins of vibrio pathogens and their role in epithelial disruption during infection. Front. Microbiol. 9, 2248. doi: 10.3389/fmicb.2018.02248
Rasmussen, J. A., Villumsen, K. R., Duchêne, D. A., Puetz, L. C., Delmont, T. O., Sveier, H., et al. (2021). Genome-resolved metagenomics suggests a mutualistic relationship between Mycoplasma and salmonid hosts. Communicat. Biol. 4, 579. doi: 10.1038/s42003-021-02105-1
Rasmussen, J. A., Villumsen, K. R., Ernst, M., Hansen, M., Forberg, T., Gopalakrishnan, S., et al. (2022a). A multi-omics approach unravels metagenomic and metabolic alterations of a probiotic and synbiotic additive in rainbow trout (Oncorhynchus mykiss). Microbiome 10, 21. doi: 10.1186/s40168-021-01221-8
Rasmussen, J. A., Villumsen, K. R., von Gersdorff Jørgensen, L., Forberg, T., Zuo, S., Kania, P. W., et al. (2022b). Integrative analyses of probiotics, pathogenic infections, and host immune response highlight the importance of gut microbiota in understanding disease recovery in rainbow trout (Oncorhynchus mykiss). J. Appl. Microbiol. 22, 1543. doi: 10.1111/jam.15433
Remien, C. H., Eckwright, M. J., and Ridenhour, B. J. (2021). Structural identifiability of the generalized Lotka–Volterra model for microbiome studies. Royal Soc. Open Sci. 8, 201378. doi: 10.1098/rsos.201378
Rimoldi, S., Gini, E., Iannini, F., Gasco, L., and Terova, G. (2019). The effects of dietary insect meal from hermetia illucens prepupae on autochthonous gut microbiota of rainbow trout (Oncorhynchus mykiss). Animals: Open Access J. MDPI 9, 4. doi: 10.3390/ani9040143
Rúa, M. A., and Umbanhowar, J. (2015). Resource availability determines stability for mutualist–pathogen–host interactions. Theoretic. Ecol. 8, 133–148. doi: 10.1007/s12080-014-0237-5
Rybicki, J., Kisdi, E., and Anttila, J. V. (2018). Model of bacterial toxin-dependent pathogenesis explains infective dose. Proceed. Nat. Acad. Sci. USA 115, 10690–10695. doi: 10.1073/pnas.1721061115
Sasse, J., Martinoia, E., and Northen, T. (2018). Feed your friends: do plant exudates shape the root microbiome? Trends Plant Sci. 23, 25–41. doi: 10.1016/j.tplants.2017.09.003
Scheuring, I., and Yu, D. W. (2012). How to assemble a beneficial microbiome in three easy steps. Ecology Lett. 15, 1300–1307. doi: 10.1111/j.1461-0248.2012.01853.x
Theis, K. R., Dheilly, N. M., Klassen, J. L., Brucker, R. M., Baines, J. F., Bosch, T. C. G., et al. (2016). Getting the hologenome concept right: an eco-evolutionary framework for hosts and their microbiomes. mSystems 1, 2. doi: 10.1128/mSystems.00028-16
Tort, L. (2011). Stress and immune modulation in fish. Development. Comparat. Immunol. 35, 1366–1375. doi: 10.1016/j.dci.2011.07.002
Wang, J., Jaramillo-Torres, A., Li, Y., Kortner, T. M., Gajardo, K., Brevik, Ø. J., et al. (2021). Microbiota in intestinal digesta of Atlantic salmon (Salmo salar), observed from late freshwater stage until one year in seawater, and effects of functional ingredients: a case study from a commercial sized research site in the Arctic region. Animal Microbiome 3, 14. doi: 10.1186/s42523-021-00075-7
Xiong, J.-B., Nie, L., and Chen, J. (2019). Current understanding on the roles of gut microbiota in fish disease and immunity. Zoologic> Res. 40, 70–76. doi: 10.24272/j.issn.2095-8137.2018.069
Zarkasi, K. Z., Abell, G. C. J., Taylor, R. S., Neuman, C., Hatje, E., Tamplin, M. L., et al. (2014). Pyrosequencing-based characterization of gastrointestinal bacteria of Atlantic salmon (Salmo salar L.) within a commercial mariculture system. J. Appl. Microbiol. 117, 18–27. doi: 10.1111/jam.12514
Zheng, D., Liwinski, T., and Elinav, E. (2020). Interaction between microbiota and immunity in health and disease. Cell Res. 30, 492–506. doi: 10.1038/s41422-020-0332-7
Keywords: bistability, mutualism, stress, pathogens, salmonids, microbiome, Mycoplasma sp., Aliivibrio sp.
Citation: Scheuring I, Rasmussen JA, Bozzi D and Limborg MT (2022) A strategic model of a host–microbe–microbe system reveals the importance of a joint host–microbe immune response to combat stress-induced gut dysbiosis. Front. Microbiol. 13:912806. doi: 10.3389/fmicb.2022.912806
Received: 04 April 2022; Accepted: 06 July 2022;
Published: 04 August 2022.
Edited by:
George Tsiamis, University of Patras, GreeceReviewed by:
Bachar Cheaib, University of Glasgow, United KingdomAmanda Carroll-Portillo, University of New Mexico, United States
Copyright © 2022 Scheuring, Rasmussen, Bozzi and Limborg. This is an open-access article distributed under the terms of the Creative Commons Attribution License (CC BY). The use, distribution or reproduction in other forums is permitted, provided the original author(s) and the copyright owner(s) are credited and that the original publication in this journal is cited, in accordance with accepted academic practice. No use, distribution or reproduction is permitted which does not comply with these terms.
*Correspondence: Morten T. Limborg, morten.limborg@sund.ku.dk