- 1Laboratory of Environmental Hygiene, School of Allied Health Sciences, Kitasato University, Sagamihara, Japan
- 2Interdisciplinary Center for River Basin Environment, University of Yamanashi, Kofu, Japan
- 3Institute of Medicine, Tribhuvan University Teaching Hospital, Kathmandu, Nepal
Antibiotic-resistant bacteria-associated infections are responsible for more than 1.2 million annual deaths worldwide. In low- and middle-income countries (LMICs), the consumption of antibiotics for human and veterinary uses is not regulated effectively. Overused and misused antibiotics can end up in aquatic environments, which may act as a conduit for antibiotic resistance dissemination. However, data on the prevalence of antibiotic resistance determinants in aquatic environments are still limited for LMICs. In this study, we evaluated the prevalence and concentration of antibiotic resistance genes (ARGs) in different drinking and environmental water sources collected from the Kathmandu Valley, Nepal, using droplet digital polymerase chain reaction to understand the current situation of ARG contamination. River water and shallow dug well water sources were the most contaminated with ARGs. Almost all samples contained sul1 (94%), and intI1 and tet(A) were detected in 83 and 60% of the samples, respectively. Maximum ARG concentration varied between 4.2 log10 copies/100 ml for mecA and 9.3 log10 copies/100 ml for sul1. Significant positive correlations were found between ARGs (r > 0.5, p < 0.01), except for mecA, qnrS, and vanA. As sul1 and intI1 were detected in almost all samples, the presence of these genes in a given sample may need to be considered as background antibiotic resistance in LMICs. Therefore, monitoring of ARGs, such as β-lactam ARGs, quinolone resistance genes, and vancomycin resistance genes, may provide a better picture of the antibiotic resistance determinants in aquatic environments of LMICs.
Introduction
Antibiotic resistance has been identified as a major health threat of the 21st century by the World Health Organization. According to a recent systematic analysis conducted using data from 204 countries, an estimated 4.95 million deaths were associated with antimicrobial resistance (AMR) in 2019, while 1.27 million deaths were attributed to AMR. South Asia had the highest number of deaths associated with and attributed to AMR (1.39 million and 389,000, respectively) (Murray et al., 2022).
Low- and middle-income countries (LMICs) have to overcome significant challenges to control antibiotic resistance dissemination. Human and animal antibiotic consumption is not regulated effectively in many LMICs, and antibiotics, antibiotic-resistant bacteria (ARB), and antibiotic resistance genes (ARGs) surveillance programs are scarce. Fraction of the antibiotics administered to humans and livestock is excreted in the active form and will end up in wastewater (Kümmerer, 2009). Around 80% of the wastewater generated in the world is discharged into aquatic environments without any treatment (United Nations World Water Assessment Programme, 2017). Moreover, available wastewater treatment infrastructure that can remove these contaminants is limited or not well maintained in LMICs, exacerbating the problem (Laxminarayan et al., 2020; Larsson and Flach, 2021). These wastewater streams containing antibiotics, ARB, and ARGs can contaminate the aquatic environments and contribute to the dissemination of antibiotic resistance (Amarasiri et al., 2020). A recent analysis highlighted the importance of improving basic sanitation in reducing global antibiotic resistance (Graham et al., 2019). Therefore, studies on the antibiotics, ARB, and antibiotic resistance gene (ARG) contamination of aquatic environments in LMICs are needed to be conducted as a first step to understand the extent of the problem and developing mitigation measures.
Earlier studies have highlighted many instances of unnecessary antibiotic prescriptions in Nepal, mostly by unqualified medical professionals (Walson et al., 2001; Acharya and Wilson, 2019). Bacterial strains conferring resistance to commonly available and cheaper antibiotics, such as tetracycline and β-lactam antibiotics, are detected even in rural areas without any healthcare facilities, while the resistant strains to antibiotics that are new, costly, and harder to obtain were found mainly in urban areas with many healthcare facilities (Walson et al., 2001). A recent review on the ARB in the human health sector of Nepal has reported high percentages of resistant strains to antibiotics, including cotrimoxazole, ciprofloxacin, ciprofloxacin, tetracyclin, erythromycin, and nalidixic acid (Acharya and Wilson, 2019). sul1, tet(B), blaCTX–M, blaNDM–1, and qnrS genes were detected in hospital effluents, wastewater treatment plant effluents, and river water, emphasizing the impact of untreated and improperly treated wastewater in contaminating aquatic environments (Thakali et al., 2021). blaTEM, ermF, mecA, and tet(A) genes were detected from the middle and downstream samples of the Bagmati River flowing through the Kathmandu Valley, confirming the anthropogenic contribution (Thakali et al., 2020). To date, there is only one study on the presence of ARGs in drinking water sources utilized in the Kathmandu Valley (Thakali et al., 2022). Therefore, in this study, we evaluated the ARG concentrations in drinking water sources (surface water and groundwater) and environmental waters in the Kathmandu Valley using the droplet digital polymerase chain reaction (ddPCR) method. sul1, tet(A), tet(M), blaKPC, blaOXA, mecA, qnrA, qnrS, and intI1 genes were selected based on previous studies reporting their presence in other aquatic samples (Thakali et al., 2020, 2021). A number of hospitals and animal health-related studies have reported the high prevalence of bacterial strains resistant to cotrimoxazole, vancomycin, and aminoglycosides (Nepal et al., 2012; Acharya and Wilson, 2019; Central Veterinary Laboratory, 2021; Pandey et al., 2021). acrD and dfr13 genes were selected to represent the aminoglycoside and cotrimoxazole resistance genes (Adrian et al., 2000; Grape et al., 2005; Szczepanowski et al., 2009). Quaternary ammonium compounds resistance gene, qacF, which was used in evaluating the anthropogenic contamination of water sources, was also quantified (Szekeres et al., 2018).
Methodology
Study site description
In this study, water samples were collected from the Kathmandu Valley. Kathmandu, the capital city of Nepal, with an area of 664 km2 and a population of 2.51 million (Central Bureau of Statistics, 2012), is situated in the central hilly region of Nepal and mainly consists of three major land covers, namely, built-up, agriculture/open space, and forest/shrub (Uddin et al., 2015; Regmi et al., 2017). The municipal piped water in the valley is supplied by Kathmandu Upatyaka Khanepani Limited (2022) that distributes treated surface and groundwater. However, there is a huge supply deficit of piped water in the valley (Kathmandu Upatyaka Khanepani Limited, 2022). Because of this, people in the valley are compelled to use alternative water sources, such as shallow dug and tube wells, deep tube wells, stone spouts, jar water, and tanker water, for drinking and domestic purposes (Shrestha et al., 2017a). However, previous studies have reported the detection of pathogens, such as Cryptosporidium, Giardia, human adenoviruses, noroviruses (Haramoto et al., 2011), and Acinetobacter spp. (Ghaju Shrestha et al., 2017), as well as human and animal fecal contamination in these water sources (Malla et al., 2015, 2018a,b, 2019). Similarly, river water, which is extensively used for irrigation of vegetable farms in the valley, is highly contaminated with pathogens (Shrestha et al., 2017b), and the interconnection between river water and groundwater of the Kathmandu Valley (Bajracharya et al., 2020) indicates a high possibility of pathogen contamination of groundwater.
Sample collection, bacterial enumeration, and deoxyribonucleic acid extraction
In total, 36 water samples were collected from deep tube wells (n = 6), rivers (n = 6), shallow dug wells (n = 11), shallow tube wells (n = 3), water springs (n = 4), and stone spouts (n = 6) which are used for drinking and other domestic purposes, between February and September 2016 (Supplementary Figure 1). Total coliforms and Escherichia coli were quantified using Colilert reagents (IDEXX Laboratories, Westbrook, CA, United States). Briefly, one pack of Colilert powder was added to 103 ml of a serially diluted water sample, shaken gently until dissolved, and poured into a Quanti-Tray 2000 and sealed. Subsequently, the tray was incubated at 37°C for 24 h. After incubation, yellow-colored large and small wells were observed which were counted as total coliforms, whereas blue-fluorescent large and small wells when observed under UV light were counted as E. coli. Finally, the result was interpreted as a most probable number (MPN) value using an MPN generating software (IDEXX Laboratories), considering the dilution ratios.
For river water samples, 10 ml was filtered using a disposable filter unit (diameter, 47 mm; pore size, 0.22 μm; Nalgene, Tokyo, Japan), while for other sample types, 100 ml was filtered. Deoxyribonucleic acid (DNA) extraction from the membrane filters was done using the CicaGeneus DNA Extraction Kit (Kanto Chemical, Tokyo, Japan) (Malla et al., 2018b; Ghaju Shrestha et al., 2019). Extracted DNA was stored at −25°C until quantification.
Droplet-digital polymerase chain reaction for antibiotic resistance gene quantification
Genes conferring resistance to commonly used antibiotics in this region were selected for the analysis (Thakali et al., 2020, 2021). ddPCR was employed in quantifying the ARGs from the drinking water sources and environmental samples speculating that ARG concentrations in drinking water sources may be low, and quantitative PCR (qPCR) may provide below the detection limit results. Primer pairs used to analyze the ARGs are shown in Supplementary Table 1 (Eurofins Genomics, Tokyo, Japan) (Demarta et al., 1999; Turner et al., 1999; Fang and Hedin, 2003; Guillard et al., 2011; Monteiro et al., 2012; Sandberg et al., 2018). gBlocks (Integrated DNA Technologies, Coralville, IA, United States) were used as positive controls, and their sequences are also shown in Supplementary Table 1. ARG concentrations in the samples were quantified using QX200 AutoDG droplet digital PCR system (Bio-Rad, Hercules, CA, United States). All the reagents were purchased from Bio-Rad, Hercules, CA, United States. The reaction mixture contained 11 μl of 2 × ddPCR EvaGreen Supermix, 0.3 μl each of forward and reverse primers (10 μM concentration), 1.1 μl of DNA template, and 9.3 μl of PCR-grade water. Prepared reaction mixtures were loaded to a ddPCR 96-well plate and sealed with a PCR plate heat seal foil using a PX1 PCR plate sealer. The sealed plate was inserted into the automated droplet generator, and droplets were generated in a new ddPCR 96-well plate. The sample plate was sealed as before, and a PCR was conducted with the following cycling conditions on generated droplets using a C1000 Touch thermal cycler. Enzyme activation at 95°C for 5 min was followed by 40 cycles of denaturation at 95°C for 30 s and annealing at 58°C for 1 min. After that, two signal stabilization steps of 5 min were conducted at 4 and 90°C. After the PCR, samples were allowed to cool at 4°C for 15 min and then transferred to the QX200 droplet reader (Bio-Rad). Data acquisition and analysis were done using QX Manager Software (version 1.1) (Bio-Rad). All the reactions resulted in more than 10,000 droplets in this study. Two positive and three negative control samples were included in each run. The threshold of each analysis was determined manually for each plate based on the positive and negative control sample data (Hughes et al., 2022). Each sample was analyzed in triplicate and considered positive if at least 2 wells were positive.
Data analysis
Absolute ARG concentrations in each sample were expressed as log10 gene copies/100 ml. The relative abundance of ARGs was calculated based on the 16S rRNA concentration of each sample. Correlation analysis between ARGs, E. coli, and total coliforms was conducted using corrplot package in R (Wei and Simko, 2021). Non-metric multidimensional scaling (NMDS) analysis was conducted using vegan and ggord packages to evaluate dissimilarities in ARG concentrations depending on the source water type (Oksanen et al., 2020; Beck, 2021). All the figures, except for the location map, were developed using the ggplot2 package using RStudio (Version 4.1.2) (Wickham, 2016; R Core Team, 2021).
Results
The presence and concentration of total coliforms and E. coli in all samples were confirmed by the Colilert method (Malla et al., 2018b; Ghaju Shrestha et al., 2019). All samples contained total coliforms ranging between 1.21 and 7.76 log10 MPN/100 ml, and a river water sample contained the highest total coliform concentration (Supplementary Figure 2). E. coli was present in all samples with concentrations ranging between −1.00 and 7.38 log10 MPN/100 ml. Similar to total coliforms, a river water sample contained the highest E. coli concentration (Supplementary Figure 2). All samples were positive for the 16S rRNA gene, and a river water sample had the highest concentration (10.73 log10 gene copies/100 ml) (Table 1).
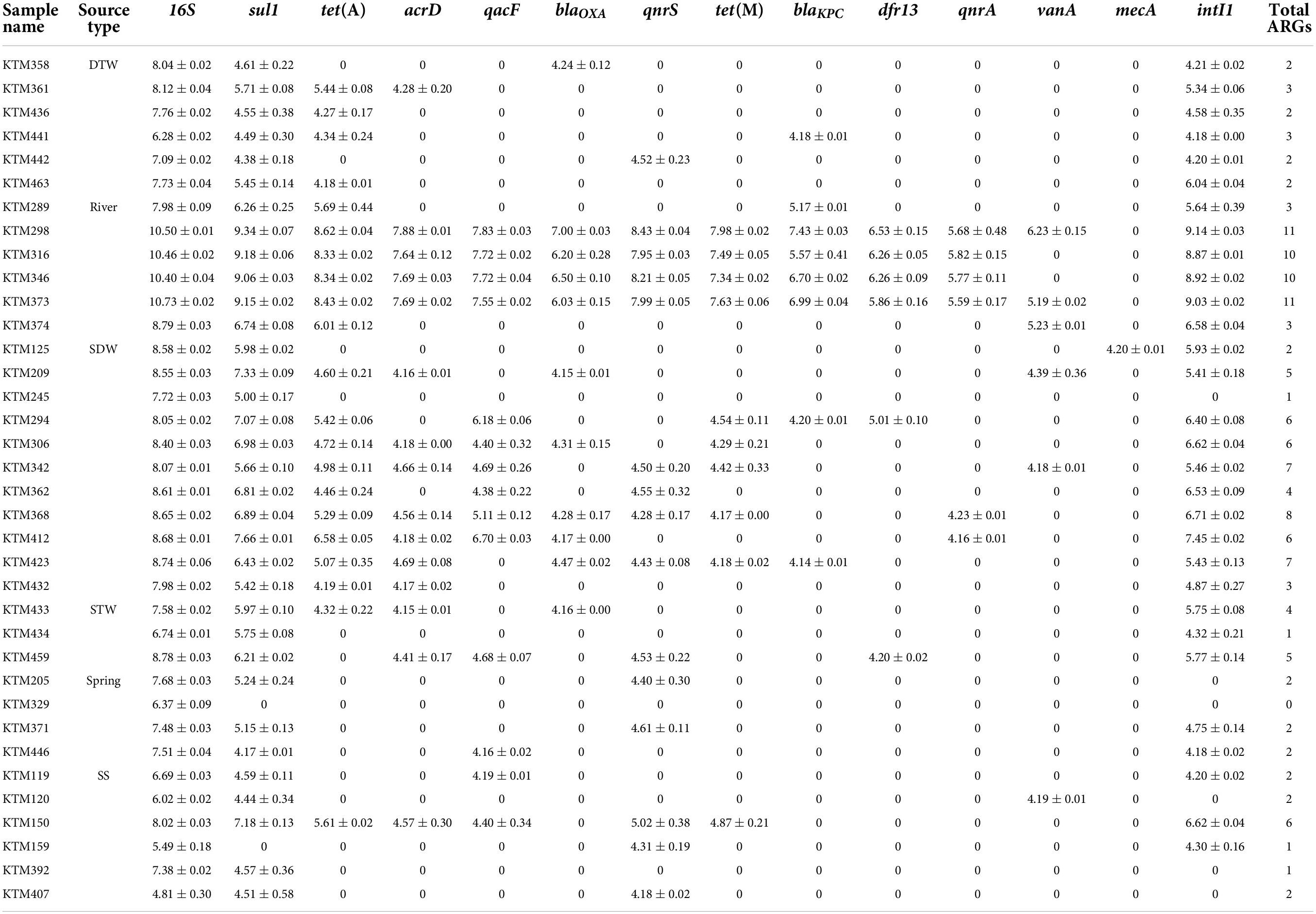
Table 1. Concentration of each antibiotic resistance gene (ARG) and intI1 in different drinking and environmental water samples (log10 copies/100 ml) (mean ± SD).
Among the analyzed ARGs, sul1 was detected in 94% (34/36) of the samples. The other ARG detected in more than 50% of the samples was tet(A) (Table 2). intI1 was detected in 83% (30/36) of the samples. Absolute ARG concentrations were highest in river water samples, followed by shallow dug well and shallow tube well water samples (Figure 1).
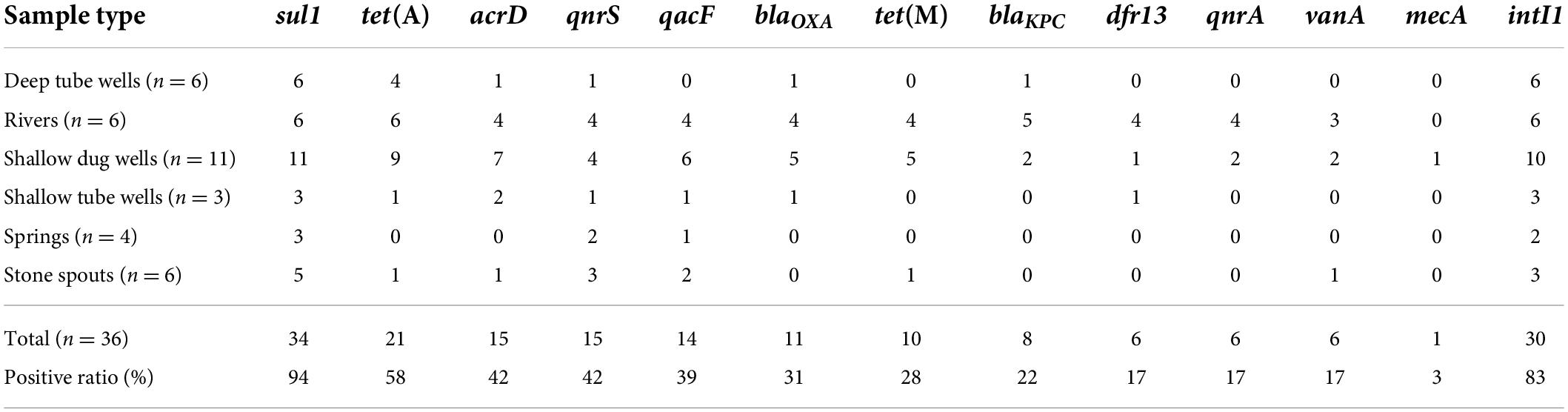
Table 2. Positive ratios of antibiotic resistance genes (ARGs) and intI1 in different drinking and environmental water samples.
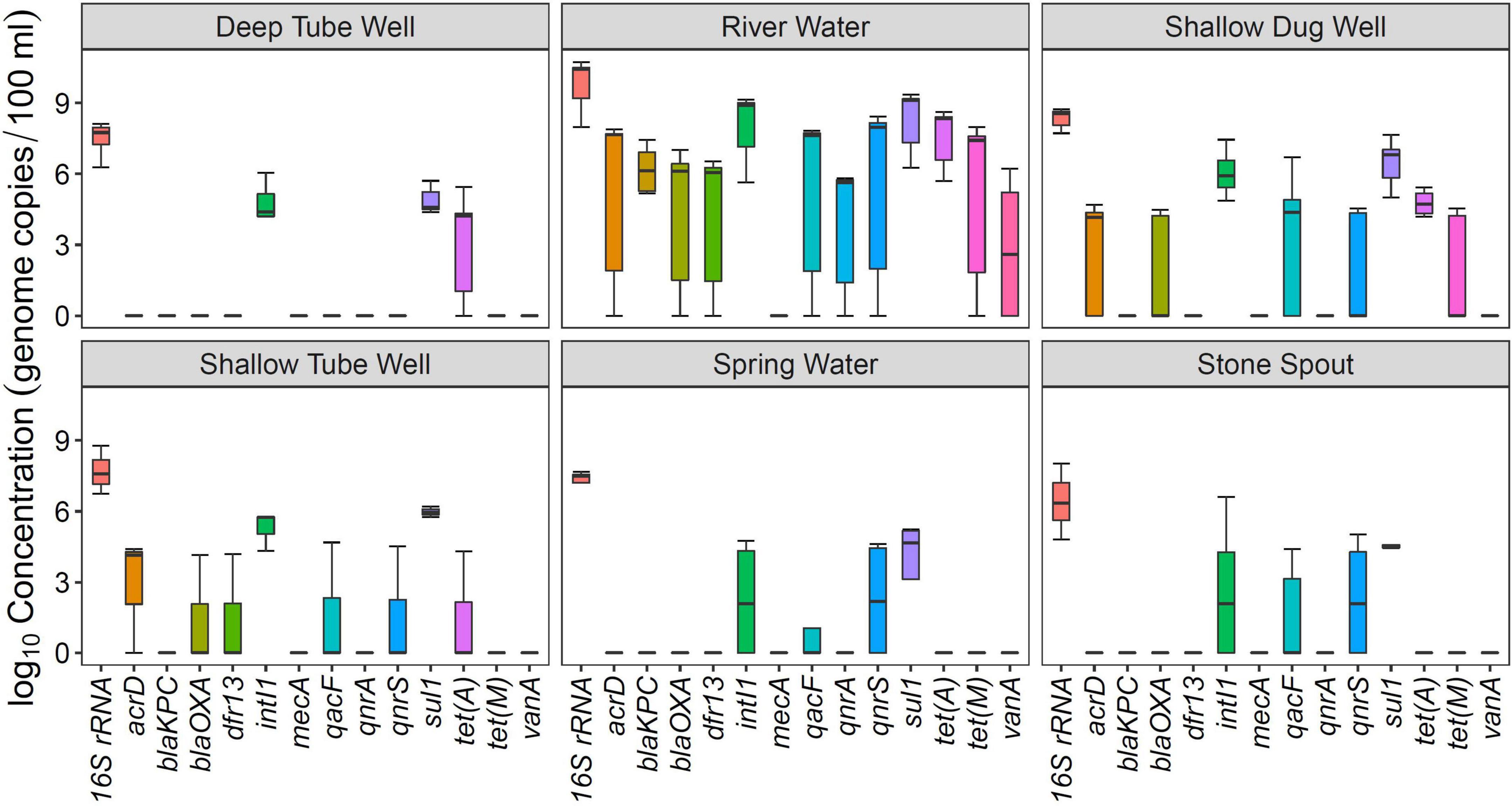
Figure 1. Concentration of antibiotic resistance genes (ARGs) in different drinking and environmental water sources.
All river water samples contained sul1, intI1, and tet(A). Two river water samples were positive for all the tested ARGs, except for the mecA gene, and two other river water samples were positive for all the tested ARGs, except for mecA and vanA genes. Water samples collected at Budhanilkantha, the upstream site of the Dhobikhola River, and from the Godavari River contained only four ARGs where blaKPC and vanA were detected in addition to the common ARGs, respectively. The sample collected from the Bishnumati River at Shovabhagavati Bridge had the highest concentration for all the ARGs, except for the qnrA gene. Absolute concentrations of 16S rRNA gene and other ARGs were usually 1–2 log10 higher in the river water samples containing 10 or 11 ARGs compared with samples containing four ARGs.
sul1 was detected in all shallow dug well samples, while most of the samples were positive for intI1 (10/11) and tet(A) (9/11). One sample contained 8 out of the 12 ARGs quantified in this study (KTM 368). dfr13 was detected in only one sample, and blaKPC, qnrA, and vanA were detected only in two out of 11 samples. Maximum concentrations of sul1 and tet(A) were 7.66 and 6.58 log10 gene copies/100 ml, respectively, while intI1 concentration was 7.45 log10 gene copies/100 ml. Concentrations of other ARGs were at least 2 log10 lower than those of sul1 and tet(A). Samples taken from the same shallow well 5 months apart showed a different ARG prevalence pattern. While the sample collected in February 2016 contained vanA, acrD, and blaOXA in addition to sul1 and tet(A), the sample collected in August 2016 was positive for qacF, qnrA, and tet(M) but not for vanA gene.
sul1 and intI1 were detected in all the shallow tube well water samples. In addition, acrD, blaOXA, dfr13, qacF, and tet(A) were sporadically detected. Concentrations of sul1 and intI1 were between 4.3 log10 and 6.2 log10 gene copies/100 ml. Other ARG concentrations were in the 4 log10 gene copies/100 ml range (Figure 1 and Table 1).
All deep tube well water samples were positive for sul1, tet(A), and intI1. acrD, blaKPC, blaOXA, and qnrS were sporadically detected in some samples, and other genes were not detected. The maximum number of ARGs detected in a deep tube well sample was three (Figure 1 and Table 1). Deep tube well samples had the lowest concentration of sul1 gene among all sample types, which varied between 4.38 and 5.71 log10 gene copies/100 ml. It was 2 to 5 log10 lower than the sul1 concentration in river water samples. intI1 concentration also followed a similar trend (Table 1). Other detected ARGs were present in concentrations between 4.18 and 4.52 log10 gene copies/100 ml.
Compared with river water and shallow dug well water samples, fewer types of ARGs were detected from spring water samples. One spring water sample did not contain any of the measured ARGs, and sul1 was the only positive ARG for the three remaining samples. qacF (1/3) and qnrS (2/3) were the other ARGs detected. intI1 was detected in two samples. sul1 was present at the highest concentration of 5.24 log10 gene copies/100 ml, while other ARGs and intI1 concentrations were between 4 and 5 log10 gene copies/100 ml (Figure 1 and Table 1).
Similar to spring water samples, fewer types of ARGs were detected in stone spout samples. The highest number of ARGs detected in one sample was 6 (KTM150), while the other samples were positive for 1 or 2 ARGs. sul1 was the predominant ARG (5/6), and the highest absolute concentration of sul1 was 7.18 log10 gene copies/100 ml. In addition, acrD, qacF, qnrS, tet(A), tet(M), and vanA were detected in several samples with concentrations ranging between 4.19 and 5.61 log10 gene copies/100 ml. intI1 was detected in 3 samples, and the concentration varied between 4.20 and 6.62 log10 gene copies/100 ml (Figure 1 and Table 1).
In general, the relative abundance of sul1 and intI1 was high for all water source types. The relative abundance of qnrS gene was comparable with sul1 and intI1 in river and stone spout samples (Figure 2). qacF gene has shown higher relative abundance in exposed water sources such as river water, shallow dug wells, and stone spouts. The relative abundance of sul1, intI1, vanA, and qnrS genes in stone spout samples was high since the 16S rRNA concentration was lower than other water sources (Figure 2).
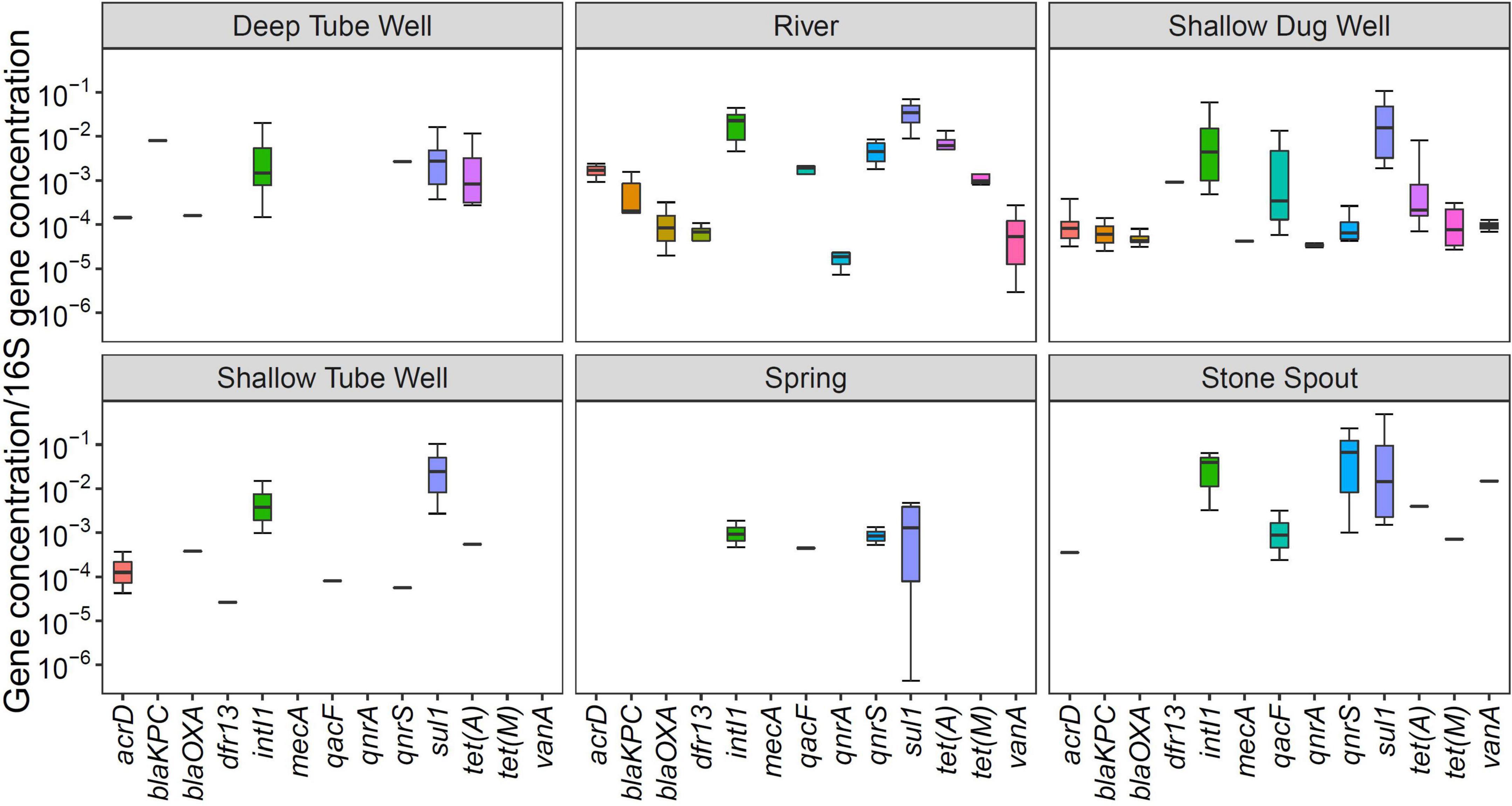
Figure 2. Relative abundance of antibiotic resistance genes (ARGs) in different drinking and environmental water sources.
Correlation analysis of ARGs showed moderate to very high correlations (r > 0.5, p < 0.01) among each other, with total coliforms and E. coli except for vanA, qnrS, and mecA (Figure 3). According to the NMDS analysis, river water samples are ordinated close together as expected, and samples containing a high number of measured ARGs are sub-clustered within the group. Stone spout samples are ordinated far apart confirming the dissimilarities in the ARGs present in each sample (Figure 4).
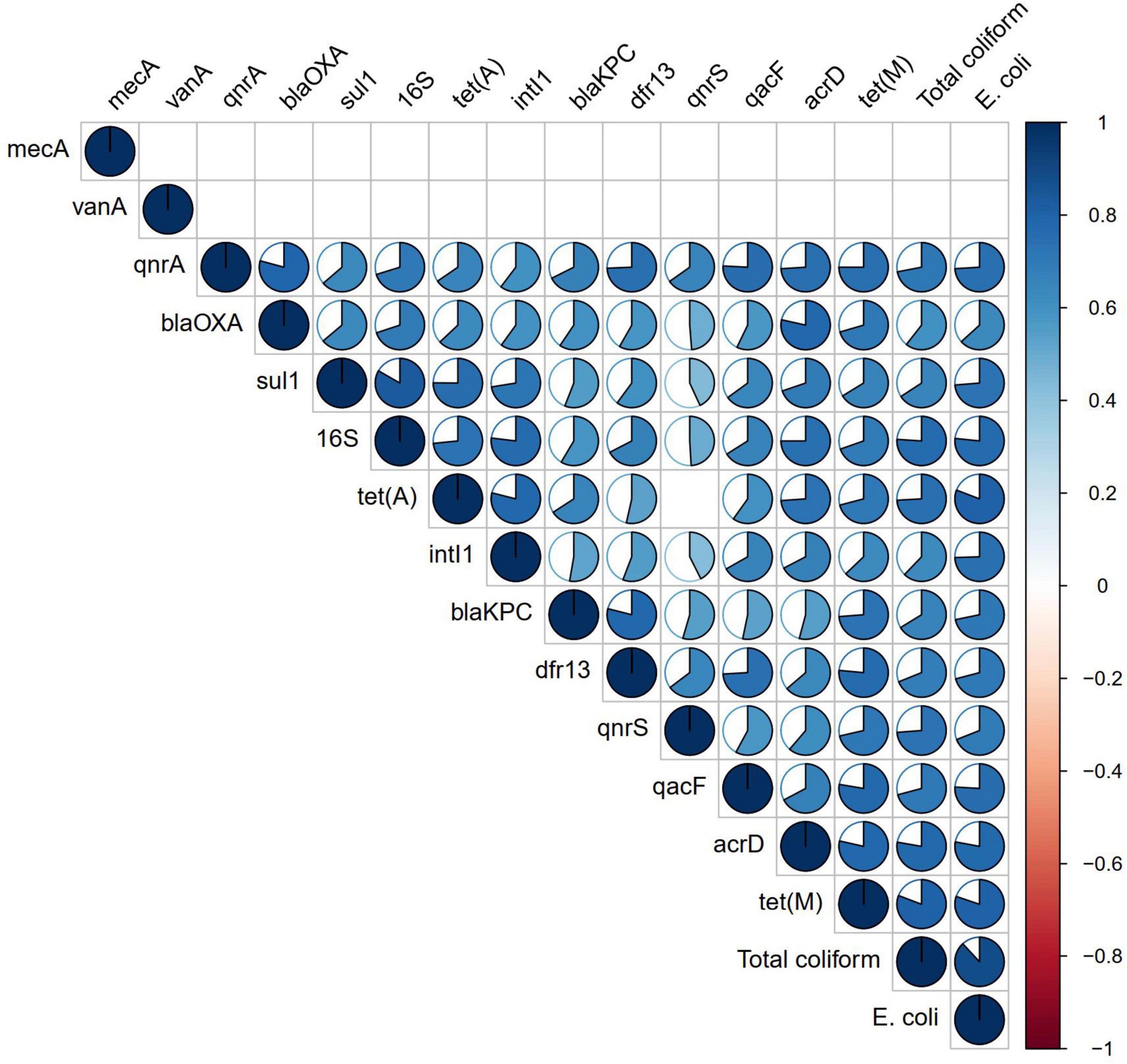
Figure 3. Correlation analysis of antibiotic resistance genes (ARGs) and fecal indicator bacteria. Correlation coefficient is shown in the pie, while non-significant correlations (p > 0.01) are kept blank.
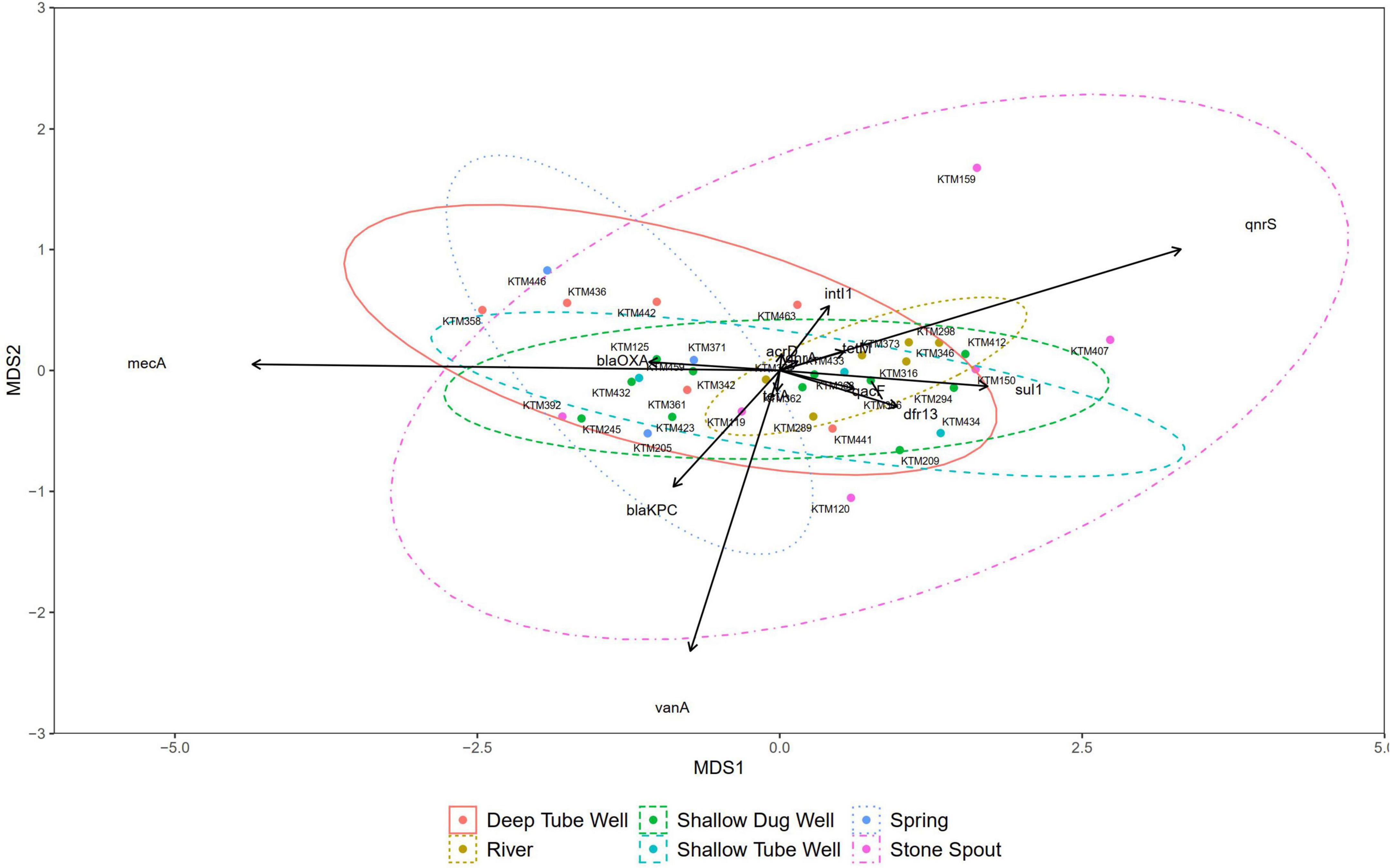
Figure 4. Non-metric multidimensional scaling (NMDS) plot of samples grouped by source type (stress = 0.088).
Discussion
The ARG concentrations in drinking water and environmental water sources collected from the Kathmandu Valley were quantified using ddPCR. Among the surface water and groundwater samples analyzed, at least one ARG was detected in 35 (97%) out of 36 samples. Maximum concentrations of different ARGs varied between 4.2 (mecA) and 9.34 (sul1) log10 gene copies/100 ml.
River water samples contained the highest concentration of all ARGs, except for the mecA gene. A recent study reported similar results where ARG concentrations in the samples from the mid-stream and downstream of the Bagmati River were 8–9 log10 copies/100 ml. dfr13 gene associated with cotrimoxazole resistance and detected in hospital isolates (Acharya and Wilson, 2019) was detected in all four highly contaminated river water samples. In Nepal, 74% of the hospitals do not have wastewater treatment facilities (Joshi et al., 2017). Direct discharge of untreated municipal and hospital wastewaters and improperly treated wastewater to rivers in highly populated areas were the possible reasons for this observation (Acharya and Wilson, 2019; Thakali et al., 2020, 2021). A low level of ARG contamination at KTM289, the upstream site of the Bagmati River, and KTM374, the upstream site of the Godavari River, with low population density corroborate this. Since river water is being extensively used for irrigation purposes, some of these ARGs may end up in fresh produce and possibly pose a human health risk (Amarasiri et al., 2020; Sorinolu et al., 2021).
Spring water and stone spout samples contained the fewest ARGs. The water in the stone spouts flows continuously in underground channels (Tripathi et al., 2018) and is less exposed to contaminants, which may have been reflected as few types of detected ARGs.
In addition to sul1 and tet(A), acrD was the most prevalent ARG in shallow dug wells. Studies on the animal samples, such as milk and poultry carcasses, have shown that bacterial isolates from such samples are resistant to aminoglycosides, such as gentamicin. Moreover, gentamycin is being used to treat mastitis diseases in cattle (Central Veterinary Laboratory, 2021). A number of meat shops, hospitals, and a pharmacy were present in the surroundings (within 200 m) of the shallow wells where 8 ARGs were detected. Contamination of shallow dug wells by wastewater from such establishments and discharging of untreated wastewater and partially treated municipal, industrial, and hospital wastewaters to rivers may have affected shallow groundwater (Mackie et al., 2006; Elliott et al., 2018; Bajracharya et al., 2020; Hubbard et al., 2020).
qacF disinfectant resistant gene was detected in all water source types, except for deep tube well samples. Quaternary ammonium compounds are ubiquitous in soaps and sanitizers. Washing activities conducted in rivers and in the vicinity of shallow wells may have acted as a selection pressure that contributed to the development of antibiotic-resistant bacterial strains (Allen and Canadian Paediatric Society, 2006; Zou et al., 2014).
The results of this study highlight that ARGs are prevalent in surface and groundwater samples that are used for drinking, bathing, and other household activities. A recent critical review highlighted the suitability of sul1, intI1, tet(A), vanA, and blaCTX–M as potential ARG targets to standardize the ARG monitoring in surface water and wastewater (Keenum et al., 2022). In this study, sul1, intI1, tet(A), and vanA were quantified not only in surface water but also in groundwater samples. The results complement the selection of the above genes and further suggest their suitability for monitoring the ARGs in groundwater.
sul1 and intI1 were present in 94 and 83% of samples analyzed in this study, respectively, implying anthropogenic contamination (Pruden et al., 2012; Gillings et al., 2015). Maximum concentrations of those genes were also 1–2 log10 higher than those of other ARGs. It is possible to speculate a similar situation in other LMICs (Devarajan et al., 2016; Rafraf et al., 2016) where antibiotic usage-related regulations are weak (Nadimpalli et al., 2018; Larsson and Flach, 2021). Under such conditions, ARGs such as sul1 and intI1 may be ubiquitous in aquatic environments and therefore may be only used to evaluate background conditions. Moreover, deep tube well water samples were positive only for sul1, tet(A), and intI1. It may suggest that these ARGs were already being present in those water sources for a long time rather than being a recent anthropogenic input. It also strengthens the suggestion of using those genes only as background ARG contamination in LMICs. However, clinically relevant ARGs, such as blaKPC, blaOXA, qnrS, and vanA, which are not frequently detected in environmental settings were sporadically detected in samples from almost all the source water types (Table 2). Clinical intI1 has shown to better correlate with ARGs (Gillings et al., 2015; Zheng et al., 2020). High concentration and relative abundance of disinfectant resistance gene, qacF, in rivers and shallow dug wells may also be representative of the anthropogenic contamination (Szekeres et al., 2018). Therefore, monitoring of such ARGs may provide valuable information on the ARGs contamination status of aquatic environments in LMICs, including Nepal.
The NMDS did not show any dissimilarities between most of the source water types, suggesting the cross-contamination or presence of a common contamination origin. However, a similar gene clustering pattern was not observed in stone spout water samples. Therefore, ARGs detected in different stone spout water samples may reflect the ARGs present in their respective origins. Further studies are necessary to identify the origins of antibiotic resistance in aquatic environments of the Kathmandu Valley.
The highest ARG concentration detected in drinking water source samples varied between 4.18 and 7.66 log10 copies/100 ml for different ARGs. Ding et al. (2020) gavaged extracellular RK2 plasmid containing apramycin resistance gene at around 1011 copies/ml concentration into mice and observed the bacterial transformation occurring in the proximal and distal jejuna and ilea for 2 days. After 2 days, transformants acted as the plasmid donors, and the RK2 plasmid was disseminated by conjugation for another 6 days before reaching a stable concentration (Ding et al., 2020). The same scenario might occur in humans exposed to ARGs in drinking water which poses a human health risk.
The highest ARG concentrations detected in river water samples varied between 5.82 and 9.34 log10 copies/100 ml for different ARGs. Wastewater treatment plant effluents in the Kathmandu Valley were shown to contain ARG concentrations between 108 and 1011 genome copies/L (Thakali et al., 2021). Those discharges may have been a major reason for the high concentrations of ARGs in river water. Long-term irrigation with treated wastewater increased the relative abundance of sul1 and intI1 in the groundwater microbiome (Kampouris et al., 2022) and intI1 concentration in dry soil (Zammit et al., 2020). A similar phenomenon can be expected in the Kathmandu Valley, where river water containing high concentrations of ARGs was used for agriculture. Even though the ARG loads in produce, such as tomatoes and beans, are 0.1–0.01% of the concentrations in dry soil (Cerqueira et al., 2019), it may still pose a human health risk when consuming raw products.
Total coliforms and E. coli are used as indicators for fecal contamination. In this study, total coliforms and E. coli were detected at the highest concentrations of 5.72 and 4.51 log10 MPN/100 ml in drinking water sources, respectively, which is 2–3 log10 lower than those in river water. Uprety et al. (2020) collected 2,822 water samples from 37 districts out of 77 in Nepal including source water, communal tap water, reservoir water, and household stored water and analyzed for the presence of E. coli. The mean E. coli concentration was 70 ± 550 CFU/100 ml. Assuming 8% of the E. coli is pathogenic in 2 L of daily water consumption, 4 × 10–2 disability adjusted life year loss (DALY Loss) per person per year (pppy) was calculated (Uprety et al., 2020). Calculated DALY loss pppy exceeds 10–4 DALYpppy which was considered an adequate margin of public-health safety related to waterborne diarrheal diseases (Mara, 2011). Even though MPN-based E. coli calculations are shown to be higher than the culture-based estimations (Prats et al., 2008), it is possible to expect that the concentration of E. coli detected in our study may also exceed the acceptable DALYpppy loss for waterborne diseases. If horizontal gene transfer occurs and the antibiotic-resistant E. coli concentrations were increased, it may significantly increase the DALYpppy loss.
In the ddPCR quantification method used in this study, the limit of quantification was around 1.2 genome copies/reaction, which is comparable to a previous study (Cavé et al., 2016). A previous study by our group which quantified ARGs in drinking water samples from Kathmandu Valley using the qPCR technique reported the limit of quantification as 5 copies/reaction which may get influenced by the amplification efficiency and the inhibitory substances (Thakali et al., 2022). Therefore, ddPCR can be suggested as a sensitive and specific quantitative analysis method usable in routine monitoring studies for ARGs in aquatic environments even when the monitoring targets are available in very low concentrations or inhibitory substances are present (Ishii, 2020).
In this study, grab sampling was conducted, and the ARG concentrations in each sample were quantified. One limitation of the grab sampling is the inability to evaluate the variations of ARG concentrations within each day. It might affect the ARG load in river water, depending on the wastewater treatment plant and hospital wastewater discharge schedules. It may not directly affect the ARG concentrations in groundwater sources unless there are anthropogenic activities in the vicinity of the unimproved shallow dug wells. As the ARG concentrations in river systems have shown seasonal variations (Harnisz et al., 2020), further studies are necessary to clarify the phenomenon in the river water of Nepal.
This study highlights the ubiquitous presence of ARGs in drinking and environmental water sources of the Kathmandu Valley. ARGs in aquatic environments have the potential to pose a human health risk if they are being transferred to human pathogens (Wright, 2010; Tomova et al., 2015). As the improvement of basic sanitation can reduce antibiotic resistance dissemination (Graham et al., 2019), interventions associated with sanitation may contribute to minimizing the ARG loads in aquatic environments. Affordable wastewater treatment systems and stringent regulations may also play a role in reducing antibiotic resistance dissemination and potential human health risks.
Data availability statement
The original contributions presented in this study are included in the article/Supplementary material, further inquiries can be directed to the corresponding author.
Author contributions
EH, JS, and KS initiated this project. MA, TF, EH, and KS contributed to the design of the experiment. BM processed the samples. TT, BM, and MA conducted the experiments and did data processing and analysis. MA, BM, TF, JS, EH, and KS wrote and edited the manuscript. All authors approved the manuscript for publication.
Funding
This study was supported by the Science and Technology Research Partnership for Sustainable Development (SATREPS) program of the Japan International Cooperation Agency (JICA) and Japan Science and Technology Agency (JST) entitled “Hydro-microbiological Approach for Water Security in Kathmandu Valley, Nepal”, and Accelerating Social Implementation for SDGs Achievement (aXis) (Grant number JPMJAS2005), the Japan Society for the Promotion of Science (JSPS) through Fund for the Promotion of Joint International Research [Fostering Joint International Research (B)] (Grant number JP18KK0297), and Grant-in-Aid for Scientific Research (B) (Grant number 20H04357).
Acknowledgments
We thank Rajani Ghaju Shrestha, Sarmila Tandukar, and Dinesh Bhandari for their support in processing the samples.
Conflict of interest
The authors declare that the research was conducted in the absence of any commercial or financial relationships that could be construed as a potential conflict of interest.
Publisher’s note
All claims expressed in this article are solely those of the authors and do not necessarily represent those of their affiliated organizations, or those of the publisher, the editors and the reviewers. Any product that may be evaluated in this article, or claim that may be made by its manufacturer, is not guaranteed or endorsed by the publisher.
Supplementary material
The Supplementary Material for this article can be found online at: https://www.frontiersin.org/articles/10.3389/fmicb.2022.894014/full#supplementary-material
References
Acharya, K. P., and Wilson, R. T. (2019). Antimicrobial resistance in Nepal. Front. Med. 6:105. doi: 10.3389/fmed.2019.00105
Adrian, P. V., Thomson, C. J., Klugman, K. P., and Amyes, S. G. B. (2000). New gene cassettes for trimethoprim resistance, dfr13, and streptomycin-spectinomycin resistance, aadA4, inserted on a class 1 integron. Antimicrob. Agents Chemother. 44, 355–361. doi: 10.1128/AAC.44.2.355-361.2000
Allen, U., Canadian Paediatric Society (2006). Antimicrobial products in the home: The evolving problem of antibiotic resistance. Paediatr. Child Health 11, 169–173. doi: 10.1093/pch/11.3.169
Amarasiri, M., Sano, D., and Suzuki, S. (2020). Understanding human health risks caused by antibiotic resistant bacteria (ARB) and antibiotic resistance genes (ARG) in water environments: Current knowledge and questions to be answered. Crit. Rev. Environ. Sci. Technol. 50, 2016–2059. doi: 10.1080/10643389.2019.1692611
Bajracharya, R., Nakamura, T., Ghimire, S., Man Shakya, B., and Kazi Tamrakar, N. (2020). Identifying groundwater and river water interconnections using hydrochemistry, stable isotopes, and statistical methods in Hanumante River, Kathmandu Valley, Central Nepal. Water 12:1524. doi: 10.3390/w12061524
Beck, M. W. (2021). ggord: Ordination Plots with ggplot2. Available online at: https://fawda123.github.io/ggord (accessed March 1, 2022).
Cavé, L., Brothier, E., Abrouk, D., Bouda, P. S., Hien, E., and Nazaret, S. (2016). Efficiency and sensitivity of the digital droplet PCR for the quantification of antibiotic resistance genes in soils and organic residues. Appl. Microbiol. Biotechnol. 100, 10597–10608. doi: 10.1007/s00253-016-7950-5
Central Bureau of Statistics (2012). National Population and Housing Census 2011 (National Report). Kathmandu: Central Bureau of Statistics.
Central Veterinary Laboratory (2021). Annual Technical Report Fiscal Year 2019/2020. Kathmandu: Central Veterinary Laboratory.
Cerqueira, F., Matamoros, V., Bayona, J. M., Berendonk, T. U., Elsinga, G., Hornstra, L. M., et al. (2019). Antibiotic resistance gene distribution in agricultural fields and crops. A soil-to-food analysis. Environ. Res. 177, 18–26. doi: 10.1016/j.envres.2019.108608
Demarta, A., Tonolla, M., Caminada, A. P., Ruggeri, N., and Peduzzi, R. (1999). Signature region within the 16S rDNA sequences of Aeromonas popoffii. FEMS Microbiol. Lett. 172, 239–246. doi: 10.1016/S0378-1097(99)00029-4
Devarajan, N., Laffite, A., Mulaji, C. K., Otamonga, J. P., Mpiana, P. T., Mubedi, J. I., et al. (2016). Occurrence of antibiotic resistance genes and bacterial markers in a tropical river receiving hospital and urban wastewaters. PLoS One 11:e0149211. doi: 10.1371/journal.pone.0149211
Ding, C., Yang, D., Ma, J., Jin, M., Shen, Z., Shi, D., et al. (2020). Effects of free antibiotic resistance genes in the environment on intestinal microecology of mice. Ecotoxicol. Environ. Saf. 204:111119. doi: 10.1016/j.ecoenv.2020.111119
Elliott, S. M., Erickson, M. L., Krall, A. L., and Adams, B. A. (2018). Concentrations of pharmaceuticals and other micropollutants in groundwater downgradient from large on-site wastewater discharges. PLoS One 13:e0206004. doi: 10.1371/journal.pone.0206004
Fang, H., and Hedin, G. (2003). Rapid screening and identification of Methicillin-Resistant Staphylococcus aureus from clinical samples by selective-broth and Real-Time PCR assay. J. Clin. Microbiol. 41, 2894–2899. doi: 10.1128/JCM.41.7.2894-2899.2003
Ghaju Shrestha, R., Tanaka, Y., Malla, B., Bhandari, D., Tandukar, S., Inoue, D., et al. (2017). Next-generation sequencing identification of pathogenic bacterial genes and their relationship with fecal indicator bacteria in different water sources in the Kathmandu Valley. Nepal. Sci. Total Environ. 601–602, 278–284. doi: 10.1016/j.scitotenv.2017.05.105
Ghaju Shrestha, R., Tanaka, Y., Sherchand, J. B., and Haramoto, E. (2019). Identification of 16s rRNA and virulence-associated genes of Arcobacter in water samples in the Kathmandu valley, Nepal. Pathogens 8, 1–9. doi: 10.3390/pathogens8030110
Gillings, M. R., Gaze, W. H., Pruden, A., Smalla, K., Tiedje, J. M., and Zhu, Y. G. (2015). Using the class 1 integron-integrase gene as a proxy for anthropogenic pollution. ISME J. 9, 1269–1279. doi: 10.1038/ismej.2014.226
Graham, D., Giesen, M., and Bunce, J. (2019). Strategic approach for prioritising local and regional sanitation interventions for reducing global antibiotic resistance. Water 11:27. doi: 10.3390/w11010027
Grape, M., Farra, A., Kronvall, G., and Sundström, L. (2005). Integrons and gene cassettes in clinical isolates of co-trimoxazole-resistant Gram-negative bacteria. Clin. Microbiol. Infect. 11, 185–192. doi: 10.1111/j.1469-0691.2004.01059.x
Guillard, T., Moret, H., Brasme, L., Carlier, A., Vernet-Garnier, V., Cambau, E., et al. (2011). Rapid detection of qnr and qepA plasmid-mediated quinolone resistance genes using real-time PCR. Diagn. Microbiol. Infect. Dis. 70, 253–259. doi: 10.1016/j.diagmicrobio.2011.01.004
Haramoto, E., Yamada, K., and Nishida, K. (2011). Prevalence of protozoa, viruses, coliphages and indicator bacteria in groundwater and river water in the Kathmandu Valley, Nepal. Trans. R. Soc. Trop. Med. Hyg. 105, 711–716. doi: 10.1016/j.trstmh.2011.08.004
Harnisz, M., Kiedrzyńska, E., Kiedrzyński, M., Korzeniewska, E., Czatzkowska, M., Koniuszewska, I., et al. (2020). The impact of WWTP size and sampling season on the prevalence of antibiotic resistance genes in wastewater and the river system. Sci. Total Environ. 741:140466. doi: 10.1016/j.scitotenv.2020.140466
Hubbard, L. E., Givens, C. E., Griffin, D. W., Iwanowicz, L. R., Meyer, M. T., and Kolpin, D. W. (2020). Poultry litter as potential source of pathogens and other contaminants in groundwater and surface water proximal to large-scale confined poultry feeding operations. Sci. Total Environ. 735:139459. doi: 10.1016/j.scitotenv.2020.139459
Hughes, B., Duong, D., White, B. J., Wigginton, K. R., Chan, E. M. G., Wolfe, M. K., et al. (2022). Respiratory Syncytial Virus (RSV) RNA in wastewater settled solids reflects RSV clinical positivity rates. Environ. Sci. Technol. Lett. 9, 173–178. doi: 10.1021/acs.estlett.1c00963
Ishii, S. (2020). Quantification of antibiotic resistance genes for environmental monitoring: Current methods and future directions. Curr. Opin. Environ. Sci. Heal. 16, 47–53. doi: 10.1016/j.coesh.2020.02.004
Joshi, H. D., Acharya, T., Ayer, R., Dhakal, P., Karki, K. B., and Dhimal, M. (2017). Health care waste management practice in health care institutions of Nepal. J. Nepal Health Res. Counc. 15, 7–11. doi: 10.3126/jnhrc.v15i1.18006
Kampouris, I. D., Alygizakis, N., Klümper, U., Agrawal, S., Lackner, S., Cacace, D., et al. (2022). Elevated levels of antibiotic resistance in groundwater during treated wastewater irrigation associated with infiltration and accumulation of antibiotic residues. J. Hazard. Mater. 423:127155. doi: 10.1016/j.jhazmat.2021.127155
Kathmandu Upatyaka Khanepani Limited (2022). 14th annual report. Kathmandu: Kathmandu Upatyaka Khanepani Limited.
Keenum, I., Liguori, K., Calarco, J., Davis, B. C., Milligan, E., Harwood, V. J., et al. (2022). A framework for standardized qPCR-targets and protocols for quantifying antibiotic resistance in surface water, recycled water and wastewater. Crit. Rev. Environ. Sci. Technol. 48, 1–25. doi: 10.1080/10643389.2021.2024739
Kümmerer, K. (2009). Antibiotics in the aquatic environment–a review–part I. Chemosphere 75, 417–434. doi: 10.1016/j.chemosphere.2008.11.086
Larsson, D. G. J., and Flach, C.-F. (2021). Antibiotic resistance in the environment. Nat. Rev. Microbiol. 20, 257–269. doi: 10.1038/s41579-021-00649-x
Laxminarayan, R., Van Boeckel, T., Frost, I., Kariuki, S., Khan, E. A., Limmathurotsakul, D., et al. (2020). The lancet infectious diseases commission on antimicrobial resistance: 6 years later. Lancet Infect. Dis. 20, e51–e60. doi: 10.1016/S1473-3099(20)30003-7
Mackie, R. I., Koike, S., Krapac, I., Chee-Sanford, J., Maxwell, S., and Aminov, R. I. (2006). Tetracycline residues and tetracycline resistance genes in groundwater impacted by swine production facilities. Anim. Biotechnol. 17, 157–176. doi: 10.1080/10495390600956953
Malla, B., Ghaju Shrestha, R., Bhandari, D., Tandukar, S., Shrestha, S., Yoshinaga, H., et al. (2015). Detection of Cryptosporidium, Giardia, fecal indicator bacteria, and total bacteria in commercial jar water in the Kathmandu Valley, Nepal. J. Inst. Med. 37, 10–15.
Malla, B., Ghaju Shrestha, R., Tandukar, S., Bhandari, D., Inoue, D., Sei, K., et al. (2018a). Validation of host-specific Bacteroidales quantitative PCR assays and their application to microbial source tracking of drinking water sources in the Kathmandu Valley, Nepal. J. Appl. Microbiol. 125, 609–619. doi: 10.1111/jam.13884
Malla, B., Shrestha, R. G., Tandukar, S., Bhandari, D., Inoue, D., Sei, K., et al. (2018b). Identification of human and animal fecal contamination in drinking water sources in the Kathmandu Valley, Nepal, using host-associated Bacteroidales Quantitative PCR Assays. Water (Switzerland) 10, 1–16. doi: 10.3390/w10121796
Malla, B., Ghaju Shrestha, R., Tandukar, S., Bhandari, D., Thakali, O., Sherchand, J. B., et al. (2019). Detection of pathogenic viruses, pathogen indicators, and fecal-source markers within tanker water and their sources in the Kathmandu Valley, Nepal. Pathogens 8:81. doi: 10.3390/pathogens8020081
Mara, D. (2011). Water-and wastewater-related disease and infection risks: What is an appropriate value for the maximum tolerable additional burden of disease? J. Water Health 9, 217–224. doi: 10.2166/wh.2010.109
Monteiro, J., Widen, R. H., Pignatari, A. C. C., Kubasek, C., and Silbert, S. (2012). Rapid detection of carbapenemase genes by multiplex real-time PCR. J. Antimicrob. Chemother. 67, 906–909. doi: 10.1093/jac/dkr563
Murray, C. J., Ikuta, K. S., Sharara, F., Swetschinski, L., Robles Aguilar, G., Gray, A., et al. (2022). Global burden of bacterial antimicrobial resistance in 2019: A systematic analysis. Lancet 399, 629–655. doi: 10.1016/S0140-6736(21)02724-0
Nadimpalli, M., Delarocque-Astagneau, E., Love, D. C., Price, L. B., Huynh, B. T., Collard, J. M., et al. (2018). Combating global antibiotic resistance: Emerging one health concerns in lower-and middle-income countries. Clin. Infect. Dis. 66, 963–969. doi: 10.1093/cid/cix879
Nepal, H., Khanal, B., Acharya, A., Gyawali, N., Jha, P., and Paudel, R. (2012). High-level gentamicin resistance and vancomycin resistance in clinical isolates of enterococci in a tertiary care hospital in eastern Nepal. J. Nepal Med. Coll. 14, 60–63.
Oksanen, J., Blanchet, F. G., Friendly, M., Kindt, R., Legendre, P., Mcglinn, D., et al. (2020). vegan: Community Ecology Package. version 2.5-7. Available online at: https://cran.r-project.org/package=vegan (accessed March 1, 2022).
Pandey, R., Mishra, S. K., and Shrestha, A. (2021). Characterisation of ESKAPE pathogens with special reference to multidrug resistance and biofilm production in a Nepalese hospital. Infect. Drug Resist. 14, 2201–2212. doi: 10.2147/IDR.S306688
Prats, J., Garcia-Armisen, T., Larrea, J., and Servais, P. (2008). Comparison of culture-based methods to enumerate Escherichia coli in tropical and temperate freshwaters. Lett. Appl. Microbiol. 46, 243–248. doi: 10.1111/j.1472-765X.2007.02292.x
Pruden, A., Arabi, M., and Storteboom, H. N. (2012). Correlation between upstream human activities and riverine antibiotic resistance genes. Environ. Sci. Technol. 46, 11541–11549. doi: 10.1021/es302657r
R Core Team (2021). R: A language and environment for statistical computing. Vienna: R foundation for statistical computing.
Rafraf, I. D., Lekunberri, I., Sànchez-Melsió, A., Aouni, M., Borrego, C. M., and Balcázar, J. L. (2016). Abundance of antibiotic resistance genes in five municipal wastewater treatment plants in the Monastir Governorate, Tunisia. Environ. Pollut. 219, 353–358. doi: 10.1016/j.envpol.2016.10.062
Regmi, R. K., Mishra, B. K., Masago, Y., Luo, P., Toyozumi-Kojima, A., and Jalilov, S. M. (2017). Applying a water quality index model to assess the water quality of the major rivers in the Kathmandu Valley, Nepal. Environ. Monit. Assess. 189:382. doi: 10.1007/s10661-017-6090-4
Sandberg, K. D., Ishii, S., and LaPara, T. M. (2018). A microfluidic quantitative polymerase chain reaction method for the simultaneous analysis of dozens of antibiotic resistance and heavy metal resistance genes. Environ. Sci. Technol. Lett. 5, 20–25. doi: 10.1021/acs.estlett.7b00552
Shrestha, S., Aihara, Y., Bhattarai, A. P., Bista, N., Rajbhandari, S., Kondo, N., et al. (2017a). Dynamics of domesticwater consumption in the Urban area of the kathmandu valley: Situation analysis pre and post 2015 Gorkha Earthquake. Water (Switzerland) 9, 1–17. doi: 10.3390/w9030222
Shrestha, S., Haramoto, E., and Shindo, J. (2017b). Assessing the infection risk of enteropathogens from consumption of raw vegetables washed with contaminated water in Kathmandu Valley. Nepal. J. Appl. Microbiol. 123, 1321–1334. doi: 10.1111/jam.13573
Sorinolu, A. J., Tyagi, N., Kumar, A., and Munir, M. (2021). Antibiotic resistance development and human health risks during wastewater reuse and biosolids application in agriculture. Chemosphere 265:129032. doi: 10.1016/j.chemosphere.2020.129032
Szczepanowski, R., Linke, B., Krahn, I., Gartemann, K. H., Gützkow, T., Eichler, W., et al. (2009). Detection of 140 clinically relevant antibiotic-resistance genes in the plasmid metagenome of wastewater treatment plant bacteria showing reduced susceptibility to selected antibiotics. Microbiology 155, 2306–2319. doi: 10.1099/mic.0.028233-0
Szekeres, E., Chiriac, C. M., Baricz, A., Szõke-Nagy, T., Lung, I., Soran, M.-L., et al. (2018). Investigating antibiotics, antibiotic resistance genes, and microbial contaminants in groundwater in relation to the proximity of urban areas. Environ. Pollut. 236, 734–744. doi: 10.1016/j.envpol.2018.01.107
Thakali, O., Malla, B., Raya, S., Sthapit, N., Sherchan, S. P., Furukawa, T., et al. (2022). Prevalence of antibiotic resistance genes in drinking water of the Kathmandu Valley, Nepal. Environ. Challenges 7:100527. doi: 10.1016/j.envc.2022.100527
Thakali, O., Malla, B., Tandukar, S., Sthapit, N., Raya, S., Furukawa, T., et al. (2021). Release of antibiotic-resistance genes from hospitals and a wastewater treatment plant in the kathmandu valley, Nepal. Water (Switzerland) 13, 2733. doi: 10.3390/w13192733
Thakali, O., Tandukar, S., Brooks, J. P., Sherchan, S. P., Sherchand, J. B., and Haramoto, E. (2020). The occurrence of antibiotic resistance genes in an Urban River in Nepal. Water (Switzerland) 12, 450. doi: 10.3390/w12020450
Tomova, A., Ivanova, L., Buschmann, A. H., Rioseco, M. L., Kalsi, R. K., Godfrey, H. P., et al. (2015). Antimicrobial resistance genes in marine bacteria and human uropathogenic Escherichia coli from a region of intensive aquaculture. Environ. Microbiol. Rep. 7, 803–809. doi: 10.1111/1758-2229.12327
Tripathi, M., Hughey, K. F. D., and Rennie, H. G. (2018). The state of traditional stone spouts in relation to their use and management in Kathmandu Valley, Nepal. Conserv. Manag. Archaeol. Sites 20, 319–339. doi: 10.1080/13505033.2018.1559421
Turner, S., Pryer, K. M., Miao, V. P. W., and Palmer, J. D. (1999). Investigating deep phylogenetic relationships among cyanobacteria and plastids by small subunit rRNA sequence analysis. J. Eukaryot. Microbiol. 46, 327–338. doi: 10.1111/j.1550-7408.1999.tb04612.x
Uddin, K., Shrestha, H. L., Murthy, M. S. R., Bajracharya, B., Shrestha, B., Gilani, H., et al. (2015). Development of 2010 national land cover database for the Nepal. J. Environ. Manage. 148, 82–90. doi: 10.1016/j.jenvman.2014.07.047
United Nations World Water Assessment Programme (2017). Wastewater: The untapped resource. Paris: United Nations Educational, Scientific and Cultural Organization.
Uprety, S., Dangol, B., Nakarmi, P., Dhakal, I., Sherchan, S. P., Shisler, J. L., et al. (2020). Assessment of microbial risks by characterization of Escherichia coli presence to analyze the public health risks from poor water quality in Nepal. Int. J. Hyg. Environ. Health 226:113484. doi: 10.1016/j.ijheh.2020.113484
Walson, J. L., Marshall, B., Pokhrel, B. M., Kafle, K. K., and Levy, S. B. (2001). Carriage of antibiotic-resistant fecal bacteria in Nepal reflects proximity to Kathmandu. J. Infect. Dis. 184, 1163–1169. doi: 10.1086/323647
Wei, T., and Simko, V. (2021). R package “corrplot”: Visualization of a Correlation Matrix (Version 0.92). Available online at: https://github.com/taiyun/corrplot (accessed March 1, 2022).
Wright, G. D. (2010). Antibiotic resistance in the environment: A link to the clinic? Curr. Opin. Microbiol. 13, 589–594. doi: 10.1016/j.mib.2010.08.005
Zammit, I., Marano, R. B. M., Marano, R. B. M., Vaiano, V., Cytryn, E., and Rizzo, L. (2020). Changes in antibiotic resistance gene levels in soil after irrigation with treated wastewater: A comparison between heterogeneous photocatalysis and chlorination. Environ. Sci. Technol. 54, 7677–7686. doi: 10.1021/acs.est.0c01565
Zheng, W., Huyan, J., Tian, Z., Zhang, Y., and Wen, X. (2020). Clinical class 1 integron-integrase gene – a promising indicator to monitor the abundance and elimination of antibiotic resistance genes in an urban wastewater treatment plant. Environ. Int. 135:105372. doi: 10.1016/j.envint.2019.105372
Keywords: antibiotic resistance genes, aquatic environments, ddPCR, drinking water, Kathmandu Valley
Citation: Amarasiri M, Takezawa T, Malla B, Furukawa T, Sherchand JB, Haramoto E and Sei K (2022) Prevalence of antibiotic resistance genes in drinking and environmental water sources of the Kathmandu Valley, Nepal. Front. Microbiol. 13:894014. doi: 10.3389/fmicb.2022.894014
Received: 11 March 2022; Accepted: 25 July 2022;
Published: 22 August 2022.
Edited by:
Amy Pruden, Virginia Tech, United StatesReviewed by:
Luther King Abia Akebe, University of KwaZulu-Natal, South AfricaRaj Nathaniel, Nicholls State University, United States
Copyright © 2022 Amarasiri, Takezawa, Malla, Furukawa, Sherchand, Haramoto and Sei. This is an open-access article distributed under the terms of the Creative Commons Attribution License (CC BY). The use, distribution or reproduction in other forums is permitted, provided the original author(s) and the copyright owner(s) are credited and that the original publication in this journal is cited, in accordance with accepted academic practice. No use, distribution or reproduction is permitted which does not comply with these terms.
*Correspondence: Mohan Amarasiri, mohan@kitasato-u.ac.jp