- 1Multimodal Laboratory Medicine Research Area, Unit of Human Microbiome, Bambino Gesù Children’s Hospital, Scientific Institute for Research, Hospitalization and Healthcare, Rome, Italy
- 2Child and Adolescent Neuropsychiatry Unit, Department of Neuroscience, Bambino Gesù Children’s Hospital, Scientific Institute for Research, Hospitalization and Healthcare, Rome, Italy
- 3GenomeUp, Rome, Italy
- 4Institute for Systems Analysis and Computer Science “Antonio Ruberti,” National Research Council, Rome, Italy
- 5Department of Diagnostics and Laboratory Medicine, Unit of Microbiology and Diagnostic Immunology, Unit of Microbiomics, Bambino Gesù Children’s Hospital, Scientific Institute for Research, Hospitalization and Healthcare, Rome, Italy
- 6Department of Diagnostic and Laboratory Medicine, Unit of Microbiology and Diagnostic Immunology, Bambino Gesù Children’s Hospital, Scientific Institute for Research, Hospitalization and Healthcare, Rome, Italy
- 7Department of Computer, Control and Management Engineering, Sapienza University of Rome, Rome, Italy
- 8CEMAD Digestive Disease Center, Fondazione Policlinico Universitario “A. Gemelli” Scientific Institute for Research, Hospitalization and Healthcare, Università Cattolica del Sacro Cuore, Rome, Italy
- 9Department of Diagnostics and Laboratory Medicine, Unit of Microbiology and Diagnostic Immunology, Unit of Microbiomics, and Multimodal Laboratory Medicine Research Area, Unit of Human Microbiome, Bambino Gesù Children’s Hospital, Scientific Institute for Research, Hospitalization and Healthcare, Rome, Italy
Autism spectrum disorders (ASDs) is a multifactorial neurodevelopmental disorder. The communication between the gastrointestinal (GI) tract and the central nervous system seems driven by gut microbiota (GM). Herein, we provide GM profiling, considering GI functional symptoms, neurological impairment, and dietary habits. Forty-one and 35 fecal samples collected from ASD and neurotypical children (CTRLs), respectively, (age range, 3–15 years) were analyzed by 16S targeted-metagenomics (the V3–V4 region) and inflammation and permeability markers (i.e., sIgA, zonulin lysozyme), and then correlated with subjects’ metadata. Our ASD cohort was characterized as follows: 30/41 (73%) with GI functional symptoms; 24/41 (58%) picky eaters (PEs), with one or more dietary needs, including 10/41 (24%) with food selectivity (FS); 36/41 (88%) presenting high and medium autism severity symptoms (HMASSs). Among the cohort with GI symptoms, 28/30 (93%) showed HMASSs, 17/30 (57%) were picky eaters and only 8/30 (27%) with food selectivity. The remaining 11/41 (27%) ASDs without GI symptoms that were characterized by HMASS for 8/11 (72%) and 7/11 (63%) were picky eaters. GM ecology was investigated for the overall ASD cohort versus CTRLs; ASDs with GI and without GI, respectively, versus CTRLs; ASD with GI versus ASD without GI; ASDs with HMASS versus low ASSs; PEs versus no-PEs; and FS versus absence of FS. In particular, the GM of ASDs, compared to CTRLs, was characterized by the increase of Proteobacteria, Bacteroidetes, Rikenellaceae, Pasteurellaceae, Klebsiella, Bacteroides, Roseburia, Lactobacillus, Prevotella, Sutterella, Staphylococcus, and Haemophilus. Moreover, Sutterella, Roseburia and Fusobacterium were associated to ASD with GI symptoms compared to CTRLs. Interestingly, ASD with GI symptoms showed higher value of zonulin and lower levels of lysozyme, which were also characterized by differentially expressed predicted functional pathways. Multiple machine learning models classified correctly 80% overall ASDs, compared with CTRLs, based on Bacteroides, Lactobacillus, Prevotella, Staphylococcus, Sutterella, and Haemophilus features. In conclusion, in our patient cohort, regardless of the evaluation of many factors potentially modulating the GM profile, the major phenotypic determinant affecting the GM was represented by GI hallmarks and patients’ age.
Introduction
Autism spectrum disorder (ASD) is a multifactorial neurodevelopmental disorder, which is characterized by deficits in social interactions, communication, and by the presence of restrictive and repetitive behaviors, interests, and activities (American Psychiatric Association [APA], 2013). In United States, across all 11 Autism and Developmental Disabilities Monitoring (ADDM) Network sites, monitored by the Center for Disease Control and Prevention (CDCP), ASD prevalence was 18.5 per 1,000 (one in 54) children aged 8 years (Maenner et al., 2020), and European studies have reported prevalence of 1 and 2% in the childhood population (Carta et al., 2020). Differences in prevalence depend on methodological approaches, demographic factors, and geographical area (Lyall et al., 2017). However, ASD is an etiologically heterogeneous disorder involving multiple factors, such as genetic (Jonsson et al., 2014; Leblond et al., 2014; Wilkinson et al., 2015) and environmental factors (Herbert, 2010; Raz et al., 2015), including pre- and/or post-natal factors (de Theije et al., 2011).
Among ASD comorbidities, gastrointestinal (GI) symptoms are of particular interest, given their reported prevalence (Buie et al., 2010; Coury et al., 2012) and correlation with ASD severity (Adams et al., 2011; Chernikova et al., 2021). GI symptoms include abdominal pain, constipation, diarrhea, bloating, and gastroesophageal reflux (GERD) (Horvath and Perman, 2002; Valicenti-McDermott et al., 2006; Nikolov et al., 2009). Among neurological symptoms, irritability (Bresnahan et al., 2015), anxiety and affective disorders (Valicenti-McDermott et al., 2006; Fulceri et al., 2016), dysregulation and externalizing problems (Horvath and Perman, 2002), rigid/compulsive behaviors (Peters et al., 2014; Marler et al., 2017), increased sensory sensitivity (Mazurek et al., 2013), and sleep problems (Horvath and Perman, 2002; Maenner et al., 2012) have been reported in patients with ASD in presence/absence of GI symptoms (Adams et al., 2011; Wang et al., 2011; Gorrindo et al., 2012; Chaidez et al., 2014).
In the last decade, many studies have highlighted the role of the gut microbiota (GM) in neurodevelopmental disorders (Hsiao et al., 2013; Borre et al., 2014; Kelly et al., 2017), particularly in ASD (Parracho et al., 2005; Finegold et al., 2010; Adams et al., 2011; Williams et al., 2011, 2012; Kang et al., 2013). Indeed, the GM is known to influence social-behavior and brain physiology through a diverse set of pathways (Flannery et al., 2020; Buffington et al., 2021), including immune activation (Furness et al., 1999; Kruglov et al., 2013), production of microbial peptides, metabolites, and various neuromodulators and neurotransmitters (Sherwin et al., 2019). In fact, the gut is linked to different brain functions acting on emotional and cognitive brain regions, such as prefrontal cortex, limbic system, and hypothalamus (Davies et al., 2021). Therefore, the communication by cross-talk interaction between the central nervous system (CNS) and the GI tract, called “gut-brain axis,” plays a key role in neurological disease pathophysiology and seems apparently driven by GM ecology and function (Collins et al., 2012; Ristori et al., 2019).
Several studies have evidenced that the gut microbial composition of the ASD enterophenotype is characterized by an increase in harmful microbes and a decrease in beneficial ones (Adams et al., 2011; Wang et al., 2011; Liu S. et al., 2019). Particularly, few studies based on targeted-metagenomics (Liu S. et al., 2019; Ma et al., 2019; Berding and Donovan, 2020) have highlighted specific overrepresented ASD-related microbial signatures, such as Ruminococcus, Sutterella, Enterococcus, Prevotella, and Fecalibacterium prausnitzii (Kang et al., 2013; De Angelis et al., 2015; Tomova et al., 2015; Fung et al., 2017).
However, GM also plays a critical role in maintaining the intestinal barrier integrity, protecting from bacterial toxins’ passage into the bloodstream, a phenomenon related to peripheral inflammation and induction of behavioral alterations and damage of a blood-brain barrier, as demonstrated in animal models (Stolp et al., 2011; Liu F. et al., 2019).
Thus, changes in intestinal permeability due to tight junctions’ modulation may reflect low GM functionality, as well as low antibacterial defense. Zonulin is a well-known biomarker of permeability, usually related to chronic diseases, such as diabetes, celiac disease, inflammatory bowel disease, or obesity (Fasano, 2012; Sturgeon and Fasano, 2016), but also to ASD-associated comorbidities (Esnafoglu et al., 2017; Fasano and Hill, 2017). Secretory IgAs (sIgAs) represent the first immune barrier of defense in the gut protecting the intestinal epithelium from pathogens and enteric toxins (Mantis et al., 2011), hence mediating the innate immune defense mechanism at the level of the gut mucosa, as also reported in patients with ASD (Zhou et al., 2018). Fecal lysozyme is an alkaline glycosidase secreted by granulocytes, macrophages, Paneth cells, Brunner’s glands, and normal colonic crypt cells, playing antimicrobial action by hydrolyzation of specific glycosidic bonds of mucopolysaccharides of Gram-positive bacteria wall.
Elevated levels of fecal lysozyme have been identified in colonic IBD when compared to healthy controls (Klass and Neale, 1978; Veer et al., 1998) but also in patients with ASD (Adams et al., 2011).
Herein, we considered an ecological and inferred functional description of the GM based on GI and severity symptoms, dietary habits, and fecal host biomarkers, underlying GI hallmarks and age as the main driving features of the GM profile in our ASD cohort.
Materials and Methods
Patient Characteristics and Sample Collection
Forty-one patients with ASD aged 3–15 years (average age, 6.5 years, SD ± 3.42), of whom 36 males and 5 females, with a male gender frequency of 88%, who were enrolled, were recruited at the Bambino Gesù Children’s Hospital (Rome, Italy) and University Hospital Agostino Gemelli (Rome, Italy). Thirty-five aged-matched neurotypical children (average age, 8 years, SD ± 3.62; 21 males and 14 females) were enrolled during an epidemiological survey carried out at the Human Microbiome Unit of Bambino Gesù Children’s Hospital in Rome (BBMRI Human Micro-biome Biobank, OPBG) to generate a reference digital biobank of neurotypical healthy subjects (controls, CTRLs).
Autism spectrum disorder inclusion criteria were: age, 3–18 years; diagnosis of ASD according to criteria of the Diagnostic and Statistical Manual of Mental Disorders, fifth edition (DSM-5); confirmation by the Autism Diagnostic Observation Schedule, second edition (ADOS-2) and, when available, by the Autism Diagnostic Interview—Revised (ADI-R). The characterization of ASD core symptoms, cognitive level, and behavioral problems was conducted by trained clinical psychologists and psychiatrists. All the patients who did not meet the inclusion criteria and did not have a diagnosis of ASD were excluded.
The participants’ metadata included clinical, anamnestic, and nutritional data [i.e., presence of food selectivity (FS) as: elimination diet, gluten free or casein free], probiotics’ supplementation and antibiotics’ administration (Supplementary Table 1 and Figure 1). Among clinical parameters (i.e., weight, height) for body mass index (BMI) calculation, the percentiles of body mass index (pBMI) were evaluated according to Center for Disease Control and Prevention (CDCP) growth charts for children and teens ages 2–19 years1 (Supplementary Table 1, sheet General Characteristics and Nutritional info and Figure 1). Fecal samples were collected at the reference node of the Human Microbiome Biobanking and Biomolecular Resources Research Infrastructure (BBMRI) of the OPBG Human Microbiome Unit and stored at −80°C until processing.
Gastrointestinal Assessment
A questionnaire based on Rome IV classification (Drossman and Hasler, 2016) was exploited to evaluate GI symptoms, such as the presence of abdominal pain, functional diarrhea, constipation, and vomiting disorders. Also, presence or absence of GERD (Wasilewska and Klukowski, 2015) and colonic eosinophilia (Katzka, 2014) from intestinal biopsies was considered when available. Hence, the patients with ASD were stratified into subgroups based on the presence or absence of one or more GI disorders to identify possible relationships with GM differences based on ASD GI-related enterophenotypes (Supplementary Table 1 sheet GI features and Figure 1). Finally, the two groups, namely, with and without GI symptoms, were considered for differential GM ecology description.
Neuropsychological Assessment
The cognitive level (intelligent quotient, IQ; and developmental quotient, DQ) was assessed according to age, language level, compliance, and timing of IQ evaluation by WISC-IV (Lang et al., 2015), non-verbal IQ by Leiter-3 (Roid et al., 2013) or general quotient (GQ) by Griffiths Mental Development Scales Extended, revised for ages 2–8 (Sannio Fancello et al., 2007).
Autism symptoms were evaluated using ADOS-2 (Lord et al., 2012), a semi-structured direct assessment of communication, social interaction, play or imaginative use of materials for individuals with a suspected diagnosis of ASD, according to the language level and ranging from non-verbal to verbal fluency. The ADOS-2 was administered and scored by licensed clinicians with a demonstrated clinical capacity of its usage. For some of the patients, the diagnosis was also confirmed by ADI-R (Lord et al., 1994), a parent semi-structured interview. To assess the severity of ASD symptomatology, total raw scores were calibrated and transformed into calibrated severity score (CSS) (Gotham et al., 2009), with a score ranging from 1 to 10. Based on the CSS, the patients were divided into three levels of severity according to ADOS scores criteria (American Psychiatric Association [APA], 2013). Behavioral and psychopathological problems were assessed by the Achenbach System of Empirically Based Assessment (ASEBA) questionnaire (Rescorla, 2005), specifically by using the 1.5–5 years’ Child Behavior Checklist (CBCL) and the 6–18 years’ CBCL questionnaires, reported by parents. The parents evaluated their children’s behavior during the preceding 6 months on a 3-point Likert scale for each item (0 = not true; 1 = somewhat or sometimes true; 2 = very true or often true). Neuropsychologists obtained scores from Internalizing, Externalizing, and Total Problems Scales. For 1.5–5 CBCL, the internalizing domain was based on four syndrome scales: emotionally reactive, anxious and depressed, somatic complaining, and withdrawn. According to the cut-off thresholds of Achenbach et al. (2003), Internalizing, Externalizing, and Total Problems’ scales were classified according to symptoms’ t-scores: clinically relevant (≥65), borderline (60–64), and non-clinical (<60) (Supplementary Table 1, sheet Neuropsycological features and Figure 1).
Ethics Statement
The study was approved by the OPBG Ethics Committees for both patients with ASD and healthy subjects’ recruitment, respectively, 1404_OPBG_2017 and 1113_OPBG_2016 protocols. The project was conducted in accordance with the Principles of Good Clinical Practice and the Declaration of Helsinki. Written informed consent was obtained from all the participants.
Fecal Markers of Intestinal Inflammation and Permeability: Secretory IgA, Lysozyme, and Zonulin
The quantitative determination of fecal markers was performed on stools collected from 41 patients with ASD and 35 CTRLs by ELISA assays. The optical density was determined by a TECAN’s Infinite F50 at 450 nm. Markers’ levels were statistically evaluated using Student’s t-test. A p-value ≤ 0.05 was considered statistically significant.
Secretory IgA
Fecal sIgAs were determined using a RIDASCREEN® sIgA kit according to the manufacturer’s instructions. One hundred mg of stools were weighed and suspended in an extraction buffer, and 100 μL of supernatant processed on the ELISA plate, along with calibrators, negative, low and high positive controls, all of them in duplicate.
Zonulin
Zonulin was measured by the Human Zonulin ELISA kit (Bioassay Technology Laboratory, China). Feces were suspended into PBS 1X (DPBS 1X Gibco®). Fifty μL of standards, 40 μL of the stool sample, 10 μL of an anti-zonulin antibody, and 50 μL of streptavidin-HRP were added to the plate incubated for 60 min at 37°C.
Lysozyme
Fecal lysozyme was assessed using the Lysozyme sandwich ELISA Kit (Immundi-agnostik AG, Cat. No. K 6900) based on two selected antibodies according to the manufacturer’s instructions. Fifteen mg of feces were suspended in 1.5 mL of a sample extraction buffer (IDK extract®) and pipetted on a plate.
Bacterial DNA Extraction From Stools and 16S rRNA Targeted-Metagenomics
DNA from 41 and 35 fecal samples of ASD and CTRLs subjects, respectively, was extracted using a QIAmp Fast DNA Stool mini kit (Qiagen, Hilden, Germany) according to the manufacturer’s instructions. Amplification of the variable region V3–V4 from the bacterial 16S rRNA gene (∼460 bp) was carried out using the primers 16S_F 5′-(TCG Qaim GCA GCG TCA GAT GTG TAT AAG AGA CAG CCT ACG GGN GGC WGC AG)-3′ and 16S_R 5′-(GTC TCG TGG GCT CGG AGA TGT GTA TAA GAG ACA GGA CTA CHV GGG TAT CTA ATC C)-3′ according to MiSeq rRNA Amplicon Sequencing protocol (Illumina, San Diego, CA, United States). The PCR reaction was set up using the 2 × KAPA Hifi HotStart ready Mix kit (KAPA Biosystems Inc., Wilmington, MA, United States). DNA amplicons were cleaned up by CleanNGS kit beads (CleanNA, Coenecoop 75, PH Wad-dinxveen, Netherlands). A second amplification step was performed to obtain a unique combination of Illumina Nextera XT dual indices for each sample. The final libraries were cleaned up using CleanNGS kit beads, quantified by Quant-iT PicoGreen dsDNA Assay Kit (Thermo Fisher Scientific, Waltham, MA, United States), and normalized to 4 nM. To generate paired-end 250- × −2 bp-length reads, normalized libraries were pooled together and run on the Illumina MiSeq platform according to the manufacturer’s specifications.
Data Processing and Quality Control
The obtained raw reads were analyzed using Quantitative Insights into Microbial Ecology software (QIIME, 1.9.1) (Caporaso et al., 2010) to perform demultiplexing, quality score checking, low length exclusion, and denoising. Chimeric reads were detected and discarded using USEARCH algorithms (Edgar, 2010). Sequences were grouped into operational taxonomic units (OTUs) by clustering at a threshold of 97% pairwise identity using UCLUST (Edgar, 2010) and submitting representative sequences to PyNAST for sequence alignment (Caporaso et al., 2010). The Greengenes database (v 13.8) was used for OTU matching.
All sequencing data associated with this study were uploaded to the NCBI bioproject database: PRJNA754695.2
16S rRNA-Targeted Metagenomics: Statistical Analysis
Unless otherwise stated, all ecological statistical analyses were performed using Python version 3.7. Statistical significance was determined at p-value ≤ 0.05 and corrected for multiple hypothesis testing by the false discovery rate (FDR) method Benjamini-Hochberg procedure (Benjamini and Hochberg, 1995). The adjusted p-value were reported. The OTU table was normalized by cumulative-sum scaling (CSS) (Paulson et al., 2013). OTUs present in less than.01% of the total sequence count, as well as unassigned taxa, were removed prior to applying statistical analysis. Genus-level comparisons were performed on a reduced matrix of 72 over 461 total OTUs. The criterion for inclusion of variables OTUs reflected their presence in at least 75% of the total sample set.
Alpha diversity statistics was calculated in QIIME (Caporaso et al., 2010) using Shannon, Chao1, Ob-served Species, PD_Whole_Tree, and Goods Coverage diversity indices, and the p-value for group comparisons was determined by ANOVA.
Principal coordinate analyses (PCoA) plots were constructed to illustrate the beta diversity of samples based on phylogenetically informed weighted and unweighted Unifrac (Lozupone and Knight, 2005) and Bray–Curtis, Euclidean distance matrices.
To test the association between the covariates and beta diversity measures, permutational analysis of variance (PERMANOVA) was used (Chen et al., 2012) as a distance-based ANOVA method based on permutation (9999 permutations, “Adonis” function in the R “Vegan” package). Linear discriminant analysis effect size (LEfSe) (Segata et al., 2011) was used to determine OTUs characterizing ASDs or CTRLs population. The non-parametric Mann-Whitney U-test and the Wilcoxon signed-rank sum test were used to compare the two independent CTRL and ASD groups. As an initial analysis, some statistics were performed on OTU tables (taxonomic levels 2–6). The interquartile range (IQR), the percentage of zeros (%0), the fold-change (FC), and the t-test with relative p-value (corrected by the FDR method) were calculated. Applying these filters: IQR ≠ 0,% 0 < 75%, and FC ≠ 0, the variables that were totally unnecessary for classification were discarded.
After applying the filters, further statistics was performed on the remaining variables: for each patient, the OTUs count with abundances > 0 at levels L2–L6, the mean and standard deviation of the abundances, and the Z-test with relative p-value between the patients and CTRLs were computed. The Z-score heat maps of OTUs distribution were performed by considering only those with a p-value < 0.05. By using Pearson’s correlation coefficient as a metric, clustering of both patients and OTUs was assessed.
To gain more insights into targeted-metagenomics-inferred functions of ASD and CTRLs microbiota, the Phylogenetic Investigation of Communities by Reconstruction of Unobserved States (PICRUSt) v1.1.4 tool was applied (Langille et al., 2013). To get KEGG description, the online database https://www.genome.jp/kegg/ko.html was used (Kanehisa et al., 2016).
In addition, to assess the influence of confounding factors for all comparisons, the Mann-Whitney U-test was applied. Particularly, for the ASD versus CTRLs, comparison confounders, such as gender (male/female) and age (<5 or ≥5 years old), were considered. The confounders taken into account, only for patients, were: age, gender, dietary habits (i.e., no picky eaters/picky eaters), autism severity (low/high-medium symptoms), FS (absence/presence) and GI symptoms (absence/presence). To verify the statistically significant differences between confounders the threshold of the p-value for all tests was set at ≤ 0.05.
Correlation Analysis on 16S rRNA-Targeted Metagenomics and Clinical Features
To correlate clinical variables and OTUs, Pearson’s correlation coefficient between all variable pairs, with relative p-value, was calculated and represented via a correlation heat map. Also, for the OTUs, the correlation analysis was performed on all variables and on features passing the filter of p-value ≤ 0.05.
Machine Learning Models
Multiple machine learning models were trained for the classification task ASD versus CTRLs at each taxonomy level and for the corresponding KOs. The training consisted of a 10-fold cross-validation with a train-test split of 70–30%. To evaluate the model, global and single class accuracies were considered. To extract the feature importance, a permutation performance of 1,000 repetitions was followed. Models tested were: Logistic Regression, SGD Classifier, Logistic Regression CV, Hist Gradient Boosting Classifier, Random Forest Classifier, Extra Trees Classifier, Gradient Boosting Classifier, Bagging Classifier, Ada Boost Classifier, XGB Classifier, XGBRF Classifier, MLP Classifier, Linear SVC, SVC, Gaussian NB, Decision Tree Classifier, Quadratic Discriminant Analysis, K Neighbors Classifier, and Gaussian Process Classifier.
The entire experimental workflow is reported in Figure 1.
Results
Subject Characteristics and Clinical Features
Forty-one patients with ASD were vaginally delivered for the 37% (15/41), while the remaining 63% (26/41) were born by cesarean birth. In addition, only 51% (21/41) ASDs were breastfed for over 3 months. The mean values for weight (average, 23 kg ± 10.7), height (average, 116 cm ± 14), and BMI (average, 16.5 kg/m2 ± 3.17) were within the normal ranges (Supplementary Table 1, sheet General Characteristics and Figure 1). Indeed, the mean BMI of 16.5 kg/m2, assigned to the 75th percentile, classified the ASD children as normal weight (Table 1).
The GI symptoms were assessed by using the questionnaire based on Rome IV criteria (Drossman and Hasler, 2016) and specific gastroenterologists’ observations. Based on functional GI disturbances (i.e., constipation, abdominal distension, diarrhea, abdominal pain, GERD, and colic eosinophilia), 73% (30/41) patients with ASD referred the presence of one or more GI symptoms; in detail, 36.6% (15/41) reported only one symptom, 24.4% (10/41) the simultaneous presence of two, 7.3% (3/41) three, and 2.4% four (1/41) and five (1/41) symptoms, respectively. Consequently, 26.8% (11/41) reported the absence of GI symptoms (Supplementary Table 1, Sheet GI symptoms and Figure 1).
Neuropsychological features are reported in Supplementary Table 1 and Figure 1 (Sheet Neuropsychological features). The scores obtained from CBCL Internalizing (CBCL_INT), Externalizing (CBCL_EXT), and Total (CBCL_TOT) Scales evidenced that, in general, a large part of the subjects presented clinical symptoms in CBCL_INT and CBCL_TOT scores, while the large part did not show clinical signs associated with CBCL_EXT scale (Supplementary Table 1, Sheet Neuropsychological features and Figure 1). Particularly, for autism symptoms’ severity, the ASD subjects showed medium for 46.3% (19/41), high for 41.5% (17/41), and low severity for 12.2% (5/41). Regarding CBCL indices (for three patients, the information was missing), those with CBCL_INT reported clinical symptoms for 60.5% (23/38), risk of behavior problems for 18.4% (7/38), and no clinical symptoms for 21.1% (8/38); based on patients with CBCL_EXT, 13.2% (5/38) showed clinical symptoms, 18.4% (7/38) risk of behavior problems, and 68.4% (26/38) no clinical symptoms; lastly, for those with CBCL_TOT, 50% (19/38) indicated clinical symptoms, 23.7% (9/38) risk of behavior problems, and 26.3% (10/38) no clinical symptoms. Remarkably, among the 30 patients with GI symptoms, 28/30 (93%) were also characterized by high (16/30) and medium (12/30) autism symptoms’ severity, respectively, with only 2/30 (7%) with low symptoms.
In addition, the intelligence measures reported as IQ/DQ evidenced that a wide part of the patients with ASD showed cognitive impairment/developmental delay, epilepsy, and drugs exposition (Supplementary Table 1, Sheet Neuropsychological features and Figure 1). In particular, for IQ/DQ measures performed on only 33/41 patients, 36.4% (12/33) were without cognitive impairment/development delay and 63.6% (21/33) reported cognitive impairment/developmental delay.
Finally, 85.4% (35/41) and 92.7% (38/41) of the patients with ASD referred to no drugs’ consumption or epilepsy, respectively (Supplementary Table 1, Sheet Neuropsychological features and Figure 1). The remaining 6/41 patients were administrated with drugs, such as Topiramate, Depakin, Abilify (antipsychotic drug, 3rd generation), Risperdal (antipsychotic drug, 2nd generation) (Supplementary Table 1, Sheet Neuropsychological features and Figure 1). Moreover, collected information on dietary needs revealed that 24% (10/41) of ASD referred to FS, 24% (10/41) followed by gluten- and 32% (13/41) casein-free diet. 17% (7/41) were exposed to probiotics’ and 24% (10/41) to antibiotics’ administration (Supplementary Table 1, Sheet Nutritional habits and probiotics/antibiotic administration and Figure 1).
Inflammation and Intestinal Permeability Markers
Levels of sIgA, lysozyme, and zonulin (μg/g), measured in stools of ASD and CTRLs subjects, are reported in Figure 2. The sIgA levels were comparable in the two groups; the lysozyme was higher for the CTRLs subjects, while zonulin appeared lower for the patients with ASD. No statistically significant differences were observed between ASD versus CTRLs (p-value ≥ 0.05). However, stratifying the patients with ASD into two subgroups based on GI symptoms presence/absence, remarkable differences were identified for both lysozyme and zonulin markers, showing in the ASD with GI symptoms a decreased median value for lysozyme (799.86 ± 672.91) (Figure 2B2) and an increased median value for zonulin (20.28 ± 5.21) (Figure 2C2), regardless of absence of statistically significant (p-value ≥ 0.05). Conversely, the sIgA levels were comparable in the two ASD subgroups (Figures 2A1–C1,A2–C2). Moreover, to exclude the influence of other factors, the comparisons of ASD with GI symptoms versus CTRLs and ASD with high/moderate autism symptoms versus CTRLs were performed, but any comparison resulted in statistically significant (p-value ≥ 0.05) (Supplementary Figure 2).
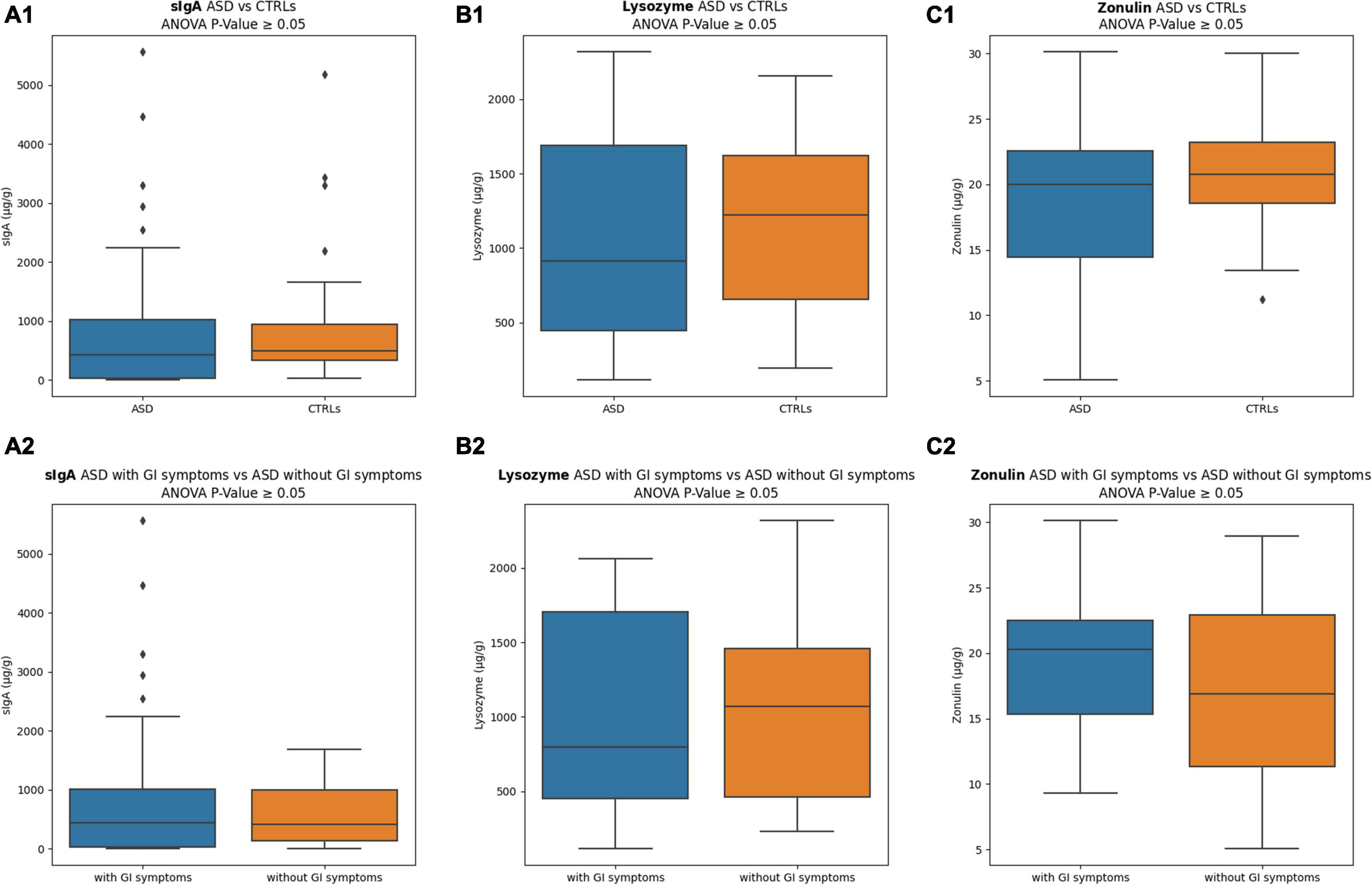
Figure 2. Inflammation and intestinal permeability markers. (A1) sIgA comparison between ASD versus CTRLs; (A2) sIgA comparison between ASD with GI symptoms versus ASD without GI symptoms; (B1) lysozyme comparison between ASD versus CTRLs; (B2) lysozyme comparison between ASD with GI symptoms versus ASD without GI symptoms; (C1) zonulin comparison between ASD versus CTRLs; (C2) zonulin comparison between ASD with GI symptoms versus ASD without GI symptoms. The interquartile range is represented by the box, and the line in the box is the median. The whiskers highest and lowest data points are reported, while the dots represent the outliers.
Gut Microbiota Ecology
After data filtering, a total of 9,525,477 sequence reads of 16S rRNA gene amplicons were obtained with an average of 70,040 reads/sample and an average length of 487 bp (calculated after primer removal). Resulting OTUs were detected by sequence-database matching (Supplementary Table 2).
The GM composition of ASD and CTRLs groups was analyzed by ecological analysis as α- and β-diversity. Statistically significant differences in the α-diversity were found in all the comparisons, except for Good Coverage and Shannon index, showing lower values of diversity for patients with ASD (Supplementary Figure 3).
The ecological analysis assessed by β-diversity algorithms provided statistically significant (p-value ≤ 0.05) differences between patients with ASD and CTRLs (Figure 3).
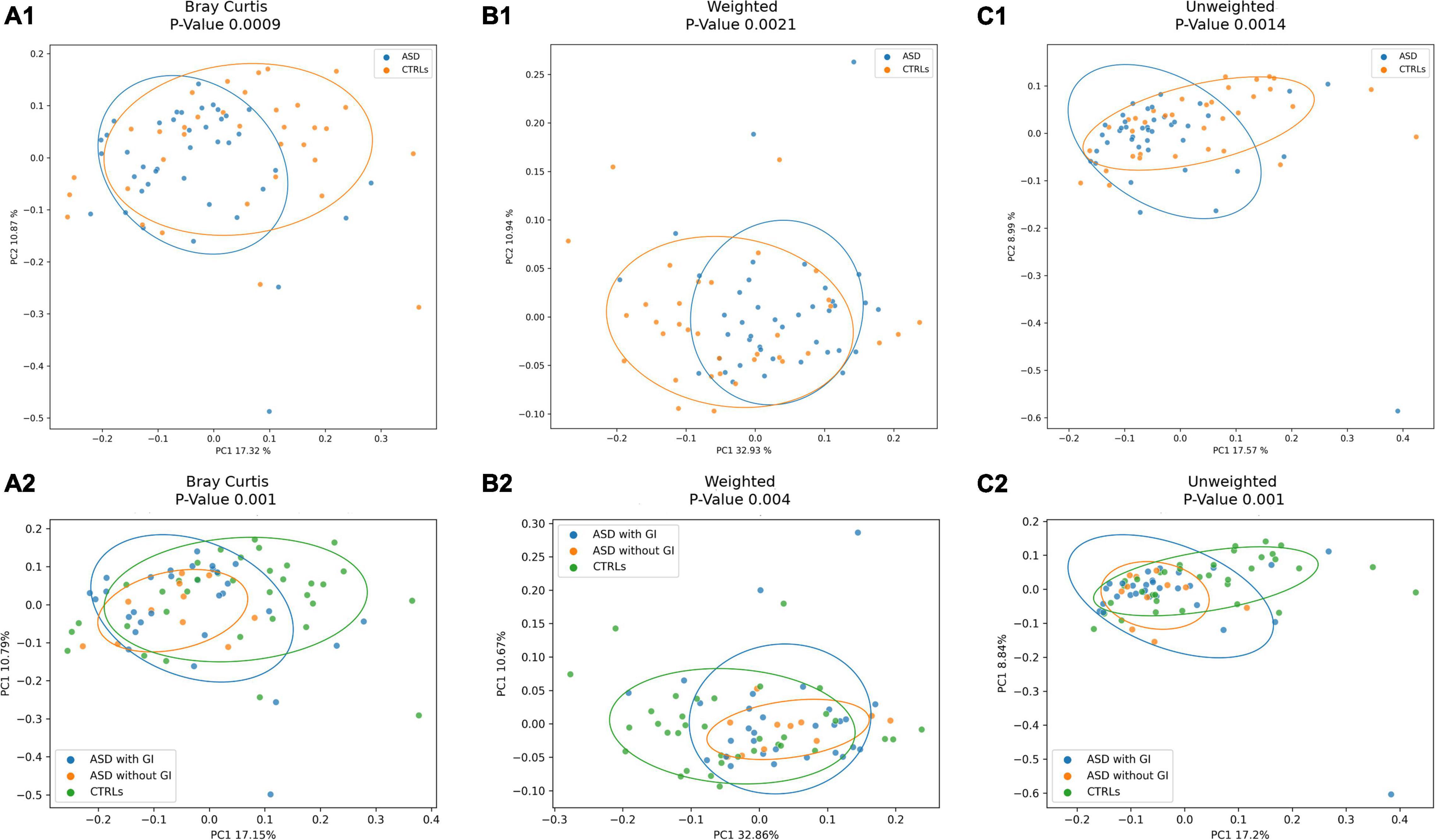
Figure 3. Beta-diversity ecological analysis. Principal Coordinate Analysis (PCoA) plots shows Bray Curtis in (A). (A1), patients with ASD versus CTRLs; (A2), patients with ASD grouped for presence of GI (with GI) or GI absence (without GI) symptoms versus CTRLs cohorts. Weighted UniFrac algorithms in (B). (B1), patients with ASD versus CTRLs; (B2), patients with ASD grouped for presence of GI (with GI) or GI absence (without GI) symptoms versus CTRLs cohorts. Unweighted UniFrac algorithms in (C). (C1), patients with ASD versus CTRLs; (C2), patients with ASD grouped for presence of GI (with GI) or GI absence (without GI) symptoms versus CTRLs cohorts.
At the phylum level (L2), for the coupled ASD versus CTRLs comparison, the OTUs abundance differences relied on Actinobacteria, Cyanobacteria, and TM7, which were higher in the CTRLs group, and on Proteobacteria and Bacteroidetes increase in ASD (Figure 4A1). By grouping the patients according to presence/absence of GI symptoms, a higher abundance of Bacteroidetes was observed in the ASD without GI symptoms group (Figure 4A2).
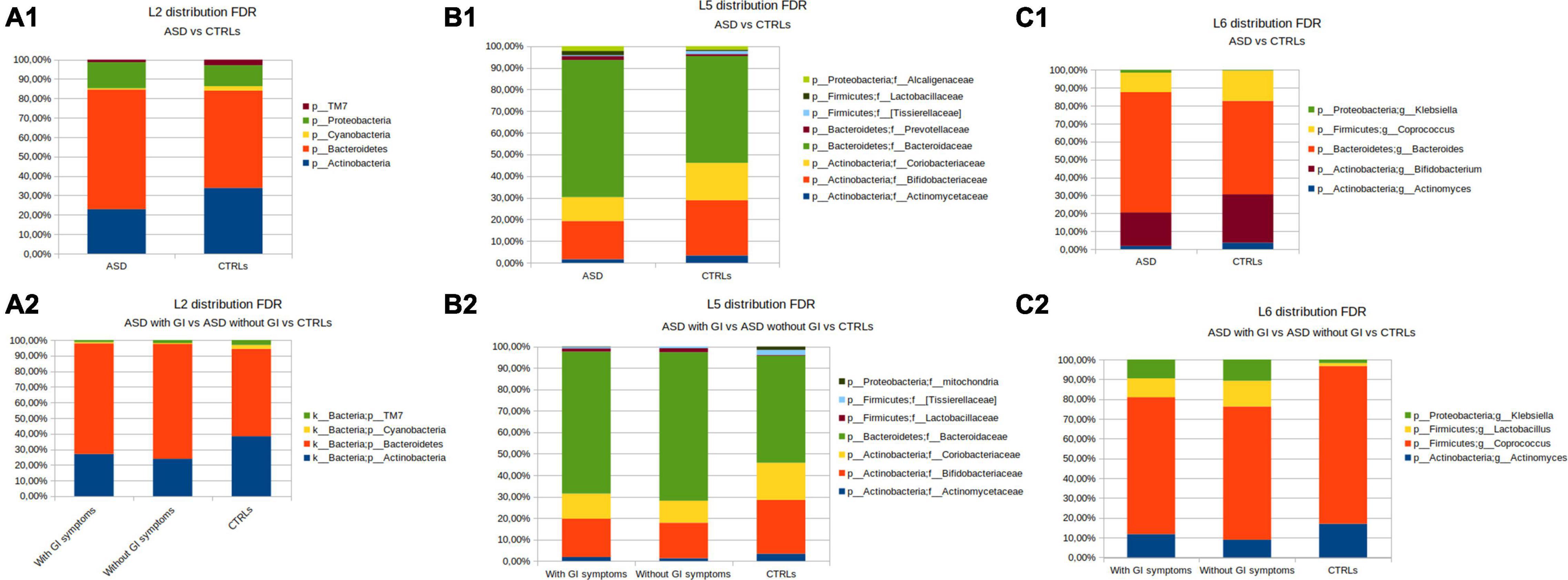
Figure 4. OTUs distribution performed by the Mann–Whitney U-test. (A) The phylum level; (A1) the patients with ASD versus CTRLs; (A2) the patients with ASD grouped for presence of GI (with GI) or GI absence (without GI) symptoms versus CTRLs cohorts. (B) The family level; (B1) the patients with ASD versus CTRLs; (B2) the patients with ASD grouped for presence of GI (with GI) or GI absence (without GI) symptoms versus CTRLs cohorts. (C) The genus level; (C1) ASD versus CTRLs; (C2) the patients with ASD grouped for presence of GI (with GI) or GI absence (without GI) symptoms versus CTRLs. Only statistically significant OTUs (Mann-Whitney U p-value FDR ≤ 0.05) were represented.
At the family level (L5), Alcaligenaceae, Lactobacillaceae, Prevotellaceaeae, and Bacteroidaceae resulted in more abundant in ASD, while, in the CTRLs subjects, Coriobacteriaceae, Bifidobacteriaceae, Actynomicetaceae, and (Tissierellaceae) were higher than in ASDs (Figure 4B1). Bacteroidaceae and Lactobacillaceae were higher in ASD without GI symptoms, while Coriobacteriaceae, Bifidobacteriaceae, (Tissierellaceae), and Actynomicetaceae appeared to increase in CTRLs (Figure 4B2).
At the genus level (L6), Bacteroides and Klebsiella were more abundant in ASDs than in CTRLs, while Coprococcus, Bifidobacterium, and Actinomyces were higher in CTRLs (Figure 4C1). In particular, the detail of the five statistically significant OTUs comparisons for ASD versus CTRL at the genus level is reported in Supplementary Figure 4. In the separation of the patients with ASD by presence/absence of GI symptoms, the comparison with CTRLs highlighted that Klebsiella and Lactobacillus were higher in the ASD without GI symptoms (Figure 4C2).
Ecological analysis in terms of α- and β-diversity and global OTUs distribution was also performed for the patients with ASD grouped according to (i) autism symptoms severity, (high/moderate and low) (Supplementary Figures 5–7); (ii) FS (presence/absence) (Supplementary Figures 8–10); and (iii) picky eaters (PEs) (presence of FS and/or gluten/casein-free diet) and no-PE (absence of FS and/or gluten/casein-free diet) (Supplementary Figures 11–13) in order to evaluate all variables affecting GM composition.
By considering these clinical and anamnestic features, both α-diversity and β-diversity were notable to discriminate any of the ASD-stratified subgroups.
In addition, the influence of confounding factors was considered. Firstly, in the comparison of ASD versus CTRLs, age and gender statistically affected the differences between the two groups (Supplementary Table 3). Thus, to investigate the effect of age, the subjects were stratified into two groups [<0–5 years old (23 ASD; 11 CTRLs) and ≥5 years old (18 ASD; 24 CTRLs)]. Indeed, the α- and β-diversity and the global OTUs distribution showed statistically significant differences between the patients and CTRLs (Supplementary Figures 14–16). Particularly, α-diversity showed differences (p-value ≤ 0.05) between ASD ≤ 5 versus CTRLs ≤ 5 years old and, also, versus the entire group of CRTLs (Supplementary Figures 14A1–D1,A2–D2).
Also, based on age subgroups’ comparison, the OTUs distribution showed for Bacteroidetes and Actinobacteria statistically significant differences (p-value ≤ 0.05) (Supplementary Figure 16A). Moreover, by considering the comparison obtained by the Mann-Whitney U-test on the entire group of CTRLs versus ASD grouped by age, Cyanobacteria and TM7 were statistically different for ASD < 5 versus CTRLs (Supplementary Figure 16B), while Cyanobacteria, Proteobacteria, Bacteroidetes, and Actinobacteria resulted in statistically different (p-value ≤ 0.05) for the comparison between ASD ≥ 5 versus CTRLs (Supplementary Figure 16B). At the family level, Gemellaceae, Streptococcaceae, Erysipelothricaceae, Carnobacteriaceae, Bifidobacteriaceae, and Actinomycetaceae were statistically different for ASD < 5 versus CTRL (Supplementary Figure 16C). In addition, for the comparison referred to the entire subgroups’ set obtained by the Mann-Whitney U-test, Alcaligenaceae, Prevotellaceae, Bacteroidaceae, Ciriobacteriaceae, Actinomycetaceae, Erysipelotrichaceae, and Bifidobacteriaceae resulted in statistically different (p-value ≤ 0.05) only for the comparison between ASD ≥ 5 versus CTRLs (Supplementary Figure 16D).
Moreover, by considering the ASD subgroups characterized in terms of dietary habits and FS, confounders’ analysis highlighted statistical significance in their comparison (Supplementary Table 3). However, adjusted results did not provide for further GM comparisons or any additional information for low sample sizing (data not shown).
Microbial Biomarkers Predictive Analysis: Linear Discriminant Analysis Effect Size
To infer taxonomic differences between the GM of the patients with ASD and CTRLs in terms of potential biomarkers, a linear discriminant analysis (LDA) effect size (LEfSe) algorithm (cutoff ≥ 2) was performed and 49 top-ranking OTUs characterizing the microbiota were identified (Figure 5).
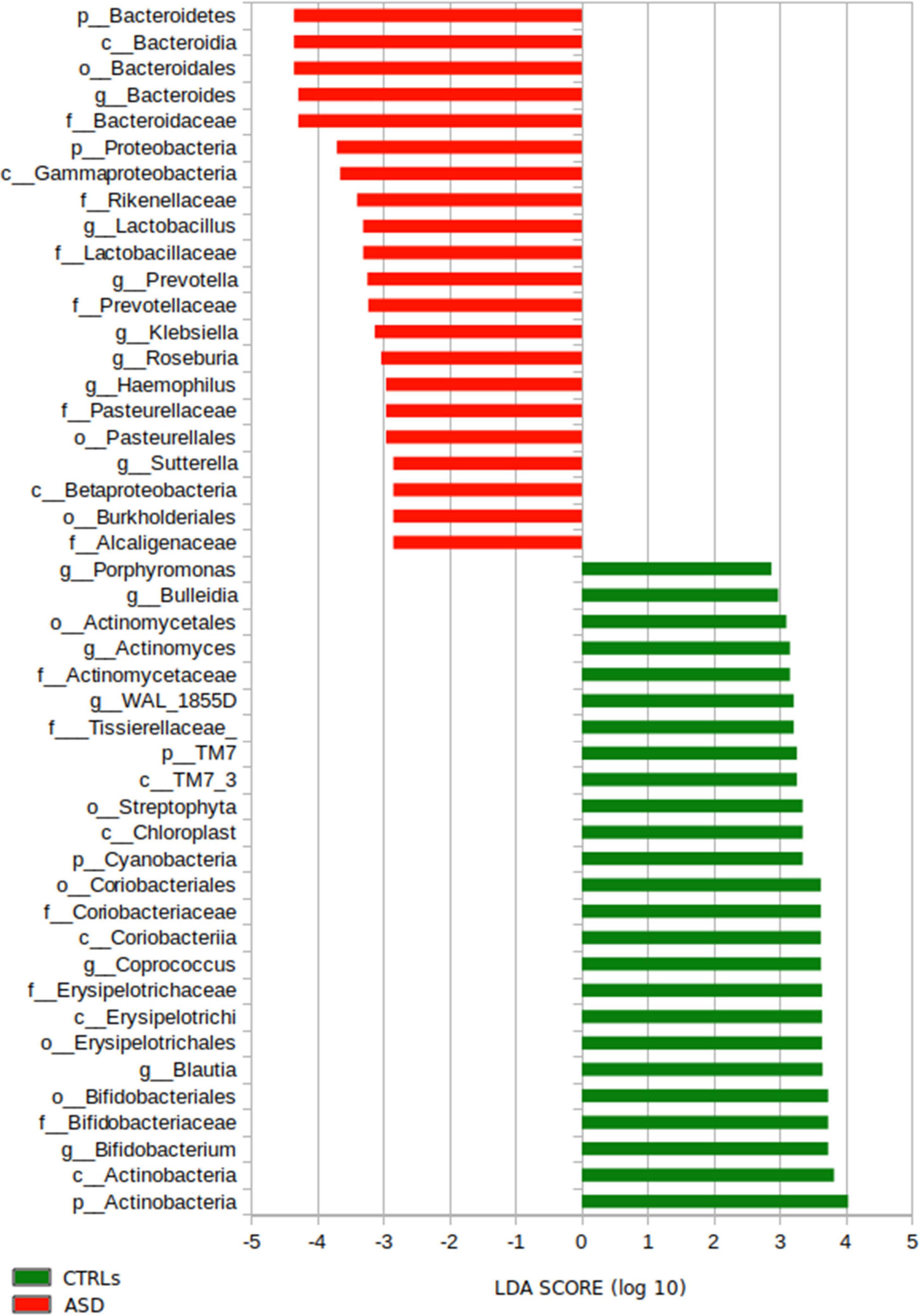
Figure 5. LEfSe analysis for the comparison between the patients with ASD and CTRLs cohorts’ gut microbiota. Histogram represents the LDA scores until L6 taxonomy filtered by statistical significance between the two groups. In red and in green are represented microbial biomarkers for the patients with ASD and the CTRLs subjects, respectively.
In particular, the analysis provided ASD-related microbial biomarkers (p-value ≤ 0.05), such as Bacteroidetes, Proteobacteria (L2); Bacteroidaceae, Rikenellaceae Lactobacillaceae, Prevotellaceae, Pasteurellaceae, and Alcaligenaceae (L5); Bacteroides, Lactobacillus, Prevotella, Klebsiella, Roseburia, Haemophilus, and Sutterella (L6) (Figure 5).
On the other hand, the GM of CTRLs was characterized (p-value < 0.05) by TM7, Cyanobacteria, and Actinobacteria (L2); Tissierellaceae, Coriobacteriaceae, Erysipelotrichaceae, and Bifidobacteriaceae (L5); Porphyromonas, Bulleidia, Actinomyces, WAL_1855D, Coprococcus, Blautia, and Bifidobacterium (L6) (Figure 5).
Moreover, the microbial predictive analysis was applied to ASDs grouped for presence/absence of GI symptoms versus CTRLs (Figures 6A,B). Significant differences in the comparison between ASD with GI symptoms versus CTRLs relied on the ASD biomarkers Haemophilus, Pasteurellaceae, Roseburia, Fusobacterium, and Enterobacteriaceae (Figure 6A), while, for the ASD subgroup without GI symptoms compared to CTRLs, Proteobacteria, Gammaproteobacteria, Ruminococcaceae, and Klebsiella (Figure 6B) were associated with ASD. On the contrary, for CTRLs, the highest scored microbial signatures were Blautia and Veillonella in the comparison versus ASD with GI symptoms, while Colinsella, Bulleidia, and Dorea better-differentiated CTRLs in the comparison versus ASD without GI symptoms (Figures 6A,B). Regarding the comparison between ASD with GI versus ASD without GI symptoms, Bilophila, Odoribacter, and Veillonella characterized ASD without GI, while ASD with GI symptoms was characterized by Roseburia (Figure 6C).
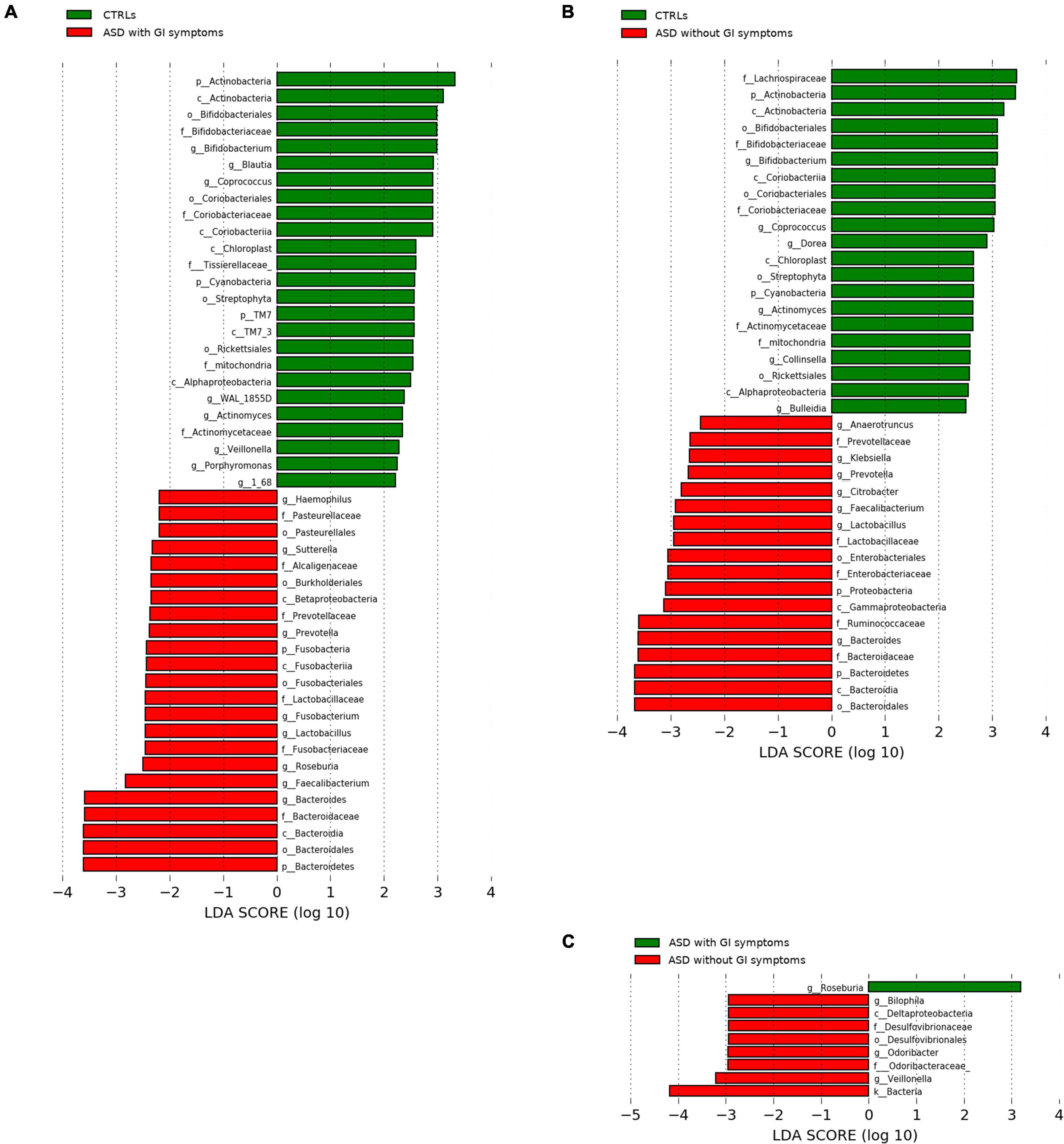
Figure 6. LEfSe analysis between the patients with ASD grouped for presence of GI (with GI) or GI absence (without GI) and CTRLs cohorts. Histogram represents the LDA scores of bacteria (L6) with statistically significant differential abundance between groups. (A) CTRLs versus ASD with GI symptoms; (B) CTRLs versus ASD without GI symptoms; (C) ASD with GI versus ASD without GI symptoms. In red, ASD; green, CTRLs. All OTUs reported were statistically significant (p-value ≤ 0.05).
Moreover, Gram-positive bacteria, such as Ruminococcaceae, resulted higher in the LEfSe analysis of the patients with ASD in the absence of GI symptoms when compared to CTRLs, while the abundance of Ruminococcaceae in PE/noPE and FS/noFS was comparable (data not shown).
Predictive Functional Analysis
Phylogenetic Investigation of Communities by Reconstruction of Unobserved States based on reference OTUs was applied to predict abundances of the KEGG orthologs (KOs) (Figure 7).
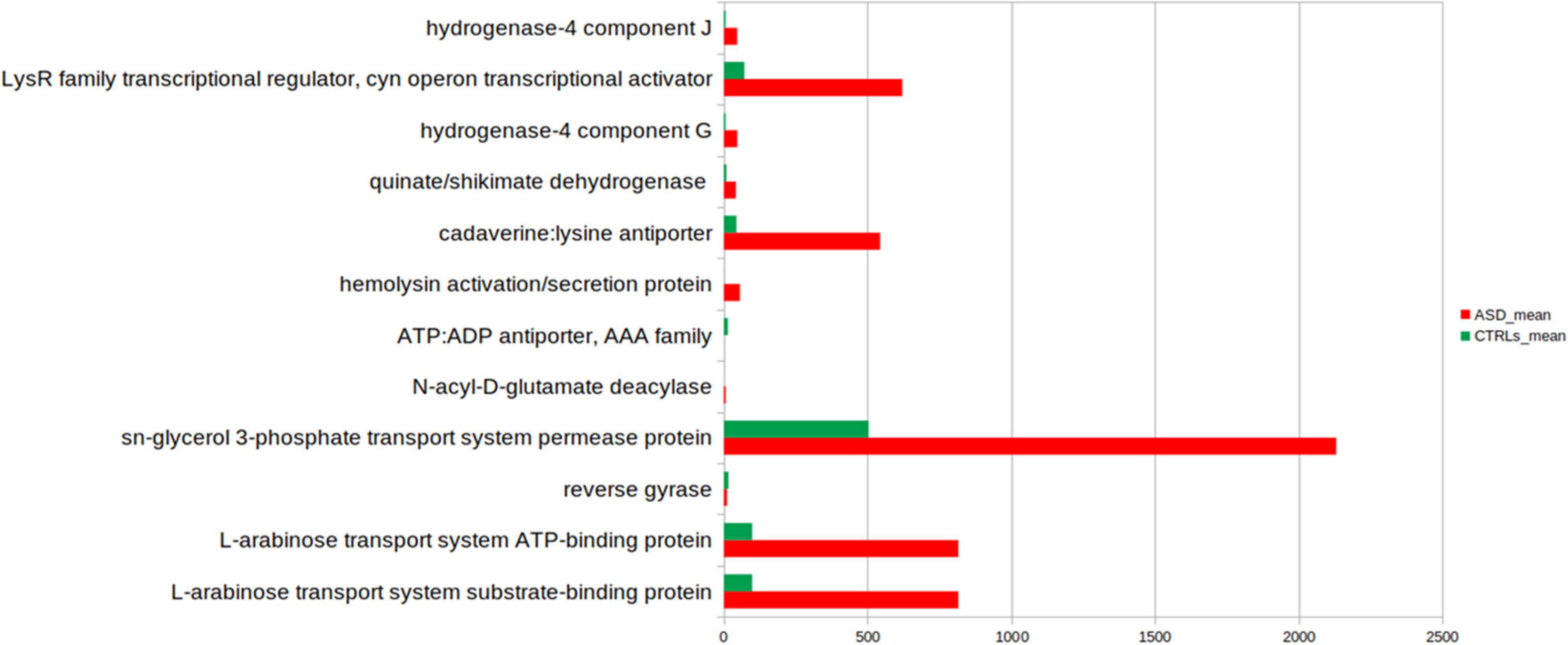
Figure 7. Kyoto Encyclopedia of Genes and Genomes (KEGG) biomarkers inferred from the whole set of OTUs of the patients with ASD and the CTLRs subjects. A linear discriminant effect size (LEfSe) analysis was performed [α = 0.05, a logarithmic discriminant analysis (LDA)] score threshold = 2.0). ASD- and CTRLs-related KEGGs entries are represented in red and green bars, respectively.
Few KOs showed significant differences in the GM of ASD and the CTRLs subjects (p-value ≤ 0.05). Twelve metabolic microbial pathways differentially expressed were related to 46 selected OTUs, and 10/12 were linked to patients with ASD (Figure 7). In particular, the top 10 pathways were hydrogenase-4 component J; LysR family transcriptional regulator cyn operon transcriptional activator; hydrogenase-4 component G; quinate/shikimate dehydrogenase; cadaverine:lysine antiporter; hemolysin activation/secretion protein; N-acyl-D-glutamate deacylase; sn-glycerol 3-phosphate transport system permease protein; L-arabinose transport system ATP-binding protein; L-arabinose transport system substrate-binding protein (Figure 7 and Table 2).
The PICRUSt analysis was also applied to ASDs subgroups compared to CTRLs (Figures 8A–C and Supplementary Tables 4–6). Significant differences (p-value ≤ 0.05) in the comparison between ASD with GI symptoms versus CTRLs particularly relied on quinate/shikimate dehydrogenase and N-glycosylase/DNA lyase for ASDs and reverse gyrase for CTRLs (Figure 8A and Supplementary Table 4).
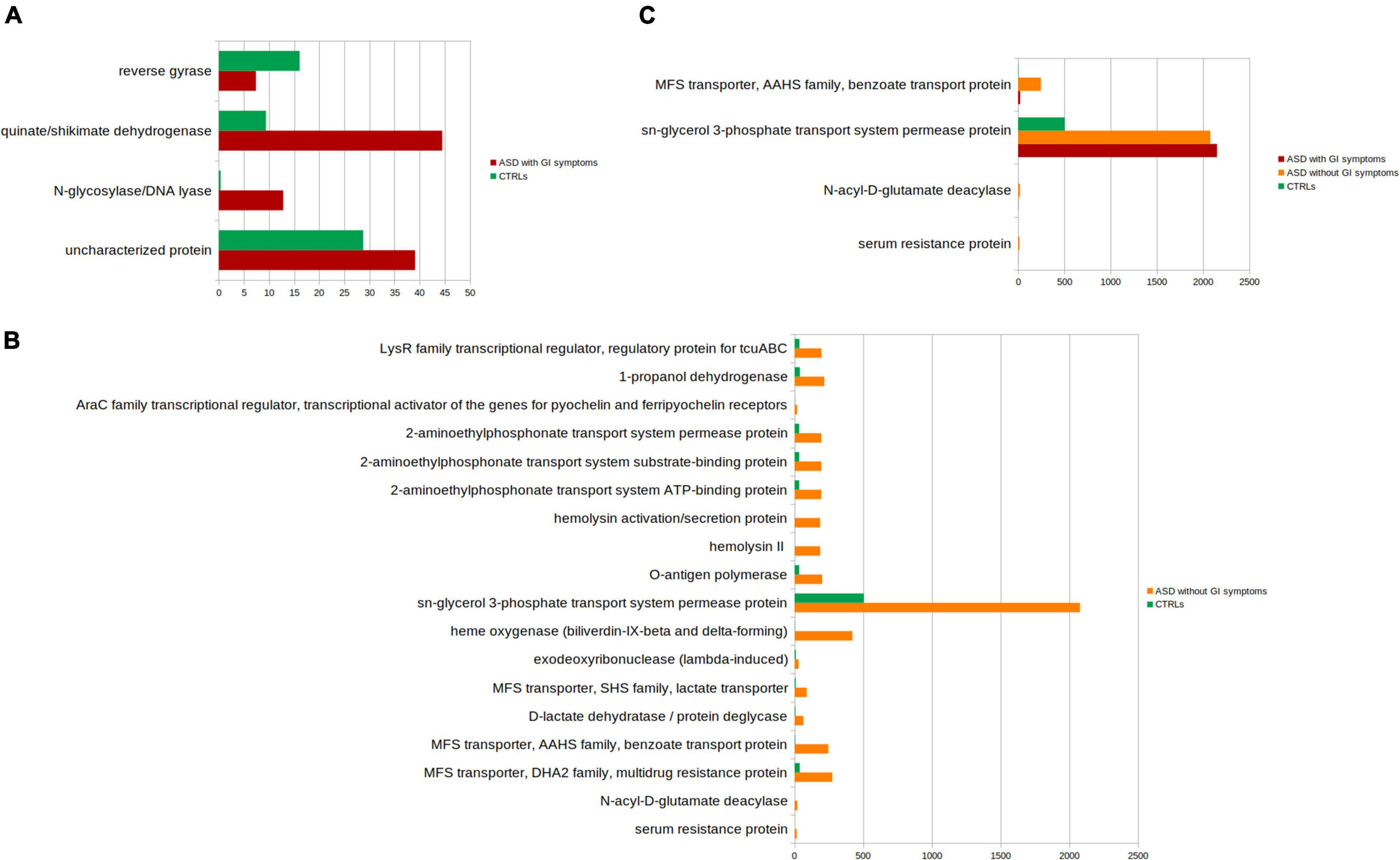
Figure 8. Kyoto Encyclopedia of Genes and Genomes (KEGG) biomarkers inferred from the whole set of OTUs of the patients with ASD grouped into the patients with GI and without GI symptoms versus the CTRLs subjects. KOs distribution for each group computed by the Kruskal-Wallis test (p-value < 0.001 and relative abundances > 10). (A) ASD with GI symptoms versus CTRLs; (B) ASD without GI symptoms CTRLs; (C) ASD with GI versus ASD without GI symptoms and versus CTRLs. In red, ASD with GI symptoms; orange, ASD without GI symptoms; green, CTRLs.
The comparison ASD without GI symptoms versus CTRLs allowed us to predict 14 overexpressed ASD-related metabolic pathways (Figure 8B and Supplementary Table 5), including sn-glycerol 3-phosphate transport system permease protein; cadaverine: lysine antiporter; 1-propanol dehydrogenase; and N-acyl-D-glutamate deacylase.
Lastly, the comparison of ASD with GI symptoms versus ASD without GI symptoms and versus CTRLs identified the sn-glycerol 3-phosphate transport system permease protein associated with both ASD with/without GI symptoms (Figure 8C and Supplementary Table 6).
The comparison between ASD with GI symptoms versus ASD without GI symptoms did not show statistically significant differences.
Hierarchical Clustering of Subjects According to Operational Taxonomic Unit Distribution
Global OTUs distribution differences between ASD and CTRLs were evaluated by hierarchical clustering in Supplementary Figure 17. The ASD and CTRL groups displayed different microbial profiles at L2 (Supplementary Figure 17A), revealing two main clusters (namely, A and B). The major part of the patients with ASD belonged to the Cluster B and was characterized by the highest abundance of Bacteroidetes and Proteobacteria. Conversely, the Cluster A was predominantly constituted by the CTRL group and represented by a major abundance of Firmicutes, Cyanobacteria, Actinobacteria, and TM7.
At L6, the heatmap showed clear separation between ASD and CTRL, displaying different microbial profiles (Supplementary Figure 17B). The Cluster A, mainly composed of the patients with ASD, was characterized by an increase in Bacteroides, Sutterella, Lactobacillus, Prevotella, Staphylococcus, and Haemophilus. On the contrary, the CTRLs mainly included in Cluster B showed an increased level of WAL_1855d, Coriobacteriaceae, Bifidobacterium, Streptophyta, Lachnospiraceae, Coprococcus, TM7-3, Gemellaceae, Eggerthella, Eryspelotrichaceae, Blautia, and Ruminococcus belonging to the Lachnosperaceae family, Actinomyces, and Streptococcus.
Correlation Network Analysis of Autism Spectrum Disorder Clinical Features and Gut Microbiota Ecology
To explore the possible relationship between clinical features and GM composition in ASD children, a correlation network was generated by Pearson’s correlation coefficient (Figure 9). At the phylum level (L2) (Figure 9A), the correlation analysis showed a significant statistically positive correlation (p-value ≤ 0.05) between few clinical variables and OTUs. Particularly, sIgA positively correlated with Proteobacteria and Actinobacteria, and drugs administration with Proteobacteria. In addition, at least one functional GI symptom, probiotics, and autism symptoms’ severity correlated positively with Bacteroidetes. On the contrary, the analysis showed a negative correlation between abdominal pain and Firmicutes; autism symptoms severity, IQ/DQ, and Cyanobacteria (Figure 9A).
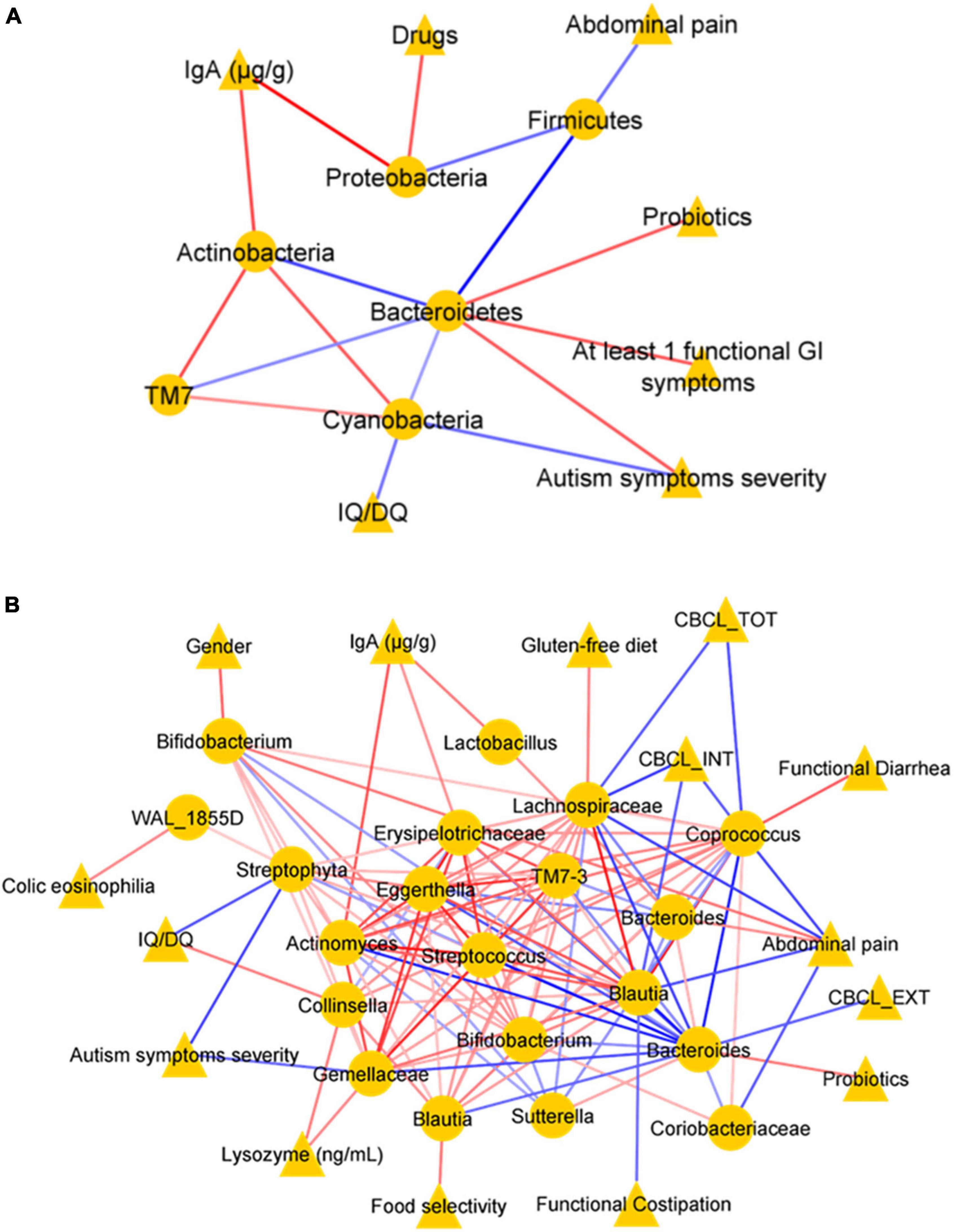
Figure 9. Correlation networks. Correlation analysis between OTUs (selected with the t-test with p-value ≤ 0.05) and clinical variables at the phylum level (L2) (A) and at the genus level (L6) (B). In each network, nodes represent the OTUs (circles) and the clinical variables (triangles), and an edge between two nodes occurs if they exhibit a statistically significant correlation (p-value ≤ 0.05). The color of the network edges indicates positive (red) and negative (blue) correlations.
In addition, at L6, the analysis showed a statistically significant (p-value ≤ 0.05) positive correlation between paired clinical variables and OTUs, such as lysozyme concentration and Gemellaceae; sIgA and Erysipelotrichaceae, antibiotic therapy, and Prevotella; diarrhea and Coprococcus; gender and Prevotella; casein-free diet and Staphylococcus; colic eosinophilia and WAL_1855D; gluten-free diet and Lachnospiraceae; abdominal functional distention and Prevotella; probiotics and Bacteroides; and between OTUs-OTUs, such as Lactobacillus and Actinomyces. Conversely, abdominal pain showed negative correlations with Coriobacteriaceae, Coprococcus, Blautia, and Lachnospiraceae; constipation with Staphylococcus (Figure 9B). In addition, almost all neuropsychological features showed statistically significant negative correlations (p-value < 0.05): autism symptoms’ severity with Gemellaceae and Streptophyta; CBCL_TOT and CBCL_EXT with Lachnospiraceae and Bacteroides; CBCL_INT with Ruminococcus, Lachnospiraceae, except for a positive correlation with Prevotella; autism symptoms severity and IQ/DQ with Streptophyta (Figure 9B).
Model Classifications Analysis Based on Operational Taxonomic Units and KEGG Orthologs Pathways’ Features
To investigate if the GM of the patients with ASD could be predictive of a disease phenotype, a model classification analysis based on machine learning (ML) was used. By this analysis, the most important features of the GM were selected at L6, revealing that the GM ecology was able to classify the 80% of the patients with ASD compared to CTRLs based on the two models: Quadratic Discriminant Analysis and Gaussian Process Classifier (Supplementary Table 7). The specific selected OTUs for ASD and CTRLs obtained by the consistent models are reported in Figure 10. Contextually, a model classification analysis based on ML identified both KOs and ko pathways able to classify the 73% of the patients with ASD versus CTRLs (p-value ≤ 0.05). The flagellar assembly (ko02040) resulted in the best KO pathway, describing ASDs (Supplementary Figure 18).
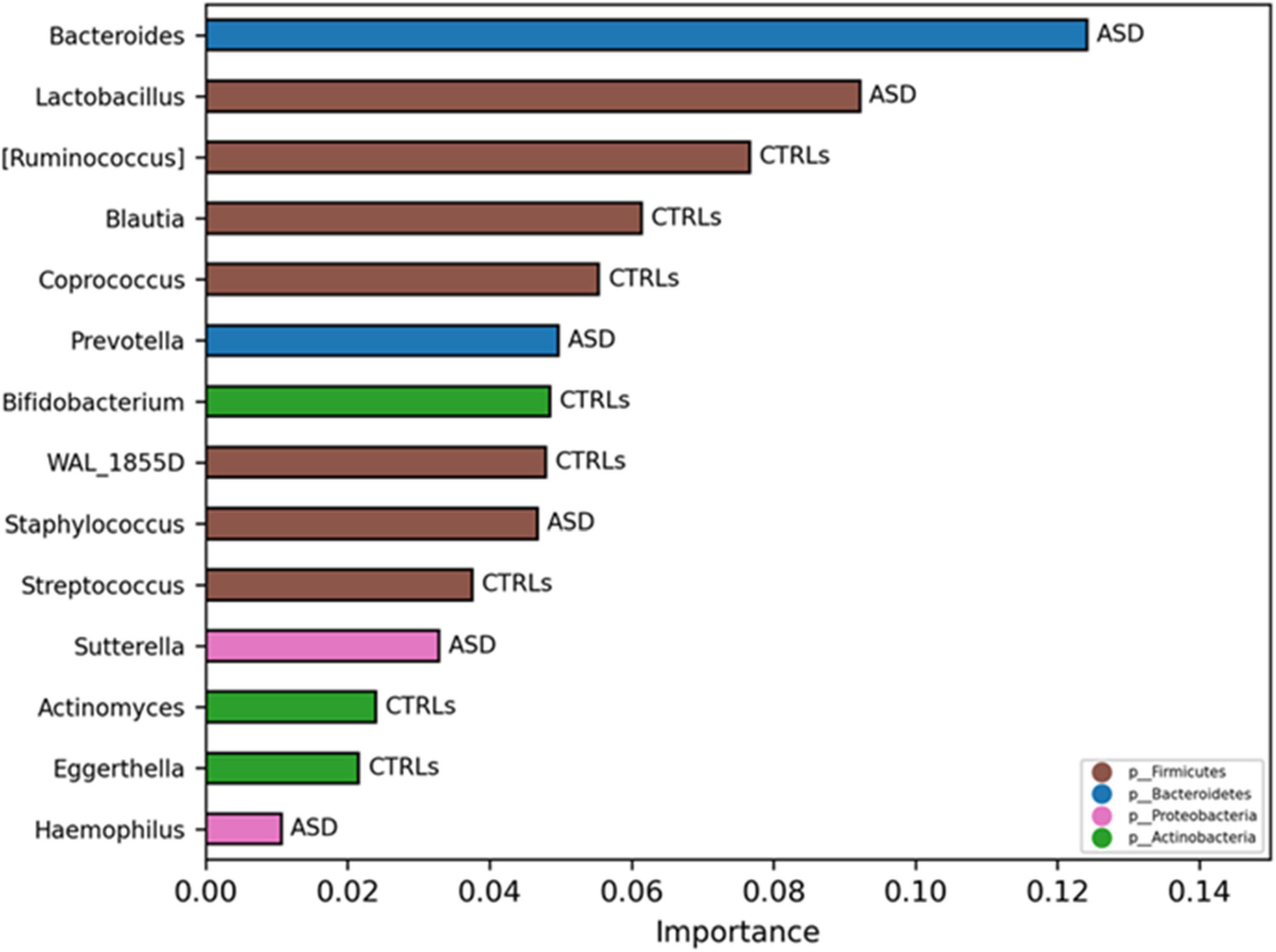
Figure 10. Significant OTUs selected by classification model analysis. The bars represent the importance scores of each OTU in the ASD prediction models versus CTRLs.
Discussion
Several aspects of individuals’ behavior and physiology can be affected by changes in the host genome and microbiome (Sharon et al., 2016; Vuong and Hsiao, 2017; Lynch and Hsiao, 2019; Sherwin et al., 2019), and their interactions (Fischbach and Segre, 2016); hence, it is crucial to understand whether ASD symptoms are influenced by modifications of the host’s GM-brain axis. Indeed, growing pieces of evidence indicate that environmental factors, including diet, family habits, etc., may play an important role in the ASD (Roussin et al., 2020; David et al., 2021; Yap et al., 2021).
Particularly, GI functional symptoms, such as constipation, abdominal pain, flatulence, and diarrhea, have been described as representing an important comorbidity (Liu S. et al., 2019) in the wide autism phenotype, if compared with children with typical development (Chaidez et al., 2014). Hence, gut inflammation related to intestinal barrier integrity and innate immune barrier defense exerted as antimicrobial action against Gram-positive bacteria were herein evaluated, determining fecal sIgAs, zonulin, and lysozyme titers.
In our dataset, the median levels of sIgA were slightly higher in stool samples of CTRLs compared with ASD, suggesting lower levels of IgA in these patients, possibly associated with impaired gut immunity (Zhou et al., 2018). Interestingly, low substantial inflammation may be due to a specific turn-over of Sutterella species (Kaakoush, 2020), identified as microbial biomarkers of the patients with ASD by LEfSe analysis and corroborated by comparable values of IgA for both the patients with ASD in presence/absence of GI symptoms.
Also median value of lysozyme appeared higher in the CTRLs group compared to ASD, with a further decrease in the patients with GI symptoms, as previously observed (Adams et al., 2011). Worth noting is that the statistically significant presence of Ruminococcus in ASD without GI symptoms may corroborate the idea that a starch-degrading bacterium (Lordan et al., 2020) could improve the GI wellness, preserving from symptoms, more responding to nutritional triggers rather than to host innate immunity (Yap et al., 2021). Typical ASD profiles, associated with GI symptoms and behavior indices, may be correlated with ASD severity (Holingue et al., 2018), hence suggesting that the communication between gut-brain axis and nutritional altered habits may be involved in ASD pathogenesis (Mayer et al., 2015; Beaudet, 2017). Moreover, the presence of Roseburia as the microbial biomarker of the patients with ASD, also in the presence/absence of GI symptoms, compared to CTRLs, may, indeed, contrast the idea that SCFAs-producing bacteria may preserve the microbiome ecology and the defense against pathogenic bacteria, without the innate immunity response of lysozyme (Adams et al., 2011).
The zonulin is involved in the intestinal permeability regulation and has been reported as reducing the expression of barrier components in the tight junctions in 75% of the patients with ASD (Fiorentino et al., 2016; Özyurt et al., 2018). In addition, zonulin is a precursor of haptoglobin 2 (Hp2) (Tripathi et al., 2009), and some previous works have suggested that the presence of the haptoglobin 2 allele could be related to a higher risk of immune-mediated diseases development (Blum et al., 2008).
However, Rose et al. (2018) observed no changes in zonulin levels in ASDs with no statistically significant over-expression of the HP2 gene product in the patients with ASD, but only a significant difference between ASD with GI symptoms and typically developing children with GI symptoms.
However, in this latter case, it is remarkable to underline that the zonulin comparison between ASDs and CTRLs subjects was meaningless based on the lack of linearity between intestinal permeability and fecal zonulin concentration in the normal-weight CTRLs subjects (Seethaler et al., 2021).
The high/medium autism severity and the presence of at least one of the GI symptoms in our patients resulted intrinsically related, possibly suggesting the GI symptom phenotype as the driving force of the underlying neuropsychological phenotype.
About global microbial distribution, the Pasteurellaceae family (Liu F. et al., 2019) was significantly associated with patients with ASD reinforcing the idea of a link to host inflammation (Shin et al., 2015). Indeed, the outgrowth of Proteobacteria promotes intestinal inflammation driven by dysregulated innate immune responses, as demonstrated by studies in mice models with genotypes influencing the adaptive immunity (Shin et al., 2015).
Moreover, the data suggested that the observed GM ecological profiles were specific for the subject affected by ASD, dependently of age, in agreement to what happens in children affected by neuropsychiatric syndromes related to pediatric autoimmune neuropsychiatric disorders associated with streptococcal infections syndrome (PANDAS), in which the GM ecological analyses showed the presence of two clear subject clusters based on the age range (Quagliariello et al., 2018).
In addition, Bacteroidetes were predominantly associated with the patients with GI symptoms underlying autism symptoms severity, as previously reported (Morais et al., 2021). Indeed, a cross-sectional study (Christian et al., 2015) reported important gut microbial differences in terms of OTUs diversity and abundance related to maternal ratings of toddlers temperament, especially among boys. In particular, the major discriminant variable was, indeed, represented by the Bacteroidetes Phylum that could be associated with infant temperament.
Our patient’s cohort was composed prevalently by males, which represent one of the variables that affect their gut microbial profile, and there are also pieces of strong evidence (Tamana et al., 2021) of positive relationships between Bacteroidetes in late infancy and subsequent neurodevelopment, most prominently among males. Particularly, concentrations of propionic acid produced by Bacteroides may play beneficial effects for the enterocytes despite of high concentrations may be related to toxic effects (Frye et al., 2016; Srikantha and Mohajeri, 2019). Moreover, Bacteroides fragilis showed a pathological role described in neurodevelopment processes, including ASDs (Nagaraju et al., 2019).
Besides Bacteroides (De Angelis et al., 2013; MacFabe, 2015), also, Roseburia was detected in higher abundance in the patients with ASD compared to CTRLs; interestingly, Roseburia degrades starch and ferments other carbohydrates to synthesize SCFAs.
Particularly, propionic acids and other SCFAs have different impacts on cellular and mitochondrial activity, which could include also the modulation of T-cell function and cytokine production (Rose et al., 2017) in a concentration-dependent manner (Frye et al., 2015). Hence, SCFAs could disrupt cellular physiology to cause functional GI disease related to ASD, such as dismotility and non-specific inflammation (Kang et al., 2017; Rose et al., 2017).
Moreover, the role of Roseburia of being a microbial biomarker of patients with ASD with GI symptoms, characterized by lysozyme defect, compared to the patients without GI symptoms, may, indeed, question the idea that SCFAs-producing bacteria may preserve the GM ecology (Tamanai-Shacoori et al., 2017) without the innate immunity response of lysozyme. On the other hand, the statistically significant presence of Ruminococcus (Ruminococcaceae) in ASD without GI symptoms may corroborate the idea that a starch-degrading bacterium may, in any case, improve the GI wellness, preserving from symptoms, and responding to nutritional triggers rather than to host innate immunity.
Moreover, Roseburia has a low capacity to degrade free amino acids (FAAs) at the gut level and, because, in ASD, the especially increased FAA has been described to be glutamate, probably its increase is related to high concentration of Roseburia (De Angelis et al., 2013). Indeed, glutamate acts as neurotransmitters in the CNS, and, since it is a potent neurotoxin, an excess could lead to neuronal death, as reported in neuropsychiatric disorders pathophysiology, including autism (Won et al., 2012; Yang and Chang, 2014). Consistently, De Angelis et al. (2015) determined FAAs in fecal samples of the patients with ASD associated with the presence of proteolytic bacteria, such as Bacteroides and Roseburia.
Prevotella and Lactobacillus were also higher in all the patients with ASD, compared to CTRLs. Previous research has indicated a correlation between dietary habits and high levels of Prevotella spp. in patients with ASD. High abundance of Prevotella spp. affects inflammation and mucosal and systemic T cell stimulation (Larsen, 2017; Iljazovic et al., 2021). However, Prevotella is also involved in the polysaccharides degradation and fermentation, leading to the production of SCFAs (Williams et al., 2017; Gálvez et al., 2020).
Sutterella distribution was higher in the GM of ASDs with GI symptoms, corroborating previous studies (Williams et al., 2012). ML actually identified this microbe as ASD features in the disease classification model.
On the contrary, beneficial bacteria, such as Bifidobacterium, decreased in all the ASD subjects regardless of presence or absence of GI symptoms, compared to CTRLs, thus reducing its protection activity against gut inflammation and cooperating in the development of the immune system (Medina et al., 2008; Salazar et al., 2008; Hashemi et al., 2017). The decrease of Bifidobacteria in the GM of ASD children may trigger rearranging of important microbes, potentially contributing to some ASD symptoms (Averina et al., 2020). Recently, Bifidobacterium has been proposed as a psycobiotics due to its ability to generate neuromodulators and to affect gut–brain relations by the interaction with other commensal microbes (Sarkar et al., 2016).
Consistently, with our results, Kang et al. (2013) detected a significantly higher abundance of Coprococcus in CTRLs compared to children with ASD. The reduction of Veillonella and Blautia in the children with ASD, regardless of GI symptoms, may be potentially linked to the presence of ASD severity symptoms rather than to GI symptoms severity or specific diet/supplement habits (i.e., FS, gluten- and casein-free diet). Thus, the reduction in ASD of these beneficial microbes might be involved in the pathogenesis of disease (Liu S. et al., 2019), indirectly influencing social brain functions (Kang et al., 2013). Conversely, the exceeding amount of ugly bacteria may increase the presence of toxic microbial by-products, passing through an irregular porous blood–brain barrier and entering into the bloodstream and the brain (Fiorentino et al., 2016).
One of the predicted biochemical pathways overexpressed in the children with ASD was the quinate/shikimate dehydrogenase, known to catabolize quinate and shikimate in Gram-negative bacteria, such as Proteobacteria, to produce aromatic AA, such as L-phenylalanine, L-tyrosine, and L-tryptophan, which represents molecular building blocks for protein biosynthesis (Herrmann and Weaver, 1999).
In addition, the sn-glycerol 3-phosphate transport system permease protein represented an overexpressed pathway in ASD. It is involved in the incorporation of exogenous FAs into phosphatidylethanolamine in Gram-negative bacteria belonging to Proteobacteria phylum (Rock and Jackowski, 1985).
The other highly represented biochemical pathways were the cadaverine lysine antiporter that represents a signaling system to acid stress in different γ-Proteobacteria (Brameyer et al., 2020); the bacterial 1-propanol dehydrogenase, associated with alcohol dehydrogenase (ADH), is able to attenuate alcohol toxicity and modulate alcohols’ interaction at the GM level (Ferraro and Finkel, 2019; Lin et al., 2021); the N-Acyl-D-glutamate amidohydrolase is produced by Gram-negative bacteria and derived from D isomers of FAAs, such as N-acetylglutamate, glutamate, aspartate, and asparagine (Sakai et al., 1991).
As diet represents a major reservoir of circulating metabolites, its modulation has been proposed to modulate intricate behaviors (Needham et al., 2018a,b, 2021; Pulikkan et al., 2019), including ASDs (David et al., 2021; Yap et al., 2021).
Moreover, the research is moving toward the understanding of GM-related metaproteomics (Levi Mortera et al., 2022) and metabolomics and microbiota-host crosstalk, trying to describe the functional ASD-related enterophenotype. In this context, the nutritional role needs to be further investigated by novel approaches, such as foodomics (Putignani and Dallapiccola, 2016), applied to patient cohorts from different geographical settings and social environments. In addition, the role of host-related immunological biomarkers and the gut intestinal barrier needs additional investigations.
Only a novel multi-omics approach, integrating all these factors in a new phenotype-“enterophenotype” model, may shed light on the complex pathophysiology of the autism disease by artificial intelligence-based algorithms to provide clinical decision support systems (CDSS) (Putignani et al., 2019). Surely, the small cohort sample size, especially for stratified groups, may represent a study limitation, and a further scale-up of our patients’ set will be performed to properly assess phenotype stratification.
In conclusion, for our patients cohort, the GM of the children with ASD was characterized by a well-differentiated gut microbial profile, featured by reduced richness and diversity as well as by specific composition and structure of bacterial communities and functions, dependently on host age and GI hallmarks.
Data Availability Statement
The datasets presented in this study can be found in online repositories. The names of the repository/repositories and accession number(s) can be found below: PRJNA754695 (https://www.ncbi.nlm.nih.gov/bioproject).
Ethics Statement
The studies involving human participants were reviewed and approved by Bambino Gesù Children’s Hospital (OPBG) Ethics Committees (1404_OPBG_2017 and 1113_OPBG_2016). Written informed consent to participate in this study was provided by the participants’ legal guardian/next of kin.
Author Contributions
PV, MVR, SV, GI, AG, and LP contributed to the conceptualization. PV, AR, SGa, EL, and SGu contributed to the methodology. LP, PP, and SGa contributed to the validation. SA-N, PV, AR, FC, VG, and SGa contributed to the formal analysis. SGu, EL, GI, SV, and LP contributed to the investigation. LP contributed to the resources. PV, LP, and GI contributed to the data curation. PV, MVR, and LP wrote the original draft. PV, LP, and SGu contributed to the writing, reviewing, and editing the manuscript. AG, LP, AR, and SV contributed to the supervision. SV and LP contributed to the project administration and funding acquisition. All authors have read and agreed to the published version of the manuscript.
Funding
This work was supported by the Italian Ministry of Health (Ricerca Corrente 2016, 2017, 2018, 2019, and 5‰ 2020, assigned to LP).
Conflict of Interest
VG and SGa were employed by GenomeUp.
The remaining authors declare that the research was conducted in the absence of any commercial or financial relationships that could be construed as a potential conflict of interest.
Publisher’s Note
All claims expressed in this article are solely those of the authors and do not necessarily represent those of their affiliated organizations, or those of the publisher, the editors and the reviewers. Any product that may be evaluated in this article, or claim that may be made by its manufacturer, is not guaranteed or endorsed by the publisher.
Supplementary Material
The Supplementary Material for this article can be found online at: https://www.frontiersin.org/articles/10.3389/fmicb.2022.871086/full#supplementary-material
Footnotes
- ^ https://www.cdc.gov/nccdphp/dnpao/growthcharts/training/overview/page5_1.html
- ^ https://www.ncbi.nlm.nih.gov/bioproject/?term=PRJNA754695
References
Achenbach, T. M., Dumenci, L., and Rescorla, L. A. (2003). DSM-Oriented and Empirically Based Approaches to Constructing Scales From the Same Item Pools. J. Clin. Child Adolesc. Psychol. 32, 328–340. doi: 10.1207/S15374424JCCP3203_02
Adams, J. B., Johansen, L. J., Powell, L. D., Quig, D., and Rubin, R. A. (2011). Gastrointestinal flora and gastrointestinal status in children with autism – comparisons to typical children and correlation with autism severity. BMC Gastroenterol. 11:22. doi: 10.1186/1471-230X-11-22
American Psychiatric Association [APA] (2013). Diagnostic and statistical manual of mental disorders: DSM-5, 5th Edn. Washington, D.C: American Psychiatric Association.
Averina, O. V., Kovtun, A. S., Polyakova, S. I., Savilova, A. M., Rebrikov, D. V., and Danilenko, V. N. (2020). The bacterial neurometabolic signature of the gut microbiota of young children with autism spectrum disorders. J. Med. Microbiol. 69, 558–571. doi: 10.1099/jmm.0.001178
Beaudet, A. L. (2017). Brain carnitine deficiency causes nonsyndromic autism with an extreme male bias: a hypothesis. Bioessays 39:1700012. doi: 10.1002/bies.201700012
Benjamini, Y., and Hochberg, Y. (1995). Controlling the False Discovery Rate: a Practical and Powerful Approach to Multiple Testing. J. R. Stat. Soc. Ser. B 57, 289–300. doi: 10.1111/j.2517-6161.1995.tb02031.x
Berding, K., and Donovan, S. M. (2020). Dietary Patterns Impact Temporal Dynamics of Fecal Microbiota Composition in Children With Autism Spectrum Disorder. Front. Nutr. 6:193. doi: 10.3389/fnut.2019.00193
Blum, S., Milman, U., Shapira, C., and Levy, A. P. (2008). Pharmacogenomic application of the haptoglobin genotype in the prevention of diabetic cardiovascular disease. Pharmacogenomics 9, 989–991. doi: 10.2217/14622416.9.8.989
Borre, Y. E., O’Keeffe, G. W., Clarke, G., Stanton, C., Dinan, T. G., and Cryan, J. F. (2014). Microbiota and neurodevelopmental windows: implications for brain disorders. Trends Mol. Med. 20, 509–518. doi: 10.1016/j.molmed.2014.05.002
Brameyer, S., Hoyer, E., Bibinger, S., Burdack, K., Lassak, J., and Jung, K. (2020). Molecular Design of a Signaling System Influences Noise in Protein Abundance under Acid Stress in Different Gammaproteobacteria. J. Bacteriol. 202, e00121–20. doi: 10.1128/JB.00121-20
Bresnahan, M., Hornig, M., Schultz, A. F., Gunnes, N., Hirtz, D., Lie, K. K., et al. (2015). Association of maternal report of infant and toddler gastrointestinal symptoms with autism: evidence from a prospective birth cohort. JAMA Psychiatry 72, 466–474. doi: 10.1001/jamapsychiatry.2014.3034
Buffington, S. A., Dooling, S. W., Sgritta, M., Noecker, C., Murillo, O. D., Felice, D. F., et al. (2021). Dissecting the contribution of host genetics and the microbiome in complex behaviors. Cell 184, 1740–1756.e16. doi: 10.1016/j.cell.2021.02.009
Buie, T., Campbell, D. B., Fuchs, G. J., Furuta, G. T., Levy, J., VandeWater, J., et al. (2010). Evaluation, Diagnosis, and Treatment of Gastrointestinal Disorders in Individuals With ASDs: a Consensus Report. Pediatrics 125, S1–S18. doi: 10.1542/peds.2009-1878C
Caporaso, J. G., Kuczynski, J., Stombaugh, J., Bittinger, K., Bushman, F. D., Costello, E. K., et al. (2010). QIIME allows analysis of high-throughput community sequencing data. Nat. Methods 7, 335–336. doi: 10.1038/nmeth.f.303
Carta, A., Fucà, E., Guerrera, S., Napoli, E., Valeri, G., and Vicari, S. (2020). Characterization of Clinical Manifestations in the Co-occurring Phenotype of Attention Deficit/Hyperactivity Disorder and Autism Spectrum Disorder. Front. Psychol. 11:861. doi: 10.3389/fpsyg.2020.00861
Chaidez, V., Hansen, R. L., and Hertz-Picciotto, I. (2014). Gastrointestinal Problems in Children with Autism, Developmental Delays or Typical Development. J. Autism Dev. Disord. 44, 1117–1127. doi: 10.1007/s10803-013-1973-x
Chen, J., Bittinger, K., Charlson, E. S., Hoffmann, C., Lewis, J., Wu, G. D., et al. (2012). Associating microbiome composition with environmental covariates using generalized UniFrac distances. Bioinformatics 28, 2106–2113. doi: 10.1093/bioinformatics/bts342
Chernikova, M. A., Flores, G. D., Kilroy, E., Labus, J. S., Mayer, E. A., and Aziz-Zadeh, L. (2021). The Brain-Gut-Microbiome System: pathways and Implications for Autism Spectrum Disorder. Nutrients 13:4497. doi: 10.3390/nu13124497
Christian, L. M., Galley, J. D., Hade, E. M., Schoppe-Sullivan, S., Kamp Dush, C., and Bailey, M. T. (2015). Gut microbiome composition is associated with temperament during early childhood. Brain Behav. Immun. 45, 118–127. doi: 10.1016/j.bbi.2014.10.018
Collins, S. M., Surette, M., and Bercik, P. (2012). The interplay between the intestinal microbiota and the brain. Nat. Rev. Microbiol. 10, 735–742. doi: 10.1038/nrmicro2876
Coury, D. L., Ashwood, P., Fasano, A., Fuchs, G., Geraghty, M., Kaul, A., et al. (2012). Gastrointestinal Conditions in Children With Autism Spectrum Disorder: developing a Research Agenda. Pediatrics 130, S160–S168. doi: 10.1542/peds.2012-0900N
David, M. M., Tataru, C., Daniels, J., Schwartz, J., Keating, J., Hampton-Marcell, J., et al. (2021). Children with Autism and Their Typically Developing Siblings Differ in Amplicon Sequence Variants and Predicted Functions of Stool-Associated Microbes. mSystems 6, e00193–20. doi: 10.1128/mSystems.00193-20
Davies, C., Mishra, D., Eshraghi, R. S., Mittal, J., Sinha, R., Bulut, E., et al. (2021). Altering the gut microbiome to potentially modulate behavioral manifestations in autism spectrum disorders: a systematic review. Neurosci. Biobehav. Rev. 128, 549–557. doi: 10.1016/j.neubiorev.2021.07.001
De Angelis, M., Francavilla, R., Piccolo, M., De Giacomo, A., and Gobbetti, M. (2015). Autism spectrum disorders and intestinal microbiota. Gut Microbes 6, 207–213. doi: 10.1080/19490976.2015.1035855
De Angelis, M., Piccolo, M., Vannini, L., Siragusa, S., De Giacomo, A., Serrazzanetti, D. I., et al. (2013). Fecal Microbiota and Metabolome of Children with Autism and Pervasive Developmental Disorder Not Otherwise Specified. PLoS One 8:e76993. doi: 10.1371/journal.pone.0076993
de Theije, C. G. M., Wu, J., da Silva, S. L., Kamphuis, P. J., Garssen, J., Korte, S. M., et al. (2011). Pathways underlying the gut-to-brain connection in autism spectrum disorders as future targets for disease management. Eur. J. Pharmacol. 668, S70–S80. doi: 10.1016/j.ejphar.2011.07.013
Drossman, D. A., and Hasler, W. L. (2016). Rome IV—Functional GI Disorders: disorders of Gut-Brain Interaction. Gastroenterology 150, 1257–1261. doi: 10.1053/j.gastro.2016.03.035
Edgar, R. C. (2010). Search and clustering orders of magnitude faster than BLAST. Bioinformatics 26, 2460–2461. doi: 10.1093/bioinformatics/btq461
Esnafoglu, E., Cırrık, S., Ayyıldız, S. N., Erdil, A., Ertürk, E. Y., Daglı, A., et al. (2017). Increased Serum Zonulin Levels as an Intestinal Permeability Marker in Autistic Subjects. J. Pediatr. 188, 240–244. doi: 10.1016/j.jpeds.2017.04.004
Fasano, A. (2012). Intestinal Permeability and Its Regulation by Zonulin: diagnostic and Therapeutic Implications. Clin. Gastroenterol. Hepatol. 10, 1096–1100. doi: 10.1016/j.cgh.2012.08.012
Fasano, A., and Hill, I. (2017). Serum Zonulin, Gut Permeability, and the Pathogenesis of Autism Spectrum Disorders: cause, Effect, or an Epiphenomenon? J. Pediatr. 188, 15–17. doi: 10.1016/j.jpeds.2017.05.038
Ferraro, C. M., and Finkel, S. E. (2019). Physiological, Genetic, and Transcriptomic Analysis of Alcohol-Induced Delay of Escherichia coli Death. Appl. Environ. Microbiol. 85, e02113–18. doi: 10.1128/AEM.02113-18
Finegold, S. M., Dowd, S. E., Gontcharova, V., Liu, C., Henley, K. E., Wolcott, R. D., et al. (2010). Pyrosequencing study of fecal microflora of autistic and control children. Anaerobe 16, 444–453. doi: 10.1016/j.anaerobe.2010.06.008
Fiorentino, M., Sapone, A., Senger, S., Camhi, S. S., Kadzielski, S. M., Buie, T. M., et al. (2016). Blood-brain barrier and intestinal epithelial barrier alterations in autism spectrum disorders. Mol. Autism 7:49. doi: 10.1186/s13229-016-0110-z
Fischbach, M. A., and Segre, J. A. (2016). Signaling in Host-Associated Microbial Communities. Cell 164, 1288–1300. doi: 10.1016/j.cell.2016.02.037
Flannery, J. E., Stagaman, K., Burns, A. R., Hickey, R. J., Roos, L. E., Giuliano, R. J., et al. (2020). Gut Feelings Begin in Childhood: the Gut Metagenome Correlates with Early Environment, Caregiving, and Behavior. mBio 11, e02780–19. doi: 10.1128/mBio.02780-19
Frye, R. E., Rose, S., Chacko, J., Wynne, R., Bennuri, S. C., Slattery, J. C., et al. (2016). Modulation of mitochondrial function by the microbiome metabolite propionic acid in autism and control cell lines. Transl. Psychiatry 6:e927. doi: 10.1038/tp.2016.189
Frye, R. E., Rose, S., Slattery, J., and MacFabe, D. F. (2015). Gastrointestinal dysfunction in autism spectrum disorder: the role of the mitochondria and the enteric microbiome. Microb. Ecol. Health Dis. 26:27458. doi: 10.3402/mehd.v26.27458
Fulceri, F., Morelli, M., Santocchi, E., Cena, H., Del Bianco, T., Narzisi, A., et al. (2016). Gastrointestinal symptoms and behavioral problems in preschoolers with Autism Spectrum Disorder. Dig. Liver Dis. 48, 248–254. doi: 10.1016/j.dld.2015.11.026
Fung, T. C., Olson, C. A., and Hsiao, E. Y. (2017). Interactions between the microbiota, immune and nervous systems in health and disease. Nat. Neurosci. 20, 145–155. doi: 10.1038/nn.4476
Furness, J. B., Kunze, W. A. A., and Clerc, N. (1999). II. The intestine as a sensory organ: neural, endocrine, and immune responses. Am. J. Physiol.-Gastrointest. Liver Physiol. 277, G922–G928. doi: 10.1152/ajpgi.1999.277.5.G922
Gálvez, E. J. C., Iljazovic, A., Amend, L., Lesker, T. R., Renault, T., Thiemann, S., et al. (2020). Distinct Polysaccharide Utilization Determines Interspecies Competition between Intestinal Prevotella spp. Cell Host Microbe 28, 838–852.e6. doi: 10.1016/j.chom.2020.09.012
Gorrindo, P., Williams, K. C., Lee, E. B., Walker, L. S., McGrew, S. G., and Levitt, P. (2012). Gastrointestinal Dysfunction in Autism: parental Report, Clinical Evaluation, and Associated Factors: gastrointestinal dysfunction in autism. Autism Res. 5, 101–108. doi: 10.1002/aur.237
Gotham, K., Pickles, A., and Lord, C. (2009). Standardizing ADOS Scores for a Measure of Severity in Autism Spectrum Disorders. J. Autism Dev. Disord. 39, 693–705. doi: 10.1007/s10803-008-0674-3
Hashemi, Z., Fouhse, J., Im, H., Chan, C., and Willing, B. (2017). Dietary Pea Fiber Supplementation Improves Glycemia and Induces Changes in the Composition of Gut Microbiota, Serum Short Chain Fatty Acid Profile and Expression of Mucins in Glucose Intolerant Rats. Nutrients 9:1236. doi: 10.3390/nu9111236
Herbert, M. R. (2010). Contributions of the environment and environmentally vulnerable physiology to autism spectrum disorders. Curr. Opin. Neurol. 23, 103–110. doi: 10.1097/WCO.0b013e328336a01f
Herrmann, K. M., and Weaver, L. M. (1999). The Shikimate Pathway. Annu. Rev. Plant Physiol. Plant Mol. Biol. 50, 473–503. doi: 10.1146/annurev.arplant.50.1.473
Holingue, C., Newill, C., Lee, L.-C., Pasricha, P. J., and Daniele Fallin, M. (2018). Gastrointestinal symptoms in autism spectrum disorder: a review of the literature on ascertainment and prevalence. Autism Res. 11, 24–36. doi: 10.1002/aur.1854
Horvath, K., and Perman, J. A. (2002). Autistic disorder and gastrointestinal disease. Curr. Opin. Pediatr. 14, 583–587. doi: 10.1097/00008480-200210000-00004
Hsiao, E. Y., McBride, S. W., Hsien, S., Sharon, G., Hyde, E. R., McCue, T., et al. (2013). Microbiota Modulate Behavioral and Physiological Abnormalities Associated with Neurodevelopmental Disorders. Cell 155, 1451–1463. doi: 10.1016/j.cell.2013.11.024
Iljazovic, A., Roy, U., Gálvez, E. J. C., Lesker, T. R., Zhao, B., Gronow, A., et al. (2021). Perturbation of the gut microbiome by Prevotella spp. enhances host susceptibility to mucosal inflammation. Mucosal Immunol. 14, 113–124. doi: 10.1038/s41385-020-0296-4
Jonsson, L., Zettergren, A., Pettersson, E., Hovey, D., Anckarsäter, H., Westberg, L., et al. (2014). Association study between autistic-like traits and polymorphisms in the autism candidate regions RELN, CNTNAP2, SHANK3, and CDH9/10. Mol. Autism 5:55. doi: 10.1186/2040-2392-5-55
Kaakoush, N. O. (2020). Sutterella Species, IgA-degrading Bacteria in Ulcerative Colitis. Trends Microbiol. 28, 519–522. doi: 10.1016/j.tim.2020.02.018
Kanehisa, M., Sato, Y., and Morishima, K. (2016). BlastKOALA and GhostKOALA: KEGG Tools for Functional Characterization of Genome and Metagenome Sequences. J. Mol. Biol. 428, 726–731. doi: 10.1016/j.jmb.2015.11.006
Kang, D.-W., Adams, J. B., Gregory, A. C., Borody, T., Chittick, L., Fasano, A., et al. (2017). Microbiota Transfer Therapy alters gut ecosystem and improves gastrointestinal and autism symptoms: an open-label study. Microbiome 5:10. doi: 10.1186/s40168-016-0225-7
Kang, D.-W., Park, J. G., Ilhan, Z. E., Wallstrom, G., LaBaer, J., Adams, J. B., et al. (2013). Reduced Incidence of Prevotella and Other Fermenters in Intestinal Microflora of Autistic Children. PLoS One 8:e68322. doi: 10.1371/journal.pone.0068322
Katzka, D. A. (2014). The Complex Relationship between Eosinophilic Esophagitis and Gastroesophageal Reflux Disease. Dig. Dis. 32, 93–97. doi: 10.1159/000357080
Kelly, J. R., Minuto, C., Cryan, J. F., Clarke, G., and Dinan, T. G. (2017). Cross Talk: the Microbiota and Neurodevelopmental Disorders. Front. Neurosci. 11:490. doi: 10.3389/fnins.2017.00490
Klass, H. J., and Neale, G. (1978). Serum and faecal lysozyme in inflammatory bowel disease. Gut 19, 233–239. doi: 10.1136/gut.19.3.233
Kruglov, A. A., Grivennikov, S. I., Kuprash, D. V., Winsauer, C., Prepens, S., Seleznik, G. M., et al. (2013). Nonredundant Function of Soluble LTα 3 Produced by Innate Lymphoid Cells in Intestinal Homeostasis. Science 342, 1243–1246. doi: 10.1126/science.1243364
Lang, M., Michelotti, C., and Bardelli, E. (2015). Wais-4.: Wechsler Adult Intelligence Scale-4.: lettura dei risultati e interpretazione clinica. Cortina: Milano.
Langille, M. G. I., Zaneveld, J., Caporaso, J. G., McDonald, D., Knights, D., Reyes, J. A., et al. (2013). Predictive functional profiling of microbial communities using 16S rRNA marker gene sequences. Nat. Biotechnol. 31, 814–821. doi: 10.1038/nbt.2676
Larsen, J. M. (2017). The immune response to Prevotella bacteria in chronic inflammatory disease. Immunology 151, 363–374. doi: 10.1111/imm.12760
Leblond, C. S., Nava, C., Polge, A., Gauthier, J., Huguet, G., Lumbroso, S., et al. (2014). Meta-analysis of SHANK Mutations in Autism Spectrum Disorders: a Gradient of Severity in Cognitive Impairments. PLoS Genet. 10:e1004580. doi: 10.1371/journal.pgen.1004580
Levi Mortera, S., Vernocchi, P., Basadonne, I., Zandonà, A., Chierici, M., Durighello, M., et al. (2022). A metaproteomic-based gut microbiota profiling in children affected by autism spectrum disorders. J. Proteomics 251:104407. doi: 10.1016/j.jprot.2021.104407
Lin, G.-H., Hsieh, M.-C., and Shu, H.-Y. (2021). Role of Iron-Containing Alcohol Dehydrogenases in Acinetobacter baumannii ATCC 19606 Stress Resistance and Virulence. Int. J. Mol. Sci. 22:9921. doi: 10.3390/ijms22189921
Liu, F., Li, J., Wu, F., Zheng, H., Peng, Q., and Zhou, H. (2019). Altered composition and function of intestinal microbiota in autism spectrum disorders: a systematic review. Transl. Psychiatry 9:43. doi: 10.1038/s41398-019-0389-6
Liu, S., Li, E., Sun, Z., Fu, D., Duan, G., Jiang, M., et al. (2019). Altered gut microbiota and short chain fatty acids in Chinese children with autism spectrum disorder. Sci. Rep. 9:287. doi: 10.1038/s41598-018-36430-z
Lord, C., Rutter, M., and Le Couteur, A. (1994). Autism Diagnostic Interview-Revised: a revised version of a diagnostic interview for caregivers of individuals with possible pervasive developmental disorders. J. Autism Dev. Disord. 24, 659–685. doi: 10.1007/BF02172145
Lord, C., Rutter, M., DiLavore, P., Risi, S., Gotham, K., Bishop, S. (2012). Autism Diagnostic Observation Schedule, Second Edition (ADOS-2) Manual (Part I): Modules 1–4, eds Colombi, C., Tancredi, R., Persico, A., and Faggioli, A. (Los Angeles, CA: Western Psychological Service).
Lordan, C., Thapa, D., Ross, R. P., and Cotter, P. D. (2020). Potential for enriching next-generation health-promoting gut bacteria through prebiotics and other dietary components. Gut Microbes 11, 1–20. doi: 10.1080/19490976.2019.1613124
Lozupone, C., and Knight, R. (2005). UniFrac: a New Phylogenetic Method for Comparing Microbial Communities. Appl. Environ. Microbiol. 71, 8228–8235. doi: 10.1128/AEM.71.12.8228-8235.2005
Lyall, K., Croen, L., Daniels, J., Fallin, M. D., Ladd-Acosta, C., Lee, B. K., et al. (2017). The Changing Epidemiology of Autism Spectrum Disorders. Annu. Rev. Public Health 38, 81–102. doi: 10.1146/annurev-publhealth-031816-044318
Lynch, J. B., and Hsiao, E. Y. (2019). Microbiomes as sources of emergent host phenotypes. Science 365, 1405–1409. doi: 10.1126/science.aay0240
Ma, B., Liang, J., Dai, M., Wang, J., Luo, J., Zhang, Z., et al. (2019). Altered Gut Microbiota in Chinese Children With Autism Spectrum Disorders. Front. Cell. Infect. Microbiol. 9:40. doi: 10.3389/fcimb.2019.00040
MacFabe, D. F. (2015). Enteric short-chain fatty acids: microbial messengers of metabolism, mitochondria, and mind: implications in autism spectrum disorders. Microb. Ecol. Health Dis. 26:28177. doi: 10.3402/mehd.v26.28177
Maenner, M. J., Arneson, C. L., Levy, S. E., Kirby, R. S., Nicholas, J. S., and Durkin, M. S. (2012). Brief Report: association Between Behavioral Features and Gastrointestinal Problems Among Children with Autism Spectrum Disorder. J. Autism Dev. Disord. 42, 1520–1525. doi: 10.1007/s10803-011-1379-6
Maenner, M. J., Shaw, K. A., Baio, J., Washington, A., and Patrick, M. (2020). Prevalence of Autism Spectrum Disorder Among Children Aged 8 Years — Autism and Developmental Disabilities Monitoring Network, 11 Sites, United States, 2016. MMWR Surveill. Summ. 69, 1–12. doi: 10.15585/mmwr.ss6904a1
Mantis, N. J., Rol, N., and Corthésy, B. (2011). Secretory IgA’s complex roles in immunity and mucosal homeostasis in the gut. Mucosal Immunol. 4, 603–611. doi: 10.1038/mi.2011.41
Marler, S., Ferguson, B. J., Lee, E. B., Peters, B., Williams, K. C., McDonnell, E., et al. (2017). Association of Rigid-Compulsive Behavior with Functional Constipation in Autism Spectrum Disorder. J. Autism Dev. Disord. 47, 1673–1681. doi: 10.1007/s10803-017-3084-6
Mayer, E. A., Tillisch, K., and Gupta, A. (2015). Gut/brain axis and the microbiota. J. Clin. Invest. 125, 926–938. doi: 10.1172/JCI76304
Mazurek, M. O., Vasa, R. A., Kalb, L. G., Kanne, S. M., Rosenberg, D., Keefer, A., et al. (2013). Anxiety, sensory over-responsivity, and gastrointestinal problems in children with autism spectrum disorders. J. Abnorm. Child Psychol. 41, 165–176. doi: 10.1007/s10802-012-9668-x
Medina, M., De Palma, G., Ribes-Koninckx, C., Calabuig, M., and Sanz, Y. (2008). Bifidobacterium strains suppress in vitro the pro-inflammatory milieu triggered by the large intestinal microbiota of coeliac patients. J. Inflamm. 5:19. doi: 10.1186/1476-9255-5-19
Morais, L. H., Schreiber, H. L., and Mazmanian, S. K. (2021). The gut microbiota–brain axis in behaviour and brain disorders. Nat. Rev. Microbiol. 19, 241–255. doi: 10.1038/s41579-020-00460-0
Nagaraju, K., Sudeep, K. S., and Kurhekar, M. P. (2019). A cellular automaton model to find the risk of developing autism through gut-mediated effects. Comput. Biol. Med. 110, 207–217. doi: 10.1016/j.compbiomed.2019.05.015
Needham, B. D., Adame, M. D., Serena, G., Rose, D. R., Preston, G. M., Conrad, M. C., et al. (2021). Plasma and Fecal Metabolite Profiles in Autism Spectrum Disorder. Biol. Psychiatry 89, 451–462. doi: 10.1016/j.biopsych.2020.09.025
Needham, B. D., Tang, W., and Wu, W.-L. (2018a). Searching for the gut microbial contributing factors to social behavior in rodent models of autism spectrum disorder. Dev. Neurobiol. 78, 474–499. doi: 10.1002/dneu.22581
Needham, B. D., Tang, W., and Wu, W.-L. (2018b). Searching for the gut microbial contributing factors to social behavior in rodent models of autism spectrum disorder: gut Microbiota and Social Behavior. Dev. Neurobiol. 78, 474–499.
Nikolov, R. N., Bearss, K. E., Lettinga, J., Erickson, C., Rodowski, M., Aman, M. G., et al. (2009). Gastrointestinal Symptoms in a Sample of Children with Pervasive Developmental Disorders. J. Autism Dev. Disord. 39, 405–413. doi: 10.1007/s10803-008-0637-8
Özyurt, G., Öztürk, Y., Appak, Y. Ç, Arslan, F. D., Baran, M., Karakoyun, I., et al. (2018). Increased zonulin is associated with hyperactivity and social dysfunctions in children with attention deficit hyperactivity disorder. Compr. Psychiatry 87, 138–142. doi: 10.1016/j.comppsych.2018.10.006
Parracho, H. M., Bingham, M. O., Gibson, G. R., and McCartney, A. L. (2005). Differences between the gut microflora of children with autistic spectrum disorders and that of healthy children. J. Med. Microbiol. 54, 987–991. doi: 10.1099/jmm.0.46101-0
Paulson, J. N., Stine, O. C., Bravo, H. C., and Pop, M. (2013). Differential abundance analysis for microbial marker-gene surveys. Nat. Methods 10, 1200–1202. doi: 10.1038/nmeth.2658
Peters, B., Williams, K. C., Gorrindo, P., Rosenberg, D., Lee, E. B., Levitt, P., et al. (2014). Rigid–Compulsive Behaviors are Associated with Mixed Bowel Symptoms in Autism Spectrum Disorder. J. Autism Dev. Disord. 44, 1425–1432. doi: 10.1007/s10803-013-2009-2
Pulikkan, J., Mazumder, A., and Grace, T. (2019). Role of the Gut Microbiome in Autism Spectrum Disorders. Adv. Exp. Med. Biol. 1118, 253–269. doi: 10.1007/978-3-030-05542-4_13
Putignani, L., and Dallapiccola, B. (2016). Foodomics as part of the host-microbiota-exposome interplay. J. Proteomics 147, 3–20.
Putignani, L., Gasbarrini, A., and Dallapiccola, B. (2019). Potential of multiomics technology in precision medicine. Curr. Opin. Gastroenterol. 35, 491–498. doi: 10.1097/MOG.0000000000000589
Quagliariello, A., Del Chierico, F., Russo, A., Reddel, S., Conte, G., Lopetuso, L. R., et al. (2018). Gut Microbiota Profiling and Gut-Brain Crosstalk in Children Affected by Pediatric Acute-Onset Neuropsychiatric Syndrome and Pediatric Autoimmune Neuropsychiatric Disorders Associated With Streptococcal Infections. Front. Microbiol. 9:675. doi: 10.3389/fmicb.2018.00675
Raz, R., Roberts, A. L., Lyall, K., Hart, J. E., Just, A. C., Laden, F., et al. (2015). Autism Spectrum Disorder and Particulate Matter Air Pollution before, during, and after Pregnancy: a Nested Case–Control Analysis within the Nurses’ Health Study II Cohort. Environ. Health Perspect. 123, 264–270. doi: 10.1289/ehp.1408133
Rescorla, L. A. (2005). Assessment of young children using the Achenbach System of Empirically Based Assessment (ASEBA). Ment. Retard. Dev. Disabil. Res. Rev. 11, 226–237. doi: 10.1002/mrdd.20071
Ristori, M. V., Quagliariello, A., Reddel, S., Ianiro, G., Vicari, S., Gasbarrini, A., et al. (2019). Autism, Gastrointestinal Symptoms and Modulation of Gut Microbiota by Nutritional Interventions. Nutrients 11:2812.
Rock, C. O., and Jackowski, S. (1985). Pathways for the incorporation of exogenous fatty acids into phosphatidylethanolamine in Escherichia coli. J. Biol. Chem. 260, 12720–12724. doi: 10.1016/S0021-9258(17)38934-2
Roid, G. H., Miller, L. J., Pomplun, M., and Koch, C. (2013). Leiter International Performance Scale, Third Edition (Leiter-3); Italian edn. Los Angeles: Western Psychological Services.
Rose, D. R., Yang, H., Serena, G., Sturgeon, C., Ma, B., Careaga, M., et al. (2018). Differential immune responses and microbiota profiles in children with autism spectrum disorders and co-morbid gastrointestinal symptoms. Brain Behav. Immun. 70, 354–368. doi: 10.1016/j.bbi.2018.03.025
Rose, S., Bennuri, S. C., Murray, K. F., Buie, T., Winter, H., and Frye, R. E. (2017). Mitochondrial dysfunction in the gastrointestinal mucosa of children with autism: a blinded case-control study. PLoS One 12:e0186377. doi: 10.1371/journal.pone.0186377
Roussin, L., Prince, N., Perez-Pardo, P., Kraneveld, A. D., Rabot, S., and Naudon, L. (2020). Role of the Gut Microbiota in the Pathophysiology of Autism Spectrum Disorder: clinical and Preclinical Evidence. Microorganisms 8:1369. doi: 10.3390/microorganisms8091369
Sakai, K., Oshima, K., and Moriguchi, M. (1991). Production and characterization of N-acyl-D-glutamate amidohydrolase from Pseudomonas sp. strain 5f-1. Appl. Environ. Microbiol. 57, 2540–2543. doi: 10.1128/aem.57.9.2540-2543.1991
Salazar, N., Gueimonde, M., Hernández-Barranco, A. M., Ruas-Madiedo, P., and de los Reyes-Gavilán, C. G. (2008). Exopolysaccharides produced by intestinal Bifidobacterium strains act as fermentable substrates for human intestinal bacteria. Appl. Environ. Microbiol. 74, 4737–4745. doi: 10.1128/AEM.00325-08
Sannio Fancello, G., Luiz, D., and Cianchetti, C. (2007). ). GMDS-ER: Griffiths mental development scales, extended revised: 2-8 anni: manuale di somministrazione. Firenze, Giunti O.S: Organizzazioni speciali.
Sarkar, A., Lehto, S. M., Harty, S., Dinan, T. G., Cryan, J. F., and Burnet, P. W. J. (2016). Psychobiotics and the Manipulation of Bacteria-Gut-Brain Signals. Trends Neurosci. 39, 763–781. doi: 10.1016/j.tins.2016.09.002
Seethaler, B., Basrai, M., Neyrinck, A. M., Nazare, J.-A., Walter, J., Delzenne, N. M., et al. (2021). Biomarkers for assessment of intestinal permeability in clinical practice. Am. J. Physiol.-Gastrointest. Liver Physiol. 321, G11–G17. doi: 10.1152/ajpgi.00113.2021
Segata, N., Izard, J., Waldron, L., Gevers, D., Miropolsky, L., Garrett, W. S., et al. (2011). Metagenomic biomarker discovery and explanation. Genome Biol. 12:R60. doi: 10.1186/gb-2011-12-6-r60
Sharon, G., Sampson, T. R., Geschwind, D. H., and Mazmanian, S. K. (2016). The Central Nervous System and the Gut Microbiome. Cell 167, 915–932. doi: 10.1016/j.cell.2016.10.027
Sherwin, E., Bordenstein, S. R., Quinn, J. L., Dinan, T. G., and Cryan, J. F. (2019). Microbiota and the social brain. Science 366:eaar2016. doi: 10.1126/science.aar2016
Shin, N.-R., Whon, T. W., and Bae, J.-W. (2015). Proteobacteria: microbial signature of dysbiosis in gut microbiota. Trends Biotechnol. 33, 496–503. doi: 10.1016/j.tibtech.2015.06.011
Srikantha, P., and Mohajeri, M. H. (2019). The Possible Role of the Microbiota-Gut-Brain-Axis in Autism Spectrum Disorder. IJMS 20:2115. doi: 10.3390/ijms20092115
Stolp, H. B., Johansson, P. A., Habgood, M. D., Dziegielewska, K. M., Saunders, N. R., and Ek, C. J. (2011). Effects of Neonatal Systemic Inflammation on Blood-Brain Barrier Permeability and Behaviour in Juvenile and Adult Rats. Cardiovasc. Psychiatry Neurol. 2011, 1–10. doi: 10.1155/2011/469046
Sturgeon, C., and Fasano, A. (2016). Zonulin, a regulator of epithelial and endothelial barrier functions, and its involvement in chronic inflammatory diseases. Tissue Barriers 4:e1251384. doi: 10.1080/21688370.2016.1251384
Tamana, S. K., Tun, H. M., Konya, T., Chari, R. S., Field, C. J., Guttman, D. S., et al. (2021). Bacteroides-dominant gut microbiome of late infancy is associated with enhanced neurodevelopment. Gut Microbes 13, 1–17. doi: 10.1080/19490976.2021.1930875
Tamanai-Shacoori, Z., Smida, I., Bousarghin, L., Loreal, O., Meuric, V., Fong, S. B., et al. (2017). Roseburia spp.: a marker of health? Future Microbiol. 12, 157–170. doi: 10.2217/fmb-2016-0130
Tomova, A., Husarova, V., Lakatosova, S., Bakos, J., Vlkova, B., Babinska, K., et al. (2015). Gastrointestinal microbiota in children with autism in Slovakia. Physiol. Behav. 138, 179–187. doi: 10.1016/j.physbeh.2014.10.033
Tripathi, A., Lammers, K. M., Goldblum, S., Shea-Donohue, T., Netzel-Arnett, S., Buzza, M. S., et al. (2009). Identification of human zonulin, a physiological modulator of tight junctions, as prehaptoglobin-2. Proc. Natl. Acad. Sci. U. S. A. 106, 16799–16804. doi: 10.1073/pnas.0906773106
Valicenti-McDermott, M., McVicar, K., Rapin, I., Wershil, B. K., Cohen, H., and Shinnar, S. (2006). Frequency of Gastrointestinal Symptoms in Children with Autistic Spectrum Disorders and Association with Family History of Autoimmune Disease. J. Dev. Behav. Pediatr. 27, S128–S136. doi: 10.1097/00004703-200604002-00011
Veer, A. V. D. S., Brouwer, J., Biemond, I., Bohbouth, G. E., Verspaget, H. W., and Lamers, C. B. H. W. (1998). Fecal lysozyme in assessment of disease activity in inflammatory bowel disease. Dig. Dis. Sci. 43, 590–595. doi: 10.1023/A:1018823426917
Vuong, H. E., and Hsiao, E. Y. (2017). Emerging Roles for the Gut Microbiome in Autism Spectrum Disorder. Biol. Psychiatry 81, 411–423. doi: 10.1016/j.biopsych.2016.08.024
Wang, L. W., Tancredi, D. J., and Thomas, D. W. (2011). The Prevalence of Gastrointestinal Problems in Children Across the United States With Autism Spectrum Disorders From Families With Multiple Affected Members. J. Dev. Behav. Pediatr. 32, 351–360. doi: 10.1097/DBP.0b013e31821bd06a
Wasilewska, J., and Klukowski, M. (2015). Gastrointestinal symptoms and autism spectrum disorder: links and risks – a possible new overlap syndrome. Pediatr. Health Med. Ther. 6, 153–166. doi: 10.2147/PHMT.S85717
Wilkinson, B., Grepo, N., Thompson, B. L., Kim, J., Wang, K., Evgrafov, O. V., et al. (2015). The autism-associated gene chromodomain helicase DNA-binding protein 8 (CHD8) regulates noncoding RNAs and autism-related genes. Transl. Psychiatry 5:e568. doi: 10.1038/tp.2015.62
Williams, B. L., Hornig, M., Buie, T., Bauman, M. L., Cho Paik, M., Wick, I., et al. (2011). Impaired Carbohydrate Digestion and Transport and Mucosal Dysbiosis in the Intestines of Children with Autism and Gastrointestinal Disturbances. PLoS One 6:e24585. doi: 10.1371/journal.pone.0024585
Williams, B. L., Hornig, M., Parekh, T., and Lipkin, W. I. (2012). Application of novel PCR-based methods for detection, quantitation, and phylogenetic characterization of Sutterella species in intestinal biopsy samples from children with autism and gastrointestinal disturbances. mBio 3, e00261–11. doi: 10.1128/mBio.00261-11
Williams, B., Grant, L., Gidley, M., and Mikkelsen, D. (2017). Gut Fermentation of Dietary Fibres: physico-Chemistry of Plant Cell Walls and Implications for Health. IJMS 18:2203. doi: 10.3390/ijms18102203
Won, H., Lee, H.-R., Gee, H. Y., Mah, W., Kim, J.-I., Lee, J., et al. (2012). Autistic-like social behaviour in Shank2-mutant mice improved by restoring NMDA receptor function. Nature 486, 261–265. doi: 10.1038/nature11208
Yang, P., and Chang, C.-L. (2014). Glutamate-mediated signaling and autism spectrum disorders: emerging treatment targets. Curr. Pharm. Des. 20, 5186–5193. doi: 10.2174/1381612819666140110120725
Yap, C. X., Henders, A. K., Alvares, G. A., Wood, D. L. A., Krause, L., Tyson, G. W., et al. (2021). Autism-related dietary preferences mediate autism-gut microbiome associations. Cell 184, 5916–5931.e17. doi: 10.1016/j.cell.2021.10.015
Keywords: autism spectrum disorders, gastrointestinal symptoms, gut microbiota ecology, fecal secretory IgA, zonulin, lysozyme, microbial and KEGG biomarkers
Citation: Vernocchi P, Ristori MV, Guerrera S, Guarrasi V, Conte F, Russo A, Lupi E, Albitar-Nehme S, Gardini S, Paci P, Ianiro G, Vicari S, Gasbarrini A and Putignani L (2022) Gut Microbiota Ecology and Inferred Functions in Children With ASD Compared to Neurotypical Subjects. Front. Microbiol. 13:871086. doi: 10.3389/fmicb.2022.871086
Received: 07 February 2022; Accepted: 19 April 2022;
Published: 09 June 2022.
Edited by:
Zongxin Ling, Zhejiang University, ChinaReviewed by:
Huajun Zheng, Shanghai Institute for Biomedical and Pharmaceutical Technologies, ChinaSilvia Turroni, University of Bologna, Italy
Copyright © 2022 Vernocchi, Ristori, Guerrera, Guarrasi, Conte, Russo, Lupi, Albitar-Nehme, Gardini, Paci, Ianiro, Vicari, Gasbarrini and Putignani. This is an open-access article distributed under the terms of the Creative Commons Attribution License (CC BY). The use, distribution or reproduction in other forums is permitted, provided the original author(s) and the copyright owner(s) are credited and that the original publication in this journal is cited, in accordance with accepted academic practice. No use, distribution or reproduction is permitted which does not comply with these terms.
*Correspondence: Lorenza Putignani, bG9yZW56YS5wdXRpZ25hbmlAb3BiZy5uZXQ=
†These authors have contributed equally to this work and share first authorship