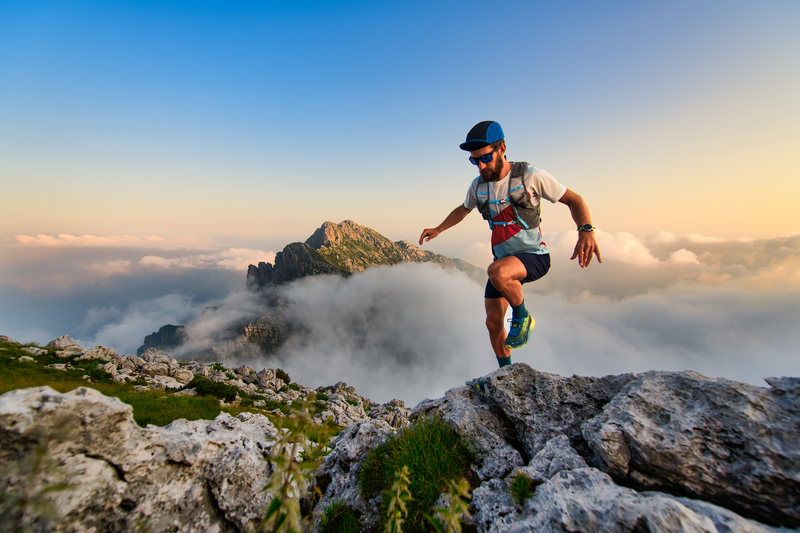
94% of researchers rate our articles as excellent or good
Learn more about the work of our research integrity team to safeguard the quality of each article we publish.
Find out more
ORIGINAL RESEARCH article
Front. Microbiol. , 28 March 2022
Sec. Microbial Symbioses
Volume 13 - 2022 | https://doi.org/10.3389/fmicb.2022.871017
Microbiomes on surfaces in kindergartens, the intermediate transfer medium for microbial exchange, can exert significant impact on the hygiene and wellbeing of young children, both individually and as a community. Here employing 2bRAD-M, a novel species-resolved metagenomics approach for low-biomass microbiomes, we surveyed over 100 samples from seven frequently contacted surfaces by children, plus individual children’s palms, in two kindergartens. Microbiome compositions, although kindergarten-specific, were grouped closely based on the type of surface within each kindergarten. Extensive microbial admixture were found among the various sampled sites, likely facilitated by contact with children’s hands. Notably, bacterial species with potential human health concerns and potentially antibiotic-resistant, although found across all sampled locations, were predominantly enriched on children’s hands instead of on the environmental sites. This first species-resolved kindergarten microbiome survey underscores the importance of good hand hygiene practices in kindergartens and provides insights into better managing hygiene levels and minimizing spread of harmful microbes in susceptible indoor environments.
Modern human beings spend most of their life time indoors. They are surrounded by indoor microbiomes, which can exert significant impact on their short-term and long-term health. For example, young children typically spend up to 8 h daily at the kindergarten. Aggravated by the high children density and the lack of proper hygiene habits in children, the kindergarten environment can be exposed to a sizeable diversity of pathogenic microorganisms (Bartlett et al., 1985; Lee et al., 2007), and thus become perfect hotspots for disease transmission (Venczel et al., 2001; Lu et al., 2004; Marziano et al., 2018). In fact, young children prevail as the most vulnerable group to infectious agents, as evidenced by their higher infection, hospital admission, and mortality rates than other age groups (Li-Kim-Moy et al., 2017; Dong et al., 2020). Therefore, knowledge of the kindergarten microbiome can provide a better understanding toward microbial hotspots, which can guide optimal crowd hygiene behavior and infection control practices for better protecting children’s health.
The prevalence of common microorganisms in the kindergarten setting has been well documented (Liu et al., 2000; Ibfelt, 2015; Ibfelt et al., 2015). However, past studies often employed culture-based methods or molecular techniques, such as quantitative polymerase chain reaction which targets only a limited number of microorganisms, thereby are incapable of detecting all potential microbes (Chiu and Miller, 2019). In addition, 16S rRNA gene amplicon sequencing used to survey surface contamination in childcare facilities lacks the required taxonomic resolution to accurately characterize microbes at the species level (Lee et al., 2007; Shin et al., 2015). In view of the above limitations, metagenomic next-generation sequencing approaches are attractive alternatives as they enable comprehensive and unbiased taxonomic characterization of the microbiome at species-level resolution (Chiu and Miller, 2019; Gu et al., 2019).
Unlike human microbiomes, samples collected from the built environment are often of low biomass and can be heavily degraded, thus applying metagenomic sequencing techniques on such samples has been difficult (Horve et al., 2020). To tackle such challenges, we have recently established a new metagenome reduction sequencing approach called 2bRAD-M and showed that it is particularly advantageous for profiling low-biomass, degraded or contaminated microbiomes at the “species” resolution (Sun et al., 2022). Therefore, employing 2bRAD-M and using kindergarten as a model for indoor environment, here we profiled the surface-associated microbiomes at the species resolution and probed possible children–environment–microbe interactions.
Samples were taken inside typical kindergartens located within the Shinan (Kindergarten 1) and the Laoshan (Kindergarten 2) residential district in Qingdao, Shandong, China. Sampling for both kindergartens was conducted on the morning and afternoon of January 3, 2020 in a single session. No conditions or restrictions in terms of the surface cleaning frequency or the children’s daily activities were imposed prior to the sampling day. A total of 99 samples from seven indoor sites were collected simultaneously, including Playroom Floor (four samples in kindergarten 1; five samples in kindergarten 2), Toilet Floor (five samples each), Color Pencil (10 samples each), Playroom Dust (five samples each), School Bag (five samples each), Picture Book (10 samples each), and Toy Surface (10 samples each). In addition, samples were taken from the palms of fifteen 3- to 6-year-old children before handwashing in kindergarten 2.
Sterile flocked swabs were moistened with sterile deionized water (0.15 M NaCl & 0.1% Tween 20) and then applied transversely and longitudinally for 20 times within an 8 cm × 8 cm area. For color pencils and toy surfaces, the swabs were wiped to cover the entire surface. For each child, the whole palm of both hands was swiped by a swab before handwashing. The collected swabs were then transferred into 2-ml Eppendorf tubes containing 500 μl sterile RNase-free buffer and stored at −80°C prior to DNA extraction. Playroom floor dust was collected by a vacuum cleaner (Panasonic, MC-WF550, 700 W) attached to a Dustream® collector (Indoor Biotechnologies, Charlottesville, United States). Dust collection was conducted for 2 min along the edge of the playroom floor for each sampling site. After sampling, the filter column was removed from the collector, sealed, and stored in Ziploc bags at −80°C prior to DNA extraction.
Genomic DNA was extracted using TIANamp Micro DNA Kit (Tiangen, Beijing, China). Carrier RNA was added to increase the yield, and extracted DNA was eluted in the 20 μl RNase-free water. After DNA extraction, 2bRAD libraries were prepared according to Sun et al. (2022). Briefly, 4 U of BcgI (NEB, United States) restriction enzyme was used to digest the genomic DNA at 37°C for 3 h. Next, ligation reaction with 0.2 μM of library-specific adaptors (Ada1, Ada2) at 4°C for 16 h was performed before heat inactivation at 65°C for 20 min for BcgI. Polymerase chain reaction (PCR) was carried out on the ligation products by including 7 μl ligated DNA, 0.1 μM primers, 0.3 mM dNTP, 1× Phusion HF buffer, and 0.4 U Phusion High Fidelity DNA polymerase (NEB, United States) at the following conditions—16–28 cycles at 98°C for 5 s, 60°C for 20 s, 72°C for 10 s, and final extension of 10 min at 72°C. The resulting libraries were purified with the QIAquick PCR purification kit (Qiagen, Valencia, CA) and sequenced on Illumina HiSeq X™ Ten platform. Library construction and sequencing were performed in OE BioTech Co., Ltd., Qingdao.
Raw sequences were processed and selected using the fastp sequence QC tool (Chen et al., 2018) with the following criteria: (i) read length of 32 bp and (ii) Phred quality score of bp >25. This yielded high-quality BcgI enzyme-digested sequence fragments of 32 bp, yielding a total of 279 million reads with an average of 2.48 million reads per sample. Taxonomic profiles were determined using the 2bRAD-M computational pipeline; GitHub: https://github.com/shihuang047/2bRAD-M (Sun et al., 2022). Briefly, reads were mapped against a pre-determined 2bRAD tag database which contains taxa-specific BcgI-derived sequences identified from 173,165 RefSeq microbial genomes. Reads coverage for each identified genome was determined and used to estimate the relative abundance of each taxon. To ensure reliable taxonomic profiling, identified taxonomic units not meeting the following criteria were discarded from downstream analysis: (i) taxonomy with taxa-specific 2bRAD-tag number < 5, and (ii) taxonomy with sequenced reads number < 15. Eventually, 495 microbial species across the 114 samples remained in our datasets for further analysis.
To check against potential cross-contamination from reagents during preparation, the ATCC® MSA-1002™ mock microbial community consisting of 20 bacteria species at even abundance of genomic DNA at ~5 ng was prepared as a positive control. Library construction & sequencing of the control were performed in conjunction with the rest of the samples. Detail taxonomic analysis revealed the detection of the 20 bacteria species at approximate equal relative abundance that is consistent with the expected profile (Supplementary Table 1). In addition, the principal coordinates analysis (PCoA) plot showed that taxonomic profile of the mock control is distinct from most of the samples (Supplementary Figure 1), suggesting minimal cross-contamination.
The R package Vegan v2.5.7 was used to calculate Shannon diversity and perform PCoA ordination (with Bray–Curtis dissimilarity as the distance measure) based on the taxonomic abundance profiles of each sample in our dataset. Difference in community structures was evaluated by PERMANOVA test conducted with 1,000 permutations. All other statistical tests were carried out with the Stats v4.0.2 and Vegan v2.5.7 R packages. SourceTracker2 (McGhee et al., 2020) version 2.0.1 was used to estimate the proportion of microbial contribution at each sampled site that comes from the other sampled sites in the same kindergarten. Identification of co-occurring samples and species was performed using SpiecEasi R package version 1.1.1 (Kurtz et al., 2015). Network graph inference was built using all species of mean relative abundance of >0.001 and with Meinshausen and Buhlmann’s neighborhood selection. All graphical plots were generated via the R packages of ggplot2, ggpubr, and ggnet2.
We started by collecting 99 indoor surface samples from seven non-host-body sites frequented by children (Playroom Floor, Toilet Floor, Playroom Dust, Color Pencil, School Bag, Toy Surface, and Picture Book) in both kindergartens and the microbiomes were sequenced (Section “Materials and Methods”). The Bacteria kingdom accounts for more than 95% of relative abundance across all samples. Fungi were mostly concentrated in the Playroom Dust and Playroom Floor, with mainly Ascomycota and Basidiomycota found (Supplementary Figure 2). At the species level, the predominant taxa shared across the sites were primarily the Gram-negative bacteria of Moraxella osloensis (Kindergarten 1: 6.1%, Kindergarten 2: 6.7%) and Acinetobacter johnsonii (Kindergarten 1: 3.9%, Kindergarten 2: 9.6%; Supplementary Figure 3), which were reported in past studies of daycare facilities (Andersson et al., 1999; Lee et al., 2007; Shin et al., 2015). However, the distinction between the two kindergartens becomes more obvious from a community standpoint, where PCoA with Bray–Curtis dissimilarity illustrates significant difference (permutational multivariate analysis of variance, PERMANOVA; R2 = 0.059, p < 0.001) in microbial compositions between the two kindergartens (Figure 1A). Compositional differences between the two kindergartens were more distinct at sites such as the Toilet Floor and Toy Surface where samples obtained from the same site in one kindergarten clustered far away from those in the other kindergarten (Supplementary Figure 1). This observation is further supported by differences in the species-level alpha diversity, where the Shannon diversity index was observed to be significantly different between the two kindergartens at the Toilet Floor (p = 0.016) and the Toy Surface (p = 0.015; Supplementary Figure 4). Conversely, Playroom Dust samples from both kindergartens were observed to cluster closely (Supplementary Figure 1), suggesting a certain level of dust microbiome homogeneity possibly due to similar levels of urbanization and environmental conditions between the districts where the two kindergartens were located (Shan et al., 2020; Park et al., 2021).
Figure 1. The species-level microbial compositional profiles of the kindergartens visualized using the principal coordinate analysis (PCoA) based on Bray–Curtis distances. (A) The PCoA together for both kindergartens revealed significantly different compositions of microbial species (PERMANOVA; R2 = 0.059, p < 0.001) between the two kindergartens. Moreover, individual PCoA was conducted separately for both (B) Kindergarten 1 and (C) Kindergarten 2, revealing significantly different composition of microbial species (kindergarten 1: PERMANOVA; R2 = 0.467, p < 0.001, kindergarten 2: PERMANOVA; R2 = 0.366, p < 0.001) between samples collected from the floor (Playroom Dust, Playroom Floor, and Toilet Floor), and those collected from hand-touched objects (Picture Book, School Bag, Toy Surface, and Color Pencil). Each point corresponds to a sample and is colored according to the kindergarten or sampling site. Ellipses correspond to 95% confidence regions.
Besides the differences between kindergartens, the microbial profiles within each kindergarten were also observed to group closely based on the type of surface where the samples were collected from. In both kindergartens, samples collected from the floor (Playroom Dust, Toilet Floor, and Playroom Floor) were observed to form a distinctive cluster that is separate from samples collected from those hand-touched objects (School Bag, Toy Surface, Picture Book, and Color Pencil), suggesting that the microbiomes between these two types of surfaces are distinct (Figures 1B,C). Not surprisingly, dominant species found on hand-touched objects across both kindergartens were largely associated with the human microbiota (e.g., Cutibacterium acnes and Streptococcus spp.), while dominant species found on the floor (e.g., Paracoccus spp. and Pseudomonas spp.) were commonly associated with the environment (Supplementary Figure 3). In particular, within each site, samples collected from the floor were relatively conserved (i.e., they cluster together based on the originated site), while those collected from objects were more diverse. Together, these observations suggest that the microbial communities of each site are heavily shaped by interaction with the environment and by human behaviors.
Despite the among-site distinctiveness of surface microbiomes, microbial exchange between surfaces is expected to be common (Meadow et al., 2014; Stephens et al., 2019). While most microbial taxa are likely to perish over time, some can lay dormant or even survive to co-inhabit with the native microbial populations (Stephens et al., 2019). Noting that hands are the most common vehicle for the transmission of microorganisms between children’s body and their environmental surfaces (Lau et al., 2012), we sought to pinpoint the potential sources of the microbes present on the child palm as well as exchanges with the kindergarten surfaces. Although a significant difference in alpha diversity was not observed on child palm as compared to the other sampled locations except the Color Pencil (Supplementary Figure 5A), PcoA analysis revealed that the child palm samples exhibit distinctive distribution of microbial composition as compared to those collected from the floor and objects (Figure 2A). This reflects on the specific ecological niche to sustain a distinct microbial community, with dominant species identified being common skin flora such as Cutibacterium acnes (Supplementary Figure 5B). Interestingly, we also observed an abundance of species associated with the oral microbiome such as Streptococcus mitis, Streptococcus oralis, and Streptococcus sanguinis on the child palm (Supplementary Figure 5B), possibly due to the increased hand-to-mouth habits of young children such as nail biting and/or finger sucking (Tulve et al., 2002). Adults on the other hand, as shown in a previous study, have a lower relative abundance of Streptococcaceae as compared to infants and children (Song et al., 2013). Additionally, we also observed overlapping of toy surface and picture book samples with the child palm cluster, suggesting similar microbial compositions between these samples.
Figure 2. Microbial associations between sampled sites with the inclusion of child palm samples. (A) Principal coordinates analysis (PCoA) ordination plot based on Bray–Curtis distance reveals significantly different composition of microbial species (PERMANOVA; R2 = 0.410, p < 0.001) among the sampled sites of Kindergarten 2. Three distinct clusters corresponding to samples obtained from the floor (Playroom Dust, Playroom Floor, and Toilet Floor), hand-touched objects (Picture Book, School Bag, Toy Surface, and Color Pencil), and the child palm were formed. Each point corresponds to a sample and is colored according to the sampled site. Ellipses correspond to 95% confidence regions. (B) Inference of microbial interaction network based on inter-sample correlation in Kindergarten 2 using SPIEC-EASI based on the relative abundance of all bacterial species. Sample-to-sample interactions were stratified by the sampling site. Each node represents a sample with the color indicating the sampling site, and no samples were omitted from the network. (C) The relative contribution from each microbial “source” community to each child palm (“sink”) microbial community was estimated using SourceTracker.
To gain insight into the possibility of children–microbe interactions across surfaces, we infer the microbial interaction network among all the sampled surfaces based on the relative abundance of all identified species. In the network, three main co-occurrence clusters were identified, namely the Toilet Floor/Playroom Floor cluster, the Child Palm/Objects cluster, and the Playroom Dust cluster (the final cluster solely consists of dust samples collected from the playroom; Figure 2B). Interestingly, species co-occurrences (as denoted by edges) between child palm samples and samples from Toy Surface and Color Pencil were observed, suggesting the inside-kindergarten cross-transmission routes as mediated by children’s hands. In addition, the observation of interconnections between samples from Color Pencil, Picture Book, School Bag, and Toy Surface suggest admixture of bacterial species, which is possibly facilitated by contact instances with children’s hands. The disconnection between the Child Palm/Objects cluster and the other two clusters can be potentially explained by lower/shorter contact or non-contact instances of the floor surfaces with the children’s hands.
Building on this observation, we then investigated the potential sources of the microbes present on child palm as well as its cross-transmission with the kindergarten surfaces. Using the Bayesian approach (Knights et al., 2011) to estimate proportion of the given “sink” samples (child palm) that are comprised of species from a potential “source” community (i.e., a surface), we found that Toy Surface is the dominant “source” with a predicted mean contribution of 33.68% across all samples (Figure 2C), followed by Picture Book (10.81%). This result supports the intuitive notion of commonly touched objects representing the primary source of bacteria found on hands, where the two locations are common contact hotspots most likely having serving as potential intermediaries for cross-transmission to/from children’s palms.
Elucidation of the microbial communities at the species resolution offers an opportunity for a comprehensive understanding of the relative microbial hazards among the surfaces within the kindergarten setting. Such hazards include potentially antibiotic-resistant bacteria and microbes which are of potential human health concerns. For example, the World Health Organization published a list of “priority bacterial pathogens” with substantial risk of antibiotic resistance that encompass a catalogue of 12 pathogenic family/genus/species which can cause food poisoning, common diseases, and severe infections (World Health Organization, 2017). Across the sampled locations from both kindergartens, a total of eight such bacteria species were detected at the species level, with at least one species detected in 74 samples, yielding an overall detection rate of 88.1% (species with mean relative abundance of >0.001%). Among the species, Pseudomonas aeruginosa was the most prevalent with a detection rate of 98.8%, followed by Acinetobacter baumannii at 52.4% (Table 1). Although the child palm was observed to harbor five out of the eight identified bacterial species with a detection rate of >80%, the relative abundance of these species was mostly low <1% (Figure 3A). In addition, we observed wide variations in the relative abundance of those potentially antibiotic-resistant bacteria as well as the microbiome among the children palms (Figures 2C, 3A). These can be attributed to the difference in the behaviors of individual child as recent studies had demonstrated that human behaviors and daily routines have a major role in spread of bacterial contaminants and fluctuation of microbiome in the indoor environments (Wang et al., 2021; Wilkins et al., 2021). Between the surfaces, the proportion of toys detected with potentially antibiotic-resistant bacteria (averagely 41.2%) is twice as much when compared to the other surfaces (averagely 20.9%), suggesting toys tend to be overlooked, which should be addressed with frequent hygiene intervention.
Table 1. Detection rate of potentially antibiotic-resistant bacteria across all sampled sites in both kindergartens.
Figure 3. Presence of potential microbial hazards in various surfaces of kindergartens. (A) Distribution of potentially antibiotic-resistant bacteria on Child Palm (n = 15) with mean relative abundance of >0.001%. (B) Significant difference in collective relative abundance of identified microbes with potential human health concerns was observed across the seven sampled locations and child palm in Kindergarten 2(p = 4.18 × 10−4, Kruskal–Wallis). With Child Palm as the reference group, Toy Surface (p = 0.0357), Picture Book (p = 0.0080), Toilet Floor (p = 0.0107), Playroom Floor (p = 0.0146) and Playroom Dust (p = 2.58 × 10−4) showed significantly lower relative abundance of pathogens. The p values above the boxplots were derived via the Mann–Whitney U-test: n.s., not significant, *p ≤ 0.05, **p ≤ 0.01, ***p ≤ 0.001. (C) Heatmap of the identified microbes of potential human health concern across the seven different sampled sites of both kindergartens and the child palms. Only those species with mean relative abundance of >0.001% across the individual sites were included.
To identify potential microbes of human health concern, a total of 299 opportunistic pathogenic bacterial species published by the Chinese Center for Disease Control and Prevention was used as a reference (Miao et al., 2017). A total of 34 distinct potentially pathogenic bacterial species (each with mean relative abundance >0.001%) were identified across all samples with dominant genera (>90% of all identified species) being Acinetobacter, Actinomyces, Prevotella, Pseudomonas, and Streptococcus (Figure 3B). In particular, Child Palm samples were found to carry the highest number of pathogenic species, while floor samples harbor the least. Notably, these species have been observed in previous studies of childcare and daycare centers (Ibfelt et al., 2015; Nygaard and Charnock, 2018), and are mostly associated with respiratory infections (e.g., Streptococcus pneumoniae and Haemophilus influenzae), gastro-intestinal infections (e.g., Helicobacter pylori and Enterococcus faecalis), and oral health concerns (e.g., Actinomyces naeslundii, Streptococcus mutans, and Streptococcus oralis). To provide a quantitative view of the potentially pathogenic bacterial species among the surfaces, the collective pathogen abundance was calculated by the summation of the individual species’ relative abundance of the 34 identified potentially pathogenic bacterial species. Notably, a significant difference was observed (p = 4.18 × 10−4, Kruskal–Wallis) across the different sites in kindergarten 2 (Figure 3C), with Toy Surface (p = 0.0357), Picture Book (p = 0.0080), Toilet Floor (p = 0.0107), Playroom Floor (p = 0.0146) and Playroom Dust (p = 2.58 × 10−4) showing significantly lower collective pathogen abundance than child palm (collective pathogen abundance: 0.349 ± 0.201). Overall, this suggests that children’s hand can possibly harbor more potentially pathogenic bacterial species than those surfaces conventionally considered “dirty” surfaces such as Toilet Floor, and highlights those surfaces where higher standards of sanitation are needed for proper hygiene control in the kindergartens.
In contrast to prior studies that have investigated environmental surfaces in kindergartens via culture-based methods or molecular techniques, this study is the first species-resolved metagenomic characterization of microbial communities present on surfaces frequented by child activities at kindergartens. The use of 2bRAD-M, a metagenome reduction sequencing approach, allowed for the profiling of low-biomass microbiomes, revealing not just the prevalence of pathogens but also the possible microbial flow and interactions among surfaces in the kindergarten. Collectively, these results confirm and deepen the findings from several previous studies which revealed the diversity of microorganisms in the kindergarten environment, microbial contact hotspots, and prevalence of potential pathogens (Lee et al., 2007; Ibfelt, 2015; Ibfelt et al., 2015; Nygaard and Charnock, 2018).
Interestingly, despite being in neighboring districts of the same city and thus presumably subjected to similar environmental factors such as relative humidity and temperature, the two kindergartens exhibit clear differences in their microbiomes. Thus, it is probable that occupational factors such as number of occupants, length of occupant stay, and occupant behavior can have a larger influence in shaping the microbiome, supporting existing findings that the building environmental conditions often have a small influence on indoor bacterial communities (Barberán et al., 2015; Stephens, 2016).
Although our analysis infers the admixture of microbes across the kindergarten surfaces which was possibly facilitated through hand contact, the detection of microbes on kindergarten surfaces does not assert their source, nor establish that they can be further transmitted from one surface to another. Surface-to-surface transmission of microbes depends on the characteristics of the microbes and surface itself and would require in-depth complementary laboratory investigations to determine if the detected microbes are indeed deposited through human contact. Nonetheless, our findings indicate that commonly touched objects are potential hotspots which can facilitate the exchange of microbes during direct hand contact, thus increasing the children’s risk of exposure to potential microbial hazards. In particular, S. pneumoniae, which was identified in 93% of all child palms in this study, have been demonstrated to spread through direct physical contact and lead to acquisition of nasopharyngeal colonization, potentially increasing the possibility of infection (Connor et al., 2018).
It is also important to note that the detection of DNA signatures arising from bacteria with pathogenic potential in our study does not equate to a higher risk of infection or disease susceptibility as these data do not provide definitive information about their strain type, viability, or pathogenicity. For instance, the likelihood of infection largely depends on the quantity of pathogen exposure, virulence of the pathogen, and whether the pathogen is metabolically active, information which might be challenging to obtain from such sequence-based metagenomic DNA data. Likewise, 2bRAD-M does not provide information about the presence of antimicrobial resistance genes, thus the detection of potentially antibiotic-resistant pathogens only provides an indication of exposure risk. Future studies based on microbial single-cell technologies instead, such as ramanome and Raman-activated Cell Sorting and Sequencing (RACS-Seq), can potentially assess the risks regarding strain type, viability, vitality, and antibiotic resistance (both phenotype and the underlying genotype; Xu et al., 2020; Jing et al., 2021) at the level of individual bacterial cells. Moreover, it should be noted that many of the detected taxa with potential implications for human health were also opportunistic pathogens such as Actinomyces naeslundii and Streptococcus mitis, which can lead to infections in oral lesions and immune-compromised patients, respectively, but also asymptomatically colonize the human body (Mitchell, 2011; Könönen and Wade, 2015). Furthermore, the values reported in this study are relative abundances, thus it is not yet clear whether a higher relative abundance between kindergartens or sampling locations is due to an increase in a certain potentially pathogenic species, or a decrease in others.
In summary, this study was the first to adopt the 2bRAD-M technology, which overcomes the low-biomass microbiome challenge, for indoor environmental samples to establish an unbiased map of microbiomes within kindergartens and microbial transmissions among indoor sites and the human inhabitants. This enabled the inference of bacterial species admixture across the kindergarten surfaces, possibly facilitated by contact instances with children’s hands. The predominant enrichment of bacterial species with potential human health concerns and antibiotic resistance on children’s hands, instead of on the environmental sites, underscores the importance of good hand hygiene practices in kindergartens. Furthermore, the understanding that fomite surface, such as toys, carries high numbers of pathogens contamination should help to identify sites which represent a risk and facilitate risk management approach for indoor hygiene in kindergarten to minimize the spread of harmful microbes indoors. Based on these findings, large-scale studies that account for multiple locations, temporal aspects and occupancy factors should help to define a “baseline of healthy ecosystem” for kindergarten, and eventually lead to a rational strategy that formulates health-promoting symbiosis between young children and their indoor environments.
The datasets presented in this study can be found in online repositories. The names of the repository/repositories and accession number(s) can be found at: https://www.ebi.ac.uk/ena, PRJEB40975.
The studies involving human participants were reviewed and approved by The Ethics Committee of Qingdao Institute of BioEnergy and Bioprocess Technology, Chinese Academy of Sciences. Written informed consent to participate in this study was provided by the participants’ legal guardian/next of kin.
TL, JX, PZ, JL, and HZ conceived and devised the overall research goals and aims. TL, JL, PZ, and LZ planned and executed the study implementation. PZ, LZ, and YD contributed to the sampling processing and performed the laboratory experiments. TL and DC processed the experimental data and performed the analysis and interpretation of the results. TL, DC, JX, and JL wrote the manuscript in consultation with PZ, LZ, YD, and HZ. JX, JL, and TL provided the overall project supervision. All authors contributed to the article and approved the submitted version.
This work was supported by P&G-CAS iMicrobiome Collaborative Initiative, Shenzhen Province Research Fund (SGDX2019081623060946) and Shandong Energy Institute.
TL, DC, JL, and HZ are employed by company Procter & Gamble.
The remaining authors declare that the research was conducted in the absence of any commercial or financial relationships that could be construed as a potential conflict of interest.
All claims expressed in this article are solely those of the authors and do not necessarily represent those of their affiliated organizations, or those of the publisher, the editors and the reviewers. Any product that may be evaluated in this article, or claim that may be made by its manufacturer, is not guaranteed or endorsed by the publisher.
The authors would like to thank Terry Lu, Andy Wu, and Amy Yu for the critical suggestions and discussions and appreciate Xiaoli Cheng, Qianrui Ren, and Fang Yang for logistic support and sample processing. We also appreciate Zheng Sun for 2bRAD-M method execution.
The Supplementary Material for this article can be found online at: https://www.frontiersin.org/articles/10.3389/fmicb.2022.871017/full#supplementary-material
Andersson, A. M., Weiss, N., Rainey, F., and Salkinoja-Salonen, M. S. (1999). Dust-borne bacteria in animal sheds, schools and children’s day care centres. J. Appl. Microbiol. 86, 622–634. doi: 10.1046/j.1365-2672.1999.00706.x
Barberán, A., Dunn, R. R., Reich, B. J., Pacifici, K., Laber, E. B., Menninger, H. L., et al. (2015). The ecology of microscopic life in household dust. Proc. R. Soc. B Biol. Sci. 282:20151139. doi: 10.1098/rspb.2015.1139
Bartlett, A. V., Moore, M., Gary, G. W., Starko, K. M., Erben, J. J., and Meredith, B. A. (1985). Diarrheal illness among infants and toddlers in day care centers. I. Epidemiology and pathogens. J. Pediatr. 107, 495–502. doi: 10.1016/s0022-3476(85)80004-4
Chen, S., Zhou, Y., Chen, Y., and Jia, G. (2018). Fastp: an ultra-fast all-in-one FASTQ preprocessor. Bioinformatics 34, i884–i890. doi: 10.1093/bioinformatics/bty560
Chiu, C. Y., and Miller, S. A. (2019). Clinical metagenomics. Nat. Rev. Genet. 20, 341–355. doi: 10.1038/s41576-019-0113-7
Connor, V., German, E., Pojar, S., Mitsi, E., Hales, C., Nikolaou, E., et al. (2018). Hands are vehicles for transmission of Streptococcus pneumoniae in novel controlled human infection study. Eur. Respir. J. 52:1800599. doi: 10.1183/13993003.00599-2018
Dong, Y., Wang, L., Burgner, D. P., Miller, J. E., Song, Y., Ren, X., et al. (2020). Infectious diseases in children and adolescents in China: analysis of national surveillance data from 2008 to 2017. BMJ 369:m1043. doi: 10.1136/bmj.m1043
Gu, W., Miller, S., and Chiu, C. Y. (2019). Clinical metagenomic next-generation sequencing for pathogen detection. Annu. Rev. Pathol. 14, 319–338. doi: 10.1146/annurev-pathmechdis-012418-012751
Horve, P. F., Lloyd, S., Mhuireach, G. A., Dietz, L., Fretz, M., MacCrone, G., et al. (2020). Building upon current knowledge and techniques of indoor microbiology to construct the next era of theory into microorganisms, health, and the built environment. J. Expo. Sci. Environ. Epidemiol. 30, 219–235. doi: 10.1038/s41370-019-0157-y
Ibfelt, T. (2015). Effect of cleaning and disinfection of toys on infectious diseases and micro-organisms in daycare nurseries. J. Hosp. Infect. 7, 109–115. doi: 10.1016/j.jhin.2014.10.007
Ibfelt, T., Engelund, E. H., Permin, A., Madsen, J. S., Schultz, A. C., and Andersen, L. P. (2015). Presence of pathogenic bacteria and viruses in the daycare environment. J. Environ. Health 78, 24–29.
Jing, X., Gong, Y., Teng, X., Meng, Y., Han, X., Xiaolu, S., et al. (2021). One-cell metabolic phenotyping and sequencing of soil microbiome by raman-activated gravity-driven encapsulation (RAGE). mSystems 6:e0018121. doi: 10.1128/mSystems.00181-21
Knights, D., Kuczynski, J., Charlson, E. S., Zaneveld, J., Mozer, M. C., Collman, R. G., et al. (2011). “Bayesian Community-Wide Culture-Independent Microbial Source Tracking,” 5.
Könönen, E., and Wade, W. G. (2015). Actinomyces and related organisms in human infections. Clin. Microbiol. Rev. 28, 419–442. doi: 10.1128/CMR.00100-14
Kurtz, Z. D., Müller, C. L., Miraldi, E. R., Littman, D. R., Blaser, M. J., and Bonneau, R. A. (2015). Sparse and compositionally robust inference of microbial ecological networks. PLoS Comput. Biol. 11:e1004226. doi: 10.1371/journal.pcbi.1004226
Lau, C. H., Springston, E. E., Sohn, M.-W., Mason, I., Gadola, E., Damitz, M., et al. (2012). Hand hygiene instruction decreases illness-related absenteeism in elementary schools: a prospective cohort study. BMC Pediatr. 12:52. doi: 10.1186/1471-2431-12-52
Lee, L., Tin, S., and Kelley, S. T. (2007). Culture-independent analysis of bacterial diversity in a child-care facility. BMC Microbiol. 13. doi: 10.1186/1471-2180-7-27
Li-Kim-Moy, J., Yin, J. K., Blyth, C. C., Kesson, A., Booy, R., Cheng, A. C., et al. (2017). Influenza hospitalizations in Australian children. Epidemiol. Infect. 145, 1451–1460. doi: 10.1017/S0950268816003381
Liu, L. J., Krahmer, M., Fox, A., Feigley, C. E., Featherstone, A., Saraf, A., et al. (2000). Investigation of the concentration of bacteria and their cell envelope components in indoor air in two elementary schools. J. Air Waste Manage. Assoc. 50, 1957–1967. doi: 10.1080/10473289.2000.10464225
Lu, N., Samuels, M. E., Shi, L., Baker, S. L., Glover, S. H., and Sanders, J. M. (2004). Child day care risks of common infectious diseases revisited. Child Care Health Dev. 30, 361–368. doi: 10.1111/j.1365-2214.2004.00411.x
Marziano, V., Poletti, P., Béraud, G., Boëlle, P.-Y., Merler, S., and Colizza, V. (2018). Modeling the impact of changes in day-care contact patterns on the dynamics of varicella transmission in France between 1991 and 2015. PLoS Comput. Biol. 14:e1006334. doi: 10.1371/journal.pcbi.1006334
McGhee, J. J., Rawson, N., Bailey, B. A., Fernandez-Guerra, A., Sisk-Hackworth, L., and Kelley, S. T. (2020). Meta-SourceTracker: application of bayesian source tracking to shotgun metagenomics. PeerJ 8:e8783. doi: 10.7717/peerj.8783
Meadow, J. F., Altrichter, A. E., Kembel, S. W., Moriyama, M., O’Connor, T. K., Womack, A. M., et al. (2014). Bacterial communities on classroom surfaces vary with human contact. Microbiome 2:7. doi: 10.1186/2049-2618-2-7
Miao, J., Han, N., Qiang, Y., Zhang, T., Li, X., and Zhang, W. (2017). 16SPIP: a comprehensive analysis pipeline for rapid pathogen detection in clinical samples based on 16S metagenomic sequencing. BMC Bioinformatics 18:568. doi: 10.1186/s12859-017-1975-3
Mitchell, J. (2011). Streptococcus mitis: walking the line between commensalism and pathogenesis. Mol Oral Microbiol 26, 89–98. doi: 10.1111/j.2041-1014.2010.00601.x
Nygaard, A. B., and Charnock, C. (2018). Longitudinal development of the dust microbiome in a newly opened norwegian kindergarten. Microbiome 6:159. doi: 10.1186/s40168-018-0553-x
Park, J.-H., Lemons, A. R., Roseman, J., Green, B. J., and Cox-Ganser, J. M. (2021). Bacterial community assemblages in classroom floor dust of 50 public schools in a large city: characterization using 16S RRNA sequences and associations with environmental factors. Microbiome 9:15. doi: 10.1186/s40168-020-00954-2
Shan, Y., Guo, J., Fan, W., Li, H., Hui, W., Song, Y., et al. (2020). Modern urbanization has reshaped the bacterial microbiome profiles of house dust in domestic environments. World Allergy Organization J. 13:100452. doi: 10.1016/j.waojou.2020.100452
Shin, S.-K., Kim, J., Ha, S.-m., Hyun-Seok, O., Chun, J., Sohn, J., et al. (2015). Metagenomic insights into the bioaerosols in the indoor and outdoor environments of childcare facilities. PLoS One 17. doi: 10.1371/journal.pone.0126960
Song, S. J., Lauber, C., Costello, E. K., Lozupone, C. A., Humphrey, G., Donna Berg-Lyons, J., et al. (2013). Cohabiting family members share microbiota with one another and with their dogs. Elife 2:e00458. doi: 10.7554/eLife.00458
Stephens, B. (2016). What have we learned about the microbiomes of indoor environments? mSystems 1:e00083-16. doi: 10.1128/mSystems.00083-16
Stephens, B., Azimi, P., Thoemmes, M. S., Heidarinejad, M., Allen, J. G., and Gilbert, J. A. (2019). Microbial exchange via fomites and implications for human health. Curr. Pollut. Rep. 5, 198–213. doi: 10.1007/s40726-019-00123-6
Sun, Z., Huang, S., Zhu, P., Tzehau, L., Zhao, H., Lv, J., et al. (2022). Species-resolved sequencing of low-biomass or degraded microbiomes using 2bRAD-M. Genome Biol. 23:36. doi: 10.1186/s13059-021-02576-9
Tulve, N. S., Suggs, J. C., Mccurdy, T., Cohen Hubal, E. A., and Moya, J. (2002). Frequency of mouthing behavior in young children. J. Expo. Sci. Environ. Epidemiol. 12, 259–264. doi: 10.1038/sj.jea.7500225
Venczel, L. V., Desai, M. M., Vertz, P. D., England, B., Hutin, Y. J., Shapiro, C. N., et al. (2001). The role of child care in a community-wide outbreak of hepatitis A. Pediatrics 108:E78. doi: 10.1542/peds.108.5.e78
Wang, P., Zhang, N., Miao, T., Chan, J. P. T., Huang, H., Lee, P. K. H., et al. (2021). Surface touch network structure determines bacterial contamination spread on surfaces and occupant exposure. J. Hazard. Mater. 416:126137. doi: 10.1016/j.jhazmat.2021.126137
Wilkins, D., Tong, X., Leung, M. H. Y., Mason, C. E., and Lee, P. K. H. (2021). Diurnal variation in the human skin microbiome affects accuracy of forensic microbiome matching. Microbiome 9:129. doi: 10.1186/s40168-021-01082-1
World Health Organization (2017). WHO publishes list of bacteria for which new antibiotics are urgently needed. Available at: https://www.who.int/news/item/27-02-2017-who-publishes-list-of-bacteria-for-which-new-antibiotics-are-urgently-needed (Accessed March 27, 2020).
Keywords: kindergarten, environmental surfaces, microbiome, metagenomics, microbial admixture
Citation: Lam T, Chew D, Zhao H, Zhu P, Zhang L, Dai Y, Liu J and Xu J (2022) Species-Resolved Metagenomics of Kindergarten Microbiomes Reveal Microbial Admixture Within Sites and Potential Microbial Hazards. Front. Microbiol. 13:871017. doi: 10.3389/fmicb.2022.871017
Received: 07 February 2022; Accepted: 02 March 2022;
Published: 28 March 2022.
Edited by:
Zhiyong Li, Shanghai Jiao Tong University, ChinaReviewed by:
Hongwei Zhou, Southern Medical University, ChinaCopyright © 2022 Lam, Chew, Zhao, Zhu, Zhang, Dai, Liu and Xu. This is an open-access article distributed under the terms of the Creative Commons Attribution License (CC BY). The use, distribution or reproduction in other forums is permitted, provided the original author(s) and the copyright owner(s) are credited and that the original publication in this journal is cited, in accordance with accepted academic practice. No use, distribution or reproduction is permitted which does not comply with these terms.
*Correspondence: Jiquan Liu, bGl1LmouMkBwZy5jb20=; Jian Xu, eHVqaWFuQHFpYmVidC5hYy5jbg==
Disclaimer: All claims expressed in this article are solely those of the authors and do not necessarily represent those of their affiliated organizations, or those of the publisher, the editors and the reviewers. Any product that may be evaluated in this article or claim that may be made by its manufacturer is not guaranteed or endorsed by the publisher.
Research integrity at Frontiers
Learn more about the work of our research integrity team to safeguard the quality of each article we publish.