- 1Department of Human Parasitology, School of Basic Medicine Science, Hubei University of Medicine, Shiyan, China
- 2Department of Infectious Diseases, Renmin Hospital, Hubei University of Medicine, Shiyan, China
Rodent malaria caused by Plasmodium yoelii 17XL (Py 17XL) is an ideal animal model for human malaria studies. Although the gut microbiota plays an important role in the occurrence and development of infectious diseases, the gut microbiota associated with Py 17XL infection remains unclear. In the current study, the gut microbiota composition of infected BALB/c mice was surveyed. Mouse fecal samples were collected at 0, 2, 5 days post-infection (dpi), and the gut microbiota was characterized by 16S rRNA sequencing. Operational taxonomic units (OTUs) were 634 ± 26 on average. Firmicutes and Bacteroidetes were typically predominant in the gut microbiota composition at the phylum level. Compared with the Ctrl, Firmicutes was significantly decreased after infection, while Bacteroidetes was notably increased. The most dominant family was Lactobacillaceae in all samples. The alpha diversity index showed that compared with that of the Ctrl, the observed OTU number was decreased at 2 dpi and then slightly increased at 5 dpi. LEfSe analysis revealed several bacterial taxa were notably related to Py-infected mice at the phylogenetic level. Several bacterial genera, such as Lactobacillus, were overrepresented in the Py-infected fecal microbiota at 2 dpi, while Muribaculaceae was overrepresented at 5 dpi. Moreover, Alistipes and Helicobacter were overrepresented at 5 dpi compared with 2 dpi. The results indicated Py infection could alter the gut microbiota composition of mice. Besides, biomarkers could serve as direct targets to elucidate their roles in the progression and pathogenesis of malaria and provide insights into studies of antimalarial drugs and malaria vaccines.
Introduction
Malaria is an acute infectious disease that threatens the health of approximately half of the world’s population and was responsible for an estimated 241 million clinical cases and approximately 627,000 deaths in 2020 (WHO, 2021). Additionally, there were 14 million more cases in 2020 than in 2019 and 69,000 more deaths. Approximately two-thirds of these additional deaths (47,000) were linked to disruptions in the provision of malaria prevention, diagnosis and treatment during the COVID-19 pandemic (WHO, 2021). Malaria is caused by protozoan parasites of the genus Plasmodium and is the most prevalent infectious disease in the WHO African Region (De et al., 2016). China, on the other hand, was officially certified as a malaria-free country by the WHO in 2021 (Feng et al., 2021). Malaria confers a large economic burden and disease burden (Waide and Schmidt, 2020). Five main species of the genus Plasmodium can cause human malaria. Fever, chills, headache, muscle aches, and tiredness are the main symptoms of malaria patients. In addition, gastrointestinal symptoms such as vomiting, abdominal pain, and diarrhea develop in falciparum malaria patients (Prasad and Virk, 1993). Under laboratory or clinical conditions, different strains or lines of malaria parasites can cause different severities of malaria in their hosts. The rodent malaria parasite Plasmodium yoelii is a representative example of parasite lines with significantly different pathogenicity. BALB/c mice infected with the parasite Py 17XL die within 7 dpi (Nair et al., 2017). Rodent malaria caused by Py 17XL is a typical rodent model for studying the pathogenesis of human malaria (Chen et al., 2010). While there is still much to explore before gut microbiota modulation becomes an effective and optimal treatment for preventing fatal malaria, recent evidence in both human studies and rodent models has indicated the gut microbiota composition is a factor in the progression of disease.
Trillions of microbes naturally reside in the human body, especially in the gastrointestinal tract (Gill et al., 2006). The gut microbiota has many important functions in the human body, including resisting pathogens to support protection, enhancing the immune system, and contributing to digestion and metabolism (Gomaa, 2020). The disturbance of the gut microbiota community is associated with several human diseases, such as inflammatory bowel diseases (IBDs) (Nishino et al., 2018), obesity and diabetes (Karlsson et al., 2013), allergies (Bunyavanich et al., 2016), autoimmune diseases (Chu et al., 2017), and cardiovascular diseases (Astudillo and Mayrovitz, 2021). Moreover, there are a lot of methods for modulating the gut microbiota composition and functions, such as the application of probiotics and fecal microbiota transplantation. Previous studies have suggested the gut microbiota could also modulate the pathogenesis of infectious diseases, indicating that gut microbiota variability influences systemic immune responses. For instance, mice colonized with a gut pathobiont could produce antibodies that reacted with Plasmodium spp. (Yilmaz et al., 2014). Besides, gastrointestinal environments, especially changes in the gut microbiota, are related to the onset of several diseases.
Malaria infections affect the gastrointestinal tract, and alterations in the intestinal environment appear to influence malaria pathogenesis. Villarino et al. (2016) showed when different vendors’ C57BL/6 mice were infected with the non-lethal rodent-specific strain Py 17XNL, the mice presented numerous differences in infection morbidity, severity, and mortality and that these differences in susceptibility were dependent on the gut microbiota. Taniguchi et al. demonstrated that C57BL/6 mice infected with the lethal rodent-specific strain P. berghei ANKA developed experimental cerebral malaria. The alterations included intestinal pathology and gut microbiota composition, which were related to the development of ECM. Moreover, Denny et al. verified that proinflammatory cells were increased in the lamina propria and changes in cecal metabolites were observed with differing susceptibility to Py 17XNL (Denny et al., 2019). However, some Plasmodium parasites appear to have developed resistance to available antimalarials. Besides, there is no practical or long-term vaccine against malaria. The relationship between human health and disease and gut microbiota has been studied widely in recent years. Hence, gut microbiota modulation may be a potential treatment for malaria (Villarino et al., 2016).
Recent studies have reported that many various diseases can alter the gut microbiota, which led us to hypothesize that the gut microbiota may be affected by malaria infections. BALB/c mice with Py 17XL infection are a lethal malaria model that can be used to clarify the possible role of Py in influencing the gut microbiota of infected BALB/c mice. Therefore, the aims of the study included (1) analyzing the gut microbiota composition obtained from the feces of Py 17XL-infected and -uninfected mice separately by targeting the V4 region of the 16S rRNA through the Illumina HiSeq2500 platform and (2) obtaining biomarkers to offer insight into malaria pathogenesis and antimalarial drugs.
Materials and Methods
Mice and Infection With Plasmodium yoelii 17XL
Six- and eight-week-old female BALB/c mice (20-25 g weight) were purchased from HNSJA Co., Ltd., Changsha, China, and maintained under specific pathogen-free conditions. The housing and feeding conditions were maintained as per recommended standards (25 ± 3°C). The mice were fed an irradiated diet and pure water. All the mice were allowed to acclimate for one week before starting the experiment.
Donor female BALB/c mice were intraperitoneally infected with Py 17XL by infected red blood cells (iRBCs) prepared from thawed blood. Fresh blood was collected from donor mice at 4 dpi, and the experimental BALB/c mice were intraperitoneally infected with 1 × 106 iRBCs (in 100 μl saline) prepared from the donor mice. Tail blood was collected for thin smears and stained with Giemsa dye at 2 and 5 dpi, respectively. Percent parasitemia assessed as percent iRBCs per total RBCs was evaluated through Giemsa-stained thin blood smears, and parasites versus whole blood cells were counted at 1,000 × magnification under a light microscope.
Sample Collection
The sixteen experimental mice were placed in the cage individually. Fecal pellets were collected at three time points as follows: before infection with Py 17XL (Ctrl), at 2 days post-infection (PyD2), and at 5 days post-infection (PyD5). Mice feces were placed in a sterile centrifuge tube using a sterile tweezer, immediately frozen in liquid nitrogen and stored at −80°C until use.
DNA Extraction, PCR Amplification, and Sequencing
DNA was extracted from the fecal samples using the CTAB or SDS method. PCR amplification and sequencing were performed using the microbial 16S rRNA primers (16S V4: 515F-806R, 392 bp) as well as Phusion® High-Fidelity PCR Master Mix (New England Biolabs). The PCR product mixture was purified using a Qiagen Gel Extraction Kit (Qiagen, Germany).
Sequencing libraries were generated using a TruSeq® DNA PCR-Free Sample Preparation Kit (Illumina, United States). The library quality was evaluated on the Qubit@ 2.0 Fluorometer (Thermo Scientific) and Agilent Bioanalyzer 2100 system. Finally, the library was sequenced on an Illumina HiSeq2500 platform. The 16S rRNA amplicons sequenced in this study were uploaded into the Sequence Read Archive (SRA) in NCBI and registered with the BioProject database (BioProject identification number PRJNA798515).
Data Processing and Statistical Analysis
Data processing and statistical analysis methods followed (Guan et al., 2021). Paired-end reads were merged using FLASH V1.2.7 (Magoč and Salzberg, 2011). Sequence analysis was performed using QIIME (V1.7.0) (Caporaso et al., 2010). The chimera sequences were determined using the UCHIME algorithm (Edgar et al., 2011) and were removed (Haas et al., 2011) to obtain the effective tags. The operational taxonomic units (OTUs) were determined by using Uparse software (Uparse v7.0.1001) (Edgar, 2013) and assigned by sequences with ≥ 97% similarity. Representative sequences for each OTU were screened for further annotation. For each representative sequence, the GreenGene Database (DeSantis et al., 2006) was used based on the RDP 3 classifier (Version 2.2) (Wang et al., 2007) algorithm to annotate the taxonomic information. To study the phylogenetic relationships of the different OTUs and the differences among the dominant species in different groups, multiple sequence alignment was conducted using MUSCLE software (Version 3.8.31) (Edgar, 2004).
Operational taxonomic units abundance information was normalized using a standard sequence number corresponding to the sample with the fewest sequences. Statistical analysis was performed by alpha diversity and beta diversity. The alpha diversity was applied to analyze the complexity of the species diversity for a sample based on six indices: Chao1, ACE, Observed-species, Shannon, Simpson, and Goods-coverage. All of these indices in our samples were calculated with QIIME (Version 1.7.0) and displayed with R software (Version 2.15.3).
In contrast, the beta diversity was evaluated by determining the similarity among the microbial communities. Both weighted and unweighted UniFrac calculations were performed by using QIIME software (Version 1.7.0). Principal coordinate analysis (PCoA) was performed to obtain the principal coordinates and visualize the complex, multidimensional data. The WGCNA package, stat packages, and ggplot2 package in R software (Version 2.15.3) were applied for PCoA analysis. The unweighted pair-group method with arithmetic means (UPGMA) clustering and LEfSe were performed to obtain differentially represented microbial taxa at different taxonomic levels.
Results
General Information
On day 0, normal mice (Figure 1A) were infected with Py 17XL parasites. At 2 and 5 dpi, the parasites were observed in thin blood smears using microscopy, representing for successful infection (Figures 1B,C). The parasitemia of the infected mice at 5 dpi was higher than that of the infected mice at 2 dpi (Figure 1D). The feces were collected on day 0, day 2, and day 5 after infection.
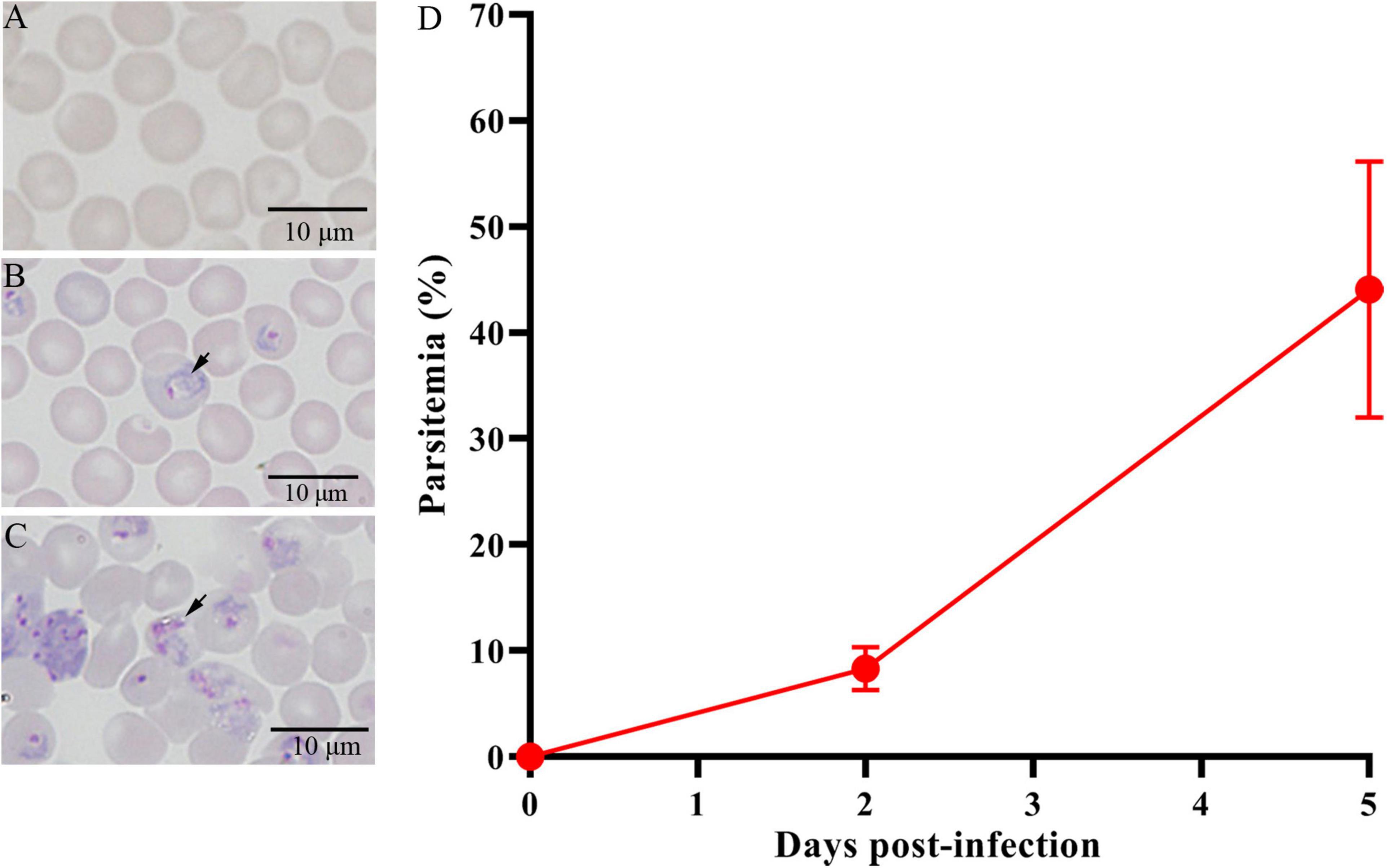
Figure 1. Giemsa’s stain to determine the parasitemia of Py 17XL in BALB/c mice. (A) The control mice (Ctrl) without parasite infection. (B) The PyD2 group with successful parasite infection at 2 dpi. (C) The PyD5 group with successful parasite infection at 5 dpi. (D) Percent parasitemia was evaluated on the corresponding days. Data are cumulative results (n = 16 mice per group) from two experiments.
Sequencing and Operational Taxonomic Units Clustering
A total of 48 mouse fecal samples were collected and sent for 16S RNA sequencing. In total, 3,805,568 raw sequences were generated from all the samples, and the sequence number varied from 70,216 to 88,910. The mean number of sequences was 79,283 ± 5,941 (standard deviation, SD) per sample. After a series of experiments, a total of 3,428,267 clean sequences remained, which ranged from 62,444 to 82,917 sequences per sample, with an average of 71,422 ± 5,514 (Table 1). The GC content in all the samples ranged from 51.78% to 53.73%, and the average was 52.68%. The high-quality reads were clustered using ≥ 97% sequence similarity into a total of 30,428 microbiota OTUs, ranging from 538 to 686. Each sample had 634 OTUs, 594 observed species, and 71,422 sequences on average (Table 1).
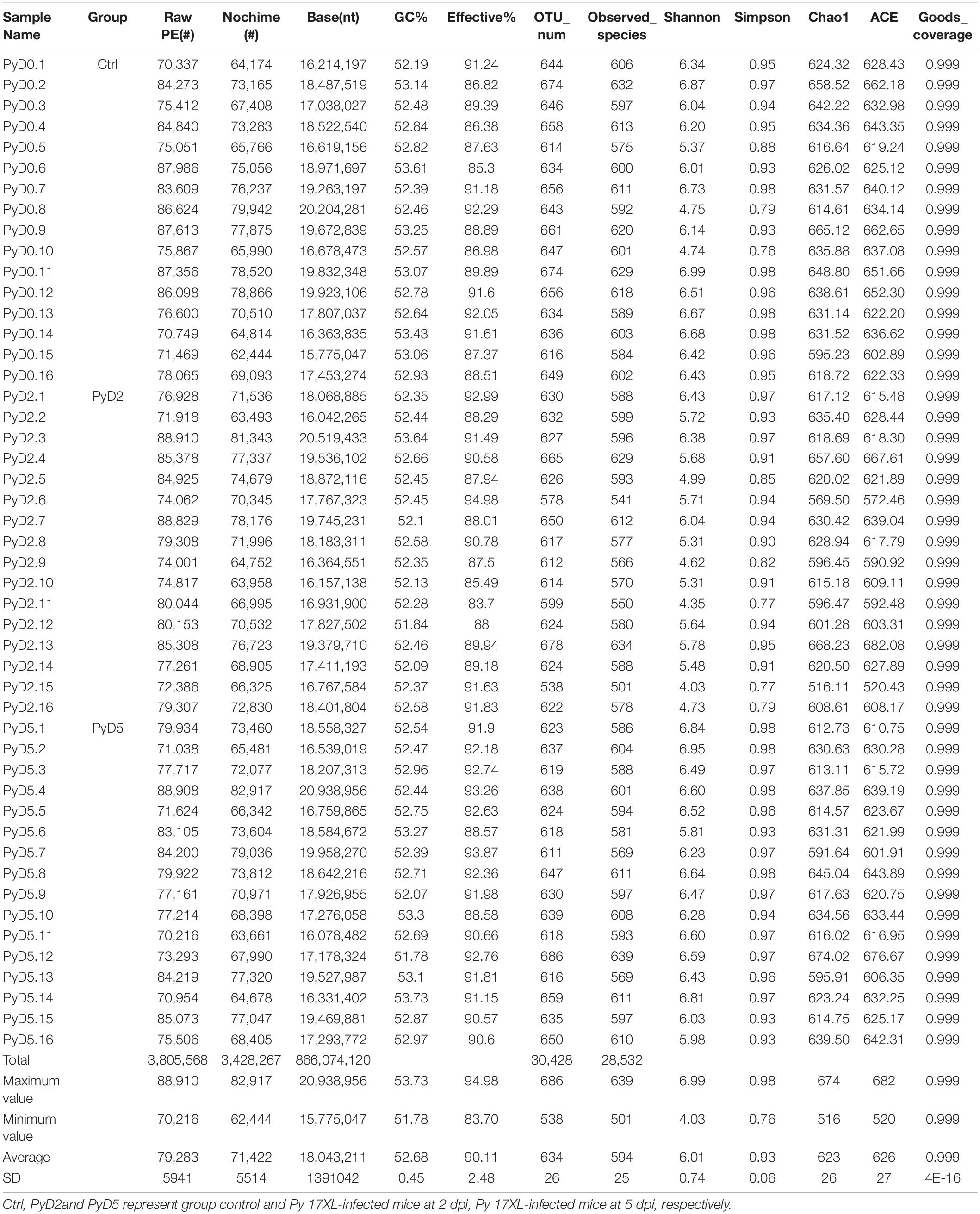
Table 1. Operational taxonomic unit (OTU)-based diversity indexes in mice gut samples during infection.
In sum, the OTU number was decreased after Py infection compared with that in the control. Table 1 lists the detailed characteristics of each sample. The Venn diagram and flower diagram were generated to compare the similarities and differences between the communities in the different groups and samples. There were a total of 1,009 OTUs in all three groups, which had 756 common OTUs. There were 39, 29, and 78 OTUs unique to the Ctrl, PyD2, and PyD5 groups, respectively. The PyD2 group had equal OTUs to the Ctrl group. The PyD5 group had 54 more OTUs than the Ctrl group. Meanwhile, the PyD5 group had 54 more OTUs than the PyD2 group (Figure 2A). For individual samples, the maximum OTUs were 80 in PyD5.12, the minimum OTUs were 1 in PyD5.2, PyD5.3, and PyD5.10, and the mean number of unique OTUs was 7 (Figures 2B–D).
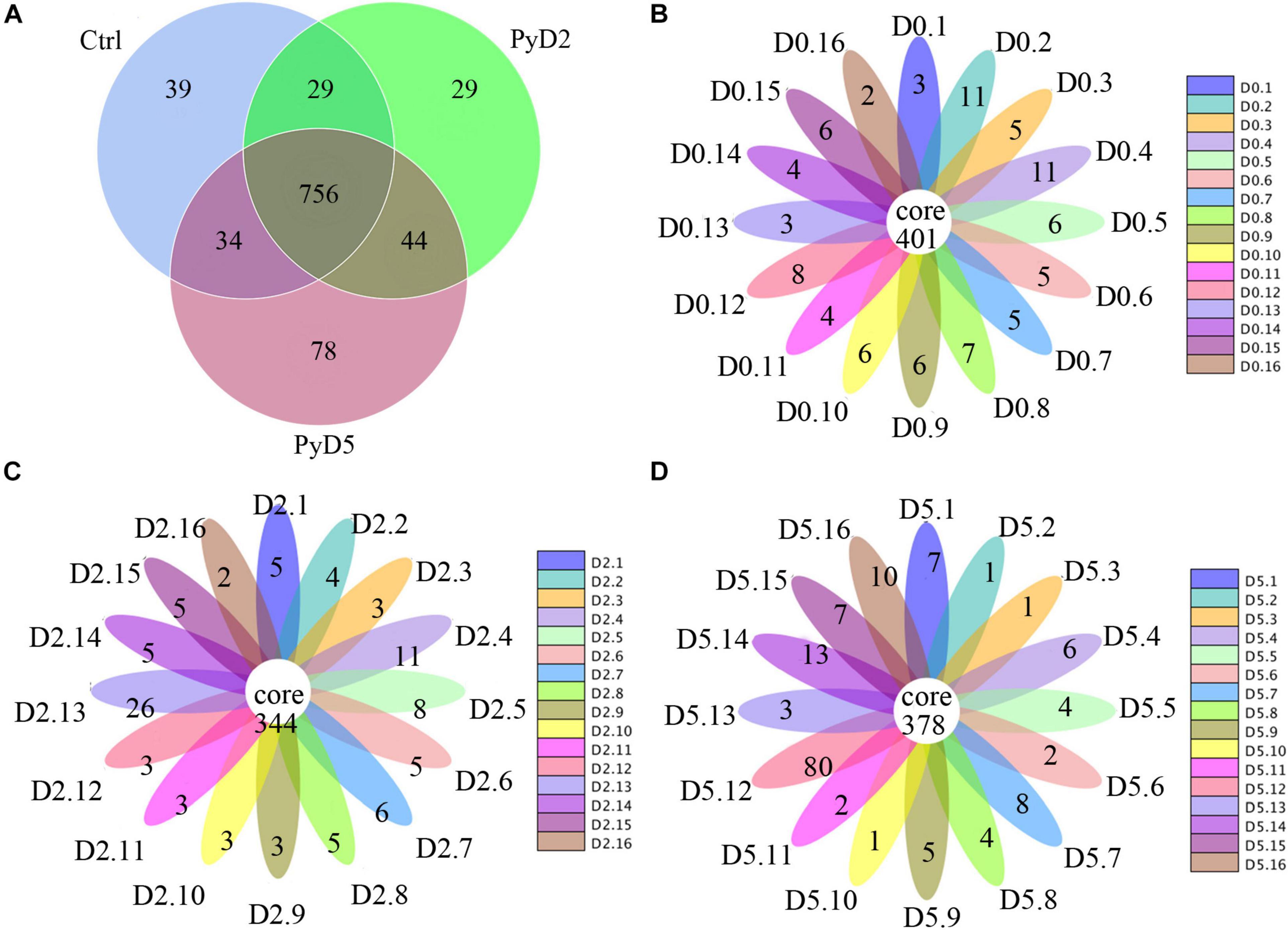
Figure 2. Operational taxonomic unit (OTU) analysis in the different fecal samples. (A) Venn diagram showing the unique and shared OTUs in the various groups and communities. (B) Flower diagram showing the shared and unique OTUs in the Ctrl samples. (C) Flower diagram showing the exclusive and mutual OTUs in the PyD2 samples. (D) The flower diagram shows the exclusive and mutual OTUs in the PyD5 samples.
The species accumulation curves represented species richness in all samples that were close to the plateau phase (Supplementary Figure 1A). Likewise, the rarefaction curves indicated that species representation in individual samples was close to the saturation number of observed species (Supplementary Figure 1B).
Species Annotation and Taxonomic Overview
Figure 3 shows the microbiota community composition of feces in all three groups of BALB/c mice. Each bar represents the average relative abundance of each microbial taxon in the stacked bar chart. The top 10 taxa with high relative abundance, which comprised 97% of the reads, are illustrated.
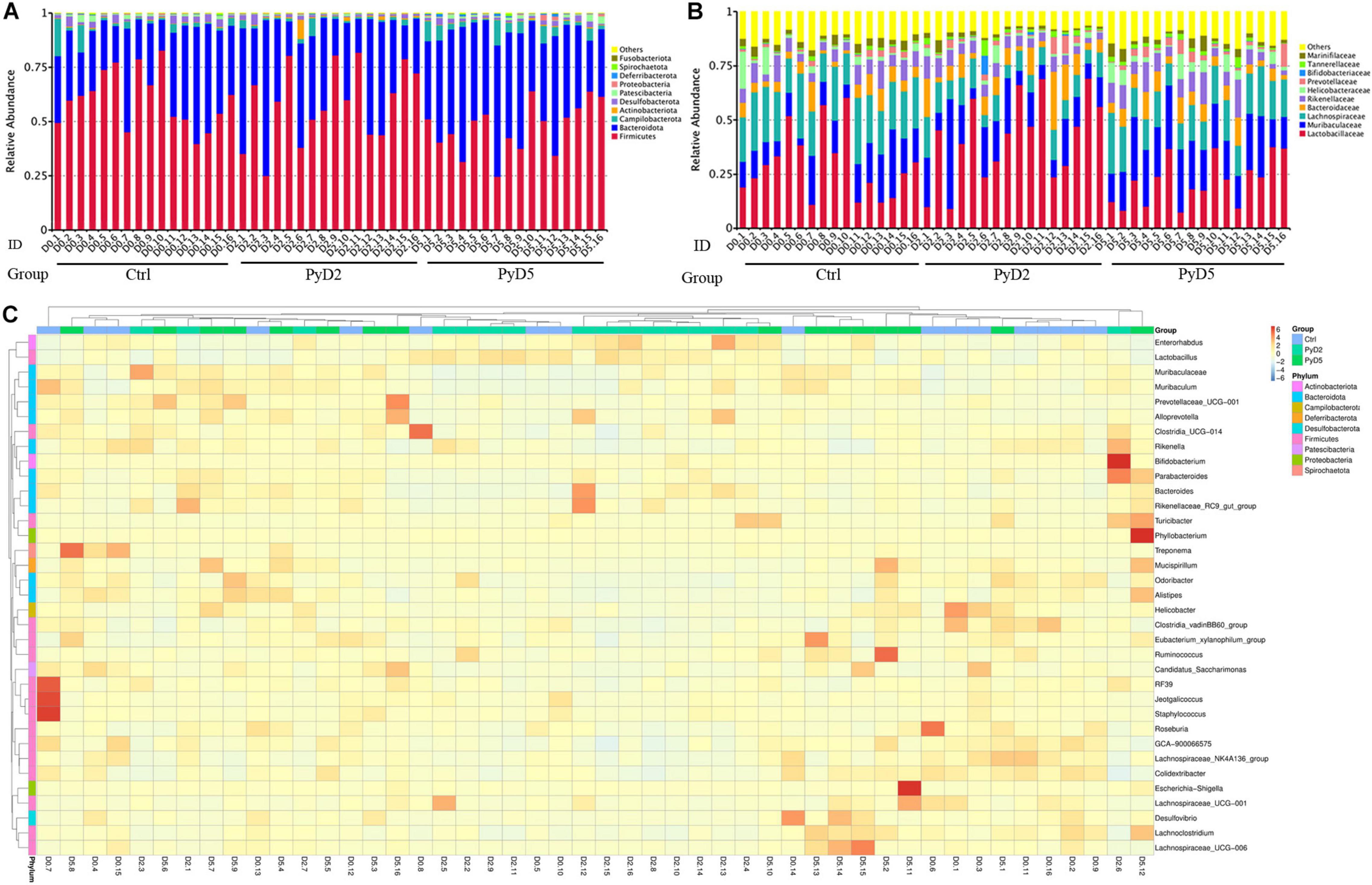
Figure 3. Changes in the gut microbiota composition. Relative abundance of fecal bacterial phyla and families existing in the BALB/c mice. Each bar represents the top 10 abundant phyla and families, whereas “Other” were obtained from low abundant and unclassified OTUs. Sample names represent samples described in Table 1. (A) Variations in the gut microbiota composition at the phylum level. (B) Variations in the gut microbiota composition at the family level; (C) Taxonomic heatmap of the three groups at the genus level. Typical relative g10 abundance of diversified genera present in the Ctrl, PyD2, and PyD5 groups. Each column represents a unique subject.
At the phylum level (Figure 3A), Firmicutes (55.39%) and Bacteroidetes (37.65%) were the major gut microbiota composition in all the samples. Compared with the Ctrl, Firmicutes was significantly decreased in post-infection samples, while Bacteroidetes was notably increased in post-infection samples.
At the family level (Figure 3B), the most dominant family was Lactobacillaceae (31.13%) in all the samples. Muribaculaceae (17.55%), Lachnospiraceae (16.53%), Bacteroidaceae (7.20%), Rikenellaceae (6.75%), Helicobacteraceae (3.11%), Marinifilaceae (2.53%), Prevotellaceae (2.19%), Tannerellaceae (1.43%), and Bifidobacteriaceae (0.30%) were the subdominant families. The abundance of Muribaculaceae, Prevotellaceae, and Tannerellaceae was increased in the post-infection samples compared with the Ctrl samples. The abundance of Lactobacillaceae, Bacteroidaceae, and Bifidobacteriaceae was dramatically increased at 2 dpi and then slightly decreased at 5 dpi. At the same time, the abundance of Lachnospiraceae, Rikenellaceae, Helicobacteraceae, and Marinifilaceae was slightly decreased at 2 dpi and then slightly increased at 5 dpi.
At the genus level, several taxa displayed significant differences among the three groups. Hierarchical clustering based on the abundance profile of the genera showed the most common genus in each group (Figure 3C). The gut microbiota in the samples of infected mice was characterized by higher abundances of Lactobacillus, Muribaculaceae, Bacteroides, Alistipes, Lachnospiraceae_NK4A136_group, Helicobacter, Odoribacter, Alloprevotella, Parabacteroides, and Bifidobacterium.
Analysis of the Bacterial Community Within Groups
Alpha diversity analysis was performed to explore the microbiota community diversity in the samples (within-group), reflecting the richness and diversity of the microbiota community. Alpha diversity metrics, including Chao1, Goods-coverage, observed species, Shannon and Simpson diversity index, and phylogenetic diversity values (Table 1), revealed a trend that infection with Py transiently changed the richness and evenness among the Ctrl, PyD2 and PyD5 groups. The estimated and observed OTUs in the three groups were different. The OTU number, observed species, and Shannon and Simpson index values were decreased at 2 dpi and then slightly increased at 5 dpi. Outliers were detected in all three groups (Supplementary Figure 2).
For Chao1 analysis (Supplementary Figure 2A), which represents an index for estimating the number of OTUs in the sample, the Ctrl samples had more estimated OTUs than the PyD2 group (p = 0.0833, t-test; p = 0.0289, Wilcox rank-sum test) and PyD5 group (p = 0.6761, t-test; p = 0.1617, Wilcox rank-sum test), while the PyD2 group had fewer OTUs than PyD5 group (p = 0.377, t-test; p = 0.408, Wilcox rank-sum test).
For observed species analysis (Supplementary Figure 2B), which represents the number of species contained in the sample, the Ctrl group samples had higher numbers than the PyD2 group (p = 0.0198, t-test; p = 0.0069, Wilcox rank-sum test) and the PyD5 group (p = 0.6650, t-test; p = 0.2384, Wilcox rank-sum test), and the PyD2 group had fewer observed species than the PyD5 group (p = 0.1388, t-test; p = 0.1084, Wilcox rank-sum test).
For Shannon diversity analysis (Supplementary Figure 2C), which assesses the richness and evenness of species composition in the sample, the samples of the Ctrl group had a higher number than the PyD2 group (p = 0.0012, t-test; p = 2.00E-04, Wilcox rank-sum test) but a lower number than the PyD5 group (p = 0.3987, t-test; p = 0.2132, Wilcox rank-sum test), while the PyD2 group had a lower number than the PyD5 group (p = 1.88E-05, t-test; p < 0.001, Wilcox rank-sum test).
For phylogenetic diversity analysis (Supplementary Figure 2D), which assesses the relationship of species in the microbiota community, samples in the Ctrl group had a slightly higher number than those in the PyD2 (p = 0.547, t-test) and PyD5 groups (p = 0.959, t-test), while the PyD2 group had a few lower numbers than the PyD5 group (p = 0.7182, t-test).
Analysis of the Bacterial Community Between Groups
For the unweighted UniFrac analysis (Supplementary Figure 2E), referring to the presence or absence of alterations in the species, beta diversity analysis was different among the Ctrl, PyD2, and PyD5 groups. The values in the PyD2 samples were increased relative to those in the Ctrl and PyD5 samples, but the values in the PyD5 samples were decreased relative to those in the Ctrl samples. That is, the samples in the PyD2 group had a higher number of OTUs than the Ctrl samples (p = 0.7244, t-test; p = 0.6495, Wilcoxon rank-sum test) and PyD5 samples (p = 0.7232, t-test; p = 0.0117, Wilcoxon rank-sum test). Moreover, the samples in the PyD5 group had a lower number of OTUs than the Ctrl group (p = 0.2843, t-test; p = 0.0382, Wilcoxon rank-sum test). It also demonstrated that the discreteness of the beta diversity increased from Ctrl to PyD2 and then decreased from PyD2 to PyD5. Meanwhile, for the weighted UniFrac analysis concerning both the presence or absence of species and the alterations in species abundance, the values among the Ctrl, PyD2, and PyD5 groups had an obviously similar trend to those of the unweighted UniFrac analysis (Supplementary Figure 2F).
The similarity degree between the BALB/c mouse gut microbiota composition in each sample was examined using the principal coordinate analysis (PCoA) based on the unweighted (Figure 4A) and weighted (Figure 4B) UniFrac distance matrices. The gut microbiota was markedly altered as Py 17XL infection progressed in BALB/c mice. On the PCoA plot, each symbol represents the gut microbiota of one mouse in the relevant stage. The similarity degree between community structures verified by PCoA was examined by comparing within-group unweighted UniFrac distances for the PC1 axis among the Ctrl, PyD2, and PyD5 groups (Figure 4A). In contrast, the similarity degree between community structures verified by PCoA was examined by comparing within-group weighted UniFrac distances for the PC2 axis among the Ctrl, PyD2, and PyD5 groups (Figure 4B).
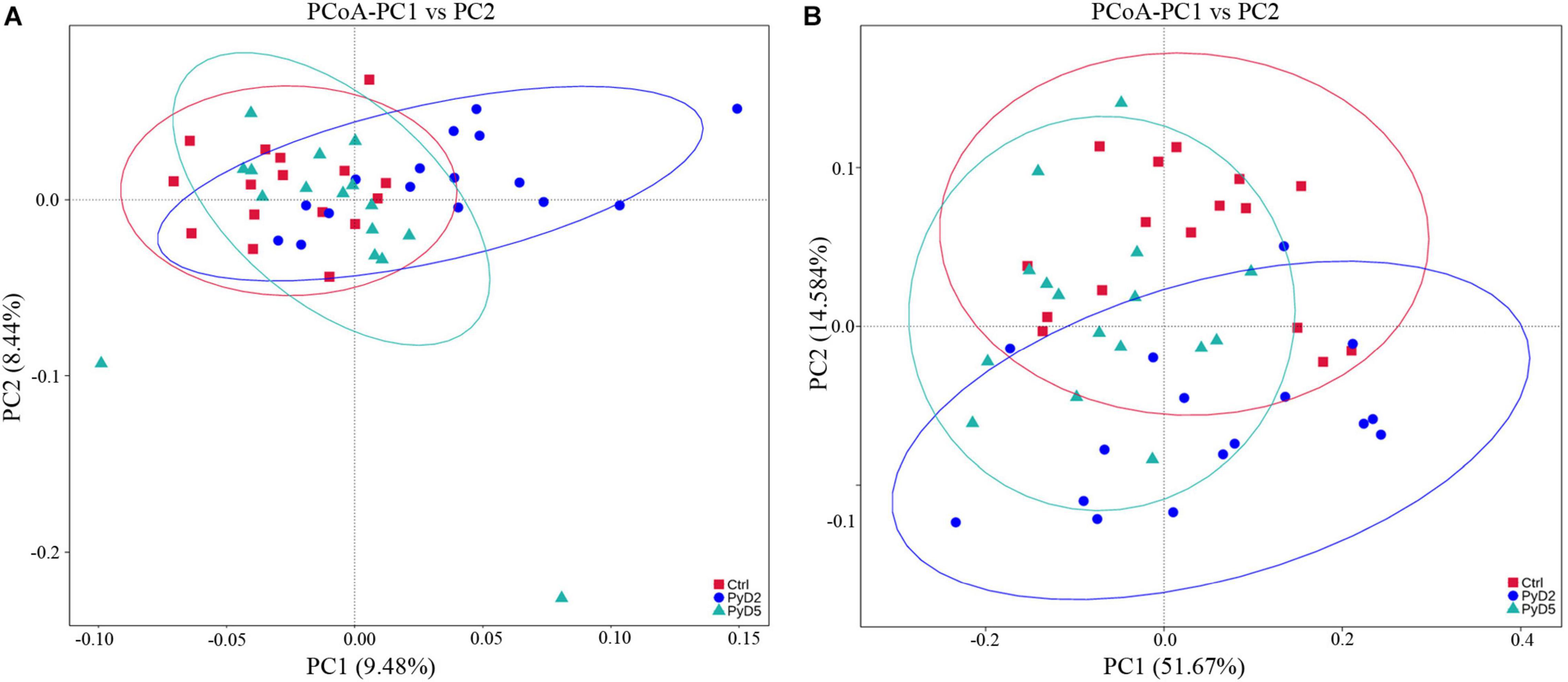
Figure 4. Principal coordinates analysis (PCoA) based on unweighted (A) and weighted (B) Unifrac distance matrices of fecal samples of BALB/c mice during infection. The fecal bacterial community components of both Py 17XL-infected and -uninfected mice were represented. Sample names are described in Table 1.
Following the PCoA plot, the between-group distances were significantly higher than the within-group distances for each group (ANOSIM, MRPP, p ≤ 0.01). The R value and P value of the ANOSIM between the Ctrl and PyD2 groups were 0.2386 and 0.002, respectively; the R value and P value of the ANOSIM between the Ctrl and PyD5 groups were 0.1739 and 0.004, respectively, and the R value and P value of the ANOSIM between PyD2 and PyD5 group were 0.3018 and 0.002, respectively. The R value indicated significant differences among the three groups, while the P value indicated statistical significance (p < 0.05). MRPP analysis (0.401, 0.384, 0.397; 0.422, 0.397, 0.423; observed-delta, expected-delta) indicated a lower difference within the groups and a higher difference between the groups. The A value of the MRPP was 0.05011, 0.03178, and 0.06291 (representing Ctrl-PyD2, Ctrl-PyD5, and PyD2-PyD5, respectively), demonstrating that the difference between groups was higher than that within groups (A > 0). Significance < 0.05 indicated a significant difference. These data demonstrated that the microbiota composition among Ctrl, PyD2 and PyD5 feces was significantly different.
Potential Biomarker Discovery
LEfSe analysis of the top 10 taxa (Figure 5) identified the different statistical biomarkers, which were the microbial taxa with significant differences among the three groups. This threshold could obtain as many taxa as possible for significant comparisons and eliminate the rarest taxa in the analysis. The potential biomarkers at different taxonomic levels were determined in all three stages (Figures 6A-D). At the genus level, the biomarker with a significant difference between the PyD2 and Ctrl groups was Lactobacillus (Lactobacillus murinus, which belongs to the Firmicutes phylum and Lactobacillaceae family) (Figures 5A,B). The biomarker with a significant difference between the PyD5 and Ctrl groups was Muribaculaceae (belonging to the Bacteroidetes phylum and Muribaculaceae family) (Figures 5A,C). In contrast, the biomarkers with a considerable difference between PyD5 and PyD2 groups were Muribaculaceae, Alistipes (belonging to the Bacteroidetes phylum and Rikenellaceae family), and Helicobacter (belonging to the Campilobacterota phylum and Helicobacteraceae family) (Figures 5A,D).
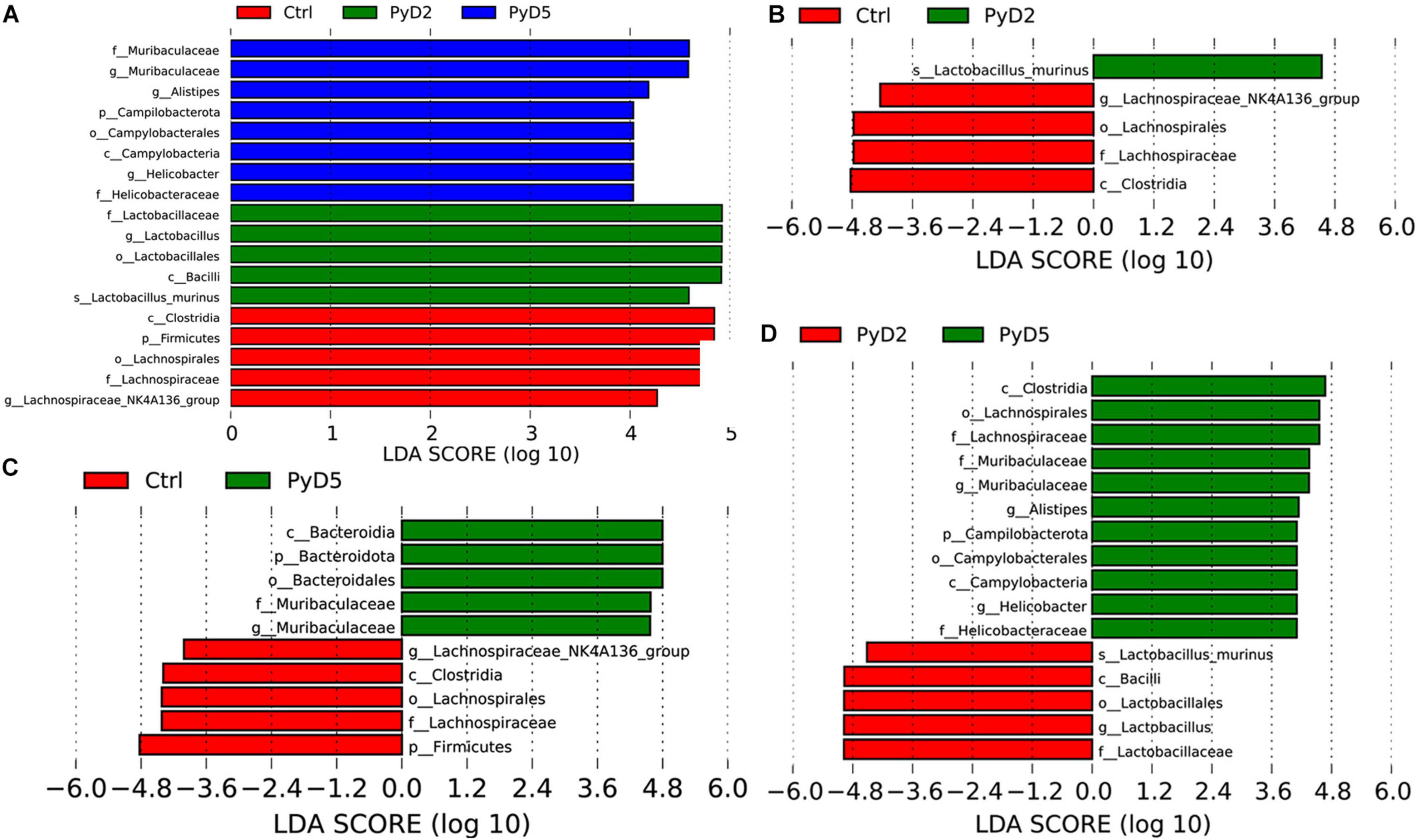
Figure 5. LEfSe identified the bacterial taxa significantly differentiated among different groups. Only taxa with the statistical differences between groups were shown in the figure, which was determined by meeting an LDA significant threshold > 4. (A) Histogram of the linear discriminant analysis (LDA) scores distribution among three groups; (B) Histogram of LDA score distribution between the Ctrl and PyD2 groups. (C) Histogram of LDA score distribution between the Ctrl and PyD5 groups. (D) Histogram of LDA score distribution between the PyD2 and PyD5 groups.
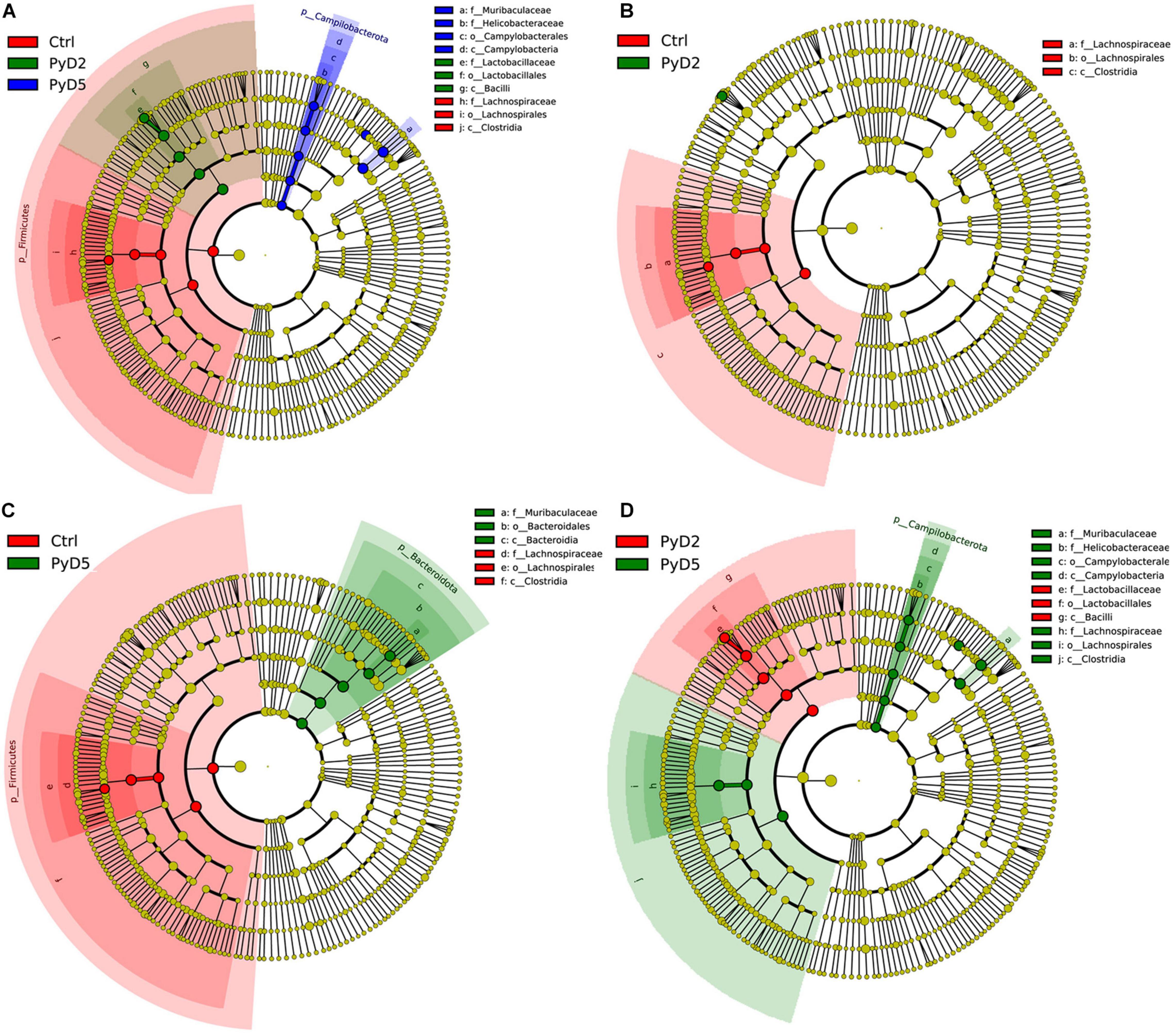
Figure 6. Cladogram of the most differentially abundant bacterial taxa among three groups. The red, green, and blue colors represent the bacterial communities of Ctrl, Py D2, and PyD5 groups, respectively. (A) Cladogram of the most differentially abundant bacterial taxa in the Ctrl, PyD2, and PyD5 groups. (B) Cladogram of the most differentially abundant bacterial taxa in the Ctrl and PyD2 groups. (C) Cladogram of the most differentially abundant bacterial taxa in the Ctrl and PyD5 groups. (D) Cladogram of the most differentially abundant bacterial taxa in the PyD2 and PyD5 groups.
Co-genetic network maps (Supplementary Figures 4, 5) were generated to identify the OTU co-abundance units and provide new insights for studying complex microbial community compositions and functions. These results presented an OTU co-abundance network approach to successfully generate associations that could generalize empirical data, and therefore, it might become a potential method for identifying microbiota associations. Co-genetic network maps can directly observe the effects on microbiota, the mutual advantage of the dominant species, and the related interactions of species after Py infection. Combined with the LEfSe of the top 10 taxa and networks, they showed that the genus Lactobacillus was associated with the genera Muribaculaceae, Bacteroides, Alloprevotella, and Parabacteroides. The genus Alistipes was correlated with the genera Rikenella, Muribaculum, Odoribacter, Mucispirillum, Bacteroides, and Parabacteroides. The genus Muribaculaceae was connected with the genera Muribaculum, NK4A214_group, Clostridia_UGG_010, and Rikenellaceae_RC9_gut_group. In contrast, the genus Helicobacter was associated with the genera Mucispirillum, Lactobacillus, and Enterococcus.
Discussion
The rodent and avian Plasmodium parasites do not infect humans and are not a threat to public health (Su and Wu, 2021). However, they may have economic impacts (Grim et al., 2008). Therefore, it is meaningful to explore the interactional effect between rodent Plasmodium parasites and mice. This study demonstrates that Plasmodium rodent species infection can alter the murine gut microbiota composition. Specifically, the gut microbiota compositions in Py 17XL-infected BALB/c mice were altered compared with Py 17XL-uninfected mice. That is, malaria caused by Plasmodium might be associated with the alteration of the gut microbiota. Consistent with this thought, a study clarified a meaningful association between the host’s microbiota composition and the proleptic risk of Plasmodium infection (Yooseph et al., 2015; Waide et al., 2020).
Both species accumulation boxplots and rarefaction curves approached the saturation level, which demonstrated almost full coverage of the whole microbiota diversity. Based on alpha and beta diversity analysis, the diversity and richness of the intestinal microbiota in all fecal samples were altered after Py 17XL infection. In the present study, the gut microbiota at the phylum level was dominated by Firmicutes and Bacteroidetes, which were consistent with the previous survey (Villarino et al., 2016).
The current data also demonstrate that the relative richness of dominant taxa was altered after infection. The abundance of Lactobacillaceae and Bifidobacteriaceae was dramatically increased at 2 dpi and then slightly decreased at 5 dpi. A previous study manifested that among differences in the gut flora were increased abundances of Lactobacillus (member of the Lactobacillaceae family) and Bifidobacterium (member of the Bifidobacteriaceae family) in resistant mice (Villarino et al., 2016). At the genus level, the gut microbiota in the samples of infected mice was characterized by higher amounts of Lactobacillus, Muribaculaceae, Bacteroides, Alistipes, and Helicobacter. Meanwhile, LEfSe analysis was performed to obtain biomarkers with significant differences between Py 17XL-infected and -uninfected mice. The biomarker with a considerable difference between the PyD2 and Ctrl groups was Lactobacillus (Lactobacillus murinus). The genus Lactobacillus was indicated to play a role in the stress response in patients (Hemarajata and Versalovic, 2013; Aizawa et al., 2018). That is, the genus Lactobacillus in the gut microbiota is related to the disease. Hu et al. verified that IBD induced with DSS caused a decrease in Lactobacillus gut microbiota at the genus level (Hu et al., 2020). The biomarker with an obvious difference between the PyD5 and Ctrl groups was Muribaculaceae. Moreover, the biomarkers with a noticeable difference between the PyD5 and PyD2 groups were Muribaculaceae, Alistipes, and Helicobacter. Parker et al. (2020) indicated that the genus Alistipes in the gut microbiota might have protective effects against some diseases such as colitis, liver fibrosis, and cancer immunotherapy. Other studies have revealed Alistipes is pathogenic in colorectal cancer (Moschen et al., 2016), and it is associated with mental signs of depression (Parker et al., 2020). A previous study manifested that the species Helicobacter pylori played a potential protective role in IBD (Sjomina et al., 2017). Kubota-Aizawa et al. (2017) performed an epidemiological survey of gastric Helicobacter spp. that were related to gastrointestinal disease in dogs.
Meanwhile, co-genetic network maps were generated to identify the OTU co-abundance units. Combined with the LEfSe on top 10 taxa and networks, they indicated that the genus Lactobacillus was associated with the genera Muribaculaceae, Bacteroides, Alloprevotella, Parabacteroides, and so on. A previous study suggested the attractive probiotic potential of the genus Bacteroides (species B. thetaiotaomicron) in IBD (Sitkin and Pokrotnieks, 2019). Another study found that genes for Bacteroides fragilis toxin encoded secreted oncotoxins correlated with colorectal cancer (Dejea et al., 2018). Chen et al. (2020) discovered that Bacteroides, Alistipes, Alloprevotella, Odoribacter, and Parabacteroides were closely correlated with short-chain fatty acid (SCFA) production, such as propionate, butyrate, and acetate. Silva et al. (2018) considered that butyrate played an essential protective role in intestinal homeostasis and IBD. In contrast, Chakravarty et al. (2019) demonstrated that the intestinal SCFA composition did not explain the effects of gut microbiota on malaria severity. The genus Alistipes was correlated with the genera Rikenella, Muribaculum, Odoribacter, and Mucispirillum. The genus Muribaculaceae was associated with the genera Muribaculum and Clostridia_UGG_010. The genus Helicobacter was correlated with the genera Mucispirillum and Lactobacillus. A previous study demonstrated that Rikenella or Rikenellaceae had an inverse relationship with body weight or fat mass (Li et al., 2017; Zhou et al., 2018) in mice and humans. Also, a high abundance of Rikenella is associated with low chronic non-infective inflammation (Miranda-Ribera et al., 2019). Brugiroux et al. (2016) displayed that Muribaculum intestinale was a strictly anaerobic bacterium that inhibited the colonization of pathogenic bacteria and regulated the balance of bacteria. Gomez-Arango et al. (2016) verified that the genus Odoribacter was known as a butyrate producer. A previous study reported that Mucispirillum schaedleri was abundant in the intestinal mucus layer of mice and humans, and that it played an essential role in inflammation (Loy et al., 2017). Besides, Lin et al. (2019) considered that patients with Parkinson’s disease had an increased abundance of M. schaedleri in the fecal microbiota. Schönherr-Hellec and Aires (2019) demonstrated that Clostridia species were responsible for necrotizing enterocolitis.
A previous study indicated that using probiotics might be a potential method to limit the reproduction of pathogenic microbiota (Pickard et al., 2017). These findings offered more insights into host gut microbiota alterations after Py 17XL infection and showed that microbiota analysis would play an important role in the early diagnosis of fatal malaria and in perceiving the pathogenesis of malaria. LEfSe analysis combined with the co-genetic OTU network showed that the genus Alistipes was a potential biomarker that may play a meaningful role in the pathogenesis and progression of Py malaria. The anaerobic bacterium Alistipes is found almost in the healthy human gastrointestinal tract (Shkoporov et al., 2015), and it is pathogenic in colorectal cancer (Moschen et al., 2016) and is associated with depression (Bangsgaard Bendtsen et al., 2012). These data indicated gut microbiota modulation can regard as a novel method of preventing severe malaria, which is consistent with the insight of Waide (Waide and Schmidt, 2020).
This article studied the alteration of the gut microbiota profile in BALB/c mice induced by Py 17XL infection and obtained potential biomarkers correlated with murine malaria. However, this research also had several limitations. In future studies, it will be important to explore the role of microbiota in the progress of malaria and to evaluate the microbiota modulation interfere with protection and less severity of the disease in a non-lethal infection model as PbA or Py 17XNL in BALB/c mice, and to assess the role of the microbiota more robustly using experimental approaches, such as non-lethal infection models, antimalarial drug therapy, and GF fecal microbiota transplantation.
The gut microbiota in mice before and after Py 17XL infection was characterized, and the overrepresented microbial taxa in infected and uninfected mice were identified. Meanwhile, several potential biomarkers at different taxonomic levels were obtained from this study. These microbiota taxa may serve as direct targets to clarify their roles in the pathogenesis and progression of lethal malaria in future studies. Also, in an association study of Py 17XL infection risk and gut microbiota composition, gut microbiota composition modulation will decrease Py 17XL infection risk and may be regarded as a standard to some antimalarial drugs or malaria vaccines.
Data Availability Statement
The datasets presented in this study can be found in online repositories. The names of the repository/repositories and accession number(s) can be found below website: https://www.ncbi.nlm.nih.gov/sra/PRJNA798515.
Ethics Statement
The animal study was reviewed and approved by the Institutional Animal Care and Use Committee of the Hubei University of Medicine under permit number HBMU-S20160414.
Author Contributions
WG and JL conceived the study, participated in its design, performed the data analysis and interpretation, and drafted the manuscript. WG, SY, and JL carried out the experiments. XS, HZ, and FL participated in analyzing and interpreting the data. All authors contributed to the article and approved the submitted version.
Funding
The Initial Project supported this study for the Principle Investigator Program of Hubei University of Medicine (HBMUPI202101), Post-Graduates of Hubei University of Medicine (Grant No. 2016QDJZR04), and the Research Project of the Hubei Provincial Department of Education (Grant No. Q20172102).
Conflict of Interest
The authors declare that the research was conducted in the absence of any commercial or financial relationships that could be construed as a potential conflict of interest.
Publisher’s Note
All claims expressed in this article are solely those of the authors and do not necessarily represent those of their affiliated organizations, or those of the publisher, the editors and the reviewers. Any product that may be evaluated in this article, or claim that may be made by its manufacturer, is not guaranteed or endorsed by the publisher.
Supplementary Material
The Supplementary Material for this article can be found online at: https://www.frontiersin.org/articles/10.3389/fmicb.2022.858897/full#supplementary-material
References
Aizawa, E., Tsuji, H., Asahara, T., Takahashi, T., Teraishi, T., Yoshida, S., et al. (2018). Bifidobacterium and counts in the gut microbiota of patients with bipolar disorder and healthy controls. Front. Psychiatry 9:730. doi: 10.3389/fpsyt.2018.00730
Astudillo, A., and Mayrovitz, H. (2021). The gut microbiome and cardiovascular disease. Cureus 13:e14519. doi: 10.7759/cureus.14519
Bangsgaard Bendtsen, K. M., Krych, L., Sorensen, D. B., Pang, W., Nielsen, D. S., Josefsen, K., et al. (2012). Gut microbiota composition is correlated to grid floor induced stress and behavior in the BALB/c mouse. PLoS One 7:e46231. doi: 10.1371/journal.pone.0046231
Brugiroux, S., Beutler, M., Pfann, C., Garzetti, D., Ruscheweyh, H. J., Ring, D., et al. (2016). Genome-guided design of a defined mouse microbiota that confers colonization resistance against Salmonella enterica serovar Typhimurium. Nat. Microbiol. 2:16215. doi: 10.1038/nmicrobiol.2016.215
Bunyavanich, S., Shen, N., Grishin, A., Wood, R., Burks, W., Dawson, P., et al. (2016). Early-life gut microbiome composition and milk allergy resolution. J. Allergy Clin. Immunol. 138, 1122–1130. doi: 10.1016/j.jaci.2016.03.041
Caporaso, J. G., Kuczynski, J., Stombaugh, J., Bittinger, K., Bushman, F. D., Costello, E. K., et al. (2010). QIIME allows analysis of high-throughput community sequencing data. Nat. Methods 7, 335–336. doi: 10.1038/nmeth.f.303
Chakravarty, S., Mandal, R., Duff, M., and Schmidt, N. (2019). Intestinal short-chain fatty acid composition does not explain gut microbiota-mediated effects on malaria severity. PloS One 14:e0214449. doi: 10.1371/journal.pone.0214449
Chen, G., Feng, H., Liu, J., Qi, Z. M., Wu, Y., Guo, S. Y., et al. (2010). Characterization of immune responses to single or mixed infections with P. yoelii 17XL and P. chabaudi AS in different strains of mice. Parasitol. Int. 59, 400–406. doi: 10.1016/j.parint.2010.05.005
Chen, H., Zhang, F., Zhang, J., Zhang, X., Guo, Y., and Yao, Q. (2020). A holistic view of berberine inhibiting intestinal carcinogenesis in conventional mice based on microbiome-metabolomics analysis. Front. Immunol. 11:588079. doi: 10.3389/fimmu.2020.588079
Chu, D., Ma, J., Prince, A., Antony, K., Seferovic, M., and Aagaard, K. (2017). Maturation of the infant microbiome community structure and function across multiple body sites and in relation to mode of delivery. Nat. Med. 23, 314–326. doi: 10.1038/nm.4272
De, S. L., Stanisic, D. I., Rivera, F., Batzloff, M. R., Engwerda, C., and Good, M. F. (2016). Plasmodium berghei bio-burden correlates with parasite lactate dehydrogenase: application to murine Plasmodium diagnostics. Malaria J. 15:3. doi: 10.1186/s12936-015-1027-2
Dejea, C., Fathi, P., Craig, J., Boleij, A., Taddese, R., Geis, A., et al. (2018). Patients with familial adenomatous polyposis harbor colonic biofilms containing tumorigenic bacteria. Science 359, 592–597. doi: 10.1126/science.aah3648
Denny, J., Powers, J., Castro, H., Zhang, J., Joshi-Barve, S., Campagna, S., et al. (2019). Differential sensitivity to plasmodium yoelii infection in C57BL/6 mice impacts gut-liver axis homeostasis. Sci. Rep. 9:3472. doi: 10.1038/s41598-019-40266-6
DeSantis, T. Z., Hugenholtz, P., Larsen, N., Rojas, M., Brodie, E. L., Keller, K., et al. (2006). Greengenes, a chimera-checked 16S rRNA gene database and workbench compatible with ARB. Appl. Environ. Microbiol. 72, 5069–5072. doi: 10.1128/AEM.03006-05
Edgar, R. C. (2004). MUSCLE: multiple sequence alignment with high accuracy and high throughput. Nucleic Acids Res. 32, 1792–1797. doi: 10.1093/nar/gkh340
Edgar, R. C. (2013). UPARSE: highly accurate OTU sequences from microbial amplicon reads. Nat. Methods 10, 996–998. doi: 10.1038/nmeth.2604
Edgar, R. C., Haas, B. J., Clemente, J. C., Quince, C., and Knight, R. (2011). UCHIME improves sensitivity and speed of chimera detection. Bioinformatics 27, 2194–2200. doi: 10.1093/bioinformatics/btr381
Feng, J., Zhang, L., Xia, Z., Zhou, S., and Xiao, N. (2021). Malaria-free certification in china: achievements and lessons learned from the national malaria elimination programme. Zoonoses 1:2. doi: 10.15212/ZOONOSES-2021-1002
Gill, S., Pop, M., Deboy, R., Eckburg, P., Turnbaugh, P., Samuel, B., et al. (2006). Metagenomic analysis of the human distal gut microbiome. Science 312, 1355–1359. doi: 10.1126/science.1124234
Gomaa, E. (2020). Human gut microbiota/microbiome in health and diseases: a review. Antonie van Leeuwenhoek 113, 2019–2040. doi: 10.1007/s10482-020-01474-7
Gomez-Arango, L. F., Barrett, H. L., McIntyre, H. D., Callaway, L. K., Morrison, M., and Dekker Nitert, M. (2016). Increased systolic and diastolic blood pressure is associated with altered gut microbiota composition and butyrate production in early pregnancy. Hypertension 68, 974–981. doi: 10.1161/hypertensionaha.116.07910
Grim, K. C., McCutchan, T., Sullivan, M., and Cranfield, M. R. (2008). Unidentified Plasmodium species in Australian black swans (Cygnus atratus) hatched and raised in North America. J. Zoo. Wildl. Med. 39, 216–220. doi: 10.1638/2007-0110r.1
Guan, W., Yang, S., Zhao, Y., Cheng, W., Song, X., Yao, Y., et al. (2021). Observation of the Gut Microbiota Profile in C57BL/6 mice induced by plasmodium berghei ANKA Infection. Front. Cell Infect. Microbiol. 11:680383. doi: 10.3389/fcimb.2021.680383
Haas, B. J., Gevers, D., Earl, A. M., Feldgarden, M., Ward, D. V., Giannoukos, G., et al. (2011). Chimeric 16S rRNA sequence formation and detection in Sanger and 454-pyrosequenced PCR amplicons. Genome Res. 21, 494–504. doi: 10.1101/gr.112730.110
Hemarajata, P., and Versalovic, J. (2013). Effects of probiotics on gut microbiota: mechanisms of intestinal immunomodulation and neuromodulation. Therapeutic. Adv. Gastroenterol. 6, 39–51. doi: 10.1177/1756283x12459294
Hu, L., Jin, L., Xia, D., Zhang, Q., Ma, L., Zheng, H., et al. (2020). Nitrate ameliorates dextran sodium sulfate-induced colitis by regulating the homeostasis of the intestinal microbiota. Free Radic Biol. Med. 152, 609–621. doi: 10.1016/j.freeradbiomed.2019.12.002
Karlsson, F., Tremaroli, V., Nielsen, J., and Backhed, F. (2013). Assessing the human gut microbiota in metabolic diseases. Diabetes 62, 3341–3349. doi: 10.2337/db13-0844
Kubota-Aizawa, S., Ohno, K., Fukushima, K., Kanemoto, H., Nakashima, K., Uchida, K., et al. (2017). Epidemiological study of gastric Helicobacter spp. in dogs with gastrointestinal disease in Japan and diversity of Helicobacter heilmannii sensu stricto. Vet. J. 225, 56–62. doi: 10.1016/j.tvjl.2017.04.004
Li, R., Wang, H., Shi, Q., Wang, N., Zhang, Z., Xiong, C., et al. (2017). Effects of oral florfenicol and azithromycin on gut microbiota and adipogenesis in mice. PLoS One 12:e0181690. doi: 10.1371/journal.pone.0181690
Lin, C. H., Chen, C. C., Chiang, H. L., Liou, J. M., Chang, C. M., Lu, T. P., et al. (2019). Altered gut microbiota and inflammatory cytokine responses in patients with Parkinson’s disease. J. Neuroinflamm. 16:129. doi: 10.1186/s12974-019-1528-y
Loy, A., Pfann, C., Steinberger, M., Hanson, B., Herp, S., Brugiroux, S., et al. (2017). Lifestyle and horizontal gene transfer-mediated evolution of mucispirillum schaedleri, a core member of the murine gut microbiota. mSystems 2, e171–e116. doi: 10.1128/mSystems.00171-16
Magoč, T., and Salzberg, S. L. (2011). FLASH: fast length adjustment of short reads to improve genome assemblies. Bioinformatics 27, 2957–2963. doi: 10.1093/bioinformatics/btr507
Miranda-Ribera, A., Ennamorati, M., Serena, G., Cetinbas, M., Lan, J., Sadreyev, R. I., et al. (2019). Exploiting the zonulin mouse model to establish the role of primary impaired gut barrier function on microbiota composition and immune profiles. Front. Immunol. 10:2233. doi: 10.3389/fimmu.2019.02233
Moschen, A. R., Gerner, R. R., Wang, J., Klepsch, V., Adolph, T. E., Reider, S. J., et al. (2016). Lipocalin 2 protects from inflammation and tumorigenesis associated with gut microbiota alterations. Cell Host. Microbe. 19, 455–469. doi: 10.1016/j.chom.2016.03.007
Nair, S., Xu, R., Pattaradilokrat, S., Wu, J., Qi, Y., Zilversmit, M., et al. (2017). A Plasmodium yoelii HECT-like E3 ubiquitin ligase regulates parasite growth and virulence. Nat. Commun. 8:223. doi: 10.1038/s41467-017-00267-3
Nishino, K., Nishida, A., Inoue, R., Kawada, Y., Ohno, M., Sakai, S., et al. (2018). Analysis of endoscopic brush samples identified mucosa-associated dysbiosis in inflammatory bowel disease. J. Gastroenterol. 53, 95–106. doi: 10.1007/s00535-017-1384-4
Parker, B. J., Wearsch, P. A., Veloo, A. C. M., and Rodriguez-Palacios, A. (2020). The genus alistipes: gut bacteria with emerging implications to inflammation, cancer, and mental health. Front. Immunol. 11:906. doi: 10.3389/fimmu.2020.00906
Pickard, J. M., Zeng, M. Y., Caruso, R., and Núñez, G. (2017). Gut microbiota: Role in pathogen colonization, immune responses, and inflammatory disease. Immunol. Rev. 279, 70–89. doi: 10.1111/imr.12567
Prasad, R. N., and Virk, K. J. (1993). Malaria as a cause of diarrhoea–a review. Papua New Guinea Med. J. 36, 337–341.
Schönherr-Hellec, S., and Aires, J. (2019). Clostridia and necrotizing enterocolitis in preterm neonates. Anaerobe 58, 6–12. doi: 10.1016/j.anaerobe.2019.04.005
Shkoporov, A. N., Chaplin, A. V., Khokhlova, E. V., Shcherbakova, V. A., Motuzova, O. V., Bozhenko, V. K., et al. (2015). Alistipes inops sp. nov. and Coprobacter secundus sp. nov., isolated from human faeces. Int. J. Syst. Evol. Microbiol. 65, 4580–4588. doi: 10.1099/ijsem.0.000617
Silva, J. P. B., Navegantes-Lima, K. C., Oliveira, A. L. B., Rodrigues, D. V. S., Gaspar, S. L. F., Monteiro, V. V. S., et al. (2018). Protective mechanisms of butyrate on inflammatory bowel disease. Curr. Pharm. Des. 24, 4154–4166. doi: 10.2174/1381612824666181001153605
Sitkin, S., and Pokrotnieks, J. (2019). Gut microbiota as a host defender and a foe: the 2 faces of commensal bacteroides thetaiotaomicron in inflammatory bowel disease. Inflamm. Bowel. Dis. 25:e71. doi: 10.1093/ibd/izy377
Sjomina, O., Heluwaert, F., Moussata, D., and Leja, M. (2017). Helicobacter pylori infection and nonmalignant diseases. Helicobacter 22:408. doi: 10.1111/hel.12408
Su, X., and Wu, J. (2021). Zoonotic transmission and host switches of malaria parasites. Zoonoses 1:11. doi: 10.15212/ZOONOSES-2021-0015
Villarino, N., LeCleir, G., Denny, J., Dearth, S., Harding, C., Sloan, S., et al. (2016). Composition of the gut microbiota modulates the severity of malaria. Proc. Nat. Acad. Sci. USA 113, 2235–2240. doi: 10.1073/pnas.1504887113
Waide, M., Polidoro, R., Powell, W., Denny, J., Kos, J., Tieri, D., et al. (2020). Gut microbiota composition modulates the magnitude and quality of germinal centers during plasmodium infections. Cell Rep. 33:108503. doi: 10.1016/j.celrep.2020.108503
Waide, M., and Schmidt, N. (2020). The gut microbiome, immunity, and Plasmodium severity. Curr. Opinion Microbiol. 58, 56–61. doi: 10.1016/j.mib.2020.08.006
Wang, Q., Garrity, G. M., Tiedje, J. M., and Cole, J. R. (2007). Naive Bayesian classifier for rapid assignment of rRNA sequences into the new bacterial taxonomy. Appl. Environ. Microbiol. 73, 5261–5267. doi: 10.1128/AEM.00062-07
Yilmaz, B., Portugal, S., Tran, T., Gozzelino, R., Ramos, S., Gomes, J., et al. (2014). Gut microbiota elicits a protective immune response against malaria transmission. Cell 159, 1277–1289. doi: 10.1016/j.cell.2014.10.053
Yooseph, S., Kirkness, E., Tran, T., Harkins, D., Jones, M., Torralba, M., et al. (2015). Stool microbiota composition is associated with the prospective risk of Plasmodium falciparum infection. BMC Genomics 16:631. doi: 10.1186/s12864-015-1819-3
Keywords: Plasmodium yoelii, malaria, gut microbiota, 16S rRNA, biomarker
Citation: Guan W, Song X, Yang S, Zhu H, Li F and Li J (2022) Observation of the Gut Microbiota Profile in BALB/c Mice Induced by Plasmodium yoelii 17XL Infection. Front. Microbiol. 13:858897. doi: 10.3389/fmicb.2022.858897
Received: 20 January 2022; Accepted: 22 February 2022;
Published: 31 March 2022.
Edited by:
George Grant, University of Aberdeen, United KingdomReviewed by:
Rabindra Kumar Mandal, Indiana University, United StatesFátima Brant, Federal University of Minas Gerais, Brazil
Copyright © 2022 Guan, Song, Yang, Zhu, Li and Li. This is an open-access article distributed under the terms of the Creative Commons Attribution License (CC BY). The use, distribution or reproduction in other forums is permitted, provided the original author(s) and the copyright owner(s) are credited and that the original publication in this journal is cited, in accordance with accepted academic practice. No use, distribution or reproduction is permitted which does not comply with these terms.
*Correspondence: Fang Li, c3lsZjIwMDlAc2luYS5jb20=; Jian Li, eXhsaWppYW5AMTYzLmNvbQ==
†These authors have contributed equally to this work