- 1Institute of Molecular and Cell Biology, Agency for Science, Technology and Research (A*STAR), Singapore, Singapore
- 2Bioprocessing Technology Institute, Agency for Science, Technology and Research (A*STAR), Singapore, Singapore
- 3Bioinformatics Institute, Agency for Science, Technology and Research (A*STAR), Singapore, Singapore
- 4School of Chemical Engineering, Sungkyunkwan University, Suwon, South Korea
- 5Department of Microbiology and Immunology, Yong Loo Lin School of Medicine, National University of Singapore, Singapore, Singapore
- 6Department of Surgery, Yong Loo Lin School of Medicine, National University of Singapore, Singapore, Singapore
The incidence and prevalence of inflammatory disorders have increased globally, and is projected to double in the next decade. Gut microbiome-based therapeutics have shown promise in ameliorating chronic inflammation. However, they are largely experimental, context- or strain-dependent and lack a clear mechanistic basis. This hinders precision probiotics and poses significant risk, especially to individuals with pre-existing conditions. Molecules secreted by gut microbiota act as ligands to several health-relevant receptors expressed in human gut, such as the G-protein coupled receptors (GPCRs), Toll-like receptor 4 (TLR4), pregnane X receptor (PXR), and aryl hydrocarbon receptor (AhR). Among these, the human AhR expressed in different tissues exhibits anti-inflammatory effects and shows activity against a wide range of ligands produced by gut bacteria. However, different AhR ligands induce varying host responses and signaling in a tissue/organ-specific manner, which remain mostly unknown. The emerging systems biology paradigm, with its powerful in silico tool repertoire, provides opportunities for comprehensive and high-throughput strain characterization. In particular, combining metabolic models with machine learning tools can be useful to delineate tissue and ligand-specific signaling and thus their causal mechanisms in disease and health. The knowledge of such a mechanistic basis is indispensable to account for strain heterogeneity and actualize precision probiotics.
Introduction
The human gut microbiome has attracted attention in the past decade for its role in inflammatory disorders (Kamada et al., 2013). Altering the gut microbiome profile through probiotic supplementation has been suggested as a potential strategy for ameliorating the symptoms associated with such diseases including inflammatory bowel disease (IBD) and colorectal cancer (Hendler and Zhang, 2018). The commercial probiotic formulations largely exist as generic supplements labeled with health claims that often remain unsubstantiated. Moreover, the existence of non-specific labels affects public perception on their proposed efficacy in treating health conditions, thereby posing risk to the probiotic market over a long run. Hence, precision probiotics with well-defined activities that target specific health conditions are imperative. The development of precision probiotics is not a trivial task, as it requires a deeper understanding of the complex molecular cross talk between gut microbiota and host. One way to address this problem is to first map possible interactions between the two in the gut environment and subsequently trace the host effects downstream to the interaction. Several human receptors have been shown to interact with the gut microbiota, including the G-protein coupled receptors (GPCRs), Toll-like receptors (TLRs), pregnane X receptor (PXR), and aryl hydrocarbon receptor (AhR) (Mohandas and Vairappan, 2017; Chang and Kao, 2019; Chen et al., 2019). The activity of each of these receptors brings about varied molecular responses that affect health. In simpler terms, the “precision” aspect of probiotics can be gauged by their net conceivable interactions with the host receptors.
Tracing the physiological effects of gut microbiota, particularly during probiotic supplementation, and the small molecules they produce is a daunting task. In particular, the pleiotropy resulting from the interaction of multiple gut microbial ligand-host receptor combinations is hard to discern using classical molecular biology techniques. Integration of multiple omics datasets has been instrumental in explaining complex biological phenomena. Further, genome-scale metabolic models (GEMs) serve as convenient tools to represent complex molecular networks in a meaningful manner, especially when constrained using omics datasets. Therefore, the future of gut microbiome therapeutics will greatly benefit from a systematic amalgamation of multiomics, GEMs and machine learning tools.
Gut Microbiota-Human Receptor Interactions
Gut microbiota interact with the host either through direct association with the intestinal epithelial cells or via secretion of small molecules. Although direct interactions are possible, they are limited to human cells in the gut lining. On the other hand, small molecules produced by the microbiota in gut environment not only interact with the host cell lining in intestine but also enter the blood circulation and access receptors expressed in nearly all human organs. Such interactions play a wide array of roles in health and disease, ranging from intestinal homeostasis, dysbiosis, inflammation and cancer. The human receptors such as GPCRs, PXR, and TLRs have been shown to play an important role in gut-microbiome mediated immunomodulation including their anti-inflammatory roles (Valentini et al., 2014; Venkatesh et al., 2014; Chen et al., 2019; Melhem et al., 2019). However, AhR is more often implicated in governing the overall gut homeostasis and inflammation (Zelante et al., 2013; Marinelli et al., 2019). Several studies have clearly demonstrated causal links between anti-inflammatory outcomes in chronic inflammatory conditions and treatment with AhR ligands or the gut microbial strains synthesizing them (Takamura et al., 2011; Fukumoto et al., 2014; Lamas et al., 2016; Abdulla et al., 2021; Zhao et al., 2021). AhR is a transcription factor, originally thought to function as a xenobiotic sensor, is now known to be an important regulator of immunity, stem cell maintenance and cellular differentiation (Gutiérrez-Vázquez and Quintana, 2018). Several signaling mechanisms downstream to AhR activation have been elucidated (Agus et al., 2018; Roager and Licht, 2018). Different gut microbial species and strains exhibit varied AhR modulating capabilities via their secretory metabolites. For example, short chain fatty acids (SCFAs) produced by gut microbiota bring about AhR activity through multiple mechanisms, including enhancement of AhR responsiveness to ligands (Jin et al., 2017), acting as a direct ligand to AhR (Marinelli et al., 2019), and increasing the expression of AhR (Yang et al., 2020). Each of these studies have indicated that the SCFA-mediated mechanisms eventually converge at promoting AhR activity and bring about anti-inflammatory effects. Similarly, tryptophan and indole derivatives activate the AhR resulting in anti-inflammatory outcomes (Lamas et al., 2016; Özçam et al., 2019). Despite their promise, the ligand- and tissue-specific effects of AhR signaling, which confound clinical studies, is not completely understood; not all AhR ligands confer anti-inflammatory phenotype (Denison et al., 2011). Therefore, characterizing strains and their tissue-specific AhR-mediated inflammation is an indispensable requirement for their use as therapeutic modalities.
Role of Systems Biology
Gut is a complex environment characterized by innumerable biotic and abiotic interactions. The chaotic regimen consisting of diet, microbiota, host secretions and gut barrier render the ligand-receptor interactions largely untraceable using conventional “top-down” experimental techniques (Veiga et al., 2020). However, the emergent network properties of such systems of interacting components have always been tractable using mathematical modeling techniques. Systems biology, a discipline that treats organisms as an integration of one or more such networks, plays an important role in today’s biological research, especially studying complex systems such as the human gut. An aspect that makes a strong case for the use of systems biology has been its tremendous success in studying metabolic networks using constraint-based modeling approach. Large-scale metabolic model reconstruction efforts have been undertaken in the past to facilitate studies deciphering interactions within and between gut microbiota and human intestinal cells (Diener et al., 2020; Heinken et al., 2021). These models largely rely on biochemical capabilities reflected by genomic data and taxonomic abundances estimated from metagenomic information, and therefore, the depth of interactions prevailing in the gut extend beyond what is usually captured by them. Fecal and serum metabolomic and transcriptomic datasets when integrated with such metabolic models can systematically reveal metabolic correlations of the microbiome. Furthermore, the use of machine learning tools in combination with omics integrated metabolic models can help derive mechanistic insights on potential health effects of specific strains (Yang et al., 2019; Lewis and Kemp, 2021). A list of publicly available systems biology tools that can aid in such an integrated analysis is shown in Table 1.
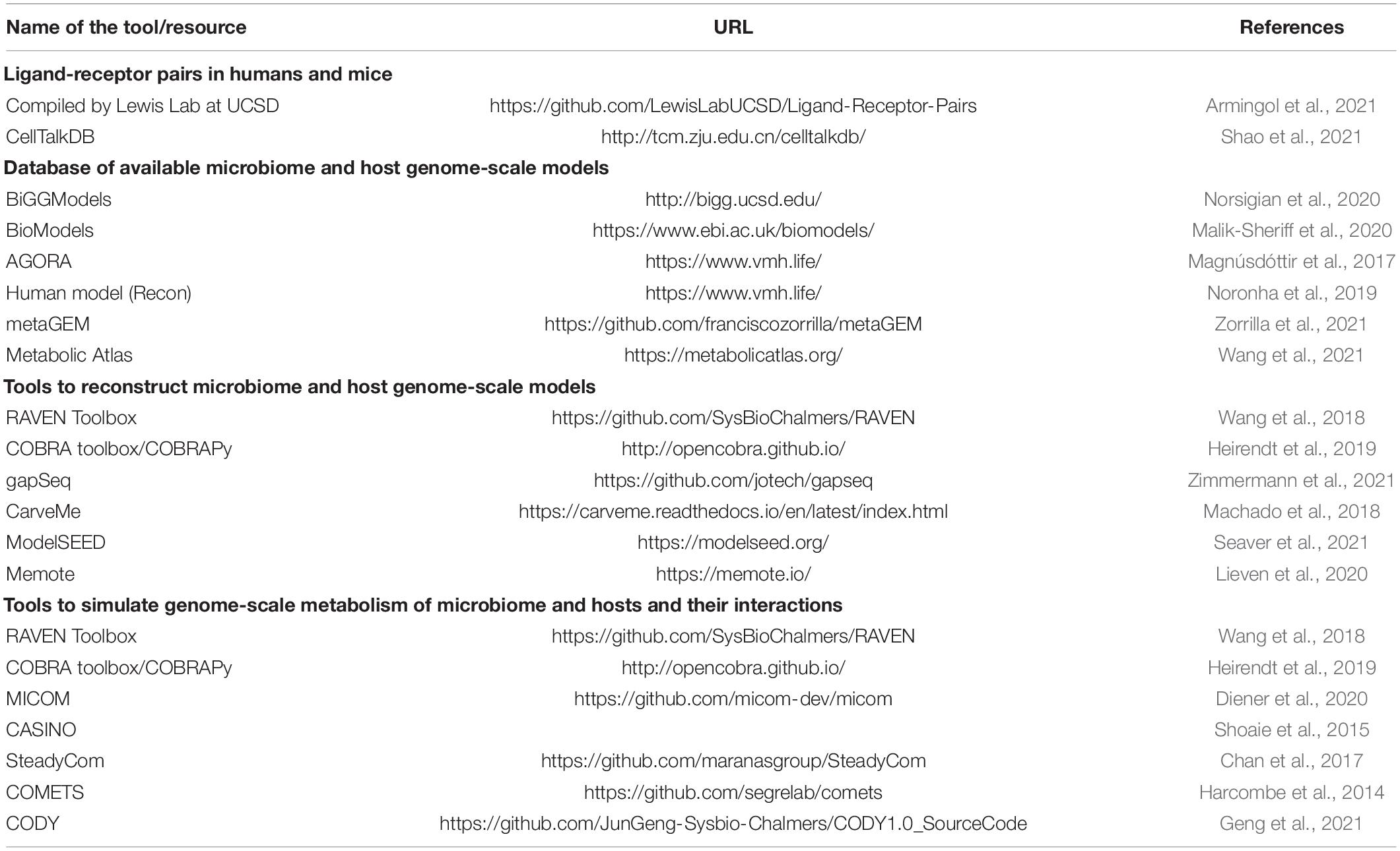
Table 1. Survey of systems biology tools and resources to study gut microbiome-host receptor interactions.
Precision Anti-Inflammatory Probiotics: Need for a Two-Pronged Approach
The current trend in systems biology has been to predict and quantify immunomodulatory effects of specific probiotic strains and metabolites that they produce in the gut environment (dos Santos et al., 2010; Fiocchi and Iliopoulos, 2021). Availability of quantitative information on microbiota-induced immunomodulation is an essential requirement to realize precision probiotics. Ligand-receptor interactions form the direct link between microbiota and immunomodulation in humans. Therefore, a systematic approach toward designing precision probiotics must first take into account the sum total interactions brought about by each bacterial strain or microbial consortia via the small molecule ligands they secrete and their possible receptors in humans. In this regard, GEMs can greatly simplify the evaluation of various strains for the biosynthesis of potential ligands. Well-curated models can further provide quantitative estimates of the ligand production, which may serve as proxies for ligand-receptor activities. For instance, we have reconstructed and used GEMs for 18 lactic acid bacterial strains to systematically evaluate their probiotic capabilities (Koduru et al., 2021). This study constitutes one of the first attempts to theoretically quantify the probiotic potential of strains. Specifically, we relied on three important metrics based on constraint-based flux analysis to evaluate the strains. First, we estimated their capacities to biosynthesize metabolites, commonly referred to as “postbiotics,” with anti-inflammatory properties such as SCFAs, tryptophan and indole, and pro-inflammatory metabolites such as lipoteichoic acids and branched chain amino acids. Subsequently, we evaluated their ability to persist in the gut environment under various dietary regimens. Finally, we assessed the nature of their potential interactions with beneficial gut commensals. These estimates can be prudently used to recommend strains to manage inflammation.
The second important requirement of precision probiotics involves understanding the nature of pleiotropic effects resulting from the cumulative downstream signaling of multiple ligand-receptor interactions. To this end, (Stein et al., 2018) have developed a computational approach to design optimal microbial consortia for induction of regulatory T-cells (Tregs), which are immune cells with an anti-inflammatory effect. The authors combined data on the contribution of 11 Clostridia strains in Treg induction with an ecological model to identify and rank different stable combinations for their ability to promote Treg activation. Importantly, they introduce and validate a computational metric called Treg Induction Score (TrIS) to facilitate the systematic selection of immunomodulatory strains. Interestingly, at least part of AhR’s anti-inflammatory effects can be attributed to its in vivo role as a potent Treg inducer (Goettel et al., 2016; Ye et al., 2017), an angle that was not pursued by Stein et al. (2018). In this regard, characterization of each of the 11 Clostridia strains for their potential to synthesize AhR ligands could further provide a thorough mechanistic basis of their Treg inducing capabilities, as well as, potentially help fine-tuning the microbial consortia for optimal activity. Therefore, while such studies constitute big steps in the direction of precision probiotics, integrating them with metabolic models and machine learning tools can yield insights into metabolites beyond the extensively investigated SCFAs, potentially paving ways to decipher the functional aspects of “large metabolic unknowns” of the gut microbial world. Furthermore, the precision aspect can be strengthened by gaining individual-specific understanding of the host physiology. An illustration of a systematic approach to integrate multiple omics datasets, metabolic models and machine learning to screen and make personalized probiotic recommendations targeting IBD is shown in Figure 1. Although the focus here is on the microbiome-host interactions in gut environment, a similar approach can be extended to such interactions in skin, oral and vaginal microbiome.
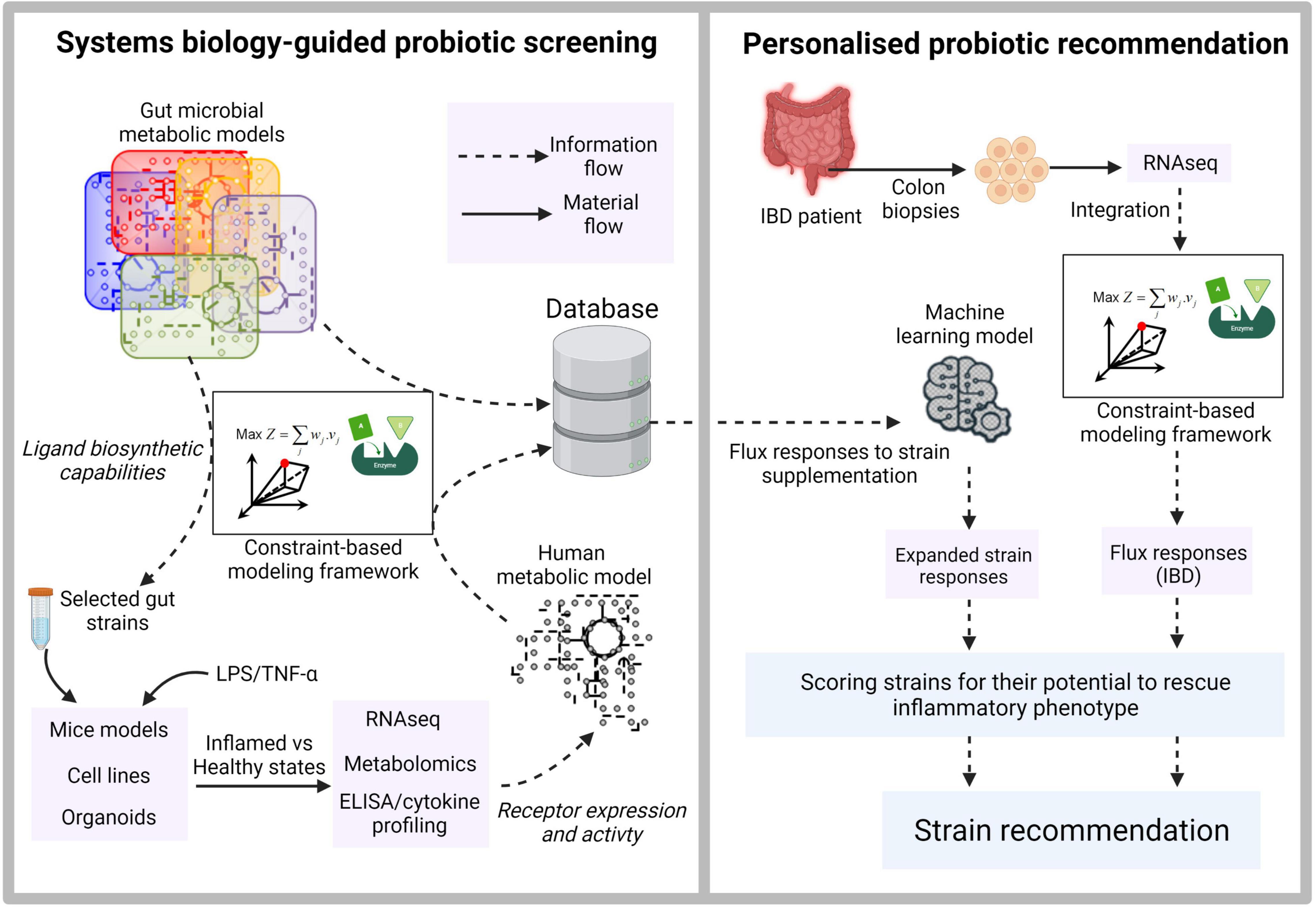
Figure 1. Illustration of systems biology-guided personalized probiotic recommendation. The first stage consists of constraint-based model-driven screening of gut microbial strains for the production of diverse ligands capable of binding to human receptors. The secretory metabolites from selected strains are then used to treat mice models, cell lines or organoids. Their inflamed and healthy states are quantified using inflammatory cytokine profiling. Paired RNAseq and metabolomic datasets derived from each probiotic supplementation scenario provide information on the expression and activity of the target receptors and serve as constraints to derive context-specific models from a generic human genome-scale metabolic network. The context-specific models are used to derive flux responses corresponding to the supplementation of each probiotic strain. The second stage involves training a machine learning model using the extensive flux response information derived in the first stage. RNAseq data derived from real IBD patients for whom the probiotic recommendations are to be made is used to generate a context-specific model and the corresponding metabolic flux distribution. This flux distribution is then used as an input to the trained machine learning model to rank strains based on their ability to rescue the inflammatory phenotype.
Conclusion
The conventional top-down approach of probiotic discovery largely relies on correlations between microbiota composition in gut or fermentative food and health or disease (Veiga et al., 2020). Although this approach has been successful in recommending candidate strains to promote gut health, the absence of a mechanistic basis limits their application to “generic” probiotic supplements. Inflammatory disorders such as IBD and colorectal cancer, being complex manifestations of unhealthy gut and microbiota dysbiosis extensively depend on host-genetic, age, diet and other environmental factors, require precision probiotic targeting. In addition, although gut microbial metabolites such as the AhR ligands have shown tremendous potential in ameliorating inflammation, their exact role might vary depending on the cells, tissue and organs expressing AhR (Cannon et al., 2022). The bottom-up approach guided by systems biology proposed here involves a two-pronged strategy – comprehensive characterization of receptor activating potential of strains and cell/tissue-specific pleiotropic signaling effects downstream to receptor activation in humans. We reason that the future transition toward precision probiotics thus lies in deciphering ligand-receptor interactions, with AhR being a key mediator in managing chronic inflammation.
Data Availability Statement
The original contributions presented in the study are included in the article/supplementary material, further inquiries can be directed to the corresponding authors.
Author Contributions
LK drafted the manuscript. ML, SH, D-YL, YL, and DO edited and proof-read the manuscript. All authors made a substantial contribution to the work and approved it for publication.
Funding
This research was supported by the Agency for Science, Technology and Research (A*STAR), Singapore, and was also funded by the Industry Alignment Fund (Pre-Positioning) Food Structure Engineering for Nutrition and Health (FSENH) programme (H18/01/a0/C11) and by the Singapore Food Story R&D Programme theme 2: Future Foods- Alternative proteins (W20W2D0005). D-YL is supported by the National Research Foundation of Korea (NRF) grant funded by the Korea government (MSIT) (No. 2020R1A2C2007192).
Conflict of Interest
The authors declare that the research was conducted in the absence of any commercial or financial relationships that could be construed as a potential conflict of interest.
Publisher’s Note
All claims expressed in this article are solely those of the authors and do not necessarily represent those of their affiliated organizations, or those of the publisher, the editors and the reviewers. Any product that may be evaluated in this article, or claim that may be made by its manufacturer, is not guaranteed or endorsed by the publisher.
References
Abdulla, O. A., Neamah, W., Sultan, M., Alghetaa, H. K., Singh, N., Busbee, P. B., et al. (2021). The Ability of AhR Ligands to Attenuate Delayed Type Hypersensitivity Reaction Is Associated With Alterations in the Gut Microbiota. Front. Immunol. 12:2505. doi: 10.3389/fimmu.2021.684727
Agus, A., Planchais, J., and Sokol, H. (2018). Gut Microbiota Regulation of Tryptophan Metabolism in Health and Disease. Cell Host Microbe 23, 716–724. doi: 10.1016/j.chom.2018.05.003
Armingol, E., Officer, A., Harismendy, O., and Lewis, N. E. (2021). Deciphering cell–cell interactions and communication from gene expression. Nat. Rev. Genet. 22, 71–88. doi: 10.1038/s41576-020-00292-x
Cannon, A. S., Nagarkatti, P. S., and Nagarkatti, M. (2022). Targeting AhR as a Novel Therapeutic Modality against Inflammatory Diseases. Int. J. Mol. Sci. 23:288. doi: 10.3390/ijms23010288
Chan, S. H. J., Simons, M. N., and Maranas, C. D. (2017). SteadyCom: predicting microbial abundances while ensuring community stability. PLoS Comput. Biol. 13:e1005539. doi: 10.1371/journal.pcbi.1005539
Chang, C.-S., and Kao, C.-Y. (2019). Current understanding of the gut microbiota shaping mechanisms. J. Biomed. Sci. 26:59. doi: 10.1186/s12929-019-0554-5
Chen, H., Nwe, P.-K., Yang, Y., Rosen, C. E., Bielecka, A. A., Kuchroo, M., et al. (2019). A forward chemical genetic screen reveals gut microbiota metabolites that modulate host physiology. Cell 177, 1217–1231. doi: 10.1016/j.cell.2019.03.036
Denison, M. S., Soshilov, A. A., He, G., Degroot, D. E., and Zhao, B. (2011). Exactly the same but different: promiscuity and diversity in the molecular mechanisms of action of the aryl hydrocarbon (dioxin) receptor. Toxicol. Sci. 124, 1–22. doi: 10.1093/toxsci/kfr218
Diener, C., Gibbons, S. M., and Resendis-Antonio, O. (2020). MICOM: metagenome-scale modeling to infer metabolic interactions in the gut microbiota. MSystems 5, e606–e619. doi: 10.1128/mSystems.00606-19
dos Santos, V. M., Müller, M., and de Vos, W. M. (2010). Systems biology of the gut: the interplay of food, microbiota and host at the mucosal interface. Curr. Opin. Biotechnol. 21, 539–550. doi: 10.1016/j.copbio.2010.08.003
Fiocchi, C., and Iliopoulos, D. (2021). IBD Systems Biology Is Here to Stay. Inflamm. Bowel Dis. 27, 760–770. doi: 10.1093/ibd/izaa343
Fukumoto, S., Toshimitsu, T., Matsuoka, S., Maruyama, A., Oh-Oka, K., Takamura, T., et al. (2014). Identification of a probiotic bacteria-derived activator of the aryl hydrocarbon receptor that inhibits colitis. Immunol. Cell Biol. 92, 460–465. doi: 10.1038/icb.2014.2
Geng, J., Ji, B., Li, G., López-Isunza, F., and Nielsen, J. (2021). CODY enables quantitatively spatiotemporal predictions on in vivo gut microbial variability induced by diet intervention. Proc. Natl. Acad. Sci. 118:e2019336118. doi: 10.1073/pnas.2019336118
Goettel, J., Gandhi, R., Kenison, J., Yeste, A., Murugaiyan, G., Sambanthamoorthy, S., et al. (2016). AHR activation is protective against colitis driven by T cells in humanized mice. Cell Rep. 17, 1318–1329. doi: 10.1016/j.celrep.2016.09.082
Gutiérrez-Vázquez, C., and Quintana, F. J. (2018). Regulation of the Immune Response by the Aryl Hydrocarbon Receptor. Immunity 48, 19–33. doi: 10.1016/j.immuni.2017.12.012
Harcombe, W. R., Riehl, W. J., Dukovski, I., Granger, B. R., Betts, A., Lang, A. H., et al. (2014). Metabolic resource allocation in individual microbes determines ecosystem interactions and spatial dynamics. Cell Rep. 7, 1104–1115. doi: 10.1016/j.celrep.2014.03.070
Heinken, A., Hertel, J., and Thiele, I. (2021). Metabolic modelling reveals broad changes in gut microbial metabolism in inflammatory bowel disease patients with dysbiosis. NPJ Syst. Biol. Appl. 7:19. doi: 10.1038/s41540-021-00178-6
Heirendt, L., Arreckx, S., Pfau, T., Mendoza, S. N., Richelle, A., Heinken, A., et al. (2019). Creation and analysis of biochemical constraint-based models using the COBRA Toolbox v. 3.0. Nat. Protoc. 14, 639–702. doi: 10.1038/s41596-018-0098-2
Hendler, R., and Zhang, Y. (2018). Probiotics in the Treatment of Colorectal Cancer. Medicines 5:101. doi: 10.3390/medicines5030101
Jin, U.-H., Cheng, Y., Park, H., Davidson, L. A., Callaway, E. S., Chapkin, R. S., et al. (2017). Short chain fatty acids enhance aryl hydrocarbon (Ah) responsiveness in mouse colonocytes and Caco-2 human colon cancer cells. Sci. Rep. 7:10163. doi: 10.1038/s41598-017-10824-x
Kamada, N., Seo, S. U., Chen, G. Y., and Núñez, G. (2013). Role of the gut microbiota in immunity and inflammatory disease. Nat. Rev. Immunol. 13, 321–335. doi: 10.1038/nri3430
Koduru, L., Lakshmanan, M., Lim, P.-Y., Ho, P.-L., Banu, M., Park, D.-S., et al. (2021). Systematic evaluation of genome-wide metabolic landscapes in lactic acid bacteria reveals diet-induced and strain-specific probiotic idiosyncrasies. BioRxiv [preprint]. doi: 10.1101/2021.06.20.449192
Lamas, B., Richard, M. L., Leducq, V., Pham, H. P., Michel, M. L., da Costa, G., et al. (2016). CARD9 impacts colitis by altering gut microbiota metabolism of tryptophan into aryl hydrocarbon receptor ligands. Nat. Med. 22, 598–605. doi: 10.1038/nm.4102
Lewis, J. E., and Kemp, M. L. (2021). Integration of machine learning and genome-scale metabolic modeling identifies multi-omics biomarkers for radiation resistance. Nat. Commun. 12:2700. doi: 10.1038/s41467-021-22989-1
Lieven, C., Beber, M. E., Olivier, B. G., Bergmann, F. T., Ataman, M., Babaei, P., et al. (2020). MEMOTE for standardized genome-scale metabolic model testing. Nat. Biotechnol. 38, 272–276.
Machado, D., Andrejev, S., Tramontano, M., and Patil, K. R. (2018). Fast automated reconstruction of genome-scale metabolic models for microbial species and communities. Nucleic Acids Res. 46, 7542–7553. doi: 10.1093/nar/gky537
Magnúsdóttir, S., Heinken, A., Kutt, L., Ravcheev, D. A., Bauer, E., Noronha, A., et al. (2017). Generation of genome-scale metabolic reconstructions for 773 members of the human gut microbiota. Nat. Biotechnol. 35, 81–89. doi: 10.1038/nbt.3703
Malik-Sheriff, R. S., Glont, M., Nguyen, T. V. N., Tiwari, K., Roberts, M. G., Xavier, A., et al. (2020). BioModels—15 years of sharing computational models in life science. Nucleic Acids Res. 48, D407–D415. doi: 10.1093/nar/gkz1055
Marinelli, L., Martin-Gallausiaux, C., Bourhis, J. M., Béguet-Crespel, F., Blottière, H. M., and Lapaque, N. (2019). Identification of the novel role of butyrate as AhR ligand in human intestinal epithelial cells. Sci. Rep. 9:643. doi: 10.1038/s41598-018-37019-2
Melhem, H., Kaya, B., Ayata, C. K., Hruz, P., and Niess, J. H. (2019). Metabolite-sensing G protein-coupled receptors connect the diet-microbiota-metabolites axis to inflammatory bowel disease. Cells 8:450. doi: 10.3390/cells8050450
Mohandas, S., and Vairappan, B. (2017). Role of pregnane X-receptor in regulating bacterial translocation in chronic liver diseases. World J. Hepatol. 9:1210. doi: 10.4254/wjh.v9.i32.1210
Noronha, A., Modamio, J., Jarosz, Y., Guerard, E., Sompairac, N., Preciat, G., et al. (2019). The Virtual Metabolic Human database: integrating human and gut microbiome metabolism with nutrition and disease. Nucleic Acids Res. 47, D614–D624. doi: 10.1093/nar/gky992
Norsigian, C. J., Pusarla, N., McConn, J. L., Yurkovich, J. T., Dräger, A., Palsson, B. O., et al. (2020). BiGG Models 2020: multi-strain genome-scale models and expansion across the phylogenetic tree. Nucleic Acids Res. 48, D402–D406. doi: 10.1093/nar/gkz1054
Özçam, M., Tocmo, R., Oh, J. H., Afrazi, A., Mezrich, J. D., Roos, S., et al. (2019). Gut symbionts Lactobacillus reuteri R2lc and 2010 encode a polyketide synthase cluster that activates the mammalian aryl hydrocarbon receptor. Appl. Environ. Microbiol. 85, e1661–e1668. doi: 10.1128/AEM.01661-18
Roager, H. M., and Licht, T. R. (2018). Microbial tryptophan catabolites in health and disease. Nat. Commun. 9:3294. doi: 10.1038/s41467-018-05470-4
Seaver, S. M. D., Liu, F., Zhang, Q., Jeffryes, J., Faria, J. P., Edirisinghe, J. N., et al. (2021). The ModelSEED Biochemistry Database for the integration of metabolic annotations and the reconstruction, comparison and analysis of metabolic models for plants, fungi and microbes. Nucleic Acids Res. 49, D575–D588.
Shao, X., Liao, J., Li, C., Lu, X., Cheng, J., and Fan, X. (2021). CellTalkDB: a manually curated database of ligand–receptor interactions in humans and mice. Brief. Bioinform. 22:bbaa269. doi: 10.1093/bib/bbaa269
Shoaie, S., Ghaffari, P., Kovatcheva-Datchary, P., Mardinoglu, A., Sen, P., Pujos-Guillot, E., et al. (2015). Quantifying diet-induced metabolic changes of the human gut microbiome. Cell Metab. 22, 320–331. doi: 10.1016/j.cmet.2015.07.001
Stein, R. R., Tanoue, T., Szabady, R. L., Bhattarai, S. K., Olle, B., Norman, J. M., et al. (2018). Computer-guided design of optimal microbial consortia for immune system modulation. Elife 7, e30916. doi: 10.7554/eLife.30916
Takamura, T., Harama, D., Fukumoto, S., Nakamura, Y., Shimokawa, N., Ishimaru, K., et al. (2011). Lactobacillus bulgaricus OLL1181 activates the aryl hydrocarbon receptor pathway and inhibits colitis. Immunol. Cell Biol. 89, 817–822. doi: 10.1038/icb.2010.165
Valentini, M., Piermattei, A., di Sante, G., Migliara, G., Delogu, G., and Ria, F. (2014). Immunomodulation by gut microbiota: role of Toll-like receptor expressed by T cells. J. Immunol. Res. 2014:586939. doi: 10.1155/2014/586939
Veiga, P., Suez, J., Derrien, M., and Elinav, E. (2020). Moving from probiotics to precision probiotics. Nat. Microbiol. 5, 878–880. doi: 10.1038/s41564-020-0721-1
Venkatesh, M., Mukherjee, S., Wang, H., Li, H., Sun, K., Benechet, A. P., et al. (2014). Symbiotic bacterial metabolites regulate gastrointestinal barrier function via the xenobiotic sensor PXR and Toll-like receptor 4. Immunity 41, 296–310. doi: 10.1016/j.immuni.2014.06.014
Wang, H., Marcišauskas, S., Sánchez, B. J., Domenzain, I., Hermansson, D., Agren, R., et al. (2018). RAVEN 2.0: a versatile toolbox for metabolic network reconstruction and a case study on Streptomyces coelicolor. PLoS Comput. Biol. 14:e1006541. doi: 10.1371/journal.pcbi.1006541
Wang, H., Robinson, J. L., Kocabas, P., Gustafsson, J., Anton, M., Cholley, P.-E., et al. (2021). Genome-scale metabolic network reconstruction of model animals as a platform for translational research. Proc. Natl. Acad. Sci. 118:e2102344118. doi: 10.1073/pnas.2102344118
Yang, J. H., Wright, S. N., Hamblin, M., McCloskey, D., Alcantar, M. A., Schrübbers, L., et al. (2019). A white-box machine learning approach for revealing antibiotic mechanisms of action. Cell 177, 1649–1661. doi: 10.1016/j.cell.2019.04.016
Yang, W., Yu, T., Huang, X., Bilotta, A. J., Xu, L., Lu, Y., et al. (2020). Intestinal microbiota-derived short-chain fatty acids regulation of immune cell IL-22 production and gut immunity. Nat. Commun. 11:4457. doi: 10.1038/s41467-020-18262-6
Ye, J., Qiu, J., Bostick, J. W., Ueda, A., Schjerven, H., Li, S., et al. (2017). The aryl hydrocarbon receptor preferentially marks and promotes gut regulatory T cells. Cell Rep. 21, 2277–2290. doi: 10.1016/j.celrep.2017.10.114
Zelante, T., Iannitti, R. G., Cunha, C., DeLuca, A., Giovannini, G., Pieraccini, G., et al. (2013). Tryptophan catabolites from microbiota engage aryl hydrocarbon receptor and balance mucosal reactivity via interleukin-22. Immunity 39, 372–385. doi: 10.1016/j.immuni.2013.08.003
Zhao, C., Hu, X., Bao, L., Wu, K., Feng, L., Qiu, M., et al. (2021). Aryl hydrocarbon receptor activation by Lactobacillus reuteri tryptophan metabolism alleviates Escherichia coli-induced mastitis in mice. PLoS Pathog. 17:e1009774. doi: 10.1371/journal.ppat.1009774
Zimmermann, J., Kaleta, C., and Waschina, S. (2021). gapseq: informed prediction of bacterial metabolic pathways and reconstruction of accurate metabolic models. Genom. Biol. 22:81. doi: 10.1186/s13059-021-02295-1
Keywords: systems biology, gut microbiota, inflammatory disorders, probiotics, postbiotics
Citation: Koduru L, Lakshmanan M, Hoon S, Lee D-Y, Lee YK and Ow DS-W (2022) Systems Biology of Gut Microbiota-Human Receptor Interactions: Toward Anti-inflammatory Probiotics. Front. Microbiol. 13:846555. doi: 10.3389/fmicb.2022.846555
Received: 31 December 2021; Accepted: 11 February 2022;
Published: 03 March 2022.
Edited by:
Qi Zhao, University of Science and Technology Liaoning, ChinaReviewed by:
Liang Wang, Xuzhou Medical University, ChinaCopyright © 2022 Koduru, Lakshmanan, Hoon, Lee, Lee and Ow. This is an open-access article distributed under the terms of the Creative Commons Attribution License (CC BY). The use, distribution or reproduction in other forums is permitted, provided the original author(s) and the copyright owner(s) are credited and that the original publication in this journal is cited, in accordance with accepted academic practice. No use, distribution or reproduction is permitted which does not comply with these terms.
*Correspondence: Lokanand Koduru, kodurul@imcb.a-star.edu.sg; Dave Siak-Wei Ow, dave_ow@bti.a-star.edu.sg