- 1Department of Pathogenic Biology, Binzhou Medical University, Yantai, China
- 2Yantai Yuhuangding Hospital, Yantai, China
- 3Institute of Health and Disease Management, Binzhou Medical University, Yantai, China
- 4 School of Nursing and Rehabilitation, Shandong University, Jinan, China
- 5Clinical Medicine School, Binzhou Medical University, Yantai, China
Growing evidence has demonstrated that hypertension was associated with dysbiosis of intestinal flora. Since intestinal microbes could critically regulate neurofunction via the intestinal–brain axis, the study aimed to reveal the role and prediction value of intestinal flora alteration in hypertension-associated cognitive impairment. A cohort of 97 participants included 63 hypertension patients and 34 healthy controls. The structure of intestinal flora was analyzed by V3–V4 16S rRNA amplicon sequencing. The cognitive function was assessed using the Montreal Cognitive Assessment (MoCA) scale, and 31 patients were considered to have cognitive impairment (MoCA < 26). Patients with cognitive impairment had considerable alterations in intestinal flora structure, composition, and function compared with normal-cognitive patients. In particular, the abundance of LPS-containing taxa (Proteobacteria, Gammaproteobacteria, Enterobacterales, Enterobacteriaceae, and Escherichia–Shigella) and SCFA-producing taxon (Prevotella) significantly changed in cognition-impaired patients. Tax4Fun predication results showed downregulation of glycan biosynthesis and metabolism in hypertension patients with cognitive impairment. Additionally, the pathway was demonstrated to be significantly correlated with LPS-containing taxa (Proteobacteria, Gammaproteobacteria, Enterobacterales, Enterobacteriaceae, and Escherichia–Shigella) and SCFA-producing taxon Prevotella. Furthermore, the taxa-based multiple joint prediction model (9×) was demonstrated to have excellent diagnostic potential for cognitive impairment of hypertension patients (AUC = 0.944). The current study revealed the involvement of intestinal microbiota dysbiosis in cognition-impaired hypertension patients and provided an objective predictive index for this cognition disorder.
Introduction
Hypertension is a worldwide public health issue. A recent study reported that the number of adults aged 30–79 with hypertension has increased from 650 million to 1.28 billion in the past 30 years (NCD Risk Factor Collaboration, 2021). It is well known that hypertension could lead to multi-system complications, including heart failure, chronic kidney disease, and mild cognitive impairment. Noteworthy, epidemiological studies found that hypertension is an important risk factor of cognitive function impairment, which significantly increased the incidence of Alzheimer’s disease (AD) and vascular dementia (Qiu et al., 2005; Abete et al., 2014). Previous studies revealed that the neuropathological features of AD, such as the number of amyloid plaques and nerve fiber tangles, were significantly increased in the cerebral cortex and hippocampus of patients with chronic hypertension (Petrovitch et al., 2000). To date, effective or disease-modifying drugs against AD are not available since the pathological factors of AD are still unclear. The lack of efficient therapy for AD has put forward the emphasis of treatments on people at risk of dementia, such as mild cognitive impairment condition (Akbari et al., 2016). Hence, it is necessary to investigate the pathogenesis of mild cognitive impairment induced by hypertension, thereby promoting strategies for this cognitive decline.
Recently, ample research has solidly proved the association of intestinal flora dysbiosis with hypertension. Yang et al. (2015) reported that the abundance and diversity of intestinal flora in hypertension patients were significantly decreased, and the structure of intestinal flora was significantly different from those of healthy people. Besides, specific metabolic pathways were considered to be relevant to hypertension (Koh et al., 2016; Sawicki et al., 2017). It is worth noting that specific functions of intestinal flora, such as those involved in short-chain fatty acid (SCFA) metabolism (Huart et al., 2019; Oyama and Node, 2019; Yang F. et al., 2020; Wu et al., 2021) and lipopolysaccharide (LPS) content (Lorenzoni and Wideman, 2008; Mell et al., 2015; Yan et al., 2017; Dubinski et al., 2021), were found to be associated with both hypertension and certain cognitive impairment diseases. Cumulative evidence indicated the pivotal role of intestinal flora in regulating brain function through the microbe–gut–brain axis (Westfall et al., 2017; Zhu et al., 2017). Imbalance of intestinal flora can lead to nervous diseases such as AD (Liu et al., 2019), ischemic stroke (Ling et al., 2020; Liu et al., 2020), and Parkinson’s disease (Sampson et al., 2016; Pietrucci et al., 2019). Hence, we hypothesized that dysbiosis of the gut microbiota might be involved in the process of hypertension-associated cognitive impairment.
The present study investigated the alteration of the intestinal microbiota and cognition by 16S rRNA amplicon sequencing and Montreal Cognitive Assessment, respectively. Moreover, we analyzed the correlation of the significantly changed specific intestinal taxa with the score of cognitive function scale among hypertension patients. Furthermore, we established a bacteria-based biological predictive model for hypertension-associated cognitive impairment, which enables sensitive diagnosis of cognitive impairment of hypertension patients, and therefore facilitates the potential novel therapy targeting at regaining gut microbiota homeostasis.
Materials and Methods
Study Cohort and Patient Characteristics
Hypertension patients were recruited from the Affiliated Hospital of Binzhou Medical University, Yantai, China, during December 2019 to June 2020. The research was approved and supervised by the Institution Review Board of Binzhou Medical University (No. 2018-085). Each subject signed a written informed consent voluntarily before enrollment. Every participant enrolled in the hypertension patients group met the following criteria: According to Chinese Guidelines for Prevention and Treatment of Hypertension (2018 Revised Version) (Joint Committee for Guideline Revision, 2019), (i) Without using antihypertensive drugs, systolic blood pressure ≤ 140 mmHg and/or diastolic blood pressure ≤ 90 mmHg; (ii) With a history of hypertension, and using antihypertensive drugs at present, even if blood pressure < 140/90 mmHg were included. Meanwhile, spouses of hypertension patients were voluntarily included as the Control group, which aimed to mitigate the impact of dietary and lifestyle habits in blood pressure. The exclusion criteria included the following: (i) current or history of neurological or chronic psychiatric disorder, schizophrenia, brain injury or stroke, major depressive disorder, or severe anxiety disorders requiring pharmacotherapy; (ii) suffering from digestive system diseases, diabetes, respiratory diseases, nervous system diseases such as AD and Parkinson’s disease, or other severe primary diseases; (iii) with severe hearing, and visual or motor deficits that may interfere with cognitive tests; (iv) treatment with antibiotics, probiotics, or prebiotics within 1 month; and (v) current or history of substance dependence. Ultimately, 63 hypertension patients and 34 healthy people were recruited in our research.
Cognitive Function Assessment
The Chinese versions of the Montreal Cognitive Assessment (MoCA) translated by Yu et al. (2012) was used to assess cognitive function (Nasreddine et al., 2005), which consists of seven domains of cognitive functional performance including visual space and executive function, naming, attention, language, abstract thinking, delayed memory, and orientation. Cronbach’s α coefficient of this MoCA scale is 0.88. The total score is 30 points (adding one point to the MoCA total score of individuals with ≤ 12 years of education). A score of MoCA ≥ 26 has been identified as “normal” cognition, and a score of MoCA < 26 indicates cognitive impairment (Nasreddine et al., 2005; Copersino et al., 2009).
V3–V4 16S rRNA Gene Sequencing Analysis
Fecal samples were freshly collected and stored at –80°C for subsequent sequencing analysis. Total genome DNA from samples was extracted using CTAB protocol. 16S rRNA genes’ V3–V4 regions were amplified used specific forward primer 341F (5′-CCTAYGGGRBGCASCAG-3′) and reverse primer 806R (5′-GGACTACNNGGGTATCTAAT-3′) with the barcode. All PCR reactions were carried out with Phusion ® High-Fidelity PCR Master Mix (New England Biolabs, Hitchin, United Kingdom). Thermal cycling consisted of initial denaturation at 98°C for 1 min, followed by 30 cycles of denaturation at 98°C for 10 s, annealing at 50°C for 30 s, and elongation at 72°C for 30 s, and, finally, 72°C for 5 min. Then, a mixture of PCR products was purified with Qiagen Gel Extraction Kit (Qiagen, Hilden, Germany). TruSeq ® DNA PCR-Free Sample Preparation Kit (Illumina, San Diego, CA, United States) was used to generate sequencing libraries. The library quality was assessed on the Qubit@ 2.0 Fluorometer (Thermo Fisher Scientific, MA, United States) and Agilent Bioanalyzer 2100 system (Agilent, Santa Clara, CA, United States), then sequenced on an Illumina NovaSeq6000 platform (Novogene, Cambridge, United Kingdom) and 250-bp paired-end reads were generated. Sequence analyses were performed by Uparse software (version 7.0.1001) (Edgar, 2013), which, with ≥ 97% similarity, were assigned to the same OTUs. For each representative sequence, the SSUrRNA of the SILVA database (version 138.1) (Quast et al., 2013) was used based on Mothur software (version 1.46.1) to annotate taxonomic information (Schloss et al., 2009). In order to study phylogenetic relationship of different OTUs, and the difference of the dominant species in different samples or groups, multiple sequence alignment was conducted using the MUSCLE software (version 3.8.31) (Edgar, 2004). QIIME software (version 1.9.1) was employed to calculate the alpha and beta diversity. Venn diagrams and rarefaction curves were plotted using R software (version 2.15.3). Principal coordinate analysis (PCoA) was performed to obtain principal coordinates and visualize complex multidimensional data, which were displayed by WGCNA package, stat packages, vegan package, and ggplot2 package in R software (version 2.15.3) (Zuo et al., 2021). Anosim analysis was performed using vegan package in R software (version 2.15.3).
Analysis of Composition and Function Differences
In order to find species with significant differences among groups, MetaStat analysis was performed using R software (version 2.15.3), and the relative abundance differences of intestinal flora at the level of phylum, class, order, family, and genus were compared. LEfSe software (version 1.0) was used for linear discriminant analysis effect size analysis, and the linear discriminant analysis (LDA) value was set to 4 (Segata et al., 2011). Based on the 16S SILVA database, the function of intestinal flora prediction was analyzed by Tax4Fun package of R software (Aßhauer et al., 2015). The 16S rRNA gene sequences extracted from KEGG prokaryote genome database1 were aligned to the SILVA SSU Ref NR 99 database2 by BLASTN algorithm, and then the functional information of KEGG prokaryote genome database was mapped to the SILVA database for functional annotation.
Statistical Analysis
The data were presented as the mean ± standard deviations (SDs). The distribution data were evaluated by Shapiro–Wilk test. Normally distributed continuous variables were analyzed using one-way analysis of variance (ANOVA) followed by Fisher’s Least Significant Difference (LSD) multiple comparisons. Non-normally distributed continuous variables were assessed by Welch’s ANOVA, and the post hoc multiple comparisons were performed using the Games–Howell test. Correlations between intestinal taxa and MoCA performance were analyzed using Spearman or Pearson correlation analysis, as appropriate. To choose the optimal model for diagnosis of cognitive impairment of hypertension patients, receiver operating characteristic (ROC) area under the curve was estimated. The Youden index was used to determine the best cutoff of the ROC curve (Fluss et al., 2005). The statistical significance was determined at p-value < 0.05. SPSS 22.0 software (IBM, Chicago, IL, United States) was used to perform the statistical analysis. Images were generated by Adobe Illustrate (version 2018CC) and R (version 4.1.1).
Results
Demographic Characteristics and Cognitive Function Analysis
The present study recruited a total of 97 subjects, including 63 hypertension patients and 32 healthy individuals (the Control group). Of the hypertension patients, 31 subjects were determined to have cognitive impairment (MoCA < 26) (the MCI group), while 32 hypertension patients were determined to have no cognitive impairment (the NMCI group). The demographic characteristics of the MCI group, NMCI group, and Control groups are presented in Table 1. There were no significant differences in age, gender, education years, and BMI indexes (p > 0.05).
Montreal Cognitive Assessment results are shown in Table 2; the total score of MoCA and the scores of specific function domains including visuospatial and executive function, attention, language, abstraction, delayed recall, and orientation were significantly lower in the MCI group than the NMCI group (p < 0.05), while there was no significant difference in naming domain among three groups (p > 0.05).
Diversity and Functional Difference of Intestinal Flora
Operational Taxonomic Units Cluster Analysis
The raw data were analyzed, followed by splicing, quality control, and chimeric filtration. Ultimately, 9,278,977 reads were generated, and 96.84% (8,985,741) passed the quality control screening. These reads were clustered to 4,940 operational taxonomic units (OTUs). The rarefaction curve for the three groups shows that the number of identified OTUs approached a plateau (Figure 1A), which means that the sequencing depth is enough for analysis. Venn diagram showed that the number of unique OTUs in the MCI group, NMCI group, and Control group was 668, 510, and 603, respectively (Figure 1B).
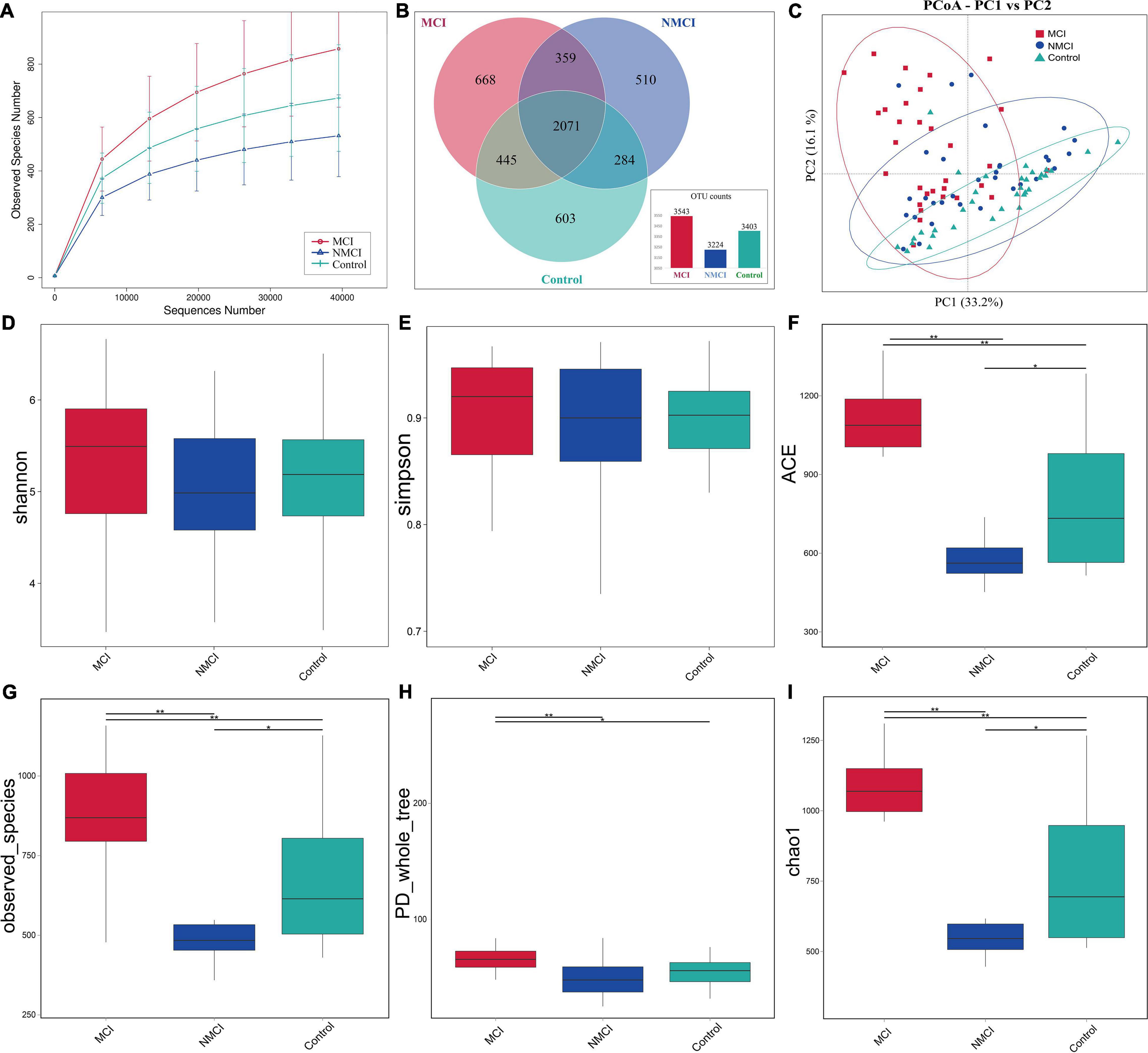
Figure 1. Comparison of the intestinal microbiota richness and diversity among three groups. (A) Rarefaction curve showed the number of identified OTUs approached a plateau, indicated that the sequencing depth is enough for analysis. (B) Venn diagram showing the shared and unique OTUs. (C) PCoA based on weighted UniFrac distance was used to analyze the structure of intestinal flora among three groups (Anosim, p < 0.01): each dot represents a sample, and different colors and shapes indicate the group to which they belong. The result is shown along the first and second axes of the PCoA plot. Numbers in parentheses represent explained variation. Ellipses represent different groups. Shannon (D), Simpson (E), ACE (F), observed_Species (G), PD_whole_tree (H), and Chao1 (I) indexes were used to assess alpha diversity. MCI, hypertension patients with cognitive impairment, NMCI, hypertension patients without cognitive impairment; Control, health control subjects.
Alpha Diversity Analysis
Alpha diversity was evaluated by Chao1, ACE, observed_Species, PD_whole_tree, Shannon, and Simpson indexes. As shown in Figures 1D–I and Table 3, compared with the Control group, Chao1, ACE, and observed_species were highly significantly lower in the NMCI group (p < 0.01). It indicated that microbial community abundance and diversity were significantly lower in hypertension patients than healthy individuals.
Compared with the NMCI group, Chao1, ACE, observed_species, and PD_whole_tree indexes were significantly higher in the MCI group (p < 0.01), indicating a significantly higher microbial community abundance and diversity in cognition-impaired patients than normal-cognition patients. The Shannon and Simpson indexes were lower in the MCI group than the NMCI group, although the difference was not significant.
Beta Diversity Analysis
Principal coordinate analysis based on weighted UniFrac distance was used to analyze the structure of intestinal flora among three groups (Anosim, p < 0.01) (Figure 1C). There was a significant separation trend in the direction of the first principal component axis (PC1) and the second principal component axis (PC2). The first principal component axis (PC1) contribution was 33.2%, and that of the second principal component axis (PC2) was 16.1%.
Differences in the Composition and Function of Intestinal Flora
MetaStat analysis and LDA effect size (LEfSe) analyses were performed to assess the difference in abundance and composition among the three groups. Figure 2A showed a summarized relative abundance of microbial taxa, Figures 2B–X displayed detailed relative abundance of each taxon.
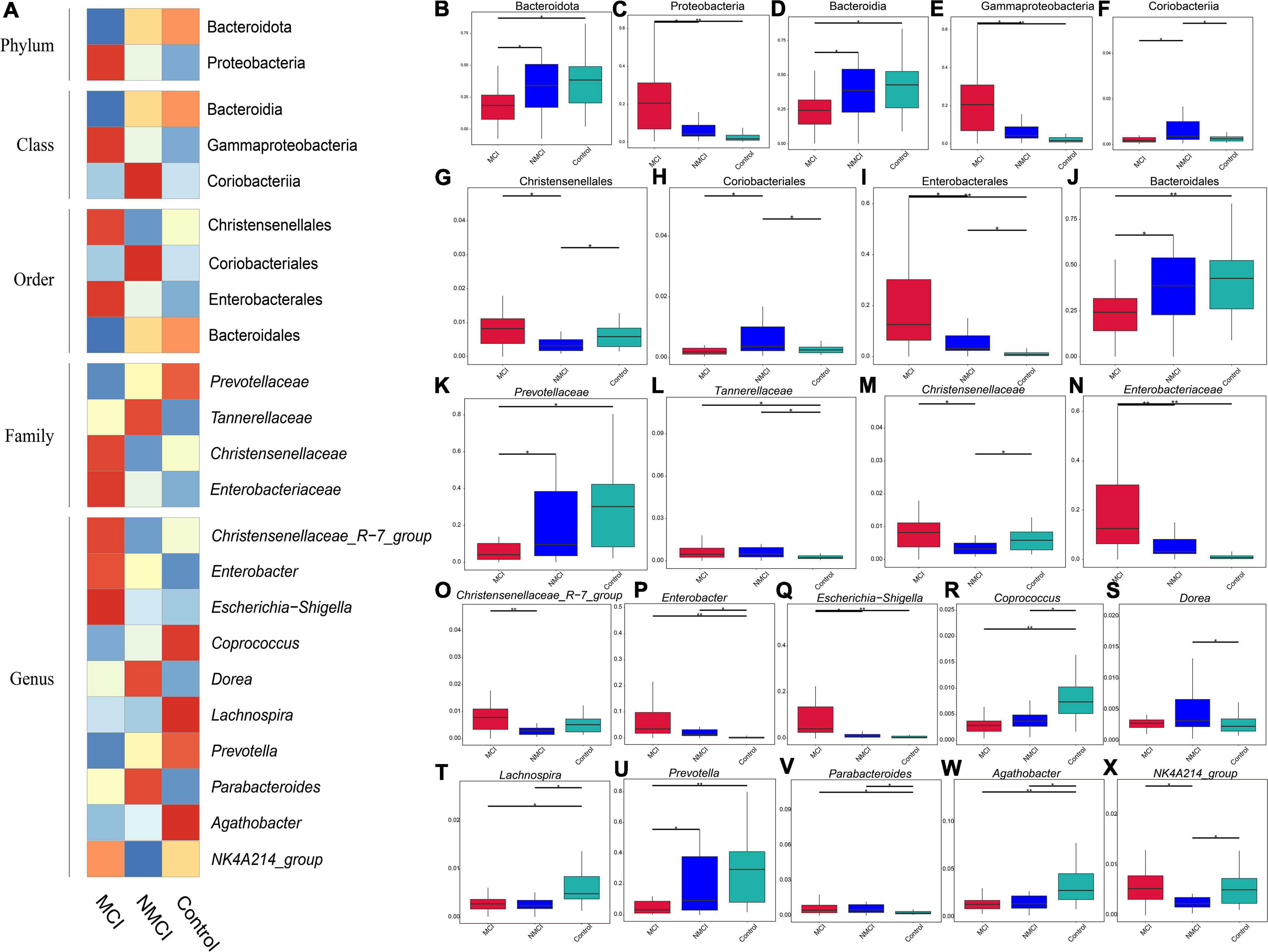
Figure 2. Differences in the abundance of intestinal flora. MetaStat analysis was performed to compare the relative abundance differences of intestinal flora. (A) Heatmap of relative abundance difference. (B,C) Phylum level. (D–F) Class level. (G–J) Order level. (K–N) Family level. (O–X) Genus level. MCI, hypertension patients with cognitive impairment; NMCI, hypertension patients without cognitive impairment; Control, health control subjects.
The results of MetaStat analysis showed that the relative abundance of 9 taxa were significantly higher in the MCI group than in the NMCI group, namely, Proteobacteria, Gammaproteobacteria, Enterobacterales, Christensenellales, Christensenellaceae, Enterobacteriaceae, Christensenellaceae_R-7_Group, Escherichia-Shigella, and NK4A214_group (21.159% vs. 9.006%, 21.104% vs. 8.843%, 19.753% vs. 7.512%, 0.955% vs. 0.438%, 0.955% vs. 0.438%, 19.736% vs. 7.491%, 0.911% vs. 0.364%, 11.40% vs. 1.97%, 0.579% vs. 0.320%; p < 0.01) (see Figures 2C,E,G,I,M–O,Q,X). Besides, the relative abundance of 7 groups was significantly lower in the MCI group than the NMCI group, which were Bacteroidota, Bacteroidia, Coriobacteriia, Coriobacteriales, Bacteroidales, Prevotellaceae, and Prevotella (24.800% vs. 37.094%, 24.800% vs. 37.094%, 0.282% vs. 0.757%, 0.282% vs. 0.757%, 24.784% vs. 37.053%, 8.168% vs. 19.128%, and 7.202% vs. 18.541%; p < 0.01) (see Figures 2B,D,F,H,J,K,U). LEfSe analysis was used to identify the key phenotypes, according to LDA scores, Phylum: Proteobacteria; Class: Gammaproteobacteria; Order: Enterobacterales; Family: Enterobacteriaceae; Genus: Escherichia–Shigella, Enterobacter; Species: Klebsiella quasipneumoniae, Bacteroides vulgatus, and Escherichia coli were distinct in the MCI group (see Figures 3A,B).
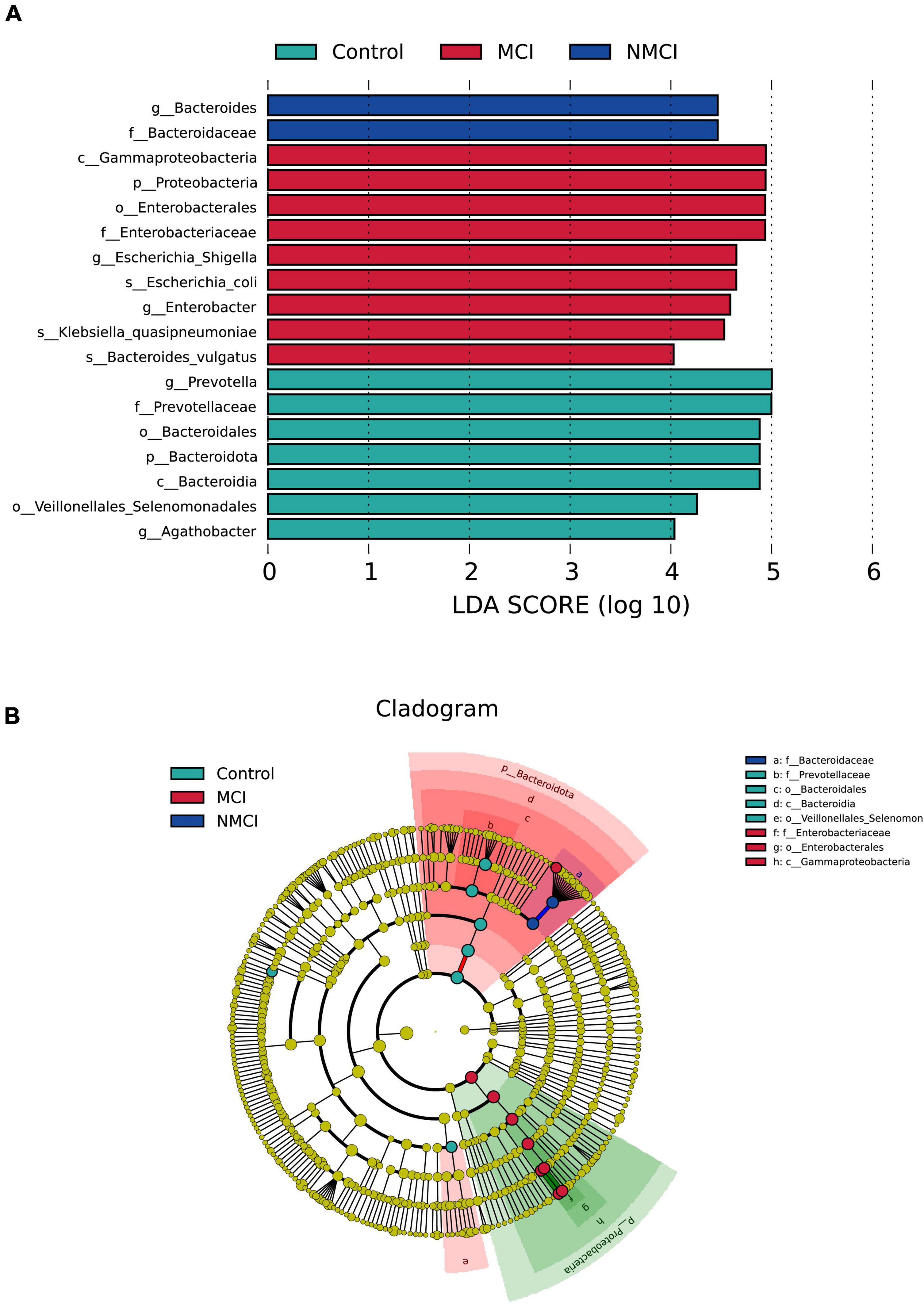
Figure 3. Differences in the composition of intestinal flora. Linear discriminant analysis (LDA) effect size (LEfSe) analyses were performed to assess the difference in composition among the three groups. (A) LDA results showed the distinct taxa. (B) LEfSe results indicating the phylogenetic distribution of microbiota. Lowercase letters represent different classification levels; p, phylum; c, class; o, order; f, family; g, genus. MCI, hypertension patients with cognitive impairment; NMCI, hypertension patients without cognitive impairment; Control, health control subjects.
To predict the functions of intestinal microflora, Tax4Fun analysis was performed, as shown in Figure 4A, at the second level of KEGG; 24 pathways, such as glycan biosynthesis and metabolism, transcription, environmental adaptation, replication and repair, and amino acid metabolism, were enriched differently between the MCI group and the NMCI group. Specifically, compared with the NMCI group, the glycan biosynthesis and metabolism were significantly weakened (p < 0.05). This pathway was significantly negatively associated with the relative abundance of Proteobacteria (p < 0.001), Gammaproteobacteria (p < 0.001), Enterobacterales (p < 0.001), Enterobacteriaceae (p < 0.001), Escherichia-Shigella (p < 0.05), Enterobacter (p < 0.01), and Klebsiella quasipneumoniae (p < 0.01) and significantly positively associated with the relative abundance of Prevotella (p < 0.001, Figure 4B).
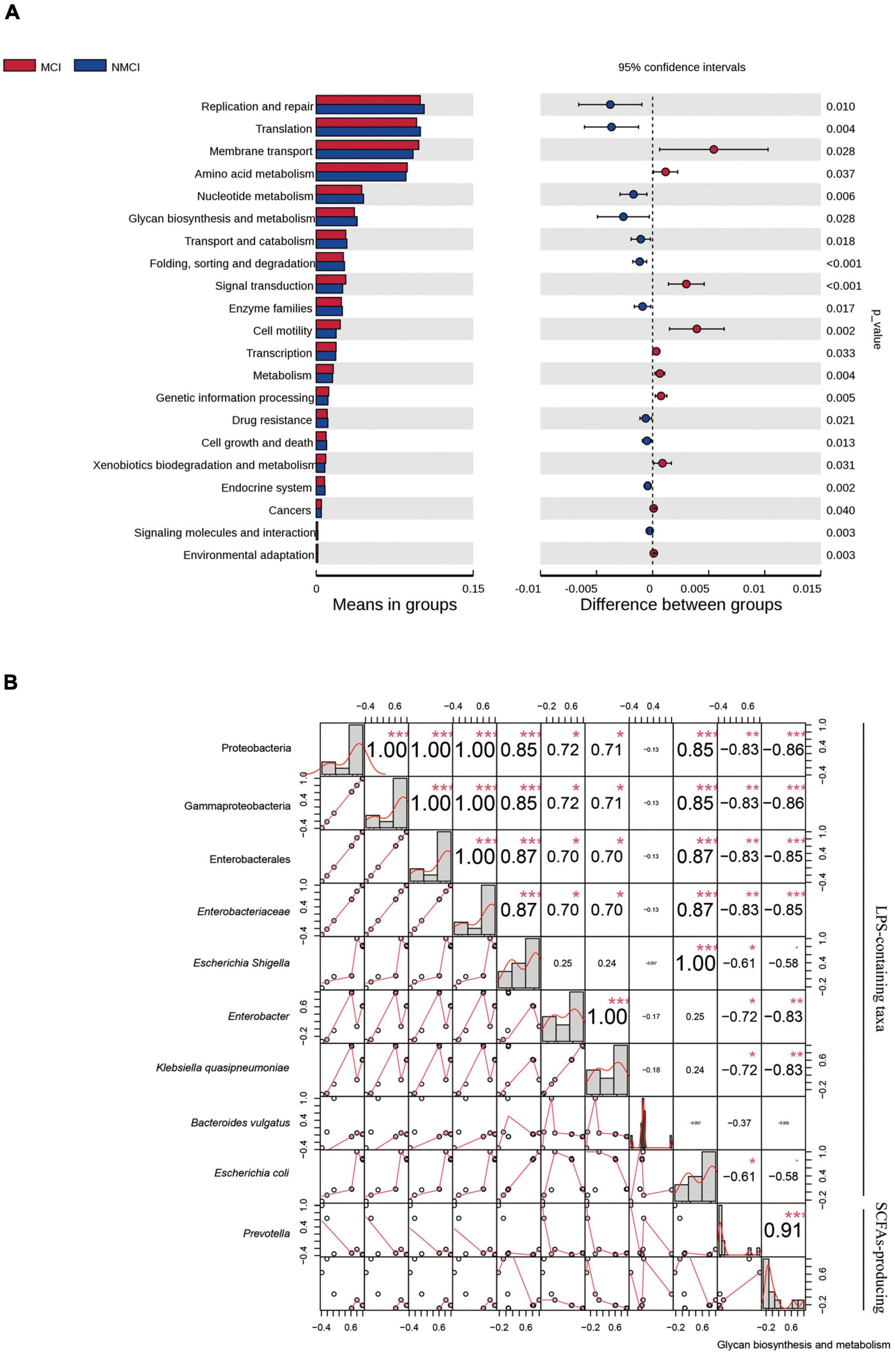
Figure 4. Function prediction and correlation analysis. (A) Function predication was inferred by using Tax4Fun package of R software, and 24 KEGG-level-2 pathways were altered. (B) Pearson correlations among the glycan biosynthesis and metabolism pathway and distinct taxa. Numbers indicated correlation coefficients, and size of points reflected the strength of the correlation. *p < 0.05, **p < 0.01, ***p < 0.001. MCI, hypertension patients with cognitive impairment; NMCI, hypertension patients without cognitive impairment; Control, health control subjects.
Correlation Analysis of Intestinal Taxa With Differential Abundance and Cognitive Function Domains
The result showed that there were nine intestinal microbiota (Proteobacteria, Gammaproteobacteria, Enterobacterales, Enterobacteriaceae, Christensenellales, Christensenellaceae, Christensenellaceae_R-7_group, Escherichia-Shigella, and NK4A214_group) that were significantly negatively correlated to the MoCA total score (p < 0.01), and two intestinal flora microbiota (Coriobacteriia and Coriobacteriales) that were highly positively correlated with the total score of the MoCA (p < 0.01). Our results indicated that these nine specific taxa (e.g., Proteobacteria, Gammaproteobacteria, and Enterobacterales) showed a potential possibility of cognitive impairment in hypertension patients, whereas Coriobacteriia and Coriobacteriales might be beneficial to the cognition of hypertension patients.
In particular, according to our results shown in Figure 5, the attention domain was significantly associated with Proteobacteria (p < 0.05), Gammaproteobacteria (p < 0.05), Enterobacterales (p < 0.05), Enterobacteriaceae (p < 0.05), Coriobacteriia (p < 0.01), Coriobacteriales (p < 0.01), and Escherichia–Shigella (p < 0.01); orientation domain was significantly associated with Coriobacteriia (p < 0.05), Coriobacteriales (p < 0.05), and Escherichia–Shigella (p < 0.01); delayed recall domain was significantly associated with Proteobacteria (p < 0.05), Gammaproteobacteria (p < 0.05), Christensenellales (p < 0.05), Christensenellaceae (p < 0.05), Enterobacterales (p < 0.05), Enterobacteriaceae (p < 0.05), Escherichia–Shigella (p < 0.05), and Christensenellaceae_R-7_group (p < 0.05); visuospatial and executive function domain was highly significantly associated with Coriobacteriia (p < 0.01), Coriobacteriales (p < 0.01), Bacteroidales (p < 0.01), and Prevotella (p < 0.01); language domain was significantly associated with Christensenellales (p < 0.01), Christensenellaceae_R-7_group (p < 0.05), Christensenellaceae (p < 0.01), and NK4A214_group (p < 0.01).
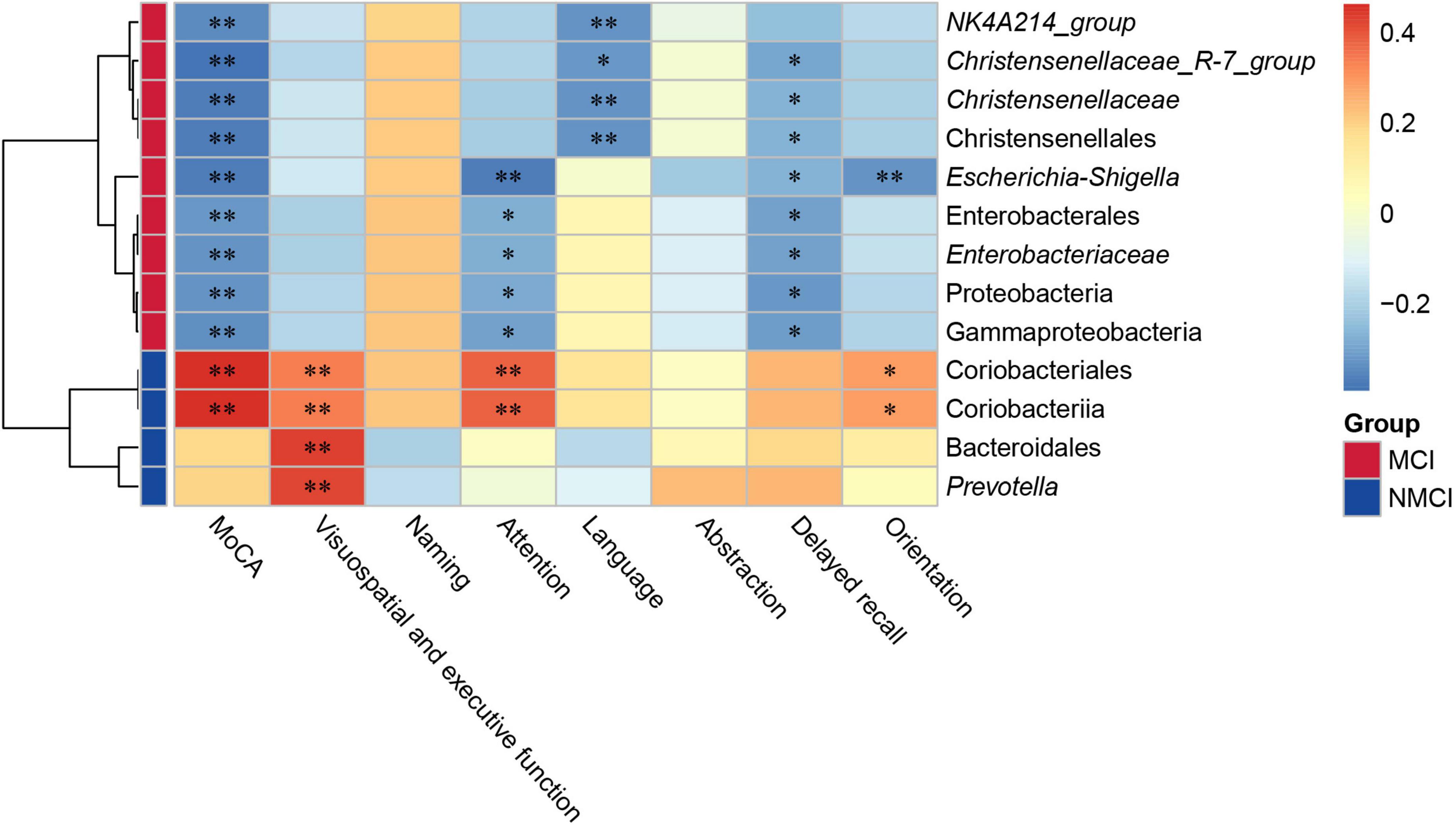
Figure 5. Correlation analysis between gut microbial and MoCA score. The depth of the color in the heatmap represented the strength of the correlation: red represents a positive correlation, whereas blue indicates a negative correlation. Group scale represented enrichment of selected taxa, blue color indicates taxa enriched in the NMCI group, orange color represents taxa enriched in the MCI group. *p < 0.05, **p < 0.01. MCI, hypertension patients with cognitive impairment; NMCI, hypertension patients without cognitive impairment; Control, health control subjects.
Discriminant Models of Mild Cognitive Impairment in Hypertension Patients Based on Selected Intestinal Taxa
Based on the above results, we used 11 candidate microbial groups (Proteobacteria, Coriobacteriia, Gammaproteobacteria, Coriobacteriales, Enterobacterales, Christensenellales, Enterobacteriaceae, Christensenellaceae, Christensenellaceae_R-7_group, Escherichia-Shigella, and NK4A214_group) significantly related to the total score of MoCA, to build a single index prediction model, separately. ROC analysis was used to assess the diagnostic ability of these indexes for the cognitive impairment of hypertension patients. As shown in Table 4 and Figure 6, the single index based on Escherichia–Shigella had the best prediction accuracy, with AUC = 0.783, p < 0.01 and Cutoff = 0.025 (Table 4 and Figure 6). To find a more effective prediction model, we built a multi-index joint prediction model based on nine intestinal bacterial taxa (Proteobacteria, Gammaproteobacteria, Coriobacteriales, Enterobacterales, Enterobacteriaceae, Christensenellaceae, Christensenellaceae_R-7_group, Escherichia-Shigella, and NK4A214_group), which demonstrated better diagnostic values (AUC = 0.944 and Cutoff = 0.584) (Table 4 and Figure 6).
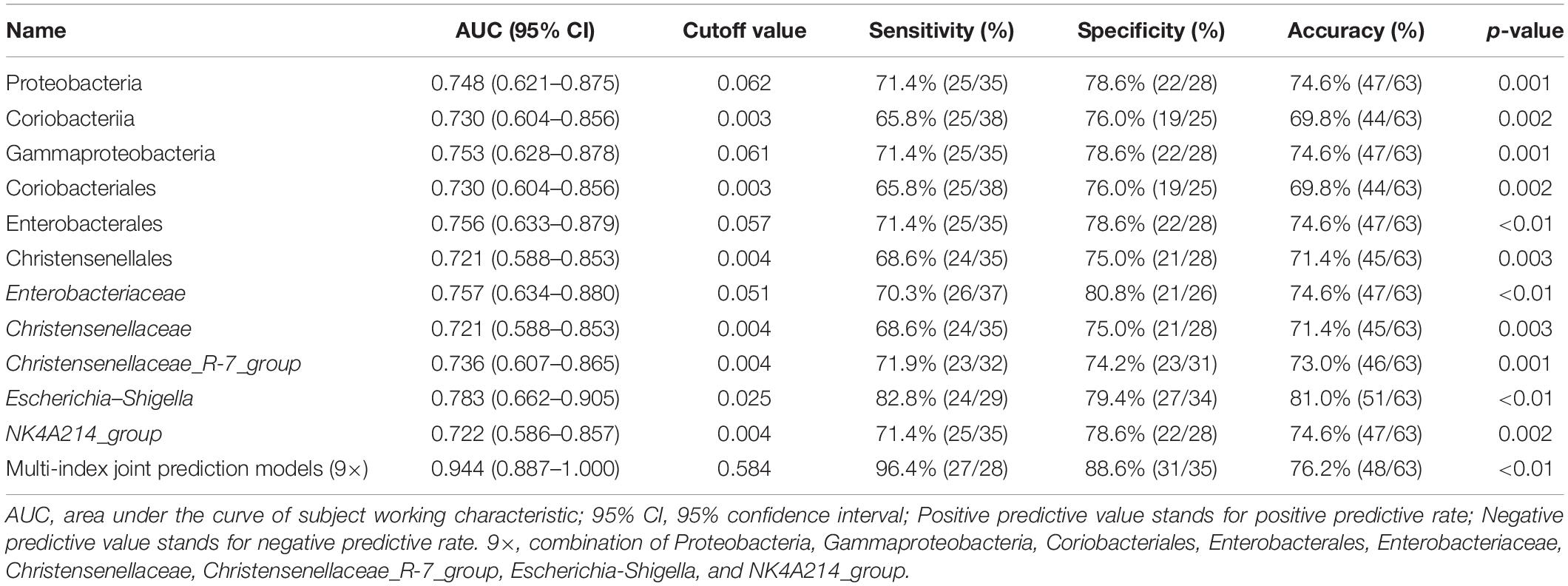
Table 4. Index prediction models and efficacy evaluation for the risk of mild cognitive impairment in hypertension patients.
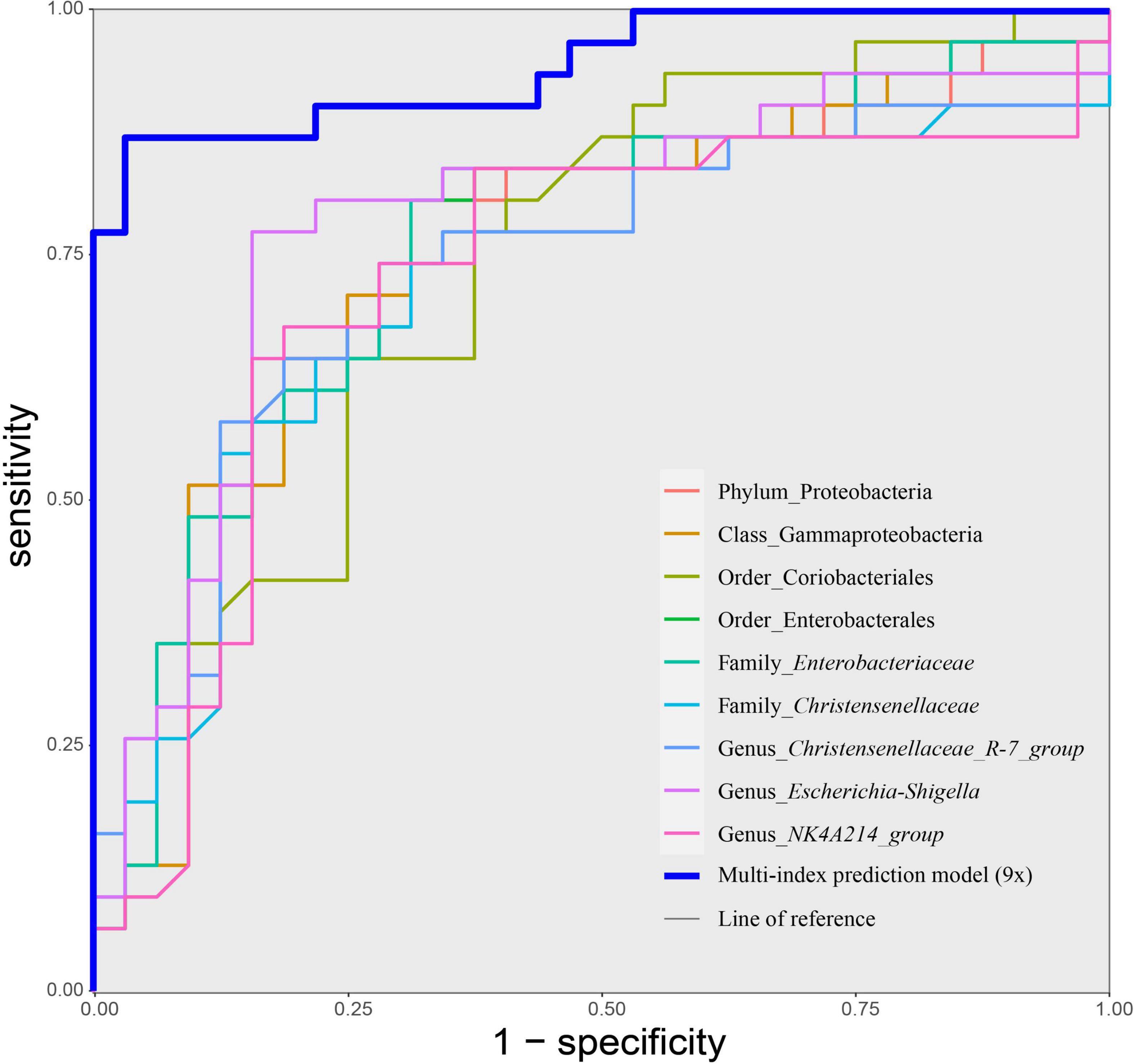
Figure 6. Receiver operating characteristic (ROC) curves of taxa-based single index and the multiple joint model (9×) for discriminating cognitive impairment of hypertension patients. Discriminatory capacity was analyzed by calculating the area under the ROC curve using logistic regression. MCI, hypertension patients with cognitive impairment; NMCI, hypertension patients without cognitive impairment; Control, health control subjects.
Discussion
Mild cognitive impairment in hypertension patients is at risk of deterioration to dementia. Sensitive diagnosis and thereby early intervention for cognitive impairment caused by hypertension can prevent the incidence of dementia. The present study found that dysbiosis of the intestinal flora is involved in the cognitive dysfunction of hypertension patients. Specifically, LPS-containing taxa (Proteobacteria, Gammaproteobacteria, Enterobacterales, Enterobacteriaceae, and Escherichia–Shigella) and SCFA-producing taxon (Prevotella) were closely correlated with cognitive function of hypertension patients (Figure 7). Moreover, we established multiple joint bacteria-based models for objective diagnosis of cognitive impairment in hypertension patients. The current research is a meaningful effort to characterize the intestinal microbiota of hypertension patients with cognitive impairment and shed new light on the objective diagnosis of cognitive decline in hypertension patients.
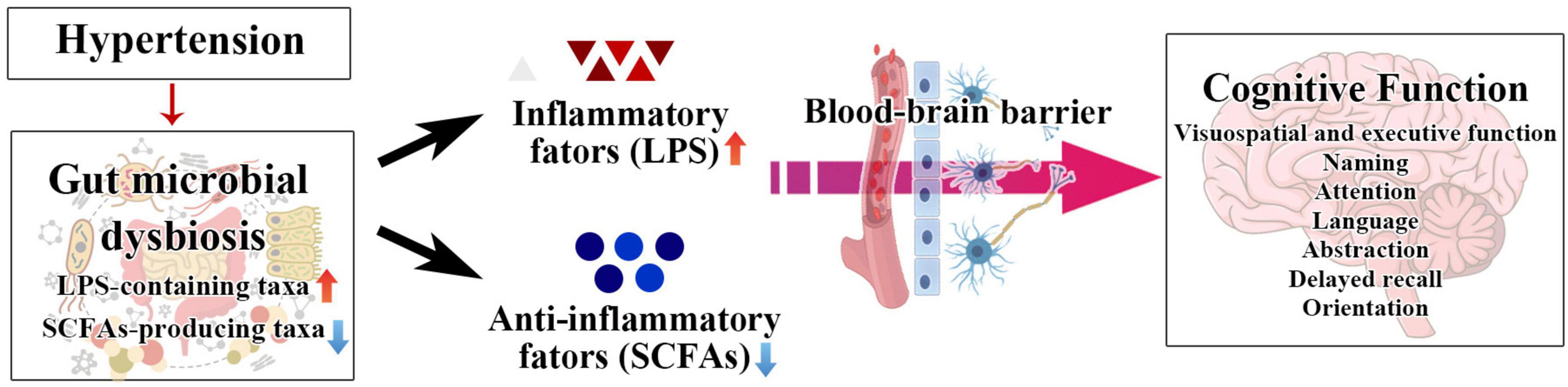
Figure 7. The schematic diagram of our hypothesis about the relationship among SCFA, LPS, hypertension, and cognitive impairment.
Substantial evidence obtained in animals and humans showed that hypertension could induce intestinal flora disorder (Mell et al., 2015; Yang et al., 2015; Marques et al., 2017; Yan et al., 2017). Previous studies indicated that dysbiosis of the intestinal flora is involved in cognitive dysfunction caused by diseases such as Parkinson’s disease and type 2 diabetes (Ren et al., 2020; Zhang et al., 2020). Hence, we hypothesized that the hypertensive-induced dysbiosis of the gut microbiota might play a role in hypertension’s cognitive function decline, which could deteriorate to AD. In the present study, 48.44% of hypertension patients were determined to have cognitive impairment (MoCA < 26). According to Chao1, ACE, observed_species, and PD_whole_tree indexes, we found that the microbial diversity and community abundance significantly rose in cognitive-impaired patients compared to patients with normal cognitive function. The unique OTUs were more frequent in cognitive impairment patients, reaching from 510 in the NMCI group to 668 in the MCI group. Specifically, our results showed that the relative abundance of LPS-containing taxa, Proteobacteria, Gammaproteobacteria, Enterobacterales, Enterobacteriaceae, and Escherichia–Shigella, was dramatically higher in hypertension patients with cognitive impairment. LPS, as a cell wall component of Gram-negative bacteria, has been previously reported to induce the production of pro-inflammatory cytokines (Oyama et al., 2004). Existing literature has shown that hypertension causes increased permeability of the blood–brain barrier (Faraco et al., 2016). Hence, the gut microbiota-derived products, such as LPS, could leak to the CNS and regulate neuroinflammation, which is a critical mechanism of cognitive impairment (Erny et al., 2015; Rothhammer et al., 2016). A recent clinical study (Liu et al., 2019) demonstrated that Gammaproteobacteria, Enterobacteriales, and Enterobacteriaceae showed a progressively enriched prevalence from health control to amnestic mild cognitive impairment and AD patients, which is similar to our results of high relative abundance of LPS-containing taxa in hypertension patients with cognitive impairment. Accumulating studies revealed that cardiovascular diseases such as hypertension and AD have overlapping neuropathological processes (Zlokovic, 2011; Rodrigue et al., 2013; Iadecola, 2016). Hence, we speculate that these specific LPS-containing taxa, including Gammaproteobacteria, Enterobacterales, and Enterobacteriaceae, which simultaneously exhibited relatively high abundance in hypertension patients with cognitive impairment and AD patients, may contribute to cognitive deterioration and play a significant role in the neuropathogenesis of hypertension’s cognitive function decline progressive to AD. Further study is required to elucidate the precise role and clarify the mechanism for this phenomenon.
Moreover, we found that the relative abundance of Prevotella, which was demonstrated as a SCFA-producing probiotic in the gut (Ren et al., 2021), was significantly decreased in the patients with cognitive impairment compared to the patients without cognitive impairment. Previous evidence demonstrated that downregulation of SCFAs is involved in cognitive impairment diseases including AD, Parkinson’s Disease, congestive heart failure, and sulfamonomethoxine exposure (Ho et al., 2018; Zhu et al., 2019; Sarah et al., 2020; He et al., 2021). SCFAs, such as propionic acid, butyric acid, isobutyric acid, and valeric acid, can inhibit inflammation by regulating histone deacetylase and binding G-protein-coupled receptors (Tan et al., 2014; Yang W. et al., 2020). Our results of Tax4Fun predication showed that the glycan biosynthesis and metabolism were significantly decreased in hypertension patients with cognitive impairment. The trend was supposed to cause carbohydrate metabolism disorder (Woting and Blaut, 2016), resulting in insufficient SCFAs by intestinal flora after digestion of carbohydrate, inducing inflammatory and subsequently affecting cognitive function (Koh et al., 2016; Sawicki et al., 2017). In the present study, we found that the enrichment of glycan biosynthesis and metabolism was significantly positively associated with the SCFA-producing probiotic Prevotella, while it was substantially negatively correlated with the relative abundance of LPS-containing taxa (Proteobacteria, Gammaproteobacteria, Enterobacterales, Escherichia–Shigella, and Enterobacteriaceae).
Additionally, we found that the total score of MoCA was strongly associated with the taxa (e.g., Proteobacteria, Coriobacteriales, and Enterobacteriaceae), which were significantly altered in the hypertension patients with cognitive impairment. In particular, visuospatial and executive function domain, attention domain, orientation domain, delayed recall domain, and language domain of the MoCA dramatically correlated with selected taxa, respectively. Notably, attention domain, orientation domain, and delayed recall domain were simultaneously correlated with Escherichia–Shigella, while attention domain, orientation domain, and visuospatial and executive function domain were consistently associated with Coriobacteriia and Coriobacteriales. Cattaneo et al. (2017) reported that cognitively impaired patients with brain amyloidosis showed a higher abundance of inflammatory bacteria taxon Escherichia–Shigella compared with both healthy controls and patients with no brain amyloidosis. The enrichment of Escherichia–Shigella is also found to be implicated in post-operative cognitive dysfunction (Lian et al., 2021). Studies investigating the effects of specific bacteria on a distinct domain of cognitive function were still limited. It was revealed by a prospective, longitudinal study that children with a history of bacterial meningitis were at greater risk of impairment in executive ability (Anderson et al., 2004). A study that employed a total 140 participants who were selected using purposive sampling from the patients within the age group of 18–60 years old at Tehran in 2016 found that Helicobacter pylori infection increases the prevalence of memory and executive dysfunction (Rezvani et al., 2017). To the best of our knowledge, our study is the first to demonstrate the corresponding associations of selected taxa with specific domains of cognitive functioning among hypertension patients. Although a possible causal relationship between these selected taxa and different aspects of cognitive functioning deserves further investigation, our results promote the possibility that the precision intervention may be achieved by targeting specific bacteria associated with distinct cognitive dysfunction of hypertension patients.
To date, the current diagnosis of neurocognitive disorders mainly relies on neuropsychological scales and expensive neuroimaging. Exploration of potential objective and cost-effective indicators of neurocognitive decline is still limited. In the current study, we aimed to establish an objective and no-invasion prediction model based on selected gut bacteria to predict mild cognitive impairment of hypertension patients. To choose the optimal model for diagnosis of cognitive impairment of hypertension patients, the area under the ROC was analyzed. Our results showed that the bacteria-based multi-index prediction model (9×) performed best on mild cognitive impairment in hypertension patients (AUC = 0.944). Additionally, the single index prediction model based on Escherichia–Shigella has also promised efficiency prediction (AUC = 0.783), despite the accuracy being less than that of the multi-index prediction model. However, because a single intestinal flora is more efficient, this single index prediction model might also be valuable in applications.
Nevertheless, our studies have some limitations. Firstly, we only recruited a limited number of participants, making it possible to ignore the slight alteration of the intestinal microbiota. Secondly, it is needed to recruit a new cohort to verify the accuracy of our prediction models. In future experiments, we will expand the sample size and conduct a longer duration of follow-up visits for further verification of the results. Thirdly, 16S amplicon sequencing is not enough for functional analysis, and microbiome metagenomics should be carried out to improve the resolution of the taxonomic functional composition of the microbiome.
Conclusion
Evidence demonstrated that hypertension-associated cognitive impairment and AD have overlapped pathophysiology. Effective diagnosis and early intervention for this neurocognitive decline could prevent the incidence of AD. The present study provides evidence for the involvement of intestinal microbiota dysbiosis in the cognitive impairment of hypertension patients. Moreover, we established efficiency prediction models based on selected taxa that strongly correlated with cognitive function performance. This work exposed a sensitive and objective index for the mild cognitive impairment of hypertension patients, and elucidate targets for preventing or reversing intestinal microbiota dysbiosis for future no-pharmacology therapeutics.
Data Availability Statement
The datasets presented in this study can be found in online repositories. The names of the repository/repositories and accession number(s) can be found below: https://www.ncbi.nlm.nih.gov/, SAMN21436827-21436923.
Ethics Statement
The studies involving human participants were reviewed and approved by Institution Review Board of Binzhou Medical University (No. 2018-085). The patients/participants provided their written informed consent to participate in this study.
Author Contributions
YW and MC conceived and supervised the project. YW, MC, LQ, and YL designed and performed the main experiments. ZD, SM, LQ, and YL participated in 16S rRNA amplicon sequencing analysis. YL, LQ, WZ, ZW, CW, RM, XJ, and TZ recruited the subjects and collected samples. YL, ZD, and LQ drafted the manuscript. MC and ZD revised the manuscript. All authors have approved the final version of the manuscript.
Funding
This research was supported by grants from the National Natural Science Foundation of China (Grant Nos. 81501950 and 81672044), the Science and Technology Plan Project of Yantai (Grant Nos. 2020YT06000973 and 2022JCYJ027), and Shandong Provincial Natural Science Foundation, China (Grant No. ZR2021QH154).
Conflict of Interest
The authors declare that the research was conducted in the absence of any commercial or financial relationships that could be construed as a potential conflict of interest.
Publisher’s Note
All claims expressed in this article are solely those of the authors and do not necessarily represent those of their affiliated organizations, or those of the publisher, the editors and the reviewers. Any product that may be evaluated in this article, or claim that may be made by its manufacturer, is not guaranteed or endorsed by the publisher.
Footnotes
References
Abete, P., Della-Morte, D., Gargiulo, G., Basile, C., Langellotto, A., Galizia, G., et al. (2014). Cognitive impairment and cardiovascular diseases in the elderly. A heart–brain continuum hypothesis. Ageing Res. Rev. 18, 41–52. doi: 10.1016/j.arr.2014.07.003
Akbari, E., Asemi, Z., Daneshvar Kakhaki, R., Bahmani, F., Kouchaki, E., Tamtaji, O. R., et al. (2016). Effect of probiotic supplementation on cognitive function and metabolic status in Alzheimer’s disease: a randomized, double-blind and controlled trial. Front. Aging Neurosci. 8:256. doi: 10.3389/fnagi.2016.00256
Anderson, V., Anderson, P., Grimwood, K., and Nolan, T. (2004). Cognitive and executive function 12 years after childhood bacterial meningitis: effect of acute neurologic complications and age of onset. J. Pediatr. Psychol. 29, 67–81. doi: 10.1093/jpepsy/jsh011
Aßhauer, K. P., Wemheuer, B., Daniel, R., and Meinicke, P. (2015). Tax4Fun: predicting functional profiles from metagenomic 16S rRNA data. Bioinformatics 31, 2882–2884. doi: 10.1093/bioinformatics/btv287
Cattaneo, A., Cattane, N., Galluzzi, S., Provasi, S., Lopizzo, N., Festari, C., et al. (2017). Association of brain amyloidosis with pro-inflammatory gut bacterial taxa and peripheral inflammation markers in cognitively impaired elderly. Neurobiol. Aging 49, 60–68. doi: 10.1016/j.neurobiolaging.2016.08.019
Copersino, M. L., Fals-Stewart, W., Fitzmaurice, G., Schretlen, D. J., Sokoloff, J., and Weiss, R. D. (2009). Rapid cognitive screening of patients with substance use disorders. Exp. Clin. Psychopharmacol 17, 337–344. doi: 10.1037/a0017260
Dubinski, P., Czarzasta, K., and Cudnoch-Jedrzejewska, A. (2021). The influence of gut microbiota on the cardiovascular system under conditions of obesity and chronic stress. Curr. Hypertens. Rep. 23:31. doi: 10.1007/s11906-021-01144-7
Edgar, R. C. (2004). MUSCLE: multiple sequence alignment with high accuracy and high throughput. Nucleic Acids Res. 32, 1792–1797. doi: 10.1093/nar/gkh340
Edgar, R. C. (2013). UPARSE: highly accurate OTU sequences from microbial amplicon reads. Nat. Methods 10, 996–998. doi: 10.1038/nmeth.2604
Erny, D., Hrabe de Angelis, A. L., Jaitin, D., Wieghofer, P., Staszewski, O., David, E., et al. (2015). Host microbiota constantly control maturation and function of microglia in the CNS. Nat. Neurosci. 18, 965–977. doi: 10.1038/nn.4030
Faraco, G., Sugiyama, Y., Lane, D., Garcia-Bonilla, L., Chang, H., Santisteban, M. M., et al. (2016). Perivascular macrophages mediate the neurovascular and cognitive dysfunction associated with hypertension. J. Clin. Invest. 126, 4674–4689. doi: 10.1172/JCI86950
Fluss, R., Faraggi, D., and Reiser, B. (2005). Estimation of the youden index and its associated cutoff point. Biom. J 47, 458–472.
He, X., Qian, Y., Xu, S., Zhang, Y., Mo, C., Guo, W., et al. (2021). Plasma short-chain fatty acids differences in multiple system atrophy from parkinson’s disease. J. Parkinsons Dis. 11, 1167–1176. doi: 10.3233/JPD-212604
Ho, L., Ono, K., Tsuji, M., Mazzola, P., Singh, R., and Pasinetti, G. M. (2018). Protective roles of intestinal microbiota derived short chain fatty acids in Alzheimer’s disease-type beta-amyloid neuropathological mechanisms. Expert Rev. Neurother. 18, 83–90. doi: 10.1080/14737175.2018.1400909
Huart, J., Leenders, J., Taminiau, B., Descy, J., Saint-Remy, A., Daube, G., et al. (2019). Gut microbiota and fecal levels of short-chain fatty acids differ upon 24-hour blood pressure levels in men. Hypertension 74, 1005–1013. doi: 10.1161/HYPERTENSIONAHA.118.12588
Iadecola, C. (2016). Vascular and metabolic factors in Alzheimer’s disease and related dementias: introduction. Cell Mol. Neurobiol. 36, 151–154. doi: 10.1007/s10571-015-0319-y
Joint Committee for Guideline Revision (2019). 2018 Chinese Guidelines for prevention and treatment of hypertension-a report of the revision committee of chinese guidelines for prevention and treatment of hypertension. J. Geriatr. Cardiol. 16, 182–241. doi: 10.11909/j.issn.1671-5411.2019.03.014
Koh, A., De Vadder, F., Kovatcheva-Datchary, P., and Bäckhed, F. (2016). From dietary fiber to host physiology: short-chain fatty acids as key bacterial metabolites. Cell 165, 1332–1345. doi: 10.1016/j.cell.2016.05.041
Lian, X., Zhu, Q., Sun, L., and Cheng, Y. (2021). Effect of Anesthesia/Surgery on gut microbiota and fecal metabolites and their relationship with cognitive dysfunction. Front. Syst. Neurosci. 15:655695. doi: 10.3389/fnsys.2021.655695
Ling, Y., Gu, Q., Zhang, J., Gong, T., Weng, X., Liu, J., et al. (2020). Structural change of gut microbiota in patients with post-stroke comorbid cognitive impairment and depression and its correlation with clinical features. J. Alzheimers Dis. 77, 1595–1608. doi: 10.3233/JAD-200315
Liu, P., Wu, L., Peng, G., Han, Y., Tang, R., and Ge, J. (2019). Altered microbiomes distinguish Alzheimer’s disease from amnestic mild cognitive impairment and health in a Chinese cohort. Brain Behav. 80, 633–643. doi: 10.1016/j.bbi.2019.05.008
Liu, Y., Kong, C., Gong, L., Zhang, X., Zhu, Y., Wang, H., et al. (2020). The association of post-stroke cognitive impairment and gut microbiota and its corresponding metabolites. J. Alzheimers Dis. 73, 1455–1466. doi: 10.3233/JAD-191066
Lorenzoni, A. G., and Wideman, R. F. (2008). Intratracheal administration of bacterial lipopolysaccharide elicits pulmonary hypertension in broilers with primed airways. Poult. Sci. 87, 645–654. doi: 10.3382/ps.2007-00483
Marques, F. Z., Nelson, E., Chu, P. Y., Horlock, D., Fiedler, A., Ziemann, M., et al. (2017). High-fiber diet and acetate supplementation change the gut microbiota and prevent the development of hypertension and heart failure in hypertensive mice. Circul. Res. 135, 964–977. doi: 10.1161/CIRCULATIONAHA.116.024545
Mell, B., Jala, V. R., Mathew, A. V., Byun, J., and Joe, B. (2015). Evidence for a link between gut microbiota and hypertension in the Dahl rat. Physiol. Genomics 47, 187–197. doi: 10.1152/physiolgenomics.00136.2014
Nasreddine, Z. S., Phillips, N. A., Bédirian, V., Charbonneau, S., Whitehead, V., Collin, I., et al. (2005). The montreal cognitive assessment, MoCA: a brief screening tool for mild cognitive impairment. J. Am. Geriatr. Soc. 53, 695–699. doi: 10.1111/j.1532-5415.2005.53221.x
NCD Risk Factor Collaboration (2021). Worldwide trends in hypertension prevalence and progress in treatment and control from 1990 to 2019: a pooled analysis of 1201 population-representative studies with 104 million participants. Lancet 398, 957–980. doi: 10.1016/S0140-6736(21)01330-1
Oyama, J. I., and Node, K. (2019). Gut microbiota and hypertension. Hypertens. Res. 42, 741–743. doi: 10.1038/s41440-41018-40203-41445
Oyama, J. I., Blais, C., Liu, X., Pu, M., and Bourcier, T. (2004). Reduced myocardial ischemia-reperfusion injury in toll-like receptor 4-deficient mice. Circulation 109, 784–789. doi: 10.1161/01.CIR.0000112575.66565.84
Petrovitch, H., White, L. R., Izmirilian, G., Ross, G. W., Havlik, R. J., Markesbery, W., et al. (2000). Midlife blood pressure and neuritic plaques, neurofibrillary tangles, and brain weight at death: the HAAS. Neurobiol. Aging 21, 57–62. doi: 10.1016/s0197-4580(00)00106-8
Pietrucci, D., Cerroni, R., Unida, V., Farcomeni, A., Pierantozzi, M., Mercuri, N. B., et al. (2019). Dysbiosis of gut microbiota in a selected population of Parkinson’s patients. Parkinsonism Relat. Disord. 65, 124–130. doi: 10.1016/j.parkreldis.2019.06.003
Qiu, C., Winblad, B., and Fratiglioni, L. (2005). The age-dependent relation of blood pressure to cognitive function and dementia. Lancet Neurol. 4, 487–499. doi: 10.1016/S1474-4422(05)70141-1
Quast, C., Pruesse, E., Yilmaz, P., Gerken, J., and Glckner, F. O. (2013). The SILVA ribosomal RNA gene database project: improved data processing and web-based tools. Nucleic Acids Res. 41, D590–D596. doi: 10.1093/nar/gks1219
Ren, T., Gao, Y., Qiu, Y., Jiang, S., Zhang, Q., Zhang, J., et al. (2020). Gut microbiota altered in mild cognitive impairment compared with normal cognition in sporadic Parkinson’s disease. Front. Neurol. 11:137. doi: 10.3389/fneur.2020.00137
Ren, W., Yan, H., Yu, B., Walsh, M. C., Yu, J., Zheng, P., et al. (2021). Prevotella-rich enterotype may benefit gut health in finishing pigs fed diet with a high amylose-to-amylopectin ratio. Anim. Nutr. 7, 400–411. doi: 10.1016/j.aninu.2020.08.007
Rezvani, F., Sayadnasiri, M., and Rezaei, O. (2017). The study of memory and executive dysfunction in patients infected with Helicobacter pylori. Neurol. Res. 39, 953–958. doi: 10.1080/01616412.2017.1363349
Rodrigue, K. M., Rieck, J. R., Kennedy, K. M., Devous, M., Diazarrastia, R., and Park, D. C. (2013). Risk factors for β-Amyloid deposition in healthy aging: vascular and genetic effects. JAMA Neurol. 70, 600–606.
Rothhammer, V., Mascanfroni, I. D., Bunse, L., Takenaka, M. C., Kenison, J. E., Mayo, L., et al. (2016). Type I interferons and microbial metabolites of tryptophan modulate astrocyte activity and central nervous system inflammation via the aryl hydrocarbon receptor. Nat. Med. 22, 586–597. doi: 10.1038/nm.4106
Sampson, T. R., Debelius, J. W., Thron, T., Janssen, S., Shastri, G. G., Ilhan, Z. E., et al. (2016). Gut microbiota regulate motor deficits and neuroinflammation in a model of Parkinson’s disease. Cell 167, 1469–1480.e12. doi: 10.1016/j.cell.2016.11.018
Sarah, K., Nicolaas, D., John, T., Gabriella, T. H., and Engelen, M. (2020). Reduced Short-Chain Fatty Acid (SCFA) plasma concentrations are associated with decreased psychological well-being in clinically stable congestive heart failure patients. Curr. Dev. Nutr. 4:42.
Sawicki, C. M., Livingston, K. A., Obin, M., Roberts, S. B., Chung, M., and McKeown, N. M. (2017). Dietary fiber and the human gut microbiota: application of evidence mapping methodology. Nutrients 9:125. doi: 10.3390/nu9020125
Schloss, P. D., Westcott, S. L., Ryabin, T., Hall, J. R., Hartmann, M., Hollister, E. B., et al. (2009). Introducing mothur: open-source, platform-independent, community-supported software for describing and comparing microbial communities. Appl. Environ. Microbiol. 75, 7537–7541. doi: 10.1128/AEM.01541-09
Segata, N., Izard, J., Waldron, L., Gevers, D., Miropolsky, L., Garrett, W. S., et al. (2011). Metagenomic biomarker discovery and explanation. Genome Biol. 12:R60. doi: 10.1186/gb-2011-12-6-r60
Tan, J., McKenzie, C., Potamitis, M., Thorburn, A. N., Mackay, C. R., and Macia, L. (2014). The role of short-chain fatty acids in health and disease. Adv. Immunol. 121, 91–119. doi: 10.1016/B978-0-12-800100-4.00003-9
Westfall, S., Lomis, N., Kahouli, I., Dia, S. Y., Singh, S. P., and Prakash, S. (2017). Microbiome, probiotics and neurodegenerative diseases: deciphering the gut brain axis. Cell Mol. Life Sci. 74, 3769–3787. doi: 10.1007/s00018-017-2550-9
Wu, Y., Xu, H., Tu, X., and Gao, Z. (2021). The role of short-chain fatty acids of gut microbiota origin in hypertension. Front. Microbiol. 12:730809. doi: 10.3389/fmicb.2021.730809
Yan, Q., Gu, Y., Li, X., Yang, W., Jia, L., Chen, C., et al. (2017). Alterations of the gut microbiome in hypertension. Front. Cell. Infect. Microbiol. 7:381. doi: 10.3389/fcimb.2017.00381
Yang, F., Chen, H., Gao, Y., An, N., Li, X., Pan, X., et al. (2020). Gut microbiota-derived short-chain fatty acids and hypertension: mechanism and treatment. Biomed. Pharmacother. 130:110503. doi: 10.1016/j.biopha.2020.110503
Yang, T., Santisteban, M. M., Rodriguez, V., Li, E., Ahmari, N., Carvajal, J. M., et al. (2015). Gut dysbiosis is linked to hypertension. Hypertension 65, 1331–1340.
Yang, W., Yu, T., Huang, X., Bilotta, A. J., Xu, L., Lu, Y., et al. (2020). Intestinal microbiota-derived short-chain fatty acids regulation of immune cell IL-22 production and gut immunity. Nat. Commun. 11:4457. doi: 10.1038/s41467-020-18262-6
Yu, J., Li, J., and Huang, X. (2012). The Beijing version of the montreal cognitive assessment as a brief screening tool for mild cognitive impairment: a community-based study. BMC Psychiatry 12:156. doi: 10.1186/1471-244X-12-156
Zhang, Y., Lu, S., Yang, Y., Wang, Z., and Yu, Z. (2020). The diversity of gut microbiota in type 2 diabetes with or without cognitive impairment. Aging Clin. Exp. Res. 33, 589–601. doi: 10.1007/s40520-020-01553-9
Zhu, X., Han, Y., Du, J., Liu, R., Jin, K., and Wei, Y. (2017). Microbiota-gut-brain axis and the central nervous system. Oncotarget 8, 53829–53838.
Zhu, Y. T., Liu, X. J., Liu, K. Y., Zhang, Q., Yang, L. S., Wei, R., et al. (2019). Persistently upregulated hippocampal mTOR signals mediated by fecal SCFAs impair memory in male pups with SMM exposure in utero. Biomed. Environ. Sci. 32, 345–356. doi: 10.3967/bes2019.046
Zlokovic, B. V. (2011). Neurovascular pathways to neurodegeneration in Alzheimer’s disease and other disorders. Nat. Rev. Neurosci. 12, 723–738. doi: 10.1038/nrn3114
Keywords: gut microbiota, hypertension, cognitive impair, prediction model, 16S/18S ribosomal RNA gene analysis
Citation: Qu L, Dong Z, Ma S, Liu Y, Zhou W, Wang Z, Wu C, Ma R, Jiang X, Zu T, Cheng M and Wu Y (2022) Gut Microbiome Signatures Are Predictive of Cognitive Impairment in Hypertension Patients—A Cohort Study. Front. Microbiol. 13:841614. doi: 10.3389/fmicb.2022.841614
Received: 22 December 2021; Accepted: 26 February 2022;
Published: 08 April 2022.
Edited by:
Hariom Yadav, USF Center for Microbiome Research, United StatesReviewed by:
Leandro Dias Teixeira, University of Florida, United StatesZhangran Chen, Xiamen University, China
Zhenlin Liao, South China Agricultural University, China
Tao Tan, Baylor College of Medicine, United States
Copyright © 2022 Qu, Dong, Ma, Liu, Zhou, Wang, Wu, Ma, Jiang, Zu, Cheng and Wu. This is an open-access article distributed under the terms of the Creative Commons Attribution License (CC BY). The use, distribution or reproduction in other forums is permitted, provided the original author(s) and the copyright owner(s) are credited and that the original publication in this journal is cited, in accordance with accepted academic practice. No use, distribution or reproduction is permitted which does not comply with these terms.
*Correspondence: Mei Cheng, chm790217@126.com; Yulong Wu, ylongwu@126.com
†These authors have contributed equally to this work