- 1Division of Plant Pathology, Indian Council of Agricultural Research (ICAR)-Indian Agricultural Research Institute, New Delhi, India
- 2Indian Council of Agricultural Research (ICAR)-National Institute for Plant Biotechnology, New Delhi, India
- 3Division of Genetics, Indian Council of Agricultural Research (ICAR)-Indian Agricultural Research Institute, New Delhi, India
Bipolaris maydis is pathogen of maize which causes maydis leaf blight disease. In India major losses occur due to the B. maydis race “O” pathogen, whereas in other parts of the world, major losses are due to the race “T” pathogen. In the present study, we conducted an in planta transcriptomics study of the B. maydis race “O” pathogen after infection on non-CMS maize resistant and susceptible genotypes by mRNA sequencing to understand the molecular basis of pathogenicity for better management of the pathogen. Approximately 23.4 GB of mRNA-seq data of B. maydis were obtained from both resistant and susceptible maize backgrounds for fungus. Differentially expressed genes (DEGs) analysis of B. maydis in two different genetic backgrounds suggested that the majority of highly DEGs were associated with mitochondrial, cell wall and chitin synthesis, sugar metabolism, peroxidase activity, mitogen-activated protein kinase (MAPK) activity, and shikimate dehydrogenase. KEGG analysis showed that the biosynthetic pathways for secondary metabolism, antibiotics, and carbon metabolism of fungus were highly enriched, respectively, in susceptible backgrounds during infection. Previous studies in other host pathogen systems suggest that these genes play a vital role in causing disease in their host plants. Our study is probably the first transcriptome study of the B. maydis race “O” pathogen and provides in-depth insight of pathogenicity on the host.
Introduction
Bipolaris maydis (Cochliobolus heterostrophus) is a necrotrophic ascomycete belonging to the order Pleosporales, which causes maydis leaf blight (MLB) or southern corn leaf blight. In India, yield losses occur due to the B. maydis race “O” pathogen in maize, unlike the rest of the world where major losses are due to the race “T” pathogen. Maize (Zea mays L.) is the third most widely grown cereal crop after rice and wheat in India (Hussain, 2011). Losses in India due to MLB disease may extend up to 70% (Kumar et al., 2016). Infected maize typically causes tan and elliptical to rectangular lesions (White, 1999) on the leaves and under the surface of foliage which later coalesce and result in an extensive blight appearance. Among the 65 major foliar diseases of maize, MLB is an important disease of maize (Rahul and Singh, 2002). MLB is reported from almost all maize growing regions in the world but more severe in areas where environmental conditions are hot and humid. Three races (C, O, and T) of B. maydis have been identified in maize crop so far. Race “O” is more prevalent than “T” in India, whereas worldwide, race “T” is a major concern; race “C” is reported only in China (Mubeen et al., 2017).
Bipolaris maydis race “O” is predominant in tropical and sub-tropical areas. It infects a broad range of maize genotypes including CMS and non-CMS maize lines. Studies reported that inoculated susceptible lines with race “O” showed a 50% yield loss (Fisher et al., 1976; Gregory et al., 1978). Typical symptoms of race “O” are small lesions which eventually become diamond-shaped and rectangular as they mature and are restricted to leaf veins (Ali et al., 2011). Race “T” attacks CMS maize which promotes Texas male-sterile cytoplasm (cms-T), this race historically caused an epidemic in the United States in 1970 and 1971. Typical symptoms of race “T” develop on leaves, husks, and ears, and produce small lesions on maize (Ullstrup, 1972). Races “O” and “T” can be identified best with a host differential test, viz., a pathogenicity test of cms-T plants, and also by physiological/morphological characteristics on culture media (Leonard, 1977; Warren et al., 1977).
Combatting losses caused by MLB resistance varieties is the best solution. Maize crop is resistant to race “T” with normal cytoplasm therefore management of race “T” can be achieved with elimination of cms-T from cultivars of high agronomic importance (Hyre, 1970; Ullstrup, 1972). In India, a broad range of maize genotypes serve as the major host of race “O,” which causes huge loss. So far, we only know that the rhm recessive gene of C. heterostrophus confers resistance to race “O” (Zaitlin et al., 1993). Various screening techniques, viz., detached leaf techniques (Lakshmi and Sharma, 1987), tissue culture (Kuehnle and Earle, 1988), and seedling assays (Tajimi et al., 1985) have been investigated for disease resistance. Conventional breeding or recurrent selection is also an effective method to improve resistance against MLB (Shieh and Lu, 1993). On the pathogen side, very few studies have been conducted to understand the race “O” pathogen. In the present study, whole transcriptome analysis was done by mRNA sequencing of the B. maydis race “O” pathogen after infection of resistant and susceptible non-CMS maize inbred lines to understand the molecular basis of pathogenicity leading to better management of the pathogen. The race “O” pathogen used in this study was re-confirmed by Venkatesh et al. (2021). So far transcriptome analysis of fungal pathogen B. sorokiniana on infected wheat (Ye et al., 2019) and B. sorghicola on sorghum (Mizuno et al., 2012; Yazawa et al., 2013) has been completed. Here we present probably the first in planta transcriptome study of the B. maydis race “O” pathogen on non-CMS maize lines. RNA-seq and fold change were calculated by comparing B. maydis infection on susceptible inoculated (SI) versus resistant inoculated (RI) lines.
Materials and Methods
Plant Material and Fungal Inoculation
Two extreme genotypes of maize inbred lines differing in their susceptibility to B. maydis were used in this study. Line SC-7-2-1-2-6-1 (SC-7) which is registered (INGR 07025) as a highly resistant non-CMS line and CM 119 which is established as a standard susceptible marker against B. maydis. The experiment was conducted under greenhouse conditions. The B. maydis New Delhi isolate was maintained in pure culture and later mass-multiplied on soaked sorghum seeds. After 30 days, old plants were inoculated with pathogen B. maydis according to the method described by Payak and Sharma (1983). Inoculated samples were collected for RNA-seq at 48 h post inoculation (disease phase, Liu et al., 2015). Symptoms started appearing and fungal signs were noticed more clearly on susceptible line CM 119 (Figure 1).
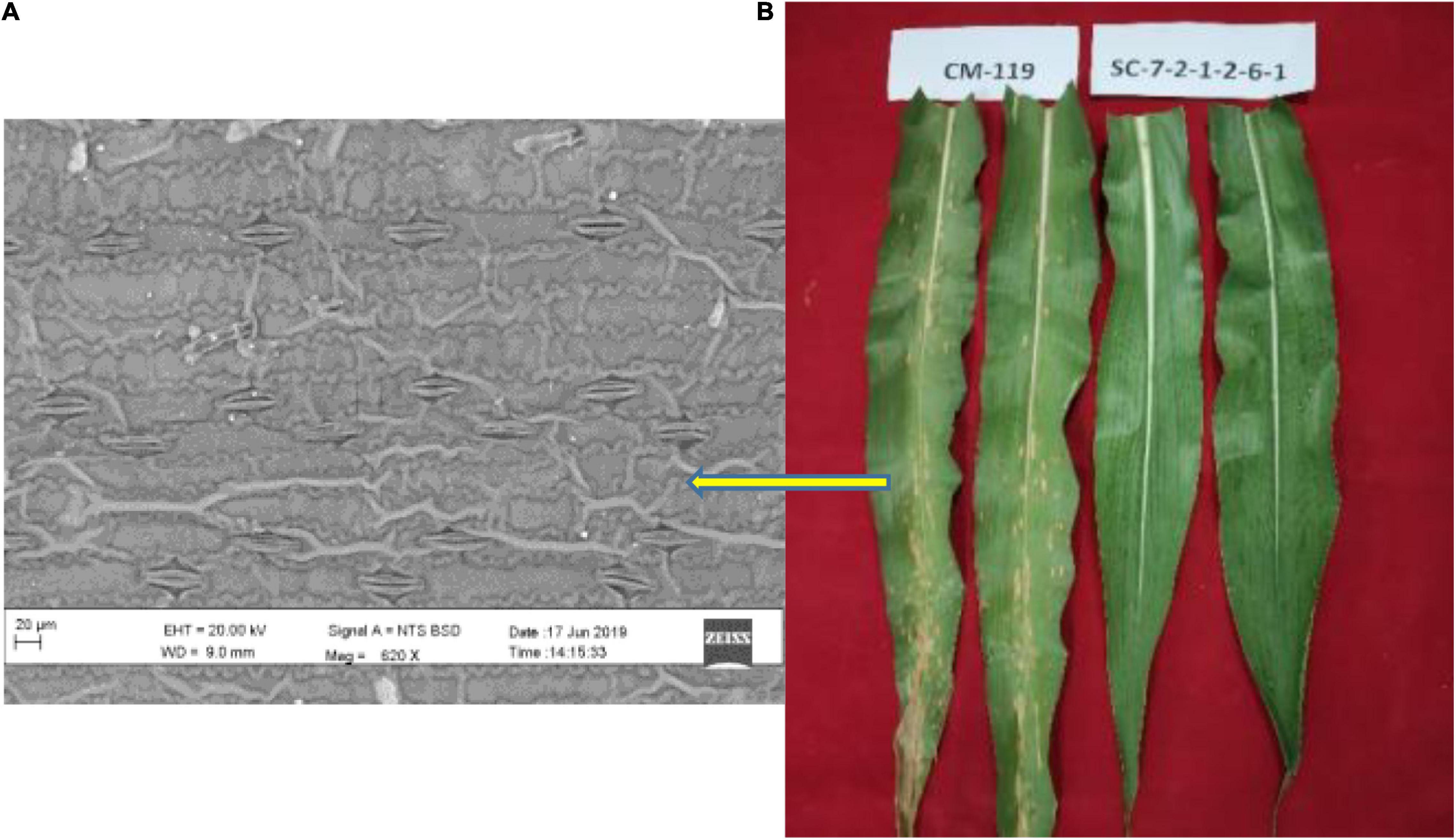
Figure 1. (A) Scanning electron micrograph showing abundant fungal hyphae in susceptible (CM 119) line after infection with Bipolaris maydis. (B) Symptoms of maydis leaf blight on susceptible CM 119 (score 4) and resistant SC-7 (score 1) and both genotypes under field conditions.
RNA Extraction, Library Preparation, and Sequencing
Total RNA was extracted from infected CM 119 and SC-7 at 48 h post inoculation and their non-inoculated controls with an RNAeasy plant mini kit (Qiagen) following the manufacturer’s instructions. Total RNA of each sample was quantified and qualified by an Agilent 2100 Bioanalyzer (Agilent Technologies, Palo Alto, CA, United States), NanoDrop (Thermo Fisher Scientific Inc.), and 1% agarose gel. One microgram of total RNA with an RIN value above 7 was used for the following library preparation. Next-generation sequencing library preparations were carried out as instructed in the manufacturer’s protocol (NEBNext® Ultra™ RNA Library Prep Kit for Illumina®).
Quality Control and Read Mapping to the Reference Genome
Quality checks for the raw fastq files were conducted through a pipeline consisting of FastQC. The minimum quality score was 30 (Qphred). HISAT2 software was selected according to the characteristics of the reference genome. The reference genome used for this study was Bipolaris_maydis_c5_gca_000338975.CocheC5_3.dna.toplevel.fa. Raw read counts mapped to each gene from the HiSat2-generated alignments were obtained using the feature counts command (Liao et al., 2014) of the subread package (Liao et al., 2013).
Differential Gene Expression Analysis
For differentially expressed gene (DEG) identification, DESEq2 V1.21.17 with a replicated package was run with parametric fit Padj < 0.05. A false discovery rate (FDR) of 0.05 and a fold change of >0 were set as thresholds for DEG calling, as previously described (Bagnaresi et al., 2012; Li and Lan, 2015) and P-value >0.05 was set. The list of all DEGs is provided (Supplementary Table 1) to allow any further DEG sub-setting based on different FDRs or fold changes.
GO Enrichment and KEGG Analyses
GO enrichment analyses were conducted with topGO, an R-bioconductor package for enrichment analysis version 2.28.0., and a P-value of 0.001 was used with classic Fisher ordering, ranks= topgoFisher. The Bioconductor package ClusterProfiler version 3.10.0 was used to generate relevant KEGG pathway pictures incorporating color-coded expression values (Padj < 0.05). A pie chart for GO enrichment is provided along with enriched genes (Supplementary Tables 2–4).
qRT-PCR for Expression of Selected Genes
The validation of the RNA-seq technique was performed by quantitative RT-PCR through monitoring the expression levels of seven selected transcripts (Figure 2 and Supplementary Table 5) after designing primers for selected genes (Supplementary Table 6). A melt curve is also provided in Supplementary Figure 1.
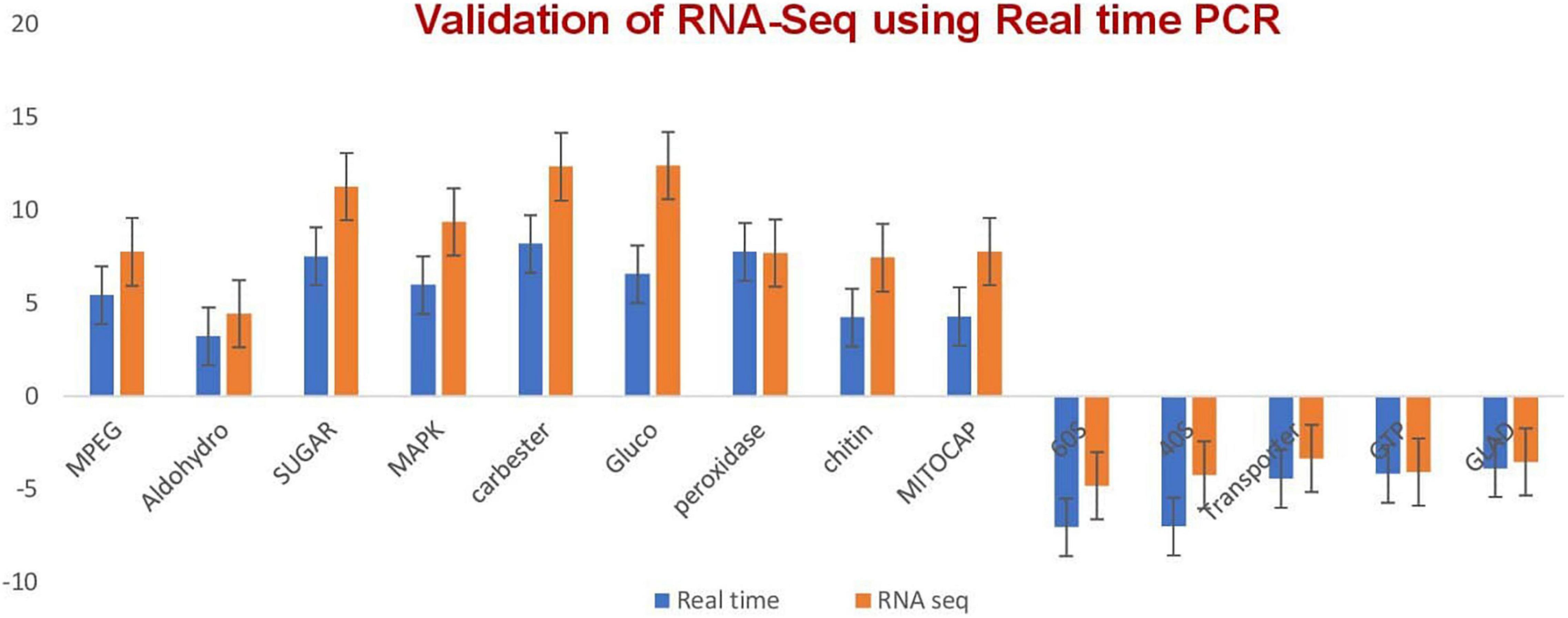
Figure 2. qRT-PCR validation of the relative expression data of genes obtained in RNA-seq analysis. Expression levels of selected transcripts are shown in dark blue (qRT-PCR) and orange (RNA-seq). The fungal actin gene was used for transcript normalization of the signal intensity which is shown on the y axis. The x axis shows comparisons of the results of the two analyses. Error bars show standard deviations for triplicate assays.
Statistical Analysis
qRT-PCR data were analyzed by analysis of variance (ANOVA) using the Statistical Package for Social Science (SPSS, IBM, Chicago, IL, United States) version 16.0. The statistical significance was judged at P < 0.05.
Results
Disease Development
There were no observable phenotypic differences between the susceptible and resistant maize inbred lines without pathogen inoculation at all-time points. We observed prominent symptoms on susceptible line CM 119. Non-inoculated controls never showed necrotic lesions. Lesions were visible from 72 h after inoculation. In the resistant line, lesions were small and fewer in numbers. There was a noticeable symptom difference between the susceptible and resistant maize inbred lines at 96 h after inoculation (Figure 1).
Sequencing and Mapping
From the sequencing of in planta libraries, approximately 31,994,420 resistant and 46,087,568 susceptible lines reads were generated (Table 1). The genome was mapped with reference genome Bipolaris_maydis_c5_gca_ 000338975.CocheC5_3.dna.toplevel.fa., and mapping statistics are provided in Supplementary Figure 2.

Table 1. Summary of the Illumina sequence reads obtained from Zea mays plants inoculated with B. maydis pathogen grown on sorghum seeds.
Differential Gene Expression Analysis
Approximately 23.4 GB of mRNA-seq data of B. maydis were obtained from both resistant and susceptible maize backgrounds. DEG analysis was conducted to detect B. maydis transcriptome changes during the pathogenesis of maize. Out of 10,363 mapped genes, 3313 genes were upregulated and only 100 genes were downregulated, and 6949 genes were commonly expressed in B. maydis SI plants compared to RI plants. A list of genes and their expression is provided in Supplementary Table 1. Log FCs and P-value are shown in Figure 3.
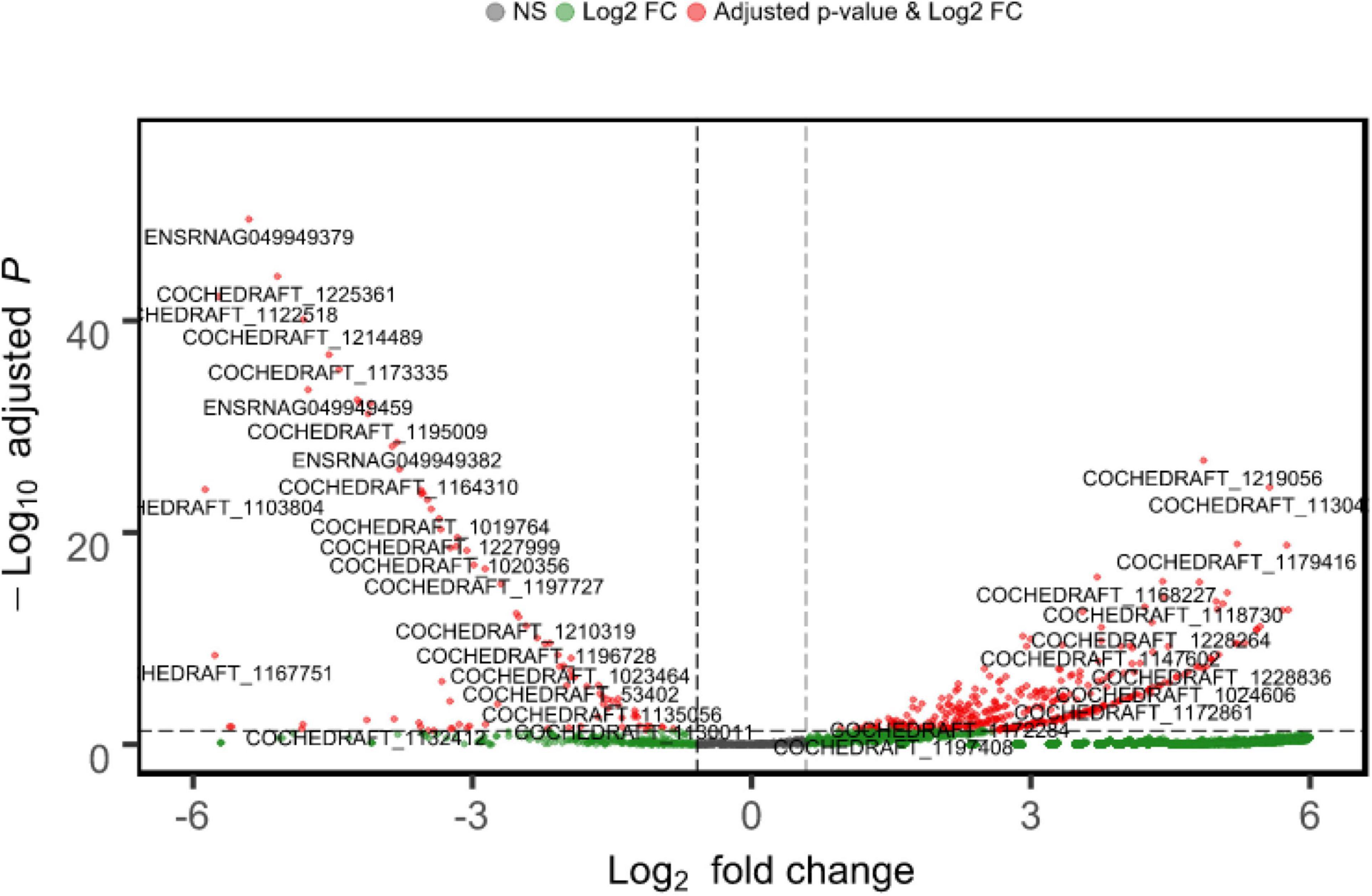
Figure 3. Mean expression versus log fold change plots (MA-plots). Transcriptional changes of B. maydis are presented in SC-7 and CM 119 48 h post inoculation. Normalized P-values are plotted versus Log2 fold changes. Genes with an FDR < 0.05 are plotted.
Transcriptome analysis of the top 40 DEGs suggested 20 upregulated and 20 downregulated genes, the detailed description of genes along with their fold change and function is provided in Tables 2, 3. Further, these DEGs were studied for genes related to pathogenesis and pathogen fitness which revealed important aspects, as shown in Table 4 and Supplementary Table 1. On the other hand, the data were also analyzed in silico to find out putative effectors using the Effector database and 22 effectors were predicted (Table 5).
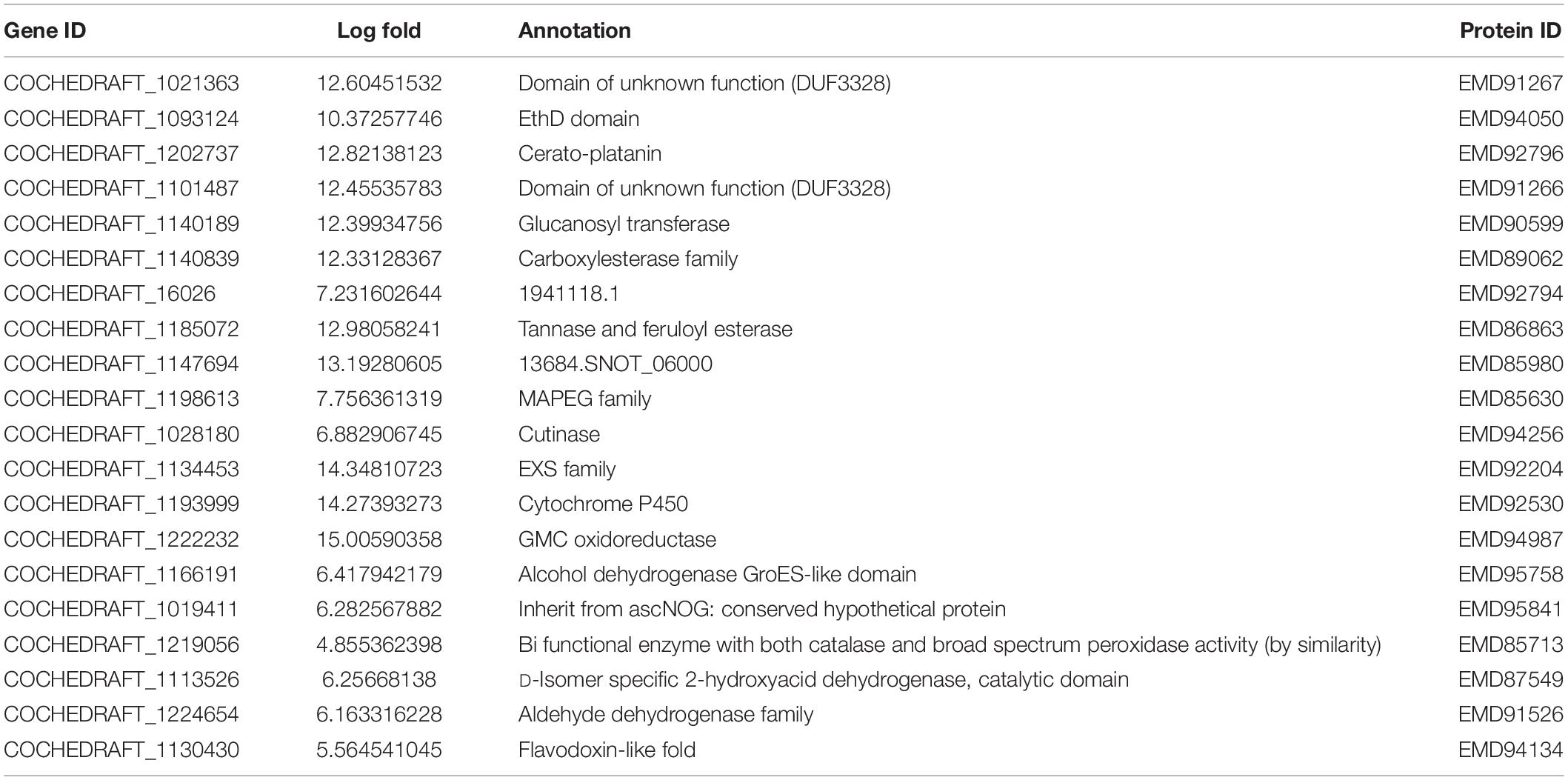
Table 2. Top 20 highly upregulated genes of B. maydis differentially expressed in inoculated resistant and susceptible plants.
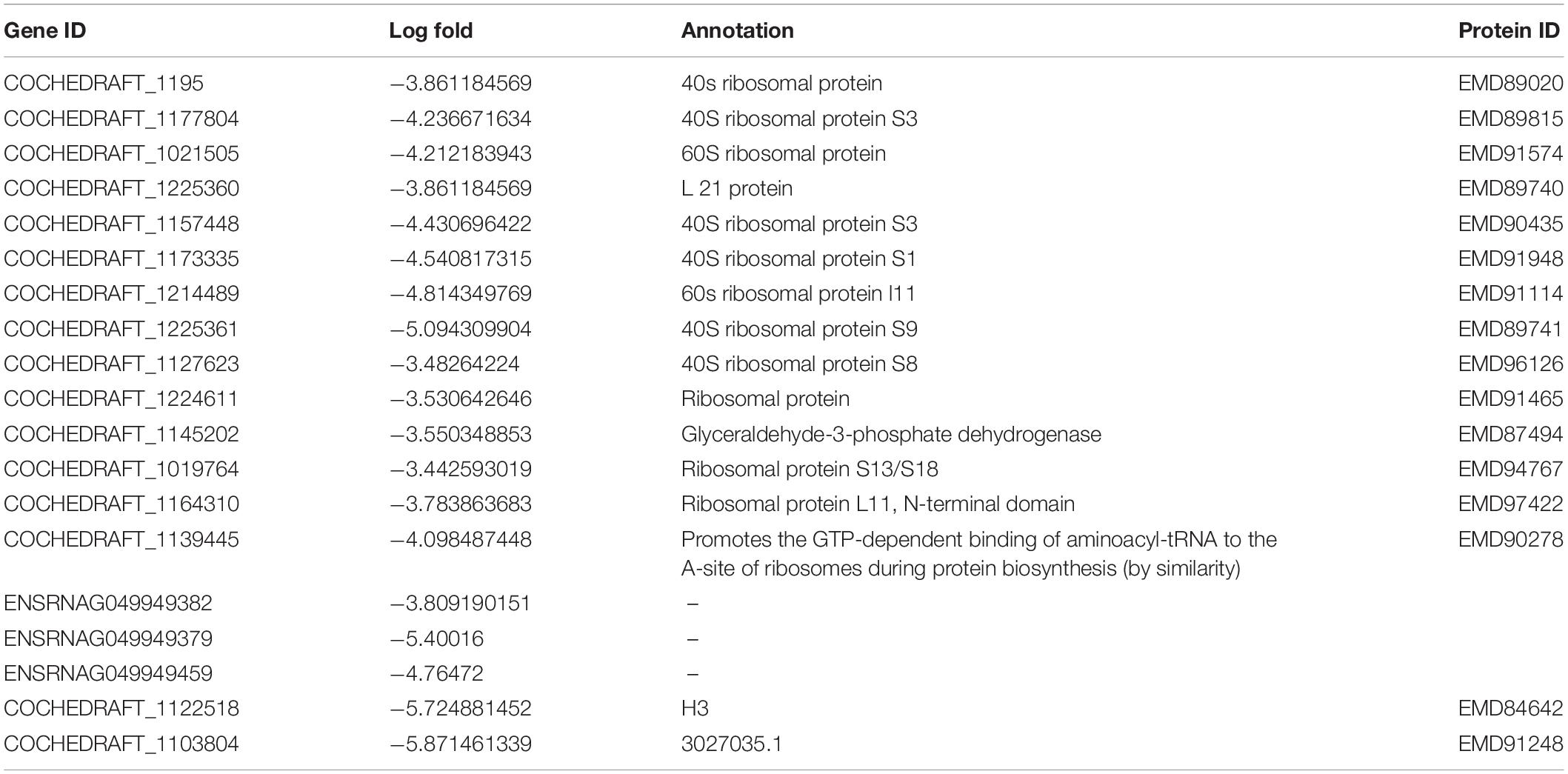
Table 3. Top 20 highly downregulated genes of B. maydis differentially expressed in inoculated resistant and susceptible plants.
GO Categories and Enrichment Analysis
GO enrichment suggested that most of the genes under DEGs were associated with pathogen fitness and reproduction. The results are presented in Figures 4–6 and described in Tables 6–8, with additional information available in Supplementary Tables 2–4.
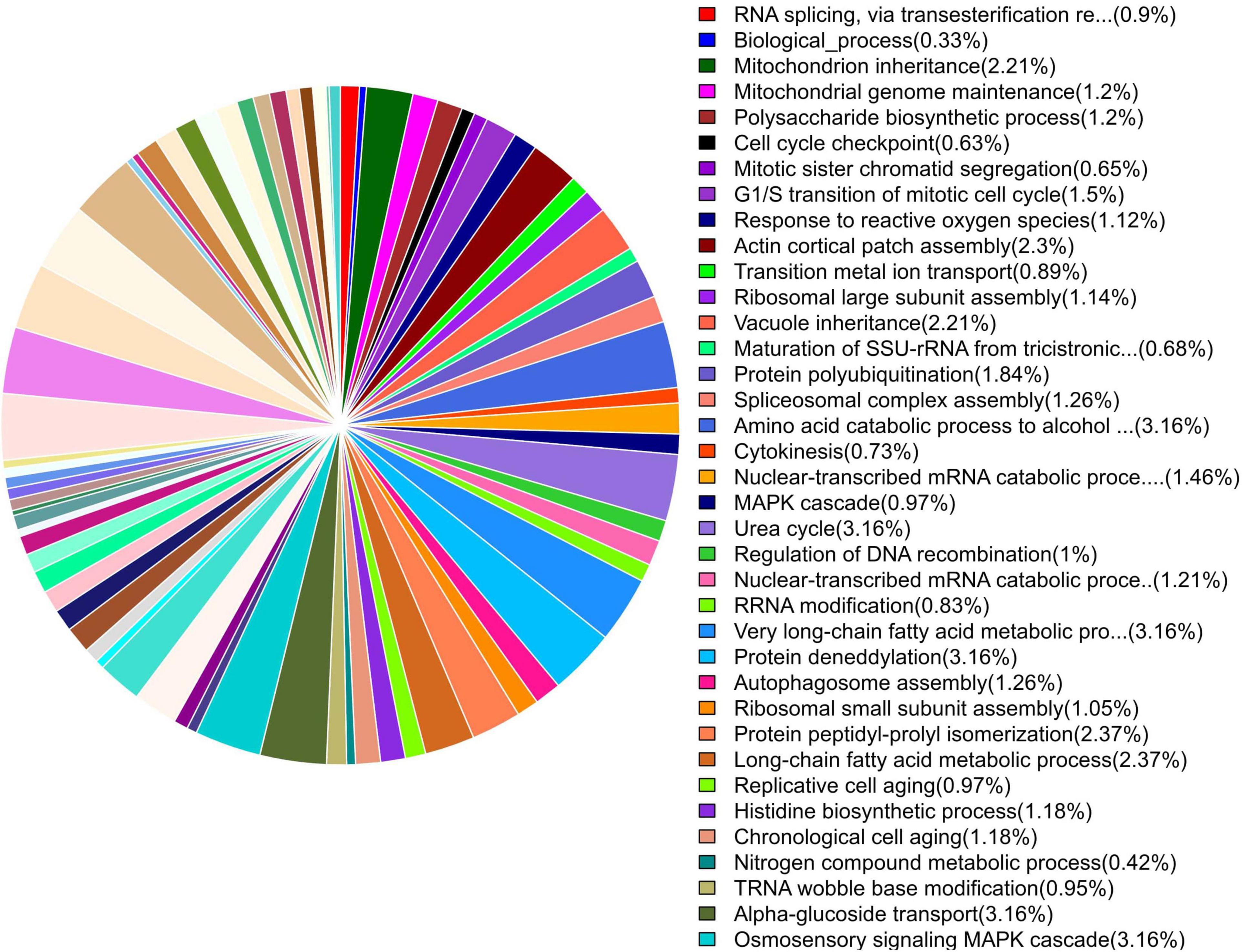
Figure 4. Pie chart of enriched GO terms of B. maydis for biological functions differentially expressed in inoculated resistant and susceptible plants.
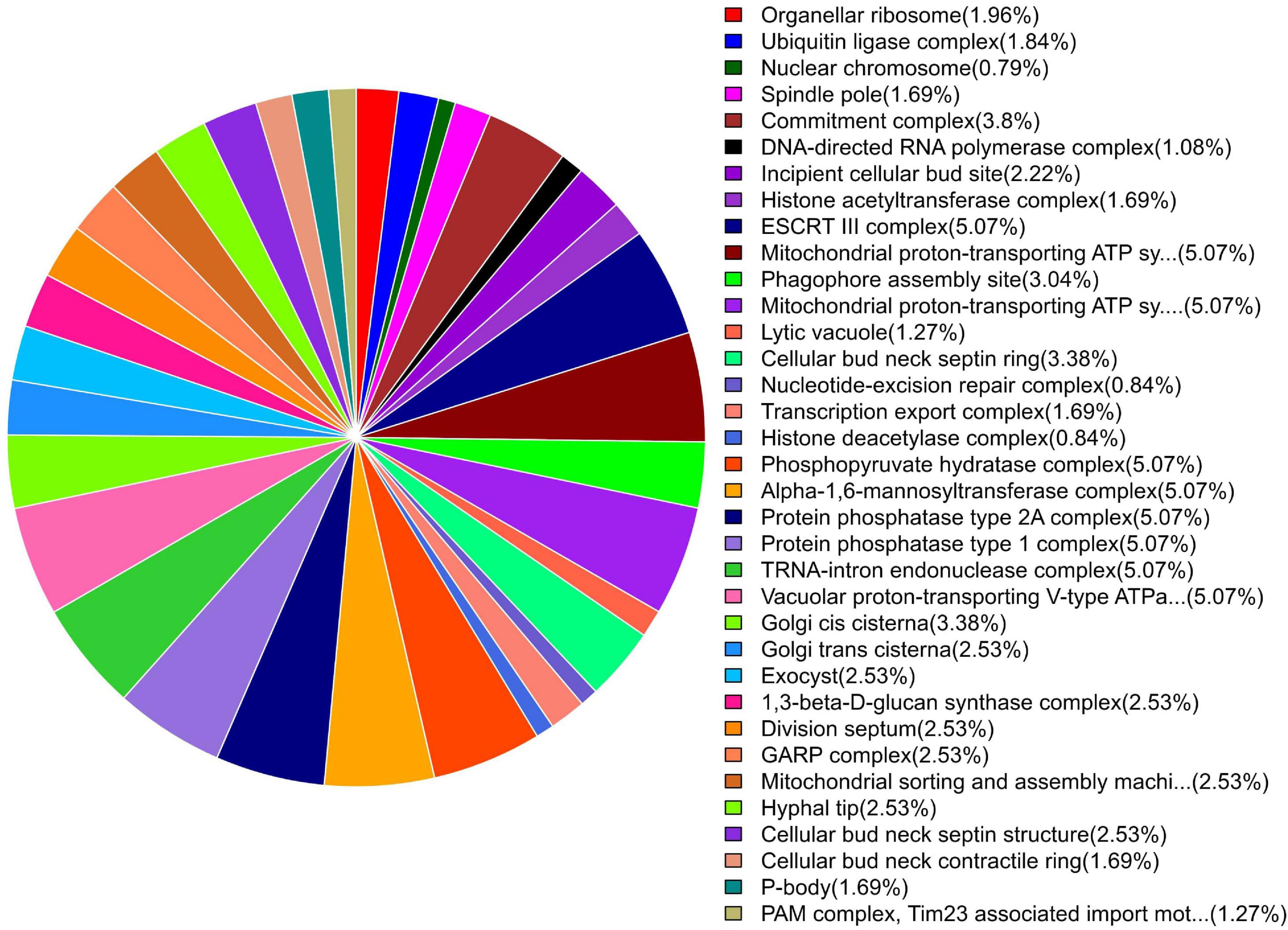
Figure 5. Pie chart of enriched GO terms of B. maydis for cellular functions differentially expressed in inoculated resistant and susceptible plants.
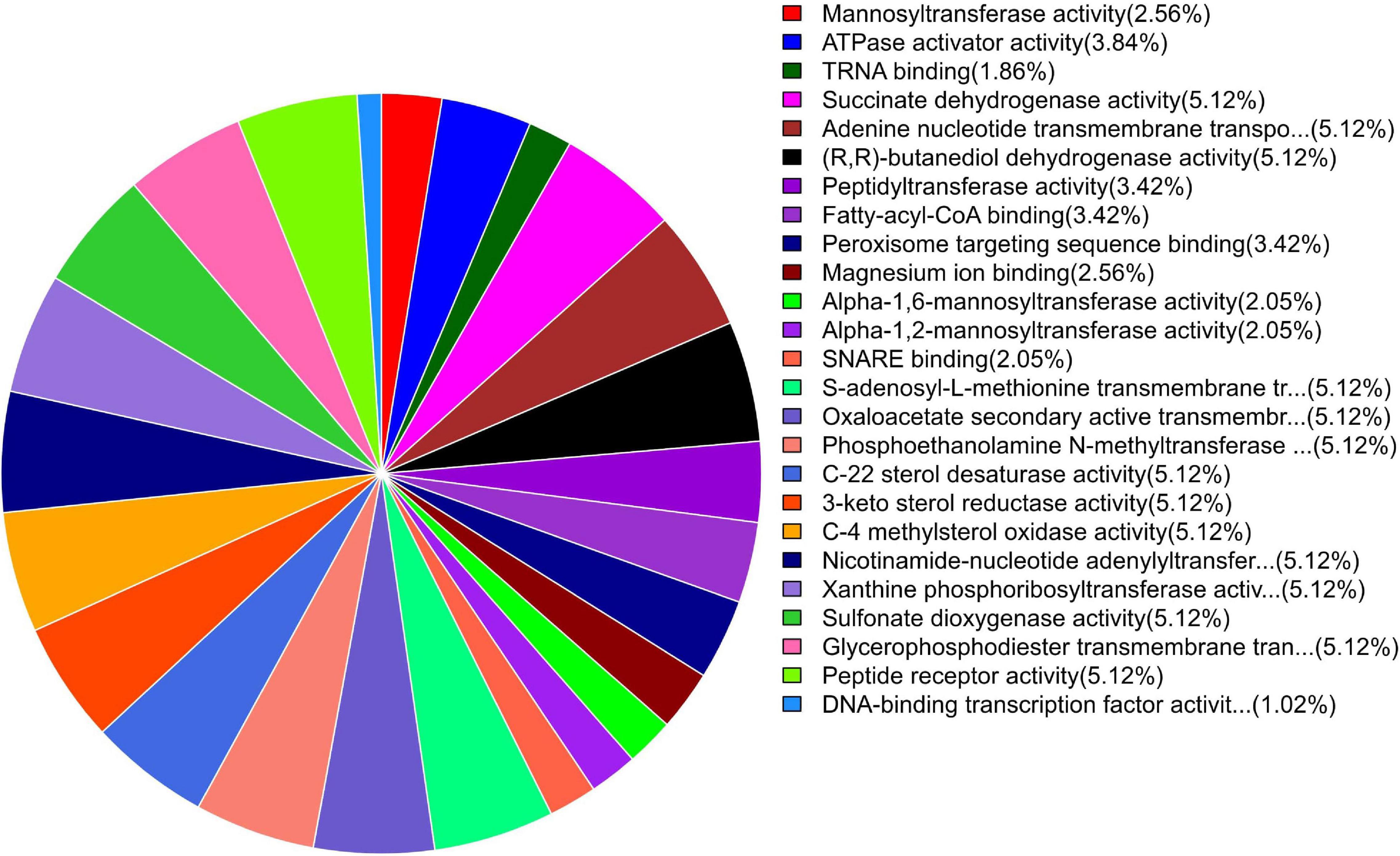
Figure 6. Pie chart of enriched GO terms of B. maydis for molecular functions differentially expressed in inoculated resistant and susceptible plants.
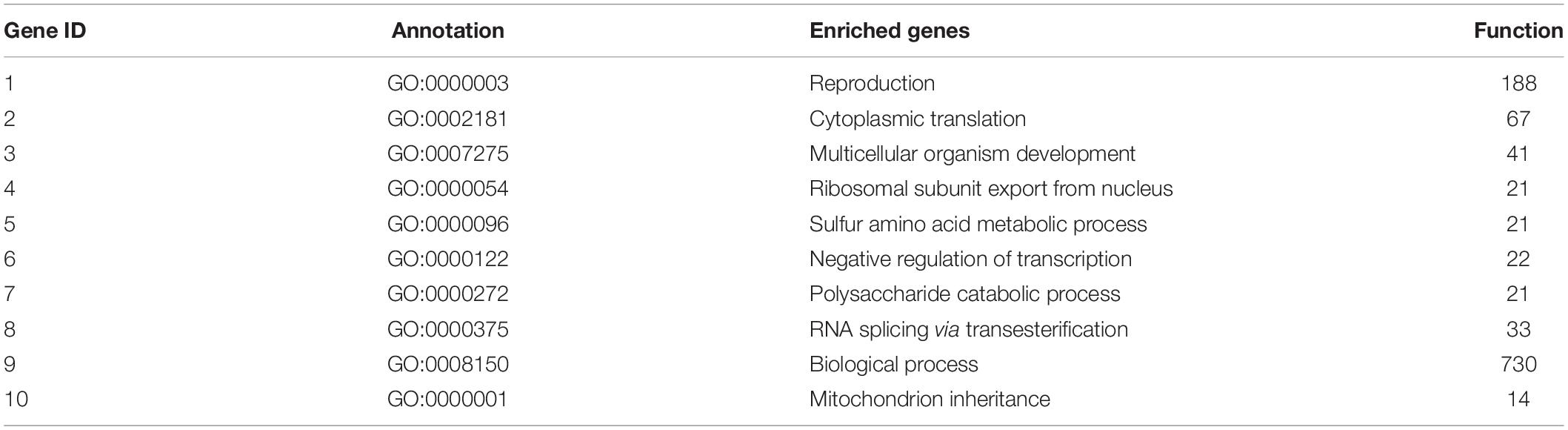
Table 6. Top 10 important enriched GO terms of B. maydis for biological functions differentially expressed in inoculated resistant and susceptible plants.
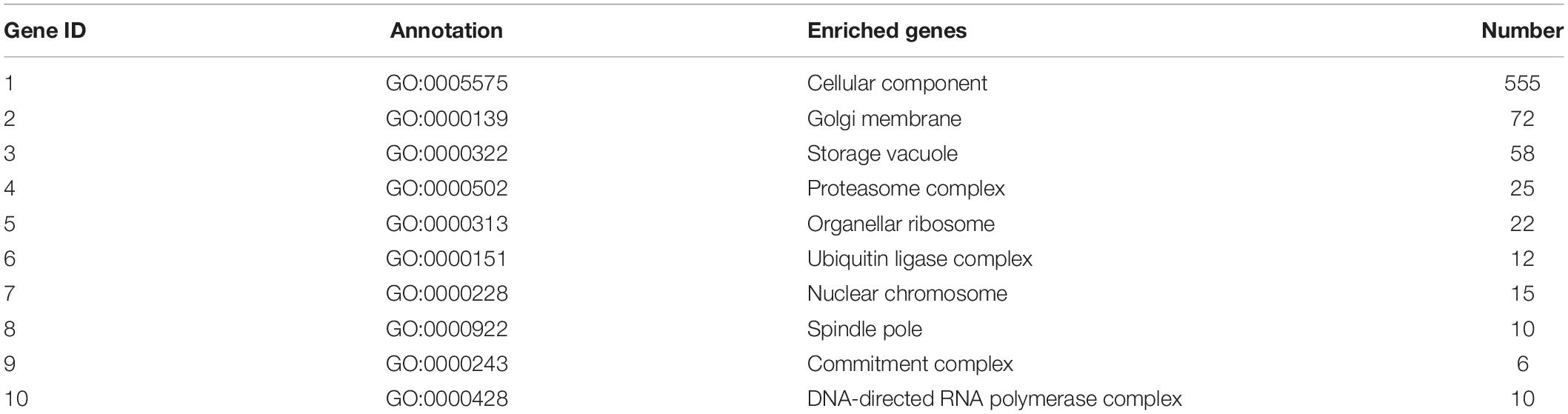
Table 7. Top 10 important enriched GO terms of B. maydis for cellular functions differentially expressed in inoculated resistant and susceptible plants.
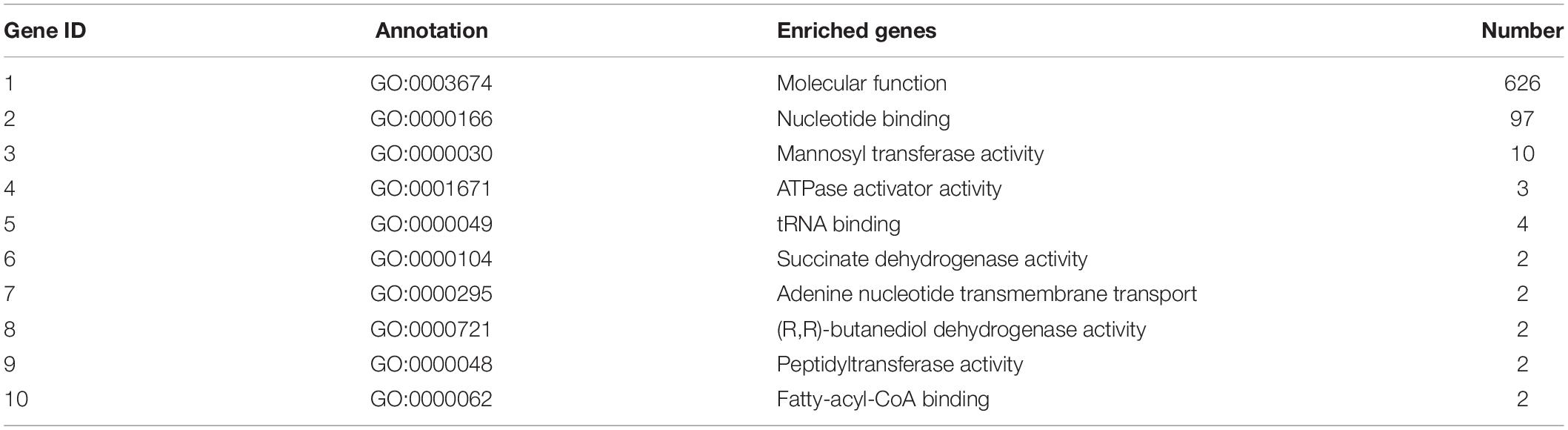
Table 8. Top 10 important enriched GO terms of B. maydis for molecular functions differentially expressed in inoculated resistant and susceptible plants.
Discussion
Enrichment analysis and the expression patterns of the highly upregulated genes indicated that successful pathogenicity of B. maydis depends on pathogen fitness genes such as mitochondrial genes, cell wall synthesis, toxin-related effector molecules, and on other hand, cell wall degradation of the host, detoxification, and host defense evasion (Figures 7–9). Understanding the pathogen’s molecular pathways during the infection process using transcriptome analysis can contribute significantly to identifying new targets for SSR control, novel genes, and pathogenicity-related pathways (Ribeiro et al., 2020; Poretti et al., 2021).
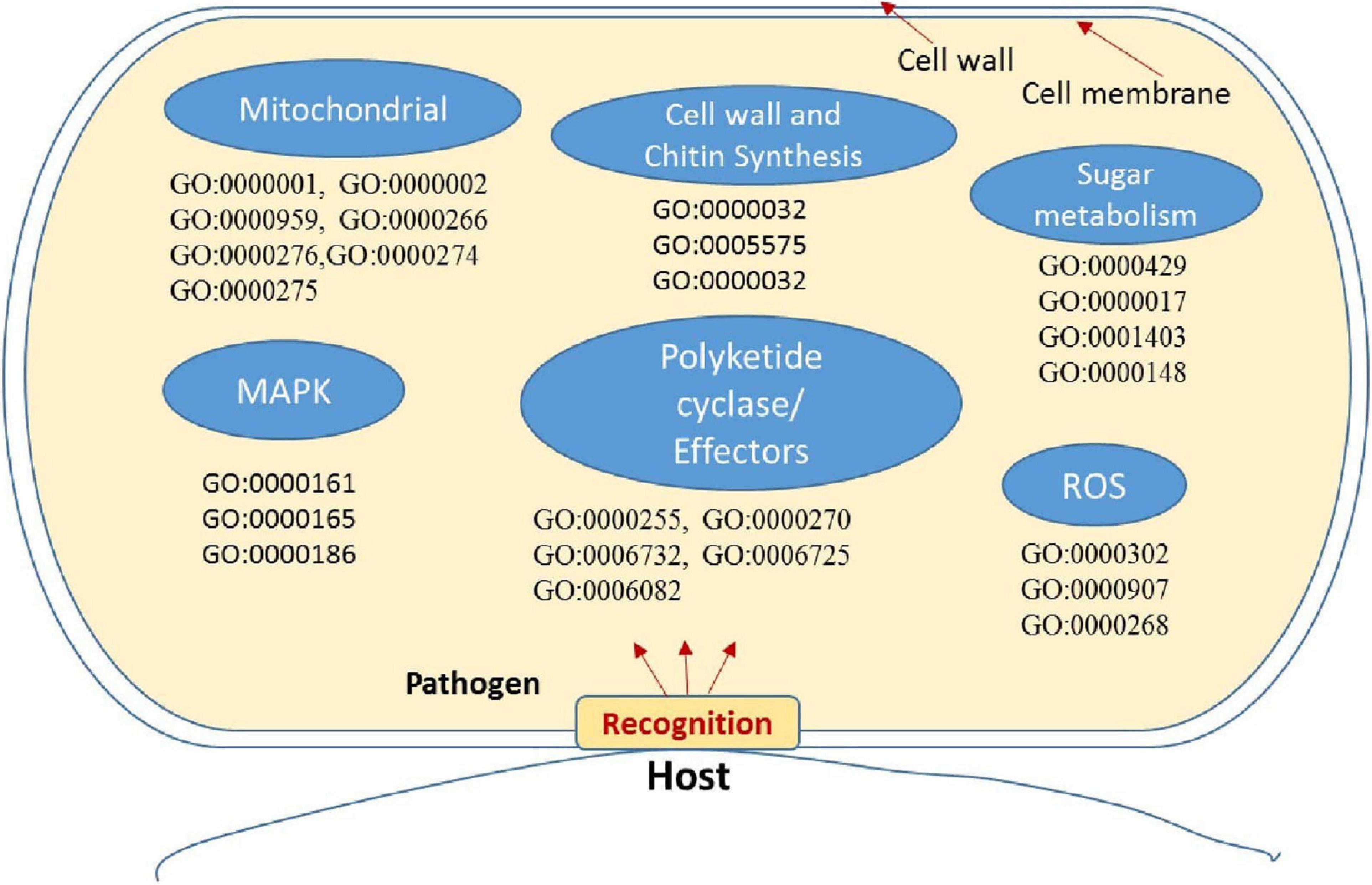
Figure 7. Putative representation of possible activation of genes and GO enriched terms in fungal pathogen Bipolaris maydis in susceptible maize (CM 119) compared to resistant maize (SC-7) during early stages of infection. GO term and associated gene IDs are provided in Supplementary Table 7.
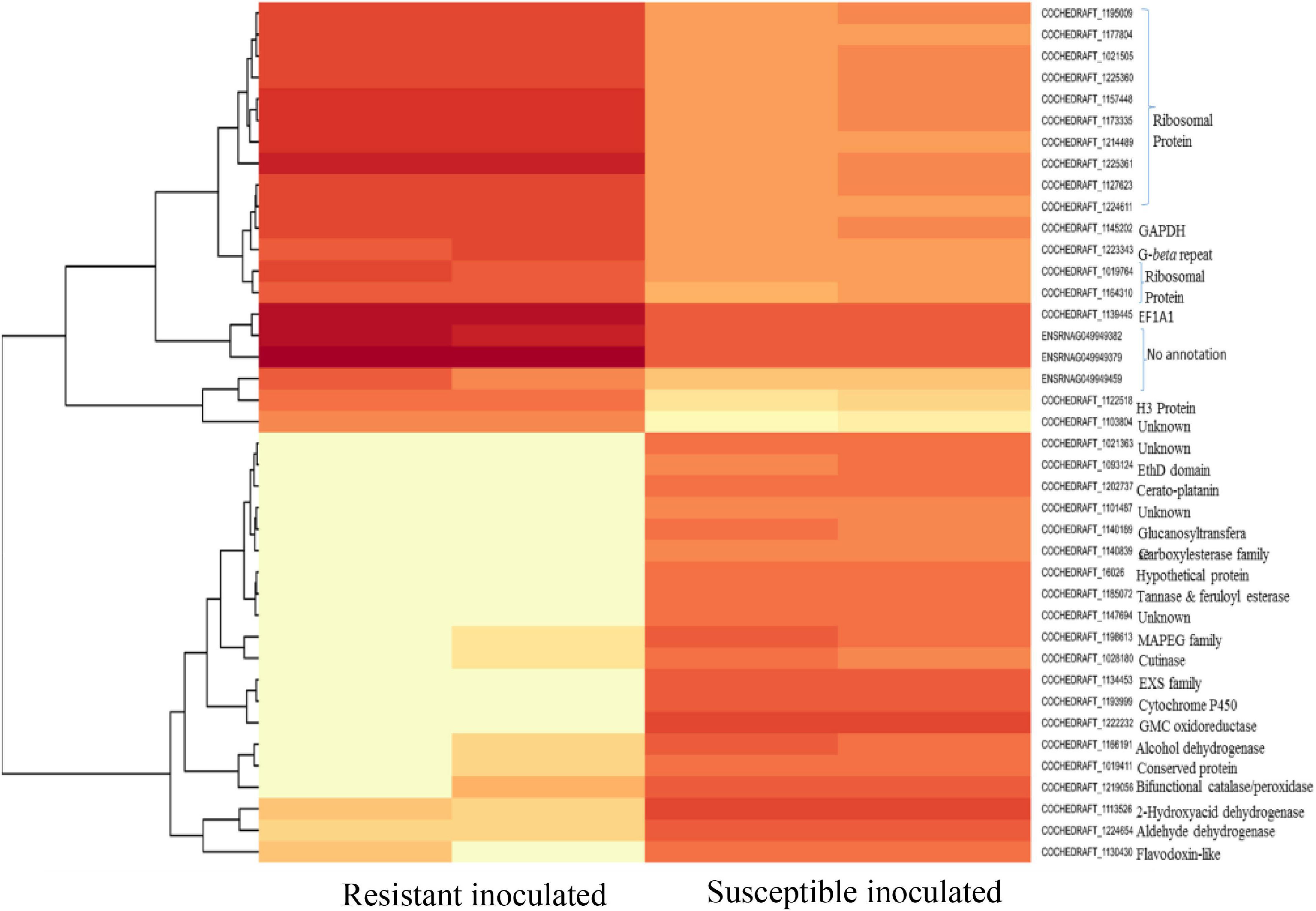
Figure 8. Heat map of top 40 differentially expressed genes of B. maydis in resistant and susceptible maize genotypes 48 h post inoculation.
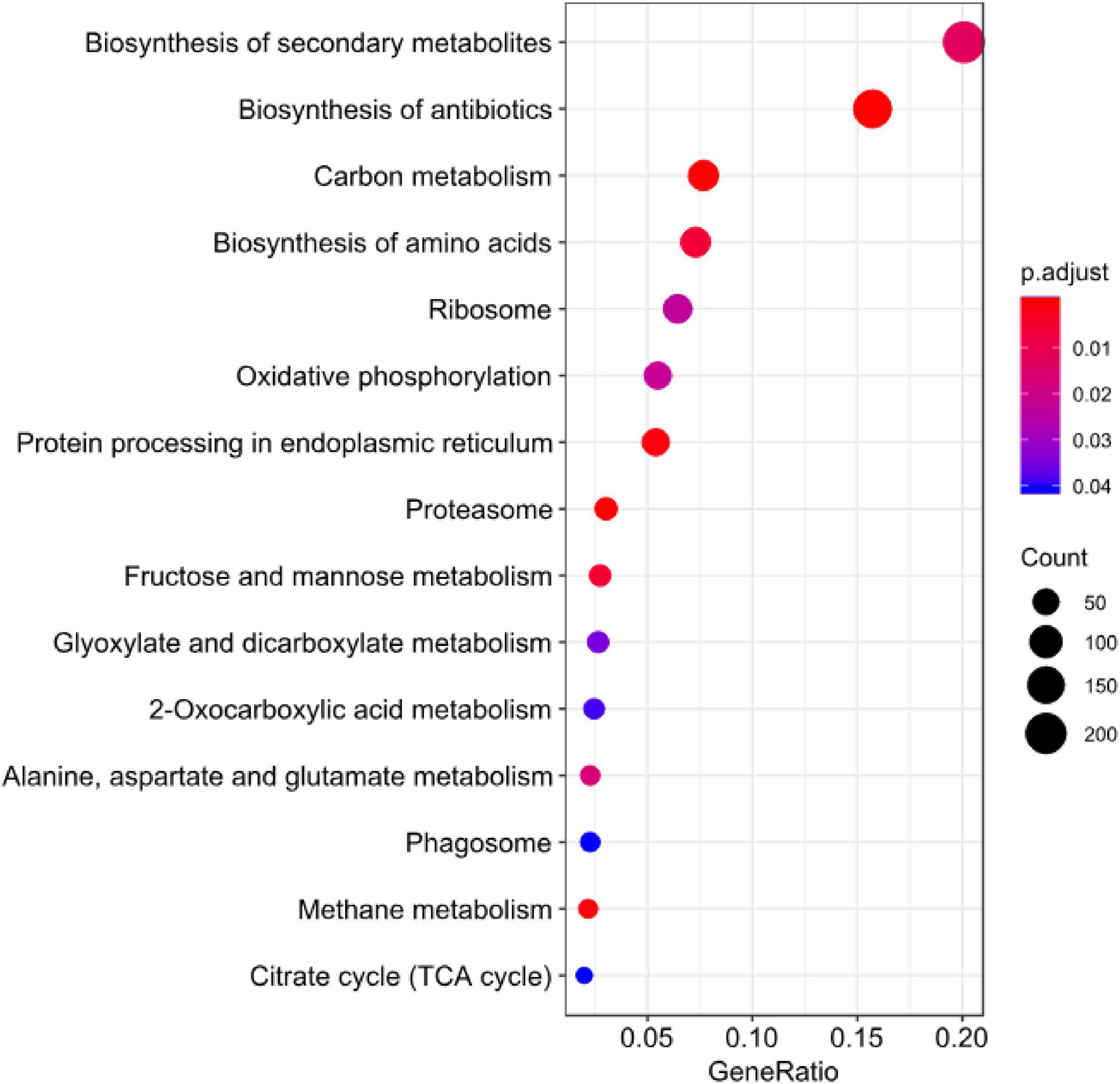
Figure 9. KEGG enrichment analysis for DEGs of B. maydis in maize susceptible and resistant genotypes at 96 h post inoculation.
Mitochondrial Genes
Mitochondria have diverse functions to perform in fungal cells. Mitochondria play a major role in fungal metabolism and fungicide resistance (Arcila et al., 2021). In the present study, genes associated with mitochondrial functions for B. maydis race “O” were upregulated in the susceptible line (CM 119), which suggests that CM 119 supports the growth of B. maydis, and on the other hand, SC-7 restricts the cellular activity of fungus (Figure 8). Previously studies suggested that fungal mitochondria play a significant role in determining fungal fitness and virulence (Calderone et al., 2015; Medina et al., 2020). A recent study suggests that endoplasmic reticulum (ER) and mitochondrial interactions along with the ER-mitochondria organizing network (ERMIONE) play important roles in adaptive responses in fungi, particularly in response to cell surface-related mechanisms that facilitate fungal invasion, growth, and stress responsive behaviors that support fungal pathogenicity (Koch et al., 2017). An investigation on C. parasitica study suggested the role of mitochondria in hypo virulence (Bertrand, 2000). Another study on the mitochondria genome of phytopathogens Synchytrium endobioticum and Phlebia radiate showed that alteration in the mitochondrial genome majorly affects the ability of fungi to adapt to changing environments (Medina et al., 2020). Overall, fungal mitochondria play a crucial role in determining pathogenicity of the host, and in susceptible backgrounds of the host, these genes are more expressed whereas resistant genotypes have a tendency to suppress the mitochondrial genes and ultimately the pathogen becomes less virulent, which we found in the present study.
Fungal Cell Wall and Chitin Synthesis Genes
The cell wall is an important component of fungal cells which mediates fungal cell interactions with its external environment and hyphal development (Castro et al., 2022). Chitin is the main component for cell wall synthesis. The cell wall protects the cell content, provides rigidity, and determines the cellular structure. It also protects the cell from various stresses including osmotic changes which are significant for healthy fungal cells. It also carries some proteins that play a role in recognition, adhesion, and receptor activity. There are several studies which establish the role of the fungal cell wall in pathogen fitness and its association with pathogenesis (Banks et al., 2005; Langner and Göhre, 2016; Pusztahelyi, 2018; Garcia-Rubio et al., 2020). It has also been investigated whether the fungal cell wall plays a crucial role in spore development (Backes et al., 2020) and in antifungal resistance activity (Díaz-Jiménez et al., 2012). In the present study, the high expression of cell wall-associated genes reconfirmed the fact that the fungal cell wall plays an essential role in disease development in susceptible hosts and also showed that the resistant genotype had the capacity to hinder the expression of fungal cell wall genes during the interaction (Figure 7).
Sugar Metabolism
Sugar transporter genes of filamentous fungi are associated with multiple physiological and biochemical processes, such as the response to various stresses (Vankuyk et al., 2004; Lv et al., 2020; Monfared et al., 2020). They were also found to be linked with many salt tolerance and sophisticated transcriptional processes. In the present transcriptome profile of B. maydis, genes of sugar metabolism (Figure 9) such as sugar transporter and glucanases were upregulated which suggests that these genes play an essential role in pathogen proliferation under susceptible backgrounds of the host.
Gene-Related Polyketides
Polyketides (PKs) play a role in mycelial growth and development of asexual and sexual structures of fungi (Gaffoor et al., 2005; Schindler and Nowrousian, 2014) including shikimate dehydrogenase (Kinghorn, 2000). A study on B. maydis race “T” demonstrated the association of a toxin-related locus with polyketide biosynthesis and high virulence on T-cytoplasm maize (Rose et al., 2002), similarly in the present study, high expression of PKs was investigated which suggests there could be a possible association of toxin “O” with PKs.
Other Important Genes Associated With Secondary Metabolites and Signaling
Recent studies proposed peroxidases, catalases, and reactive oxygen species (ROS) as components of the antioxidant defense system in fungal pathogens and were also associated with conidial production (Zhang et al., 2020). A study on fungal pathogen Magnaporthe oryzae suggested significant and positive correlations among sensitivity to H2O2, peroxidase activity, and fungal pathogenicity (Garre et al., 1998; Hansberg et al., 2012; Mir et al., 2015; Chittem et al., 2020). On the other hand, mitogen-activated protein kinase (MAPK) signaling pathways play an important role in cell cycle control, mating, morphogenesis, response to different stresses, resistance to UV radiation, temperature changes, cell wall assembly and integrity, degradation of cellular organelles, virulence, cell–cell signaling, fungus-plant interaction, and response to damage-associated molecular patterns (DAMPs) (Martínez-Soto and Ruiz-Herrera, 2017). Upregulation of these genes in the B. myadis race “O” pathogen indicated the interconnected nature in determining the fungal infection strategy in susceptible hosts (Figure 7).
Candidate Effector Genes Identified in Bipolaris maydis
At least 22 transcripts showing homology to genes previously reported to be involved in fungal infection were predicted as effector proteins (Table 5 and Figure 9) in the Effector database using the CSIRO tool EffectorP2 (a machine learning method for fungal effector prediction in secretomes) (Sperschneider et al., 2018). The majority of them showed a probability above 60–90%. This fact can provide evidence for the pathogenicity behavior of B. myadis race “O” on susceptible lines.
Conclusion
Based on the observation of present and previous studies in other host pathogen systems, we suggest that the above cited genes play a vital role in causing disease in their host plants. The DEG study of pathogen genes can provide evidence for its sensitive targets, virulence toward hosts, and resistance against chemicals. This is probably the first transcriptome study of the B. maydis pathogen during infection in a non-CMS maize genotype, differing in their susceptibility to the pathogen. The findings from this study emphasize the role of mitochondrial-associated genes and pathways. In addition, cell wall synthesis, genes related to synthesis of polyketides, toxins, and putative candidate effector genes were found to be the key compounds underlying the pathogenesis of the B. maydis race “O” pathogen.
Data Availability Statement
The datasets presented in this study can be found in online repositories. The names of the repository/repositories and accession number(s) can be found below: NCBI BioProject – PRJNA689117.
Author Contributions
SM, RG, BB, PM, FH, and AK were involved in the conceptualization of the project, study design, critical inputs, and finalization of the manuscript. PM, AK, and BB were involved in wet lab experiments. BB and PM were involved in bio-informatics analyses and data compilation. SM, RG, BB, and PM drafted the manuscript. All authors have read and approved the final manuscript.
Funding
SM was thankful to Centres for Advanced Agricultural Science and Technology – National Agricultural Higher Education Project (CAAST-NAHEP) for providing financial support and the Division of Plant Pathology, ICAR-IARI, New Delhi.
Conflict of Interest
The authors declare that the research was conducted in the absence of any commercial or financial relationships that could be construed as a potential conflict of interest.
Publisher’s Note
All claims expressed in this article are solely those of the authors and do not necessarily represent those of their affiliated organizations, or those of the publisher, the editors and the reviewers. Any product that may be evaluated in this article, or claim that may be made by its manufacturer, is not guaranteed or endorsed by the publisher.
Supplementary Material
The Supplementary Material for this article can be found online at: https://www.frontiersin.org/articles/10.3389/fmicb.2022.837056/full#supplementary-material
References
Ali, F., Rahman, H., Durrishahwar, N. F., Munir, M., and Ullah, H. (2011). Genetic analysis of maturity and morphological traits under maydis leaf blight (MLB) epiphytotics in maize (Zea mays L.). J. Agric. Biol. Sci. 6, 13–11.
Arcila, J. E., Arango, R. E., Torres, J. M., and Arias, T. (2021). Comparative genomics in plant fungal pathogens (Mycosphaerellaceae): variation in mitochondrial composition due to at least five independent intron invasions. Life 11:215.
Backes, A., Hausman, J. F., Renaut, J., Ait Barka, E., Jacquard, C., and Guerriero, G. (2020). Expression analysis of cell wall-related genes in the plant pathogenic fungus drechslera teres. Genes 11:300. doi: 10.3390/genes11030300
Bagnaresi, P., Biselli, C., Orrù, L., Urso, S., Crispino, L., Abbruscato, P., et al. (2012). Comparative transcriptome profiling of the early response to Magnaporthe oryzae in durable resistant vs susceptible rice (Oryza sativa L.) genotypes. PloS One 7:e51609. doi: 10.1371/journal.pone.0051609
Banks, I. R., Specht, C. A., Donlin, M. J., Gerik, K. J., Levitz, S. M., and Lodge, J. K. (2005). A chitin synthase and its regulator protein are critical for chitosan production and growth of the fungal pathogen Cryptococcus neoformans. Eukaryot. Cell 4, 1902–1912. doi: 10.1128/EC.4.11.1902-1912.2005
Bertrand, H. (2000). Role of mitochondrial DNA in the senescence and hypovirulence of fungi and potential for plant disease control. Annu. Rev. Phytopathol. 38, 397–422. doi: 10.1146/annurev.phyto.38.1.397
Calderone, R., Li, D., and Traven, A. (2015). System-level impact of mitochondria on fungal virulence: to metabolism and beyond. FEMS Yeast. Res. 15:fov027. doi: 10.1093/femsyr/fov027
Castro, I. S. L., Freitas-Lopes, R. D. L., Ferreira, S. D. S., Maciel, T. E. F., Florez, J. C., Zambolim, E. M., et al. (2022). Transcriptome Analysis Uncovers the Gene Expression Profile of Hemileia vastatrix (Race XXXIII) during the Interactions with Resistant and Susceptible Coffee. Agronomy 12:444.
Chittem, K., Yajima, W. R., Goswami, R. S., and del Río Mendoza, L. E. (2020). Transcriptome analysis of the plant pathogen Sclerotinia sclerotiorum interaction with resistant and susceptible canola (Brassica napus) lines. PLoS One 15:e0229844. doi: 10.1371/journal.pone.0229844
Díaz-Jiménez, D. F., Pérez-García, L. A., Martínez-Álvarez, J. A., and Mora-Montes, H. M. (2012). Role of the fungal cell wall in pathogenesis and antifungal resistance. Curr. Fungal Infect. Rep. 6, 275–282.
Fisher, D. E., Hooker, A. L., Lim, S. M., and Smith, D. R. (1976). Leaf infection and yield loss caused by four Helminthosporium leaf diseases of corn. Phytopath 66, 942–944.
Gaffoor, I., Brown, D. W., Plattner, R., Proctor, R. H., Qi, W., and Trail, F. (2005). Functional analysis of the polyketide synthase genes in the filamentous fungus Gibberella zeae (anamorph Fusarium graminearum). Eukaryot. Cell 4, 1926–1933. doi: 10.1128/EC.4.11.1926-1933.2005
Garcia-Rubio, R., de Oliveira, H. C., Rivera, J., and Trevijano-Contador, N. (2020). The fungal cell wall: Candida, Cryptococcus, and Aspergillus species. Front. Microbiol. 10:2993. doi: 10.3389/fmicb.2019.02993
Garre, V., Tenberge, K. B., and Eising, R. (1998). Secretion of a fungal extracellular catalase by Claviceps purpurea during infection of rye: putative role in pathogenicity and suppression of host defense. Phytopath 88, 744–753. doi: 10.1094/PHYTO.1998.88.8.744
Gregory, L. V., Ayers, J. E., and Nelson, R. R. (1978). Predicting yield losses in corn from southern corn leaf blight. Phytopath 68, 517–521.
Hansberg, W., Salas-Lizana, R., and Domínguez, L. (2012). Fungal catalases: function, phylogenetic origin and structure. Arch. Biochem. Biophys. 525, 170–180. doi: 10.1016/j.abb.2012.05.014
Hussain, N. (2011). Screening of maize varieties for grain yield at Dera Ismail Khan. J. Anim. Plant Sci. 21, 626–628.
Hyre, R. A. (1970). Epidemiology of southern corn leaf blight exploratory experiments. Plant Dis. Rep. 54, 1131–1133.
Kinghorn, A. D. (2000). Volume 1. Polyketides and Other Secondary Metabolites Including Fatty Acids and Their Derivatives Edited by Ushio Sankawa. Japan: Toyama Medical and Pharmaceutical University.
Koch, B., Tucey, T. M., Lo, T. L., Novakovic, S., Boag, P., and Traven, A. (2017). The mitochondrial GTPase Gem1 contributes to the cell wall stress response and invasive growth of Candida albicans. Front. Microbiol. 8:2555. doi: 10.3389/fmicb.2017.02555
Kuehnle, A. R., and Earle, E. D. (1988). Evaluation of tissue culture-derived methomyl resistant cms-T lines. Maize Genet. Coop. Newslett. 62:68.
Kumar, R., Mina, U., Gogoi, R., Bhatia, A., and Harit, R. C. (2016). Effect of elevated temperature and carbon dioxide levels on maydis leaf blight disease tolerance attributes in maize. Agric. Ecosyst. Enviro. 231, 98–104.
Lakshmi, P., and Sharma, R. C. (1987). Evaluation of maize germplasm to Helminthosporium maydis using detached leaf technique. Ann. Agric. Res. 8, 34–40.
Langner, T., and Göhre, V. (2016). Fungal chitinases: function, regulation, and potential roles in plant/pathogen interactions. Curr. Genet. 62, 243–254. doi: 10.1007/s00294-015-0530-x
Leonard, K. J. (1977). Races of Bipolaris maydis in the South eastern US from 1974-1976. Plant Dis. Rep. 61, 914–915.
Li, W., and Lan, P. (2015). Re-analysis of RNA-seq transcriptome data reveals new aspects of gene activity in Arabidopsis root hairs. Front. Plant Sci. 6:421. doi: 10.3389/fpls.2015.00421
Liao, Y., Smyth, G. K., and Shi, W. (2013). The Subread aligner: fast, accurate and scalable read mapping by seed-and-vote. Nucleic Acids Res. 41, e108–e108. doi: 10.1093/nar/gkt214
Liao, Y., Smyth, G. K., and Shi, W. (2014). featureCounts: an efficient general purpose program for assigning sequence reads to genomic features. Bioinformatics 30, 923–930. doi: 10.1093/bioinformatics/btt656
Liu, M., Gao, J., Yin, F., Gong, G., Qin, C., Ye, K., et al. (2015). Transcriptome analysis of maize leaf systemic symptom infected by Bipolaris zeicola. Plos One 10:e0119858. doi: 10.1371/journal.pone.0119858
Lv, G., Jiang, C., Liang, T., Tu, Y., Cheng, X., Zeng, B., et al. (2020). Identification and Expression Analysis of Sugar Transporter Gene Family in Aspergillus oryzae. Int. J. Genomics 2020:7146701. doi: 10.1155/2020/7146701
Martínez-Soto, D., and Ruiz-Herrera, J. (2017). Functional analysis of the MAPK pathways in fungi. Rev. Iberoam. Micol. 34, 192–202. doi: 10.1016/j.riam.2017.02.006
Medina, R., Franco, M. E. E., Bartel, L. C., Alcántara, V. M., Saparrat, M. C. N., and Balatti, P. A. (2020). Fungal Mitogenomes: Relevant Features to Planning Plant Disease Management. Front. Microbiol. 11:978. doi: 10.3389/fmicb.2020.00978
Mir, A. A., Park, S. Y., Sadat, M. A., Kim, S., Choi, J., Jeon, J., et al. (2015). Systematic characterization of the peroxidase gene family provides new insights into fungal pathogenicity in Magnaporthe oryzae. Sci. Rep. 5, 1–14. doi: 10.1038/srep11831
Mizuno, H., Kawahigashi, H., Kawahara, Y., Kanamori, H., Ogata, J., Minami, H., et al. (2012). Global transcriptome analysis reveals distinct expression among duplicated genes during sorghum-Bipolaris sorghicola interaction. BMC Plant Biol. 12:1–15. doi: 10.1186/1471-2229-12-121
Monfared, H. H., Chew, J. K., Azizi, P., Xue, G. P., Ee, S. F., Kadkhodaei, S., et al. (2020). Overexpression of a rice monosaccharide transporter gene (OsMST6) confers enhanced tolerance to drought and salinity stress in Arabidopsis thaliana. Plant Mol. Biol. Rep. 38, 151–164.
Mubeen, S., Rafique, M., Munis, M. F. H., and Chaudhary, H. J. (2017). Study of southern corn leaf blight (SCLB) on maize genotypes and its effect on yield. J. Saudi Soc. Agric. Sci. 16, 210–217.
Payak, M. M., and Sharma, R. C. (1983). Disease Rating Scales in Maize in India. In Techniques of Scoring for Resistance to Disease of Maize in India. All India Co- ordinated Maize Improvement project. New Delhi: IARI, 1–4.
Poretti, M., Sotiropoulos, A. G., Graf, J., Jung, E., Bourras, S., Krattinger, S. G., et al. (2021). Comparative transcriptome analysis of wheat lines in the field reveals multiple essential biochemical pathways suppressed by obligate pathogens. Front. Plant Sci. 17:70462. doi: 10.3389/fpls.2021.720462
Pusztahelyi, T. (2018). Chitin and chitin-related compounds in plant–fungal interactions. Mycology 9, 189–201. doi: 10.1080/21501203.2018.1473299
Rahul, K., and Singh, I. S. (2002). Inheritance of resistance to banded leaf and sheath blight (Rhizoctonia solam f. sp. Sasakii) of maize. Proc. 8th Asian Reg. Maize Works. Bangkok, Thailand 5, 356–365.
Ribeiro, T. H. C., Fernandes-Brum, C. N., de Souza, C. R., Dias, F. A. N., Almeida-Junior, O. D., Regina, M. D. A., et al. (2020). Transcriptome analyses suggest that changes in fungal endophyte lifestyle could be involved in grapevine bud necrosis. Sci. Rep. 10, 1–13. doi: 10.1038/s41598-020-66500-0
Rose, M. S., Yun, S. H., Asvarak, T., Lu, S. W., Yoder, O. C., and Turgeon, B. G. (2002). A decarboxylase encoded at the Cochliobolus heterostrophus translocation-associated Tox1B locus is required for polyketide (T-toxin) biosynthesis and high virulence on T-cytoplasm maize. Mol. Plant Microbe Interact. 15, 883–893. doi: 10.1094/MPMI.2002.15.9.883
Schindler, D., and Nowrousian, M. (2014). The polyketide synthase gene pks4 is essential for sexual development and regulates fruiting body morphology in Sordaria macrospora. Fung. Genet. Biol. 68, 48–59. doi: 10.1016/j.fgb.2014.04.008
Shieh, G. J., and Lu, H. S. (1993). Diallel analysis of mature plant resistance to Helminthosporium maydis in maize. J. Agric. Res. China 42, 12–18.
Sperschneider, J., Dodds, P. N., Gardiner, D. M., Singh, K. B., and Taylor, J. M. (2018). Improved prediction of fungal effector proteins from secretomes with EffectorP 2.0. Mol. Plant Pathol. 19, 2094–2110. doi: 10.1111/mpp.12682
Tajimi, A., Ando, N., and Teranaka, M. (1985). Seedling test for resistance to southern leaf blight in corn under greenhouse conditions. III Virulence of six isolates of Bipolaris maydis collected at six localities in southwest Japan. Bull. Natl. Grassl. Res. Inst. 31, 68–72.
Ullstrup, A. J. (1972). The impacts of the southern corn leaf blight epidemics of 1970-1971. Annu. Rev. Phytopathol. 10, 37–50.
Vankuyk, P. A., Diderich, J. A., MacCABE, A. P., Hererro, O., Ruijter, G. J., and Visser, J. (2004). Aspergillus niger mstA encodes a high-affinity sugar/H+ symporter which is regulated in response to extracellular pH. Biochem. J. 379, 375–383. doi: 10.1042/BJ20030624
Venkatesh, I., Gogoi, R., Hossain, F., Kumar, A., Aggarwal, R., and Mandal, P. K. (2021). Confirmation of physiological race of Bipolaris maydis causing maydis leaf blight of maize in India. Ind. J. Agri. Sci. 91, 613–618.
Warren, H. L., Jones, A., and Huber, D. M. (1977). Morphological and physiological differences between Bipolaris maydis races O and T. Mycologia 69, 773–782.
White, D. G. (1999). Compendium of Corn Diseases, third ed. St. Paul, MN, USA: American Phytopathological Society.
Yazawa, T., Kawahigashi, H., Matsumoto, T., and Mizuno, H. (2013). Simultaneous transcriptome analysis of Sorghum and Bipolaris sorghicola by using RNA-seq in combination with de novo transcriptome assembly. PloS One 8:e62460. doi: 10.1371/journal.pone.0062460
Ye, W., Liu, T., Zhang, W., Li, S., Zhu, M., Li, H., et al. (2019). Disclosure of the Molecular Mechanism of Wheat Leaf Spot Disease Caused by Bipolaris sorokiniana through Comparative Transcriptome and Metabolomics Analysis. Int. J. Mol. Sci. 20:6090. doi: 10.3390/ijms20236090
Zaitlin, D., DeMars, S., and Ma, Y. (1993). Linkage of rhm, a recessive gene for resistance to southern corn leaf blight, to RFLP marker loci in maize (Zea mays) seedlings. Genome 36, 555–564. doi: 10.1139/g93-076
Zhang, M. Z., Sun, C. H., Liu, Y., Feng, H. Q., Chang, H. W., Cao, S. N., et al. (2020). Transcriptome analysis and functional validation reveal a novel gene, BcCGF1, that enhances fungal virulence by promoting infection-related development and host penetration. Mol. Plant Path. 21, 834–853. doi: 10.1111/mpp.12934
Keywords: Bipolaris maydis race “O”, non-CMS maize, RNA-seq, host–pathogen interaction, differentially expressed genes (DEGs), effectors
Citation: Meshram S, Gogoi R, Bashyal BM, Kumar A, Mandal PK and Hossain F (2022) Comparative Transcriptome Analysis of Fungal Pathogen Bipolaris maydis to Understand Pathogenicity Behavior on Resistant and Susceptible Non-CMS Maize Genotypes. Front. Microbiol. 13:837056. doi: 10.3389/fmicb.2022.837056
Received: 16 December 2021; Accepted: 02 March 2022;
Published: 29 April 2022.
Edited by:
Marco Scortichini, Council for Agricultural and Economics Research (CREA), ItalyReviewed by:
Rui Shi, North Carolina State University, United StatesJialing Bao, Southwest University, China
Copyright © 2022 Meshram, Gogoi, Bashyal, Kumar, Mandal and Hossain. This is an open-access article distributed under the terms of the Creative Commons Attribution License (CC BY). The use, distribution or reproduction in other forums is permitted, provided the original author(s) and the copyright owner(s) are credited and that the original publication in this journal is cited, in accordance with accepted academic practice. No use, distribution or reproduction is permitted which does not comply with these terms.
*Correspondence: Robin Gogoi, ci5nb2dvaWlhcmlAZ21haWwuY29t