- 1Biomedical Research Center (CINBIO), Universidade de Vigo, Vigo, Spain
- 2Hospital Álvaro Cunqueiro, Galicia Sur Health Research Institute (IIS-GS), Vigo, Spain
While Tuberculosis (TB) infection remains a serious challenge worldwide, big data and “omic” approaches have greatly contributed to the understanding of the disease. Transcriptomics have been used to tackle a wide variety of queries including diagnosis, treatment evolution, latency and reactivation, novel target discovery, vaccine response or biomarkers of protection. Although a powerful tool, the elevated cost and difficulties in data interpretation may hinder transcriptomics complete potential. Technology evolution and collaborative efforts among multidisciplinary groups might be key in its exploitation. Here, we discuss the main fields explored in TB using transcriptomics, and identify the challenges that need to be addressed for a real implementation in TB diagnosis, prevention and therapy.
Introduction
The Challenge of Tuberculosis Disease
Tuberculosis (TB) remains a relentless disease caused by the bacillus Mycobacterium tuberculosis. With more than 1.5 million deaths annually, it is one of the top causes of death worldwide and the second leading cause from a single infectious agent, after COVID-19 (World Health Organization [WHO], 2021). Tuberculosis disease displays a continuous spectrum. After the bacteria enters the body, it can be rapidly eliminated by the innate immune system (“early clearance”) or it can persist, triggering the response of the adaptive immune system. Only 5–10% of the infected people directly progress to the active disease, whereas in most cases the mycobacteria enters into a dormant state and persists in the organism trough years, as latent TB infection (LTBI). This latent stage can eventually lead toward bacteria reactivation (“post-primary TB”) or the disease resolution.
LTBI has classically included those asymptomatic people that developed immune memory against TB. It is now stablished that other scenarios might occur, including rapid (“incipient disease,” with no evidence of active Mtb) or slow (“subclinical tuberculosis,” with radiological abnormalities or microbiological evidence of active Mtb) progression toward the active disease, cycling between stages, or the effective elimination of the infection after developing memory (Drain et al., 2018). Nonetheless, most routine diagnostic tests do not differentiate among these stages. It is estimated that about one-quarter of the world’s population has contacted with the bacilli and developed immune memory reflected in the definition of LTBI.
Understanding the factors that promote the diverse and dynamic stages of the infection, including early clearance of the pathogen and active disease, is therefore key to develop an appropriate strategy in the fight against TB.
The economic barriers between high and low-income areas usually affect TB diagnosis and treatment, as well as the outcome of the patient. Moreover, the increasing rates of multidrug-resistant (MDR-TB) and extensively drug-resistant TB (XDR-TB) threats TB management in the future. The failure in developing an effective vaccine for adult pulmonary TB, opens another front in TB research and in the control of the disease.
Development of high-throughput (HT) technologies were expected to impulse the understanding of the complexity of this disease. They enabled the revolution of the omic sciences, including genomics, transcriptomics, proteomics, epigenomics, or metabolomics.
The term transcriptomics refers to the study of the complete set of RNA transcripts produced by the genome at a specific time or circumstance. Their studies are being used in many areas of knowledge like toxicology, immunology, microbiology, environmental studies or evolutionary relationships among species. In medicine, it is a powerful tool for the understanding of many human diseases, including infections, cancer or autoimmune diseases.
Transcriptomics cannot predict the post-transcriptional protein modifications that usually affect the protein’s function. As a matter of fact, the comparison among transcriptome and proteome commonly fails to show a good correlation (Kumar et al., 2016). Nonetheless, the complexity of proteome analysis limits its understanding. Therefore, gene expression analysis are routinely accepted as a proxy for biological activity, being more affordable and manageable than proteomics. Furthermore, transcriptomics allows the detection of low abundant gene transcripts, and the identification of novel isoforms resulting from alternative splicing or single nucleotide polymorphisms, where proteomic fails. Researchers must understand and accept the limitations of both systems when choosing their approach.
Given these limitations, studies that include both approaches can provide a more complete picture of the processes that characterize the disease. A combination of omic approaches with immune profiling techniques, such as flow cytometry, in vitro cell activation or measurement of cytokine secretion, etc., can help to identify the immunological mechanisms involved in the different stages of the disease, which is key in the development of both diagnostic and treatment approaches.
Transcriptome Studies: Exploring the Past…
Transcriptome can be partially studied using targeted expression approaches, as real-time quantitative reverse transcription PCR (RT-qPCR) or differential display for measuring gene expression, or RNA interference for exploring gene function. Nonetheless, the usage of high throughput technologies allows the unbiased acquisition of tens of thousands of potential candidate genes in just one step.
The first approaches for the gene expression analysis emerged in the early 1990 ìs and were based on Sanger sequencing. They included Expressed Sequence Tag (EST) libraries, Serial Analysis of Gene Expression (SAGE), Cap Analysis of Gene Expression (CAGE), and Massively Parallel Signature Sequencing (MPSS). They had various limitations, including high costs due to the Sanger sequencing technology, dependence on enzyme recognition sites, and limitations in detecting isoforms (Wang et al., 2009). Reverse-transcriptase multiplex ligation-dependent probe amplification (RT-MLPA) is also used to quantify expression of a predefined list of target genes, but fails in assessing hundreds of genes simultaneously (Eldering et al., 2003).
These methods were soon replaced by hybridization-based microarray technology which has a much better affordability for large scale studies. Next-generation sequencing, and thus RNA-Seq, arose later, in mid 2000s (Figure 1). Currently, high-throughput transcriptomics (HTTr) rely on those two platforms.
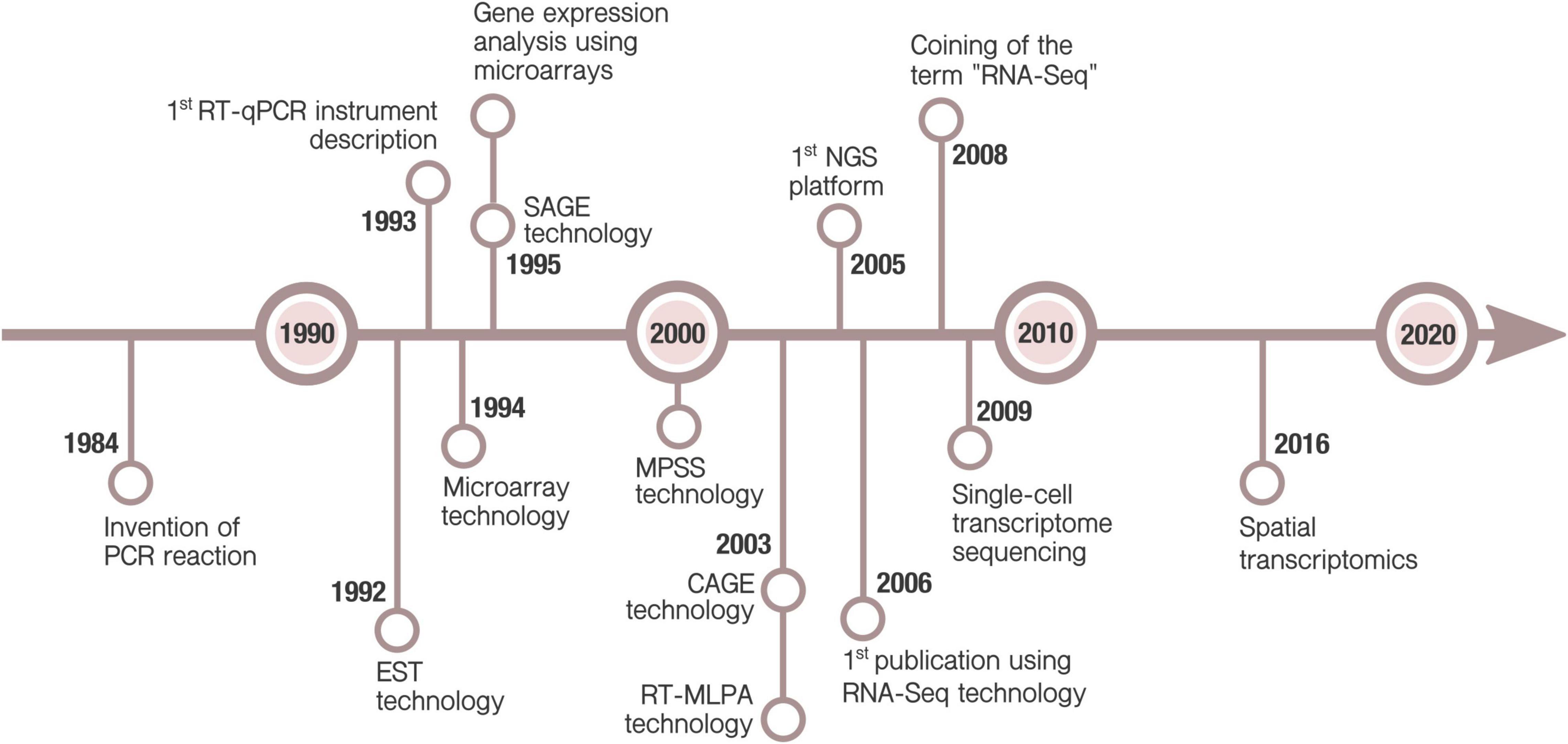
Figure 1. Timeline of main milestones in technologies for gene expression. Polymerase Chain Reaction (PCR) was invented in 1984 by Mullis (1990). A decade later, Real-Time qPCR (RT-qPCR) technology enabled the detection of PCR products in real-time (Higuchi et al., 1993). This allowed, for the first time, quantify mRNA expression of selected genes. Simultaneous analysis of multiple genes was possible due to microarray, Expressed Sequence Tag (EST) (Okubo et al., 1992), Serial Analysis of Gene Expression (SAGE) (Velculescu et al., 1995), Massively Parallel Signature Sequencing (MPSS) (Brenner et al., 2000), Cap Analysis of Gene Expression (CAGE) (Shiraki et al., 2003) and Reverse-transcriptase multiplex ligation-dependent probe amplification (RT-MLPA) (Eldering et al., 2003) technologies. Although the DNA hybridization method was described earlier, the microarray technology is considered to be firstly commercialized by Affymetrix in 1994 (Lenoir and Giannella, 2006). It was promptly applied to measure gene expression (Schena et al., 1995). It continues to be one of the most popular methods for gene expression, allowing the analysis of hundreds or thousands of genes. Development of Next Generation Sequencing (NGS) in the onset of 2000s supposed a revolution for both genomics and transcriptomics (Mardis, 2011). The first publication using NGS RNA-Seq technology was in 2006 (Bainbridge et al., 2006), but it was not until 2008 when the term RNA-seq started to be used (Lister et al., 2008; Mortazavi et al., 2008). RNA-Seq was applied to single-cell technology in 2009 for the first time (Tang et al., 2009). Emerging applications, as spatial transcriptomics (Ståhl et al., 2016), hold the promise of new advances in transcriptomics research.
For some time, RNA microarrays were nonetheless preferred over RNA-Seq because of its reduced price. Now, the equalization of costs of both techniques have favored the popularity of RNA-Seq for exploratory research, as which present various advantages over microarrays, but also have a few shortcomings (Table 1). In TB, where the discovery of novel biomarkers is still required, transcriptomics and RNA-Seq are considered the most informative and cost effective assays to start with. Once established a set of genes of interest, further exploration can be done using a well-designed microarray or by RT-qPCR, reducing complexity and costs.
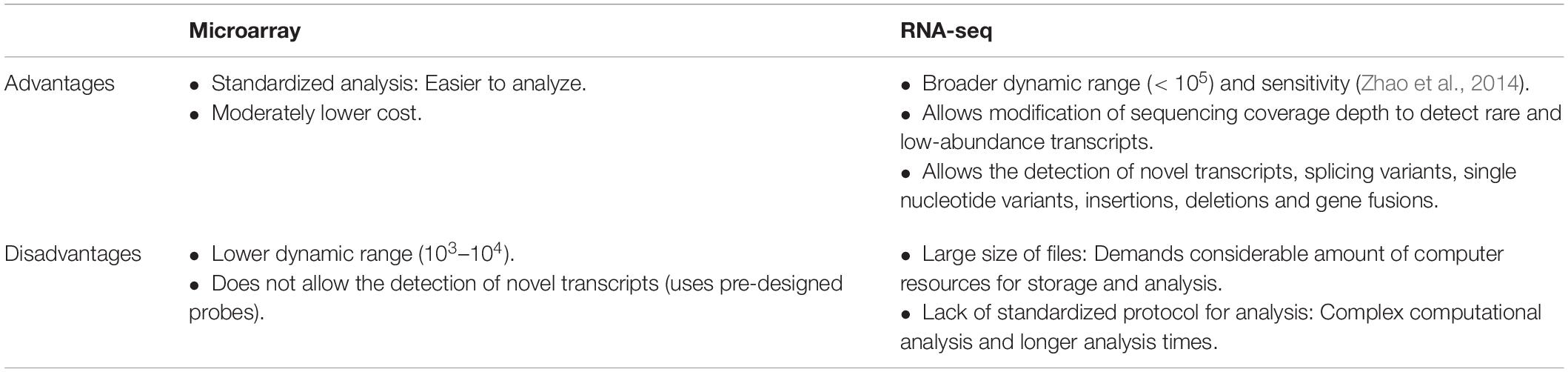
Table 1. Advantages and disadvantages of microarrays and RNA-sequencing for gene expression analysis.
Another major contributor to the transcriptomics success is the development of single-cell RNA-Seq technology and spatial transcriptomics. While single-cell proteomics is still in its infancy, single-cell transcriptomics allows an unprecedent analysis of gene expression and its heterogeneity amongst different cell populations, rare cell detection or complex interactions among tissues (Cai et al., 2020; Nathan et al., 2021; Pisu et al., 2021). Some drawbacks are its elevated cost, with reagents 10–20 times more expensive than conventional RNA-Seq, and the complexity of its analysis, facing new computational challenges such as the necessity of dimensionality reduction and robust statistics to detect true heterogeneity (Adil et al., 2021). Spatial transcriptomics, which combines gene expression analysis and histology, allows for the first time the localization of mRNA molecules within a tissue or identifying their subcellular location (Ståhl et al., 2016). It has rapidly become a promising tool in research and diagnostics, with special applications in assessing tissue heterogeneity and cell interactions.
HTTr studies have boosted the amount of available information to levels never reached before. The large-scale data obtained requires of bioinformatics tools to be adequately processed and understood. Data can be stored in either private or public storage. Most scientific publications require the transcriptomic raw data to be available in public repositories, where it can be freely downloaded. Some examples of public repositories for transcriptomic data can be found in Table 2.
Nonetheless, special care must be taken when comparing data from different sources. A meticulous annotation must be done when sharing our data, including the experimental protocol, procedure for sample isolation, relevant donor information, microarray design or RNA-Seq technology used or selection of either total RNA or mRNA fraction for RNA-Seq. The availability of a complete and curated metadata will ease further in silico analysis comparing data worldwide.
An increasing number of bioinformatics tools are developed for the different steps of the transcriptome analysis, with many alternatives available for each step. RNA-Seq analysis share some common steps (Figure 2), but there is not a unique pipeline that one can follow and the pipeline of choice depends mainly on the biological question of interest. To take full advantage of our data, it is desired to keep updating our knowledge of useful and reliable software. The sustained software development also hinders experts in the field to review and criticize how most data is analyzed. Lastly, communication issues among biological scientists and data analysts may also become a handicap to get the most out of the experimental data.
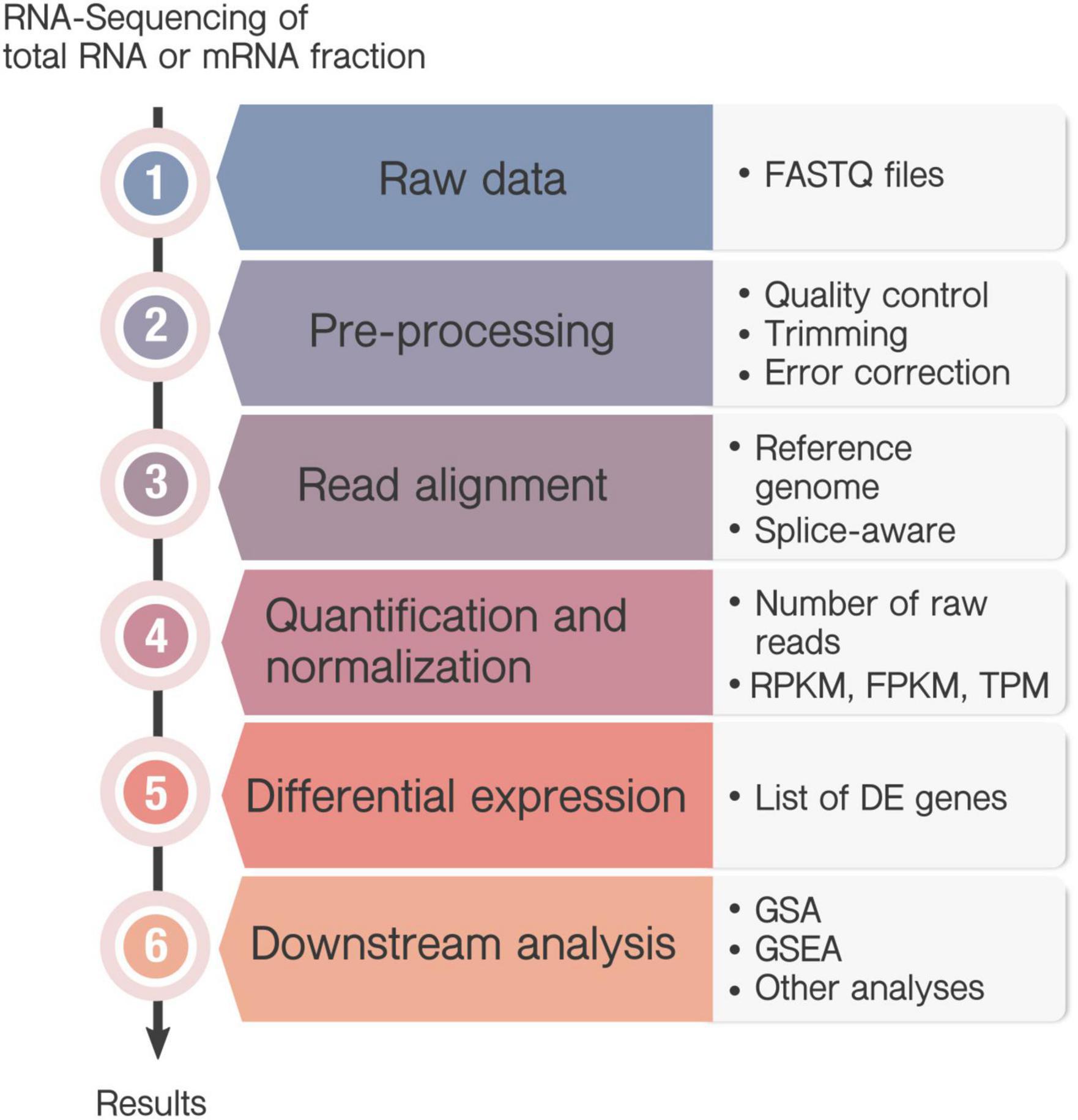
Figure 2. Workflow in RNA-Seq analysis. Transcriptome can be sequenced either from messenger RNA (mRNA) fraction, or total RNA, which includes also ribosomal RNA and transfer RNA. (1) RNA-sequencing generate a large amount of data from the millions of sequenced fragments (reads), and converts the information into a FASTQ file. (2) Pre-processing steps are commonly performed including quality check, trimming, filtering or error correction. (3) If an annotated genome is available, the sequenced reads are mapped onto the reference genome to identify each transcript and the correspondent gene. In this case, it is recommended to use splice-aware aligners, that align reads across splice junctions. However, if a reference genome is not available, then the reads will be assembled de novo by their overlapping regions to form contigs. (4) Next, quantification determines the number of raw reads that map to each transcript or gene and commonly normalized them to be compared between samples. The most commonly used normalizations are the “Reads Per Kilobase Million” (RPKM) or its alternative “Fragments Per Kilobase Million” (FPKM) and the “Transcripts per Kilobase” (TPM). (5) Then, differential expression (DE) analysis allows the identification of those genes whose expression change under particular circumstances indicates the gene expression profile associated to a certain condition through different statistical methods. (6) The result of a differential expression analysis is a list of DE genes that can sometimes contain hundreds or even thousands of genes. A downstream analysis is usually needed to interpret the results, as Gene Set Analysis (GSA) or Gene Set Enrichment Analysis (GSEA). Besides, there are many other options for the analysis of RNA-seq data, as the identification of Single Nucleotide Polymorphisms, or nucleotide insertions and deletions.
Transcriptomics Applied to Tuberculosis Research
It has been two decades since the first HTTr studies in TB were published (Ehrt et al., 2001; Ragno et al., 2001). HTTr have been used to explore different features, of both host and bacteria. Work is commonly not limited to Mtb but it includes the Mycobacterium tuberculosis complex (MTBC) species, the genetically related etiologic agents of tuberculosis in humans or other animals.
In this review, we will navigate through some of the most relevant HTTr studies performed in TB to date. We will not delve into the posterior evaluation and validation of the postulated biomarkers. Studies have been classified in sections according to their contributions, although they usually served to more than one purpose. A summary of applications and references can be found in Table 3.
Mechanisms of Tuberculosis Infection
TB HTTr studies can be addressed from two different perspectives: (i) one focused on the mechanisms used by the mycobacteria to infect the host and escape the immune system, or (ii) on the host’s immune defense itself.
Focusing on the mycobacteria mechanisms of infection, Mtb is able to adapt to different scenarios through the course of infection, changing its gene expression accordingly. The characterization of the bacteria response in different microenvironment will improve our understanding of TB pathogenesis and how it escapes from the immune response.
In vitro culture is one of the easiest way to study the mycobacteria. Microarray and RNA-Seq have been used to study its growth in broth culture (either exponential or stationary phase), or after being exposed to a variety of perturbations that mimic the infection process. Low pH (Fisher et al., 2002; Tan et al., 2013), low oxygen (Bacon et al., 2004; Rustad et al., 2008), oxidative stress (Ohno et al., 2003; Mehra and Kaushal, 2009; Voskuil et al., 2011) or other perturbations (Stewart et al., 2002; Tan et al., 2013; Lin et al., 2016; Saini et al., 2020) are commonly used to recreate the intra-macrophage phagosomal environment. These studies have shown that environmental stress triggers different survival mechanisms in Mtb. For instance, the two-component regulator phoPR in response to Cl- in synergy with pH (Tan et al., 2013); genes involved in the non-ribosomal-peptide synthesis and fatty acid metabolism in low-pH environment (Fisher et al., 2002); the biosynthesis of cell wall precursors in response to low oxygen (Bacon et al., 2004), sigma factors (Mehra and Kaushal, 2009) or gene expression regulation induced by reactive nitrogen species, via overlapping signal transduction pathways (Ohno et al., 2003).
Diversity of MTBC members are used: wild-type common research strains (Abdallah et al., 2015), mutant strains (Manganelli et al., 2002; Stewart et al., 2002; Saini et al., 2020), or clinical isolates to characterize them (Gao et al., 2005; Rose et al., 2013; Eldholm et al., 2014; Gomez-Gonzalez et al., 2019). A great part of the work done with mutants use genetically modified isogenic strains, constructed to assess the function of a certain gene. This input has been key to comprehend most bacterial virulence factors, including the acr-2 gene, one of the most prominent features of the Mtb heat-shock response (Stewart et al., 2002).
However, in vitro bacterial growth cannot completely simulate the host intracellular environment. Capturing the transcriptome of mycobacteria inside macrophages or dendritic cells is technically difficult because of the low abundance and quality of bacterial RNAs (Hinton et al., 2004) and hence there are limited data available. Pioneer work by Schnappinger et al. (2003) captured for the first time the intra-phagosomal Mtb transcriptome. They infected bone-marrow macrophages from wild-type and mutant mice and profiled the Mtb transcriptome in different conditions. Data revealed how Mtb upregulate genes in the phagosome required for fatty acid degradation, siderophore synthesis, DNA repair, cell envelope remodeling, lead to anaerobic respiration and increase iron uptake. Other studies followed their lead, including in vitro infection of human (Chaussabel et al., 2003; Cappelli et al., 2006; Fontán et al., 2008a,b; Zimmermann et al., 2017) or mouse macrophages (Rachman et al., 2006a; Rohde et al., 2007, 2012).
It is of special interest to define the bacterial expression changes occurring inside pulmonary tissue, the main site of infection, and especially from human TB samples. Nevertheless, in vivo infection has been mostly studied using animal models (Talaat et al., 2004; Rengarajan et al., 2005; Gautam et al., 2015; Coppola et al., 2016; Pisu et al., 2020, 2021) and have described how the bacteria changes its transcriptome within days of infection (Talaat et al., 2004; Coppola et al., 2016). Only a few articles have worked directly with samples from infected TB patients (Rachman et al., 2006b; Sharma et al., 2017). Another area explored by HTTr is the biofilm production by Mycobacterium species (Flores-Valdez et al., 2020).
Mycobacterial features drawn from these studies could also be used as targets for novel TB drugs and therapies. However, further research is required due to the plasticity of the response depending on the infection status, the microenvironment and the differences in methodology among publications.
Mechanisms of Mtb Latency
One of the most remarkable Mtb features is its ability to adapt to stress conditions generated by the host by entering into latency. This dormant phase is characterized by growth arrest, anaerobic respiration and antibiotic tolerance. Understanding the mechanisms behind the latent phase and how it is switched would help battling persistent TB. In physiological conditions, latent bacteria will be mostly contained into granulomas, multicellular structures comprised of macrophages, T cells, other cell types and biomolecules.
In vitro studies have tried to recreate the conditions believed to occur inside the granuloma. In order to do that, HTTr have been applied to MTBC cultures in hypoxia (Sherman et al., 2001; Bacon et al., 2004; Muttucumaru et al., 2004; Voskuil et al., 2004; McGillivray et al., 2015), starvation (Betts et al., 2002; Hampshire et al., 2004) or a combination of conditions (Beste et al., 2007; Deb et al., 2009; Aguilar-Ayala et al., 2017).
Isogenic mutant strains were tested in dormancy-like conditions to understand the role of diverse genes in latency (Park et al., 2003; McGillivray et al., 2015). Some key genes involved in transition to latency were discovered trough these HTTr studies, as the DosR regulon (Park et al., 2003). A meta-analysis of Mtb under latency-like conditions analyzed sets of genes regulated by transcription factors, and found differences during its adaptation (Balázsi et al., 2008). They agreed that dosR-controlled genes were the most responsive early during infection, while nadR, sigE, sigC, and furB regulons peaked later, after day 20. Other meta-analysis searched promising latency targets for drug discovery (Murphy and Brown, 2007).
Study of latent mycobacteria in vivo gets more problematic. The most widely used laboratory mouse strains do not generate caseous granulomas. As a consequence, specific mice strain (C3HeB/FeJ) or other animals as guinea pigs are commonly used, raising the experimental costs. Regarding human studies, obtaining granulomas from living patients requires delicate surgery, which is only recommended for patients with severe lung disease. To the best of our knowledge, transcriptome data from latent MTBC bacilli in vivo are not publicly available yet. Exploring TB latency remains technically challenging, but warrant further investigation.
Mechanisms of Host Immune Response
Along with the mycobacteria, the host response can swing the outcome of the disease. There are many unsolved questions about how some individuals develop the active disease while others go through latent infection or are able to clear the mycobacteria early.
Host transcriptional studies in human using easily collectible samples, like blood or saliva, had helped both exploring the immunopathogenesis of TB and identifying diagnostic markers. A pioneer work was conducted by Berry et al. (2010), demonstrating that blood transcriptome could reflect part of the events taking place at the site of infection. Such study provided a 393-gene signature associated with active TB able to discriminate between active and LTBI.
Following their steps, posterior studies have searched for specific features of active and/or latent TB disease (Berry et al., 2010; Maertzdorf et al., 2011a; Ottenhoff et al., 2012; Singhania et al., 2018) that have provided a greater insight into the host immune mechanisms that take place during the infection. Crucial TB biomarkers were derived from these works, including the first evidence of the importance of type I interferon in the pathogenesis of human tuberculosis (Berry et al., 2010); alteration of pathways related to regulation of immune responses; signal transduction and activation of leukocyte populations; intracellular protein trafficking; cell structure and motility changes; cell cycle control, phagocytosis, endocytosis and activation of the complement cascade. The diagnostic applications of these and other studies are further detailed in the TB diagnosis section.
Integration of results from independent studies can offer a new vision, such as the suggestion of a strong dominant role for myeloid cells in TB inflammation (Joosten et al., 2013). Additionally, application of single-cell transcriptomics enabled the identification of novel biomarkers and cell populations participating in TB previously undescribed, such as the depletion of the natural killer cell subset CD3–CD7+GMZMB+in TB (Cai et al., 2020).
Further efforts profiled the host response by isolating certain cell subtypes from blood and analyzing their specific transcriptional response (Jacobsen et al., 2010; Arlehamn et al., 2014). The bases of early clearance have also been investigated, being able to predict conversion to tuberculin skin test positive of household contacts, prior to any signs of infection (Weiner et al., 2020). Nonetheless, blood profiling may miss factors of biological relevance only present in the real environment of infection.
Transcriptomic studies using cells from the site of infection from real TB patients remain scarce, mainly owing to the difficulty of obtaining samples. Kim et al. (2010) successfully isolated caseous granulomas from TB patients, finding abundant transcripts involved in various destructive tissue pathologies and upregulated genes for lipid sequestration and metabolism. Importantly, the granuloma transcriptome did not entirely correlate with published TB blood signatures. Despite the difficulty, a proteomic human granuloma signature has also been accomplished (Marakalala et al., 2016). Future work deciphering the granuloma environment will have to deal with the enormous heterogeneity of granulomas within the host. Other approaches have captured the transcriptional response of cells after in vitro Mtb infection, using either human (Chaussabel et al., 2003; Volpe et al., 2006; Fontán et al., 2008a; Salamon et al., 2014; Zimmermann et al., 2017) or animal cells (Ehrt et al., 2001; Schnappinger et al., 2003; Shi et al., 2003; Roy et al., 2018).
In these settings, in vivo infection in animal models are crucial. They allow not only to harvest a higher amount of sample than in patients, but also grant control and decision over infection conditions. Transcriptome analysis has been commonly done in blood (Moreira-Teixeira et al., 2020a,b) or lungs (Seiler et al., 2003; Mehra et al., 2010, 2015; Huang et al., 2018; Ahmed et al., 2020; Moreira-Teixeira et al., 2020b; Pisu et al., 2020) from mice or macaques. Some of these works beautifully isolated alveolar (AM) and interstitial macrophages (IM) from lung, obtaining their unique signatures after infection (Huang et al., 2018; Pisu et al., 2020). Other projects made progress toward the correlation of the TB signature among human and animal models. Ahmed et al. (2020) identified common transcriptional immune correlates of TB across lung from animal models and human blood. Moreira-Teixeira et al. (2020b) identified a blood signature in TB-susceptible C3HeB/FeJ mice infected with Mtb HN878 that is compatible with the human signature, whereas it is minimally altered in blood from TB-resistant C57BL/6J mice infected with Mtb H37Rv.
Again, single-cell RNA-Seq technology also provided novel insights in the field. Pisu et al. (2021) described the functional heterogeneity in AM and IM populations in mouse infected lungs. An outstanding work by Khan et al. (2020) using bulk and single-cell RNA-seq, demonstrated that Mtb is able to reprogram hematopoietic stem cells in the bone marrow, limiting myelopoiesis and impairing trained macrophage immunity. HTTr can also be used to analyze changes in the microRNA fraction (Liu et al., 2011; Sharbati et al., 2011; Wang et al., 2011) during mycobacteria infections.
Lastly, the usage of dual transcriptomic analysis, analyzing in parallel both the host and the bacteria transcriptomes, is an interesting approach with growing presence (Fontán et al., 2008a; Rienksma et al., 2015; Zimmermann et al., 2017; Pisu et al., 2020, 2021). This method provides a complete vision of how both organisms interact within each other at a precise time. For instance, it was found a link between bacterial stress, induction of drug tolerance and the expression levels of CD11c in macrophages (Pisu et al., 2021). In other work, Mtb growing inside AMs (their favorite niche) were reported to upregulate genes of oxidative phosphorylation, mitochondrial function, iron storage, cell division and growth (Pisu et al., 2020). Contrarily, Mtb inside IMs expressed more genes of bacterial stress, concurring with the upregulation of the host pro-inflammatory response by those macrophages. Other studies linked the bacterial SigE regulon with the modulation of the inflammatory response (Fontán et al., 2008a); or decipher the pathogen’s diet inside the macrophages (Zimmermann et al., 2017) by combining dual sequencing and metabolomics.
Tuberculosis Diagnosis
TB diagnosis remain challenging. The current available tests present diverse shortcomings; i.e., microscopy has poor sensibility with low bacteria abundance; culture of Mtb requires weeks to grow; ex vivo Mtb gene amplification test Xpert MTB/RIF is expensive and fails to discriminate cleared infections; etc. Besides, it is common to mislead TB with other diseases due to its variable clinical signs. An accurate diagnosis would translate into a more effective use of drug resources and to the identification of individuals that will benefit from preventive treatment. The advances in transcriptomic studies hold the promise of finding new biomarkers that will serve to accurately diagnose the different stages of TB infection.
The first ever published HTTr work in human TB diagnostic was performed by Mistry et al. (2007). They compared whole-blood from a reduced number of patients with active tuberculosis, latently infected, cured TB or recurrent TB. Ensuing works followed their lead, while the technological progress allowed a better characterization of the disease, with higher number of target genes and larger number of samples.
Although slight differences can be found in several studies, the majority characterizes the TB response comparing between active TB patients (TBI) with uninfected individuals (NoTBI) and latent healthy patients (LTBI) (Jacobsen et al., 2007; Berry et al., 2010; Maertzdorf et al., 2011a,b; Anderson et al., 2014; Cai et al., 2014; Singhania et al., 2018; Estévez et al., 2020). In most published studies, the differential expression of a group of genes is usually sufficient to classify these groups. Some highlighted genes or pathways include an interferon-inducible neutrophil-driven gene signature (Berry et al., 2010), lactoferrin, CD64, Ras-associated GTPase 33A (Jacobsen et al., 2007), changes in FCGR1B signaling (Maertzdorf et al., 2011b), or complement pathways (Cai et al., 2014). MicroRNAs (Fu et al., 2011; Zhang et al., 2013) have also been screened for TB diagnostic purposes.
The scientific community has made efforts in discriminating the TB gene signature from extrapulmonary TB (Roe et al., 2016) and other diseases (Berry et al., 2010; Maertzdorf et al., 2012; Bloom et al., 2013), specially other pulmonary diseases or co-infecting diseases that are highly prevalent in TB endemic areas. Multicenter studies (Kaforou et al., 2013; Anderson et al., 2014; Hoang et al., 2021) included a superior number of patients suffering from TB and other diseases, including HIV-coinfection.
Despite efforts, human TB biomarkers have shown little overlap between studies. An endless variety of factors may contribute to the signature diversity: differences in cohorts, variations in study design, diagnostic method for confirming TB, misclassified or undetected pathologies, missing data, BCG vaccination, transcriptomics platforms or analysis pipeline. Metadata analysis have exploited available data sets in an attempt to conceal existing studies (Sweeney et al., 2016; Warsinske et al., 2019; Gupta et al., 2020). A three-gene set (GBP5, DUSP3, and KLF2) defined by Sweeney et al. (2016), ascertain later in an independent study (Warsinske et al., 2019), was robust enough to diagnose active tuberculosis among their data.
Nonetheless, there is still a need for TB diagnostic biomarkers to be used in a heterogeneous population. Most successful studies were based on cohorts of hundreds of cases, with standardized sample processing methods and correct identification of the patients. The use of a validation cohort from another ethnicity and TB endemicity seems to be key to test the robustness of the transcriptomic signature. It is also highly desirable to compare to diseases closely related to TB in order to ensure the specificity of the infection. Moreover, the usage of samples different from blood, such as sputum or bronchoalveolar lavage, could reflect better what is happening at the site of disease, as they might expose useful biomarkers that are not so evident in blood. However, the difficulty in obtaining those samples and its limited reproducibility are major drawbacks.
Biomarkers of Treatment Evolution
HTTr have also been applied to screen blood biomarkers that correlate with the response to anti-TB treatment. Those biomarkers would permit a rapid assessment of the treatment’s success or failure and detect patients that require a change in the treatment regimen. Implementation of the treatment monitoring in clinical practice will have a beneficial impact on patient wellness and health care costs.
Changes in the blood transcriptome of TB patients have been detected during different stages of the treatment or after treatment completion (Berry et al., 2010; Bloom et al., 2012; Ottenhoff et al., 2012; Cliff et al., 2013; Tabone et al., 2021). Generally, authors found a down-regulation of immune or inflammation-related genes coinciding with the disease resolution. A set of differentially expressed genes previously identified as TB progression biomarkers (Zak et al., 2016) also proved to be successful in treatment monitoring (Penn-Nicholson et al., 2020). Furthermore, an appealing study highlighted a signature of treatment failure before treatment initiation (Thompson et al., 2017), while other work presented a signature for detecting relapse after successful treatment (Cliff et al., 2016).
Progression to Tuberculosis Infection
Although there are many evidences that suggest a continuous spectrum of TB infection (Drain et al., 2018), its diagnosis is currently divided into two different stages: latent or active TB. A major drawback of the fight against TB is the inability to differentiate between latently infected patients that are at a higher risk of transitioning to an active disease.
Many efforts have been made into understanding and predicting progression to active TBI, both amongst latently infected population (Maertzdorf et al., 2011b; Zak et al., 2016; Scriba et al., 2017; Burel et al., 2021), contacts of TB patients (Suliman et al., 2018; Estévez et al., 2020; Roe et al., 2020; Nathan et al., 2021), or combined cohorts in a meta-analysis of published data (Warsinske et al., 2019; Gupta et al., 2020; Moreira-Teixeira et al., 2020b). These studies differentiate TBI progressors and non-progressors. Some results suggest that people at risk of progression show an inflammatory profile that include Type I/II interferon and complement genes in early stages before progression to active TB, and a higher expression of lymphoid, monocyte and neutrophil genes proximal to the disease onset (Scriba et al., 2017).
One of the most cited works identified and validated a 16 gene-signature that differentiate people at risk of developing the active disease (Zak et al., 2016). Warsinske et al. (2019) compared 16 published gene signatures and assessed that the three-gene set selected by Sweeney et al. (2016) for TB diagnosis also served to accurately identify individuals at high risk of progression. Comparison of TB risk across species showed that the mentioned 16-gene signature is enriched in progressors across animal models, and specified the genes that mediate protective (as Stat1 and Tap1) or detrimental (Batf2, Fcgr1, and Scarf1) responses in mouse model (Ahmed et al., 2020). The study of Esaulova et al. (2021) used single-cell RNA-seq and CyTOF technology to study TB in macaques. They reported an augment of CD27+ NK cells in lungs during latency Mtb-control, whereas progression was characterized by plasmacytoid dendritic cells and T cells producing IFN responses. Our group applied a machine-learning prediction model to identify a subset of LTBI contacts whose transcriptional profile are suggestive of having a higher probability of developing active TB (Estévez et al., 2020). Tabone et al. (2021) have recently published a unique study of blood signatures across the TB spectrum, including incipient, subclinical, and active TB. Changes in blood gene expression were minimal in incipient TB, increased in patients with subclinical TB and became maximal when clinical TB was diagnosed. They also assessed the effect of anti-TB treatment, obtaining differential responses among the defined TB subgroups. Other related studies included the characterization of patients at risk for recurrent tuberculosis (Mistry et al., 2007).
Predicting potential progression to active TB using biomarkers could be usefully translated into clinical care, by treating latent infected cases prior to develop the disease or augmenting medical monitoring.
Drug Resistance and Search for Novel Drugs
The emergence of MDR and XDR Mtb strains severely compromises TB treatment, and urges the discovery of new therapeutic anti-mycobacterial compounds. We previously assessed how comprehension of the bacterial transcriptome helped in identifying potential drug candidates against both active and dormant tuberculosis. Comparative analysis of Mtb transcriptome treated with different drugs has also been used to elucidate the mode of action of uncharacterized anti-mycobacterial compounds, to identify new mycobacterial targets, or to predict effective drug combinations. Besides, gene expression comparison of resistant vs. sensitive strains can be used to discern mechanisms of antibiotic resistance.
The transcriptome response of sensitive mycobacteria strains to diverse drugs has been explored, including isoniazid (Wilson et al., 1999; Betts et al., 2003; Boshoff et al., 2004; Waddell et al., 2004), rifampicin (Boshoff et al., 2004), ciprofloxacin (Sullivan et al., 2008), capreomycin (Fu and Shinnick, 2007), or vancomycin (Provvedi et al., 2009). It has also been applied to unravel the mechanisms of novel compounds that demonstrated good antimycobacterial activity in vitro (Manjunatha et al., 2009; Foo et al., 2018).
Some mechanisms of Mycobacterium antibiotic resistance are already known, while others remain undiscovered. To inspect further into those tolerance mechanisms, the transcriptome response of mutant-resistant and sensitive-wild type Mtb strains were compared in diverse studies. They included single-drug (Knegt et al., 2013; Zheng et al., 2018), or multiple drug resistance (Keren et al., 2011; Chatterjee et al., 2013; Peñuelas-Urquides et al., 2013; Eldholm et al., 2014). Some of the main findings of these studies included a possible role of genes Rv0559c and Rv0560c (Knegt et al., 2013) in rifampicine resistance; they also found a set of genes upregulated in Mtb persisters, including UspA, SigF, or dnaE2 (Keren et al., 2011); described the downregulation of diverse genes in multidrug resistant isolates (Chatterjee et al., 2013), and the differential expression drug efflux and mycolic acid synthesis pathways (Eldholm et al., 2014). One work assessed the transcriptional changes triggered by SMARt-420, a molecule that reverses ethionamide-acquired resistance in Mtb (Blondiaux et al., 2017).
The majority of published studies were performed under in vitro laboratory growth condition. Few works have explored the transcriptional response of Mtb from clinical samples or in vivo models during treatment (Karakousis et al., 2008; Shaikh et al., 2021). Furthermore, latent bacteria are largely tolerant to antibiotics, mostly because common antibiotics target proteins expressed in replicating bacilli. It has been described an altered transcriptional response to antibiotics in the dormant stage (Karakousis et al., 2008; Keren et al., 2011). In this setting, Mtb genes expressed during latency are obvious candidates for drug targeting. Various studies addressed this issue (Defelipe et al., 2016; Aguilar-Ayala et al., 2017; Banerjee et al., 2020) and identified potential candidates, as phosphate synthase I3PS or specific proteins Rv1994c, Rv2780, and Rv3515c. However, when drawing conclusions, we must bear in mind that most data are collected during Mtb transition into dormancy, rather than during the rigorous latency stage.
In sum, discoveries in the field have helped to predict the mechanism of action of previously uncharacterized compounds, identifying potential drugs, novel drug targets, or understanding resistance. One of the difficulties of the analysis remains in differentiating the expression changes directly corresponding to the drug target, from those derived, including compensatory effects.
Immune Response to Novel Vaccines and Correlates of Protection
The vaccine containing the Bacille Calmette-Gueìrin (BCG) is currently the only approved TB vaccine, but it shows a highly variable efficacy against pulmonary TB in adults. It consists in a live-attenuated form of Mycobacterium bovis. As the classical dogma of TB protection, attributed to interferon-γ and CD4 T cells, has partially failed (Kagina et al., 2010), new reliable biomarkers of protection are searched to direct the development of novel vaccines or therapies for TB.
Gene expression profiling of blood from BCG-vaccinated donors revealed interesting findings, as a decrease in the expression of cell adhesion molecules (Fletcher et al., 2009) or the upregulation of several immune-related genes (Fletcher et al., 2016). Currently, it is highly accepted that BCG triggers epigenetic changes in the immune cells, inducing trained innate memory (Kaufmann et al., 2018). A couple of publications have addressed the changes in both the transcriptome and the epigenome induced by the vaccine in humans (Arts et al., 2018; Cirovic et al., 2020).
Pulmonary transcriptome after BCG vaccination has been analyzed in various animal models, mostly in mice and macaques (Mollenkopf et al., 2006; Aranday Cortes et al., 2010; Mehra et al., 2013; Kunnath-Velayudhan et al., 2017). A singular study analyzing the granuloma lesions in BCG-vaccinated macaques found the upregulation of various cytokines and chemokines in the vaccinated individuals (Mehra et al., 2013).
Importantly, distinct BCG strains may generate different transcriptomic profiles in the host, which seems in line with the high heterogeneity of the BCG vaccine efficacy. Numerous publications focused on comparing the bacterial transcriptomes of the diverse BCG variants in order to understand their differences (Behr et al., 1999; Brosch et al., 2007; Abdallah et al., 2015).
Novel vaccine candidates are continuously being developed to substitute or boost BCG, and commonly the protective results are compared to BCG itself. A common approach has been developing mutants of BCG, which also helps in the understanding of the mechanisms of BCG functioning. Both the BCG bacterial transcriptome (Flores-Valdez et al., 2018) or the host transcriptional response (Gengenbacher et al., 2016; Hoft et al., 2016) are studied to characterize those new BCG-based vaccines. Other novel vaccine strategies included peptide/protein-based (Santoro et al., 2018; Martínez-Pérez et al., 2021), viral vectors (Hansen et al., 2018) or DNA vaccines (Zárate-Bladés et al., 2009). The transcriptome analysis usually find a reduced TB disease-associated signature, upregulation of antigen presenting pathways, and suggest potential biomarkers of protection.
The human transcriptomic profile to novel TB vaccines is scarce. Valuable lessons may be drawn from the most advanced candidates: M72/AS01E (van den Berg et al., 2018) and VPM1002 (Loxton et al., 2017).
Discoveries of protective mechanisms can also be drawn from therapeutic or early protection studies, discussed in previous sections. Host-directed therapies is a new and emerging concept in the treatment of TB, where the host response can be boosted using diverse biological compounds, with or without adjunct antibiotics.
Although HTTr studies can prioritize some candidate biomarkers, further testing must be done to validate their true effectiveness. Powerful correlates of TB protection will accelerate the progress of new TB vaccine development and therapies.
Discussion
To Shape the Future
The future of transcriptomics seems bright. Comparing with what has happened with genome sequencing, we will expect that new equipment, cheaper reagents, specialized groups and better software, will come in the next years. Hopefully, those advances will continue addressing the complexity of TB disease.
RNA-Seq and microarrays are yet prohibitive for routine clinical use or even some research projects, because of their costs and expertise required. Commonly, RT-qPCR is used instead at large scale studies to measure a limited signature of candidate genes. This procedure is faster, cheaper, easier to use, and requires minimal electric power. Nonetheless, it is expected that technical progress will end up reducing prize and complexity. Currently, the five platforms most commonly used for RNA-seq include Illumina, SOLiD, Ion Torrent, PacBio and 454 Life sciences, with differences in read length and accuracy. It is likely that, in the near future, more companies will appear in the market, with new reagents and kits available.
The standardization of the process, including preparation of samples and analysis pipelines, would allow a better comparison between laboratories. The goal is to obtain a robust workflow requiring minimal resources to ensure feasibility and data reproducibility amongst the highly variable clinical infrastructures worldwide.
It is also crucial to count on reliable databases. Metadata must be meticulously filled when uploading our results to a public database. They must include a detailed experimental procedure, cellular composition, RNA extraction details and sequencing methods. Better integration among databases will also contribute to easily find gene sets of interest.
There is a growing need of specialists in bioinformatic infrastructures, as Supercomputing Centers, to process and store data. A large combination of bioinformatics software tools and resources is required to process the large volume of raw sequences and the multiple steps in data analysis.
Education in Biocomputing at different levels (university, master, doctorate), will increase the number of persons with expertise in this field. Furthermore, translating big data into simple and useful biological conclusions is usually challenging. The expansion of knowledge in the biological context will help finding interactions among differentially expressed (DE) genes or pathways of biological significance.
One of the most promising approaches to boost the HTTr data utilities is the application of machine learning technology. Machine learning methods benefit from large datasets in order to identify complex relationships between gene candidates. It allows the integration of different datasets into one single analysis, helping to overcome the limitations of typically small sample sizes. Using this approach, one can benefit from all data available in public repositories to learn from large, heterogeneous datasets and build class-prediction models that help on the classification of any sample, regardless of its origin. This is particularly important in TB, where it is of special interest to find a gene combination that could be used for diagnosis in multiple TB-burden settings.
As it has happened with DNA sequencing, emerging technologies will update the current transcriptomic platforms. New technologies that could be more reliable, faster, requiring less amount of sample, and able to detect low abundant transcripts, are expected to come in the next years. As an example, a new generation of sensing technology that uses nanopore, developed by Oxford Nanopore, is suitable for sequencing RNA without conversion to cDNA. Furthermore, gene expression technology can be combined with other platforms, as is the case of spatial transcriptomics. It uses spatially barcoded mRNA-binding oligonucleotides, to obtain a 2D picture of gene expression within a histological section of tissue. Nevertheless, this technology does not allow yet the study of the complete transcriptome.
Integration of information coming from different sources (clinical, immunological) and other omics data (genomic, transcriptomic, epigenomics, proteomic, metabolomics, glycomics, glycoproteomics) will provide an integrative analysis of the factors involved in disease, embryology, evolution or other processes. This field of study, termed systems biology, may improve with the help of new sophisticated computational tools. In recent years, researchers tried to integrate transcriptomic and proteomic data in TB, finding usually a poor correlation. It is expected for systems biology to explain the complex regulatory mechanism that control RNA and protein levels.
As we have seen along this review, HTTr has proven to be an important tool to reveal interesting biological pathways in TB. Nonetheless, forthcoming studies must take into consideration some critical points:
• Many external factors can affect the transcriptional results. They include the nature of the sample collection (bronchoalveolar lavage, lung parenchyma, saliva, blood), sample processing (whole sample, cell subset isolation, cell subset-depletion) or sequencing platform. Mtb strains, ethnically diverse cohorts and misclassification of patient, will also affect in the transcriptomic signature. Findings in a cohort might not be effectively translated into global clinical use. Researchers must be aware of the variables when interpreting results and designing experiments (Figure 3).
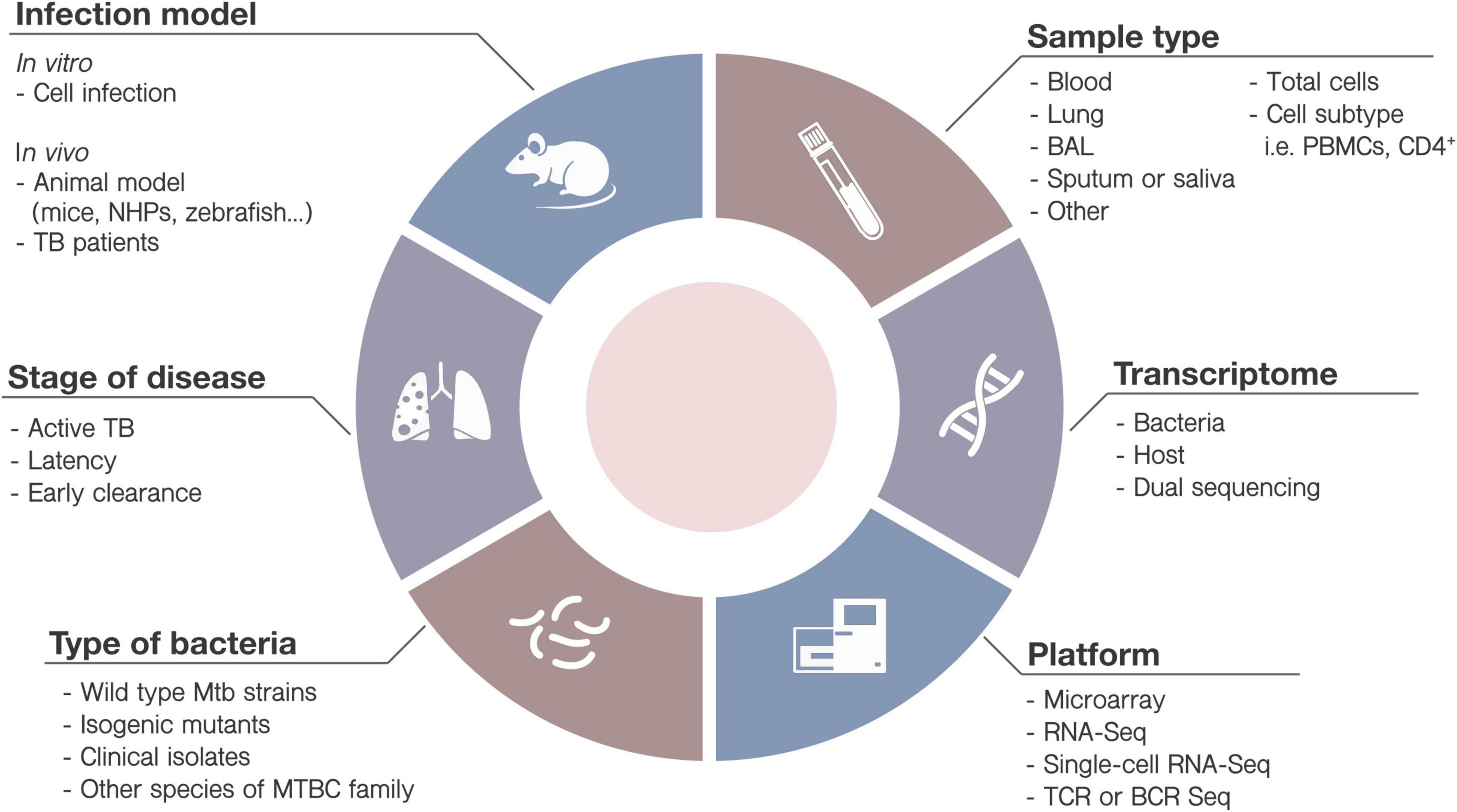
Figure 3. Variables when designing high-throughput transcriptomic studies. Diverse parameters must be taken into account when designing new TB studies involving HTTr. Some of them include: (1) the infection model: in vitro (cell infection) or in vivo (animals or TB patients) (2) type of bacteria (i.e., strain, mutated or wild-type) and culture conditions; (3) samples to be collected (saliva, sputum, blood, etc.), including analysis of immune cells or subpopulations, at basal level or after stimulation; (4) the stage of the disease (i.e., active disease, latency, early clearance); (5) target element: bacteria, host or both, and (6) the HTTr platform most adequate for the study.
• There must be a partial reliability of the blood transcriptome, as it may not truly represent the processes occurring within the lungs. On the other hand, animal models may not be the best way to study the human TB disease. Some models recreate better the pathology of human TB (guinea pig, macaques) than others (mice). Differences regarding number of exposures, bacterial concentration, challenge route, kinetics, type of lesions produced or illness severity, should also be taken into consideration.
• Further biological studies and clinical validation must be pursued to elucidate the role of the product of DE candidate genes. Moreover, gene expression methods cannot predict the final protein existence, nor their post-translational modifications or evaluate the presence other molecules like polysaccharides, lipopolysaccharides and glycolipids. Complementary studies are required in order to confirm the role of candidate molecules.
• Lastly, special adaptations of the transcriptomic technology are opening new horizons: Single-cell RNA sequencing is a promising technique to explore cell heterogeneity within a population and reveal novel signatures and cell subsets. T cell receptor (TCR) and B cell receptor (BCR) sequencing can use bulk or single-cell methods to characterize complex T and B cell responses, respectively. These techniques allow the identification of all potential clonotypes in a diverse repertoire of TCRs and BCRs, and it is a powerful tool for studying the distribution of lymphocyte repertoires.
Conclusion
Once accepted the extraordinary plasticity of mechanisms involved in Mycobacterium tuberculosis-host interactions, a lot of work remains. HTTr have been immensely valuable in advancing on TB research. It can be applied to a variety of samples and experimental conditions depending on the question asked. Unfortunately, differences in experimental procedures, sequencing platforms, or incomplete metadata usually hamper further exploitation of public-available data.
Currently, RNA-Seq offers key advantages over the rest of existing technologies of HTTr, although it might be replaced in the near future. There is a growing need of bioinformatics specialists and infrastructures that must be met to fully exploit the generated HT data. Lastly, we encourage the creation of interdisciplinary projects that integrates HTTr with the rest of omic sciences.
Search Strategy and Selection Criteria
References were searched through PubMed by use of the terms “Tuberculosis,” “Transcriptomics,” “Microarray,” and “RNA-Sequencing,” for articles published from 1980 to November 2021. Then, articles were ascertain to use high-throughput transcriptomics methods. A selection of most relevant publications was performed based on number of citations, quality and impact of publication journal or relevance in the field.
Author Contributions
AM-P and OE performed literature search, figures, and writing of the manuscript. ÁG-F made the critical revision of the manuscript and contributed with funding acquisition. All authors contributed to the article and approved the submitted version.
Funding
We gratefully acknowledge funding by project EU Horizon 2020 “Eliciting Mucosal Immunity in Tuberculosis” (EMI-TB) project (Grant No. 643558) and Xunta de Galicia (Grupo de Referencia Competitiva) (ED431C 2016/041). AM-P acknowledges a fellowship from the Xunta de Galicia Programa de Axudas á etapa predoutoral (Consellería de Cultura, Educación e Ordenación Universitaria) (ED481A-2018/230).
Conflict of Interest
The authors declare that the research was conducted in the absence of any commercial or financial relationships that could be construed as a potential conflict of interest.
Publisher’s Note
All claims expressed in this article are solely those of the authors and do not necessarily represent those of their affiliated organizations, or those of the publisher, the editors and the reviewers. Any product that may be evaluated in this article, or claim that may be made by its manufacturer, is not guaranteed or endorsed by the publisher.
References
Abdallah, A. M., Hill-Cawthorne, G. A., Otto, T. D., Coll, F., Guerra-Assunção, J. A., Gao, G., et al. (2015). Genomic expression catalogue of a global collection of BCG vaccine strains show evidence for highly diverged metabolic and cell-wall adaptations. Sci. Rep. 5:15443. doi: 10.1038/srep15443
Adil, A., Kumar, V., Jan, A. T., and Asger, M. (2021). Single-Cell transcriptomics: current methods and challenges in data acquisition and analysis. Front. Neurosci. 15:591122. doi: 10.3389/fnins.2021.591122
Aguilar-Ayala, D. A., Tilleman, L., Van Nieuwerburgh, F., Deforce, D., Palomino, J. C., Vandamme, P., et al. (2017). The transcriptome of Mycobacterium tuberculosis in a lipid-rich dormancy model through RNAseq analysis. Sci. Rep. 7:17665. doi: 10.1038/s41598-017-17751-x
Ahmed, M., Thirunavukkarasu, S., Rosa, B. A., Thomas, K. A., Das, S., Rangel-Moreno, J., et al. (2020). Immune correlates of tuberculosis disease and risk translate across species. Sci. Transl. Med. 12:eaay0233. doi: 10.1126/scitranslmed.aay0233
Anderson, S. T., Kaforou, M., Brent, A. J., Wright, V. J., Banwell, C. M., Chagaluka, G., et al. (2014). Diagnosis of childhood tuberculosis and host RNA Expression in Africa. N Engl. J. Med. 370, 1712–1723. doi: 10.1056/NEJMoa1303657
Aranday Cortes, E., Kaveh, D., Nunez-Garcia, J., Hogarth, P. J., and Vordermeier, M. H. (2010). Mycobacterium bovis-BCG vaccination induces specific pulmonary transcriptome biosignatures in mice. PLoS One 5:e11319. doi: 10.1371/journal.pone.0011319
Arlehamn, C. L., Seumois, G., Gerasimova, A., Huang, C., Fu, Z., Yue, X., et al. (2014). Transcriptional profile of tuberculosis antigen–specific T Cells reveals novel multifunctional features. J. Immunol. 193, 2931–2940. doi: 10.4049/jimmunol.1401151
Arts, R. J. W., Moorlag, S. J. C. F. M., Novakovic, B., Li, Y., Wang, S. Y., Oosting, M., et al. (2018). BCG Vaccination protects against experimental viral infection in humans through the induction of cytokines associated with trained immunity. Cell Host Microbe. 23, 89–100. doi: 10.1016/j.chom.2017.12.010
Bacon, J., James, B. W., Wernisch, L., Williams, A., Morley, K. A., Hatch, G. J., et al. (2004). The influence of reduced oxygen availability on pathogenicity and gene expression in Mycobacterium tuberculosis. Tuberculosis 84, 205–217. doi: 10.1016/j.tube.2003.12.011
Bainbridge, M. N., Warren, R. L., Hirst, M., Romanuik, T., Zeng, T., Go, A., et al. (2006). Analysis of the prostate cancer cell line LNCaP transcriptome using a sequencing-by-synthesis approach. BMC Genomics 7:246. doi: 10.1186/1471-2164-7-246
Balázsi, G., Heath, A. P., Shi, L., and Gennaro, M. L. (2008). The temporal response of the Mycobacterium tuberculosis gene regulatory network during growth arrest. Mol. Syst. Biol. 4:225. doi: 10.1038/msb.2008.63
Banerjee, U., Sankar, S., Singh, A., and Chandra, N. A. (2020). Multi-Pronged computational pipeline for prioritizing drug target strategies for latent tuberculosis. Front. Chem. 8:593497. doi: 10.3389/fchem.2020.593497
Behr, M. A., Wilson, M. A., Gill, W. P., Salamon, H., Schoolnik, G. K., Rane, S., et al. (1999). Comparative genomics of BCG vaccines by whole-genome DNA microarray. Science 284, 1520–1523. doi: 10.1126/science.284.5419.1520
Berry, M. P. R., Graham, C. M., McNab, F. W., Xu, Z., Bloch, S. A. A., Oni, T., et al. (2010). An interferon-inducible neutrophil-driven blood transcriptional signature in human tuberculosis. Nature 466, 973–977. doi: 10.1038/nature09247
Beste, D. J. V., Laing, E., Bonde, B., Avignone-Rossa, C., Bushell, M. E., and McFadden, J. J. (2007). Transcriptomic analysis identifies growth rate modulation as a component of the adaptation of mycobacteria to survival inside the macrophage. J. Bacteriol. 189, 3969–3976. doi: 10.1128/JB.01787-06
Betts, J. C., Lukey, P. T., Robb, L. C., McAdam, R. A., and Duncan, K. (2002). Evaluation of a nutrient starvation model of Mycobacterium tuberculosis persistence by gene and protein expression profiling. Mol. Microbiol. 43, 717–731. doi: 10.1046/j.1365-2958.2002.02779.x
Betts, J. C., Mclaren, A., Lennon, M. G., Kelly, F. M., Lukey, P. T., Blakemore, S. J., et al. (2003). Signature gene expression profiles discriminate between isoniazid-, thiolactomycin-, and triclosan-treated mycobacterium tuberculosis. Antimicrob. Agents Chemother. 47, 2903–2913. doi: 10.1128/AAC.47.9.2903-2913.2003
Blondiaux, N., Moune, M., Desroses, M., Frita, R., Flipo, M., Mathys, V., et al. (2017). Reversion of antibiotic resistance in Mycobacterium tuberculosis by spiroisoxazoline SMARt-420. Science 355, 1206–1211. doi: 10.1126/science.aag1006
Bloom, C. I., Graham, C. M., Berry, M. P. R., Rozakeas, F., Redford, P. S., Wang, Y., et al. (2013). Transcriptional blood signatures distinguish pulmonary tuberculosis, pulmonary sarcoidosis, pneumonias and lung cancers. PLoS One 8:e70630. doi: 10.1371/journal.pone.0070630
Bloom, C. I., Graham, C. M., Berry, M. P. R., Wilkinson, K. A., Oni, T., Rozakeas, F., et al. (2012). Detectable changes in the blood transcriptome are present after two weeks of antituberculosis therapy. PLoS One 7:e46191. doi: 10.1371/journal.pone.0046191
Boshoff, H. I. M., Myers, T. G., Copp, B. R., Mcneil, M. R., Wilson, M. A., and Iii, C. E. B. (2004). The transcriptional responses of mycobacterium tuberculosis to inhibitors of metabolism. J. Biol. Chem. 279, 40174–40184. doi: 10.1074/jbc.M406796200
Brenner, S., Johnson, M., Bridgham, J., Golda, G., Lloyd, D. H., Johnson, D., et al. (2000). Gene expression analysis by massively parallel signature sequencing (MPSS) on microbead arrays. Nat. Biotechnol. 18, 630–634. doi: 10.1038/76469
Brosch, R., Gordon, S. V., Garnier, T., Eiglmeier, K., Frigui, W., Valenti, P., et al. (2007). Genome plasticity of BCG and impact on vaccine efficacy. Proc. Natl. Acad. Sci. USA 104, 5596–5601. doi: 10.1073/pnas.0700869104
Burel, J. G., Singhania, A., Dubelko, P., Muller, J., Tanner, R., Parizotto, E., et al. (2021). Distinct blood transcriptomic signature of treatment in latent tuberculosis infected individuals at risk of developing active disease. Tuberculosis 131:102127. doi: 10.1016/j.tube.2021.102127
Cai, Y., Dai, Y., Wang, Y., Yang, Q., Guo, J., Wei, C., et al. (2020). Single-cell transcriptomics of blood reveals a natural killer cell subset depletion in tuberculosis. EBioMedicine 53:102686. doi: 10.1016/j.ebiom.2020.102686
Cai, Y., Yang, Q., Tang, Y., Zhang, M., Liu, H., Zhang, G., et al. (2014). Increased complement C1q level marks active disease in human tuberculosis. PLoS One 9:e92340. doi: 10.1371/journal.pone.0092340
Cappelli, G., Volpe, E., Grassi, M., Liseo, B., Colizzi, V., and Mariani, F. (2006). Profiling of Mycobacterium tuberculosis gene expression during human macrophage infection: Upregulation of the alternative sigma factor G, a group of transcriptional regulators, and proteins with unknown function. Res. Microbiol. 157, 445–455. doi: 10.1016/j.resmic.2005.10.007
Chatterjee, A., Saranath, D., Bhatter, P., and Mistry, N. (2013). Global Transcriptional profiling of longitudinal clinical isolates of mycobacterium tuberculosis exhibiting rapid accumulation of drug resistance. PLoS One 8:e54717. doi: 10.1371/journal.pone.0054717
Chaussabel, D., Semnani, R. T., McDowell, M. A., Sacks, D., Sher, A., and Nutman, T. B. (2003). Unique gene expression profiles of human macrophages and dendritic cells to phylogenetically distinct parasites. Blood 102, 672–681. doi: 10.1182/blood-2002-10-3232
Cirovic, B., de Bree, L. C. J., Groh, L., Blok, B. A., Chan, J., van der Velden, W. J. F. M., et al. (2020). BCG vaccination in humans elicits trained immunity via the hematopoietic progenitor compartment. Cell Host Microbe. 28, 322–334. doi: 10.1016/j.chom.2020.05.014
Cliff, J. M., Cho, J. E., Lee, J. S., Ronacher, K., King, E. C., Van Helden, P., et al. (2016). Excessive cytolytic responses predict tuberculosis relapse after apparently successful treatment. J. Infect. Dis. 213, 485–495. doi: 10.1093/infdis/jiv447
Cliff, J. M., Lee, J. S., Constantinou, N., Cho, J. E., Clark, T. G., Ronacher, K., et al. (2013). Distinct phases of blood gene expression pattern through tuberculosis treatment reflect modulation of the humoral immune response. J. Infect. Dis. 207, 18–29. doi: 10.1093/infdis/jis499
Coppola, M., Van Meijgaarden, K. E., Franken, K. L. M. C., Commandeur, S., Dolganov, G., Kramnik, I., et al. (2016). New genome-wide algorithm identifies novel in-vivo expressed mycobacterium tuberculosis antigens inducing human t-cell responses with classical and unconventional cytokine profiles. Sci. Rep. 6:37793. doi: 10.1038/srep37793
Deb, C., Lee, C. M., Dubey, V. S., Daniel, J., Abomoelak, B., Sirakova, T. D., et al. (2009). A novel in vitro multiple-stress dormancy model for mycobacterium tuberculosis generates a lipid-loaded, drug-tolerant, dormant pathogen. PLoS One 4:e6077. doi: 10.1371/journal.pone.0006077
Defelipe, L. A., Do Porto, D. F., Pereira Ramos, P. I., Nicolás, M. F., Sosa, E., Radusky, L., et al. (2016). A whole genome bioinformatic approach to determine potential latent phase specific targets in Mycobacterium tuberculosis. Tuberculosis 97, 181–192.
Drain, P. K., Bajema, K. L., Dowdy, D., Dheda, K., Naidoo, K., and Schumacher, S. G. (2018). Incipient and subclinical tuberculosis?: a clinical review of early stages and progression of infection the spectrum of tuberculosis infection to disease. Clin. Microbiol. Rev. 31, e18–e21. doi: 10.1128/CMR.00021-18
Ehrt, S., Schnappinger, D., Bekiranov, S., Drenkow, J., Shi, S., Gingeras, T. R., et al. (2001). Reprogramming of the Macrophage Transcriptome in Response to Interferon-γ and Mycobacterium tuberculosis. J. Exp. Med. 194, 1123–1140. doi: 10.1084/jem.194.8.1123
Eldering, E., Spek, C. A., Aberson, H. L., Grummels, A., Derks, I. A., de Vos, A. F., et al. (2003). Expression profiling via novel multiplex assay allows rapid assessment of gene regulation in defined signalling pathways. Nucleic Acids Res. 31:e153. doi: 10.1093/nar/gng153
Eldholm, V., Norheim, G., vonderLippe, B., Kinander, W., Dahle, U. R., Caugant, D. A., et al. (2014). Evolution of extensively drug-resistant Mycobacterium tuberculosis from a susceptible ancestor in a single patient. Genome Biol. 15:490. doi: 10.1186/s13059-014-0490-3
Esaulova, E., Das, S., Singh, D. K., Choreño-Parra, J. A., Swain, A., Arthur, L., et al. (2021). The immune landscape in tuberculosis reveals populations linked to disease and latency. Cell Host Microbe. 29, 165–178. doi: 10.1016/j.chom.2020.11.013
Estévez, O., Anibarro, L., Garet, E., Pallares, A., Barcia, L., and Calvino, L. (2020). An RNA-seq based machine learning approach identifies latent tuberculosis patients with an active tuberculosis Profile. Front. Immunol. 11:1470. doi: 10.3389/fimmu.2020.01470
Fisher, M. A., Plikaytis, B. B., and Shinnick, T. M. (2002). Microarray analysis of the Mycobacterium tuberculosis transcriptional response to the acidic conditions found in phagosomes. J. Bacteriol. 184, 4025–4032. doi: 10.1128/JB.184.14.4025-4032.2002
Fletcher, H. A., Filali-Mouhim, A., Nemes, E., Hawkridge, A., Keyser, A., Njikan, S., et al. (2016). Human newborn bacille Calmette-Guérin vaccination and risk of tuberculosis disease: A case-control study. BMC Med. 14:76. doi: 10.1186/s12916-016-0617-3
Fletcher, H. A., Keyser, A., Bowmaker, M., Sayles, P. C., Kaplan, G., Hussey, G., et al. (2009). Transcriptional profiling of mycobacterial antigen-induced responses in infants vaccinated with BCG at birth. BMC Med. Genomics. 2:10. doi: 10.1186/1755-8794-2-10
Flores-Valdez, M. A., Aceves-Sánchez, M., Peterson, E. J. R., Baliga, N., Bravo-Madrigal, J., and De la Cruz-Villegas, M. A. (2020). Transcriptional portrait of M. bovis BCG during biofilm production shows genes differentially expressed during intercellular aggregation and substrate attachment. Sci. Rep. 10:12578. doi: 10.1038/s41598-020-69152-2
Flores-Valdez, M. A., Pedroza-Roldán, C., Aceves-Sánchez, M., De, J., Peterson, E. J. R., Baliga, N. S., et al. (2018). The BCGΔBCG1419c vaccine candidate reduces lung pathology, IL-6, TNF-α, and IL-10 during chronic TB infection. Front. Microbiol. 9:1281. doi: 10.3389/fmicb.2018.01281
Fontán, P., Aris, V., Alvarez, M. E., Ghanny, S., Cheng, J., Soteropoulos, P., et al. (2008a). Mycobacterium tuberculosis sigma factor E regulon modulates the host inflammatory response. J. Infect. Dis. 198, 877–885. doi: 10.1086/591098
Fontán, P., Aris, V., Ghanny, S., Soteropoulos, P., and Smith, I. (2008b). Global transcriptional profile of Mycobacterium tuberculosis during THP-1 human macrophage infection. Infect. Immun. 76, 717–725. doi: 10.1128/IAI.00974-07
Foo, C. S., Lupien, A., Kienle, M., Vocat, A., Benjak, A., Sommer, R., et al. (2018). Arylvinylpiperazine amides, a new class of potent inhibitors targeting QcrB of mycobacterium tuberculosis. MBio 9, e1218–e1276. doi: 10.1128/mBio.01276-18
Fu, L. M., and Shinnick, T. M. (2007). Genome-wide exploration of the drug action of capreomycin on Mycobacterium tuberculosis using Affymetrix oligonucleotide GeneChips. J. Infect. 54, 277–284. doi: 10.1016/j.jinf.2006.05.012
Fu, Y., Yi, Z., Wu, X., Li, J., and Xu, F. (2011). Circulating microRNAs in patients with active pulmonary tuberculosis. J. Clin. Microbiol. 49, 4246–4251. doi: 10.1128/JCM.05459-11
Gao, Q., Kripke, K. E., Saldanha, A. J., Yan, W., Holmes, S., and Small, P. M. (2005). Gene expression diversity among Mycobacterium tuberculosis clinical isolates. Microbiology 151(Pt 1), 5–14. doi: 10.1099/mic.0.27539-0
Gautam, U. S., Mehra, S., and Kaushal, D. (2015). In-vivo gene signatures of Mycobacterium tuberculosis in C3HeB/FeJ mice. PLoS One 10:e0135208. doi: 10.1371/journal.pone.0135208
Gengenbacher, M., Nieuwenhuizen, N., Vogelzang, A., Liu, H., Kaiser, P., Schuerer, S., et al. (2016). Deletion of nuoG from the vaccine candidate Mycobacterium bovis BCG ΔureC:: Hly improves protection against tuberculosis. MBio 7, e619–e676. doi: 10.1128/mBio.00679-16
Gomez-Gonzalez, P. J., Andreu, N., Phelan, J. E., de Sessions, P. F., Glynn, J. R., Crampin, A. C., et al. (2019). An integrated whole genome analysis of Mycobacterium tuberculosis reveals insights into relationship between its genome, transcriptome and methylome. Sci. Rep. 9:5204. doi: 10.1038/s41598-019-41692-2
Gupta, R. K., Turner, C. T., Venturini, C., Esmail, H., Rangaka, M. X., Copas, A., et al. (2020). Concise whole blood transcriptional signatures for incipient tuberculosis: a systematic review and patient-level pooled meta-analysis. Lancet Respir. Med. 8, 395–406. doi: 10.1016/S2213-2600(19)30282-6
Hampshire, T., Soneji, S., Bacon, J., James, B. W., Hinds, J., Laing, K., et al. (2004). Stationary phase gene expression of Mycobacterium tuberculosis following a progressive nutrient depletion: A model for persistent organisms? Tuberculosis 84, 228–238. doi: 10.1016/j.tube.2003.12.010
Hansen, S. G., Zak, D. E., Xu, G., Ford, J. C., Marshall, E. E., Malouli, D., et al. (2018). Prevention of tuberculosis in rhesus macaques by a cytomegalovirus-based vaccine. Nat. Med. 24, 130–143. doi: 10.1038/nm.4473
Higuchi, R., Fockler, C., Dollinger, G., and Watson, R. (1993). Kinetic PCR analysis: Real-time monitoring of DNA amplification reactions. BioTechnology 11, 1026–1030. doi: 10.1038/nbt0993-1026
Hinton, J. C. D., Hautefort, I., Eriksson, S., Thompson, A., and Rhen, M. (2004). Benefits and pitfalls of using microarrays to monitor bacterial gene expression during infection. Curr. Opin. Microbiol. 7, 277–282. doi: 10.1016/j.mib.2004.04.009
Hoang, L. T., Jain, P., Pillay, T. D., Tolosa-Wright, M., Niazi, U., Takwoingi, Y., et al. (2021). Transcriptomic signatures for diagnosing tuberculosis in clinical practice: a prospective, multicentre cohort study. Lancet Infect. Dis. 21, 366–375. doi: 10.1016/S1473-3099(20)30928-2
Hoft, D. F., Blazevic, A., Selimovic, A., Turan, A., Tennant, J., Abate, G., et al. (2016). Safety and Immunogenicity of the Recombinant BCG Vaccine AERAS-422 in Healthy BCG-naïve adults: a randomized, active-controlled, first-in-human phase 1 Trial. EBioMedicine 7, 278–286. doi: 10.1016/j.ebiom.2016.04.010
Huang, L., Nazarova, E. V., Tan, S., Liu, Y., and Russell, D. G. (2018). Growth of Mycobacterium tuberculosis in vivo segregates with host macrophage metabolism and ontogeny. J. Exp. Med. 215, 1135–1152. doi: 10.1084/jem.20172020
Jacobsen, M., Repsilber, D., Gutschmidt, A., Neher, A., Feldmann, K., Mollenkopf, H. J., et al. (2007). Candidate biomarkers for discrimination between infection and disease caused by Mycobacterium tuberculosis. J. Mol. Med. 85, 613–621. doi: 10.1007/s00109-007-0157-6
Jacobsen, M., Repsilber, D., Kleinsteuber, K., Gutschmidt, A., Schommer-Leitner, S., Black, G., et al. (2010). Suppressor of cytokine signaling-3 is affected in T-cells from tuberculosis TB patients. Clin. Microbiol. Infect. 17, 1323–1331. doi: 10.1111/j.1469-0691.2010.03326.x
Joosten, S. A., Fletcher, H. A., and Ottenhoff, T. H. M. A. (2013). Helicopter Perspective on tb biomarkers: pathway and process based analysis of gene expression data provides new insight into TB Pathogenesis. PLoS One 8:e73230. doi: 10.1371/journal.pone.0073230
Kaforou, M., Wright, V. J., Oni, T., French, N., Anderson, S. T., Bangani, N., et al. (2013). Detection of Tuberculosis in HIV-Infected and -Uninfected African Adults Using Whole Blood RNA Expression Signatures: A Case-Control Study. PLoS Med. 10:e1001538. doi: 10.1371/journal.pmed.1001538
Kagina, B. M. N., Abel, B., Scriba, T. J., Hughes, E. J., Keyser, A., Soares, A., et al. (2010). Specific T cell frequency and cytokine expression profile do not correlate with protection against tuberculosis after bacillus Calmette-Guérin vaccination of newborns. Am. J. Respir. Crit. Care Med. 182, 1073–1079.
Karakousis, P. C., Williams, E. P., and Bishai, W. R. (2008). Altered expression of isoniazid-regulated genes in drug-treated dormant Mycobacterium tuberculosis. J. Antimicrob. Chemother. 61, 323–331. doi: 10.1093/jac/dkm485
Kaufmann, E., Sanz, J., Dunn, J. L., Khan, N., Mendonça, L. E., Pacis, A., et al. (2018). BCG Educates hematopoietic stem cells to generate protective innate immunity against tuberculosis. Cell 172, 176–190. doi: 10.1016/j.cell.2017.12.031
Keren, I., Minami, S., Rubin, E., and Lewis, K. (2011). Characterization and transcriptome analysis of mycobacterium tuberculosis persisters. MBio 2, 3–12. doi: 10.1128/mBio.00100-11
Khan, N., Downey, J., Sanz, J., Kaufmann, E., Blankenhaus, B., Pacis, A., et al. (2020). M. tuberculosis reprograms hematopoietic stem cells to limit myelopoiesis and impair trained Immunity. Cell 183, 752–770. doi: 10.1016/j.cell.2020.09.062
Kim, M. J., Wainwright, H. C., Locketz, M., Bekker, L. G., Walther, G. B., Dittrich, C., et al. (2010). Caseation of human tuberculosis granulomas correlates with elevated host lipid metabolism. EMBO Mol. Med. 2, 258–274. doi: 10.1002/emmm.201000079
Knegt, G. J., DeBruning, O., Marian, T., and De, Jong M, Van, Belkum A, and Endtz, H. P. (2013). Rifampicin-induced transcriptome response in rifampicin-resistant Mycobacterium tuberculosis. Tuberculosis 93, 96–101. doi: 10.1016/j.tube.2012.10.013
Kumar, D., Bansal, G., Narang, A., Basak, T., Abbas, T., and Dash, D. (2016). Integrating transcriptome and proteome profiling: Strategies and applications. Proteomics 16, 2533–2544. doi: 10.1002/pmic.201600140
Kunnath-Velayudhan, S., Goldberg, M. F., Saini, N. K., Johndrow, C. T., Ng, T. W., Johnson, A. J., et al. (2017). Transcriptome Analysis of Mycobacteria-Specific CD4 + T Cells Identified by Activation-Induced Expression of CD154. J. Immunol. 199, 2596–2606. doi: 10.4049/jimmunol.1700654
Lenoir, T., and Giannella, E. (2006). The emergence and diffusion of DNA microarray technology. J. Biomed. Discov. Collab. 1:11. doi: 10.1186/1747-5333-1-11
Lin, W., De Sessions, P. F., Teoh, G. H. K., Mohamed, A. N. N., Zhu, Y. O., Koh, V. H. Q., et al. (2016). Transcriptional profiling of Mycobacterium tuberculosis exposed to In Vitro lysosomal stress. Infect. Immun. 84, 2505–2523. doi: 10.1128/IAI.00072-16
Lister, R., O’Malley, R. C., Tonti-Filippini, J., Gregory, B. D., Berry, C. C., Millar, A. H., et al. (2008). Highly integrated single-base resolution maps of the epigenome in arabidopsis. Cell 133, 523–536. doi: 10.1016/j.cell.2008.03.029
Liu, Y., Wang, X., Jiang, J., Cao, Z., Yang, B., and Cheng, X. (2011). Modulation of T cell cytokine production by miR-144* with elevated expression in patients with pulmonary tuberculosis. Mol. Immunol. 48, 1084–1090. doi: 10.1016/j.molimm.2011.02.001
Loxton, A. G., Knaul, J. K., Grode, L., Gutschmidt, A., Meller, C., Eisele, B., et al. (2017). Safety and immunogenicity of the recombinant mycobacterium bovis BCG vaccine VPM1002 in HIV-unexposed newborn infants in South Africa. Clin. Vaccine Immunol. 24, e419–e439. doi: 10.1128/CVI.00439-16
Maertzdorf, J., Ota, M., Repsilber, D., Mollenkopf, H. J., Weiner, J., Hill, P. C., et al. (2011a). Functional correlations of pathogenesis-driven gene expression signatures in tuberculosis. PLoS One 6:e26938. doi: 10.1371/journal.pone.0026938
Maertzdorf, J., Repsilber, D., Parida, S. K., Stanley, K., Roberts, T., Black, G., et al. (2011b). Human gene expression profiles of susceptibility and resistance in tuberculosis. Genes Immun. 12, 15–22. doi: 10.1038/gene.2010.51
Maertzdorf, J., Weiner, J., Mollenkopf, H. J., Network, T. B. T., Bauer, T., and Prasse, A. (2012). Common patterns and disease-related signatures in tuberculosis and sarcoidosis. Proc. Natl. Acad. Sci. USA 109, 7853–7858. doi: 10.1073/pnas.1121072109
Manganelli, R., Voskuil, M. I., Schoolnik, G. K., Dubnau, E., Gomez, M., and Smith, I. (2002). Role of the extracytoplasmic-function σ factor σH in Mycobacterium tuberculosis global gene expression. Mol. Microbiol. 45, 365–374. doi: 10.1046/j.1365-2958.2002.03005.x
Manjunatha, U., Boshoff, H. I. M., and Barry, C. E. (2009). The mechanism of action of PA-824. Commun. Integr. Biol. 2, 215–218. doi: 10.4161/cib.2.3.7926
Marakalala, M. J., Raju, R. M., Sharma, K., Zhang, Y. J., Eugenin, E. A., Prideaux, B., et al. (2016). Inflammatory signaling in human tuberculosis granulomas is spatially organized. Nat. Med. 22, 531–538. doi: 10.1038/nm.4073
Mardis, E. R. (2011). A decade’s perspective on DNA sequencing technology. Nature 470, 198–203. doi: 10.1038/nature09796
Martínez-Pérez, A., Igea, A., Estévez, O., Ferreira, C. M., Torrado, E., Castro, A. G., et al. (2021). Changes in the immune phenotype and gene expression profile driven by a novel tuberculosis nanovaccine: short and long-term post-immunization. Front. Immunol. 11:589863. doi: 10.3389/fimmu.2020.589863
McGillivray, A., Golden, N. A., and Kaushal, D. (2015). The Mycobacterium tuberculosis Clp gene regulator is required for in vitro reactivation from hypoxia-induced dormancy. J. Biol. Chem. 290, 2351–2367. doi: 10.1074/jbc.M114.615534
Mehra, S., Alvarez, X., Didier, P. J., Doyle, L. A., Blanchard, J. L., Lackner, A. A., et al. (2013). Granuloma correlates of protection against tuberculosis and mechanisms of immune modulation by Mycobacterium tuberculosis. J. Infect. Dis. 207, 1115–1127. doi: 10.1093/infdis/jis778
Mehra, S., and Kaushal, D. (2009). Functional genomics reveals extended roles of the Mycobacterium tuberculosis stress response factor. J. Bacteriol. 191, 3965–3980. doi: 10.1128/JB.00064-09
Mehra, S., Foreman, T. W., Didier, P. J., Ahsan, M. H., Hudock, T. A., Kissee, R., et al. (2015). The DosR regulon modulates adaptive immunity and is essential for mycobacterium tuberculosis persistence. Am. J. Respir. Crit. Care Med. 191, 1185–1196. doi: 10.1164/rccm.201408-1502OC
Mehra, S., Pahar, B., Dutta, N. K., Conerly, C. N., Philippi-Falkenstein, K., Alvarez, X., et al. (2010). Transcriptional reprogramming in nonhuman primate (Rhesus Macaque) tuberculosis granulomas. PLoS One 5:e12266. doi: 10.1371/journal.pone.0012266
Mistry, R., Cliff, J. M., Clayfon, C. L., Beyers, N., Mohamed, Y. S., Wilson, P. A., et al. (2007). Gene-expression patterns in whole blood identify subjects at risk for recurrent tuberculosis. J. Infect. Dis. 195, 357–365. doi: 10.1086/510397
Mollenkopf, H. J., Hahnke, K., and Kaufmann, S. H. E. (2006). Transcriptional responses in mouse lungs induced by vaccination with Mycobacterium bovis BCG and infection with Mycobacterium tuberculosis. Microb. Infect. 8, 136–144. doi: 10.1016/j.micinf.2005.06.015
Moreira-Teixeira, L., Stimpson, P. J., Stavropoulos, E., Hadebe, S., Chakravarty, P., Ioannou, M., et al. (2020a). Type I IFN exacerbates disease in tuberculosis-susceptible mice by inducing neutrophil-mediated lung inflammation and NETosis. Nat. Commun. 11:5566.
Moreira-Teixeira, L., Tabone, O., Graham, C. M., Singhania, A., Stavropoulos, E., Redford, P. S., et al. (2020b). Mouse transcriptome reveals potential signatures of protection and pathogenesis in human tuberculosis. Nat. Immunol. 21, 464–476. doi: 10.1038/s41590-020-0610-z
Mortazavi, A., Williams, B. A., McCue, K., Schaeffer, L., and Wold, B. (2008). Mapping and quantifying mammalian transcriptomes by RNA-Seq. Nat. Methods. 5, 621–628. doi: 10.1038/nmeth.1226
Mullis, K. B. (1990). The unusual origin of the polymerase chain reaction. Sci. Am. 262, 56–61. doi: 10.1038/scientificamerican0490-56
Murphy, D. J., and Brown, J. R. (2007). Identification of gene targets against dormant phase Mycobacterium tuberculosis infections. BMC Infect. Dis. 7:84. doi: 10.1186/1471-2334-7-84
Muttucumaru, D. G. N., Roberts, G., Hinds, J., Stabler, R. A., and Parish, T. (2004). Gene expression profile of Mycobacterium tuberculosis in a non-replicating state. Tuberculosis 84, 239–246. doi: 10.1016/j.tube.2003.12.006
Nathan, A., Beynor, J. I., Baglaenko, Y., Suliman, S., Ishigaki, K., Asgari, S., et al. (2021). Multimodally profiling memory T cells from a tuberculosis cohort identifies cell state associations with demographics, environment and disease. Nat. Immunol. 22, 781–793. doi: 10.1038/s41590-021-00933-1
Ohno, H., Zhu, G., Mohan, V. P., Chu, D., Kohno, S., Jacobs, W. R., et al. (2003). The effects of reactive nitrogen intermediates on gene expression in Mycobacterium tuberculosis. Cell Microbiol. 5, 637–648. doi: 10.1046/j.1462-5822.2003.00307.x
Okubo, K., Hori, N., Matoba, R., Niiyama, T., Fukushima, A., Kojima, Y., et al. (1992). Large scale cDNA sequencing for analysis of quantitative and qualitative aspects of gene expression. Nat. Genet. 2, 173–179. doi: 10.1038/ng1192-173
Ottenhoff, T. H. M., Dass, R. H., Yang, N., Zhang, M. M., Wong, H. E. E., Sahiratmadja, E., et al. (2012). Genome-wide expression profiling identifies type 1 interferon response pathways in active tuberculosis. PLoS One 7:e45839. doi: 10.1371/journal.pone.0045839
Park, H. D., Guinn, K. M., Harrell, M. I., Liao, R., Voskuil, M. I., Tompa, M., et al. (2003). Rv3133c/dosR is a transcription factor that mediates the hypoxic response of Mycobacterium tuberculosis. Mol. Microbiol. 48, 833–843. doi: 10.1046/j.1365-2958.2003.03474.x
Penn-Nicholson, A., Mbandi, S. K., Thompson, E., Mendelsohn, S. C., Suliman, S., Chegou, N. N., et al. (2020). RISK6, a 6-gene transcriptomic signature of TB disease risk, diagnosis and treatment response. Sci. Rep. 10:8629. doi: 10.1038/s41598-020-65043-8
Peñuelas-Urquides, K., González-Escalante, L., Villarreal-Treviño, L., Silva-Ramírez, B., Gutiérrez-Fuentes, D. J., Mojica-Espinosa, R., et al. (2013). Comparison of gene expression profiles between pansensitive and multidrug-resistant strains of mycobacterium tuberculosis. Curr. Microbiol. 67, 362–371. doi: 10.1007/s00284-013-0376-8
Pisu, D., Huang, L., Grenier, J. K., and Russell, D. G. (2020). Dual RNA-Seq of Mtb-infected macrophages in vivo reveals ontologically distinct host-pathogen interactions. Cell Rep. 30, 335–350. doi: 10.1016/j.celrep.2019.12.033
Pisu, D., Huang, L., Narang, V., Theriault, M., Lê-Bury, G., Lee, B., et al. (2021). Single cell analysis of M. tuberculosis phenotype and macrophage lineages in the infected lung. J. Exp. Med. 218:e20210615. doi: 10.1084/jem.20210615
Provvedi, R., Boldrin, F., Falciani, F., and Palu, G. (2009). Global transcriptional response to vancomycin in Mycobacterium tuberculosis Printed in Great Britain. Microbiology 155, 1093–1102. doi: 10.1099/mic.0.024802-0
Rachman, H., Strong, M., Schaible, U., Schuchhardt, J., Hagens, K., Mollenkopf, H., et al. (2006a). Mycobacterium tuberculosis gene expression profiling within the context of protein networks. Microb. Infect. 8, 747–757. doi: 10.1016/j.micinf.2005.09.011
Rachman, H., Strong, M., Ulrichs, T., Grode, L., Schuchhardt, J., Mollenkopf, H., et al. (2006b). Unique transcriptome signature of Mycobacterium tuberculosis in pulmonary tuberculosis. Infect. Immun. 74, 1233–1242. doi: 10.1128/IAI.74.2.1233-1242.2006
Ragno, S., Romano, M., Howell, S., Pappin, D. J. C., Jenner, P. J., and Colston, M. J. (2001). Changes in gene expression in macrophages infected with Mycobacterium tuberculosis: A combined transcriptomic and proteomic approach. Immunology 104, 99–108. doi: 10.1046/j.0019-2805.2001.01274.x
Rengarajan, J., Bloom, B. R., and Rubin, E. J. (2005). Genome-wide requirements for Mycobacterium tuberculosis adaptation and survival in macrophages. Proc. Natl. Acad. Sci. USA 102, 8327–8332. doi: 10.1073/pnas.0503272102
Rienksma, R. A., Suarez-Diez, M., Mollenkopf, H. J., Dolganov, G. M., Dorhoi, A., Schoolnik, G. K., et al. (2015). Comprehensive insights into transcriptional adaptation of intracellular mycobacteria by microbe-enriched dual RNA sequencing. BMC Genomics 16:34. doi: 10.1186/s12864-014-1197-2
Roe, J. K., Thomas, N., Gil, E., Best, K., and Tsaliki, E. (2016). Blood transcriptomic diagnosis of pulmonary and extrapulmonary tuberculosis. JCI Insight 1:e87238. doi: 10.1172/jci.insight.87238
Roe, J., Venturini, C., Gupta, R. K., Gurry, C., Chain, B. M., Sun, Y., et al. (2020). Blood transcriptomic stratification of short-term risk in contacts of tuberculosis. Clin. Infect. Dis. 70, 731–737. doi: 10.1093/cid/ciz252
Rohde, K. H., Abramovitch, R. B., and Russell, D. G. (2007). Mycobacterium tuberculosis Invasion of macrophages: linking bacterial gene expression to environmental Cues. Cell Host Microbe. 2, 352–364. doi: 10.1016/j.chom.2007.09.006
Rohde, K. H., Veiga, D. F. T., Caldwell, S., Balázsi, G., and Russell, D. G. (2012). Linking the transcriptional profiles and the physiological states of Mycobacterium tuberculosis during an extended intracellular infection. PLoS Pathog. 8:e1002769. doi: 10.1371/journal.ppat.1002769
Rose, G., Cortes, T., Comas, I., Coscolla, M., Gagneux, S., and Young, D. B. (2013). Mapping of genotype-phenotype diversity among clinical isolates of Mycobacterium tuberculosis by sequence-based transcriptional profiling. Genome Biol. Evol. 5, 1849–1862. doi: 10.1093/gbe/evt138
Roy, S., Schmeier, S., Kaczkowski, B., Arner, E., Alam, T., Ozturk, M., et al. (2018). Transcriptional landscape of Mycobacterium tuberculosis infection in macrophages. Sci. Rep. 8:6758. doi: 10.1038/s41598-018-24509-6
Rustad, T. R., Harrell, M. I., Liao, R., and Sherman, D. R. (2008). The enduring hypoxic response of Mycobacterium tuberculosis. PLoS One 3:e1502.
Saini, V., Chinta, K. C., Reddy, V. P., Glasgow, J. N., Stein, A., Lamprecht, D. A., et al. (2020). Hydrogen sulfide stimulates Mycobacterium tuberculosis respiration, growth and pathogenesis. Nat. Commun. 11:557. doi: 10.1038/s41467-019-14132-y
Salamon, H., Bruiners, N., Lakehal, K., Shi, L., Ravi, J., Yamaguchi, K. D., et al. (2014). Cutting Edge: Vitamin D regulates lipid metabolism in mycobacterium tuberculosis Infection. J. Immunol. 193, 30–34. doi: 10.4049/jimmunol.1400736
Santoro, F., Pettini, E., Kazmin, D., Ciabattini, A., Fiorino, F., Gilfillan, G. D., et al. (2018). Transcriptomics of the vaccine immune response: Priming with adjuvant modulates recall innate responses after boosting. Front. Immunol. 9:1248. doi: 10.3389/fimmu.2018.01248
Schena, M., Shalon, D., Davis, R. W., and Brown, P. O. (1995). Quantitative monitoring of gene expression patterns with a complementary DNA microarray. Science 270, 467–470. doi: 10.1126/science.270.5235.467
Schnappinger, D., Ehrt, S., Voskuil, M. I., Liu, Y., Mangan, J. A., Monahan, I. M., et al. (2003). Transcriptional adaptation of Mycobacterium tuberculosis within macrophages: Insights into the phagosomal environment. J. Exp. Med. 198, 693–704. doi: 10.1084/jem.20030846
Scriba, T. J., Penn-Nicholson, A., Shankar, S., Hraha, T., Thompson, E. G., Sterling, D., et al. (2017). Sequential inflammatory processes define human progression from M. tuberculosis infection to tuberculosis disease. PLoS Pathog. 13:e1006687. doi: 10.1371/journal.ppat.1006687
Seiler, P., Aichele, P., Bandermann, S., Hauser, A. E., Lu, B., Gerard, N. P., et al. (2003). Early granuloma formation after aerosol Mycobacterium tuberculosis infection is regulated by neutrophils via CXCR3-signaling chemokines. Eur. J. Immunol. 33, 2676–2686. doi: 10.1002/eji.200323956
Shaikh, A., Sriraman, K., Vaswani, S., Oswal, V., Rao, S., and Mistry, N. (2021). Early phase of effective treatment induces distinct transcriptional changes in Mycobacterium tuberculosis expelled by pulmonary tuberculosis patients. Sci. Rep. 11:17812. doi: 10.1038/s41598-021-96902-7
Sharbati, J., Lewin, A., Kutz-Lohroff, B., Kamal, E., Einspanier, R., and Sharbati, S. (2011). Integrated microrna-mrna-analysis of human monocyte derived macrophages upon mycobacterium avium subsp. hominissuis infection. PLoS One 6:e20258. doi: 10.1371/journal.pone.0020258
Sharma, S., Ryndak, M. B., Aggarwal, A. N., Yadav, R., Sethi, S., Masih, S., et al. (2017). Transcriptome analysis of mycobacteria in sputum samples of pulmonary tuberculosis patients. PLoS One 12:e0173508. doi: 10.1371/journal.pone.0173508
Sherman, D. R., Voskuil, M., Schnappinger, D., Liao, R., Harrell, M. I., and Schoolnik, G. K. (2001). Regulation of the mycobacterium tuberculosis hypoxic response gene encoding α-crystallin. Proc. Natl. Acad. Sci. USA 98, 7534–7539. doi: 10.1073/pnas.121172498
Shi, S., Nathan, C., Schnappinger, D., Drenkow, J., Fuortes, M., Block, E., et al. (2003). MyD88 primes macrophages for full-scale activation by interferon-γ yet mediates few responses to Mycobacterium tuberculosis. J. Exp. Med. 198, 987–997. doi: 10.1084/jem.20030603
Shiraki, T., Kondo, S., Katayama, S., Waki, K., Kasukawa, T., Kawaji, H., et al. (2003). Cap analysis gene expression for high-throughput analysis of transcriptional starting point and identification of promoter usage. Proc. Natl. Acad. Sci. USA 100, 15776–15781. doi: 10.1073/pnas.2136655100
Singhania, A., Verma, R., Graham, C. M., Lee, J., Tran, T., Richardson, M., et al. (2018). A modular transcriptional signature identifies phenotypic heterogeneity of human tuberculosis infection. Nat. Commun. 9:2308. doi: 10.1038/s41467-018-04579-w
Ståhl, P. L., Salmén, F., Vickovic, S., Lundmark, A., Navarro, J. F., Magnusson, J., et al. (2016). Visualization and analysis of gene expression in tissue sections by spatial transcriptomics. Science 353, 78–82. doi: 10.1126/science.aaf2403
Stewart, G. R., Wernisch, L., Stabler, R., Mangan, J. A., Hinds, J., Laing, K. G., et al. (2002). Dissection of the heat-shock response in Mycobacterium tuberculosis using mutants and microarrays. Microbiology 148, 3129–3138. doi: 10.1099/00221287-148-10-3129
Suliman, S., Thompson, E. G., Sutherland, J., Weiner, J., Ota, M. O. C., Shankar, S., et al. (2018). Four-gene pan-African blood signature predicts progression to tuberculosis. Am. J. Respir. Crit. Care Med. 197, 1198–1208. doi: 10.1164/rccm.201711-2340OC
Sullivan, D. M. O., Hinds, J., Butcher, P. D., Gillespie, S. H., Mchugh, T. D., Medicine, M., et al. (2008). Mycobacterium tuberculosis DNA repair in response to subinhibitory concentrations of ciprofloxacin. J. Antimicrob. Chemother. 62, 1199–1202. doi: 10.1093/jac/dkn387
Sweeney, T. E., Braviak, L., Tato, C. M., and Khatri, P. (2016). Genome-wide expression for diagnosis of pulmonary tuberculosis: a multicohort analysis. Lancet Respir. Med. 4, 213–224. doi: 10.1016/S2213-2600(16)00048-5
Tabone, O., Verma, R., Singhania, A., Chakravarty, P., Branchett, W. J., Graham, C. M., et al. (2021). Blood transcriptomics reveal the evolution and resolution of the immune response in tuberculosis. J. Exp. Med. 218, e20210915. doi: 10.1084/jem.20210915
Talaat, A. M., Lyons, R., Howard, S. T., and Johnston, S. A. (2004). The temporal expression profile of Mycobacterium tuberculosis infection in mice. Proc. Natl. Acad. Sci. USA 101, 4602–4607. doi: 10.1073/pnas.0306023101
Tan, S., Sukumar, N., Abramovitch, R. B., Parish, T., and Russell, D. G. (2013). Mycobacterium tuberculosis Responds to Chloride and pH as Synergistic Cues to the Immune Status of its Host Cell. PLoS Pathog. 9:e1003282. doi: 10.1371/journal.ppat.1003282
Tang, F., Barbacioru, C., Wang, Y., Nordman, E., Lee, C., Xu, N., et al. (2009). mRNA-Seq whole-transcriptome analysis of a single cell. Nat. Methods 6, 377–382. doi: 10.1038/nmeth.1315
Thompson, E. G., Du, Y., Malherbe, S. T., Shankar, S., Braun, J., Valvo, J., et al. (2017). Host blood RNA signatures predict the outcome of tuberculosis treatment. Tuberculosis 107, 48–58. doi: 10.1016/j.tube.2017.08.004
van den Berg, R. A., De Mot, L., Leroux-Roels, G., Bechtold, V., Clement, F., Coccia, M., et al. (2018). Adjuvant-associated peripheral blood mRNA profiles and kinetics induced by the adjuvanted recombinant protein candidate tuberculosis vaccine M72/AS01 in Bacillus Calmette-Guérin-vaccinated adults. Front. Immunol. 9:564. doi: 10.3389/fimmu.2018.00564
Velculescu, V. E., Zhang, L., Vogelstein, B., and Kinzler, K. W. (1995). Serial analysis of gene expression. Science 270, 484–487.
Volpe, E., Cappelli, G., Grassi, M., Martino, A., Serafino, A., Colizzi, V., et al. (2006). Gene expression profiling of human macrophages at late time of infection with Mycobacterium tuberculosis. Immunology 118, 449–460. doi: 10.1111/j.1365-2567.2006.02378.x
Voskuil, M. I., Bartek, I. L., Visconti, K., and Schoolnik, G. K. (2011). The response of Mycobacterium tuberculosis to reactive oxygen and nitrogen species. Front. Microbiol. 2:105.
Voskuil, M. I., Visconti, K. C., and Schoolnik, G. K. (2004). Mycobacterium tuberculosis gene expression during adaptation to stationary phase and low-oxygen dormancy. Tuberculosis 84, 218–227. doi: 10.1016/j.tube.2004.02.003
Waddell, S. J., Stabler, R. A., Laing, K., Kremer, L., Reynolds, R. C., and Besra, G. S. (2004). The use of microarray analysis to determine the gene expression profiles of Mycobacterium tuberculosis in response to anti-bacterial compounds. Tuberculosis 84, 263–274. doi: 10.1016/j.tube.2003.12.005
Wang, C., Yang, S., Sun, G., Tang, X., Lu, S., Neyrolles, O., et al. (2011). Comparative miRNA expression profiles in individuals with latent and active tuberculosis. PLoS One 6:e25832. doi: 10.1371/journal.pone.0025832
Wang, Z., Gerstein, M., and Snyder, M. R. N. A. - (2009). Seq: a revolutionary tool for transcriptomics. Nat. Rev. Genet. 10, 57–63. doi: 10.1038/nrg2484
Warsinske, H., Vashisht, R., and Khatri, P. (2019). Host-response-based gene signatures for tuberculosis diagnosis: A systematic comparison of 16 signatures. PLoS Med. 16:e1002786. doi: 10.1371/journal.pmed.1002786
Weiner, J., Domaszewska, T., Donkor, S., Kaufmann, S. H. E., Hill, P. C., and Sutherland, J. S. (2020). Changes in transcript, metabolite, and antibody reactivity during the early protective immune response in humans to mycobacterium tuberculosis infection. Clin. Infect. Dis. 71, 30–40. doi: 10.1093/cid/ciz785
Wilson, M., Derisi, J., Kristensen, H., Imboden, P., Rane, S., Brown, P. O., et al. (1999). Exploring drug-induced alterations in gene expression in Mycobacterium tuberculosis by microarray hybridization. Proc. Natl. Acad. Sci. USA 96, 12833–12838. doi: 10.1073/pnas.96.22.12833
Zak, D. E., Penn-Nicholson, A., Scriba, T. J., Thompson, E., Suliman, S., Amon, L. M., et al. (2016). A blood RNA signature for tuberculosis disease risk: a prospective cohort study. Lancet 387, 2312–2322. doi: 10.1016/S0140-6736(15)01316-1
Zárate-Bladés, C. R., Bonato, V. L. D., da Silveira, E. L. V., OliveiraePaula, M., Junta, C. M., and Sandrin-Garcia, P. (2009). Comprehensive gene expression profiling in lungs of mice infected with Mycobacterium tuberculosis following DNAhsp65 immunotherapy. J. Gene. Med. 11, 66–78. doi: 10.1002/jgm.1269
Zhang, X., Guo, J., Fan, S., Li, Y., Wei, L., Yang, X., et al. (2013). Screening and identification of six serum microRNAs As novel potential combination biomarkers for pulmonary tuberculosis diagnosis. PLoS One 8:e81076. doi: 10.1371/journal.pone.0081076
Zhao, S., Fung-Leung, W. P., Bittner, A., Ngo, K., and Liu, X. (2014). Comparison of RNA-Seq and microarray in transcriptome profiling of activated T cells. PLoS One 9:e78644. doi: 10.1371/journal.pone.0078644
Zheng, H., Williams, J. T., Coulson, G. B., Haiderer, E. R., and Abramovitch, R. B. (2018). HC2091 kills mycobacterium tuberculosis by targeting the MmpL3 mycolic acid transporter. Antimicrob. Agents Chemother. 62, e2417–e2459. doi: 10.1128/AAC.02459-17
Keywords: transcriptomics, tuberculosis, mycobacteria, RNA-sequencing, microarray, immune response, drug resistance
Citation: Martínez-Pérez A, Estévez O and González-Fernández Á (2022) Contribution and Future of High-Throughput Transcriptomics in Battling Tuberculosis. Front. Microbiol. 13:835620. doi: 10.3389/fmicb.2022.835620
Received: 14 December 2021; Accepted: 25 January 2022;
Published: 24 February 2022.
Edited by:
Joanna Kirman, University of Otago, New ZealandReviewed by:
Gang Cao, Huazhong Agricultural University, ChinaMargarida Saraiva, Universidade do Porto, Portugal
Htin Lin Aung, University of Otago, New Zealand
Copyright © 2022 Martínez-Pérez, Estévez and González-Fernández. This is an open-access article distributed under the terms of the Creative Commons Attribution License (CC BY). The use, distribution or reproduction in other forums is permitted, provided the original author(s) and the copyright owner(s) are credited and that the original publication in this journal is cited, in accordance with accepted academic practice. No use, distribution or reproduction is permitted which does not comply with these terms.
*Correspondence: Amparo Martínez-Pérez, ammartinez@uvigo.es