- 1National Food Institute, Technical University of Denmark, Kongens Lyngby, Denmark
- 2Department of Bacteria, Parasites and Fungi, Statens Serum Institut, Copenhagen, Denmark
- 3Department of Clinical Microbiology, Herlev and Gentofte Hospital, Herlev, Denmark
- 4Department of Clinical Microbiology, Aalborg University Hospital, Aalborg, Denmark
- 5Department of Clinical Medicine, Aalborg University, Aalborg, Denmark
- 6Department of Clinical Microbiology, Aarhus University Hospital, Aarhus, Denmark
- 7Department of Clinical Microbiology, Odense University Hospital, Odense, Denmark
- 8Department of Clinical Microbiology, Slagelse Hospital, Slagelse, Denmark
- 9Department of Clinical Microbiology, Rigshospitalet, Copenhagen, Denmark
- 10Department of Clinical Microbiology, Hospital of Southern Jutland, Sønderborg, Denmark
- 11Department of Clinical Microbiology, Hospital of South West Jutland, Esbjerg, Denmark
- 12Department of Clinical Microbiology, Vejle Hospital, Vejle, Denmark
- 13Department of Clinical Microbiology, Hvidovre Hospital, Copenhagen University Hospital – Amager and Hvidovre, Hvidovre, Denmark
- 14Department of Clinical Medicine, University of Copenhagen, Copenhagen, Denmark
Antimicrobial susceptibility testing (AST) should be fast and accurate, leading to proper interventions and therapeutic success. Clinical microbiology laboratories rely on phenotypic methods, but the continuous improvement and decrease in the cost of whole-genome sequencing (WGS) technologies make them an attractive alternative. Studies evaluating the performance of WGS-based prediction of antimicrobial resistance (AMR) for selected bacterial species have shown promising results. There are, however, significant gaps in the literature evaluating the applicability of WGS as a diagnostics method in real-life clinical settings against the range of bacterial pathogens experienced there. Thus, we compared standard phenotypic AST results with WGS-based predictions of AMR profiles in bacterial isolates without preselection of defined species, to evaluate the applicability of WGS as a diagnostics method in clinical settings. We collected all bacterial isolates processed by all Danish Clinical Microbiology Laboratories in 1 day. We randomly selected 500 isolates without any preselection of species. We performed AST through standard broth microdilution (BMD) for 488 isolates (n = 6,487 phenotypic AST results) and compared results with in silico antibiograms obtained through WGS (Illumina NextSeq) followed by bioinformatics analyses using ResFinder 4.0 (n = 5,229 comparisons). A higher proportion of AMR was observed for Gram-negative bacteria (10.9%) than for Gram-positive bacteria (6.1%). Comparison of BMD with WGS data yielded a concordance of 91.7%, with discordant results mainly due to phenotypically susceptible isolates harboring genetic AMR determinants. These cases correspond to 6.2% of all isolate-antimicrobial combinations analyzed and to 6.8% of all phenotypically susceptible combinations. We detected fewer cases of phenotypically resistant isolates without any known genetic resistance mechanism, particularly 2.1% of all combinations analyzed, which corresponded to 26.4% of all detected phenotypic resistances. Most discordances were observed for specific combinations of species-antimicrobial: macrolides and tetracycline in streptococci, ciprofloxacin and β-lactams in combination with β-lactamase inhibitors in Enterobacterales, and most antimicrobials in Pseudomonas aeruginosa. WGS has the potential to be used for surveillance and routine clinical microbiology. However, in clinical microbiology settings and especially for certain species and antimicrobial agent combinations, further developments in AMR gene databases are needed to ensure higher concordance between in silico predictions and expected phenotypic AMR profiles.
Introduction
Antimicrobial susceptibility testing (AST) is one of the main components of clinical microbiology diagnostics. With the emergence of new antimicrobial resistance (AMR) mechanisms and multi- or pan-drug resistant organisms, it becomes increasingly important to ensure that adequate antimicrobial susceptibility profiles are available in a fast and accurate manner, leading to proper interventions, drug prescriptions, and therapeutic success (Cassini et al., 2019).
Broth microdilution (BMD) is the AST method endorsed by the European Committee on Antimicrobial Susceptibility Testing (EUCAST), performed according to the recommendations from the International Organization for Standardization [ISO 20776-1:2006 (International Organization for Standardization, 2006) and ISO 20776-1:2019 (International Organization for Standardization, 2019)]. BMD requires relatively simple techniques and limited costs for well-developed laboratories (Jorgensen and Ferraro, 2009; Benkova et al., 2020). However, the reproducibility of BMD and other phenotypic AST protocols, such as disk diffusion, remains a concern. Small variations in, for example, operators’ methodology, laboratory materials and reagents, machinery, and culture conditions may translate into different results and respective interpretations (Wiegand et al., 2008). Furthermore, the agreement between results derived from different phenotypic methods and cross-interpretation of the same is not perfect (Jean et al., 2017; Matuschek et al., 2018; Mojica et al., 2020).
Studies have been performed to compare classical phenotypic AST results with in silico antibiograms obtained through whole-genome sequencing (WGS) technologies. Notably, EUCAST performed an in-depth review of phenotype–genotype AMR concordance in important human pathogens, concluding that for certain bacterial groups (such as Enterobacteriaceae and staphylococci), results have been promising with high levels of concordance, while for other species (e.g., Pseudomonas aeruginosa) prove much more difficult to interpret (Ellington et al., 2017). These findings are corroborated by other projects that have shown good concordance between WGS-based antimicrobial susceptibility predictions and minimum inhibitory concentration (MIC) determinations for Enterobacterales (Zankari et al., 2012; Stoesser et al., 2013; Pecora et al., 2015; Clausen et al., 2016; Do Nascimento et al., 2017; Shelburne et al., 2017; Ruppé et al., 2020), enterococci, staphylococci, and streptococci (Aanensen et al., 2016; Metcalf et al., 2016, 2017; Hammerum et al., 2017; Mason et al., 2018; Stewart et al., 2020), while remaining more variables for P. aeruginosa (Kos et al., 2015; Subedi et al., 2018; Cortes-Lara et al., 2021). The main caveat of these and other similar projects is their focus on selected bacterial species, thus not reflecting the diversity observed during routine testing (Tagini and Greub, 2017; Su et al., 2018). Studies evaluating WGS data of non-preselected bacterial isolates collected in a clinical setting exist but are comprised of small collections or mainly focus on species identification and epidemiological analyses, without exploring the use of genomic data for AMR prediction (Long et al., 2013; Hasman et al., 2014; Roach et al., 2015; Galata et al., 2019).
This study was conducted to compare AST results obtained with reference laboratory MIC determinations and WGS predictions, based on a random selection of clinical isolates obtained during a single day from all clinical microbiological laboratories in Denmark.
Materials and Methods
Bacterial Isolates
We collected all clinically relevant isolates (n = 2,073) processed by the 11 Danish Clinical Microbiology Laboratories (DCM) (Herlev and Gentofte Hospital, Herlev; Hvidovre Hospital, Hvidovre; Nykøbing F. Sygehus, Nykøbing F; Odense Universitetshospital, Odense; Rigshospitalet, København; Slagelse Sygehus, Slagelse; Sydvestjysk Sygehus, Esbjerg; Sygehus Lillebælt, Vejle; Sygehus Sønderjylland, Sønderborg; Aalborg Universitetshospital, Aalborg; and Aarhus Universitetshospital, Skejby) on January 10, 2018, as previously described (Rebelo et al., 2022). According to the accompanying metadata, 2,024 of these corresponded to bacterial isolates. Before any analyses or laboratory proceedings, we performed a random selection of 500 isolates using the “=RAND” function on Microsoft Excel to attribute a random number to each of the 2,024 presumptive bacterial isolates, locking the obtained values, sorting them by value from lowest to highest, and selecting the first 500.
Phenotypic Antimicrobial Susceptibility Testing
Antimicrobial susceptibility testing was performed at European Union Reference Laboratory for Antimicrobial Resistance (EURL-AR), at the Technical University of Denmark (DTU) by BMD using Thermo Scientific™ Sensititre™ panels, in particular GN3F (n = 266), GPALL1F (n = 35), EUSTAPF (n = 103), STP6F (n = 70), HPB1 (n = 14), and FRCOL (n = 265, of which 251 were used in combination with GN3F and 14 in combination with HPB1), and performed through agar dilution for anaerobic bacteria (n = 7) according to international standards [ISO 20776-1:2006 (International Organization for Standardization, 2006), EUCAST guidelines (European Committee on Antimicrobial Susceptibility Testing, 2017), and CLSI M11-A8 (Clinical and Laboratory Standards Institute, 2012)] and according to the manufacturer’s specifications.
Interpretation of MIC results was performed using EUCAST clinical breakpoints version 12.0. When different breakpoints existed for “meningitis” and “indications other than meningitis,” the latter was applied due to the absence of isolates from cerebrospinal fluid in this collection. When breakpoints only referred to urinary tract infections, they were still applied to allow for phenotype–genotype comparison. For easier description and discussion of results, we grouped the “susceptible, standard dosing regimen” (S) and “susceptible, increased exposure” (I) categories under the term “susceptible,” as currently recommended by EUCAST (European Committee on Antimicrobial Susceptibility Testing, 2021). All cut-off values are described in Supplementary Table 3.
Whole-Genome Sequencing-Based Antimicrobial Susceptibility Testing
Whole-genome sequencing and bioinformatics analyses were performed at DTU. Genomic DNA was extracted from all bacterial isolates using the Easy-DNA™ Kit (Invitrogen, Carlsbad, CA, United States), and DNA concentrations were determined using the Qubit™ dsDNA high-sensitivity (HS) and/or broad-range (BR) assay kits (Invitrogen, Carlsbad, CA, United States). Genomic DNA was prepared for Illumina paired-end sequencing using the Illumina (Illumina, Inc., San Diego, CA, United States) NexteraXT® DNA Library Prep Reference Guide (Document #15031942, v03, February 2018) and NextSeq System Denature and Dilute Libraries Guide (Document #15048776, v03, April 2018). The libraries were sequenced using the Illumina NextSeq 500 platform. The raw reads were de novo assembled using the Centre for Genomic Epidemiology FoodQCPipeline1 for assembly and quality control. Quality thresholds were set at maximum 500 contigs per genome and maximum 0.5 million base pairs of deviation from the expected genome size. Species identification was performed as previously described (Rebelo et al., 2022) through matrix-assisted laser desorption/ionization – time-of-flight mass spectrometry (MALDI-TOF MS), KmerFinder2 (Larsen et al., 2014; Clausen et al., 2018), and rMLST3 (Jolley et al., 2012).
In silico AST was performed using ResFinder 4.04 (Bortolaia et al., 2020). The program was run in batch by grouping the isolates per species, using the Danish National Supercomputer for Life Sciences5 and using the default threshold values found in the corresponding online tool (minimum accepted alignment of 60% and minimum accepted identity of 90%). Results were manually curated for trimethoprim/sulfamethoxazole in all relevant species (because resistance genes for each antimicrobial are provided separately using the ResFinder 4.0 tool).
We classified WGS results as “resistant” when one or several antimicrobial resistance genes (ARGs) or chromosomal point mutations (PMs) were identified by ResFinder and allocated as the mechanism of AMR to that antimicrobial, and as “susceptible” when no ARG or PM was found.
Raw sequence data have been submitted to the European Nucleotide Archive6 under study accession no.: PRJEB37711. A complete list of genomic sequence data is available in the Supplementary Material.
Comparison of Phenotype and Genotype
We compared MIC results and WGS results for all bacterial species-antimicrobial combinations where EUCAST clinical breakpoints were available and included in the Sensititre™ panel range (excluding intrinsic resistances), while simultaneously being present in the ResFinder 4.0 database (n = 5,229).
The antimicrobials present in the BMD panels but missing from the ResFinder 4.0 database, and thus automatically excluded from the comparison, were as follows: ampicillin/sulbactam, cefaclor, cefazolin, cefpodoxime, ceftaroline, cefuroxime, clarithromycin, daptomycin, levofloxacin, moxifloxacin, nitrofurantoin, norfloxacin, oxacillin, sparfloxacin, and telavancin. The antimicrobials present in the panels and in the ResFinder database but not analyzed due to lack of breakpoints or due to these values being outside of the panel range are available on a species-antimicrobial basis in the Supplementary Material.
Results
Bacterial Isolates
Of the 500 randomly selected bacterial isolates, two were non-viable from the beginning of laboratory work (presumptive Neisseria gonorrhoeae isolates) and one died during laboratory work (presumptive Aerococcus sp. isolate). For one isolate, no MIC values were obtained (Anaerococcus hydrogenalis), and for another, they were not interpretable (undetermined species). Seven anaerobic isolates were excluded due to failure to obtain MIC values in the admitted range for the ATCC control strains.
The MIC and WGS results were obtained and interpreted for a total of 488 bacterial isolates, of which the main species were Escherichia coli (n = 171, 35%) and Staphylococcus aureus (n = 89, 18.2%). The complete distribution of isolates by genera and species can be found in Table 1 and in Supplementary Table 1. Most isolates had been recovered from urine samples (n = 257, 52.7%) or skin/soft tissue samples (n = 61, 12.5%). The complete distribution of the isolated source is found in Figure 1.
Classical Methods for Antimicrobial Susceptibility Testing
For the 488 bacterial isolates, the total number of obtained MIC results was 10,874, including cefoxitin, gentamicin, and streptomycin screens and D-tests. The total number of MIC values interpreted was 6,487 (Tables 2, 3). The remaining MIC values were not interpreted due to lack of breakpoints or due to intrinsic resistance of the respective species to the respective antimicrobial but are provided in the Supplementary Material. The screens and D-tests were interpreted for relevant species. In summary, from the 6,487 isolate-antimicrobial combinations interpreted, 91% (n = 5,901) corresponded to susceptible phenotypes (S or I) and 9% (n = 586) to resistance phenotypes (R).
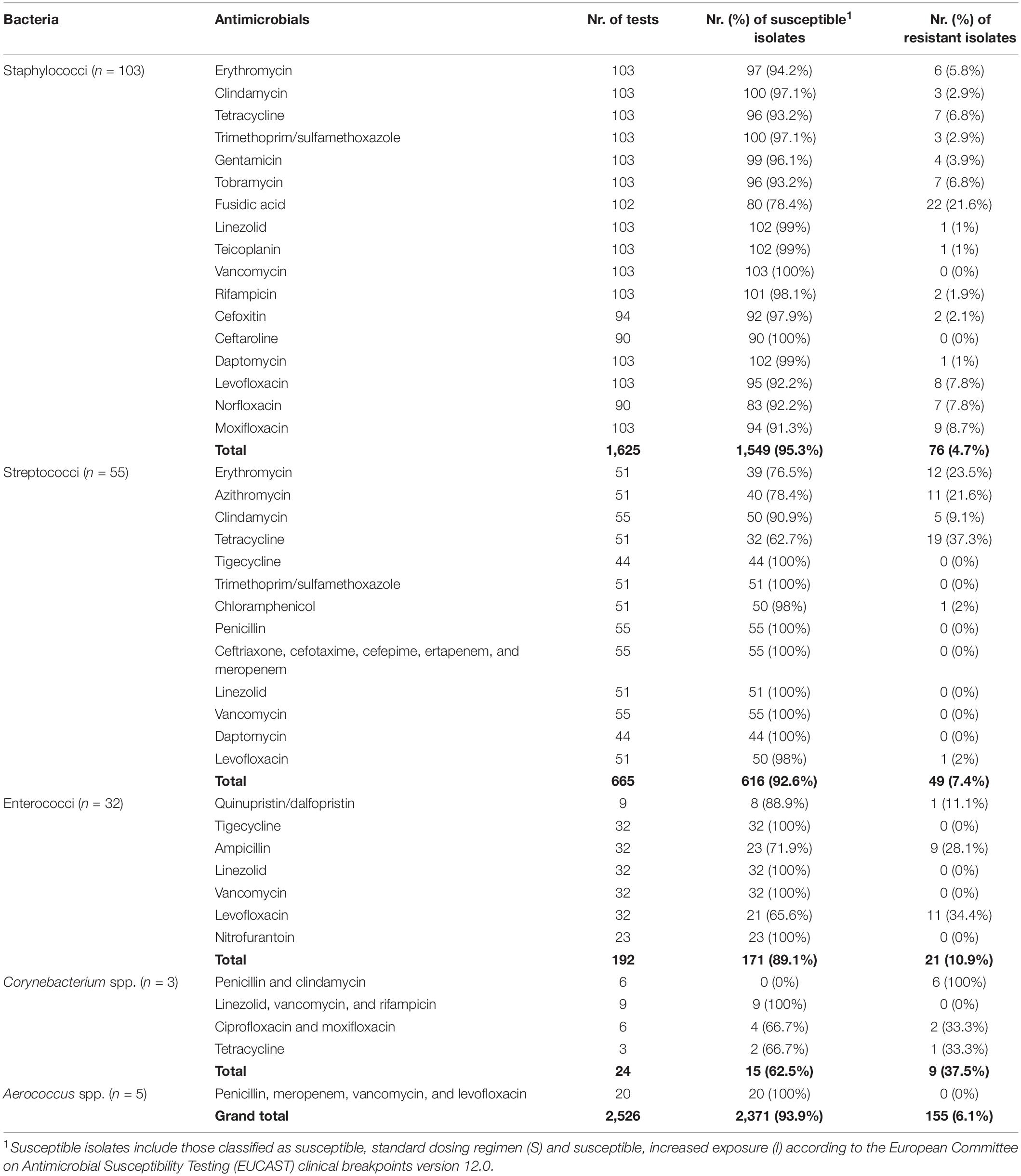
Table 2. Phenotypic antimicrobial susceptibility testing (AST) results obtained through broth microdilution (BMD) for the main Gram-positive bacterial taxa in this study.
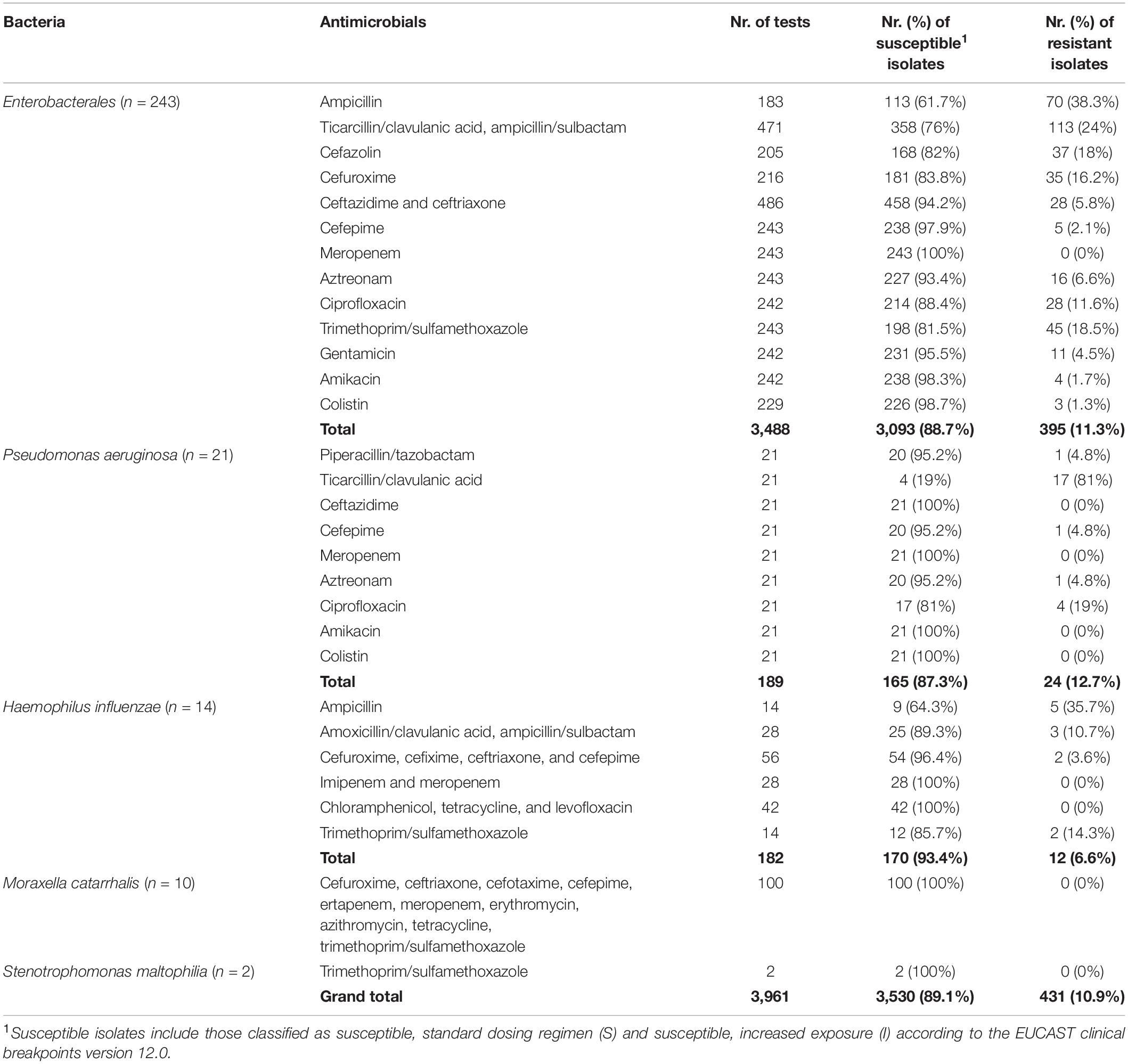
Table 3. Phenotypic AST results obtained through BMD for the main Gram-negative bacterial taxa in this study.
Gram-positive bacteria presented lower proportions of phenotypical resistance (6.1%) than Gram-negative bacteria (10.9%).
Comparison of Phenotype and Genotype
Out of the 5,229 isolate-antimicrobial combinations for which MIC and WGS data were compared, 434 (8.3%) had discordances in phenotypic and genotypic antimicrobial susceptibility profiles. The remaining 4,795 combinations (91.7%) showed a concordance between phenotype and genotype (Figure 2). Of the observed discordances, 75 were observed in Gram-positive bacteria, which corresponded to 3.8% of all 1,969 combinations analyzed in those isolates. Among Gram-negative bacteria, there were 359 discordances, or 11% of the 3,260 combinations analyzed in those taxa (Figure 2).
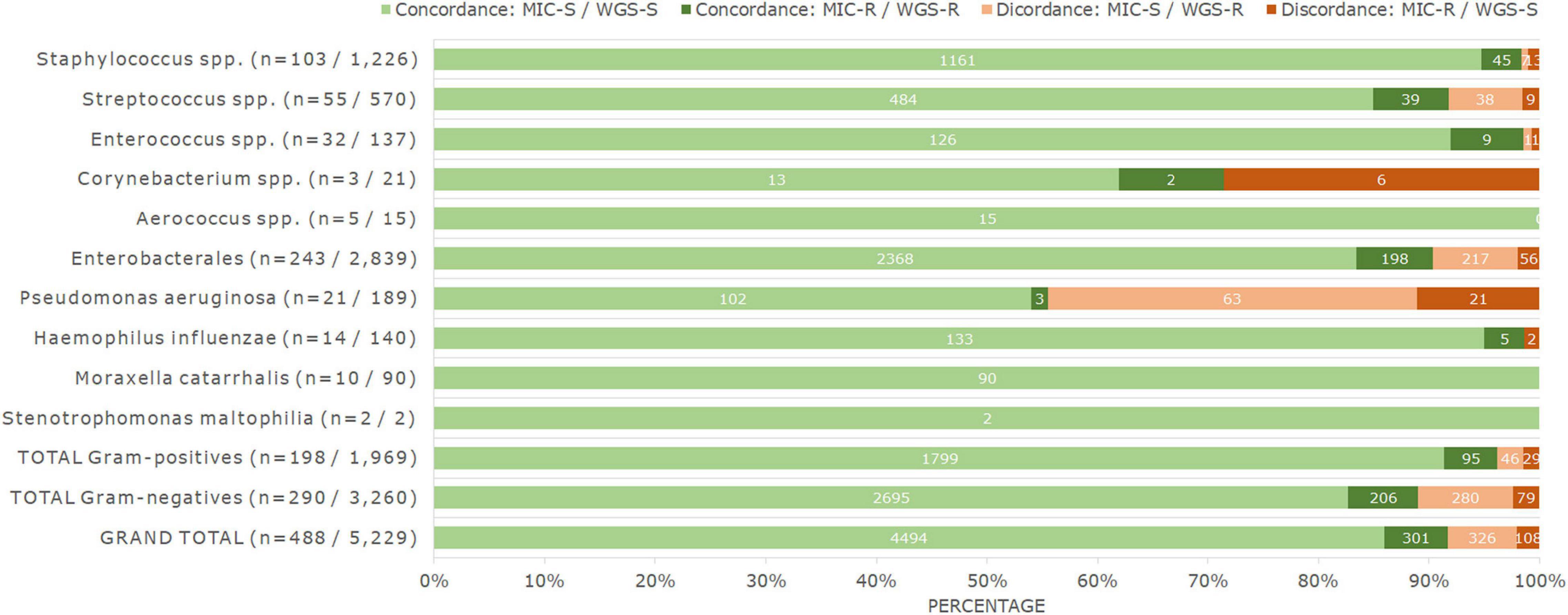
Figure 2. Percentage and number of genotype–phenotype concordances and discordances observed for all bacteria analyzed in this study, when comparing MIC and WGS results. Numbers in brackets for each taxon correspond to the number of isolates, and to the number of isolate-antimicrobial combinations tested. Numbers on top of the bars correspond to the respective number of results. MIC-S cases correspond to S and I phenotypes according to EUCAST clinical breakpoints v12.0, and MIC-R cases correspond to R phenotypes according to the same guideline. WGS-S correspond to cases where no acquired ARGs nor chromosomal PMs have been detected, and WGS-R correspond to cases where they have.
The discordant cases were not equally distributed. For 326 isolate-antimicrobial combinations, ARGs or PMs were detected but the isolate was phenotypically susceptible (major errors). This number corresponded to 6.2% of all combinations analyzed and to 6.8% of all phenotypically susceptible combinations (n = 4,820). The remaining 108 discordances were phenotypically resistant isolates in which no genetic determinants of AMR were detected (very major errors), corresponding to 2.1% of all combinations analyzed and 26.4% of all the detected phenotypic resistances (n = 409) (Figure 2).
In staphylococci, we analyzed 103 isolates with 12 antimicrobials, yielding a total of 1,226 isolate-antimicrobial combinations. In total, 1.6% (n = 20) of results were discordant. Notably, 25% of these discordances occurred for fusidic acid (n = 5 or 4.9% of 102 combinations analyzed for this antimicrobial). Furthermore, we observed one, two, or three discordances for all remaining antimicrobials tested except vancomycin and erythromycin, which presented none.
For streptococci, we analyzed 55 isolates with 15 antimicrobials (a total of 570 combinations). The worst phenotype–genotype concordance was observed for macrolide antimicrobials. Of all isolate-macrolide combinations (n = 102), 34.3% (n = 35) corresponded to discordances, mainly due to phenotypic susceptibility with the presence of ARGs (n = 30). Furthermore, we detected eight discordances for tetracycline (15.7% of tetracycline results). These two classes were responsible for 91.5% of all discordances observed in streptococci. The remaining discordances were found in clindamycin (n = 3 or 5.5% of results for clindamycin) and chloramphenicol (n = 1 or 2% of chloramphenicol results). In total, 8.2% (n = 47) of results were discordant.
In enterococci, we analyzed the results of 32 isolates and four antimicrobials, as well as quinupristin/dalfopristin results in nine Enterococcus faecium isolates, with a total of 137 isolate-antimicrobial combinations. Discordances were only observed for quinupristin/dalfopristin (n = 2 or 22.2% of quinupristin/dalfopristin results), with one resistant isolate missing dalfopristin resistance genes and one susceptible (I) isolate harboring quinupristin and dalfopristin resistance genes. Although this percentage is high, in total, only those two results were discordant (1.5% of all results).
Of the remaining Gram-positive bacteria, no discordances were found in the 15 isolate-antimicrobial combinations analyzed for the five isolates of Aerococcus spp. The three isolates of Corynebacterium spp. were analyzed with seven antimicrobials (n = 21 combinations), and we observed six discordances (28.6% of all results). All these discordances corresponded to phenotypically resistant isolates with no known resistance genes, for penicillin (n = 3, 100%), clindamycin (n = 2, 66.6%), and ciprofloxacin (n = 1, 33.3%). Tetracycline, vancomycin, rifampicin, and linezolid results were concordant in this genus.
In Enterobacterales, 243 isolates were analyzed with 12 antimicrobials (except when certain species were intrinsically resistant or lacked breakpoints), yielding a total of 2,839 combinations. In total, 9.6% (n = 273) of results were discordant. Ciprofloxacin concordance was poor, with discordances observed in 28.5% of results for this antimicrobial, which corresponded to 25.3% of all detected discordances. The remaining discordances were mainly observed for the β-lactam antibiotics (n = 187) and together were responsible for 68.5% of all discordances observed in Enterobacterales. In particular, combinations of penicillins with β-lactamase inhibitor results were discordant in 28.8% of cases (n = 70 of 243 results), followed by cephalosporins (12.3% or n = 90 of 729 results) and aztreonam (12.1% or n = 26 of 243 results). These issues were mainly due to phenotypic susceptibility profiles with the presence of ARGs (139 out of 186 discordances in these antimicrobials). However, no discordances were observed for meropenem and only one (0.5%) for ampicillin. Only 17 other cases of discordances were observed for this bacterial group, with no other antimicrobial exceeding 2.5% of discordant results.
Pseudomonas aeruginosa had the highest percentage of discordant results. Concordance was analyzed for 21 isolates with nine antimicrobials (n = 189 combinations), and 44.4% of results showed discordance between phenotype and genotype (n = 84). Several antimicrobials showed over 50% of phenotype–genotype discordance, in particular ceftazidime (100%), cefepime (95.2%), meropenem (61.9%), and ciprofloxacin (52.4%), with genetic determinants present in phenotypically susceptible isolates, and ticarcillin/clavulanic acid (81%) with resistant isolates harboring no known genetic AMR determinants. The remaining β-lactam antibiotics, namely, piperacillin/tazobactam and aztreonam also had poor results, with 4.8% of discordances each. Only amikacin and colistin showed full genotype–phenotype concordance.
Of the 140 combinations analyzed for Haemophilus influenzae (14 isolates with 10 antimicrobials), two (1.4%) were discordant, observed for trimethoprim/sulfamethoxazole. Due to the small number of isolates, these corresponded to 14.3% of the results for this antimicrobial. All other combinations (n = 138) were concordant.
No discordances were found for Moraxella catarrhalis (10 isolates with nine antimicrobials, yielding 90 combinations) nor Stenotrophomonas maltophilia (two isolates with one antimicrobial).
All individual and grouped genotype–phenotype comparison results are available as Supplementary Material.
Discussion
In this study, we analyzed both phenotypic and genotypic AMR profiles of a random subset of clinically relevant bacterial isolates obtained from an original collection containing all isolates processed during one day in all Danish Clinical Microbiology Laboratories, without any a priori selection of bacterial species nor biological sample type.
Only 9% (n = 586) of all phenotypic AST results (n = 6,487) determined by the standard BMD corresponded to phenotypical resistance, which can be considered as a low occurrence of AMR when compared with results observed in similar settings, such as other countries in the European Union (European Centre for Disease Prevention and Control [ECDC], 2020). The AMR profiles observed in this study are mostly in agreement with what has been observed in surveillance results for urine and/or invasive isolates analyzed in the last decade, country-wide (Statens Serum Institut and National Food Institute, 2018, 2019, 2020). Noteworthy observations are that Staphylococcus epidermidis and Streptococcus agalactiae were the species with the highest prevalence of AMR in the Staphylococcus and Streptococcus genera, respectively (Supplementary Tables 2,3). Furthermore, the occurrence of ampicillin resistance in Enterococcus spp. corresponded to 100% of resistance in E. faecium isolates and full susceptibility in Enterococcus faecalis (Supplementary Tables 2,3). In both Enterobacterales and P. aeruginosa, resistance to combinations of β-lactams with β-lactamase inhibitors and to ciprofloxacin was high, and other prevalent resistance phenotypes were detected (Table 3 and Supplementary Tables 2,3).
The main focus of this study was the comparison of BMD AST results with in silico antibiograms obtained through WGS followed by bioinformatics analysis. WGS-based AMR predictions were discordant from BMD results in 8.3% of all species-antimicrobial combinations analyzed, from which 6.2% corresponded to major errors and 2.1% to very major errors. Most of these discordances were restricted to particular species-antimicrobial class combinations, in particular, macrolides and tetracycline in streptococci, penicillins in association with β-lactamase inhibitors, ciprofloxacin in Enterobacterales, and most antimicrobials in P. aeruginosa.
Most of the streptococci-macrolide discordances (n = 30) were due to the presence of the ARG mre(A) in phenotypically susceptible isolates. The gene was also observed in phenotypically resistant isolates but in combination with erm, mef, or msr genes, which are associated with macrolide resistance (Garland et al., 2011; Metcalf et al., 2017). As such, it appears that mre(A) by itself is unable to increase the macrolides’ MIC above resistance thresholds in streptococci, in opposition to what had been previously suggested (Clarebout et al., 2001). Tetracycline resistance genotypic determinants tet(M) and tet(O) were more heterogeneously distributed: of the 21 isolates harboring these ARGs 16 were phenotypically resistant, but five were susceptible. Furthermore, three isolates with tetracycline MIC values in the resistant range presented no known genes associated with resistance, indicating that further studies are necessary to elucidate the correlation between genetic determinants and phenotypes, as well as to discover other genetic mechanisms potentially responsible for tetracycline resistance in streptococci. The poor correlation between the presence or absence of tet genes and phenotypic tetracycline-resistance has been observed in other instances (Stewart et al., 2020).
In Enterobacterales, the discordances observed in piperacillin/tazobactam were equally distributed between the presence of ARGs in phenotypically susceptible isolates (n = 35) and the opposite situation of phenotypic resistance without any known AMR determinants (n = 35). Predicting the activity of these combinations through genomic analyses has proven difficult in the past, as alterations in gene expression, mutations in promoter regions, and number of gene copies, among other factors, can contribute to altered phenotypes while being difficult to detect through these WGS methods (Stoesser et al., 2013; Schechter et al., 2018; Ruppé et al., 2020). Ciprofloxacin discordances were mainly due to the presence, in phenotypically susceptible isolates, of single PMs in gyrase and topoisomerase genes and to the presence of oqx genes. The oqxA and oqxB ARGs increase MIC when compared with values observed in wild-type isolates but are not always responsible for an increase that would lead to a classification of clinical resistance. However, they can lead to such an increase and thus cannot be discarded from in silico antibiograms (Hansen et al., 2007; Wong et al., 2015). Furthermore, it has been previously shown that the number of gyrase and topoisomerase point mutations is correlated with the observed increase in quinolone-MIC values: accumulation of more mutations leads to higher MICs (Vila et al., 1994; Everett et al., 1996; Sáenz et al., 2003; Hopkins et al., 2005). As such, we would suggest that it is necessary to use a different approach for predicting ciprofloxacin resistance than the usual detection of the presence/absence of genotypic determinants. Although the absence of PMs or ARGs is a good predictor of susceptibility, algorithms should consider both the type and number of mutations detected in each isolate, as well as the association with acquired ARGs when attempting to predict phenotypic resistance.
In P. aeruginosa, discordances were abundant and distributed throughout several different antimicrobial classes. Against what should be expected according to previous studies, the presence of β-lactamases from the blaOXA and blaPAO families and of the crpP gene did not correlate to phenotypic resistance to β-lactam drugs and ciprofloxacin, respectively (Rodríguez-Martínez et al., 2009;Chávez-Jacobo et al., 2018; Subedi et al., 2018; Madaha et al., 2020). AMR is often exacerbated or mediated by complex genetic mechanisms leading to overexpression of ampC-encoded β-lactamases and efflux pumps in this species, which confounds genotypic detection of resistance without resorting to, for example, transcriptomics or proteomics analyses (Tam et al., 2007; Lister et al., 2009; Cabot et al., 2011).
We have shown that WGS can potentially be applied as a diagnostic method for AST in clinical microbiology settings. There are clear limitations when considering certain species-antimicrobial combinations where mechanisms related to increased gene expression are involved, but the phenotype–genotype concordance for most isolates processed in the clinical microbiology laboratories was very high. However, as previously pointed out, this study was conducted in a collection presenting relatively low percentages of phenotypic AMR. We observed very major errors in 26.4% of all phenotypically resistant isolate-antimicrobial combinations, but these cases only represented 2.1% of all our results. Thus, phenotype–genotype concordance might be lower in settings with a higher prevalence of AMR; therefore, a future perspective is to employ this methodology of data comparison in bacterial collections with more challenging phenotypes. This approach will elucidate which species-antimicrobial combinations should be further investigated regarding currently unknown genetic determinants of AMR. For these combinations, analyzing large datasets of MIC distributions and comparing those with the genome sequences can reveal new ARGs or PMs of interest, and their contribution to phenotypic resistance profiles can be then confirmed in vitro through, for example, transformation experiments (Hadjirin et al., 2021). This procedure can also be used to confirm if specific genetic determinants that apparently do not confer phenotypic resistance can be removed from bioinformatics databases, despite previous literature suggesting that association. Machine learning approaches can also be employed to identify further genetic candidates associated with AMR (Peiffer-Smadja et al., 2020; Anahtar et al., 2021).
Besides the need to improve our understanding of phenotypic–genotypic correlations for the discordant cases and curate bioinformatics databases according to the state of the art, it is necessary to create an adequate framework that allows for the use of these results in a clinical setting. In this study, the choice of clinical breakpoints referring to “indications other than meningitis” and the inclusion of breakpoints exclusive for uncomplicated urinary tract infection were made to allow the inclusion of larger amounts of data for phenotype and genotype comparison, due to the most common sample sources observed in this collection of bacterial isolates. However, in a clinical setting, the difference in breakpoints cannot be ignored, and data analysis systems must take into account sample sources and other important clinical manifestations of the disease. One potential solution is to associate warning messages to antimicrobial-species combinations for which there is a meningitis breakpoint, when the breakpoint only applies to urinary tract infection or other specific situations. Furthermore, current data are insufficient to effectively predict differences between S and I categories through genotypic characterization, thus systems must also warn users when the susceptibility categories include both S and I ranges (or only I ranges). These details must be confirmed and updated any time the breakpoints are revised, similarly to the current practice of updating the interpretation of phenotypic results. In this study, we have provided the MIC distributions in addition to their interpretation, so it is possible to review phenotype–genotype concordance when breakpoints are revised, or new breakpoints are included.
Implementation of in silico prediction of AMR profiles in clinical settings must be gradual. Laboratories should start with benchmarking their WGS and bioinformatics approaches, by employing the technology in parallel with their currently used diagnostics methods for well-described bacterial species, from uncomplicated infections, and with simple expected phenotypes. Only after optimizing all processes related to WGS, bioinformatics analysis, and data management, should laboratories consider an exclusive WGS-based characterization of isolates (Florian Fricke and Rasko, 2014; Gargis et al., 2016). Depending on the specific local bacterial epidemiology patterns, laboratories can choose to proceed with the inclusion of all isolates processed in their settings, or apply WGS technologies only in selected cases (such as for species included in national surveillance systems, species associated with frequent nosocomial or community outbreaks, or species with emerging epidemiological relevance in neighboring settings).
It is furthermore important to note that a major caveat of performing genotypic predictions of antimicrobial susceptibility profiles is the lack of detection of new resistance determinants. Bioinformatics tools screen for ARGs or chromosomal PMs included in their databases and are thus unable to detect any unknown AMR determinants. Therefore, it is likely that some national laboratories must continue to perform sentinel or routine phenotypic AST so that variations in incidence or prevalence of phenotypic resistance can serve as a warning that new mechanisms of resistance might have been acquired or might be emerging. Together, these limitations make it unlikely that phenotypic methods can be completely replaced by in silico antibiograms. However, the possibility of applying WGS technologies to other steps of the clinical diagnostics pipeline (such as species identification, serotyping, and sequence typing, among others) and for infection control purposes (through phylogenetic or cluster analyses), the constantly decreasing cost of sequencing machines and reagents and the ease of storing, processing, and transferring data make it an attractive option in clinical settings that should be further explored and optimized (Didelot et al., 2012; Deurenberg et al., 2017; Parcell et al., 2021).
Data Availability Statement
The datasets presented in this study can be found in online repositories. The names of the repository/repositories and accession number(s) can be found in the article/Supplementary Material.
Author Contributions
All authors contributed to the conception and design of the study. DH, HN, SE-E, MK, BR, NF-M, TS, JC, CØ, and HW provided the bacterial isolates and the respective metadata, as well as routine clinical diagnostics results. AR performed antimicrobial susceptibility testing through broth microdilution, DNA extraction, whole-genome sequencing, bioinformatics analysis, data analysis, and wrote the first draft of the manuscript. All authors contributed to manuscript revision, read, and approved the submitted version.
Funding
This project was supported by the Novo Nordisk Foundation (NNF16OC0021856) – Global Surveillance of Antimicrobial Resistance and the H2020 project VEO (grant number 874735).
Conflict of Interest
The authors declare that the research was conducted in the absence of any commercial or financial relationships that could be construed as a potential conflict of interest.
The reviewer PD declared a shared parent affiliation with the HW to the handling editor at the time of review.
Publisher’s Note
All claims expressed in this article are solely those of the authors and do not necessarily represent those of their affiliated organizations, or those of the publisher, the editors and the reviewers. Any product that may be evaluated in this article, or claim that may be made by its manufacturer, is not guaranteed or endorsed by the publisher.
Acknowledgments
We thank Marie Stengaard Jensen for her assistance in collecting and organizing the samples. We also thank Birthe S. Rosenqvist Lund, Christina Aaby Svendsen, Gunhild Larsen, Hanne Mordhorst, Hanne Nørgaard Nielsen, Inge Marianne Hansen, Jacob Dyring Jensen, and Kate Vina Vibefeldt for their help with all laboratory work included in this project. Finally, we thank the staff of the Danish Departments of Clinical Microbiology for enabling the project.
Supplementary Material
The Supplementary Material for this article can be found online at: https://www.frontiersin.org/articles/10.3389/fmicb.2022.804627/full#supplementary-material
Footnotes
- ^ https://bitbucket.org/genomicepidemiology/foodqcpipeline/src/master/
- ^ https://cge.food.dtu.dk/services/KmerFinder/
- ^ https://pubmlst.org/rmlst/
- ^ https://cge.food.dtu.dk/services/ResFinder/
- ^ https://www.computerome.dk
- ^ http://www.ebi.ac.uk/ena
References
Aanensen, D. M., Feil, E. J., Holden, M. T. G., Dordel, J., Yeats, C. A., Fedosejev, A., et al. (2016). Whole-Genome sequencing for routine pathogen surveillance in public health: a population snapshot of invasive Staphylococcus aureus in Europe. mBio 7:e00444-16. doi: 10.1128/mBio.00444-416
Anahtar, M. N., Yang, J. H., and Kanjilal, S. (2021). Applications of machine learning to the problem of antimicrobial resistance: an emerging model for translational research. J. Clin. Microbiol. 59:e0126020. doi: 10.1128/JCM.01260-1220
Benkova, M., Soukup, O., and Marek, J. (2020). Antimicrobial susceptibility testing: currently used methods and devices and the near future in clinical practice. J. Appl. Microbiol. 129, 806–822. doi: 10.1111/jam.14704
Bortolaia, V., Kaas, R. S., Ruppe, E., Roberts, M. C., Schwarz, S., Cattoir, V., et al. (2020). ResFinder 4.0 for predictions of phenotypes from genotypes. J. Antimicrob. Chemother. 75, 3491–3500. doi: 10.1093/jac/dkaa345
Cabot, G., Ocampo-Sosa, A. A., Tubau, F., Macia, M. D., Rodríguez, C., Moya, B., et al. (2011). Overexpression of AmpC and efflux pumps in Pseudomonas aeruginosa isolates from bloodstream infections: prevalence and impact on resistance in a Spanish multicenter study. Antimicrob. Agents Chemother. 55, 1906–1911. doi: 10.1128/AAC.01645-1610
Cassini, A., Högberg, L. D., Plachouras, D., Quattrocchi, A., Hoxha, A., Simonsen, G. S., et al. (2019). Attributable deaths and disability-adjusted life-years caused by infections with antibiotic-resistant bacteria in the EU and the European Economic Area in 2015: a population-level modelling analysis. Lancet Infect. Dis. 19, 56–66. doi: 10.1016/S1473-3099(18)30605-30604
Chávez-Jacobo, V. M., Hernández-Ramírez, K. C., Romo-Rodríguez, P., Pérez-Gallardo, R. V., Campos-García, J., Gutiérrez-Corona, J. F., et al. (2018). CrpP is a novel ciprofloxacin-modifying enzyme encoded by the Pseudomonas aeruginosa pUM505 Plasmid. Antimicrob. Agents Chemother. 62:e02629-17. doi: 10.1128/AAC.02629-2617
Clarebout, G., Villers, C., and Leclercq, R. (2001). Macrolide resistance gene mreA of Streptococcus agalactiae encodes a flavokinase. Antimicrob. Agents Chemother. 45, 2280–2286. doi: 10.1128/AAC.45.8.2280-2286.2001
Clausen, P. T. L. C., Aarestrup, F. M., and Lund, O. (2018). Rapid and precise alignment of raw reads against redundant databases with KMA. BMC Bioinformatics 19:307. doi: 10.1186/s12859-018-2336-2336
Clausen, P. T. L. C., Zankari, E., Aarestrup, F. M., and Lund, O. (2016). Benchmarking of methods for identification of antimicrobial resistance genes in bacterial whole genome data. J. Antimicrob. Chemother. 71, 2484–2488. doi: 10.1093/jac/dkw184
Clinical and Laboratory Standards Institute (2012). M11-A8: Methods for Antimicrobial Susceptibility Testing of Anaerobic Bacteria; Approved Standard 8th Edn. Wayne, PA: CLSI.
Cortes-Lara, S., Barrio-Tofiño, E., del, López-Causapé, C., Oliver, A., Martínez-Martínez, L., Bou, G., et al. (2021). Predicting Pseudomonas aeruginosa susceptibility phenotypes from whole genome sequence resistome analysis. Clin. Microbiol. Infect. 27, 1631–1637. doi: 10.1016/j.cmi.2021.05.011
Deurenberg, R. H., Bathoorn, E., Chlebowicz, M. A., Couto, N., Ferdous, M., García-Cobos, S., et al. (2017). Application of next generation sequencing in clinical microbiology and infection prevention. J. Biotechnol. 243, 16–24. doi: 10.1016/j.jbiotec.2016.12.022
Didelot, X., Bowden, R., Wilson, D. J., Peto, T. E. A., and Crook, D. W. (2012). Transforming clinical microbiology with bacterial genome sequencing. Nat. Rev. Genet. 13, 601–612. doi: 10.1038/nrg3226
Do Nascimento, V., Day, M. R., Doumith, M., Hopkins, K. L., Woodford, N., Godbole, G., et al. (2017). Comparison of phenotypic and WGS-derived antimicrobial resistance profiles of enteroaggregative Escherichia coli isolated from cases of diarrhoeal disease in England, 2015-16. J. Antimicrob. Chemother. 72, 3288–3297. doi: 10.1093/jac/dkx301
Ellington, M. J., Ekelund, O., Aarestrup, F. M., Canton, R., Doumith, M., Giske, C., et al. (2017). The role of whole genome sequencing in antimicrobial susceptibility testing of bacteria: report from the EUCAST Subcommittee. Clin. Microbiol. Infect. 23, 2–22. doi: 10.1016/j.cmi.2016.11.012
European Centre for Disease Prevention and Control [ECDC] (2020). Surveillance Report. Antimicrobial Resistance in the EU/EEA (EARS-Net) – Annual Epidemiological. Sweden: ECDC. (Report)for 2019.
European Committee on Antimicrobial Susceptibility Testing (2017). Media Preparation for EUCAST Disk Diffusion Testing and for Determination of MIC Values by the Broth Microdilution Method, v5.0.2-6. Sweden: European Committee on Antimicrobial Susceptibility Testing.
European Committee on Antimicrobial Susceptibility Testing (2021). EUCAST Communication to Clinical Colleagues: On Recent Changes in Clinical Microbiology Susceptibility Reports – New Interpretation of Susceptibility Categories S, I and R. 1-3. Sweden: European Committee on Antimicrobial Susceptibility Testing.
Everett, M. J., Jin, Y. F., Ricci, V., and Piddock, L. J. V. (1996). Contributions of individual mechanisms to fluoroquinolone resistance in 36 Escherichia coli strains isolated from humans and animals. Antimicrob. Agents Chemother. 40, 2380–2386. doi: 10.1128/aac.40.10.2380
Florian Fricke, W., and Rasko, D. A. (2014). Bacterial genome sequencing in the clinic: bioinformatic challenges and solutions. Nat. Rev. Genet. 15, 49–55. doi: 10.1038/nrg3624
Galata, V., Laczny, C. C., Backes, C., Hemmrich-Stanisak, G., Schmolke, S., Franke, A., et al. (2019). Integrating culture-based antibiotic resistance profiles with whole-genome sequencing data for 11,087 clinical isolates. Genomics Proteomics Bioinforma 17, 169–182. doi: 10.1016/j.gpb.2018.11.002
Gargis, A. S., Kalman, L., and Lubin, I. M. (2016). Assuring the quality of next-generation sequencing in clinical microbiology and public health laboratories. J. Clin. Microbiol. 54, 2857–2865. doi: 10.1128/JCM.00949-916
Garland, S. M., Cottrill, E., Markowski, L., Pearce, C., Clifford, V., Ndisang, D., et al. (2011). Antimicrobial resistance in group B streptococcus: the Australian experience. J. Med. Microbiol. 60, 230–235. doi: 10.1099/jmm.0.022616-0
Hadjirin, N. F., Miller, E. L., Murray, G. G. R., Yen, P. L. K., Phuc, H. D., Wileman, T. M., et al. (2021). Large-scale genomic analysis of antimicrobial resistance in the zoonotic pathogen Streptococcus suis. BMC Biol. 19:191. doi: 10.1186/s12915-021-01094-1091
Hammerum, A. M., Baig, S., Kamel, Y., Roer, L., Pinholt, M., Gumpert, H., et al. (2017). Emergence of vanA Enterococcus faecium in Denmark, 2005-15. J. Antimicrob. Chemother. 72, 2184–2190. doi: 10.1093/jac/dkx138
Hansen, L. H., Jensen, L. B., Sørensen, H. I., and Sørensen, S. J. (2007). Substrate specificity of the OqxAB multidrug resistance pump in Escherichia coli and selected enteric bacteria. J. Antimicrob. Chemother. 60, 145–147. doi: 10.1093/jac/dkm167
Hasman, H., Saputra, D., Sicheritz-Ponten, T., Lund, O., Svendsen, C. A., Frimodt-Moller, N., et al. (2014). Rapid whole-genome sequencing for detection and characterization of microorganisms directly from clinical samples. J. Clin. Microbiol. 52, 139–146. doi: 10.1128/JCM.02452-2413
Hopkins, K. L., Davies, R. H., and Threlfall, E. J. (2005). Mechanisms of quinolone resistance in Escherichia coli and Salmonella: recent developments. Int. J. Antimicrob. Agents 25, 358–373. doi: 10.1016/j.ijantimicag.2005.02.006
International Organization for Standardization (2006). ISO 20776-1:2006 Clinical Laboratory Testing and in Vitro Diagnostic Test Systems – Susceptibility Testing of Infectious Agents and Evaluation of Performance of Antimicrobial Susceptibility Test Devices – Part 1: Reference Method for Testing the in Vitro Activity of Antimicrobial Agents Against Rapidly Growing Aerobic Bacteria Involved in Infectious Diseases. Geneva: International Organization for Standardization.
International Organization for Standardization (2019). ISO 20776-1:2019 – Susceptibility Testing of Infectious Agents and Evaluation of Performance of Antimicrobial Susceptibility Test Devices – Part 1: Broth Micro-dilution Reference Method for Testing the in Vitro Activity of Antimicrobial Agents Against Rapidly Growing Aerobic Bacteria Involved in Infectious Diseases. Geneva: International Organization for Standardization.
Jean, S.-S., Liao, C.-H., Sheng, W.-H., Lee, W.-S., and Hsueh, P.-R. (2017). Comparison of commonly used antimicrobial susceptibility testing methods for evaluating susceptibilities of clinical isolates of Enterobacteriaceae and nonfermentative Gram-negative bacilli to cefoperazone-sulbactam. J. Microbiol. Immunol. Infect. 50, 454–463. doi: 10.1016/j.jmii.2015.08.024
Jolley, K. A., Bliss, C. M., Bennett, J. S., Bratcher, H. B., Brehony, C., Colles, F. M., et al. (2012). Ribosomal multilocus sequence typing: universal characterization of bacteria from domain to strain. Microbiology 158, 1005–1015. doi: 10.1099/mic.0.055459-55450
Jorgensen, J. H., and Ferraro, M. J. (2009). Antimicrobial susceptibility testing: a review of general principles and contemporary practices. Clin. Infect. Dis. 49, 1749–1755. doi: 10.1086/647952
Kos, V. N., Déraspe, M., McLaughlin, R. E., Whiteaker, J. D., Roy, P. H., Alm, R. A., et al. (2015). The resistome of Pseudomonas aeruginosa in relationship to phenotypic susceptibility. Antimicrob. Agents Chemother. 59, 427–436. doi: 10.1128/AAC.03954-3914
Larsen, M. V., Cosentino, S., Lukjancenko, O., Saputra, D., Rasmussen, S., Hasman, H., et al. (2014). Benchmarking of methods for genomic taxonomy. J. Clin. Microbiol. 52, 1529–1539. doi: 10.1128/JCM.02981-2913
Lister, P. D., Wolter, D. J., and Hanson, N. D. (2009). Antibacterial-Resistant Pseudomonas aeruginosa: clinical impact and complex regulation of chromosomally encoded resistance mechanisms. Clin. Microbiol. Rev. 22, 582–610. doi: 10.1128/CMR.00040-49
Long, S. W., Williams, D., Valson, C., Cantu, C. C., Cernoch, P., Musser, J. M., et al. (2013). A genomic day in the life of a clinical microbiology laboratory. J. Clin. Microbiol. 51, 1272–1277. doi: 10.1128/JCM.03237-3212
Madaha, E. L., Mienie, C., Gonsu, H. K., Bughe, R. N., Fonkoua, M. C., Mbacham, W. F., et al. (2020). Whole-genome sequence of multi-drug resistant Pseudomonas aeruginosa strains UY1PSABAL and UY1PSABAL2 isolated from human broncho-alveolar lavage. Yaoundé, Cameroon. PLoS One 15:e0238390. doi: 10.1371/journal.pone.0238390
Mason, A., Foster, D., Bradley, P., Golubchik, T., Doumith, M., Gordon, N. C., et al. (2018). Accuracy of different bioinformatics methods in detecting antibiotic resistance and virulence factors from staphylococcus aureus whole-genome sequences. J. Clin. Microbiol. 56, e1815–e1817. doi: 10.1128/JCM.01815-1817
Matuschek, E., Åhman, J., Webster, C., and Kahlmeter, G. (2018). Antimicrobial susceptibility testing of colistin – evaluation of seven commercial MIC products against standard broth microdilution for Escherichia coli, Klebsiella pneumoniae, Pseudomonas aeruginosa, and Acinetobacter spp. Clin. Microbiol. Infect. 24, 865–870. doi: 10.1016/j.cmi.2017.11.020
Metcalf, B. J., Chochua, S., Gertz, R. E., Hawkins, P. A., Ricaldi, J., Li, Z., et al. (2017). Short-read whole genome sequencing for determination of antimicrobial resistance mechanisms and capsular serotypes of current invasive Streptococcus agalactiae recovered in the USA. Clin. Microbiol. Infect 23, 574.e7–574.e14. doi: 10.1016/j.cmi.2017.02.021.
Metcalf, B. J., Chochua, S., Gertz, R. E., Li, Z., Walker, H., Tran, T., et al. (2016). Using whole genome sequencing to identify resistance determinants and predict antimicrobial resistance phenotypes for year 2015 invasive pneumococcal disease isolates recovered in the United States. Clin. Microbiol. Infect. 22, 1002.e1–1002.e8. doi: 10.1016/j.cmi.2016.08.001.
Mojica, M. F., De La Cadena, E., Hernández-Gómez, C., Correa, A., Appel, T. M., Pallares, C. J., et al. (2020). Performance of disk diffusion and broth microdilution for fosfomycin susceptibility testing of multidrug-resistant clinical isolates of Enterobacterales and Pseudomonas aeruginosa. J. Glob. Antimicrob. Resist. 21, 391–395. doi: 10.1016/j.jgar.2020.01.003
Parcell, B. J., Gillespie, S. H., Pettigrew, K. A., and Holden, M. T. G. (2021). Clinical perspectives in integrating whole-genome sequencing into the investigation of healthcare and public health outbreaks – hype or help? J. Hosp. Infect. 109, 1–9. doi: 10.1016/j.jhin.2020.11.001
Pecora, N. D., Li, N., Allard, M., Li, C., Albano, E., Delaney, M., et al. (2015). Genomically informed surveillance for carbapenem-resistant Enterobacteriaceae in a Health Care System. mBio 6:e01030-15. doi: 10.1128/mBio.01030-1015
Peiffer-Smadja, N., Dellière, S., Rodriguez, C., Birgand, G., Lescure, F. X., Fourati, S., et al. (2020). Machine learning in the clinical microbiology laboratory: has the time come for routine practice? Clin. Microbiol. Infect. 26, 1300–1309. doi: 10.1016/j.cmi.2020.02.006
Rebelo, A. R., Ibfelt, T., Bortolaia, V., Leekitcharoenphon, P., Hansen, D. S., Nielsen, H. L., et al. (2022). One Day in Denmark: nationwide point-prevalence survey of human bacterial isolates and comparison of classical and whole-genome sequence-based species identification methods. PLoS One 17:e0261999. doi: 10.1371/journal.pone.0261999
Roach, D. J., Burton, J. N., Lee, C., Stackhouse, B., Butler-Wu, S. M., Cookson, B. T., et al. (2015). A year of infection in the intensive care unit: prospective whole genome sequencing of bacterial clinical isolates reveals cryptic transmissions and novel microbiota. PLoS Genet 11:e1005413. doi: 10.1371/journal.pgen.1005413
Rodríguez-Martínez, J. M., Poirel, L., and Nordmann, P. (2009). Extended-spectrum cephalosporinases in Pseudomonas aeruginosa. Antimicrob. Agents Chemother. 53, 1766–1771. doi: 10.1128/AAC.01410-1418
Ruppé, E., Cherkaoui, A., Charretier, Y., Girard, M., Schicklin, S., Lazarevic, V., et al. (2020). From genotype to antibiotic susceptibility phenotype in the order Enterobacterales: a clinical perspective. Clin. Microbiol. Infect. 26, 643.e1–643.e7. doi: 10.1016/j.cmi.2019.09.018.
Sáenz, Y., Zarazaga, M., Briñas, L., Ruiz-Larrea, F., and Torres, C. (2003). Mutations in gyrA and parC genes in nalidixic acid-resistant Escherichia coli strains from food products, humans and animals. J. Antimicrob. Chemother. 51, 1001–1005. doi: 10.1093/jac/dkg168
Schechter, L. M., Creely, D. P., Garner, C. D., Shortridge, D., Nguyen, H., Chen, L., et al. (2018). Extensive gene amplification as a mechanism for piperacillin-tazobactam resistance in Escherichia coli. mBio 9:e00583-18. doi: 10.1128/mBio.00583-518
Shelburne, S. A., Kim, J., Munita, J. M., Sahasrabhojane, P., Shields, R. K., Press, E. G., et al. (2017). Whole-Genome sequencing accurately identifies resistance to extended-spectrum β-Lactams for major gram-negative bacterial pathogens. Clin. Infect. Dis. 65, 738–745. doi: 10.1093/cid/cix417
Statens Serum Institut and National Food Institute (2018). Danmap 2018. Denmark: Statens Serum Institut and National Food Institute.
Statens Serum Institut and National Food Institute (2019). Danmap 2019. Denmark: Statens Serum Institut and National Food Institute.
Statens Serum Institut and National Food Institute (2020). Danmap 2020. Denmark: Statens Serum Institut and National Food Institute.
Stewart, A. G., Burnard, D., Sowden, D., and McMillan, D. (2020). Whole genome sequencing for antimicrobial resistance mechanisms, virulence factors and clonality in invasive Streptococcus agalactiae blood culture isolates recovered in Australia. Pathology 52, 694–699. doi: 10.1016/j.pathol.2020.06.006
Stoesser, N., Batty, E. M., Eyre, D. W., Morgan, M., Wyllie, D. H., and Del Ojo, et al. (2013). Predicting antimicrobial susceptibilities for Escherichia coli and Klebsiella pneumoniae isolates using whole genomic sequence data. J. Antimicrob. Chemother. 68, 2234–2244. doi: 10.1093/jac/dkt180
Su, M., Satola, S. W., and Read, T. D. (2018). Genome-based prediction of bacterial antibiotic resistance. J. Clin. Microbiol. 57, e1405–e1418. doi: 10.1128/JCM.01405-1418
Subedi, D., Vijay, A. K., Kohli, G. S., Rice, S. A., and Willcox, M. (2018). Comparative genomics of clinical strains of Pseudomonas aeruginosa strains isolated from different geographic sites. Sci. Rep. 8:15668. doi: 10.1038/s41598-018-34020-34027
Tagini, F., and Greub, G. (2017). Bacterial genome sequencing in clinical microbiology: a pathogen-oriented review. Eur. J. Clin. Microbiol. Infect. Dis. 36, 2007–2020. doi: 10.1007/s10096-017-3024-3026
Tam, V. H., Schilling, A. N., LaRocco, M. T., Gentry, L. O., Lolans, K., Quinn, J. P., et al. (2007). Prevalence of AmpC over-expression in bloodstream isolates of Pseudomonas aeruginosa. Clin. Microbiol. Infect. 13, 413–418. doi: 10.1111/j.1469-0691.2006.01674.x
Vila, J., Ruiz, J., Marco, F., Barcelo, A., Goni, P., Giralt, E., et al. (1994). Association between double mutation in gyrA gene of ciprofloxacin-resistant clinical isolates of Escherichia coli and MICs. Antimicrob. Agents Chemother. 38, 2477–2479. doi: 10.1128/AAC.38.10.2477
Wiegand, I., Hilpert, K., and Hancock, R. E. W. (2008). Agar and broth dilution methods to determine the minimal inhibitory concentration (MIC) of antimicrobial substances. Nat. Protoc. 3, 163–175. doi: 10.1038/nprot.2007.521
Wong, M. H. Y., Chan, E. W. C., and Chen, S. (2015). Evolution and dissemination of OqxAB-like efflux pumps, an emerging quinolone resistance determinant among members of Enterobacteriaceae. Antimicrob. Agents Chemother. 59, 3290–3297. doi: 10.1128/AAC.00310-315
Keywords: whole-genome sequencing (WGS), antimicrobial resistance (AMR), antimicrobial resistance genes (ARGs), genotype, phenotype, concordance, in silico antibiogram
Citation: Rebelo AR, Bortolaia V, Leekitcharoenphon P, Hansen DS, Nielsen HL, Ellermann-Eriksen S, Kemp M, Røder BL, Frimodt-Møller N, Søndergaard TS, Coia JE, Østergaard C, Westh H and Aarestrup FM (2022) One Day in Denmark: Comparison of Phenotypic and Genotypic Antimicrobial Susceptibility Testing in Bacterial Isolates From Clinical Settings. Front. Microbiol. 13:804627. doi: 10.3389/fmicb.2022.804627
Received: 29 October 2021; Accepted: 10 May 2022;
Published: 10 June 2022.
Edited by:
Jesus L. Romalde, University of Santiago de Compostela, SpainReviewed by:
Ben Pascoe, University of Bath, United KingdomPeter Damborg, University of Copenhagen, Denmark
Copyright © 2022 Rebelo, Bortolaia, Leekitcharoenphon, Hansen, Nielsen, Ellermann-Eriksen, Kemp, Røder, Frimodt-Møller, Søndergaard, Coia, Østergaard, Westh and Aarestrup. This is an open-access article distributed under the terms of the Creative Commons Attribution License (CC BY). The use, distribution or reproduction in other forums is permitted, provided the original author(s) and the copyright owner(s) are credited and that the original publication in this journal is cited, in accordance with accepted academic practice. No use, distribution or reproduction is permitted which does not comply with these terms.
*Correspondence: Ana Rita Rebelo, YW5yaXJlQGZvb2QuZHR1LmRr