- 1Department of Biology, University of Mississippi, Oxford, MS, United States
- 2Department of Biological Sciences, University of Alabama, Tuscaloosa, AL, United States
The Asian clam Corbicula fluminea (Family: Cyneridae) has aggressively invaded freshwater habitats worldwide, resulting in dramatic ecological changes and declines of native bivalves such as freshwater mussels (Family: Unionidae), one of the most imperiled faunal groups. Despite increases in our knowledge of invasive C. fluminea biology, little is known of how intrinsic and extrinsic factors, including co-occurring native species, influence its microbiome. We investigated the gut bacterial microbiome across genetically differentiated populations of C. fluminea in the Tennessee and Mobile River Basins in the Southeastern United States and compared them to those of six co-occurring species of native freshwater mussels. The gut microbiome of C. fluminea was diverse, differed with environmental conditions and varied spatially among rivers, but was unrelated to host genetic variation. Microbial source tracking suggested that the gut microbiome of C. fluminea may be influenced by the presence of co-occurring native mussels. Inferred functions from 16S rRNA gene data using PICRUST2 predicted a high prevalence and diversity of degradation functions in the C. fluminea microbiome, especially the degradation of carbohydrates and aromatic compounds. Such modularity and functional diversity of the microbiome of C. fluminea may be an asset, allowing to acclimate to an extensive range of nutritional sources in invaded habitats, which could play a vital role in its invasive success.
Introduction
The introduction of species outside of their native range through human activities is an accelerating phenomenon worldwide (Seebens et al., 2017). Invasive species, spreading aggressively after introduction, have important negative ecological consequences on the ecosystem they invade and are the second leading cause of species endangerment and extinction (Bellard et al., 2016). As with other organisms, invasive species live and interact with a diverse community of microorganisms, their microbiome, which is an integral part of their biology and ecology (Bahrndorff et al., 2016). While the composition and function of microbiomes have been examined for many host species, they have rarely been assessed in an invasion context (but see, e.g., Goddard-Dwyer et al., 2021).
The influence of the microbiome on invasive species success, while increasingly recognized in plants (Kowalski et al., 2015), is still largely unknown in animals (Bahrndorff et al., 2016). This is striking given that numerous hypotheses for the success or failure of the invasion process involve mutualistic or antagonistic partners of invasive and native species, which can include microbes (Lockwood et al., 2013a,b). It has been suggested that a higher variability or diversity of functions displayed by the gut microbiome may benefit a host’s capacity to adapt to a more extensive range of nutritional niches, therefore facilitating its establishment in invaded areas (Bahrndorff et al., 2016; Goddard-Dwyer et al., 2021). The microorganisms transported by an invasive species may also pose a risk to native populations through the transmission of pathogens (Lymbery et al., 2014), or through the modification of environmental microbial communities that may be less beneficial to the native hosts (Coats and Rumpho, 2014).
As with any organism, microbiomes associated with invasive species can be driven by both host-specific (e.g., host condition, life history, genetics) and environmental factors (e.g., water physichochemistry, diet) (Chiarello et al., 2019). Additionally, while invasive species may carry microorganisms that are co-introduced from their native range, they may also lose much of their microbiome during an invasion and thus may acquire novel microbes locally (Parker et al., 2006). This makes the invasion history, such as the number of distinct introductions and subsequent patterns of spatial expansion, important when assessing the microbiome diversity of invasive species (Parker et al., 2020). Interactions with local native communities may be particularly important when the invasive species occupies the same functional role as co-occurring native fauna, as similar niche requirements should increase encounters between native and invasive species, therefore increasing the potential for microbe acquisition (Parker et al., 2006; Shelby et al., 2016). Thus, investigating the microbiome of established invasive populations across ecological gradients provides an opportunity to understand the relative influence of host-specific and environmental influences in shaping the microbiome of invasive species during the invasion process.
Invasive species in freshwater habitats are of particular concern, as these ecosystems are among the most diverse and vulnerable on the planet and have exhibited the most dramatic decreases in biological diversity since the 1970s (Darwall et al., 2018; Lopes-Lima et al., 2018; Albert et al., 2021). Asian clams in the genus Corbicula are among the most problematic invasive freshwater species, having spread rapidly from their native range in Eastern Asia, Australia, and Eastern Africa, to a worldwide distribution in just a few decades (Lee et al., 2005; Sousa et al., 2008). The exact number of species within the genus Corbicula is still unclear, but most invasive lineages are classified as Corbicula fluminea or fluminalis (Sousa et al., 2008). The United States populations are referred to as Corbicula fluminea (National Invasive Species Information Center1, February 2021), but belong to five genetically distinct morphotypes (Haponski and Foighil, 2019). Corbicula fluminea reproduces androgenetically, where the nuclear DNA of juveniles is derived entirely from the male parent (Ishibashi et al., 2003), and clonal reproduction of introduced lineages has contributed to their rapid expansions (Lee et al., 2005; Haponski and Foighil, 2019).
Corbicula fluminea invasions are especially concerning because of their potential impact on native bivalve populations, particularly mussels in the family Unionidae (Sousa et al., 2008; Haag, 2019). Freshwater mussels play important roles in ecosystems through their filter-feeding (Vaughn and Hoellein, 2018) and are among the most threatened faunal groups worldwide, with 45% of the described species being threatened, endangered, or extinct (Lopes-Lima et al., 2014a,2018). Freshwater mussels are typically slow growing and long-lived (most living ∼6-50 years, Haag, 2012) and often occur in dense multi-species aggregations, yet both their diversity and abundance have been declining dramatically (Haag, 2019). C. fluminea occupies the same functional guild as unionids (i.e., filter-feeding bivalves) but shows a faster growth rate and shorter life span (1-5 years) that, together with earlier sexual maturity and clonal reproduction, allows it to reproduce and disperse more rapidly than native mussels and to recover more quickly following perturbations (McMahon, 2002; Sousa et al., 2008; Ferreira-Rodríguez et al., 2018b). Increasing densities of C. fluminea may thus replace unionid populations in the wild (McMahon, 2002; Ferreira-Rodríguez et al., 2018b) and pose a risk to remaining populations (Sousa et al., 2008; Haag, 2019). While field assessments in Western Europe and North America document variable spatial overlap between invasive C. fluminea and native mussel populations (Vaughn and Spooner, 2006; Ferreira-Rodríguez et al., 2018a), experiments have revealed the negative impact of C. fluminea on mussel growth and physiology, with the most likely cause being competition for space and/or resources, as C. fluminea typically displays higher individual filtration rates than native mussels (Ferreira-Rodríguez et al., 2018a,b; Haag, 2019).
Despite these possible threats to native mussels, the microbiome of C. fluminea and its relationship to native mussel microbiomes have never been examined. The unionid microbiome has been increasingly studied over the past few years, suggesting some degree of species-specificity, where mussel species collected from the same environment have distinct microbiomes (Weingarten et al., 2019; Liu et al., 2020; McCauley et al., 2021). However, while opportunistic bacteria are detected within moribund mussels or during mass mortality events, little is known about potential bacterial pathogens of mussel populations in the wild (Grizzle and Brunner, 2009; Leis et al., 2019; McElwain, 2019; Richard et al., 2021), or the risk of a transmission of such pathogens from exotic species such as C. fluminea. Whether C. fluminea likewise harbors its own distinctive microbiome, influences the microbiome of native freshwater mussels, or has acquired a microbial community that reflects that of the local environment and/or one of the native mussels remains unknown. Further, the unique invasion history and reproductive mode of C. fluminea clonal lineages may influence microbiome diversity and transmission within and among populations. Thus, understanding the distribution of different clonal lineages or fine-scale population genetic structure could provide additional clues into the respective roles of host-specific and environmental influences. Given the potential negative impacts on native mussel communities, investigating the diverse factors that may contribute to C. fluminea microbiome diversity alongside native mussel microbiome will help better elucidate possible impacts on freshwater mussel communities.
We report the first investigation into the microbiome of C. fluminea populations that co-occur along a gradient of native freshwater mussel assemblage density in the Mobile and Tennessee River Basins (Figure 1; Hopper et al., 2021). We describe the diversity and inferred function of the microbiome of C. fluminea and assess the main differences compared to that of co-occurring native mussels. By integrating population genomic data, we examine the genetic structure of C. fluminea in the Mobile and Tennessee Basins and test whether ancestry contributes to microbiome diversity, either from clonal lineage or within-lineage genetic variation. Lastly, we examine if the presence and/or higher densities of C. fluminea may lead to changes in the microbiome of co-occurring native mussels and assess the potential reciprocal influence of the native mussel microbiome on that of C. fluminea. By examining how spatial-environmental variation, population genetic structure, and co-occurring mussel communities interact to influence microbiome composition, we provide insights into host-specific and environmental factors driving the microbiome of established invasive freshwater species.
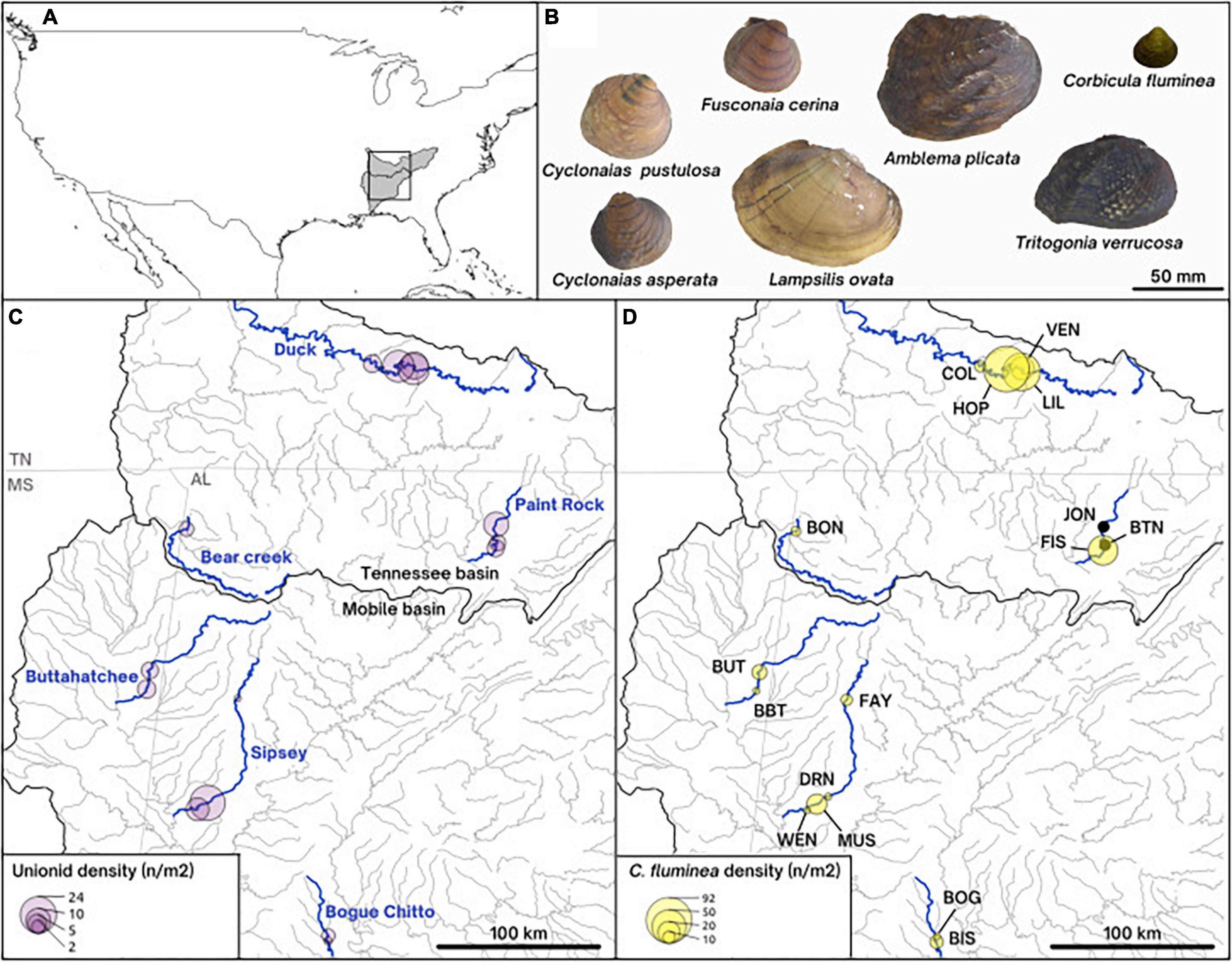
Figure 1. Map of the collecting sites (A,C,D) and pictures of the shells (B) of the species studied. (A) Overall map of the United States of America, showing the Mobile and the Tennessee river basins shaded in gray. (C) Density of unionids on our collecting sites along the six rivers of study. (D) Density of the invasive Asian clam Corbicula fluminea on collecting sites and site names represented by three letters. (B) On-scale pictures of representative shells of the native unionid species included in this paper (Lampsilis ovata, Cyclonaias pustulosa, Cyclonaias asperata, Fusconaia cerina, Tritogonia verrucosa, and Amblema plicata) and the invasive Corbicula fluminea.
Materials and Methods
Study Area
Samples were collected from six rivers in the Mobile and Tennessee River Basins, in the southeastern United States (Figure 1). The southeastern United States is a hotspot for freshwater mussel biodiversity, containing ∼90% of the North American diversity (Parmalee and Bogan, 1998; Williams et al., 2008). This extraordinarily diverse region has been severely degraded by anthropogenic activity, such that 95% of the 70 United States federally protected mussel species occur in this region (Williams et al., 2008). Although C. fluminea has been considered established in the southeastern United States for more than 50 years (US Geological Survey, 2021), complete accounts of C. fluminea invasion timing and quantitative population estimates are rarely available where mussels are found (Benson and Williams, 2021). On our 16 study sites (1-4 per river), C. fluminea density ranged from low to very high (averaging 0.5-92 individuals/m2) and was generally correlated to native mussel densities, which ranged from 0.6 to 23 individuals/m2 (as reported by Hopper et al., 2021; Figure 1).
Specimen Collection and Environmental Measures
We collected 180 specimens of C. fluminea and 144 specimens of Unionidae belonging to six species (Lampsilis ovata, Cyclonaias pustulosa, Cyclonaias asperata, Fusconaia cerina, Tritogonia verrucosa, and Amblema plicata) between July and September 2019 (Figure 1 and S1-Supplementary Table 1). We collected 3-28 individuals of C. fluminea per site, along with up to 10 individuals each from up to three native mussel species. Mussel species were identified morphologically by author C.L.A.
All specimens were placed on ice and transported back to the University of Alabama on the same day of collection, where they were flash-frozen and stored at −80°C. The entire gastrointestinal tracts of mussels and C. fluminea were subsequently excised using sterile dissecting equipment and transported on dry ice to the University of Mississippi for microbiome analysis. For population genomic analysis, C. fluminea mantle tissue was clipped and stored in molecular biology grade absolute ethanol at −80°C.
Three surface sediment and three 120-mL water samples were collected from each site at the time of sampling. Water samples were filtered through sterile 1 μm pore size glassfiber filters (25 mm diameter; Millipore) placed in sterile tubes. Water temperature, pH, conductivity, and dissolved oxygen were measured at each site using aYSI DO Probe (YSI Inc., Yellow Springs, OH, United States), and 50 mL water samples were collected to determine concentrations of dissolved organic carbon (DOC), Soluble Reactive Phosphorus (SRP), soluble ammonium (NH4+), soluble nitrite (NO2−), soluble nitrate (NO3−). Sediment granulometry was also determined for each sample site. Values of these parameters and methodology are described in S1-Supplementary Table 2.
Microbial DNA Extraction, 16S rRNA Gene Sequencing, and Sequence Processing
A subset of 3-8 C. fluminea specimens per site was selected for microbiome analysis for a total sample size of 80 C. fluminea and 144 native mussels (S1-Supplementary Table 2). Bivalve gut tissue was ground using sterile pellet pestles with the extraction buffer from a PowerSoil Pro kit (Qiagen, Germantown, MD), and bacterial DNA was extracted as described previously (McCauley et al., 2021). DNA from sediment and filtered water (seston) samples were extracted following PowerSoil Pro kit instructions. Dual-indexed barcoded primers were used to amplify the V4 region of the 16S rRNA gene of the extracted DNA from each sample following established techniques (Kozich et al., 2013; McCauley et al., 2021). This hypervariable region was chosen following previous work on unionid microbiome using Illumina MiSeq technology (Weingarten et al., 2019; Aceves et al., 2020; Richard et al., 2021), and recommendations from the Earth Microbiome Project2 (01/2022). The amplified 16S rRNA gene fragments were combined and spiked with 20% PhiX before being sequenced on an Illumina MiSeq at the University of Mississippi Medical Center Molecular and Genomics Core Facility.
Raw sequences were processed using the DADA2 R-package in R version 3.6.3 (R Core Team, 2020b). We followed the general methodology available on the DADA2 Github3 (11/2020). We filtered sequences with more than two and five estimated errors on forward and reverse reads, respectively, and truncated reads on their 3′ end at the first base where quality dropped under a quality score of 2 (TrunQ = 2). After estimation of error rates, Amplified Sequence Variants (ASVs) were predicted and merged using default parameters. Chimeras were removed using the consensus method in “removeBimeraDenovo” function. Any final ASV out of a range of 243-263 base pairs was then removed, leaving a total of 57,556 ASVs in the un-rarefied dataset of 318 samples. Bivalve microbiomes contained 4,042 to 196,506 sequences. To ensure comparable alpha- and beta-diversities, we randomly rarefied bivalve samples to 4,000 sequences. Environmental samples (sediment, seston) contained fewer sequences (2,374 - 16,587 and 2,100 - 34,963, respectively), and were rarefied to 2,000 sequences per sample. After rarefaction, 31,091 ASVs remained in the entire dataset containing all bivalves and environmental samples. Coverage was assessed by Chao’s non-parametric indicator using the “entropart” R-package (Marcon and Hérault, 2015) and averaged 0.98 ± 0.02 (Mean ± Standard Deviation, here and elsewhere) across samples after rarefaction.
Microbial Phylogeny and Diversity Indices
Alpha-diversity (Shannon alpha-diversity) was assessed using the ‘vegan’ R-package and expressed in an equivalent number of species (Dixon, 2003; Jost, 2007). To compute phylogenetic diversity, ASV sequences were incorporated into the GreenGenes 99% phylogenetic tree version 13.8 (McDonald et al., 2012) using SEPP software (Janssen et al., 2018) implemented in QIIME2 (Bolyen et al., 2019), using default parameters. Phylogenetic richness, based on the phylogenetic distance between ASVs, and phylogenetic diversity, taking into account both phylogenetic distances and ASV relative abundance, were, respectively, assessed using Faith’s PD and the index of Allen using “picante” (Kembel et al., 2010) and “entropart” R-packages. Phylogenetic beta-diversity was assessed using the weighted (W-) and unweighted (U-) versions of Unifrac on microbial phylogeny using GUniFrac R-package (Chen, 2021).
Statistical Analysis of Phylogenetic and Taxonomic Data
All data visualizations were made using the “ggplot2” R-package (Wickham, 2016). Comparison of alpha-diversity between C. fluminea and native mussels was assessed using Wilcoxon signed-rank tests, using all native mussel species at once, and separately for each mussel species. Rarefaction curves for each sample were obtained using “vegan” and averaged per species, before plotting. To assess differences in the overall structure of the gut microbiome, U- and W-Unifrac dissimilarities were plotted along the first two axes of a Principal Coordinates Analysis (PCoA) ordination, and significant differences between species, sites, and rivers were assessed using PERMANOVAs. Post hoc pairwise comparisons of microbiome structure were assessed using ‘‘pairewiseAdonis4.” Correlation between microbiome dissimilarities and physicochemical characteristics on site were assessed using the envfit function in “vegan,” removing sites for which we didn’t have all measurements (S1-Supplementary Table 2). Tritogonia verrucosa specimens presented the highest variability and made the ordinations difficult to interpret. Therefore, to ease visualizations, we performed PCoAs without T. verrucosa. Separate PCoAs, including T. verrucosa, are provided in Supplementary Information S2. To assess the geographical variation within C. fluminea microbiome, Spearman correlation tests were performed on U- and W-Unifrac dissimilarities and geographical distances between sites along the same river.
Individual LefSe analyses were computed to identify which microbial taxa differed between C. fluminea and each mussel species consistently across all sites (Segata et al., 2011). Co-variation of mussel and co-occurring C. fluminea gut microbiomes were assessed using 500 Mantel tests computed in “vegan,” on random subsamples of the same number of C. fluminea and native mussel specimens on each site. The median of the 500 p-values was computed and the distribution of Spearman’s correlation values was visualized using boxplots. The potential contribution of the microbiome of C. fluminea to that of native mussels was assessed using FEAST (Shenhav et al., 2019) with each native mussel specimen as a “sink” and all other co-occurring microbial communities (microbiomes of other mussels, C. fluminea, seston, and sediment) as “sources.” Conversely, the potential influence of the microbiome of native mussels over co-occurring C. fluminea was assessed using each native mussel specimen as a “source” and C. fluminea as a “sink.” Such reciprocal influence was then compared across recipient or source species (i.e., distinct mussel species and C. fluminea) and sites using separated Kruskal-Wallis tests (KW). They were then correlated with physicochemical and biotic variables (native mussel and C. fluminea density, native mussel richness) using separated Pearson’s correlation tests on scaled data using the “psych” R-package (Revelle, 2021).
Microbial Functional Inferences and Functional Diversity
Functional inferences from the ASV community table and ASV sequences were obtained using PICRUST2 using default parameters except for a similarity cutoff of 0.75 to remove poorly aligned sequences (Douglas et al., 2020). The inferred enzymatic functions were aggregated into metabolic pathways according to MetaCyc database release May 2020, using the same software. Predictive abundances of pathways were transformed using a clr transformation of predicted expression levels using the “microbiome” R-package (Lahti et al., 2019), and samples were visualized using a Principal Component Analysis (PCA) in the “phyloseq” R-package (McMurdie and Holmes, 2013). Functional Bray-Curtis dissimilarities were computed on the transformed functional table, using “vegan” in order to test the overall differences in predicted functions from mussel vs. C. fluminea microbiome, using PERMANOVAs in “vegan.” To identify which pathways were significantly enriched in C. fluminea compared to native mussels, we performed a DESeq2 analysis with a negative binomial generalized linear model (P < 0.05) using “phyloseq” and “DESeq2” R-packages (Love et al., 2014). The parent class for each significant pathway was manually recorded from the MetaCyc database to simplify result visualization.
Potential structural diversity of degraded compounds was assessed by recording the Simplified Molecular Input Line-Entry System (SMILES) code of each entry compound of all pathways belonging to the Degradation-Utilization-Assimilation class. Pairwise structural dissimilarities between every compound were computed using RDKit (Landrum, 2021), and averaged for each degradation pathway. The obtained dissimilarities were then used to reconstruct a dendrogram using the ‘stats’ R-package, which was used as an input in GUniFrac to assess the predicted structural richness (unweighted Unifrac) of potentially degraded compounds (hereafter, “degradation potential”).
Population Genomics of Corbicula fluminea
Restriction Site-Associated DNA Library Construction and Sequencing
To evaluate the population structure of C. fluminea, we used the Best-RAD protocol (Ali et al., 2016) for reduced representation genomic sequencing (Ali et al., 2016; Andrews et al., 2016) of 185 C. fluminea from the sites described above (Figure 1 and S1-Supplementary Table 1). DNA was isolated from mantle clips using Qiagen DNeasy kits (Germantown, ML, United States). DNA was normalized to 10 ng/μl and digested with the restriction enzyme SbfI-HF (New England Biolabs, Ipswich, MA, United States), followed by ligation of oligonucleotides containing unique 8 base pair Hamming barcodes for each individual. Barcoded samples were combined into two sample pools (94 and 91 haphazardly distributed samples each) and sonicated using a Covaris M220 focused ultrasonicator (COVARIS, INC, Woburn, MA, United States) to generate fragments with a mean of 550 bp. RAD-tag fragments for each sample pool were isolated with streptavidin beads, and biotinylated groups were removed by Sbf-1 digestion. A NEBNext Ultra™ kit (New England Biolabs, Ipswich, MA) was used to prepare Illumina sequencing libraries with unique dual index primers for each sample pool, using 12 PCR cycles to amplify the RAD-tags. Samples were sequenced on an Illumina HiSeq X (Illumina Inc., San Diego, CA, United States) to produce 150 bp paired-end reads (Psomagen, Rockville, ML, United States).
Bioinformatics
Illumina reads were demultiplexed and quality filtered using Stacks v2.53 (Catchen et al., 2013) process_radtags (parameters -c, -q, -r, –best-rad, others default). 173 samples (> 500,000 reads per sample) were retained for downstream analyses. Reads were mapped to the 18 major linkage groups in the chromosome-level C. fluminea genome assembly (Zhang et al., 2021) using BWA-mem v (Li and Durbin, 2009). Alignments were sorted with samtools v1.10 (Li et al., 2009), and duplicate reads were removed with picard v2.18.9 MarkDuplicates5. Single Nucleotide Polymorphisms (SNPs) were called using freebayes v1.2.0 (Garrison and Marth, 2012), with the minimum coverage across samples set at 100 and including monomorphic loci (–report-monomorphic). All data processing of variant call files (vcf) used VCFtools v0.1.16 (Danecek et al., 2011) and statistical analyses using R version 4.0.3 (R Core Team, 2020a). We first removed indels and retained sites with a maximum of two alleles, a minimum sequence coverage of five, and a maximum of 10% missing data. This vcf was used to calculate nucleotide diversity (π) for each site, including monomorphic sites, using –site-pi in vcftools; π was averaged for each chromosome for individuals in each river. After calculating π, for other population genetic analyses, the data set was filtered to only include variant sites (–min-alleles 2) with a minimum quality threshold of 10 (–minQ 10) and a minor allele frequency of 5% (–maf 0.05). SNPs with a minor allele frequency < 0.05 were removed to reduce the impact of low frequency alleles and possible genotyping error (Rochette et al., 2019). Given that C. fluminea is clonal and based on other population genomic studies of this species (Haponski and Foighil, 2019), it was anticipated that there would be substantial excesses in observed heterozygosity (Balloux et al., 2003; Stoeckel et al., 2006). The inbreeding coefficient FIS was thus calculated in “hierfstat” R-package v0.5-7 using the function boot. Pfis with 1,000 bootstraps (Goudet and Jombart, 2021). After observing strongly negative FIS values (see Results), an inspection of the data revealed many SNPs that were fully heterozygous in all samples. We elected to remove SNPs with fixed observed heterozygosity prior to population structure analyses and then thinned loci to ≤ 1 SNP per kb to retain no more than one SNP per RAD-tag locus. This final dataset of 5,225 SNPs was used for all population structure and genetic distance estimations below.
The R-package “vcfR” v1.12.0 was used for import and data format conversion (Knaus and Grünwald, 2017). Individual-level differentiation was examined using a dissimilarity matrix. The “poppr” v2.9.2 package function diss.dist was used to create a distance matrix of the percent allelic differences between individuals (Kamvar et al., 2014, 2015). Population structure was visualized using discriminant analysis of principal components (DAPC) performed in “adegenet” v2.1.4 (Jombart, 2008) and plotted using “ggplot2.” To examine the genetic differentiation among populations, pairwise FST was calculated (significance tested by 1,000 bootstraps) in “StAMPP” v1.6.2 (Pembleton et al., 2013). To assess the geographical patterns within C. fluminea genetics, Spearman tests were performed on individual genetic dissimilarities and river distances between sites along the same river. The overall correlation between individual genetic variation and microbiome was then computed using Mantel tests performed on the 73 individuals in common the microbiome and RADSeq datasets using “vegan” (999 permutations), performed both globally and within each river. A population-level Mantel test was also performed to assess a possible correlation between pairwise FST and U- and W-Unifrac distances computed for site-averaged microbiomes (i.e., averaged ASV relative abundances for all C. fluminea from the same site).
DNA Barcoding
To confirm the clonal lineage assignment of individuals included in the analysis, a subset of individuals from each location (N = 47 total) were amplified for the mitochondrial cytochrome c oxidase subunit I (COI) gene using the LCO1490 and HCO2198 (Folmer et al., 1994) DNA barcoding primers. PCR and sequencing (in both primer directions) following methods in Lozier et al. (2020). Geneious R10 (BioMatters, Ltd.) was used for all sequence inspection, editing, assembly, and alignment. Sequence ends were automatically trimmed (2.5% error limit) followed by manual inspection. Consensus sequences for each sample were aligned with the MAFFT plug-in. Clone identity was determined using NCBI BLAST (default megablast).
Results
Gut Microbiome Diversity and Structure
Phylogenetic richness based on presence-absence data and diversity weighted by relative abundances of ASVs were higher in C. fluminea microbiome than that of native mussels (Figure 2 and S2-Supplementary Table 1, Wilcoxon tests, P < 0.001). The C. fluminea microbiome was richer phylogenetically and taxonomically than every co-occurring mussel species sampled, except for that of L. ovata, which reached similar levels (Figure 2 and S2-Supplementary Table 1). Phylogenetic and taxonomic and diversity accounting for relative abundance were also significantly higher in C. fluminea when compared to two and four of the native mussel species, respectively (S2-Supplementary Table 1). Conversely, estimated coverage was significantly higher for every mussel species compared to C. fluminea, excepted for L. ovata (S2-Supplementary Table 1). While C. fluminea shared 21.4-26.9% of its ASVs with co-occurring mussel species, each mussel species shared 31.5-53.1% of their ASVs with co-occurring C. fluminea.
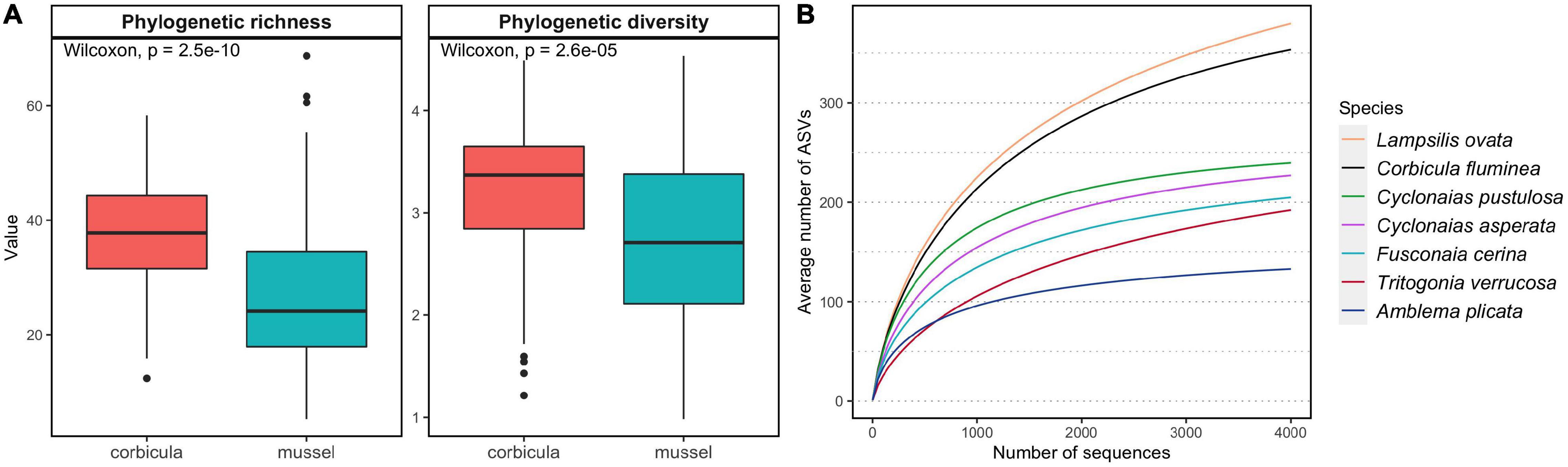
Figure 2. Alpha diversity of the gut microbiomes of six native freshwater mussel species and the invasive clam Corbicula fluminea, collected from six rivers in the Tennessee and Mobile River Basins, United States. (A) Distribution of phylogenetic richness (Faith’s PD) and phylogenetic diversity (Allen index of diversity) in C. fluminea and mussels. (B) Average accumulation curves representing the number of amplified sequence variants (ASVs) in random subsamples of 1 to 4000 sequences per sample. Other descriptors of alpha diversity and coverage are available in S2-Supplementary Table 1.
The overall structure of the C. fluminea gut microbiome was distinct from that of native mussels as a group (PERMANOVA, P = 0.001, R2 = 0.09 and 0.03 for W- and U-Unifrac, respectively, Figures 3A,C); more distinct from seston bacterial communities (P = 0.001, R2 = 0.17 for both indices); and even more distinct from sediment bacterial communities (P = 0.001, R2 = 0.47 and 0.50). The structure of the C. fluminea microbiome and that of each mussel species were systematically distinct, especially based on W-Unifrac (R2 = 0.11-0.33 depending on the mussel species considered; S2-Supplementary Table 2). The mussels T. verrucosa and A. plicata hosted the most distinctive microbiomes compared to that of C. fluminea, and T. verrucosa sampled from the Buttahatchee and Sipsey Rivers exhibited the most unique microbiome structure compared to all other species (Figure 3 and S2-Supplementary Table 2, S2-Supplementary Figure 1). Variability in microbiome structure was equivalent for C. fluminea and each mussel species, other than greater variability in T. verrucosa and C. asperata (permutation tests on betadisper, P < 0.05).
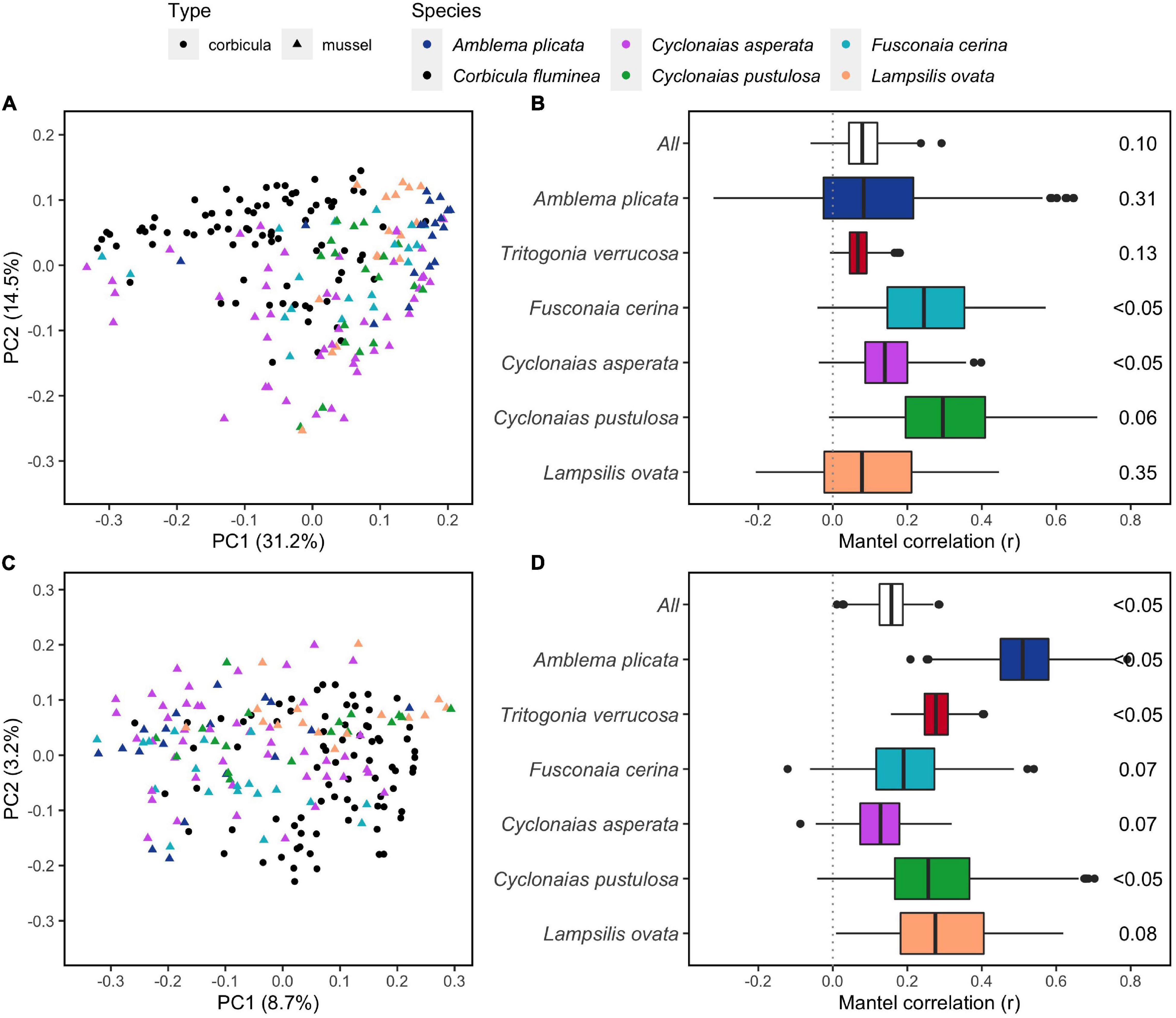
Figure 3. Dissimilarities between gut microbiomes of six native freshwater mussel species and the invasive clam Corbicula fluminea, collected from rivers in the Tennessee and Mobile River Basins. A and C: Principal Coordinate Analyses (PCoAs) representing the weighted (A) and unweighted (C) versions of Unifrac phylogenetic dissimilarity between individual microbiomes of five of the mussel species, and C. fluminea (PCoAs with all six species including T. verrucosa are available in S2-Supplementary Figure 1). B and D: correlations between dissimilarities of W-Unifrac (B) and U-Unifrac (D) of C. fluminea and mussel microbiomes, assessed by 500 Mantel tests on random subsamples of the same number of specimens at each site (either with all mussel species – “All,” or per species). The median of the 500 p-values, indicating an overall significant co-variation between C. fluminea and mussel microbiomes, is displayed on the right.
The mean dissimilarity between C. fluminea and native mussels (U-Unifrac = 0.73 ± 0.06; W-Unifrac = 0.36 ± 0.10) was similar to that between native mussel species (U-Unifrac = 0.73 ± 0.06; W-Unifrac = 0.39 ± 0.12). When the analyses were performed excluding T. verrucosa, to eliminate the greater influence of this mussel species, C. fluminea vs. mussel dissimilarity (U-Unifrac = 0.73 ± 0.04; W-Unifrac = 0.33 ± 0.08) remained similar to that within native mussel species (U-Unifrac = 0.73 ± 0.05; W-Unifrac = 0.34 ± 0.08).
Additionally, the gut microbiome of C. fluminea tended to co-vary with that of native mussels, especially based on the presence-absence of ASVs (500 Mantel tests on U-Unifrac, median P < 0.1, Figure 3). However, this correlation was not significant in T. verrucosa, A. plicata, and L. ovata when considering relative abundance weighted dissimilarities (median P > 0.1, Figure 3).
Geographic Variation in the Gut Microbiome
Structures of C. fluminea and native mussel microbiomes varied across rivers and sampling sites, and these effects were higher for W-Unifrac than U-Unifrac (PERMANOVAs, P < 0.01 and R2 = 0.14-0.38 for every mussel species, and P < 0.01 and C. fluminea, R2 = 0.15-0.58, respectively; S2-Supplementary Table 3). Microbiome dissimilarities of C. fluminea showed a positive correlation with river distance, i.e., more distant individuals along the same river tended to have more distinct microbiomes (Spearman test, P < 0.001, S2-Supplementary Figure 2). Among environmental variables, soluble nitrate (P = 0.001, R2 = 0.40), nitrite (P = 0.001, R2 = 0.32), SRP (P = 0.02, R2 = 0.14), water temperature (P = 0.02, R2 = 0.14) and dissolved oxygen (P = 0.03, R2 = 0.12), were significantly correlated to variations in the structure of C. fluminea microbiome (Figure 4).
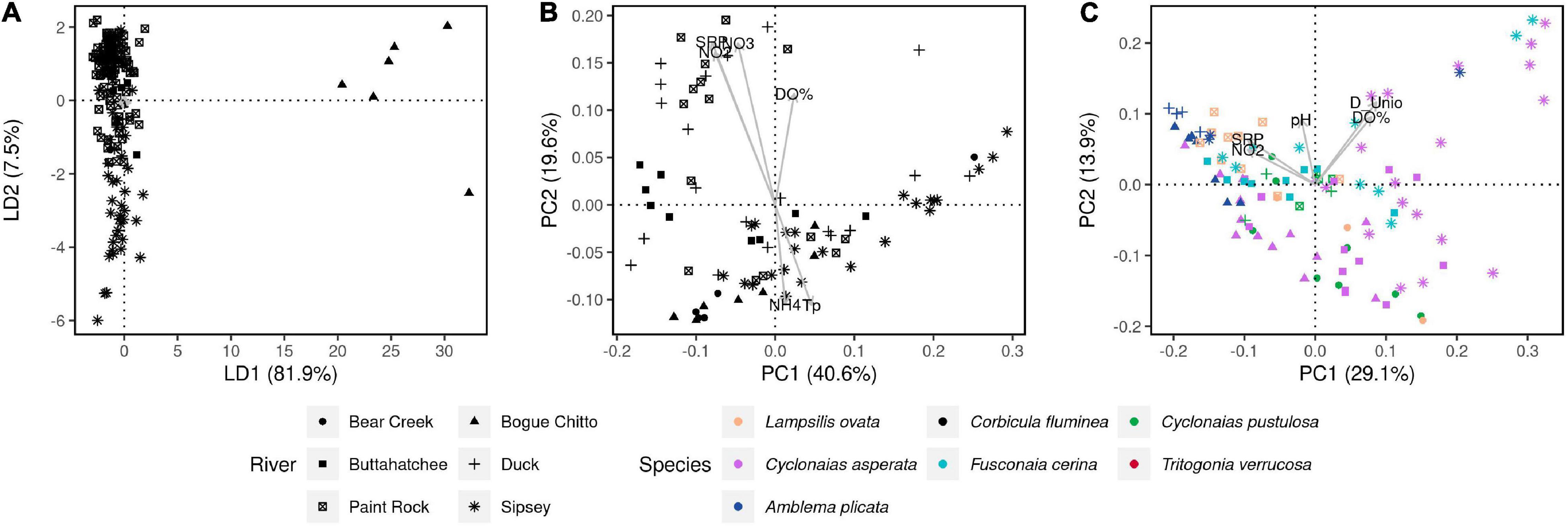
Figure 4. Genetic and microbial dissimilarities in the invasive clam C. fluminea and six native freshwater mussel species, collected from six rivers in the Tennessee and Mobile River Basins. (A) DAPC showing the genetic dissimilarities between all 173 C. fluminea specimens that were included in the population genomics analysis (RADSeq). (B) PCoA displaying W-Unifrac dissimilarities between the gut microbiomes of the 80 individuals analyzed for their microbiome. (C) PCoA displaying W-Unifrac dissimilarities between the gut microbiomes of the native freshwater mussels collected. On plots (B,C), Physicochemical and biological variables were correlated to the coordinates of each individual microbiome and are represented by arrows. Only parameters showing a significant correlation (P < 0.05) are shown. D_Unio: unionid density; D_Corb: C. fluminea density; S: unionid richness; NO2: water nitrite; NO3: water nitrate; SRP: soluble reactive phosphorus in water; Tp: water temperature.
The microbiome dissimilarities between native mussels (other than T. verrucosa) were correlated to unionid density (P = 0.002, R2 = 0.23), dissolved oxygen (P = 0.002, R2 = 0.17), nitrite (P = 0.001, R2 = 0.17), SRP (P = 0.005, R2 = 0.13), pH (P = 0.003, R2 = 0.12), and water temperature (P = 0.02, R2 = 0.08) (envfit, Figure 4). The microbiome dissimilarities between T. verrucosa only correlated to SRP (P = 0.02, R2 = 0.22). There was no significant correlation between C. fluminea density and the microbiome dissimilarities between native mussels (envfit, P = 0.38).
Population Genomic Variation in Corbicula fluminea and Its Relationship to the Gut Microbiome
DNA barcoding with COI confirmed that samples were identical to the C. fluminea lineage “A” (S3-Supplementary Figure 1), the most common in North America. Clonal lineage thus does not appear to have a major contribution to microbiome dissimilarities across C. fluminea individuals in these rivers. 3,580,850 bp were sequenced by RAD-tag sequencing to a mean coverage of 44.15x per site sample, with a mean of 2.4% missing data per sample. Nucleotide diversity was similar among populations (π = 0.0049 - 0.0053 for all rivers) and thus was also not considered a major predictor of microbiome variation (S3-Supplementary Table 1). Consistent with extensive clonal reproduction (Haponski and Foighil, 2019), FIS was strongly negative in all populations (FIS values −0.92 to −0.85, S3-Supplementary Table 1), and there were many SNPs (7,213 of 34,646 SNPs) that were heterozygotes across all sequenced individuals. After removing these sites and thinning to one SNP per kb, 5,225 SNPs remained for analysis of population structure. Pairwise FST showed weak but significant differentiation of C. fluminea across rivers (S3-Supplementary Table 2). DAPC analysis also indicated within clonal lineage variation in diversity that was primarily structured by river, although not by basin; samples from the Bogue Chitto River largely separated on Axis 1 and Axis 2 transitioned from Sipsey River samples (negatively loading) to Paint Rock and Bear Creek River samples, to Duck and Buttahatchee River samples (positively loading) (Figure 4). Within rivers, genetic dissimilarities of C. fluminea exhibited a positive correlation with geographical distance, i.e., more distant individuals tended to have more distinct genomes (Spearman correlation testS, P < 0.001, S2-Supplementary Figure 2). Despite this structuring of C. fluminea genetic diversity and geographic variation in gut microbiomes, no significant correlations between genetic distances and microbiome dissimilarities were detected, either globally (Mantel tests on W- and U-Unifrac and individual genetic dissimilarities, P > 0.05) or within rivers (Spearman’s correlation test on dissimilarities between individuals collected along the same river, P = 0.02, r = −0.1, Figure 4 and S2-Supplementary Figure 2). Similarly, no correlation was detected based on FST between rivers (Mantel tests on averaged W- and U-Unifrac per river and FST, P > 0.05).
Composition
The C. fluminea gut microbiome consisted of 69 different bacterial phyla and 126 bacterial classes, with the classes accounting for the greatest proportion of sequences being similar to those in the microbiome of the six native mussel species. These included Clostridia (34.7 ± 21.2% and 14.1 ± 20.3% of the sequences for C. fluminea and native mussels, respectively), Planctomycetacia (18.1 ± 9.3% and 23.5 ± 15.3%), Alphaproteobacteria (10.6 ± 5.1% and 12.7 ± 8.9%), Gammaproteobacteria (9.7 ± 10.6% and 8.2 ± 7.4%), and Bacilli (4.3 ± 4.9% and 3.5 ± 5.7%). Native mussels also presented high percentages of Mollicutes, which were of a lower abundance in C. fluminea (12.8 ± 14.9% in mussels vs. 4.5 ± 5.5%). Compared to all species of native mussels and across all sites, the C. fluminea microbiome contained higher proportions of Actinobacteriota (LefSE log10 LDA score = 3.0), Verrucomicrobiota (2.9), and Planctomycetes (3.5), especially the Gemmataceae (3.2) and Pirellulaceae (3.0). Eleven of the most abundant ASVs were found to be significantly enriched in C. fluminea compared to native mussels, the most numerous ones classified as members of genera Romboutsia (Clostridiales), Stenotrophomonas (Xanthomonadales), Epulopiscium (Lachnospirales), and Paraclostridium (Peptostreptococcales) (S2-Supplementary Figure 3).
Potential Reciprocal Influences of Corbicula fluminea and Mussel Microbiomes
The estimated influence of the C. fluminea microbiome on that of the co-occurring mussels was on average of 5.8 ± 8.0%, while the influence of the native mussel microbiome on the C. fluminea microbiome was 14.0 ± 12.2% (KW on FEAST results, P < 0.001) (Figure 5). The estimated influence of the C. fluminea microbiome over that of native mussels was primarily dependent on the recipient mussel species (KW, P < 0.001), and independent from the collection site (KW, P = 0.08). Mussel species that were under the greatest influence from the C. fluminea microbiome were Cyclonaias pustulosa (11.6 ± 13.5%) and Lampsilis ovata (7.4 ± 7.7%), while the species that was the least influenced was Tritogonia verrucosa (2.4 ± 3.8%).
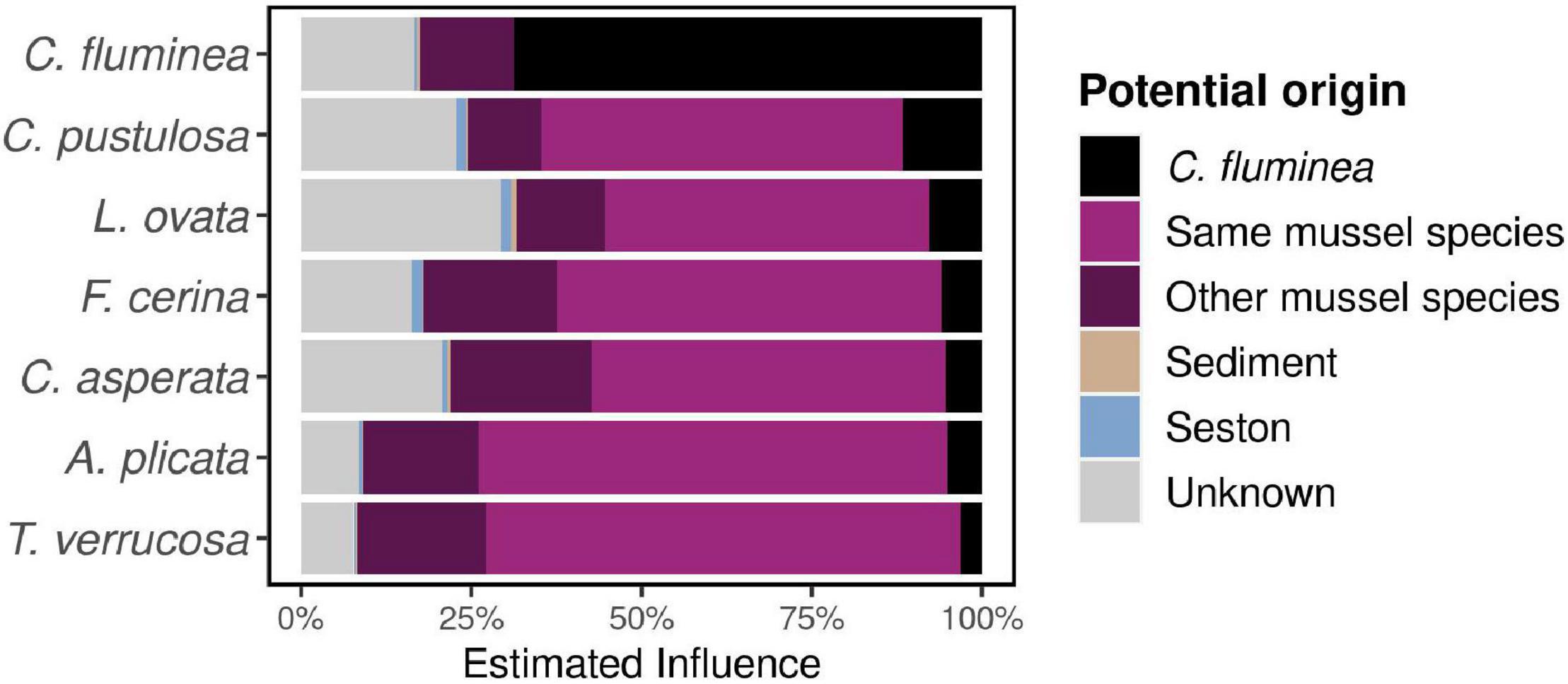
Figure 5. Influence of co-occurring host-associated, seston, and sediment microbes over individual microbiomes, estimated using FEAST software and averaged per species. Globally, individual bivalves presenting a higher reciprocal influence (native mussels over C. fluminea, and reversely) presented a higher phylogenetic richness (Pearson’s correlation test on log-transformed data, P < 0.001, r = 0.6).
In contrast, the influence of the native mussel microbiome on that of C. fluminea was significantly distinct across sites, varying from 4.67% on site FAY (Sipsey river) to 15.3% on BTN (Paint Rock river, KW, P = 0.01), but not across source mussel species (KW, P = 0.051, varying from 4.39% from T. verrucosa to 9.47% from C. pustulosa although no mussel species were sampled on every site, making such comparison difficult, Figure 1 and S1-Supplementary Table 1). However, neither water physicochemical characteristics nor biotic variables (C. fluminea and unionid densities) explained such variation of mussel contribution across sites variation (Spearman’s correlation tests, P > 0.05).
Inferred Functions and Degradation Potential
The 436 functional pathways were inferred from the C. fluminea gut microbiome sequence data compared to 443 for native mussels. The functional potential of C. fluminea was distinct overall from that of native mussels (PERMANOVA based on Bray-Curtis, P = 0.001, R2 = 0.07), especially from that of T. verrucosa, A. plicata, and C. asperata (PERMANOVA’s pairwise post hoc tests, P < 0.05). Compared to native mussels, the functional potential of the C. fluminea gut microbiome was enriched in pathways related to the degradation of various compounds (especially carbohydrates and carboxylates, aromatic compounds). In contrast, the microbiome of mussels included higher potential for biosynthesis (especially cofactors and vitamins, nucleotides, and nucleosides) (Figure 6). Among the seven pathways that contributed the most to the differences between the C. fluminea and mussel microbiomes, six were classified as a degradation or assimilation function, and only one to biosynthesis (methylquinone). All seven revealed a potential for higher expression in C. fluminea than in native mussels (S2-Supplementary Figure 4). Accordingly, the degradation potential of each microbiome, taking into account all degradation pathways, was significantly higher for C. fluminea than for any native mussel species except L. ovata, where it reached similar levels (S2-Supplementary Figure 5).
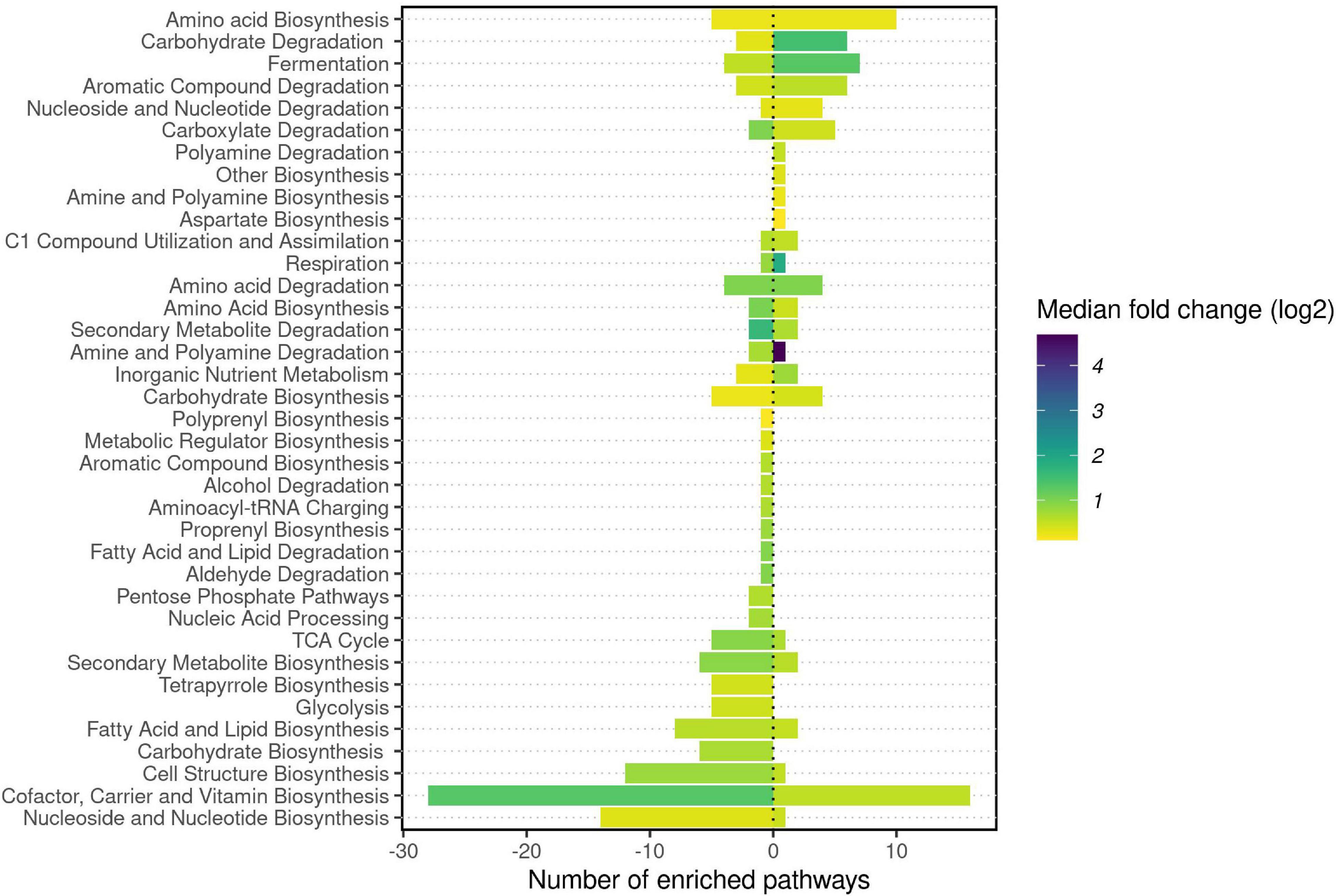
Figure 6. DESeq2 analysis of inferred microbial functions from 16S rRNA gene data of six freshwater mussel species and the invasive clam Corbicula fluminea collected from six rivers in the Tennessee and Mobile River Basins. DESeq2 was used to determine which inferred pathways were significantly enriched in C. fluminea compared to native mussels, before the class of each pathway was recorded from the MetaCyc database and the number of pathways significantly enriched in each class was reported. Pathways enriched in C. fluminea are presented by positive values, while the negative values represent those enriched in mussels. For each host type and each class of pathway, the median of the log2 Fold change was used to color the bars.
Discussion
Here we document for the first time the gut microbiome of the invasive C. fluminea. We demonstrate that while this species’ microbiome is diverse, it is not highly distinctive from that of the co-occurring native mussels. The high correlation of the structure of the C. fluminea microbiome with local environmental parameters, together with the influence of co-occurring mussel species and the lack of influence of host genetic ancestry, suggests that the microbiome of this invasive species may be largely dependent on extrinsic factors such as environmental conditions and local bacterial occurrence.
Differences in the Microbiome Between Corbicula fluminea and Native Mussels
The microbiome structure of C. fluminea was only partially distinct from that of six co-occurring native mussel species, with similar dominant classes of Bacteria but differences occurring at a finer taxonomic level. Overall similarities between the microbiome of native mussels and C. fluminea could be explained by the trophic similarity between these organisms, primarily filter-feeding on suspended particles and deposit-feeding within the sediment (Sousa et al., 2008; Schartum et al., 2017). However, given the species-specificity of microbiomes within freshwater mussels (i.e., distinct species occupying the same habitat host distinct microbiomes, (Weingarten et al., 2019; McCauley et al., 2021), we expected a more distinct C. fluminea microbiome given its contrasting phylogeny and life history traits to those of freshwater mussels. We also found no evidence that intra-specific genetic ancestry or diversity was related to microbiome variation within C. fluminea. All individuals sampled belong to the clonal lineage A (Haponski and Foighil, 2019) and although SNP data revealed significant within-lineage genetic differentiation across rivers, there was no effect of genetic differentiation among individuals or populations on their microbiomes. Instead, the structure of C. fluminea microbiome was tightly correlated to local environmental variables, with nitrates, nitrites, and SRP being the primary drivers.
Studies on other invertebrates have demonstrated a consistent effect of host genotype on the microbiome when hosts are reared under controlled or germ-free conditions (Wegner et al., 2013; Callens et al., 2020), so that it is likely that the lack of correlation between the genotype of C. fluminea and its gut microbiome in this study is related to variable field conditions. Further studies under controlled conditions would be needed to confirm the absence of a host genotype-microbiome link in this aquatic filter-feeder.
Diversity of the Microbiome of Corbicula fluminea and Inferred Functions
We observed a greater microbial richness in the gut microbiome of C. fluminea compared to co-occurring native mussels, except for L. ovata, which had similar levels of microbiome diversity. Such higher diversity may be related to differences in feeding behavior, as a higher clearance rate or a lower selectivity in ingested particles could induce gut colonization by a greater diversity of bacteria. C. fluminea has a higher filter-feeding rate than the few native mussels that have been studied so far (Hills et al., 2020; Pouil et al., 2021), and while systematic comparisons are lacking, C. fluminea also shows a low prey selectivity (seemingly limited to an avoidance of toxic bacterioplankton (Bolam et al., 2019) and assimilates a wider range of substrates (Atkinson et al., 2010). Unionid mussels exhibit a higher degree of filtration selection and a narrower range of substrate assimilation (Beck and Neves, 2003; Atkinson et al., 2010; Lopes-Lima et al., 2014b). The greater bacterial diversity hosted by C. fluminea may allow them to consume a broader range of nutritional sources. This could provide a competitive advantage for C. fluminea and, accordingly, functional inferences predicted a greater diversity of degradation potential in the gut microbiome of C. fluminea compared to native mussels, and most metabolic pathways that were enriched in C. fluminea were associated with degradative functions. This hypothesis is based on functional inferences and should be studied further with appropriate metagenomic analysis of the C. fluminea gut microbiome to confirm such higher prevalence of degradation functions, combined with experiments to assess the relationship between the C. fluminea microbiome and the nature of successfully digested particles.
Reciprocal Influences Between Invasive and Native Species
Overall, native mussels shared a substantial fraction of their microbiomes with co-occurring C. fluminea (31.5-53.1% of ASVs depending on mussel species), and C. fluminea and native mussel microbiomes tended to co-vary across sites. The FEAST estimate of mussel influence over co-occurring C. fluminea microbiome was more than twice that of the reverse interaction. This may indicate that C. fluminea may host gut populations that are obtained locally, including from native mussels that may be indirectly transferred through the water column or the sediment via feces and pseudofeces. While such potential contamination should be further explored in controlled conditions, it may confirm evidence from plants that suggests that invasive species can suffer from the loss of their natural bacterial symbionts when they are introduced to a new environment, and must develop novel interactions with local bacteria, potentially bacteria associated with co-occurring native species (Parker et al., 2006; Shelby et al., 2016).
Accordingly, while comparisons of the microbiome of invasive animal species across native vs. introduced range are lacking, a study on invasive tunicates documented a more variable and more diverse microbiome in individuals in the introduced range than those in their native range, which may also suggest this hypothesis could be confirmed in invasive animals (Utermann et al., 2020). Moreover, in our study, while the estimated influence of mussel microbiome over that of C. fluminea was variable across sites, it was independent of local unionid density. Although appropriate density experiments must confirm this, this may indicate that even low densities of native species may provide microbial partners to C. fluminea microbiome.
Conclusion
We show that the gut microbiome of the invasive clam C. fluminea presents several characteristics (e.g., higher diversity, higher functional potential, and potentially a lower selectivity and a higher rate of horizontal transmission from native counterparts) that may be beneficial for such a globally invasive organism to acclimate to a non-native area. This work should be further developed, focusing on understanding which bacterial partners may be more beneficial to C. fluminea to assess whether these partners are also enriched in populations of C. fluminea in their native range, or are indicative of invasive areas. Studies evaluating geographic regions with additional clonal lineages would also be valuable for fully understanding how genetic diversity contributes to microbiome variation in this invasive species. Focused analyses could pave the way for a more nuanced understanding of the transport of symbionts within invasive animal species, which is still a poorly investigated topic, and has the potential to develop pre- or probiotics for endangered native species.
Data Availability Statement
The datasets presented in this study can be found in online repositories. The names of the repository/repositories and accession number(s) can be found below: https://www.ncbi.nlm.nih.gov/genbank/, OK047371-OK047417; https://www.ncbi.nlm.nih.gov/sra, PRJNA757758, PRJNA757734, PRJNA740316, and PRJNA761344.
Author Contributions
MC and CJ conceptualized the study. CA, GH, IS, JB, MM, and MC participated in sample collection and fieldwork. MC and SV processed the microbiome samples and prepared the libraries.MC analyzed the microbiome data. JB and JL performed RADseq and DNA barcoding and analyzed the data. MC wrote the manuscript, with contributions from JB and JL for the genomic analyses. All authors read and approved the final manuscript.
Funding
Funding was provided from National Science Foundation awards DEB-1831531 to CJ, DEB-1831512 to CA and JL, and an award from Conchologists of America to MC.
Conflict of Interest
The authors declare that the research was conducted in the absence of any commercial or financial relationships that could be construed as a potential conflict of interest.
Publisher’s Note
All claims expressed in this article are solely those of the authors and do not necessarily represent those of their affiliated organizations, or those of the publisher, the editors and the reviewers. Any product that may be evaluated in this article, or claim that may be made by its manufacturer, is not guaranteed or endorsed by the publisher.
Acknowledgments
Native mussels were collected under permits (USFWS permit #TE68616B-1 and ALCDNR permit #2016077745468680) issued by the US Fish and Wildlife Service and Alabama Department of Conservation and Natural Resources to CA. We thank the Alabama Aquatic Biodiversity Center, Todd Amacker, Austin Beets, Ansley Hamid, Madison Knapp, Megan Kubala, Matt Lodato, Taylor Kelley, Josh Millwood, Austin Omer, and Brian van Ee for help in the field. Megan Kubala helped with dissections. Anne Bell analyzed water samples for N and P.
Supplementary Material
The Supplementary Material for this article can be found online at: https://www.frontiersin.org/articles/10.3389/fmicb.2022.800061/full#supplementary-material
Footnotes
- ^ https://www.invasivespeciesinfo.gov/
- ^ https://earthmicrobiome.org/protocols-and-standards/16s/
- ^ https://benjjneb.github.io/dada2/tutorial.html
- ^ https://github.com/pmartinezarbizu/pairwiseAdonis
- ^ http://broadinstitute.github.io/picard
References
Aceves, A. K., Johnson, P. D., Atkinson, C. L., van Ee, B. C., Bullard, S. A., and Arias, C. R. (2020). Digestive gland microbiome of Pleurobema cordatum: mesocosms induce dysbiosis. J. Molluscan Stud. 86, 280–289. doi: 10.1093/mollus/eyaa024
Albert, J. S., Destouni, G., Duke-Sylvester, S. M., Magurran, A. E., Oberdorff, T., Reis, R. E., et al. (2021). Scientists’ warning to humanity on the freshwater biodiversity crisis. Ambio 50, 85–94. doi: 10.1007/s13280-020-01318-8
Ali, O. A., O’Rourke, S. M., Amish, S. J., Meek, M. H., Luikart, G., Jeffres, C., et al. (2016). Rad capture (Rapture): flexible and efficient sequence-based genotyping. Genetics 202, 389–400. doi: 10.1534/genetics.115.183665
Andrews, K. R., Good, J. M., Miller, M. R., Luikart, G., and Hohenlohe, P. A. (2016). Harnessing the power of RADseq for ecological and evolutionary genomics. Nat. Rev. Genet. 17, 81–92. doi: 10.1038/nrg.2015.28
Atkinson, C. L., Opsahl, S. P., Covich, A. P., Golladay, S. W., and Conner, L. M. (2010). Stable isotopic signatures, tissue stoichiometry, and nutrient cycling (C and N) of native and invasive freshwater bivalves. J. North Am. Benthol. Soc. 29, 496–505. doi: 10.1899/09-083.1
Bahrndorff, S., Alemu, T., Alemneh, T., and Lund Nielsen, J. (2016). The microbiome of animals: implications for conservation biology. Int. J. Genomics 2016, 1–7. doi: 10.1155/2016/5304028
Balloux, F., Lehmann, L., and de Meeûs, T. (2003). The population genetics of clonal and partially clonal diploids. Genetics 164, 1635–1644. doi: 10.1093/genetics/164.4.1635
Beck, K., and Neves, R. J. (2003). An evaluation of selective feeding by three age-groups of the rainbow mussel Villosa iris. North Am. J. Aquacult. 65, 203–209. doi: 10.1577/C02-031
Bellard, C., Cassey, P., and Blackburn, T. M. (2016). Alien species as a driver of recent extinctions. Biol. Lett. 12:20150623. doi: 10.1098/rsbl.2015.0623
Benson, A. J., and Williams, J. D. (2021). Review of the Invasive Asian Clam Corbicula spp. (Bivalvia: Cyrenidae) Distribution in North America, 1924–2019 (Scientific Investigations Report N° 2021–5001). Reston, VA: US Geological Survey, 66.
Bolam, B. A., Rollwagen-Bollens, G., and Bollens, S. M. (2019). Feeding rates and prey selection of the invasive Asian clam, Corbicula fluminea, on microplankton in the Columbia River, USA. Hydrobiologia 833, 107–123. doi: 10.1007/s10750-019-3893-z
Bolyen, E., Rideout, J. R., Dillon, M. R., Bokulich, N. A., Abnet, C. C., Al-Ghalith, G. A., et al. (2019). Reproducible, interactive, scalable and extensible microbiome data science using QIIME 2. Nat. Biotechnol. 37, 852–857. doi: 10.1038/s41587-019-0209-9
Callens, M., De Meester, L., Muylaert, K., Mukherjee, S., and Decaestecker, E. (2020). The bacterioplankton community composition and a host genotype dependent occurrence of taxa shape the Daphnia magna gut bacterial community. FEMS Microbiol. Ecol. 96:fiaa128.
Catchen, J., Hohenlohe, P. A., Bassham, S., Amores, A., and Cresko, W. A. (2013). Stacks: an analysis tool set for population genomics. Mol. Ecol. 22, 3124–3140. doi: 10.1111/mec.12354
Chen, J. (2021). Package GUniFrac (1.3) [Computer Software]. The Comprehensive R Archive Network (CRAN). Available online at: https://CRAN.R-project.org/package=GUniFrac (accessed May 2021).
Chiarello, M., Paz-Vinas, I., Veyssière, C., Santoul, F., Loot, G., Ferriol, J., et al. (2019). Environmental conditions and neutral processes shape the skin microbiome of European catfish (Silurus glanis) populations of Southwestern France. Environ. Microbiol. Rep. 11, 605–614. doi: 10.1111/1758-2229.12774
Coats, V. C., and Rumpho, M. E. (2014). The rhizosphere microbiota of plant invaders: an overview of recent advances in the microbiomics of invasive plants. Front. Microbiol. 5:368. doi: 10.3389/fmicb.2014.00368
Danecek, P., Auton, A., Abecasis, G., Albers, C. A., Banks, E., DePristo, M. A., et al. (2011). The variant call format and VCFtools. Bioinformatics 27, 2156–2158. doi: 10.1093/bioinformatics/btr330
Darwall, W., Bremerich, V., De Wever, A., Dell, A. I., Freyhof, J., Gessner, M. O., et al. (2018). The Alliance for Freshwater Life: a global call to unite efforts for freshwater biodiversity science and conservation. Aquatic Conserv.: Mar. Freshw. Ecosyst. 28, 1015–1022. doi: 10.1002/aqc.2958
Dixon, P. (2003). VEGAN, a package of R functions for community ecology. J. Vegetation Sci. 14, 927–930. doi: 10.1111/j.1654-1103.2003.tb02228.x
Douglas, G. M., Maffei, V. J., Zaneveld, J. R., Yurgel, S. N., Brown, J. R., Taylor, C. M., et al. (2020). PICRUSt2 for prediction of metagenome functions. Nat. Biotechnol. 38, 685–688. doi: 10.1038/s41587-020-0548-6
Ferreira-Rodríguez, N., Sousa, R., and Pardo, I. (2018b). Negative effects of Corbicula fluminea over native freshwater mussels. Hydrobiologia 810, 85–95. doi: 10.1007/s10750-016-3059-1
Ferreira-Rodríguez, N., Fandiño, L., Pedreira, A., and Pardo, I. (2018a). First evidence of asymmetric competition between the non-native clam Corbicula fluminea and the native freshwater mussel Unio delphinus during a summer heat wave. Aquatic Conserv.: Mar. Freshw. Ecosyst. 28, 1105–1113. doi: 10.1002/aqc.2964
Folmer, O., Black, M., Hoeh, W., Lutz, R., and Vrijenhoek, R. (1994). DNA primers for amplification of mitochondrial cytochrome c oxidase subunit I from diverse metazoan invertebrates. Mol. Mar. Biol. Biotechnol. 3, 294–299.
Garrison, E., and Marth, G. (2012). Haplotype-based variant detection from short-read sequencing. arXiv preprint
Goddard-Dwyer, M., López-Legentil, S., and Erwin, P. M. (2021). Microbiome variability across the native and invasive ranges of the ascidian Clavelina oblonga. Appl. Environ. Microbiol. 87, e2233–e2220. doi: 10.1128/AEM.02233-20
Goudet, J., and Jombart, T. (2021). Package Hierfstat (0.5–10) [Computer Software]. The Comprehensive R Archive Network (CRAN). Available online at: https://www.r-project.org
Grizzle, J. M., and Brunner, C. J. (2009). Infectious diseases of freshwater mussels and other freshwater bivalve mollusks. Rev. Fisheries Sci. 17, 425–467. doi: 10.1080/10641260902879000
Haag, W. R. (2012). Chapter 6. In North American Freshwater Mussels: Natural History, Ecology, and Conservation. Cambridge: Cambridge University Press, 180–430.
Haag, W. R. (2019). Reassessing enigmatic mussel declines in the united states. Freshw. Mollusk Biol. Conserv. 22:43. doi: 10.31931/fmbc.v22i2.2019.43-60
Haponski, A. E., and Foighil, D.O. (2019). Phylogenomic analyses confirm a novel invasive North American Corbicula (Bivalvia: cyrenidae) lineage. PeerJ 7:e7484. doi: 10.7717/peerj.7484
Hills, A., Pouil, S., Hua, D., and Mathews, T. J. (2020). Clearance rates of freshwater bivalves Corbicula fluminea and Utterbackia imbecillis in the presence and absence of light. Aquatic Ecol. 54, 1059–1066. doi: 10.1007/s10452-020-09793-7
Hopper, G. W., Chen, S., Sánchez González, I., Bucholz, J. R., Lu, Y., and Atkinson, C. L. (2021). Aggregated filter-feeders govern the flux and stoichiometry of locally available energy and nutrients in rivers. Funct. Ecol. 35, 1183–1195. doi: 10.1111/1365-2435.13778
Ishibashi, R., Ookubo, K., Aoki, M., Utaki, M., Komaru, A., and Kawamura, K. (2003). Androgenetic reproduction in a freshwater diploid clam Corbicula fluminea (Bivalvia: corbiculidae). Zool. Sci. 20, 727–732. doi: 10.2108/zsj.20.727
Janssen, S., McDonald, D., Gonzalez, A., Navas-Molina, J. A., Jiang, L., Xu, Z. Z., et al. (2018). Phylogenetic placement of exact amplicon sequences improves associations with clinical information. MSystems 3, e21–e18. doi: 10.1128/mSystems.00021-18
Jombart, T. (2008). Adegenet: a R package for the multivariate analysis of genetic markers. Bioinformatics 24, 1403–1405. doi: 10.1093/bioinformatics/btn129
Jost, L. (2007). Partitioning diversity into independent alpha and beta components. Ecology 88, 2427–2439. doi: 10.1890/06-1736.1
Kamvar, Z. N., Brooks, J. C., and Grünwald, N. J. (2015). Novel R tools for analysis of genome-wide population genetic data with emphasis on clonality. Front. Genet. 6:208. doi: 10.3389/fgene.2015.00208
Kamvar, Z. N., Tabima, J. F., and Grünwald, N. J. (2014). poppr: an R package for genetic analysis of populations with clonal, partially clonal, and/or sexual reproduction. PeerJ 2:e281. doi: 10.7717/peerj.281
Kembel, S. W., Cowan, P. D., Helmus, M. R., Cornwell, W. K., Morlon, H., Ackerly, D. D., et al. (2010). Picante: R tools for integrating phylogenies and ecology. Bioinformatics 26, 1463–1464. doi: 10.1093/bioinformatics/btq166
Knaus, B. J., and Grünwald, N. J. (2017). VCFR: a package to manipulate and visualize variant call format data in R. Mol. Ecol. Resour. 17, 44–53. doi: 10.1111/1755-0998.12549
Kowalski, K. P., Bacon, C., Bickford, W., Braun, H., Clay, K., Leduc-Lapierre, M., et al. (2015). Advancing the science of microbial symbiosis to support invasive species management: a case study on Phragmites in the Great Lakes. Front. Microbiol. 6:95. doi: 10.3389/fmicb.2015.00095
Kozich, J. J., Westcott, S. L., Baxter, N. T., Highlander, S. K., and Schloss, P. D. (2013). Development of a dual-index sequencing strategy and curation pipeline for analyzing amplicon sequence data on the miseq illumina sequencing platform. Appl. Environ. Microbiol. 79, 5112–5120. doi: 10.1128/AEM.01043-13
Lahti, L., Shetty, S., Blake, T., and Salojarvi, J. (2019). Microbiome R Package (3.9) [Computer Software]. Available online at: http://microbiome.github.io (accessed May 2021).
Landrum, G. (2021). RDKit: Open-Source Cheminformatics (2021.03) [Computer Software]. Available online at: http://www.rdkit.org (accessed May 2021).
Lee, T., Siripattrawan, S., Ituarte, C. F., and Foighil, D. O. (2005). Invasion of the clonal clams: corbicula lineages in the New World. Am. Malacol. Bull. 20, 113–122.
Leis, E., Erickson, S., Waller, D., Richard, J., and Goldberg, T. (2019). A comparison of bacteria cultured from unionid mussel hemolymph between stable populations in the upper mississippi river basin and populations affected by a mortality event in the clinch river. Freshw. Mollusk Biol. Conserv. 22, 70–80. doi: 10.31931/fmbc.v22i2.2019.7080
Li, H., and Durbin, R. (2009). Fast and accurate short read alignment with Burrows-Wheeler transform. Bioinformatics 25, 1754–1760. doi: 10.1093/bioinformatics/btp324
Li, H., Handsaker, B., Wysoker, A., Fennell, T., Ruan, J., Homer, N., et al. (2009). The sequence alignment/map format and samtools. Bioinformatics 25, 2078–2079. doi: 10.1093/bioinformatics/btp352
Liu, X., Cao, Y., Ouyang, S., and Wu, X. (2020). Comparative analysis of gut microbiota diversity in endangered, economical, and common freshwater mussels using 16S rRNA gene sequencing. Ecol. Evol. 10, 12015–12023. doi: 10.1002/ece3.6796
Lockwood, J. L., Hoopes, M. F., and Marchetti, M. P. (2013a). “Modeling the geographical spread of invasive species,” in Invasion Ecology, 2nd Edn. ed. Wiley-Blackwell (Hoboken, NJ: John Wiley & Sons), 157–187.
Lockwood, J. L., Hoopes, M. F., and Marchetti, M. P. (2013b). “Establishment success: the influence of biotic interactions,” in Invasion Ecology, 2nd Edn. ed. Wiley-Blackwell (Hoboken, NJ: John Wiley & Sons), 129–155.
Lopes-Lima, M., Burlakova, L. E., Karatayev, A. Y., Mehler, K., Seddon, M., and Sousa, R. (2018). Conservation of freshwater bivalves at the global scale: diversity, threats and research needs. Hydrobiologia 810, 1–14. doi: 10.1007/s10750-017-3486-7
Lopes-Lima, M., Lima, P., Hinzmann, M., Rocha, A., and Machado, J. (2014a). Selective feeding by Anodonta cygnea (Linnaeus, 1771): the effects of seasonal changes and nutritional demands. Limnologica 44, 18–22. doi: 10.1016/j.limno.2013.07.001
Lopes-Lima, M., Teixeira, A., Froufe, E., Lopes, A., Varandas, S., and Sousa, R. (2014b). Biology and conservation of freshwater bivalves: past, present and future perspectives. Hydrobiologia 735, 1–13. doi: 10.1007/s10750-014-1902-9
Love, M. I., Huber, W., and Anders, S. (2014). Moderated estimation of fold change and dispersion for RNA-seq data with DESeq2. Genome Biol. 15:550. doi: 10.1186/s13059-014-0550-8
Lozier, J. D., Ols, C. N., Pitsenberger, C. A., Marshall, V. M., and Watkins, M. H. M. (2020). Partitioning of bee diversity at a small spatial scale in an urban arboretum. Southeastern Naturalist 19:22. doi: 10.1656/058.019.0103
Lymbery, A. J., Morine, M., Kanani, H. G., Beatty, S. J., and Morgan, D. L. (2014). Co-invaders: the effects of alien parasites on native hosts. Int. J. Parasitol.: Parasites Wildlife 3, 171–177. doi: 10.1016/j.ijppaw.2014.04.002
Marcon, E., and Hérault, B. (2015). Entropart: an R package to measure and partition diversity. J. Stat. Softw. 67, 1–26. doi: 10.18637/jss.v067.i08
McCauley, M., Chiarello, M., Atkinson, C. L., and Jackson, C. R. (2021). Gut microbiomes of freshwater mussels (Unionidae) are taxonomically and phylogenetically variable across years but remain functionally stable. Microorganisms 9:411. doi: 10.3390/microorganisms9020411
McDonald, D., Price, M. N., Goodrich, J., Nawrocki, E. P., DeSantis, T. Z., Probst, A., et al. (2012). An improved Greengenes taxonomy with explicit ranks for ecological and evolutionary analyses of bacteria and archaea. ISME J. 6, 610–618. doi: 10.1038/ismej.2011.139
McElwain, A. (2019). Are parasites and diseases contributing to the decline of freshwater mussels (Bivalvia, Unionida) BioOne 22, 85–89.
McMahon, R. F. (2002). Evolutionary and physiological adaptations of aquatic invasive animals: R selection versus resistance. Canadian J. Fish. Aquatic Sci. 59, 1235–1244. doi: 10.1139/f02-105
McMurdie, P. J., and Holmes, S. (2013). Phyloseq: an R package for reproducible interactive analysis and graphics of microbiome census data. PLoS One 8:e61217. doi: 10.1371/journal.pone.0061217
Parker, E. S., Newton, I. L. G., and Moczek, A. P. (2020). (My microbiome)Would walk 10,000 miles: maintenance and turnover of microbial communities in introduced dung beetles. Microb. Ecol. 80, 435–446. doi: 10.1007/s00248-020-01514-9
Parker, M. A., Malek, W., and Parker, I. M. (2006). Growth of an invasive legume is symbiont limited in newly occupied habitats. Diversity Distribut. 12, 563–571. doi: 10.1111/j.1366-9516.2006.00255.x
Parmalee, P. W., and Bogan, A. E. (1998). The Freshwater Mussels of Tennessee, 1st Edn. Knoxville, TN: University of Tennessee Press.
Pembleton, L. W., Cogan, N. O. I., and Forster, J. W. (2013). StAMPP: an R package for calculation of genetic differentiation and structure of mixed-ploidy level populations. Mol. Ecol. Resour. 13, 946–952. doi: 10.1111/1755-0998.12129
Pouil, S., Hills, A., and Mathews, T. J. (2021). The effects of food quantity, light, and temperature on clearance rates in freshwater bivalves (Cyrenidae and Unionidae). Hydrobiologia 848, 675–689. doi: 10.1007/s10750-020-04472-y
R Core Team (2020b). R: A Language and Environment for Statistical Computing (3.6.3) [Ubuntu 20.04.2 LTS]. Vienna: R Foundation for Statistical Computing.
R Core Team (2020a). R: A Language and Environment for Statistical Computing (4.0.3) [Computer Software]. Vienna: R Foundation for Statistical Computing.
Revelle, W. (2021). Psych: Procedures for Personality and Psychological Research (2.0.9) [Computer Software]. Available online at: https://CRAN.R-project.org/package=psych (accessed May 2021).
Richard, J. C., Campbell, L. J., Leis, E. M., Agbalog, R. E., Dunn, C. D., Waller, D. L., et al. (2021). Mussel mass mortality and the microbiome: evidence for shifts in the bacterial microbiome of a declining freshwater bivalve. Microorganisms 9:1976. doi: 10.3390/microorganisms9091976
Rochette, N. C., Rivera-Colon, A. G., and Catchen, J. M. (2019). Stacks 2: analytical methods for paired-end sequencing improve RADseq-based population genomics. Mol. Ecol. 28, 4737–4754. doi: 10.1111/mec.15253
Schartum, E., Mortensen, S., Pittman, K., and Jakobsen, P. J. (2017). From pedal to filter feeding: ctenidial organogenesis and implications for feeding in the postlarval freshwater pearl mussel Margaritifera margaritifera (Linnaeus, 1758). J. Molluscan Stud. 83, 36–42. doi: 10.1093/mollus/eyw037
Seebens, H., Blackburn, T. M., Dyer, E. E., Genovesi, P., Hulme, P. E., Jeschke, J. M., et al. (2017). No saturation in the accumulation of alien species worldwide. Nat. Commun. 8:14435. doi: 10.1038/ncomms14435
Segata, N., Izard, J., Waldron, L., Gevers, D., Miropolsky, L., Garrett, W. S., et al. (2011). Metagenomic biomarker discovery and explanation. Genome Biol. 12:R60. doi: 10.1186/gb-2011-12-6-r60
Shelby, N., Duncan, R. P., Putten, W. H., McGinn, K. J., Weser, C., and Hulme, P. E. (2016). Plant mutualisms with rhizosphere microbiota in introduced versus native ranges. J. Ecol. 104, 1259–1270. doi: 10.1111/1365-2745.12609
Shenhav, L., Thompson, M., Joseph, T. A., Briscoe, L., Furman, O., Bogumil, D., et al. (2019). FEAST: fast expectation-maximization for microbial source tracking. Nat. Methods 16, 627–632. doi: 10.1038/s41592-019-0431-x
Sousa, R., Antunes, C., and Guilhermino, L. (2008). Ecology of the invasive Asian clam Corbicula fluminea (Müller, 1774) in aquatic ecosystems: an overview. Ann. Limnol.-Int. J. Limnol. 44, 85–94. doi: 10.1051/limn:2008017
Stoeckel, S., Grange, J., Fernández-Manjarres, J. F., Bilger, I., Frascaria-Lacoste, N., and Mariette, S. (2006). Heterozygote excess in a self-incompatible and partially clonal forest tree species—Prunus avium L. Mol. Ecol. 15, 2109–2118. doi: 10.1111/j.1365-294X.2006.02926.x
US Geological Survey (2021). Nonindigenous Aquatic Species Database. Reston, VA: US Geological Survey.
Utermann, C., Blümel, M., Busch, K., Buedenbender, L., Lin, Y., Haltli, B. A., et al. (2020). Comparative microbiome and metabolome analyses of the marine tunicate Ciona intestinalis from native and invaded habitats. Microorganisms 8:2022. doi: 10.3390/microorganisms8122022
Vaughn, C. C., and Hoellein, T. J. (2018). Bivalve impacts in freshwater and marine ecosystems. Annu. Rev. Ecol. Evol. Syst. 49, 183–208. doi: 10.1146/annurev-ecolsys-110617-062703
Vaughn, C. C., and Spooner, D. E. (2006). Scale-dependent associations between native freshwater mussels and invasive Corbicula. Hydrobiologia 568, 331–339. doi: 10.1007/s10750-006-0210-4
Wegner, K. M., Volkenborn, N., Peter, H., and Eiler, A. (2013). Disturbance induced decoupling between host genetics and composition of the associated microbiome. BMC Microbiol. 13:252. doi: 10.1186/1471-2180-13-252
Weingarten, E. A., Atkinson, C. L., and Jackson, C. R. (2019). The gut microbiome of freshwater Unionidae mussels is determined by host species and is selectively retained from filtered seston. PLoS One 14:e0224796. doi: 10.1371/journal.pone.0224796
Williams, J. D., Bogan, A. E., and Garner, J. T. (2008). Freshwater mussels of Alabama and the Mobile Basin in Georgia, Mississippi and Tennessee. Tuscaloosa, AL: University of Alabama Press.
Keywords: freshwater biodiversity, invasive species microbiome, microbial source tracking, RADseq, species interactions, Unionidae
Citation: Chiarello M, Bucholz JR, McCauley M, Vaughn SN, Hopper GW, Sánchez González I, Atkinson CL, Lozier JD and Jackson CR (2022) Environment and Co-occurring Native Mussel Species, but Not Host Genetics, Impact the Microbiome of a Freshwater Invasive Species (Corbicula fluminea). Front. Microbiol. 13:800061. doi: 10.3389/fmicb.2022.800061
Received: 22 October 2021; Accepted: 21 February 2022;
Published: 04 April 2022.
Edited by:
Jinbo Xiong, Ningbo University, ChinaReviewed by:
Olga Aksenova, Federal Center for Integrated Arctic Research (RAS), RussiaEllen Decaestecker, KU Leuven, Belgium
Copyright © 2022 Chiarello, Bucholz, McCauley, Vaughn, Hopper, Sánchez González, Atkinson, Lozier and Jackson. This is an open-access article distributed under the terms of the Creative Commons Attribution License (CC BY). The use, distribution or reproduction in other forums is permitted, provided the original author(s) and the copyright owner(s) are credited and that the original publication in this journal is cited, in accordance with accepted academic practice. No use, distribution or reproduction is permitted which does not comply with these terms.
*Correspondence: Marlène Chiarello, marlene.chiarello@gmail.com