- 1Centre for Agricultural Bioinformatics, ICAR-Indian Agricultural Statistics Research Institute, New Delhi, India
- 2School of Interdisciplinary and Applied Sciences, Central University of Haryana, Mahendergarh, Haryana, India
Agricultural productivity is highly influenced by its associated microbial community. With advancements in omics technology, metagenomics is known to play a vital role in microbial world studies by unlocking the uncultured microbial populations present in the environment. Metagenomics is a diagnostic tool to target unique signature loci of plant and animal pathogens as well as beneficial microorganisms from samples. Here, we reviewed various aspects of metagenomics from experimental methods to techniques used for sequencing, as well as diversified computational resources, including databases and software tools. Exhaustive focus and study are conducted on the application of metagenomics in agriculture, deciphering various areas, including pathogen and plant disease identification, disease resistance breeding, plant pest control, weed management, abiotic stress management, post-harvest management, discoveries in agriculture, source of novel molecules/compounds, biosurfactants and natural product, identification of biosynthetic molecules, use in genetically modified crops, and antibiotic-resistant genes. Metagenomics-wide association studies study in agriculture on crop productivity rates, intercropping analysis, and agronomic field is analyzed. This article is the first of its comprehensive study and prospects from an agriculture perspective, focusing on a wider range of applications of metagenomics and its association studies.
Introduction
Metagenomics (community and environmental genomics), once described as a major demand in microbial analysis, is uncovered through methods that drive microbial community population studies on heterogeneity and complexity over time. The goal for any metagenomic study is to completely characterize a microbial community, i.e., “who’s present?”, “what are their actions?”. This helps to decipher the structure of the microbial community, the functional activity of each microbial member, and the intra-species heterogeneity information (Scholz et al., 2012).
The term “metagenomics” was coined in 1998 to capture the analysis knowledge of a set of similar but not identical organisms, as in meta-analysis, which is defined as the analysis of analyses (Handelsman et al., 1998). Stepwise advancement in metagenomics study includes proposal involving the cloning of DNA from environmental-related samples, phage vector study, and construction of metagenomic library from DNA. The metagenomics field was initially defined by constructing the libraries from prokaryotic organisms in seawater with 16S rRNA (Schmidt et al., 1991; Stein et al., 1996). Later, similar studies were reported using 16S rRNA from soil samples (Henne et al., 1999). Soil is considered the most challenging environment due to the size and diversity of the microbiome (Daniel, 2005). A study estimated one gram of microbial population in forest soil containing 4 × 107 prokaryotic cells (Richter and Markewitz, 1995) while cultivated soils and grasslands contained an estimated 2 × 109 prokaryotic cells (Paul and Clark, 1989). Later on, ‘metagenomic’ was termed as ‘the functional and sequence-based analysis of the collective microbial genomes of an environmental sample’ (Allen and Banfield, 2005; Sarangi et al., 2019).
The revolution of metagenomics analysis started with computational technology and advancement in sequencing. The decreasing cost of next-generation sequencing and increase in analytical applications have led to a major transformation in the field of the microbial world. Microbial community characterization is progressive using various sequencing methods, namely, Sanger, pyrosequencing, ABI-solid, 454, Illumina, assembled, and so on (Table 1). Metagenomics involves DNA extraction and cloning from a collection of microorganisms. It is emanated from inevitable studies that microorganisms represent a massive population probably in all environments on earth especially 16S rRNA analyses approach facilitates the detection of ample new microbial life lineages (Dhanjal et al., 2020a). Though 16s rRNA revolutionized the detection of the microbial community, metagenomics has led to a detailed study on environmental microorganisms in the context of ecology and physiology.
The need for metagenomics has gained significant concern among microbiologists due to earlier microbial techniques. It involves the growth of microbes in pure culture in the laboratory due to which many organisms might have missed or gone unnoticed during culturing. This cultivation bottleneck is overcome by metagenomics analysis by providing a relatively unbiased view of species richness, diversity, and their potential activity in the community population (Links et al., 2012). In general, a powerful combination of genome sequencing and bioinformatics analysis of data has transformed our understanding and knowledge about how organisms evolve, function, and interact with each other, their hosts, and with the environment, providing new channels of inquiries and advances for translational impact (Moreno-Indias and Tinahones, 2020). Garrido-Cardenas and Manzano-Agugliaro’s 2017 article on worldwide research in metagenomics shows an exponential rise every year in the study and application of metagenomics approaches from the beginning of the metagenomics era (Figure 1).
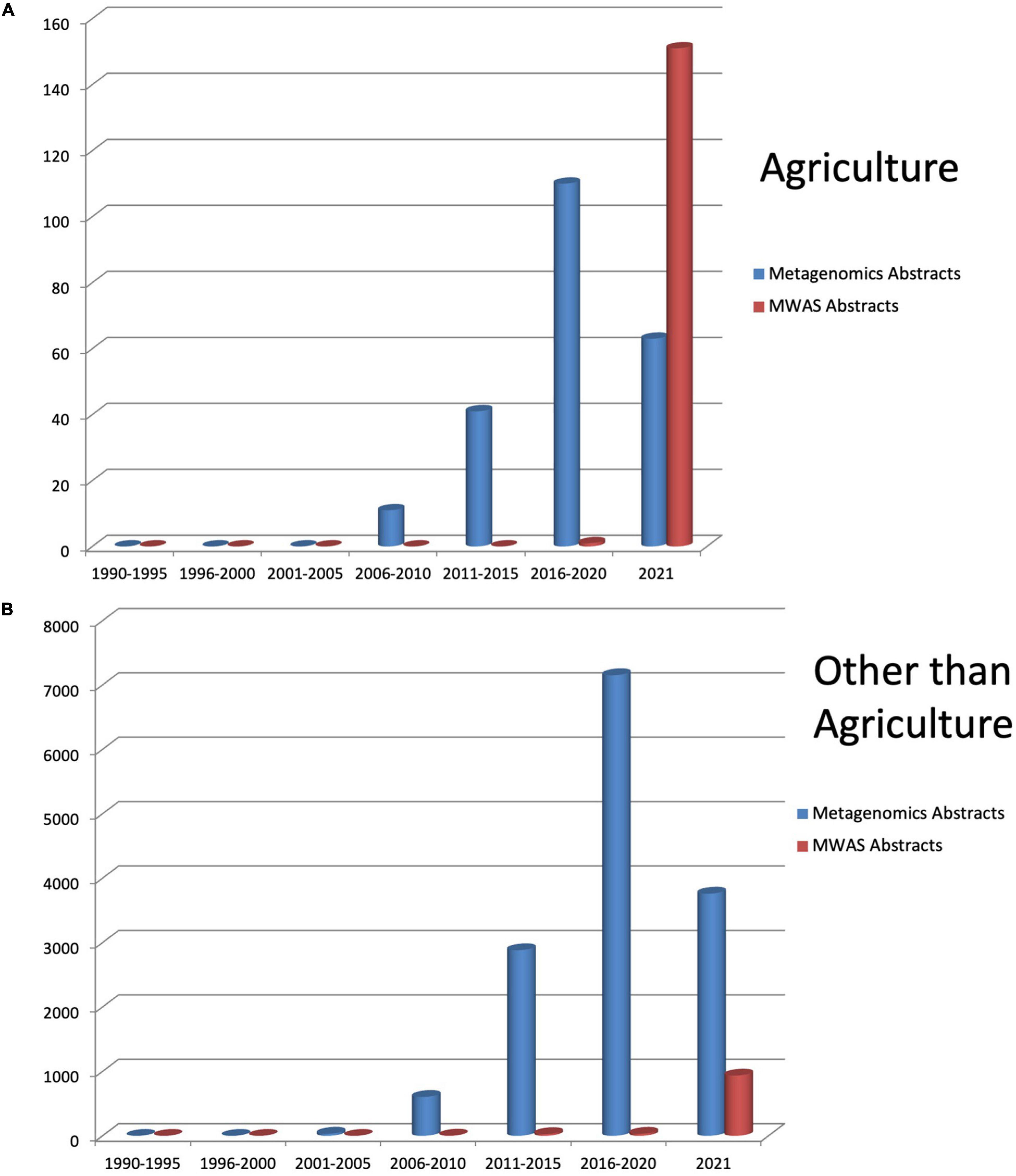
Figure 1. Literature reported in PubMed for metagenomics and metagenomics wide association studies from 1990 till 2021 in (A) agriculture and (B) other than agriculture.
Metagenomics mainly deals with the analysis of environmental samples from diversified sources like agriculture and seawater. The environment is a major reservoir of microbial species diversity and the complexity of this diversity depends on various factors like pH, temperature, water content, biotic activity, soil structure, and climatic variations. Although microbial communities are key players in the functioning of all ecosystems, their identification and understanding role of uncultivable microorganisms in natural ecosystems are uncertain (Tyson et al., 2004). Also, metagenomics helps in unlocking the uncultured microbial population present in an environment, especially, novel molecules related to therapeutic, biotechnological, and sustainable agricultural applications. With this versatility, in this review, we present its wider applications in agriculture, including livestock health and disease concerns, crop production and effect of environmental factors, such as abiotic stress, novel products identified through this approach, and its industrial applications.
Spatial Dimensions of Metagenomic Studies in the Biosphere
Metagenomics study is not limited to air (Núñez et al., 2016), water samples like aquatic (Doxey et al., 2015), freshwater (Edge et al., 2020), soil (Delmont et al., 2011), gut microbiota (Zhou et al., 2020), coral (Meenatchi et al., 2020), terragenome (Vogel et al., 2009), marine (Woyke et al., 2009), and oil-contaminated sediments from the Deepwater Horizon spill (Kimes et al., 2013). Based on human gut microbiota studies through metagenomics, a database atlas on gutMEGA was developed by Zhou et al. (2020). Various studies related to mass bleaching to understand the global impact on coral microorganisms (Meenatchi et al., 2020), iron intake trends by microbes prevailing on the surface ocean (Toulza et al., 2012; Meenatchi et al., 2020), ecobiomics projects to estimate and check soil health and quality of water (Edge et al., 2020), virus diversity and host interactions (Obiol et al., 2020; Schulz et al., 2020) have been undertaken. Recently, a study identified 1200 metagenome-assembled genomes from African cattle rumen and provided wider insights on the rumen functionality at extremely harsh conditions like food scarcity (Wilkinson et al., 2020). Another work estimated about 400 novel species and their ecological preferences in freshwater ecosystems (Rodriguez-R et al., 2020).
A recent study by Kwok et al. (2020) on zoonotic origin infectious diseases, stated the role of metagenomic Next-Generation Sequencing (mNGS) in identifying and characterizing novel etiologies and viruses from diversified samples in livestock, such as pig, cattle, poultry, and small ruminants (Kwok et al., 2020). Further, recent studies on human microbiota in disease association stated the impact and importance of microbial ecology of livestock in achieving better food quality and a healthy environment for the host (Frank, 2011). Features, such as plant fitness, soil biogeochemical properties, quality traits, and crop yield, play a key role in agriculture depend majorly on the microbiome of soil, plant, and livestock (Esposito et al., 2016). Miller et al. (2013) elaborated on the role of metagenomics in the public health sector for new pathogen detection (Miller et al., 2013). The study also commented on constantly evolving and zoonotic in origin microorganisms like H7N9 influenza and Middle East respiratory syndrome coronavirus (MERS-CoV). All these studies show the broad application of metagenomics in agriculture and other sectors (Taş et al., 2021; Figure 2).
Various Approaches and Tools for Metagenomics Data Analyses
Next-generation sequencing (NGS) is a powerful diagnostic tool in agriculture metagenomics due to its scope to target various unique signature loci of plant and animal pathogens as well as beneficial microorganisms from samples. The various approaches used in metagenomic studies include:
Amplicon-Based Methods
These are read-based reconstructions of the functional and taxonomic components of the metagenome. It is a targeted strategy, which involves a pre-sequencing PCR amplification to selectively target a taxonomic marker rRNA gene. Amplicon sequences are PCR products that specify a region within a gene and are specific to a particular organism. Strategies used through this method in bacterial characterization are 16S rRNA, RNA polymerase and heat shock protein 90, protozoan, and fungal identification with 18s rRNA gene (Iliev et al., 2012). In the case of viral population studies, viral RNA polymerase is used. In deep sequencing with next-generation technology, multiple different amplicons can be sequenced in a sample These sequences are assembled to a reference database to identify the conserved gene of a specific organism. This method identifies the organism along with its relative abundance. Overall, the sensitivity of this method increases in strain identification that is targeted to a particular species through amplicons. Though potential bias does exist in this method, such as the abundance ratio, due to artificially inflated counts of certain taxa during PCR amplification or with the use of universal primers which may mislead and may ignore the identification of certain organisms (Gonzalez et al., 2012).
Shotgun Metagenomics
It is a broader strategy that involves entire genome sequencing present in an environmental sample which facilitates a better understanding of the functions along with taxonomic profiling of the microbes (Enagbonma et al., 2019). The sequenced reads are compared to the reference database which is different and large in comparison with amplicon-based methods. This method is computationally intense, less biased, and reflects the better microbial community structure in a sample (Shakya et al., 2013). Moreover, this method has the power to differentiate the closely related species or strains (Miller et al., 2013). This method can also be used in the identification of stable microbial community structures in agricultural soil as well as novel ammonia oxidizers in fertilization (Orellana et al., 2018).
The general workflow of metagenomics includes: (i) processing of biological samples after sample collection and DNA/RNA extraction, (ii) sequencing, and (iii) bioinformatics analyses using various tools. Each step involving various approaches and tools is discussed precisely (Figure 3).
Processing of Biological Samples
Based on the purpose of the study, metagenomic analysis involves the extraction of the entire nucleic acid from a sample. Depending on the strategy, either DNA or RNA is targeted based on the microbial population to be studied, like, bacteria or viruses. In the case of virus studies in metagenomics, virions are extracted by removing cellular material followed by treatment of nucleases to remove non-viral nucleic acids and to extract viral nucleic acids intact within the nucleocapsid (Thurber et al., 2009). Technical issues related to very fewer amounts of nucleic acid in a sample can also be handled with library preparation kits for metagenomics, such as Nextera XT (Illumina, San Diego, CA, United States), random PCR, multiple displacement amplification, and higher sequencing depth (Loman et al., 2013).
Another important aspect in metagenomics is the collection of environmental sample associated data, i.e., metadata, that include temperature, pH, and salinity, as well as the collection of geographical data, such as global positioning system coordinates, depth, height, date of sample collection, extraction method, and clone library information (Field et al., 2008). Similarly, metadata with clinical samples varies like height, weight, sex, age, symptoms, heredity diseases, and so on. Databases for metagenomics also include the diverse degrees of metadata and also facilitate comparative communities study along environmental, longitudinal, or spatial gradients (Seshadri et al., 2007).
Sequencing
Sequencing is a crucial step that depends on various factors, such as samples being sequenced, sample size and cost. Various sequencing platforms available are discussed in Table 1. The most commonly used sequencing platforms in metagenomics analysis are Illumina and 454 pyrosequencing.
Bioinformatics Analysis
The computational analysis depends on sequencing methods, which use two main approaches in genome reconstruction: (i) Assembling of reads, followed by taxonomic and functional classification: Shotgun sequencing (ii) read-based reconstruction method: Amplicon sequencing. Due to a wide study on bacterial 16S rRNA and fungal ITS amplicon sequencing and whole sample sequencing by shotgun metagenomics, bioinformatics algorithms and tools are specially designed and developed. This plays a significant role in understanding diverse microbes inhabiting a varied environment.
Computational Approaches
Various approaches for reference and taxonomic profiling are implemented during sequence data analysis. In the case of OTU’s mapping for amplicon data, based on sequence similarity, three approaches are implemented, which are the following: (a) reference-based methods, which include mapping to reference databases like Greengenes and Silva database; (b) de novo OTU clustering method, which is for those data for which reference database match was very minimum or for those who rely on the de novo method (like UPARSE and UCLUST); (c) hybrid approach, which generally is initiated with reference mapping and unmapped sequences are further mapped by de novo clustering method, for instance, SortMeRNA (Edgar, 2013).
Databases
Various databases are developed and used as reference datasets during the taxonomic classification of the sequence data. The most common databases are Ribosomal Database Project, Greengenes, SILVA, and UNITE database (Pruesse et al., 2007; Cole et al., 2009; Abarenkov et al., 2010). Specific pipelines for the identification of any specific category of microbes are also developed. For instance, identification of specific fungal species from ITS data includes CloVR-ITS, Clotu, PIPITS, and Plutof (Abarenkov et al., 2010; White et al., 2013; Gweon et al., 2015).
Toolkits
Some of the frequently used bioinformatics toolkits in amplicon data analysis include QIIME (Caporaso et al., 2010), BioMaS (Fosso et al., 2015), and MOTHUR (Schloss et al., 2009). These toolkits facilitate multiple analyses at each step, starting from various kinds of sequence data preprocessing, like, denoising, merging of pair-end data, binning, demultiplexing, error correction, barcode-type analysis (Semenov, 2021) to diversity estimation. DADA2 algorithm is a recent pipeline for taxonomic identification, which uniquely produces amplicon sequence variant analysis rather than operational taxonomic units (OTU) (Callahan et al., 2016).
Workflow management systems available for genomic and metagenomic data where the users can apply the customized features and can build analysis and share data, including CAMERA (Seshadri et al., 2007), Galaxy (Giardine et al., 2005), and Ergatis (Orvis et al., 2010). Galaxy metagenomics toolkit contains six tools: fetching taxonomy, summarizing taxonomy, drawing phylogeny, finding of diagnostic hits, classification of diagnostic hits, and the Poisson two-sample test. Windshield splatter analysis is one of the first studies on metagenomic analysis using the NGS data analysis pipeline within the Galaxy workflow management system. Currently, there are many modular pipelines developed using such workflow systems.
There also exist stand-alone data analysis packages, developed using open-source tools by the Nanopore community, like Oxford Nanopore Technologies (ONT) cloud-based What’s In My Pot (WIMP) software, and MinION Detection Software (MINDS) for rapid species identification. Several bioinformatics applications have been developed implementing ONT’s framework for long reads’ analysis (Deshpande et al., 2019).
Tools
Computational tools and algorithms differ depending on the sequencing of data like amplicon-based or shotgun. In the case of shotgun data analysis, different software is used at different steps. Metagenome assembly is supported by the following algorithms starting from the traditional approach that includes overlap-based assembly and the most frequently used Kmer approach. Others include apriori algorithm, association rule mining algorithm, a binning algorithm like MaxBin 2, SPHINX, MetaCluster 5, sequence classification algorithm, and so on (Tandon et al., 2016; Saha et al., 2019). Classical metagenome gene predictors include Fgenesb and Critica/Glimmer which were evaluated by simulated data sets in a study by Kunin et al. (2008).
The shotgun based analysis is performed in various steps and tools used are the following: (i) assemblers, such as SOAP, Ray Meta, Snowball, MetaVelvet, MetaSPAdes, Meta-IDBA, MEGAHIT (Boisvert et al., 2012; Luo et al., 2012; Nurk et al., 2013; Li et al., 2015; Gregor et al., 2016); (ii) Binning of sequence fragments can be processed by taxator-tk, MaxBin2, Kraken, PhyloPytiaS+, Metaphlan, MEGAN and MetaBAT (Wood and Salzberg, 2014; Wu et al., 2014; Dröge et al., 2015; Kang et al., 2015; Gregor et al., 2016; Huson et al., 2016); (iii) metagenomics annotation and classification includes MetaGeneMark, PRODIGAL, KEGG, TIGRFAMs, COG, PFAM, eggNOG, and SEED (Overbeek et al., 2005; Zhu et al., 2010; Kanehisa et al., 2014; Powell et al., 2014; Garrido Oter, 2018).
Tools developed are even specific in mapping and characterization of organism type, like bacterial and archaeal annotations implemented by the functional annotation and gene calling pipeline, such as the command-line cg-pipeline and Ergatis web-based workflow management system. The electronic probe Diagnostic Nucleic acid Analysis (EDNA) tool utilizes NGS data for the detection of eukaryotic plant pathogens like fungi and oomycetes (Espindola et al., 2015). Similarly, virus-specific workflows are Viral MetaGenome Annotation Pipeline that was developed by using knowledge of specialized databases, such as mobile genetic elements collections and environmental metagenomes, to enhance the identification, classification, and functional analysis of viral gene products (Lorenzi et al., 2011).
Online servers for the study of metagenomics data uploaded by users can be comprehensively analyzed with MG-RAST, CAMERA, and IMG-M (Meyer et al., 2008). They also provide functional annotations and pathway reconstruction and facilitate the user for depositing, analyzing, sharing, both in public and private mode and visualization of the data in a single platform. All these resources help the researchers without access to high-performance computing facilities.
Comparative metagenomics, as its name indicates, is for multiple metagenome comparisons among different environments based on taxonomical and functional assignments in another important part of metagenome study. The first comparative metagenomics study introduced the knowledge of a gene-centric view of different environments (Tringe et al., 2005). Various studies on comparative metagenomics include Daphnia symbionts, modeling ecological drivers in marine viral communities, the role of microbes in human biology, poly-microbial black band disease of corals, biogas producing microbial communities, organic management, etc. (Meyer et al., 2017). This comparison platform will act as a tool in gaining a scientific understanding of microbial communities related to environmental perturbations. METAREP is one such tool that facilitates comparative metagenomics analysis along with statistical analysis like principal component analysis (PCA), non-metric multidimensional scaling (NMDS), and multivariate analysis1. Similarly, Integrated Microbial Genomes (IMG) system also serves as a comparative analysis resource facilitating the annotation and visualization of data2.
Metagenomics-wide association studies (MWAS) are a broader branch of metagenomics of recent interest. With its wider application, this study is successful but its use and implementation are still in its infancy stage because of various challenges. One such challenge focuses on the choice of tools, methods, and workflow (James et al., 2019). The study has also elaborated on the classification of tools in MWAS based on their analysis that includes phylogeny, non-phylogeny (statistical), hybrid tools (both statistical and phylogenetic methods), and machine-learning tools. Tools and software available for MWAS studies include the following:
(a) Command-line software: SEER (Lees et al., 2016), bugwas (Earle et al., 2016), Phenotype Seeker (Aun et al., 2018), PySEER (Lees et al., 2018), and HAWK (Rahman et al., 2018) and
(b) Graphical user interface software: Scoary (Brynildsrud et al., 2016) but also supports command-line function (Table 2).
Metagenomics and Sustainable Agriculture
Agriculture challenges are the major concern in terms of the growing demand for food and bioenergy all over the world which has to be effectively addressed (Tilman et al., 2011). Environmental threats, including biodiversity loss, climatic change, water and land pollution, the low organic content of the soil, and degradation, have to be balanced accordingly. Many studies and strategies are planned in agricultural ecosystem biology but still need to be deciphered to gain deeper awareness and to meet the growing needs and negative impacts of the environment (Power, 2010). Soil fertility is a key player and depends on various factors, like, water availability and contamination, elements fluxes in soil like carbon, nitrogen, and phosphorus, and climatic conditions. But the most crucial is soil microorganisms’ dynamics. Under such cases, metagenomics will help in resolving challenges in agricultural sectors as it considers biotic and abiotic factors associated with it (Scholes and Scholes, 2013). Furthermore, the use and demand for biopesticides and biofertilizers in agriculture and advancement in sequencing and metagenomics analysis have led to the discovery of beneficial microbes for agriculture (Park et al., 2013).
Soil microbiomes are largely responsible for the biogeochemical cycles that support life on earth where they mediate the nitrogen cycle, carbon, and sulfur cycles, as well as in the transformation of oxidative and reductive states of metals like iron and mercury. They particularly influence the health of plants and their dependents by providing nutrients and vitamins, influencing developmental processes, and protecting the host from attack from virulence factors (Handelsman, 2003). All these show the positive phase of the microbial population with a balanced natural system. If this system is disrupted, it can lead to harmful effects like acid mine drainage, exposure of coal or copper mines to oxygen, and disruption of the earth leading to the production of high damaging waste. Most of these factors are currently addressed on a broader scale with metagenomics for instance soil microorganism dynamics is studied through diversity and functional analysis of soil microbial populations (Fierer et al., 2013).
Rhizosphere harbors powerful microbiomes which play a significant role in maintaining plant health and productivity, nutrient cycling, and enhancing soil fertility. These microbiome complexities vary by plant species, soil type, host genotype, and land tillage system. They are the most complex ecosystem on earth and the rhizosphere microbiomes of various agricultural crops and their biocontrol characteristics have been studied (Subrahmanyam et al., 2020; Alawiye and Babalola, 2021). These studies and evidence reflect the importance of metagenomics application as a tool in unlocking the knowledge of biota that are influenced by abiotic factors in sustainable agriculture. Major challenges currently faced in agriculture in maintaining a balance between sustainability and increasing fiscal growth rate are the environmental threats, social threats, economic threats, and other factors like global warming, urbanization, and pollution (Gupta et al., 2018; Figure 4).
Soil metagenomics advancement stimulates new soil conceptions as “living” and “laboring” (Granjou and Phillips, 2019). It is also stated as a new agroecological revolution period with the use of biota for soil functioning. Overall soil metagenomics helps in reconfiguring biopolitical and bioeconomic relations and it may influence future (micro) biopolitical studies.
Dimensions of Metagenomics Data in the Agricultural Ecosystem
The major perspective of the present review is to understand metagenomics applications in agriculture, including: (i) livestock health and disease, (ii) crop production and its environmental impact mainly abiotic stress, and (iii) design and development of novel products and their industrial applications. Among many such studies reported in the literature, we are presenting a few studies in Table 3 of this decade focused mainly on the agriculture sector.
Antibiotic-resistant genes in soil are majorly produced by the action of complex antibiotic-producing microbes which include aminoglycoside acetyltransferase, ADP-ribosyl transferase, ribosomal protection protein, aminoglycoside 6-adenyltransferase, transporters, and other antibiotic-resistant determinants and their resistant mechanisms are identified through functional metagenomics approach. The distribution of antibiotic-resistant genes in agricultural and non-agricultural metagenomic samples has been investigated and it was observed that a large percent of antibiotic resistance genes was produced by diverse bacterial communities in agricultural and gastrointestinal-associated metagenomes compared to marine and Antarctic samples (Durso et al., 2012).
Studies on the microbial establishment in the developing rumen are said to have implications in farm animals ecologically and physiologically that ultimately lead to productivity efficiency in mature animals. Metagenomic studies on fish gastrointestinal communities are focused on the identification of a healthy microbiome and its role in vertebrate health (Tarnecki et al., 2017).
Applications of Metagenomics in Agriculture
Pathogen and Plant Disease Identification
Plant disease control, identification and characterization of the microbes, and prevention are successful and efficient with early detection of plant disease outbreaks and accurate diagnostic methods. Plant pathogens and pests have significant economic, ecological, and evolutionary consequences in agricultural ecosystems. Symptoms of attack of pathogens on plants are the major factors that help in diagnosing a disease by a plant pathologist. However, this is not a highly desirable method, as there is a gap existing in strain-level/species identification of the causative agent. For instance, in tomatoes “bacterial speck disease symptoms” are caused by different species of Pseudomonas syringae pathovar (pv.) tomato (Pto) (Cai et al., 2011). Similarly, “bacterial spot disease symptoms” on tomato leaves are caused by genus Xanthomonas but involve four different species (Jones et al., 2004). Another significant fact is that during diagnosis and crop rotation, different species of the same pathogen may have varied host ranges. In P. syringae pathovar (pv.) tomato (Pto), strain DC3000 could affect not only tomatoes but also leafy greens by existing in weeds of the Brassicaceae family (Yan et al., 2008).
Various methods are used in plant disease diagnosis for species identification, including gene sequence-based techniques with pure culture, 16S rRNA gene method but involve low resolution, whole-genome sequencing using pure culture, and antibody-based assays, such as ELISA (El Sheikha and Ray, 2014). However, limitations associated with these methods are a lengthy pathogen identification protocol to obtain pure culture and prior knowledge of pathogens. In the current scenario, all these limitations are overcome with metagenome sequencing and identification methods in agriculture.
Metagenomics in pathogen diagnosis has the potency to target multiple unique signature loci of pathogens in an infected plant. This approach has already been used to detect unknown pathogens in different organisms, including mammals, plants, and insects (Adams et al., 2009). Also, various farming practices influence the taxonomic diversity of the phyllosphere microbial community leading to unique signatures (Khoiri et al., 2021). Nevertheless, the protocol of this method should be targeted toward precise identification of causative agents as this involves DNA extraction, which may contain host sequences, other microbe sequences apart from pathogens of interest. Electronic-probe Diagnostic Nucleic acid Analysis or EDNA overcomes this with simulated metagenomes.
The bacterial tomato pathogen identification at the strain-level was achieved by metagenomics (Mechan Llontop et al., 2020). Fusarium wilt is a common vascular wilt fungal disease in many plants. In banana, a study with fusarium wilt infected fields was conducted by analyzing endophytic communities, which led to the recognition of bacterial communities having an impact on disease development, including Flavobacteriales (Kaushal et al., 2020). Simulated metagenome data analysis using EDNA was done for detecting fungal and oomycete plant pathogens (Espindola et al., 2015). Soil may also act as disease suppressive agent in agriculture by protecting the crops from harmful microbes. Mendes et al. (2011), with PhyloChip-based metagenomics, diagnosed key bacterial taxa and genes comprising 33,000 bacterial and archaeal species from soil to be involved in the suppression of fungal root pathogens (Mendes et al., 2011). This soil microbial consortia act as superorganisms in controlling and protecting plants and crops from harmful invading microbes.
Plant disease management can be made successful as in medicine through the transfer of microbiome by mixing disease suppressive soils with disease conducive soils (Gopal et al., 2013). Blackroot rot disease of tobacco in suppressive and conducive soil with rhizobacterial community study resulted in novel bacterial taxa that help as indicators of disease suppressiveness. Bacterial taxa with plant beneficial properties in disease suppressive soils were Pseudomonas, Azospirillum, Gluconacetobacter, Comamonas, Burkholderia, and Sphingomonadaceae, whereas Mycobacterium, Rhodobacteraceae, Bradyrhizobium, Rhodospirillum were more frequent in the conducive soil (Kyselková et al., 2009). Another global problem faced by potato growers annually is potato common scab caused by Streptomyces species. A similar study on suppressive and conducive soil identified various bacterial genera (predominantly Bacillus genus) in the disease-conducive soil (Rosenzweig et al., 2012).
Disease Resistance Breeding
Application and manipulation of plant-microbe interactions and microbe-microbe interactions with a strong scientific basis are being extensively used in agro-ecosystems. Their roles in improving plant health and productivity are a well-recognized in various studies. Microbial community engineering through plant breeding is another way of harnessing plant-microbe interactions (PMI). These interactions also provide insights in improving resistance breeding against diseases in crops, which is a promising approach in achieving sustainable agriculture (Rubiales et al., 2015).
Plant resistance breeding usually targets developing cultivars with the best performance over diversified environments. However, it is a very challenging task as holobiont varies in different environments. A study on lupine cultivars for resistance against Fusarium avenaceum in Canada was not successful, whereas it was found effective in Denmark and Germany (Chang et al., 2014). Therefore, it is also attributed to geographical location and environmental conditions. These studies show that selection under targeted environmental conditions will help in producing resistant cultivars. In grain legumes, PMI plays an important role in resistance breeding against root diseases (Wille et al., 2019). This is based on the hypothesis that plant functioning is mediated by plant metagenomes that encompass both internal and external microbes (Berg et al., 2017). Plants and plant metagenomes are comprehensively termed as holobiont necessary for plant-resistant breeding strategies (Wille et al., 2019).
Metagenomics application under the influence of resistance breeding in Phaseolus vulgaris (common beans) against fungal root pathogen Fusarium oxysporum (Fox) with rhizosphere microbiomes was conducted. As rhizosphere microbiomes are involved as the first line of defense against root infections and diseases, the use of metagenomics in rhizosphere engineering has also been studied (Hakim et al., 2022). This study identified microbes, including Pseudomonadaceae, bacillaceae, solibacteraceae, and cytophagaceae, to be predominant in resistant cultivars of common beans. Metagenome analyses further explained that unique functional traits like protein secretion systems and biosynthesis genes of antifungal phenazines and rhamnolipids were dominant in the rhizobacterial community in Fox-resistant cultivars (Mendes et al., 2018).
In another study, comparative analyses of rhizosphere metagenomes from resistant tomato variety, Hawaii 7996 and susceptible variety, Moneymaker to soil-borne pathogen Ralstonia solanacearum revealed the abundant presence of flavobacterium genome in resistant variety, following which rhizosphere microbiota from resistant plants when transplanted suppressed disease symptoms in susceptible plants (Kwak et al., 2018). All these pieces of evidence prove the impact of metagenomics in resistance crop breeding.
Plant Pest Control
Microbes act as prominent biocontrol agents in the following ways: inhibition of pathogenic genes by impairing the pathogens with quorum sensing, production of antagonistic molecules in plant tissues, influence on plant defense mechanism through hormones production. There are also microbes that support pathogens through their mutual relationship like the Enterobacteriaceae family (Erlacher et al., 2014). So in the biocontrol mechanism microbes having negative benefits on pathogens can be used, on the contrary, microbes showing positive effects with pathogens can be targeted to break the symbiotic relationship in plant protection methods (Berg et al., 2017).
Plant microbiota act as substitutes to environmentally unfriendly pesticides and agrochemicals. It also mediates significant functions, including fitness, nutrient availability, pathogen or pest control, and stress tolerance. Microbial population manipulation in the rhizosphere is directly associated with crop health and productivity of crops. This microbial population is studied extensively using metagenomics as a prime tool. These strategies studied with metagenomics will be helpful for the control of plant diseases (Parakhia and Golakiya, 2018). Plant-associated metagenomes help in recognizing a large number of signatures involved in these interactions, but the traits produced through these interactions are yet to be extensively studied. Metagenomics the emerging omics technology shows that analysis of the microbiome and its function may provide insights and a paradigm shift in deciphering the holobiont functioning related to health and associated biocontrol measures.
The recent study by Poudel et al. (2019) on tomatoes with a metagenomics approach observed rootstock genotypes on endosphere and rhizosphere microbiomes which have the potential to select a candidate for the biocontrol process (Poudel et al., 2019). Organic crops are potentially enriched with their own biocontrol agents. Schmid et al. (2011) study on the grapevine-associated microbiome in organic and conventional fields evidenced the role of a biocontrol agent Aureobasidium pullulans, as well as the copper-detoxifying fungus in the organic field (Schmid et al., 2011).
Metagenomics study on disease and surviving plant microbiota for future plant health demonstrated that surviving plant microbiomes are linked with specific rare taxa having pathogen-suppressive tendencies or else involved in encoding antimicrobial compounds, these taxa included bacterial microbes like Pseudomonas and Bacillus (Wei et al., 2019). Fusarium Head Blight (FHB) is a devastating global disease caused by Fusarium graminearum, a fungus affecting small grain cereals. It is devastating, as it poses threat related to health diseases by producing mycotoxins which affect yield and seed germination. Therefore, a project using the metagenomics approach is proposed to interpret the wheat head microbiome and its dynamics during FHB disease development3. It also has the potential to reveal growth-promoting microbes or new pathogen antagonists, thereby aiding in the identification of novel biocontrol promoters.
Pest management strategies also involve microbial and viral enzymes like chitinases for use as bio-pesticides and have promising fungicidal, nematicidal, and insecticidal activities. Unraveling novel chitinases for use as a biocontrol is possible with a powerful tool, such as metagenomics. Metagenome-sourced chitinases have been studied in terrestrial and aquatic ecosystems with their distribution varying among abiotic factors. One such study is the identification of Chi18H8b, a novel chitinase with antifungal activity against Fusarium graminearum and Rhizoctonia solani (Berini et al., 2017). These novel metagenome-sourced chitinases were extracted from wastewater contaminated soil, chitin-enriched soil samples, pig feces, and sediments (Cretoiu et al., 2015). Metagenomics contribution in biocontrol agent identification is promising and expected to show greater application in near future.
Weed Management
With the global increase of herbicide-resistant weed communities, there is a need for a strategy targeting the different mechanisms of action. Development of metagenomics application for natural product discovery paves way for approach in weed management research which leads to innovative control strategies in cropping and farming systems. This approach based on high-throughput technology can accelerate the rate and scale of herbicide development in the agriculture and pest management sectors.
Transformation of traditional methods with metagenomics techniques in the discovery of novel and natural products like enzymes and antibiotics from microbes having antimicrobial and phytotoxic effects are increasing (Dhanjal et al., 2020b; Wani et al., 2022). Metagenomics-based functional screens offer a natural drug discovery potential for the segregation of weed suppressive compounds from microbes that targets the wider plant range. The recent focus on bioherbicide research is targeting major crops and turfgrass landscapes.
Metagenomics-based functional screens in weed management involve two approaches (i) novel herbicide isolation and (ii) herbicide resistance genes identification. Lucas (2011), Genetically modified crops resistant to herbicides through recognition of herbicide resistance genes from soil microbes were studied (Lucas, 2011). Another study was conducted, leading to the discovery of glyphosate resistance and glyphosate degrading abilities using microbes (Staub et al., 2012). It is estimated that the finding of a novel antibiotic in actinomycetes may involve hundreds of clone screening, but metagenomics along with robotics techniques may speed up the acceleration process. A similar study has been done on cellulase with hundreds of clone libraries (Mewis et al., 2011).
Although, the number of studies linking metagenomics in weed management is limited there are many promising studies conducted to show its potential applications. For example, application of metagenomics and metatranscriptomics approaches in studying seed microbiome population and function for identification of novel bioherbicides (Müller-Stöver et al., 2016) and use of metagenomics functional screening approach for microbiome selection on small plants, such as duckweed (Lemna minor L.), algae or leaf spot assays, to test success rate, following a large-scale screening in the greenhouse (Kao-Kniffin et al., 2013). Metagenomics along with the metabolomics approach helps in the identification of novel herbicide compound discovery. Numerous antibiotics were obtained from Streptomyces spp. using the metagenomics approach have the ability to suppress the weed. The role of new sequencing technologies for weed establishment and weed prevention along with the exploitation of the knowledge in search of new biocontrol agents against weeds based on soil and plant microbial communities is well reported (Trognitz et al., 2016).
Abiotic Stress Management
Microbes associated with plants/crops, including soil microbes and rhizosphere microbiomes, contribute to a wide range of functions that are needed for plant productivity, like mineralization of soil organic matter, nutrient cycling, stimulating disease resistance, and responding to abiotic stresses like drought, salinity, pollution, etc. There is extensive information about the ability of specific soil microbes to influence abiotic stress tolerance in plants, for example, the impact of rhizosphere microbiome on some plant species survival under extreme stress conditions (Jorquera et al., 2012).
Abiotic stress players are non-living components but pose a great threat and unfavorable conditions and challenges in agriculture. Plant response depends on the type of stress like extreme temperature, drought, salinity, waterlogging, soil pollutants, hazardous compounds, such as heavy metals, and the extent to which the condition is affecting the plant. They are the foremost limiting factors in crop productivity. Crop plants need to cope with these adverse external pressures of the environmental conditions with their innate biological mechanisms, failing which plant growth, development, and productivity suffer (Meena et al., 2017).
To perceive the cumulative role of these multiple interactions in plants along with its ability in abiotic stress management (ASM), a broader scale study, such as metagenomics, is needed. Zolla et al. (2013), work on drought stress conditions in Arabidopsis thaliana using the pyrosequencing approach reported the robust set of soil microbes having an ability to sense abiotic stress and increase plant biomass production along with reduced expression of drought response marker genes (Zolla et al., 2013). A similar study on wheat rhizosphere for abiotic stress management reported the wider and diversified role of metagenomics in ASM where metagenomics of wheat along with its application in climate-resilient genotypes development is elaborated (Ahlawat et al., 2018).
Soil pollution is another important abiotic feature associated with plant damage and disease susceptible environments. Response to polycyclic aromatic hydrocarbons pollution using 16S rRNA gene pyrosequencing approach was studied on Trifolium (Kawasaki et al., 2012). Pollutants like detergents, oxidants, and glucose also pose severe losses to agriculturists. The promising use of functional metagenomics in unrevealing proteins that are tolerant to such pollutants from agricultural soil is well reported. Alkaline β-glucosidases enzymes showing high resistance toward severe detergents, glucose, and oxidants are identified by this technique.
In another study on proteins like ACC-deaminase, genes involved in stress alleviation, using metagenomics libraries reported the functioning of acdS operon from uncultivated endophyte in potato (Nikolic et al., 2011). Salt-tolerant genes from pond water metagenomics libraries can be used in developing salt-tolerant recombinant microbes and transgenic plants. Comparable study on acid mine drainage led to the identification of genes that tolerate low temperatures like cold-shock proteins, anti-freeze protein, pH homeostasis, and compatible solutes production pathways using the metagenomics approach (Liljeqvist et al., 2015). Functions involved in improving plant stress resistance activity like detoxification of ROS and quorum sensing can also be identified through the metagenomics approach with endophytic bacterial residents of rice roots (Sessitsch et al., 2012).
A novel approach using endophytic bacteria to alleviate various stress conditions is gaining significance in agriculture. Kunda et al. (2018) study on bacterial endophytes from roots of rice plants grown in the coastal saline zone identified the microbial genera with plant growth-promoting potentials in a high salt environment and also proposed the use of these microbial genera for designing cultivation strategies in saline conditions (Kunda et al., 2018). Methe et al. (2017) study on functional genes that differentiate maize phyllosphere metagenomes in two distinct conditions: drought and well-watered identified unique taxa involved with potential growth-promoting traits (Methe et al., 2017).
Post-harvest Management
Post-harvest loss is one of the major concerns worldwide. It is a wider sector, covering a long chain of processes from production in a field to food in consumer plates (Buchholz et al., 2018). It includes several steps, such as harvesting, protection, preservation, preparing, packaging, transportation, and marketing. It is stated by the Food and Agriculture Organization (FAO) that ‘Hunger is still one of the most demanding challenges, still the agriculture throughout the world is producing more than enough food.’, but a huge amount of food produced gets lost on its way from the field to consumer. It is also estimated by FAO that about 15--50% of food produced in developing countries is lost after harvest due to various abiotic factors like drought, high temperature, huge rainfall, the wrong procedure of harvesting, physical damage, or contamination by microbes4.
Post-harvest food loss is defined, as measurable qualitative and quantitative food loss along the food process chain, beginning from the time of harvest until its consumption. Food losses are attributed to loss during harvest, transport, loss in quality due to undesired microbial growth or pathogen attack, rancidity, water loss, etc. (El Sheikha and Xu, 2018). Post-harvest loss in potato production in Switzerland showed that about 50–55% losses are due to pathogen attack, water loss, saccharification, etc. (Willersinn et al., 2015). However, plant microbiota dynamics have a symbolic role in post-harvest food loss by influencing the storage of crops. However, many studies on plant microbiota are focused on crop productivity, but still, the plant microbiome’s role and impact on processing, packaging, storing, and marketing is mostly unexplored.
Pathogen-induced post-harvest crop loss caused by microbial activity, including bacteria and molds, is obvious. Plant microbiomes consist of complex microbial interactions of potentially mutualistic, commensal, and pathogenic microbes colonizing as in crop plants. The dynamics and role of the bacterial community of potato tubers during infection with soft rot pathogen named Pectobacterium atrosepticum was studied (Kõiv et al., 2015). The soil microbial communities on flowering phenology and reproductive fitness of Boechera stricta, a wild relative of Arabidopsis were tested and it was concluded that the soil microbiome is the possible driver contributing at flowering time, to differential selection observed between habitats through the metagenomics approach (Wagner et al., 2014).
The Vitis vinifera L. cv. Corvina grapes are popular for the production of unique wines, like Amaron. This unique feature is strongly linked to the post-harvest grape withering process. With the use of whole metagenome sequencing, insights into the microbiome of Corvina withered berries revealed pertinent variations attributable to post-harvest withering conditions. This knowledge and management of the withering process in Corvina will have an impact on winemaking (Salvetti et al., 2016). Meta-omics technologies involving meta-genomics, meta-transcriptomics, meta-proteomics, and meta-metabolomics are at the earlier stage in post-harvest studies. This omics approach will certainly bring revolution in our understanding and knowledge of post-harvest biocontrol systems, post-harvest physiology, and foodborne pathogens (El Sheikha et al., 2018).
An important feature that has to be noted is microflora associated with different parts of a fruit/vegetable should be considered when preparing strategies for biocontrol systems involving post-harvest disease management. A work by Abdelfattah et al. (2016) reported the diversity in fungal microflora among various parts of harvested apples and as well as in farming techniques like organic or conventional methods (Abdelfattah et al., 2016). Mazzola and Freilich (2017) also emphasized the need for meta-omic studies to understand the potential and functional aspects of the microbial community in post-harvest management strategy (Mazzola and Freilich, 2017).
Discoveries From Metagenomics in Agriculture
Metagenomics as a tool resulted in many discoveries. First, bacteriorhodopsin was discovered due to metagenomics as a novel gene product. Discoveries on microbial rhodopsin through functional metagenomics studies were stated (Pushkarev et al., 2018). Heliorhodopsins, which include diverse members of the rhodopsin family, are detected from microorganisms distributed globally. Further, novel biomolecules with antimicrobial activity and new proteins of known families like DNA polymerase and RecA were also identified. Microbial communities are agents and insight providers on biogeochemical cycling, energy and nutrient cycling, and metabolic potential. Multi-metagenome complete genome recovery from metagenome has led to link genomic analysis, which includes observations on gene function, genome structure, lateral gene transfers, and population genetics.
Metagenomics is probably used as a tool in the pathogen detection of agriculture. Synthetic metagenomics involves the identification of novel genes through mining sequence databases and metagenomics data for the gene of interest, followed by its chemical synthesis. This approach has led to the identification of the enzyme, methyl halide transferase, which is prominently used in biofuel production in the agriculture industry (Culligan et al., 2014). Biosurfactants have been identified through metagenomics studies, which are used as emulsifiers and detergents in various sectors, including pharmaceutical, agricultural and ecological functions (Thies et al., 2016).
Source of Novel Molecules/Compounds
The metagenomics approach has led the way in novel discoveries of genes, enzymes, and antibiotics. Functional metagenomics will accelerate the rate of discovery of new molecules from the microbiome community and it paves ways to investigate natural products from uncultured microbes. Metagenomics has been used as routine tools for novel natural product discovery during larger-scale exploration of bacteria coupled with genome engineering of naturally privileged heterologous expression hosts under larger-insert environmental DNA cloning methods (Iqbal et al., 2016). These natural products, which were discovered through the metagenomics approach have a number of characteristics, such as antimicrobial, hydrolytic, and phytotoxic compounds, and are being used in industry, agriculture, and biomedicine fields.
In agriculture, these novel products are antibiotics and have the potential to suppress weeds, act as biocontrol agents by plant-growth promotion, pathogen suppression, and plant disease resistance as well as effective pesticides. For example, buffalo rumen is used to isolate enzymes with unique features that can survive in extreme temperatures or pH (Singh et al., 2012). In another examination, novel hydrolase diversity was retrieved from bovine rumen microflora with a metagenome library and had a role in biocatalysis. Similarly, novel endo-α-1,5-L-Arabinanase was discovered from cow rumen with an endo-acting mechanism with arabinotriose as the final product (Wong et al., 2009).
Agricultural soil is also a rich source of novel compounds with diverse applications in industry. Novel phytase genes were identified from an agricultural soil microbial community for identification of phytase activity in a metagenomic library (Tan et al., 2014). In a different survey, a novel salt-tolerant, chitobiosidase was derived from chitin-amended disease-suppressive agricultural soil with the use of a metagenomic library (Cretoiu et al., 2015). Rhizospheric soil samples collected from three different Solanum phureja farms located in the Cundinamarca Andean Plateau, Colombia revealed unique lipase, esterase, and protease enzymes that have a biotechnological application (Calderon et al., 2019).
Source of Biosurfactants
Another promising role of functional metagenomics is in green biosurfactants analysis from uncultured microbes in the agriculture industry. It has the potential to replace harsh chemical surfactants. In biosurfactants synthesized by the rhizosphere, plant-associated microbes play a vital role and have a wider application in industries, including agriculture. They are responsible for biofilm formation, motility, signaling process, plant-pathogen destruction, the bioavailability of nutrients, agricultural soil quality enhancement through soil remediation, plant growth promotion, etc. There are different types of biosurfactants obtained from bacteria, yeast, and fungi based on their physiochemical properties, such as lipopeptides, glycolipids, phospholipids, neutral lipids, polymeric, and fatty acids. In the bioremediation process, a biosurfactant is employed for the effective removal of hydrocarbon and metal pollutants that are tightly bound to soil (Pacwa-Płociniczak et al., 2011; Sharma et al., 2021) for enhancing the degradation of certain chemical insecticides in agricultural soil. The first report of biosurfactant identification was by using the metagenomic library from environmental DNA that is N-acyltyrosines with N-myristoyltyrosine (Thies et al., 2016). The application of metagenomics in deciphering the novel biosurfactants from the marine environment is also reported (Gudiña et al., 2016). Moreover, N-acyltyrosines exhibited antibiotic activity across varied bacteria.
Source of Natural Product
Different industries depend on various agricultural products in their application. Here, products discovered using a metagenomics approach from agriculture-related fields to use in industries are discussed. In second-generation biofuel production, the focus is on the use of sustainable and alternative energy management. In this case, the industrially reasonable and economic conversion process involves the use and conversion of lignocellulosic biomass into biofuel molecules with cellulolytic enzymes. Tiwari et al. (2018), elaborated a study on deciphering the novel and effective cellulases from different environmental niches by uncultivable metagenomic approaches for their strong utilization in bio-refineries (Tiwari et al., 2018). Agricultural production-scale biogas plants provided new insights into microbial community composition and the genetic potential of important communities involved in biogas production (Bremges et al., 2015). Hemicellulolytic enzymes used in biofuel production are obtained from ruminal metagenomic libraries (Duque et al., 2018). Asymmetric synthesis of chiral alcohols using microbes is also studied (Saravanan and Suneetha, 2017). Table 4 describes the novel products detected using metagenomics approaches.
Other industries include pharmacy, which involves antibiotics and pharmaceuticals identified from agricultural soil. Antibiotics discovered are indirubin, deoxyviolacein and violacein, N-acyltyrosine, terragine (mycobacterium-inhibiting antibiotic), acyltyrosines, and turbomucin A and B.
Identification of Biosynthetic Molecules
Functional metagenomics has led to the detection of carotenoids and metatricycloene, a biosynthetically and structurally significant polyene. Metatricycloene is the first biosynthetically complex natural product to be obtained from Streptomyces species by metagenomics screening methods. Large numbers of herbicidal compounds have been isolated from soil bacteria which paved the way for the development of popular herbicides, like glufosinate (Kao-Kniffin et al., 2013).
Biosynthetic genes and proteins associated with insecticidal activity are discovered through this approach. For instance, insecticidal active proteins were isolated from Xenorhabdus nematophilus, and discovery of pyripyroprene an insecticide from Penicillium coprobium PF1169 (Hu et al., 2011). Pest management strategies, which were discussed in this review, explain the application and design of microbial-derived management strategies in agriculture. A study on Serratia entomophila strain Mor4.1, a bacterial pathogen of several soil pests from genera: Phyllophaga and Anomala lead to the discovery of cell membrane protein having a toxic effect, which is proposed to be used in designing biocontrol project (Rodríguez-Segura et al., 2012).
Use in Genetically Modified Crops
Metagenomics application for genetically modified crops is through identification of herbicide resistance genes from soil microbes, which can be used in engineering genetically modified crops. The best example is the discovery of glyphosate resistance and glyphosate degrading abilities from microorganisms (Staub et al., 2012).
Antibiotic-Resistant Genes
Metagenomics analyses play important role in the identification of antibiotics resistant genes in agriculture. This antibiotic resistance gene from agricultural soil contaminates groundwater. In addition, the clinical consequences caused by it will have an adverse effect, if, consumed by humans and animals. Antibiotics resistance genes of about 9 aminoglycosides and one tetracycline were identified from soil (Riesenfeld et al., 2004).
Metagenome-Wide Association Studies
Predictive Biology of Crops
Numerous studies on metagenomics in agriculture have led to the association of microbial communities in crop growth factors like crop productivity, growth rate decline, etc. Recent works of research in agricultural metagenomics are being focused on Metagenomics Wide Association Studies (MWAS). MGWAS and Genome-Wide Association Studies (GWAS) have striking similarities between challenges and pitfalls and elaborated on three key points in MWAS analysis (Weissbrod et al., 2018). These are (i) uniform data format adoption (ii) stringent statistical criteria and (iii) treating microbiome and host genome as specific entities. It aims at identifying genetic variants in microbial genomes that are associated with host variation in microbe phenotypes, like genetic variation affecting phenotypes, including carriage (in humans) and virulence in microbes.
In the MWAS approach, the microbial community identified through the metagenomics approach is used as a tool for analysis along with its abundance. The abundance of taxa is used as an explanatory variable for MWAS studies. MWAS is used to study many host-microbe interactions as a way to uncover microbial communities that are important for host health. A study by Crespo-Piazuelo et al. (2019), suggested that about 39 candidate genes may be involved in regulating microbiota composition, as well as responsible for the association between host genome and gut microbiota in pigs through MWAS (Crespo-Piazuelo et al., 2019). It is used in defining Enterohemorrhagic Escherichia coli Colonization. Further, it has been found that the plant loci responsible for defense and cell wall integrity affect microbial community variation in Arabidopsis thaliana (Horton et al., 2014).
Metagenomics-wide association studies has also been applied to regulate genes that are important for species-specific phenotypes in Helicobacter pylori, human diseases like asthma, obesity, and diabetes (Dutilh et al., 2013; Karlsson et al., 2013). Very few MWAS studies have been done in an agricultural context. Some of these are on crops like soybeans, millets, grapes, and strawberries (Debenport et al., 2015; Chang et al., 2017). In these studies, the linking of MWAS based on taxon abundance and crop productivity rates are emphasized, which is elaborately discussed in the following sections.
Theories, such as neutral (stochastic) and niche (deterministic), are subject to managing ecological populations. Rhizosphere microbiome is regulated by a deterministic approach, while, habitats in bulk soil are dictated by stochastic theory. Niche mechanisms depend on local habitat circumstances and their niche requirements. Further, the shaping of niches and helping of microbial community assembly is through physical-chemical soil characteristics and products derived from plant roots. A total of 25 abiotic soil characteristics, including latitude and longitude of sampling places, clay, silt, sand, and 12 types of elements percentage, pH, organic matter, cation exchange capacity along with percent saturation of elements and their influence on agricultural crops, were studied (Chang et al., 2017).
Genetic variation in microbes is altogether different from human genetics. The three major variations are (i) single nucleotide polymorphisms (SNPs) and insertion-deletion mutations (INDELs), (ii) gene presence and absence, and (iii) bi-allelic SNP variation that is responsible for the phenotype of interest. Understanding the type of variation is a fundamental key in selecting tools and methods in MWAS analysis. Various tools used for the MWAS study are elaborated in detail in the tools and approaches section.
Application of Metagenomics-Wide Association Studies
Crop Productivity Rates
Keen interest in crop productivity linked to MWAS is exponentially increasing, studying the differences in crop productivity rates at a location within a field, despite having the same features related to crop genotype, management practices, and cropping history. Assumptions behind this crop productivity differences are attributed to unequal distribution of bulk soil biotic and abiotic factors in a location within agricultural fields.
With regard to crop productivity, it is a quantitative trait influenced by several factors, which includes physical and chemical analysis for abiotic factors and the next-generation metagenomics sequencing approach in relation to biotic factors. There are several abiotic features but few that significantly impact crop productivity, including water, weather conditions like temperature, rainfall, and nitrogen availability. Further, soil characteristics that are much important like soil moisture, soil physical compaction, and drainage differences within agronomic fields should also be considered.
Limiting factors of productivity in relation to biotic stress are (i) yield performance, which is affected by pest, pathogen and influenced by root nodulation (ii) genotype of crop variety includes genetics related to the following features: water and nitrogen utilization efficiency, photosynthesis, and productivity performance, disease, and pest resistance. de Almeida Lopes et al. (2016), described the influence of crop genetic features on rhizosphere microbiome structuring (de Almeida Lopes et al., 2016).
In addition, plant growth-promoting rhizobacteria (PGPR) are playing a key role in crop productivity leading to high crop production and suppressing the development of diseases. Microbiome structure in the phyllosphere and rhizosphere differ between plant species, and also with species genotype. Peiffer et al. (2013), studied the plant-microbe interactions through genome wide-associations which can be integrated into plant breeding (Peiffer et al., 2013).
Intercropping Analysis
Intercropping systems are known to positively affect crop growth and yield by potentially recruiting favorable microorganisms at the root zone of a crop. The impact of intercropping on the structure of microbiomes is widely studied by methods from terminal restriction fragment length polymorphism, denaturing gradient gel electrophoresis, phospholipid fatty acid analysis to high throughput sequence-based approaches. Intercropped soil samples showed frequently large-magnitude differences in the abundance of beneficial microorganisms in crops.
Applications of intercropping strategy have led to stimulation of microbial ecology that increased soil enzyme activity and resulted in high yield (Debenport et al., 2015). Benefits of intercropping include improvement in nutrient content, soil moisture profiles, microfauna of soil, soil enzyme activity, phospholipid fatty acid biomarkers, root zone microbiome, increase in crop production, soil organic carbon, etc. Soil organic matter plays a very potent role in agriculture and its biodegradability depends on compounds like organic and humic acids, proteins, and lignin along with pH and oxygen level, including microbial quality, composition, size, and activity.
Microorganisms’ spatial and temporal interactions with organic matter dictate the overall microbial population at a specific site. The increase in soil organic content depends on soil type. For instance, in semi-arid soils, it influences the arbuscular mycorrhizal diversity, whereas, in polar desert soil as well as in medium and low-level saline soils, bacterial communities are dominated (Van Horn et al., 2014).
Agronomic Field: Rotation With More Crops
Rotation is a cultural practice in agriculture to restrict soil-borne pathogens causing diseases. It has been observed that the same cropping pattern over a long period of time leads to reduced yield potential. Examples are apples, almonds, strawberries (Mazzola and Manici, 2012). The soil-borne pathogen, which affects a large number of plant species usually, penetrates through the roots from infected soil or damages the host roots, making them susceptible to infection. An example includes fungal pathogens Verticillium dahliae Kleb, Rhizoctonia sp., Cylindrocarpon destructans, Fusarium oxysporum, Fusarium solani, Pestalotia longiseta, Pythium spp., Aphanomyces euteiches, Macrophomina phaseolina, Sclerotium and nematodes, such as Pratylenchus penetrans, Meloidogyne hapla, and Globodera pallida.
Recent advances in agriculture are using biofumigation and anaerobic soil disinfestation (ASD) methods in controlling these pathogens and pests. Biofumigation is preferred over chemical methods of fumigation involving methyl bromide and chloropicrin. Biofumigation is used for brassica species that generate isothiocyanate as biologically active green manures. Crop rotation with non-host crops resulted in decreased incidence of infections and an increase in crop production. For example, Neubauer et al. (2014), assessed systematically the use of brassicaceous green manures to Verticillium dahlia but the results concluded that their efficiency in controlling the pathogen depends on soil organic conditions (Neubauer et al., 2014). In another study, wilt occurrence in strawberries is reduced by broccoli or lettuce crop rotation (Njoroge et al., 2009). During the biofumigation process release of volatiles suggests that control measures could be used to improve the control of soil-borne diseases (Mercier and Jiménez, 2009). A study in crop fields in Arkansas to investigate the impact of crop rotation and soil cultivation methods on rhizosphere microbial diversity was found not to significantly affect bacterial diversity (Oliveira et al., 2022).
Anaerobic soil disinfestation methods were traditionally used for grasses, but their effect was unknown. Later, it was studied that organic materials, including organic wastes, animal and green manure, compost, and peats, are found to be effective mainly against pests and partially for pathogens (Runia et al., 2012). But these studies showed the effectiveness and production of gasses (CO2, CH4, and N2O, among others) depending on physical factors, including soil type, organic materials, temperature, exposure time, and dosage. Organic amendments included organic wastes, animal and green manure, compost, and peats. In a metastudy, the characteristics of organic soil amendments that improve soil fertility, plant health and suppress soil-borne plant diseases were identified (Bonanomi et al., 2010). Effective treatment against Verticillium wilt in plants through the treatment of organic amendments was done (Vitullo et al., 2013). In short, organic matter possesses positive agronomic properties in agriculture. For the effective soil disinfectant, studies on complete mechanisms that are effective, quick, and predictable are needed.
Future Trends in Metagenomic Studies
Metagenomic studies itself is a potent tool. However, metagenomics along with wide association studies discussed in this review and transcriptomics studies termed metatranscriptomics will strengthen this approach as a whole. Similarly, proteome study of microbial communities is a new emerging area that aims at analyzing the catalytic potential of a microbial population. Along with agriculture, metagenomics has also been used in food obtained as agriculture byproducts. For example, it is applied in food industries for the identification of novel enzymes and enhances the production of recombinant enzymes (El Sheikha, 2018; Hua et al., 2018). The role of metagenomics in the identification of biocontrol agents as future plant health detectors and influencers in biopolitics and bioeconomics is highly promising for application in near future.
Conclusion
Enormous studies by a group of researchers from multiple fields have made extraordinary advances in metagenomics over more than three decades. To solve the intricacies of underlying processes of microbial communities, it is important to understand the diversity and dynamics of such microbial communities. This review is a vast resource of knowledge discovery with product discovery. The appreciable NGS cost reduction and community approach can be promising in uncovering the metagenome. Understanding of genome alone of any agricultural microorganism is not enough, as productivity is the cumulative role of genomes rather than the genome of a single organism, be it agricultural crop or fish, or domestic animal. More investment and attention are required to improve the model of genome-wide association studies or genomic selection, adding a metagenome profile in the mixed model. Genome manipulation is inadequate for genetic gain in breeding programs if we neglect the metagenome as one of the major variables. This review highlights the unexplored high potential area, whereas more research attention is required to have better agricultural productivity with sustainability. With the advanced data generation and analyses technologies, this field of metagenomics will widen and improve the knowledge of microorganisms.
Author Contributions
DK, MI, SJ, and JJ contributed to conceptualization, data curation, formal analysis, funding acquisition, investigation, methodology, project administration, resources, software, supervision, validation, visualization, and writing the original draft. AR, DK, and RP contributed to manuscript editing and finalization. All authors contributed to the article and approved the submitted version.
Funding
This work was supported by the Indian Council of Agricultural Research; Ministry of Agriculture and Farmer’s Welfare, Government of India by providing financial assistance in the form of a CABin grant (F.No. Agril. Edn.4-1/2013-A&P).
Conflict of Interest
The authors declare that the research was conducted in the absence of any commercial or financial relationships that could be construed as a potential conflict of interest.
Publisher’s Note
All claims expressed in this article are solely those of the authors and do not necessarily represent those of their affiliated organizations, or those of the publisher, the editors and the reviewers. Any product that may be evaluated in this article, or claim that may be made by its manufacturer, is not guaranteed or endorsed by the publisher.
Acknowledgments
We are thankful to Indian Council of Agricultural Research, Ministry of Agriculture and Farmers’ Welfare, Govt. of India for infrastructure setup and other facilities at ICAR-IASRI, New Delhi, India created under National Agricultural Innovation Project, funded by World Bank at ICAR-IASRI, New Delhi. Financial support from the CABin Grant of ICAR-IASRI, New Delhi in the form of fellowship to JJ is also thankfully acknowledged.
Footnotes
- ^ https://www.jcvi.org/research/metarep
- ^ https://img.jgi.doe.gov/
- ^ https://gfo.ca/research-projects/w2017id03/
- ^ https://www.ipcc.ch/srccl/chapter/chapter-5/
References
Abarenkov, K., Nilsson, R. H., Larsson, K.-H., Alexander, I. J., Eberhardt, U., Erland, S., et al. (2010). The UNITE database for molecular identification of fungi–recent updates and future perspectives. New Phytol. 186, 281–285. doi: 10.1111/j.1469-8137.2009.03160.x
Abdelfattah, A., Wisniewski, M., Droby, S., and Schena, L. (2016). Spatial and compositional variation in the fungal communities of organic and conventionally grown apple fruit at the consumer point-of-purchase. Hortic. Res. 3, 1–12.
Abe, T., Kukita, A., Akiyama, K., Naito, T., and Uemura, D. (2012). Isolation and structure of a novel biindole pigment substituted with an ethyl group from a metagenomic library derived from the marine sponge Halichondria okadai. Chem. Lett. 41, 728–729. doi: 10.1246/cl.2012.728
Adams, I. P., Glover, R. H., Monger, W. A., Mumford, R., Jackeviciene, E., Navalinskiene, M., et al. (2009). Next-generation sequencing and metagenomic analysis: a universal diagnostic tool in plant virology. Mol. Plant Pathol. 10, 537–545. doi: 10.1111/j.1364-3703.2009.00545.x
Ahlawat, O. P., Tiwari, R., and Singh, G. P. (2018). Metagenomics of wheat rhizosphere for abiotic stress managment. Wheat Barley Res. 10, 64–77.
Alawiye, T. T., and Babalola, O. O. (2021). Metagenomic insight into the community structure and functional genes in the sunflower rhizosphere microbiome. Agriculture 11:167. doi: 10.3390/agriculture11020167
Allen, E. E., and Banfield, J. F. (2005). Community genomics in microbial ecology and evolution. Nat. Rev. Microbiol. 3, 489–498. doi: 10.1038/nrmicro1157
Arakane, Y., Hogenkamp, D. G., Zhu, Y. C., Kramer, K. J., Specht, C. A., Beeman, R. W., et al. (2004). Characterization of two chitin synthase genes of the red flour beetle, Tribolium castaneum, and alternate exon usage in one of the genes during development. Insect Biochem. Mol. Biol. 34, 291–304. doi: 10.1016/j.ibmb.2003.11.004
Arjun, J. K., Aneesh, B. P., Kavitha, T., and Harikrishnan, K. (2018). Characterization of a novel asparaginase from soil metagenomic libraries generated from forest soil. Biotechnol. Lett. 40, 343–348. doi: 10.1007/s10529-017-2470-7
Aun, E., Brauer, A., Kisand, V., Tenson, T., and Remm, M. (2018). A k-mer-based method for the identification of phenotype-associated genomic biomarkers and predicting phenotypes of sequenced bacteria. PLoS Comput. Biol. 14:e1006434. doi: 10.1371/journal.pcbi.1006434
Bandla, A., Pavagadhi, S., Sudarshan, A. S., Poh, M. C. H., and Swarup, S. (2020). 910 metagenome-assembled genomes from the phytobiomes of three urban-farmed leafy Asian greens. Sci. Data 7:278. doi: 10.1038/s41597-020-00617-9
Bayer, T. S., Widmaier, D. M., Temme, K., Mirsky, E. A., Santi, D. V., and Voigt, C. A. (2009). Synthesis of methyl halides from biomass using engineered microbes. J. Am. Chem. Soc. 131, 6508–6515. doi: 10.1021/ja809461u
Berg, G., Köberl, M., Rybakova, D., Müller, H., Grosch, R., and Smalla, K. (2017). Plant microbial diversity is suggested as the key to future biocontrol and health trends. FEMS Microbiol. Ecol. 93:fix050. doi: 10.1093/femsec/fix050
Berini, F., Casciello, C., Marcone, G. L., and Marinelli, F. (2017). Metagenomics: novel enzymes from non-culturable microbes. FEMS Microbiol. Lett. 364:fnx211.
Bertazzon, N., Chitarra, W., Angelini, E., and Nerva, L. (2020). Two new putative plant viruses from wood metagenomics analysis of an esca diseased vineyard. Plants 9:835. doi: 10.3390/plants9070835
Boisvert, S., Raymond, F., Godzaridis, É, Laviolette, F., and Corbeil, J. (2012). Ray Meta: scalable de novo metagenome assembly and profiling. Genome Biol. 13:R122. doi: 10.1186/gb-2012-13-12-r122
Bonanomi, G., Antignani, V., Capodilupo, M., and Scala, F. (2010). Identifying the characteristics of organic soil amendments that suppress soilborne plant diseases. Soil Biol. Biochem. 42, 136–144. doi: 10.1007/s11356-015-4816-9
Brady, S. F., Chao, C. J., and Clardy, J. (2002). New natural product families from an environmental DNA (eDNA) gene cluster. J. Am. Chem. Soc. 124, 9968–9969. doi: 10.1021/ja0268985
Brady, S. F., Chao, C. J., and Clardy, J. (2004). Long-chain N-acyltyrosine synthases from environmental DNA. Appl. Environ. Microbiol. 70, 6865–6870. doi: 10.1128/AEM.70.11.6865-6870.2004
Brady, S. F., Chao, C. J., Handelsman, J., and Clardy, J. (2001). Cloning and heterologous expression of a natural product biosynthetic gene cluster from eDNA. Org. Lett. 3, 1981–1984. doi: 10.1021/ol015949k
Brady, S. F., and Clardy, J. (2000). Long-chain N-acyl amino acid antibiotics isolated from heterologously expressed environmental DNA. J. Am. Chem. Soc. 122, 12903–12904. doi: 10.1021/ja002990u
Brynildsrud, O., Bohlin, J., Scheffer, L., and Eldholm, V. (2016). Rapid scoring of genes in microbial pan-genome-wide association studies with Scoary. Genome Biol. 17:238. doi: 10.1186/s13059-016-1108-8
Bremges, A., Maus, I., Belmann, P., Eikmeyer, F., Winkler, A., Albersmeier, A., et al. (2015). Deeply sequenced metagenome and metatranscriptome of a biogas-producing microbial community from an agricultural production-scale biogas plant. Gigascience 4:33. doi: 10.1186/s13742-015-0073-6
Buchholz, F., Kostić, T., Sessitsch, A., and Mitter, B. (2018). The potential of plant microbiota in reducing postharvest food loss. Microb. Biotechnol. 11, 971–975. doi: 10.1111/1751-7915.13252
Cai, R., Lewis, J., Yan, S., Liu, H., Clarke, C. R., Campanile, F., et al. (2011). The plant pathogen Pseudomonas syringae pv. tomato is genetically monomorphic and under strong selection to evade tomato immunity. PLoS Pathog. 7:e1002130. doi: 10.1371/journal.ppat.1002130
Calderon, D., Peña, L., Suarez, A., Villamil, C., Ramirez-Rojas, A., Anzola, J. M., et al. (2019). Recovery and functional validation of hidden soil enzymes in metagenomic libraries. Microbiologyopen 8:e00572. doi: 10.1002/mbo3.572
Callahan, B. J., McMurdie, P. J., Rosen, M. J., Han, A. W., Johnson, A. J. A., and Holmes, S. P. (2016). DADA2: high-resolution sample inference from Illumina amplicon data. Nat. Methods 13, 581–583. doi: 10.1038/nmeth.3869
Caporaso, J. G., Kuczynski, J., Stombaugh, J., Bittinger, K., Bushman, F. D., Costello, E. K., et al. (2010). QIIME allows analysis of high-throughput community sequencing data. Nat. Methods 7, 335–336. doi: 10.1038/nmeth.f.303
Cardoso, A. M., Cavalcante, J. J. V., Cantão, M. E., Thompson, C. E., Flatschart, R. B., Glogauer, A., et al. (2012). Metagenomic analysis of the microbiota from the crop of an invasive snail reveals a rich reservoir of novel genes. PLoS One 7:e48505. doi: 10.1371/journal.pone.0048505
Chang, H.-X., Haudenshield, J. S., Bowen, C. R., and Hartman, G. L. (2017). Metagenome-wide association study and machine learning prediction of bulk soil microbiome and crop productivity. Front. Microbiol. 8:519. doi: 10.3389/fmicb.2017.00519
Chang, K. F., Hwang, S. F., Ahmed, H. U., Strelkov, S. E., Gossen, B. D., Turnbull, G. D., et al. (2014). Disease reaction to Fusarium avenaceum and yield losses in narrow-leafed lupin lines. Can. J. Plant Sci. 94, 1211–1218. doi: 10.4141/cjps2013-243
Chuzel, L., Ganatra, M. B., Rapp, E., Henrissat, B., and Taron, C. H. (2018). Functional metagenomics identifies an exosialidase with an inverting catalytic mechanism that defines a new glycoside hydrolase family (GH156). J. Biol. Chem. 293, 18138–18150. doi: 10.1074/jbc.RA118.003302
Claverie, S., Bernardo, P., Kraberger, S., Hartnady, P., Lefeuvre, P., Lett, J.-M., et al. (2018). From spatial metagenomics to molecular characterization of plant viruses: a geminivirus case study. Adv. Virus Res. 101, 55–83. doi: 10.1016/bs.aivir.2018.02.003
Cole, J. R., Wang, Q., Cardenas, E., Fish, J., Chai, B., Farris, R. J., et al. (2009). The ribosomal database project: improved alignments and new tools for rRNA analysis. Nucleic Acids Res. 37, D141–D145. doi: 10.1093/nar/gkn879
Crespo-Piazuelo, D., Migura-Garcia, L., Estellé, J., Criado-Mesas, L., Revilla, M., Castelló, A., et al. (2019). Association between the pig genome and its gut microbiota composition. Sci. Rep. 9:8791. doi: 10.1038/s41598-019-45066-6
Cretoiu, M. S., Berini, F., Kielak, A. M., Marinelli, F., and van Elsas, J. D. (2015). A novel salt-tolerant chitobiosidase discovered by genetic screening of a metagenomic library derived from chitin-amended agricultural soil. Appl. Microbiol. Biotechnol. 99, 8199–8215. doi: 10.1007/s00253-015-6639-5
Culligan, E. P., Sleator, R. D., Marchesi, J. R., and Hill, C. (2014). Metagenomics and novel gene discovery: promise and potential for novel therapeutics. Virulence 5, 399–412. doi: 10.4161/viru.27208
de Almeida Lopes, K. B., Carpentieri-Pipolo, V., Oro, T. H., Stefani Pagliosa, E., and Degrassi, G. (2016). Culturable endophytic bacterial communities associated with field-grown soybean. J. Appl. Microbiol. 120, 740–755. doi: 10.1111/jam.13046
de Nazaré Almeida dos Reis, L., Fonseca, M. E. N., Ribeiro, S. G., Naito, F. Y. B., Boiteux, L. S., and Pereira-Carvalho, R. C. (2020). Metagenomics of neotropical single-stranded DNA viruses in tomato cultivars with and without the Ty-1 gene. Viruses 12:819. doi: 10.3390/v12080819
Debenport, S. J., Assigbetse, K., Bayala, R., Chapuis-Lardy, L., Dick, R. P., and Gardener, B. B. M. (2015). Association of shifting populations in the root zone microbiome of millet with enhanced crop productivity in the Sahel region (Africa). Appl. Environ. Microbiol. 81, 2841–2851. doi: 10.1128/AEM.04122-14
Delmont, T. O., Robe, P., Clark, I., Simonet, P., and Vogel, T. M. (2011). Metagenomic comparison of direct and indirect soil DNA extraction approaches. J. Microbiol. Methods 86, 397–400. doi: 10.1016/j.mimet.2011.06.013
Deshpande, S. V., Reed, T. M., Sullivan, R. F., Kerkhof, L. J., Beigel, K. M., and Wade, M. M. (2019). Offline next generation metagenomics sequence analysis using MinION detection Software (MINDS). Genes (Basel) 10:578. doi: 10.3390/genes10080578
Dhanjal, D. S., Chopra, C., and Chopra, R. S. (2020a). “Metagenomic DNA sequencing: technological advances and applications,” in Metagenomics: Techniques, Applications, Challenges and Opportunities, eds R. S. Chopra, C. Chopra, and N. R. Sharma (Singapore: Springer), 37–53. doi: 10.1007/978-981-15-6529-8_3
Dhanjal, D. S., Chopra, R. S., and Chopra, C. (2020b). “Metagenomics and enzymes: the novelty perspective,” in Metagenomics: Techniques, Applications, Challenges and Opportunities, eds R. S. Chopra, C. Chopra, and N. R. Sharma (Singapore: Springer), 109–131. doi: 10.1007/978-981-15-6529-8_7
Donia, M. S., Cimermancic, P., Schulze, C. J., Brown, L. C. W., Martin, J., Mitreva, M., et al. (2014). A systematic analysis of biosynthetic gene clusters in the human microbiome reveals a common family of antibiotics. Cell 158, 1402–1414. doi: 10.1016/j.cell.2014.08.032
Doxey, A. C., Kurtz, D. A., Lynch, M. D. J., Sauder, L. A., and Neufeld, J. D. (2015). Aquatic metagenomes implicate Thaumarchaeota in global cobalamin production. ISME J. 9, 461–471. doi: 10.1038/ismej.2014.142
Dröge, J., Gregor, I., and McHardy, A. C. (2015). Taxator-tk: precise taxonomic assignment of metagenomes by fast approximation of evolutionary neighborhoods. Bioinformatics 31, 817–824. doi: 10.1093/bioinformatics/btu745
Duque, E., Daddaoua, A., Cordero, B. F., Udaondo, Z., Molina-Santiago, C., Roca, A., et al. (2018). Ruminal metagenomic libraries as a source of relevant hemicellulolytic enzymes for biofuel production. Microb. Biotechnol. 11, 781–787. doi: 10.1111/1751-7915.13269
Durso, L. M., Miller, D. N., and Wienhold, B. J. (2012). Distribution and quantification of antibiotic resistant genes and bacteria across agricultural and non-agricultural metagenomes. PLoS One 7:e48325. doi: 10.1371/journal.pone.0048325
Dutilh, B. E., Backus, L., Edwards, R. A., Wels, M., Bayjanov, J. R., and van Hijum, S. A. F. T. (2013). Explaining microbial phenotypes on a genomic scale: GWAS for microbes. Brief. Funct. Genomics 12, 366–380. doi: 10.1093/bfgp/elt008
Earle, S. G., Wu, C.-H., Charlesworth, J., Stoesser, N., Gordon, N. C., Walker, T. M., et al. (2016). Identifying lineage effects when controlling for population structure improves power in bacterial association studies. Nat. Microbiol. 1:16041. doi: 10.1038/nmicrobiol.2016.41
Edgar, R. C. (2013). UPARSE: highly accurate OTU sequences from microbial amplicon reads. Nat. Methods 10, 996–998. doi: 10.1038/nmeth.2604
Edge, T. A., Baird, D. J., Bilodeau, G., Gagné, N., Greer, C., Konkin, D., et al. (2020). The ecobiomics project: advancing metagenomics assessment of soil health and freshwater quality in Canada. Sci. Total Environ. 710:135906. doi: 10.1016/j.scitotenv.2019.135906
El Sheikha, A. F. (2018). “Advances in genetic engineering for higher production and quality improvement of food and beverages,” in Innovations in Technologies for Fermented Food and Beverage Industries, eds S. Panda and P. Shetty (Cham: Springer), 221–255. doi: 10.1007/978-3-319-74820-7_12
El Sheikha, A. F., Levin, R., and Xu, J. (2018). Molecular Techniques in Food Biology. Hoboken, NJ: Wiley Online Library.
El Sheikha, A. F., and Ray, R. C. (2014). Is Pcr-Dgge an Innovative Molecular Tool for the Detection of Microbial Plant Pathogens. Biol. Control. Prev. Food deterior. Chichester: John Wiley Sons Ltd, 409–433.
El Sheikha, A. F., and Xu, J. (2018). Molecular techniques and foodstuffs: innovative fingerprints, then what? Mol. Tech. Food Biol. Safety, Biotechnol. Authent. Traceability 421–434. doi: 10.1002/9781119374633.ch19
Enagbonma, B. J., Aremu, B. R., and Babalola, O. O. (2019). Profiling the functional diversity of termite mound soil bacteria as revealed by shotgun sequencing. Genes (Basel) 10:637. doi: 10.3390/genes10090637
Entcheva, P., Liebl, W., Johann, A., Hartsch, T., and Streit, W. R. (2001). Direct cloning from enrichment cultures, a reliable strategy for isolation of complete operons and genes from microbial consortia. Appl. Environ. Microbiol. 67, 89–99. doi: 10.1128/AEM.67.1.89-99.2001
Erlacher, A., Cardinale, M., Grosch, R., Grube, M., and Berg, G. (2014). The impact of the pathogen Rhizoctonia solani and its beneficial counterpart Bacillus amyloliquefaciens on the indigenous lettuce microbiome. Front. Microbiol. 5:175. doi: 10.3389/fmicb.2014.00175
Espindola, A., Schneider, W., Hoyt, P. R., Marek, S. M., and Garzon, C. (2015). A new approach for detecting fungal and oomycete plant pathogens in next generation sequencing metagenome data utilising electronic probes. Int. J. Data Min. Bioinform. 12, 115–128. doi: 10.1504/ijdmb.2015.069422
Esposito, A., Colantuono, C., Ruggieri, V., and Chiusano, M. L. (2016). Bioinformatics for agriculture in the Next-Generation sequencing era. Chem. Biol. Technol. Agric. 3:9.
Fadiji, A. E., Ayangbenro, A. S., and Babalola, O. O. (2020). Metagenomic profiling of the community structure, diversity, and nutrient pathways of bacterial endophytes in maize plant. Antonie Van Leeuwenhoek 113, 1559–1571. doi: 10.1007/s10482-020-01463-w
Feng, Z., Chakraborty, D., Dewell, S. B., Reddy, B. V. B., and Brady, S. F. (2012). Environmental DNA-encoded antibiotics fasamycins A and B inhibit FabF in type II fatty acid biosynthesis. J. Am. Chem. Soc. 134, 2981–2987. doi: 10.1021/ja207662w
Field, D., Garrity, G., Gray, T., Morrison, N., Selengut, J., Sterk, P., et al. (2008). The minimum information about a genome sequence (MIGS) specification. Nat. Biotechnol. 26, 541–547.
Fierer, N., Ladau, J., Clemente, J. C., Leff, J. W., Owens, S. M., Pollard, K. S., et al. (2013). Reconstructing the microbial diversity and function of pre-agricultural tallgrass prairie soils in the United States. Science 342, 621–624. doi: 10.1126/science.1243768
Fosso, B., Santamaria, M., Marzano, M., Alonso-Alemany, D., Valiente, G., Donvito, G., et al. (2015). BioMaS: a modular pipeline for bioinformatic analysis of metagenomic AmpliconS. BMC Bioinformatics 16:203. doi: 10.1186/s12859-015-0595-z
Frank, D. N. (2011). Growth and Development Symposium: promoting healthier humans through healthier livestock: animal agriculture enters the metagenomics era. J. Anim. Sci. 89, 835–844. doi: 10.2527/jas.2010-3392
Gabor, E. M. (2004). Harvesting Novel Biocatalysts From the Metagenome. Ph.D. thesis. Groningen: University of Groningen.
Garrido Oter, R. (2018). Computational Methods for the Study of Plant-Associated Microbial Communities. Ph.D dissertation. Halle: Universitäts- und Landesbibliothek.
Giardine, B., Riemer, C., Hardison, R. C., Burhans, R., Elnitski, L., Shah, P., et al. (2005). Galaxy: a platform for interactive large-scale genome analysis. Genome Res. 15, 1451–1455. doi: 10.1101/gr.4086505
Gillespie, D. E., Brady, S. F., Bettermann, A. D., Cianciotto, N. P., Liles, M. R., Rondon, M. R., et al. (2002). Isolation of antibiotics turbomycin A and B from a metagenomic library of soil microbial DNA. Appl. Environ. Microbiol. 68, 4301–4306. doi: 10.1128/AEM.68.9.4301-4306.2002
Ginolhac, A., Jarrin, C., Gillet, B., Robe, P., Pujic, P., Tuphile, K., et al. (2004). Phylogenetic analysis of polyketide synthase I domains from soil metagenomic libraries allows selection of promising clones. Appl. Environ. Microbiol. 70, 5522–5527. doi: 10.1128/AEM.70.9.5522-5527.2004
Gonzalez, J. M., Portillo, M. C., Belda-Ferre, P., and Mira, A. (2012). Amplification by PCR artificially reduces the proportion of the rare biosphere in microbial communities. PLoS One 7:e29973. doi: 10.1371/journal.pone.0029973
Gopal, M., Gupta, A., and Thomas, G. V. (2013). Bespoke microbiome therapy to manage plant diseases. Front. Microbiol. 4:355. doi: 10.3389/fmicb.2013.00355
Granjou, C., and Phillips, C. (2019). Living and labouring soils: metagenomic ecology and a new agricultural revolution? Biosocieties 14, 393–415. doi: 10.1057/s41292-018-0133-0
Gregor, I., Dröge, J., Schirmer, M., Quince, C., and McHardy, A. C. (2016). PhyloPythiaS+: a self-training method for the rapid reconstruction of low-ranking taxonomic bins from metagenomes. PeerJ 4:e1603. doi: 10.7717/peerj.1603
Gudiña, E. J., Teixeira, J. A., and Rodrigues, L. R. (2016). Biosurfactants produced by marine microorganisms with therapeutic applications. Mar. Drugs 14:38. doi: 10.3390/md14020038
Gupta, N., Vats, S., and Bhargava, P. (2018). “Sustainable agriculture: role of metagenomics and metabolomics in exploring the soil microbiota,” in Silico Approach for Sustainable Agriculture, eds D. Choudhary, M. Kumar, R. Prasad, and V. Kumar (Singapore: Springer), 183–199. doi: 10.1007/978-981-13-0347-0_11
Gweon, H. S., Oliver, A., Taylor, J., Booth, T., Gibbs, M., Read, D. S., et al. (2015). PIPITS: an automated pipeline for analyses of fungal internal transcribed spacer sequences from the Illumina sequencing platform. Methods Ecol. Evol. 6, 973–980. doi: 10.1111/2041-210X.12399
Hakim, S., Nawaz, M. S., Siddique, M. J., Hayat, M., Gulzar, U., and Imran, A. (2022). “Metagenomics for rhizosphere engineering,” in Rhizosphere Engineering, eds R. Dubey and P. Kumar (Amsterdam: Elsevier), 395–416.
Handelsman, J. (2003). Soils—the metagenomics approach. Microb. Divers. Bioprospecting 109–119. doi: 10.1128/9781555817770.ch11
Handelsman, J., Rondon, M. R., Brady, S. F., Clardy, J., and Goodman, R. M. (1998). Molecular biological access to the chemistry of unknown soil microbes: a new frontier for natural products. Chem. Biol. 5, R245–R249. doi: 10.1016/s1074-5521(98)90108-9
Henne, A., Daniel, R., Schmitz, R. A., and Gottschalk, G. (1999). Construction of environmental DNA libraries inEscherichia coli and screening for the presence of genes conferring utilization of 4-hydroxybutyrate. Appl. Environ. Microbiol. 65, 3901–3907. doi: 10.1128/AEM.65.9.3901-3907.1999
Hjort, K., Presti, I., Elväng, A., Marinelli, F., and Sjöling, S. (2014). Bacterial chitinase with phytopathogen control capacity from suppressive soil revealed by functional metagenomics. Appl. Microbiol. Biotechnol. 98, 2819–2828. doi: 10.1007/s00253-013-5287-x
Horton, M. W., Bodenhausen, N., Beilsmith, K., Meng, D., Muegge, B. D., Subramanian, S., et al. (2014). Genome-wide association study of Arabidopsis thaliana leaf microbial community. Nat. Commun. 5:5320. doi: 10.1038/ncomms6320
Hu, J., Okawa, H., Yamamoto, K., Oyama, K., Mitomi, M., and Anzai, H. (2011). Characterization of two cytochrome P450 monooxygenase genes of the pyripyropene biosynthetic gene cluster from Penicillium coprobium. J. Antibiot. (Tokyo) 64, 221–227. doi: 10.1038/ja.2010.162
Hua, W., El Sheikha, A. F., and Xu, J. (2018). “Molecular techniques for making recombinant enzymes used in food processing,” in Molecular Techniques in Food Biology: Safety, Biotechnology, Authenticity & Traceability, 1 Edn, eds A. El Sheikha, R. Levin, and J. Xu (Hoboken, NJ: John Wiley & Sons), 95–114. doi: 10.1002/9781119374633.ch5
Huson, D. H., Beier, S., Flade, I., Górska, A., El-Hadidi, M., Mitra, S., et al. (2016). MEGAN community edition-interactive exploration and analysis of large-scale microbiome sequencing data. PLoS Comput. Biol. 12:e1004957. doi: 10.1371/journal.pcbi.1004957
Iliev, I. D., Funari, V. A., Taylor, K. D., Nguyen, Q., Reyes, C. N., Strom, S. P., et al. (2012). Interactions between commensal fungi and the C-type lectin receptor Dectin-1 influence colitis. Science 336, 1314–1317. doi: 10.1126/science.1221789
Iqbal, H. A., Low-Beinart, L., Obiajulu, J. U., and Brady, S. F. (2016). Natural product discovery through improved functional metagenomics in Streptomyces. J. Am. Chem. Soc. 138, 9341–9344. doi: 10.1021/jacs.6b02921
James, S. E., de Oliveira, T., Baichoo, S., Fonseca, V., Kanzi, A. M., Moosa, Y., et al. (2019). Current affairs of microbial genome-wide association studies: approaches, bottlenecks and analytical pitfalls. Front. Microbiol. 10:3119. doi: 10.3389/fmicb.2019.03119
Jo, Y., Back, C.-G., Choi, H., and Cho, W. K. (2020). Comparative microbiome study of mummified peach fruits by metagenomics and metatranscriptomics. Plants 9:1052. doi: 10.3390/plants9081052
Jones, J. B., Lacy, G. H., Bouzar, H., Stall, R. E., and Schaad, N. W. (2004). Reclassification of the xanthomonads associated with bacterial spot disease of tomato and pepper. Syst Appl Microbiol. 27, 755–762. doi: 10.1078/0723202042369884
Jorquera, M. A., Shaharoona, B., Nadeem, S. M., de la Luz Mora, M., and Crowley, D. E. (2012). Plant growth-promoting rhizobacteria associated with ancient clones of creosote bush (Larrea tridentata). Microb. Ecol. 64, 1008–1017. doi: 10.1007/s00248-012-0071-5
Kanehisa, M., Goto, S., Sato, Y., Kawashima, M., Furumichi, M., and Tanabe, M. (2014). Data, information, knowledge and principle: back to metabolism in KEGG. Nucleic Acids Res. 42, D199–D205. doi: 10.1093/nar/gkt1076
Kang, D. D., Froula, J., Egan, R., and Wang, Z. (2015). MetaBAT, an efficient tool for accurately reconstructing single genomes from complex microbial communities. PeerJ 3:e1165. doi: 10.7717/peerj.1165
Kao-Kniffin, J., Carver, S. M., and DiTommaso, A. (2013). Advancing weed management strategies using metagenomic techniques. Weed Sci. 61, 171–184. doi: 10.1614/ws-d-12-00114.1
Karlsson, F. H., Tremaroli, V., Nookaew, I., Bergström, G., Behre, C. J., Fagerberg, B., et al. (2013). Gut metagenome in European women with normal, impaired and diabetic glucose control. Nature 498, 99–103. doi: 10.1038/nature12198
Kaushal, M., Mahuku, G., and Swennen, R. (2020). Metagenomic insights of the root colonizing microbiome associated with symptomatic and non-symptomatic bananas in fusarium wilt infected fields. Plants 9:263. doi: 10.3390/plants9020263
Kawasaki, A., Watson, E. R., and Kertesz, M. A. (2012). Indirect effects of polycyclic aromatic hydrocarbon contamination on microbial communities in legume and grass rhizospheres. Plant Soil 358, 169–182. doi: 10.1007/s11104-011-1089-z
Khoiri, A. N., Cheevadhanarak, S., Jirakkakul, J., Dulsawat, S., Prommeenate, P., Tachaleat, A., et al. (2021). Comparative metagenomics reveals microbial signatures of sugarcane phyllosphere in organic management. Front. Microbiol. 12:310. doi: 10.3389/fmicb.2021.623799
Kimes, N. E., Callaghan, A. V., Aktas, D. F., Smith, W. L., Sunner, J., Golding, B. T., et al. (2013). Metagenomic analysis and metabolite profiling of deep–sea sediments from the Gulf of Mexico following the Deepwater Horizon oil spill. Front. Microbiol. 4:50. doi: 10.3389/fmicb.2013.00050
King, R. W., Bauer, J. D., and Brady, S. F. (2009). An environmental DNA-derived type II polyketide biosynthetic pathway encodes the biosynthesis of the novel pentacyclic polyketide, erdacin. Angew. Chemie Int. Ed. 48, 6257–6261. doi: 10.1002/anie.200901209
Knietsch, A., Waschkowitz, T., Bowien, S., Henne, A., and Daniel, R. (2003). Construction and screening of metagenomic libraries derived from enrichment cultures: generation of a gene bank for genes conferring alcohol oxidoreductase activity on Escherichia coli. Appl. Environ. Microbiol. 69, 1408–1416. doi: 10.1128/aem.69.3.1408-1416.2003
Kõiv, V., Roosaare, M., Vedler, E., Kivistik, P. A., Toppi, K., Schryer, D. W., et al. (2015). Microbial population dynamics in response to Pectobacterium atrosepticum infection in potato tubers. Sci. Rep. 5:11606. doi: 10.1038/srep11606
Kunda, P., Dhal, P. K., and Mukherjee, A. (2018). Endophytic bacterial community of rice (Oryza sativa L.) from coastal saline zone of West Bengal: 16S rRNA gene based metagenomics approach. Meta Gene 18, 79–86. doi: 10.1016/j.mgene.2018.08.004
Kunin, V., Copeland, A., Lapidus, A., Mavromatis, K., and Hugenholtz, P. (2008). A bioinformatician’s guide to metagenomics. Microbiol. Mol. Biol. Rev. 72, 557–578. doi: 10.1128/MMBR.00009-08
Kwak, M.-J., Kong, H. G., Choi, K., Kwon, S.-K., Song, J. Y., Lee, J., et al. (2018). Rhizosphere microbiome structure alters to enable wilt resistance in tomato. Nat. Biotechnol. 36, 1100–1109. doi: 10.1038/nbt.4232
Kwok, K. T. T., Nieuwenhuijse, D. F., Phan, M. V. T., and Koopmans, M. P. G. (2020). Virus metagenomics in farm animals: a systematic review. Viruses 12:107. doi: 10.3390/v12010107
Kyselková, M., Kopecký, J., Frapolli, M., Défago, G., Ságová-Marečková, M., Grundmann, G. L., et al. (2009). Comparison of rhizobacterial community composition in soil suppressive or conducive to tobacco black root rot disease. ISME J. 3, 1127–1138. doi: 10.1038/ismej.2009.61
Larke-Mejia, N. L., Crombie, A., Pratscher, J., McGenity, T. J., and Murrell, C. (2019). Novel isoprene-degrading Proteobacteria from soil and leaves identified by cultivation and metagenomics analysis of stable isotope probing experiments. Front. Microbiol. 10:2700. doi: 10.3389/fmicb.2019.02700
Lavecchia, A., Curci, M., Jangid, K., Whitman, W. B., Ricciuti, P., Pascazio, S., et al. (2015). Microbial 16S gene-based composition of a sorghum cropped rhizosphere soil under different fertilization managements. Biol. Fertil. Soils 51, 661–672. doi: 10.1007/s00374-015-1017-0
Lees, J. A., Galardini, M., Bentley, S. D., Weiser, J. N., and Corander, J. (2018). Pyseer: a comprehensive tool for microbial pangenome-wide association studies. Bioinformatics 34, 4310–4312. doi: 10.1093/bioinformatics/bty539
Lees, J. A., Vehkala, M., Välimäki, N., Harris, S. R., Chewapreecha, C., Croucher, N. J., et al. (2016). Sequence element enrichment analysis to determine the genetic basis of bacterial phenotypes. Nat. Commun. 7:12797. doi: 10.1038/ncomms12797
Li, D., Liu, C.-M., Luo, R., Sadakane, K., and Lam, T.-W. (2015). MEGAHIT: an ultra-fast single-node solution for large and complex metagenomics assembly via succinct de Bruijn graph. Bioinformatics 31, 1674–1676. doi: 10.1093/bioinformatics/btv033
Liu, P., Wang, W., Zhao, J., and Wei, D. (2019). Screening novel β-galactosidases from a sequence-based metagenome and characterization of an alkaline β-galactosidase for the enzymatic synthesis of galactooligosaccharides. Protein Expr. Purif. 155, 104–111. doi: 10.1016/j.pep.2018.12.001
Liljeqvist, M., Ossandon, F. J., González, C., Rajan, S., Stell, A., Valdes, J., et al. (2015). Metagenomic analysis reveals adaptations to a cold-adapted lifestyle in a low-temperature acid mine drainage stream. FEMS Microbiol. Ecol. 91:fiv011. doi: 10.1093/femsec/fiv011
Links, M. G., Dumonceaux, T. J., Hemmingsen, S. M., and Hill, J. E. (2012). The chaperonin-60 universal target is a barcode for bacteria that enables de novo assembly of metagenomic sequence data. PLoS One 7:e49755. doi: 10.1371/journal.pone.0049755
Loman, N. J., Constantinidou, C., Christner, M., Rohde, H., Chan, J. Z.-M., Quick, J., et al. (2013). A culture-independent sequence-based metagenomics approach to the investigation of an outbreak of Shiga-toxigenic Escherichia coli O104: H4. JAMA 309, 1502–1510. doi: 10.1001/jama.2013.3231
Lorenzi, H. A., Hoover, J., Inman, J., Safford, T., Murphy, S., Kagan, L., et al. (2011). The Viral MetaGenome Annotation Pipeline (VMGAP): an automated tool for the functional annotation of viral Metagenomic shotgun sequencing data. Stand. Genomic Sci. 4, 418–429. doi: 10.4056/sigs.1694706
Lucas, J. A. (2011). Advances in plant disease and pest management. J. Agric. Sci. 149:91. doi: 10.1017/s0021859610000997
Luo, R., Liu, B., Xie, Y., Li, Z., Huang, W., Yuan, J., et al. (2012). SOAPdenovo2: an empirically improved memory-efficient short-read de novo assembler. Gigascience 1:18. doi: 10.1186/2047-217X-1-18
MacNeil, I. A., Tiong, C. L., Minor, C., August, P. R., Grossman, T. H., Loiacono, K. A., et al. (2001). Expression and isolation of antimicrobial small molecules from soil DNA libraries. J. Mol. Microbiol. Biotechnol. 3, 301–308.
Maruthamuthu, M., Jiménez, D. J., Stevens, P., and van Elsas, J. D. (2016). A multi-substrate approach for functional metagenomics-based screening for (hemi) cellulases in two wheat straw-degrading microbial consortia unveils novel thermoalkaliphilic enzymes. BMC Genomics 17:86. doi: 10.1186/s12864-016-2404-0
Meyer, F., Paarmann, D., D’Souza, M., Olson, R., Glass, E. M., Kubal, M., et al. (2008). The metagenomics RAST server–a public resource for the automatic phylogenetic and functional analysis of metagenomes. BMC Bioinformatics 9:386. doi: 10.1186/1471-2105-9-386
Mazzola, M., and Freilich, S. (2017). Prospects for biological soilborne disease control: application of indigenous versus synthetic microbiomes. Phytopathology 107, 256–263. doi: 10.1094/PHYTO-09-16-0330-RVW
Mazzola, M., and Manici, L. M. (2012). Apple replant disease: role of microbial ecology in cause and control. Annu. Rev. Phytopathol. 50, 45–65. doi: 10.1146/annurev-phyto-081211-173005
Mechan Llontop, M. E., Sharma, P., Aguilera Flores, M., Yang, S., Pollok, J., Tian, L., et al. (2020). Strain-level identification of bacterial tomato pathogens directly from metagenomic sequences. Phytopathology 110, 768–779. doi: 10.1094/PHYTO-09-19-0351-R
Meena, K. K., Sorty, A. M., Bitla, U. M., Choudhary, K., Gupta, P., Pareek, A., et al. (2017). Abiotic stress responses and microbe-mediated mitigation in plants: the omics strategies. Front. Plant Sci. 8:172. doi: 10.3389/fpls.2017.00172
Meenatchi, R., Thinesh, T., Brindangnanam, P., Hassan, S., Kiran, G. S., and Selvin, J. (2020). Revealing the impact of global mass bleaching on coral microbiome through 16S rRNA gene-based metagenomic analysis. Microbiol. Res. 233:126408. doi: 10.1016/j.micres.2019.126408
Mendes, L. W., Raaijmakers, J. M., de Hollander, M., Mendes, R., and Tsai, S. M. (2018). Influence of resistance breeding in common bean on rhizosphere microbiome composition and function. ISME J. 12, 212–224. doi: 10.1038/ismej.2017.158
Mendes, R., Kruijt, M., De Bruijn, I., Dekkers, E., van der Voort, M., Schneider, J. H. M., et al. (2011). Deciphering the rhizosphere microbiome for disease-suppressive bacteria. Science 332, 1097–1100. doi: 10.1126/science.1203980
Mercier, J., and Jiménez, J. I. (2009). Demonstration of the biofumigation activity of Muscodor albus against Rhizoctonia solani in soil and potting mix. Biocontrol 54:797. doi: 10.1007/s10526-009-9223-z
Methe, B. A., Li, K., Talley, S. P., Gupta, N., Frank, B., Xu, W., et al. (2017). Functional genes that distinguish Maize phyllosphere metagenomes in drought and well-watered conditions. bioRxiv [Preprint] doi: 10.1101/104331
Mewis, K., Taupp, M., and Hallam, S. J. (2011). A high throughput screen for biomining cellulase activity from metagenomic libraries. J. Vis. Exp. 48:e2461. doi: 10.3791/2461
Meyer, J. L., Paul, V. J., Raymundo, L. J., and Teplitski, M. (2017). Comparative metagenomics of the polymicrobial black band disease of corals. Front. Microbiol. 8:618. doi: 10.3389/fmicb.2017.00618
Miller, R. R., Montoya, V., Gardy, J. L., Patrick, D. M., and Tang, P. (2013). Metagenomics for pathogen detection in public health. Genome Med. 5:81. doi: 10.1186/gm485
Moreno-Indias, I., and Tinahones, F. J. (2020). “Metagenomics,” in Principles of Nutrigenetics and Nutrigenomics, eds R. Caterina, A. Martinez, and M. Kohlmeier (Amsterdam: Elsevier), 81–87.
Müller-Stöver, D., Nybroe, O., Baraibar, B., Loddo, D., Eizenberg, H., French, K., et al. (2016). Contribution of the seed microbiome to weed management. Weed Res. 56, 335–339. doi: 10.1111/wre.12218
Neubauer, C., Heitmann, B., and Müller, C. (2014). Biofumigation potential of Brassicaceae cultivars to Verticillium dahliae. Eur. J. Plant Pathol. 140, 341–352. doi: 10.1007/s10658-014-0467-9
Nikolic, B., Schwab, H., and Sessitsch, A. (2011). Metagenomic analysis of the 1-aminocyclopropane-1-carboxylate deaminase gene (acdS) operon of an uncultured bacterial endophyte colonizing Solanum tuberosum L. Arch. Microbiol. 193, 665–676. doi: 10.1007/s00203-011-0703-z
Njoroge, S. M. C., Kabir, Z., Martin, F. N., Koike, S. T., and Subbarao, K. V. (2009). Comparison of crop rotation for Verticillium wilt management and effect on Pythium species in conventional and organic strawberry production. Plant Dis. 93, 519–527. doi: 10.1094/PDIS-93-5-0519
Nurk, S., Bankevich, A., Antipov, D., Gurevich, A. A., Korobeynikov, A., Lapidus, A., et al. (2013). Assembling single-cell genomes and mini-metagenomes from chimeric MDA products. J. Comput. Biol. 20, 714–737. doi: 10.1089/cmb.2013.0084
Núñez, A., Amo de Paz, G., Rastrojo, A., García, A. M., Alcamí, A., Gutiérrez-Bustillo, A. M., et al. (2016). Monitoring of airborne biological particles in outdoor atmosphere. Part 2: metagenomics applied to urban environments. Int. Microbiol. 19, 69–80. doi: 10.2436/20.1501.01.265
Obiol, A., Giner, C. R., Sánchez, P., Duarte, C. M., Acinas, S. G., and Massana, R. (2020). A metagenomic assessment of microbial eukaryotic diversity in the global ocean. Mol. Ecol. Resour. 20, 718–731. doi: 10.1111/1755-0998.13147
Oliveira, C., Shakiba, E., North, D., McGraw, M., Ballard, E., Barrett-D’Amico, M., et al. (2022). 16S rRNA gene-based metagenomic analysis of rhizosphere soil bacteria in arkansas rice crop fields. Agronomy 12:222. doi: 10.3390/agronomy12010222
Orellana, L. H., Chee-Sanford, J. C., Sanford, R. A., Löffler, F. E., and Konstantinidis, K. T. (2018). Year-round shotgun metagenomes reveal stable microbial communities in agricultural soils and novel ammonia oxidizers responding to fertilization. Appl. Environ. Microbiol. 84:e01646–17. doi: 10.1128/AEM.01646-17
Orvis, J., Crabtree, J., Galens, K., Gussman, A., Inman, J. M., Lee, E., et al. (2010). Ergatis: a web interface and scalable software system for bioinformatics workflows. Bioinformatics 26, 1488–1492. doi: 10.1093/bioinformatics/btq167
Overbeek, R., Begley, T., Butler, R. M., Choudhuri, J. V., Chuang, H.-Y., Cohoon, M., et al. (2005). The subsystems approach to genome annotation and its use in the project to annotate 1000 genomes. Nucleic Acids Res. 33, 5691–5702. doi: 10.1093/nar/gki866
Owen, J. G., Reddy, B. V. B., Ternei, M. A., Charlop-Powers, Z., Calle, P. Y., Kim, J. H., et al. (2013). Mapping gene clusters within arrayed metagenomic libraries to expand the structural diversity of biomedically relevant natural products. Proc. Natl. Acad. Sci. U.S.A. 110, 11797–11802. doi: 10.1073/pnas.1222159110
Pacwa-Płociniczak, M., Płaza, G. A., Piotrowska-Seget, Z., and Cameotra, S. S. (2011). Environmental applications of biosurfactants: recent advances. Int. J. Mol. Sci. 12, 633–654. doi: 10.3390/ijms12010633
Parakhia, M. V., and Golakiya, B. A. (2018). Manipulation of phytobiome: a new concept to control the plant disease and improve the productivity. J Bacteriol Mycol Open Access 6, 322–324. doi: 10.15406/jbmoa.2018.06.00227
Park, J.-K., Lee, S.-H., Han, S., Kim, J.-C., Cheol, Y., and Gardener, B. M. (2013). Marker-assisted selection of novel bacteria contributing to soil-borne plant disease suppression. Mol. Microb. Ecol. Rhizosph. 2, 637–642. doi: 10.1002/9781118297674.ch60
Paul, E. A., and Clark, F. E. (1989). Soil Microbiology and Biochemistry. San Diego, CA: Academic Press. Inc.
Pawar, S. V., Hallam, S. J., and Yadav, V. G. (2018). Metagenomic discovery of a novel transaminase for valorization of monoaromatic compounds. RSC Adv. 8, 22490–22497. doi: 10.1039/c8ra02764a
Peiffer, J. A., Spor, A., Koren, O., Jin, Z., Tringe, S. G., Dangl, J. L., et al. (2013). Diversity and heritability of the maize rhizosphere microbiome under field conditions. Proc. Natl. Acad. Sci. U.S.A. 110, 6548–6553. doi: 10.1073/pnas.1302837110
Pii, Y., Borruso, L., Brusetti, L., Crecchio, C., Cesco, S., and Mimmo, T. (2016). The interaction between iron nutrition, plant species and soil type shapes the rhizosphere microbiome. Plant Physiol. Biochem. 99, 39–48. doi: 10.1016/j.plaphy.2015.12.002
Pooja, S., Pushpanathan, M., Jayashree, S., Gunasekaran, P., and Rajendhran, J. (2015). Identification of periplasmic α-amlyase from cow dung metagenome by product induced gene expression profiling (pigex). Indian J. Microbiol. 55, 57–65. doi: 10.1007/s12088-014-0487-3
Poudel, R., Jumpponen, A., Kennelly, M. M., Rivard, C. L., Gomez-Montano, L., and Garrett, K. A. (2019). Rootstocks shape the rhizobiome: rhizosphere and endosphere bacterial communities in the grafted tomato system. Appl. Environ. Microbiol. 85:e01765-18. doi: 10.1128/AEM.01765-18
Powell, S., Forslund, K., Szklarczyk, D., Trachana, K., Roth, A., Huerta-Cepas, J., et al. (2014). eggNOG v4. 0: nested orthology inference across 3686 organisms. Nucleic Acids Res. 42, D231–D239. doi: 10.1093/nar/gkt1253
Power, A. G. (2010). Ecosystem services and agriculture: tradeoffs and synergies. Philos. Trans. R. Soc. B Biol. Sci. 365, 2959–2971. doi: 10.1098/rstb.2010.0143
Pruesse, E., Quast, C., Knittel, K., Fuchs, B. M., Ludwig, W., Peplies, J., et al. (2007). SILVA: a comprehensive online resource for quality checked and aligned ribosomal RNA sequence data compatible with ARB. Nucleic Acids Res. 35, 7188–7196. doi: 10.1093/nar/gkm864
Pushkarev, A., Inoue, K., Larom, S., Flores-Uribe, J., Singh, M., Konno, M., et al. (2018). A distinct abundant group of microbial rhodopsins discovered using functional metagenomics. Nature 558, 595–599. doi: 10.1038/s41586-018-0225-9
Rahman, A., Hallgrímsdóttir, I., Eisen, M., and Pachter, L. (2018). Association mapping from sequencing reads using k-mers. Elife 7:e32920. doi: 10.7554/eLife.32920
Rees, H. C., Grant, S., Jones, B., Grant, W. D., and Heaphy, S. (2003). Detecting cellulase and esterase enzyme activities encoded by novel genes present in environmental DNA libraries. Extremophiles 7, 415–421. doi: 10.1007/s00792-003-0339-2
Richter, D. D., and Markewitz, D. (1995). How deep is soil? Bioscience 45, 600–609. doi: 10.1097/01.hp.0000142499.92778.76
Riesenfeld, C. S., Goodman, R. M., and Handelsman, J. (2004). Uncultured soil bacteria are a reservoir of new antibiotic resistance genes. Environ. Microbiol. 6, 981–989. doi: 10.1111/j.1462-2920.2004.00664.x
Rodríguez-Segura, Z., Chen, J., Villalobos, F. J., Gill, S., and Nuñez-Valdez, M. E. (2012). The lipopolysaccharide biosynthesis core of the Mexican pathogenic strain Serratia entomophila is associated with toxicity to larvae of Phyllophaga blanchardi. J. Invertebr. Pathol. 110, 24–32. doi: 10.1016/j.jip.2012.01.008
Rodriguez-R, L. M., Tsementzi, D., Luo, C., and Konstantinidis, K. T. (2020). Iterative subtractive binning of freshwater chronoseries metagenomes identifies over 400 novel species and their ecologic preferences. Environ. Microbiol. 22, 3394–3412. doi: 10.1111/1462-2920.15112
Rosenzweig, N., Tiedje, J. M., Quensen, J. F. III, Meng, Q., and Hao, J. J. (2012). Microbial communities associated with potato common scab-suppressive soil determined by pyrosequencing analyses. Plant Dis. 96, 718–725. doi: 10.1094/PDIS-07-11-0571
Rubiales, D., Fondevilla, S., Chen, W., Gentzbittel, L., Higgins, T. J. V., Castillejo, M. A., et al. (2015). Achievements and challenges in legume breeding for pest and disease resistance. CRC Crit. Rev. Plant Sci. 34, 195–236. doi: 10.1080/07352689.2014.898445
Runia, W. T., Molendijk, L. P. G., Ludeking, D. J. W., and Schomaker, C. H. (2012). Improvement of anaerobic soil disinfestation. Commun. Agric. Appl. Biol. Sci. 77, 753–762.
Sachdev, D. P., and Cameotra, S. S. (2013). Biosurfactants in agriculture. Appl. Microbiol. Biotechnol. 97, 1005–1016.
Saha, S., Johnson, J., Pal, S., Weinstock, G. M., and Rajasekaran, S. (2019). MSC: a metagenomic sequence classification algorithm. Bioinformatics 35, 2932–2940. doi: 10.1093/bioinformatics/bty1071
Salvetti, E., Campanaro, S., Campedelli, I., Fracchetti, F., Gobbi, A., Tornielli, G. B., et al. (2016). Whole-metagenome-sequencing-based community profiles of Vitis vinifera L. cv. Corvina berries withered in two post-harvest conditions. Front. Microbiol. 7:937. doi: 10.3389/fmicb.2016.00937
Sarangi, M., Chopra, C., Usman, Y. A., Dhanjal, D. S., and Chopra, R. S. (2019). Accessing genetic diversity and phylogenetic analysis of microbial population of soil from Hygam Wetland of Kashmir Valley. Res. J. Pharm. Technol. 12, 2323–2326. doi: 10.5958/0974-360x.2019.00391.3
Saravanan, J., and Suneetha, V. (2017). Asymmetric synthesis of chiral alcohols for API production using microorganisms-A mini review. Res. J. Pharm. Technol. 10, 1881–1885. doi: 10.5958/0974-360x.2017.00330.4
Schloss, P. D., Westcott, S. L., Ryabin, T., Hall, J. R., Hartmann, M., Hollister, E. B., et al. (2009). Introducing mothur: open-source, platform-independent, community-supported software for describing and comparing microbial communities. Appl. Environ. Microbiol. 75, 7537–7541. doi: 10.1128/AEM.01541-09
Schmid, F., Moser, G., Müller, H., and Berg, G. (2011). Functional and structural microbial diversity in organic and conventional viticulture: organic farming benefits natural biocontrol agents. Appl. Environ. Microbiol. 77, 2188–2191. doi: 10.1128/AEM.02187-10
Schmidt, T. M., DeLong, E. F., and Pace, N. R. (1991). Analysis of a marine picoplankton community by 16S rRNA gene cloning and sequencing. J. Bacteriol. 173, 4371–4378. doi: 10.1128/jb.173.14.4371-4378.1991
Scholes, M. C., and Scholes, R. J. (2013). Dust unto dust. Science 342, 565–566. doi: 10.1126/science.1244579
Scholz, M. B., Lo, C.-C., and Chain, P. S. G. (2012). Next generation sequencing and bioinformatic bottlenecks: the current state of metagenomic data analysis. Curr. Opin. Biotechnol. 23, 9–15. doi: 10.1016/j.copbio.2011.11.013
Schulz, F., Roux, S., Paez-Espino, D., Jungbluth, S., Walsh, D. A., Denef, V. J., et al. (2020). Giant virus diversity and host interactions through global metagenomics. Nature 578, 432–436. doi: 10.1038/s41586-020-1957-x
Semenov, M. V. (2021). Metabarcoding and metagenomics in soil ecology research: achievements, challenges, and prospects. Biol. Bull. Rev. 11, 40–53. doi: 10.1134/s2079086421010084
Sengupta, S., Ganguli, S., and Singh, P. K. (2017). Metagenome analysis of the root endophytic microbial community of Indian rice (O. sativa L.). Genomics Data 12, 41–43. doi: 10.1016/j.gdata.2017.02.010
Seshadri, R., Kravitz, S. A., Smarr, L., Gilna, P., and Frazier, M. (2007). CAMERA: a community resource for metagenomics. PLoS Biol 5:e75. doi: 10.1371/journal.pbio.0050075
Sessitsch, A., Hardoim, P., Döring, J., Weilharter, A., Krause, A., Woyke, T., et al. (2012). Functional characteristics of an endophyte community colonizing rice roots as revealed by metagenomic analysis. Mol. Plant Microbe Interact. 25, 28–36. doi: 10.1094/MPMI-08-11-0204
Shah, R. K., Patel, A. K., Davla, D. M., Parikh, I. K., Subramanian, R. B., Patel, K. C., et al. (2017). Molecular cloning, heterologous expression, and functional characterization of a cellulolytic enzyme (Cel PRII) from buffalo rumen metagenome. 3 Biotech 7:257. doi: 10.1007/s13205-017-0895-2
Shakya, M., Quince, C., Campbell, J. H., Yang, Z. K., Schadt, C. W., and Podar, M. (2013). Comparative metagenomic and rRNA microbial diversity characterization using archaeal and bacterial synthetic communities. Environ. Microbiol. 15, 1882–1899. doi: 10.1111/1462-2920.12086
Sharma, P., Kumar, S., and Pandey, A. (2021). Bioremediated techniques for remediation of metal pollutants using metagenomics approaches: a review. J. Environ. Chem. Eng. 9:105684. doi: 10.1007/398_2019_24
Singh, K. M., Ahir, V. B., Tripathi, A. K., Ramani, U. V., Sajnani, M., Koringa, P. G., et al. (2012). Metagenomic analysis of Surti buffalo (Bubalus bubalis) rumen: a preliminary study. Mol. Biol. Rep. 39, 4841–4848. doi: 10.1007/s11033-011-1278-0
Soares, F. L., Marcon, J., Pereira E Silva, M. D. C., Khakhum, N., Cerdeira, L. T., Ottoni, J. R., et al. (2017). A novel multifunctional β-N-acetylhexosaminidase revealed through metagenomics of an oil-spilled mangrove. Bioengineering 4:62. doi: 10.3390/bioengineering4030062
Sree, B. R., Sowjanya, B., and Divakar, K. (2019). Metagenomic bioprospecting of novel oxygen insensitive nitroreductase for degradation of nitro aromatic compounds. Int. Biodeterior. Biodegradation 143:104737. doi: 10.1016/j.ibiod.2019.104737
Srivastava, R., Srivastava, A. K., Ramteke, P. W., Gupta, V. K., and Srivastava, A. K. (2020). Metagenome dataset of wheat rhizosphere from Ghazipur region of Eastern Uttar Pradesh. Data Brief 28:105094. doi: 10.1016/j.dib.2019.105094
Staub, J. M., Brand, L., Tran, M., Kong, Y., and Rogers, S. G. (2012). Bacterial glyphosate resistance conferred by overexpression of an E. coli membrane efflux transporter. J. Ind. Microbiol. Biotechnol. 39, 641–647. doi: 10.1007/s10295-011-1057-x
Stein, J. L., Marsh, T. L., Wu, K. Y., Shizuya, H., and DeLong, E. F. (1996). Characterization of uncultivated prokaryotes: isolation and analysis of a 40-kilobase-pair genome fragment from a planktonic marine archaeon. J. Bacteriol. 178, 591–599. doi: 10.1128/jb.178.3.591-599.1996
Subrahmanyam, G., Kumar, A., Sandilya, S. P., Chutia, M., and Yadav, A. N. (2020). “Diversity, plant growth promoting attributes, and agricultural applications of rhizospheric microbes,” in Plant Microbiomes for Sustainable Agriculture, eds A. Yadav, J. Singh, A. Rastegari, and N. Yadav (Cham: Springer), 1–52. doi: 10.1007/s42729-021-00451-x
Suenaga, H., Ohnuki, T., and Miyazaki, K. (2007). Functional screening of a metagenomic library for genes involved in microbial degradation of aromatic compounds. Environ. Microbiol. 9, 2289–2297. doi: 10.1111/j.1462-2920.2007.01342.x
Sutherland, T. D., Horne, I., Russell, R. J., and Oakeshott, J. G. (2002). Gene cloning and molecular characterization of a two-enzyme system catalyzing the oxidative detoxification of β-endosulfan. Appl. Environ. Microbiol. 68, 6237–6245. doi: 10.1128/AEM.68.12.6237-6245.2002
Tan, H., Mooij, M. J., Barret, M., Hegarty, P. M., Harington, C., Dobson, A. D., et al. (2014). Identification of novel phytase genes from an agricultural soil-derived metagenome. J. Microbiol. Biotechnol. 24, 113–118. doi: 10.4014/jmb.1307.07007
Tandon, D., Haque, M. M., and Mande, S. S. (2016). Inferring intra-community microbial interaction patterns from metagenomic datasets using associative rule mining techniques. PLoS One 11:e0154493. doi: 10.1371/journal.pone.0154493
Tarnecki, A. M., Burgos, F. A., Ray, C. L., and Arias, C. R. (2017). Fish intestinal microbiome: diversity and symbiosis unravelled by metagenomics. J. Appl. Microbiol. 123, 2–17. doi: 10.1111/jam.13415
Taş, N., de Jong, A. E. E., Li, Y., Trubl, G., Xue, Y., and Dove, N. C. (2021). Metagenomic tools in microbial ecology research. Curr. Opin. Biotechnol. 67, 184–191. doi: 10.1016/j.copbio.2021.01.019
Thies, S., Rausch, S. C., Kovacic, F., Schmidt-Thaler, A., Wilhelm, S., Rosenau, F., et al. (2016). Metagenomic discovery of novel enzymes and biosurfactants in a slaughterhouse biofilm microbial community. Sci. Rep. 6:27035. doi: 10.1038/srep27035
Thornbury, M., Sicheri, J., Slaine, P., Getz, L. J., Finlayson-Trick, E., Cook, J., et al. (2019). Characterization of novel lignocellulose-degrading enzymes from the porcupine microbiome using synthetic metagenomics. PLoS One 14:e0209221. doi: 10.1371/journal.pone.0209221
Thurber, R. V., Haynes, M., Breitbart, M., Wegley, L., and Rohwer, F. (2009). Laboratory procedures to generate viral metagenomes. Nat. Protoc. 4:470. doi: 10.1038/nprot.2009.10
Tilman, D., Balzer, C., Hill, J., and Befort, B. L. (2011). Global food demand and the sustainable intensification of agriculture. Proc. Natl. Acad. Sci. U.S.A. 108, 20260–20264. doi: 10.1073/pnas.1116437108
Tiwari, R., Nain, L., Labrou, N. E., and Shukla, P. (2018). Bioprospecting of functional cellulases from metagenome for second generation biofuel production: a review. Crit. Rev. Microbiol. 44, 244–257. doi: 10.1080/1040841X.2017.1337713
Toulza, E., Tagliabue, A., Blain, S., and Piganeau, G. (2012). Analysis of the global ocean sampling (GOS) project for trends in iron uptake by surface ocean microbes. PLoS One 7:e30931. doi: 10.1371/journal.pone.0030931
Tringe, S. G., Von Mering, C., Kobayashi, A., Salamov, A. A., Chen, K., Chang, H. W., et al. (2005). Comparative metagenomics of microbial communities. Science 308, 554–557.
Trognitz, F., Hackl, E., Widhalm, S., and Sessitsch, A. (2016). The role of plant–microbiome interactions in weed establishment and control. FEMS Microbiol. Ecol. 92:fiw138. doi: 10.1093/femsec/fiw138
Tyson, G. W., Chapman, J., Hugenholtz, P., Allen, E. E., Ram, R. J., Richardson, P. M., et al. (2004). Community structure and metabolism through reconstruction of microbial genomes from the environment. Nature 428, 37–43. doi: 10.1038/nature02340
Van Horn, D. J., Okie, J. G., Buelow, H. N., Gooseff, M. N., Barrett, J. E., and Takacs-Vesbach, C. D. (2014). Soil microbial responses to increased moisture and organic resources along a salinity gradient in a polar desert. Appl. Environ. Microbiol. 80, 3034–3043. doi: 10.1128/AEM.03414-13
Vitullo, D., Altieri, R., Esposito, A., Nigro, F., Ferrara, M., Alfano, G., et al. (2013). Suppressive biomasses and antagonist bacteria for an eco-compatible control of Verticillium dahliae on nursery-grown olive plants. Int. J. Environ. Sci. Technol. 10, 209–220. doi: 10.1007/s13762-012-0145-4
Vogel, T. M., Simonet, P., Jansson, J. K., Hirsch, P. R., Tiedje, J. M., Van Elsas, J. D., et al. (2009). TerraGenome: a consortium for the sequencing of a soil metagenome. Nat. Rev. Microbiol. 7, 252. doi: 10.1038/nrmicro2119
Voget, S., Leggewie, C., Uesbeck, A., Raasch, C., Jaeger, K.-E., and Streit, W. R. (2003). Prospecting for novel biocatalysts in a soil metagenome. Appl. Environ. Microbiol. 69, 6235–6242. doi: 10.1128/AEM.69.10.6235-6242.2003
Wagner, M. R., Lundberg, D. S., Coleman-Derr, D., Tringe, S. G., Dangl, J. L., and Mitchell-Olds, T. (2014). Natural soil microbes alter flowering phenology and the intensity of selection on flowering time in a wild Arabidopsis relative. Ecol. Lett. 17, 717–726. doi: 10.1111/ele.12276
Wang, G.-Y.-S., Graziani, E., Waters, B., Pan, W., Li, X., McDermott, J., et al. (2000). Novel natural products from soil DNA libraries in a streptomycete host. Org. Lett. 2, 2401–2404. doi: 10.1021/ol005860z
Wani, A. K., Rahayu, F., Kadarwati, F. T., Suhara, C., Singh, R., Dhanjal, D. S., et al. (2022). “Metagenomic screening strategies for bioprospecting enzymes from environmental samples,” in Proceeedings of the 2nd IOP Conference Series: Earth and Environmental Science, (Bristol: IOP Publishing), 12003.
Wei, Z., Gu, Y., Friman, V.-P., Kowalchuk, G. A., Xu, Y., Shen, Q., et al. (2019). Initial soil microbiome composition and functioning predetermine future plant health. Sci. Adv. 5:eaaw0759. doi: 10.1126/sciadv.aaw0759
Weissbrod, O., Rothschild, D., Barkan, E., and Segal, E. (2018). Host genetics and microbiome associations through the lens of genome wide association studies. Curr. Opin. Microbiol. 44, 9–19. doi: 10.1016/j.mib.2018.05.003
White, J. R., Maddox, C., White, O., Angiuoli, S. V., and Fricke, W. F. (2013). CloVR-ITS: automated internal transcribed spacer amplicon sequence analysis pipeline for the characterization of fungal microbiota. Microbiome 1:6. doi: 10.1186/2049-2618-1-6
Wilkinson, T., Korir, D., Ogugo, M., Stewart, R. D., Watson, M., Paxton, E., et al. (2020). 1200 high-quality metagenome-assembled genomes from the rumen of African cattle and their relevance in the context of sub-optimal feeding. Genome Biol. 21, 1–25. doi: 10.1186/s13059-020-02144-7
Wille, L., Messmer, M. M., Studer, B., and Hohmann, P. (2019). Insights to plant–microbe interactions provide opportunities to improve resistance breeding against root diseases in grain legumes. Plant. Cell Environ. 42, 20–40. doi: 10.1111/pce.13214
Willersinn, C., Mack, G., Mouron, P., Keiser, A., and Siegrist, M. (2015). Quantity and quality of food losses along the Swiss potato supply chain: stepwise investigation and the influence of quality standards on losses. Waste Manag. 46, 120–132. doi: 10.1016/j.wasman.2015.08.033
Wood, D. E., and Salzberg, S. L. (2014). Kraken: ultrafast metagenomic sequence classification using exact alignments. Genome Biol. 15:R46. doi: 10.1186/gb-2014-15-3-r46
Wong, D. W. S., Chan, V. J., and McCormack, A. A. (2009). Functional cloning and expression of a novel endo-α-1, 5-L-arabinanase from a metagenomic library. Protein Pept. Lett. 16, 1435–1441. doi: 10.2174/092986609789839313
Woyke, T., Xie, G., Copeland, A., Gonzalez, J. M., Han, C., Kiss, H., et al. (2009). Assembling the marine metagenome, one cell at a time. PLoS One 4:e5299. doi: 10.1371/journal.pone.0005299
Wu, Y.-W., Tang, Y.-H., Tringe, S. G., Simmons, B. A., and Singer, S. W. (2014). MaxBin: an automated binning method to recover individual genomes from metagenomes using an expectation-maximization algorithm. Microbiome 2:26. doi: 10.1186/2049-2618-2-26
Xin, Y., Guo, T., Zhang, Y., Wu, J., and Kong, J. (2019). A new β-galactosidase extracted from the infant feces with high hydrolytic and transgalactosylation activity. Appl. Microbiol. Biotechnol. 103, 8439–8448. doi: 10.1007/s00253-019-10092-x
Yan, S., Liu, H., Mohr, T. J., Jenrette, J., Chiodini, R., Zaccardelli, M., et al. (2008). Role of recombination in the evolution of the model plant pathogen Pseudomonas syringae pv. tomato DC3000, a very atypical tomato strain. Appl. Environ. Microbiol. 74, 3171–3181. doi: 10.1128/AEM.00180-08
Zhou, C., Zhao, H., Xiao, X., Guo, R., Wang, Q., Chen, H., et al. (2020). Metagenomic profiling of the pro-inflammatory gut microbiota in ankylosing spondylitis. J. Autoimmun. 107:102360. doi: 10.1016/j.jaut.2019.102360
Zhu, W., Lomsadze, A., and Borodovsky, M. (2010). Ab initio gene identification in metagenomic sequences. Nucleic Acids Res. 38:e132. doi: 10.1093/nar/gkq275
Zipperer, A., Konnerth, M. C., Laux, C., Berscheid, A., Janek, D., Weidenmaier, C., et al. (2016). Human commensals producing a novel antibiotic impair pathogen colonization. Nature 535, 511–516. doi: 10.1038/nature18634
Keywords: agriculture, metagenomics-wide association studies, metagenome, software tools, web resources
Citation: Iquebal MA, Jagannadham J, Jaiswal S, Prabha R, Rai A and Kumar D (2022) Potential Use of Microbial Community Genomes in Various Dimensions of Agriculture Productivity and Its Management: A Review. Front. Microbiol. 13:708335. doi: 10.3389/fmicb.2022.708335
Received: 11 May 2021; Accepted: 17 March 2022;
Published: 17 May 2022.
Edited by:
Robert Czajkowski, University of Gdańsk, PolandReviewed by:
Aly Farag El Sheikha, Jiangxi Agricultural University, ChinaDaljeet Singh Dhanjal, Lovely Professional University, India
Copyright © 2022 Iquebal, Jagannadham, Jaiswal, Prabha, Rai and Kumar. This is an open-access article distributed under the terms of the Creative Commons Attribution License (CC BY). The use, distribution or reproduction in other forums is permitted, provided the original author(s) and the copyright owner(s) are credited and that the original publication in this journal is cited, in accordance with accepted academic practice. No use, distribution or reproduction is permitted which does not comply with these terms.
*Correspondence: Dinesh Kumar, dinesh.kumar@icar.gov.in
†These authors have contributed equally to this work