- 1Department of Breast Surgical Oncology, Clinical Oncology School of Fujian Medical University, Fujian Cancer Hospital, Fuzhou, China
- 2Department of Pathology, The First Affiliated Hospital of Guangdong University of Pharmacy, Guangzhou, China
- 3Institute of Medical Biology, Chinese Academy of Medical Sciences and Peking Union Medical College, Kunming, China
- 4Department of Pathology, Maternity and Child Healthcare Hospital of Longhua District, Shenzhen, China
- 5Department of Nuclear Medicine, Clinical Oncology School of Fujian Medical University, Fujian Cancer Hospital, Fuzhou, China
Introduction: In December 2019, a novel epidemic of coronavirus pneumonia (COVID-19) was reported,and population-based studies had shown that cancer was a risk factor for death from COVID-19 infection. However, the molecular mechanism between COVID-19 and cancer remains indistinct. In this paper, we analyzed the nucleic acid sensor (DDX58) of SARS-CoV-2 virus, which is a significant gene related to virus infection. For purpose of clarifying the characteristics of DDX58 expression in malignant tumors, this study began to systematically analyze the DDX58 expression profile in the entire cancer type spectrum.
Methods: Using TCGA pan-cancer database and related data resources, we analyzed the expression, survival analysis, methylation expression, mutation status, microsatellite instability (MSI), immune related microenvironment, gene related network, function and drug sensitivity of DDX58.
Results: The expression level of DDX58 mRNA in most cancers was higher than the expression level in normal tissues. Through TIMER algorithm mining, we found that DDX58 expression was closely related to various levels of immune infiltration in pan-cancer. The promoter methylation level of DDX58 was significantly increased in multiple cancers. In addition, abnormal expression of DDX58 was related to MSI and TMB in multiple cancers, and the most common type of genomic mutation was “mutation.” In the protein–protein interaction (PPI) network, we found that type I interferon, phagocytosis, ubiquitinase, and tumor pathways were significantly enriched. Finally, according to the expression of DDX58 indicated potential sensitive drugs such as Cediranib, VE−821, Itraconazole, JNJ−42756493, IWR−1, and Linsitinib.
Discussion: In conclusion, we had gained new insights into how DDX58 might contribute to tumor development, and DDX58 could be used as an immune-related biomarker and as a potential immunotherapeutic target for COVID-19 infected cancer patients.
1. Introduction
Globally, 590 million cases of COVID-19 infection and 6.4 million deaths have been reported as of August 15, 2022. A recent study found that about 66 cancer patients were immunosuppressed with increasing susceptibility to infection and risk of serious complications (Al-Quteimat and Amer, 2020). Compared with other diseases, the genomes of 68 cancers have been fully studied. However, the gene information associated with COVID-19 remains largely unknown.
Genome-wide association study on COVID-19 patients with severe and critical illness showed that DDX58 gene was closely associated to severe COVID-19. It is urgent to study the role of this gene in different cancers. RIG-I or DExD/H-box helicase 58 (DDX58) is a protein that recognizes viral double-stranded RNA and produces type I interferon, an antiviral and innate immune response medium as previous described (Morelli et al., 2021). At the same time, DDX58 is considered as a potential novel target for COVID-19 treatment and a key component of COVID-19 infection and progress (Yamada et al., 2021). In cancer patients exposed to viruses, their condition worsened and their mortality increased (Han et al., 2021). Therefore, we aimed to find the role of DDX58 in cancer immunotherapy, in order to provide a more suitable treatment idea for cancer patients infected with COVID-19.
Here, we showed the landscape analysis of DDX58 expression level in healthy tissues and pan-cancer tissues using GTEx and TCGA, and then studied the relationship between DDX58 and various tumor prognoses. We explored the relationship between DDX58 and immune cell infiltration in specific tumor patients and studied the potential role of DDX58 in tumor patients, we also analyzed the methylation profile of DDX58 promoter and the mutation of DDX58 in the UALCAN database. These findings might have important significance in preventing SARS CoV-2 infection and mitigate cytokine storm in patients infected with cancer. This study might also point out the therapeutic potential of DDX58 inhibitors in preventing or mitigating SARS CoV-2 infection in specific cancer patients.
2. Materials and methods
2.1. Transcriptome data analysis
TCGA database and genotypic tissue expression (GTEx) database were used to obtain gene expression profiles. An analysis of 31 normal tissues was performed using mRNA data obtained from the GTEx project. Cancer cell lines were analyzed in 31 tissues according to their expression levels, and then the Kruskal Wallis test was performed on the mRNA data between adjacent tissues and tumor tissues, as well as healthy tissues and tumor tissues, to determine the difference of DDX58 expression. DDX58 expression levels were compared between healthy tissues and tumor tissues, as well as between adjacent tumor tissues and tumor tissues.
HPA1 contains normal tissue and tumor tissue protein levels of human gene expression profile information. In this study, we compared the expression of DDX58 protein in normal tissues and cancer tissues of four different organs by HPA. The significance of the difference was calculated using the Wilcoxon test. p < 0.05 suggests that the expression of tumor tissue is different from that of normal tissue.
2.2. Clinical relevance analysis
The expression level of DDX58 was examined using univariate COX regression analysis to determine whether it was associated with tumor prognosis in various cancers. According to the median of DDX58, samples were divided into two groups based on their expression levels: high-and low-expression groups of DDX58. In order to determine the importance of survival differences, a log rank test was used, with a threshold of p = 0.05. What’s more, we used the limma package to learn the relationship between DDX58 and T stage in pan-cancer.
2.3. Construction and enrichment analysis of gene-gene, protein-protein and gene-disease networks
We constructed gene–gene interaction network through GeneMANIA2 and built PPI network through STRING database.3 We had further constructed a gene disease network on the OPENTARGET platform. Gene ontology (GO) terminology, Kyoto Encyclopedia of Genes and Genomes (KEGG) and GSEA were used to gene enrichment analysis. The term “GO” refers to molecular function (MF), cellular components (CC), and biological processes (BP). Use the “ClusterProfiler” package to perform GO, KEGG analysis, and GSEA. The TIMER4 was a comprehensive online database, analysis of a wide variety of cancer types related to immune infifiltrating. In this study, we used TIMER to determine the relationship between DDX58 expression and ACE2.
2.4. Epigenetic methylation analysis and association analysis of methyltransferase
As a form of DNA chemical modification, DNA methylation controls gene expression by changing epigenetics without changing DNA sequence. To analyze the methylation level of tumor and normal tissues, we obtained them from the methylation module of UALCAN database. Later, from UCSC5 database, we have downloaded a standardized pan-cancer dataset: TCGA Pan Cancer (PANCAN, N = 10,535, G = 60,499), from which we further extracted the expression data of DDX58 gene and 44 marker genes of three kinds of RNA modified m6A genes in each sample. We filtered the samples from: Primary Blood Derived Cancer-Peripheral Blood, Primary Tumor. Further, log2 (x + 1) transformation has been performed for each expression value. Next, we had calculated the spearman correlation between DDX58 and marker genes of five different immune pathways.
2.5. Analysis of tumor mutation load and genome changes in pan-cancer
The total number of substitutions, insertions and deletions per megabase in the coding region of tumor gene exons was used to calculate the tumor mutation load (TMB). We got the expression data of DDX58 gene in every sample from the previously downloaded datasets, combined with the previously screened samples. In addition, we also had download the Simple Nucleotide Variation dataset of level4 of all TCGA samples processed through MuTect2 software from GDC (https://portal.gdc.cancer.gov/; Beroukhim et al., 2010). To calculate the tumor mutation burden (TMB), we used the TMB function of the R software package maftools (version 2.8.01). Then we integrated the TMB and gene expression data of the samples. Finally, we obtained the expression data of 37 cancer species after removing those with fewer than three samples in a single cancer species. Through cBioPortal resources,6 we had analyzed the genetic changes of DDX58 in the TCGA dataset (Reimer et al., 2021). The gene changes and mutation sites of DDX58 were obtained in the “Oncoprint,” “Cancer Type Summary,” and “Mutations” sub modules.
2.6. Analysis of immune checkpoint genes and new immune antigens
Biological phenomena such as gene fusion, deletion mutation and point mutation are called new antigens encoded by mutated genes in tumor cells. We had calculated the binding affinity score of epitopes with 8–11 amino acids of a certain length and the epitopes with a score less than 500 nm were defined as new antigens. Then, we ranked the predicted new antigens according to antigenicity index value, affinity and mutation allele frequency. In each tumor sample, scannedo was used to count the new antigens and analyze the relationship between DDX58 expression and new antigens. The immune checkpoint genes had been extracted and analyzed along with the DDX58 expression to further investigate their relationship.
2.7. DDX58 expression and microsatellite instability analysis
From UCSC (see Footnote 5) database we had downloaded a standardized pan-cancer dataset: TCGA Pan Cancer (PANCAN, N = 10,535, G = 60,499). Based on the previously extracted expression data and screened samples, we obtained MSI (Microsatellite instance) scores of each tumor from the previous study (Gounder et al., 2022). Next, the MSI and gene expression data of the samples were integrated.
2.8. Immune infiltration analysis
We screened the metastatic samples from the following sources: Primary Blood Derived Cancer - Peripheral Blood (TCGA-LAML), Primary Tumor, and TCGA-SKCM. The gene expression profiles of each tumor were extracted, mapped to GeneSymbol, and further analyzed using the Timer method of the R software package IOBR (version 0.99.9, https://www.ncbi.nlm.nih.gov/pmc/articles/PMC8283787/; Li et al., 2017). The B cell, T cell CD4, T cell CD8, Neutrophil, Macrophage and DC infiltration scores of each patient in each tumor were reevaluated according to gene expression.
2.9. Drug sensitivity of DDX58 in pan-cancer
To study the drug sensitivity of pan-cancer patients to DDX58, the CallMinerTM database was used7 to get activity data and RNA seq expression profile of NCI-60 compounds. In order to analyze and select drugs approved by FDA or clinical trials, R packages “impute,” “limma,” “ggplot2,” and “ggpubr” were used for analysis.
3. Results
3.1. Differential DDX58 expression analysis in pan-cancer tissues and normal tissues
The analysis of gene disease network interaction showed that DDX58 was mainly related to genetic, familial or genetic disease, immune system disease, infectious disease, benign tumor, etc. In particular, DDX58 had a certain relationship with benign tumor (Figure 1A). Subsequently, we investigated the role of human DDX58 expression in pan-cancer. A comparison of the expression levels of DDX58 in tumors and normal tissues was performed using the TCGA database. As compared to normal tissues, DDX58 was found to be highly expressed in BRCA, ESCA, STES, KIPAN, STAD, HNSC, KIRC, LIHC, CHOL, while it was low expressed in LUAD, COAD, READ, KIRP, LUSC, KICH (Figures 1B,C). At the same time, DDX58 protein levels in four different organs and tissues also showed significant differences (Figure 1D).
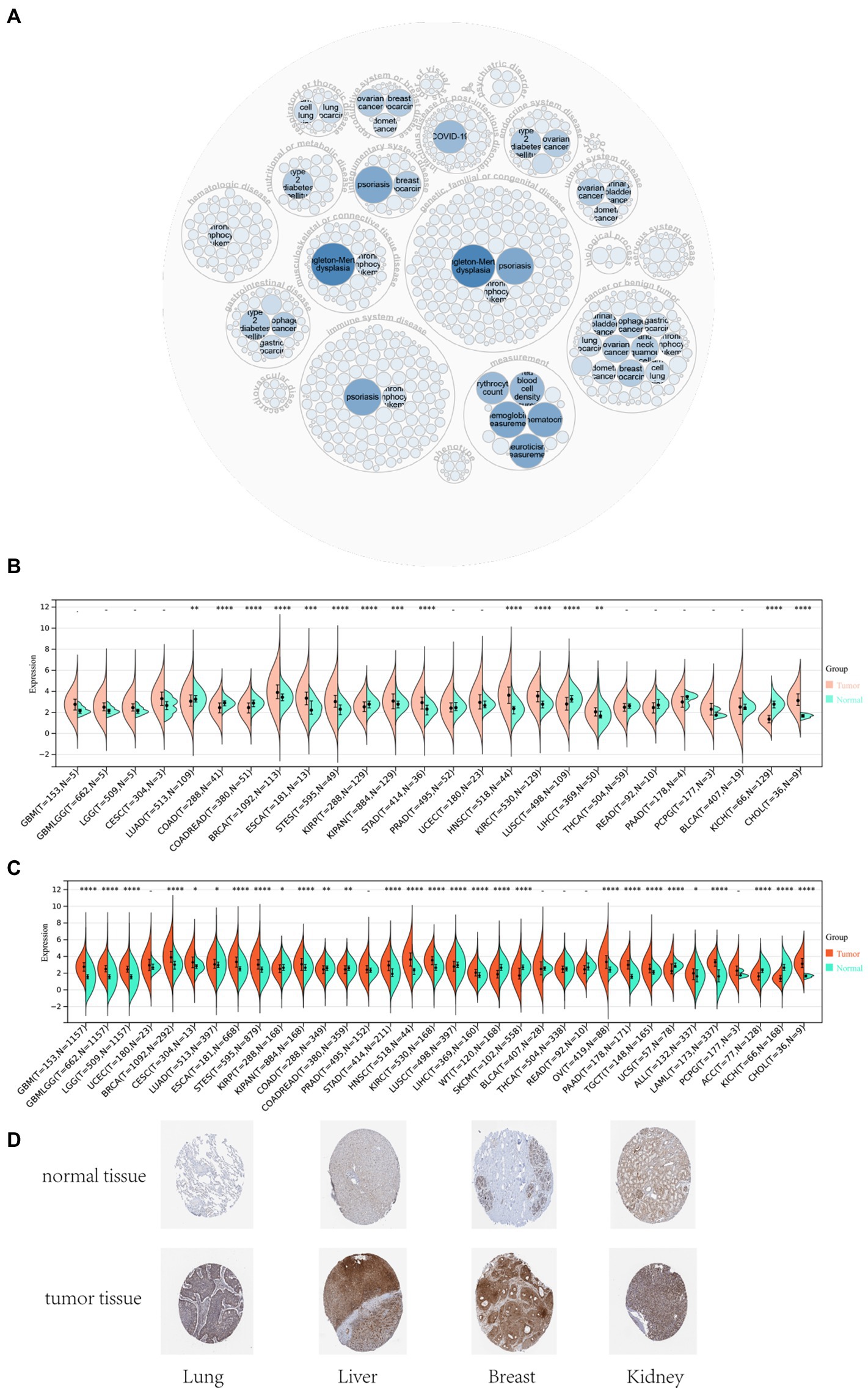
Figure 1. Differential expression analysis of DDX58 in pan-cancer tissues and normal tissues. (A) DDX58 related disease prediction (B) cancer and normal tissues in TCGA database (C) cancer and normal tissues in GTEx database (D) the protein expression level of DDX58 in normal and tumor tissues of four different organs *p < 0.05, **p < 0.01, ***p < 0.001. BLCA, Bladder Urothelial Carcinoma; BRCA, Bladder Urothelial Carcinoma; CHOL, Cholangiocarcinoma, COAD, Colon adenocarcinoma; ESCA, Esophageal carcinoma, GBM, Glioblastoma multiforme; HNSC, Head and Neck squamous cell carcinoma; KICH, Kidney Chromophobe; KIRC, Kidney renal clear cell carcinoma; KIRP, Kidney renal papillary cell carcinoma; LIHC, Liver hepatocellular carcinoma; LUAD, Lung adenocarcinoma; LUSC, Lung squamous cell carcinoma; PRAD, Prostate adenocarcinoma; READ, Rectum adenocarcinoma; STAD, Stomach adenocarcinoma; THCA, Thyroid carcinoma; UCEC, Uterine Corpus Endometrial Carcinoma.
3.2. Pan-cancer analysis of prognostic value of DDX58 expression in different stages of cancers
Next, an analysis of DDX58 expression and cancer prognosis was conducted using univariate Cox regression. According to the forest map of pan-cancer, the expression of DDX58 had a significant impact on the OS of LGG, KIRC, SKCM, MESO, TGCT, PAAD, LUAD patients (Figure 2). In addition, we also analyzed the expression of DDX58 in different cancer T stages, and the results showed that there were significant differences in the expression of DDX58 in different stages of 10 cancers (Supplementary Figure S1).
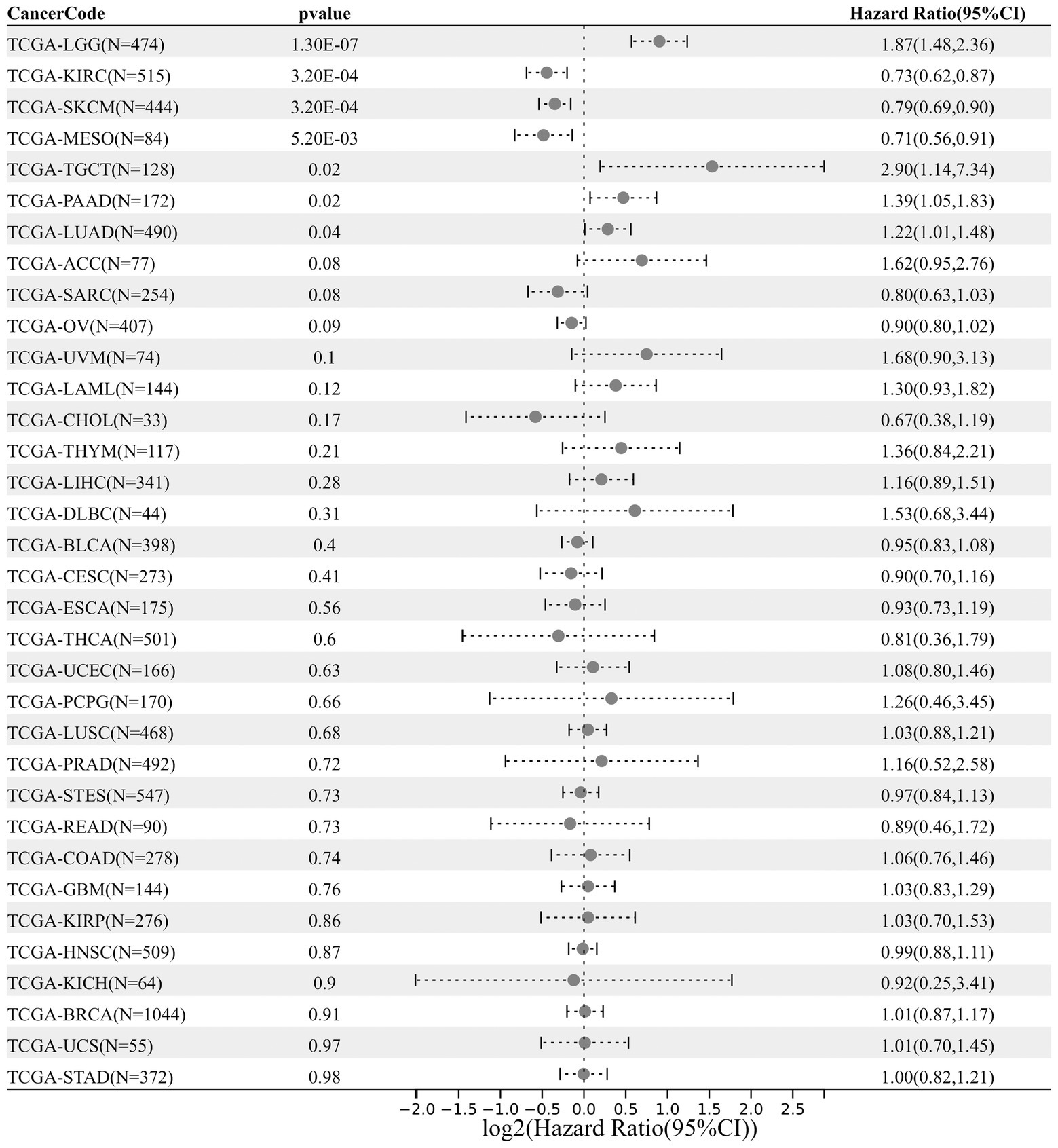
Figure 2. Pancancerous analysis of the diagnostic and prognostic value of DDX58 expression. The forest map shows the HR and 95% CI of DDX58 expression related to cancer OS.
3.3. Construction of DDX58 gene, protein, disease network correlation with SARS CoV-2 receptor–ACE2
In order to understand the DDX58 related network, the STRING and GeneMANIA protein-protein and gene-gene interaction networks that interact with DDX58 were used (20 potential related genes were selected respectively; Figures 3B,C). We obtained 8 genes from the intersection of two data sets (Figure 3A) and carried out GO and KEGG analysis on 9 genes including DDX58 (Figure 3D). We found that BP was enriched in negative regulation of type I interchange production, regulation of type I interchange production, type I interchange production. CC was mainly enriched in phagophore assembly site membrane, phagophore assembly site, phagocytic vascular membrane. MF was significantly enriched in protein tag, Lys63 specific dehydrogenase activity, and Lys48 specific dehydrogenase activity. KEGG analysis showed that many related pathways were significantly enriched, including RIG-I-like receiver signaling pathway, NF kappa B signaling pathway, Influenza A. In addition, it could be seen from the correlation analysis with AEC2 (SARS CoV-2 receptor) that there was a positive correlation between the expression of DDX58 and ACE2 in many cancers (Supplementary Figure S2).
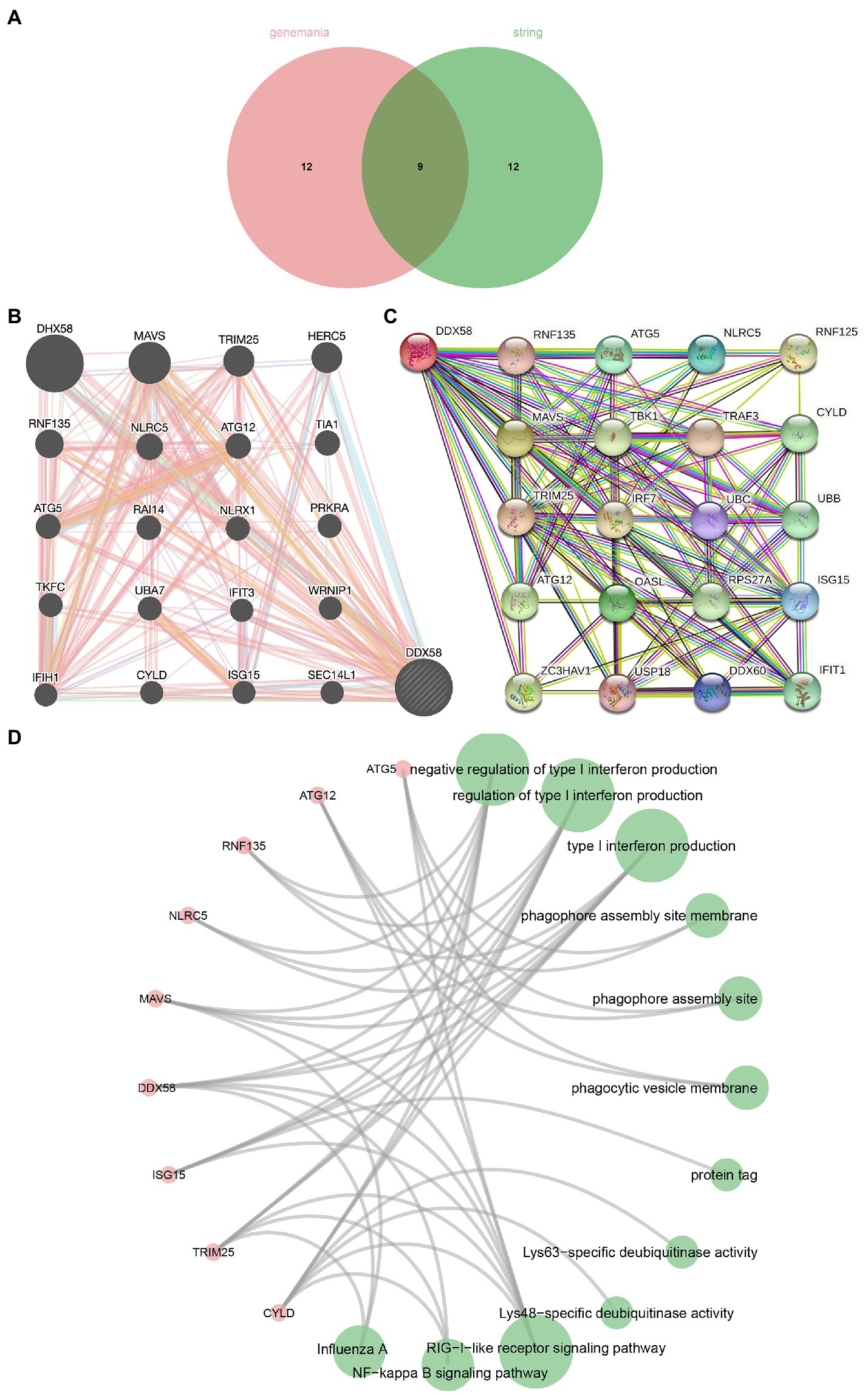
Figure 3. Gene, protein and disease networks use the DDX58 related gene network mapped by GeneMANIA. (A) The Venn diagram where STRING and GeneMANIA intersect. (B) DDX58 related gene network mapped by GeneMANIA (C) DDX58 related protein network mapped by STRING. (D) Enrichment analysis of cross genes.
3.4. Epigenetic modification of DDX58
According to promoter methylation analysis, DDX58 is hypermethylated in a variety of cancer types (Figure 4A). DDX58 methylation seems to be correlated with the level of DNA methyltransferase mRNA expression in various cancers (all p < 0.05; Figure 4B). As we all know, DNA methylation is the result of DNA methyltransferase, which plays a role by covalently binding to the methyl at the 5′ carbon position of cytosine, a CpG dinucleotide in the genome. A correlation was found between methyl related genes and various cancers. There was a positive correlation between the expression of DDX58 in pan-cancer and methyl-related genes, which meant that DDX58 may mediate tumor genesis and progression by regulating epigenetic status. Moreover, it was worth noting that the correlation coefficient was higher in DLBC and UVM.
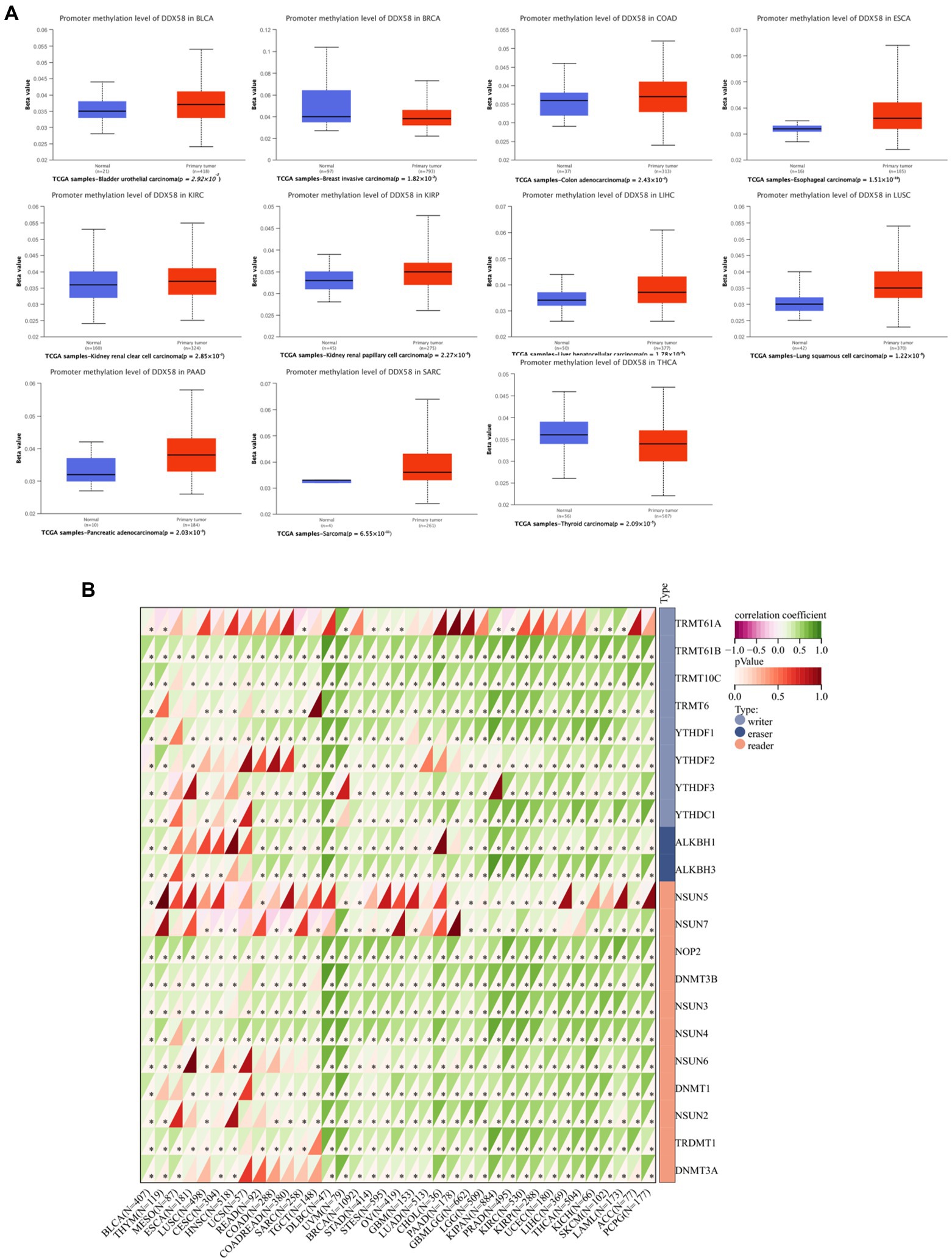
Figure 4. Correlation analysis between DDX58 methylation level and methyltransferase expression level in pan-cancer tissues. (A) Display the difference of DDX58 methylation level between tumor and adjacent normal tissues in TCGA database (β Value). (B) Correlation between DDX58 expression and methylation related gene expression.
3.5. Genetic variation analysis of DDX58 in pan carcinoma
Based on the cBioPortal database, we found that there were higher DDX58 gene changes in LUSC, UCEC, STAD, and SKCM, and mutation was the main type (Figure 5A). It further proved the type, location and quantity of DDX58 gene modification. R244K/I changes were detected in 4 patients with DDX58 (Figure 5B). Then the 3D structure of DDX58 protein at this mutation site was mapped (Figure 5C). The most common type of mutation found in pan-cancer analysis were gain and diploid (Figure 5D). In addition, TRAJ6, TMEM158, YY1P2, TTN, TAF1L, TP53, TOPORS, MUC16, ACO1, RYR2 gene changes were more common in the altered group than in the unchanged group (Figure 5E).
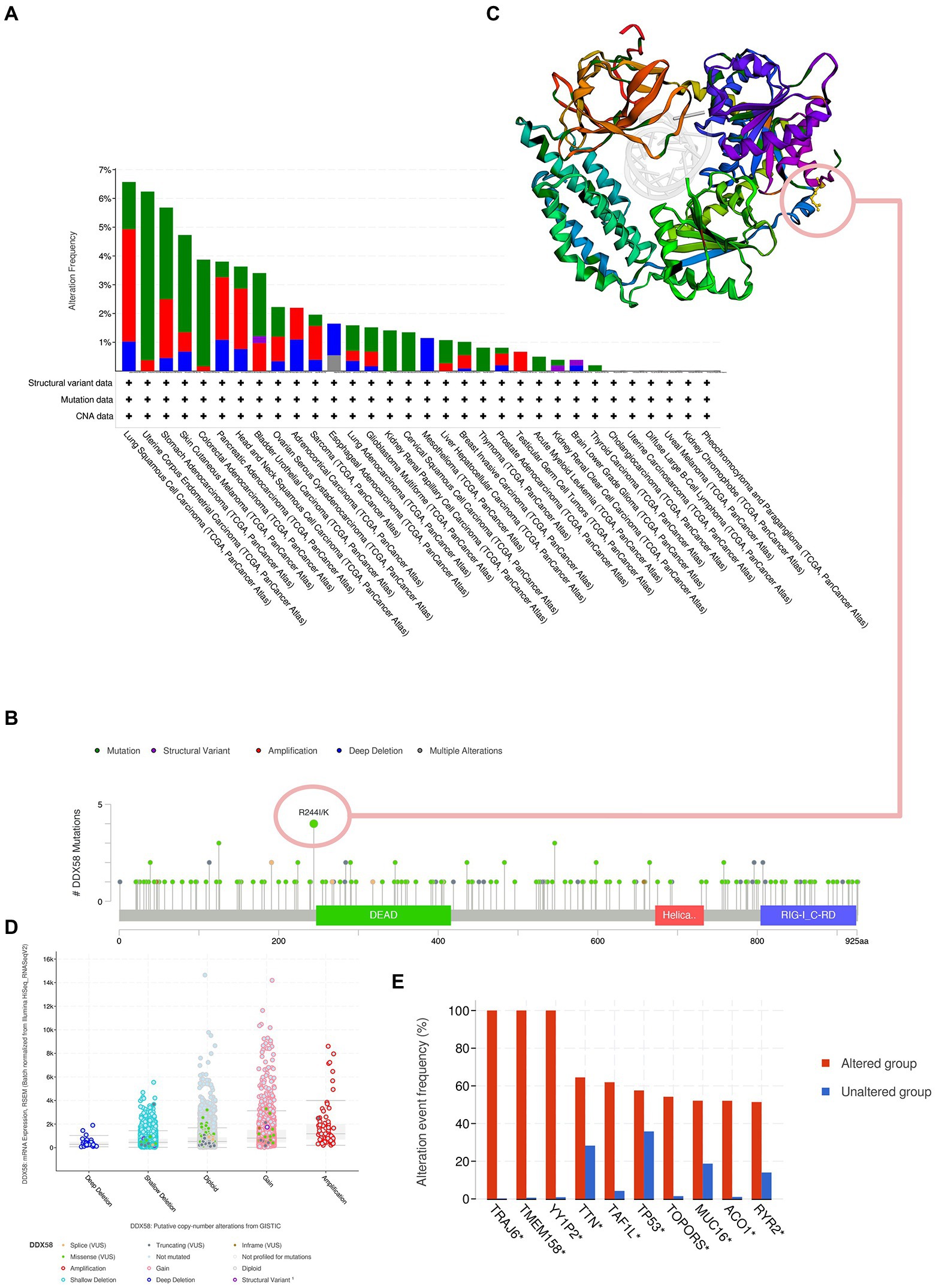
Figure 5. Genetic changes of DDX58. (A) Summary of DDX58 changes in TCGA pan-cancer dataset. (B) The type, number and location of mutations in DDX58 gene changes. (C) The 3D structure of DDX58 at 232 mutation site. (D) The type of DDX58 change in pan carcinoma. (E) Change frequency of related genes in DDX58 changed and unchanged groups.
3.6. DDX58 is associated with TMB and MSI in some cancers
TMB and MSI are effective prognostic biomarkers and indicators of immunotherapeutic response in many tumors. From these two analyses, we can conclude the relationship between DDX58 and immunotherapy prognosis of specific cancer types.
The tumor cell genome’s TMB is usually expressed as the total number of non-synonymous mutations within an average 1 M base region. In some cases, it is also expressed directly as the number of somatic mutations. Base substitution, frameshift mutation, deletion mutation, insertion mutation and other mutation types are the most common mutation type. In tumor cells, TMB is a quantifiable indicator of mutation frequency. The correlation between DDX58 and TMB was calculated for each tumor. Ten tumors showed a significant correlation, including a significant positive correlation in 6 tumors, such as GBMLGG (N = 650; R = 0.1430, p = 0.0002), COAD (N = 282; R = 0.1328, p = 0.0257), COADREAD (N = 372; R = 0.1103, p = 0.0334), KIPAN (N = 679; R = 0.194, p = 3.4926e-7), UCS (N = 57; R = 0.3099, p = 0.01895), BLCA (N = 407; R = 0.0996, p = 0.0444), significantly negative correlation in 4 tumors, for example: BRCA (N = 981; R = −0.0669, p = 0.0361), HNSC (N = 498; R = −0.1226, p = 0.0061), THCA (N = 489; R = −0.2149, p = 0.00001), UVM (N = 79; R = −0.3177, p = 0.0043; Figure 6A).
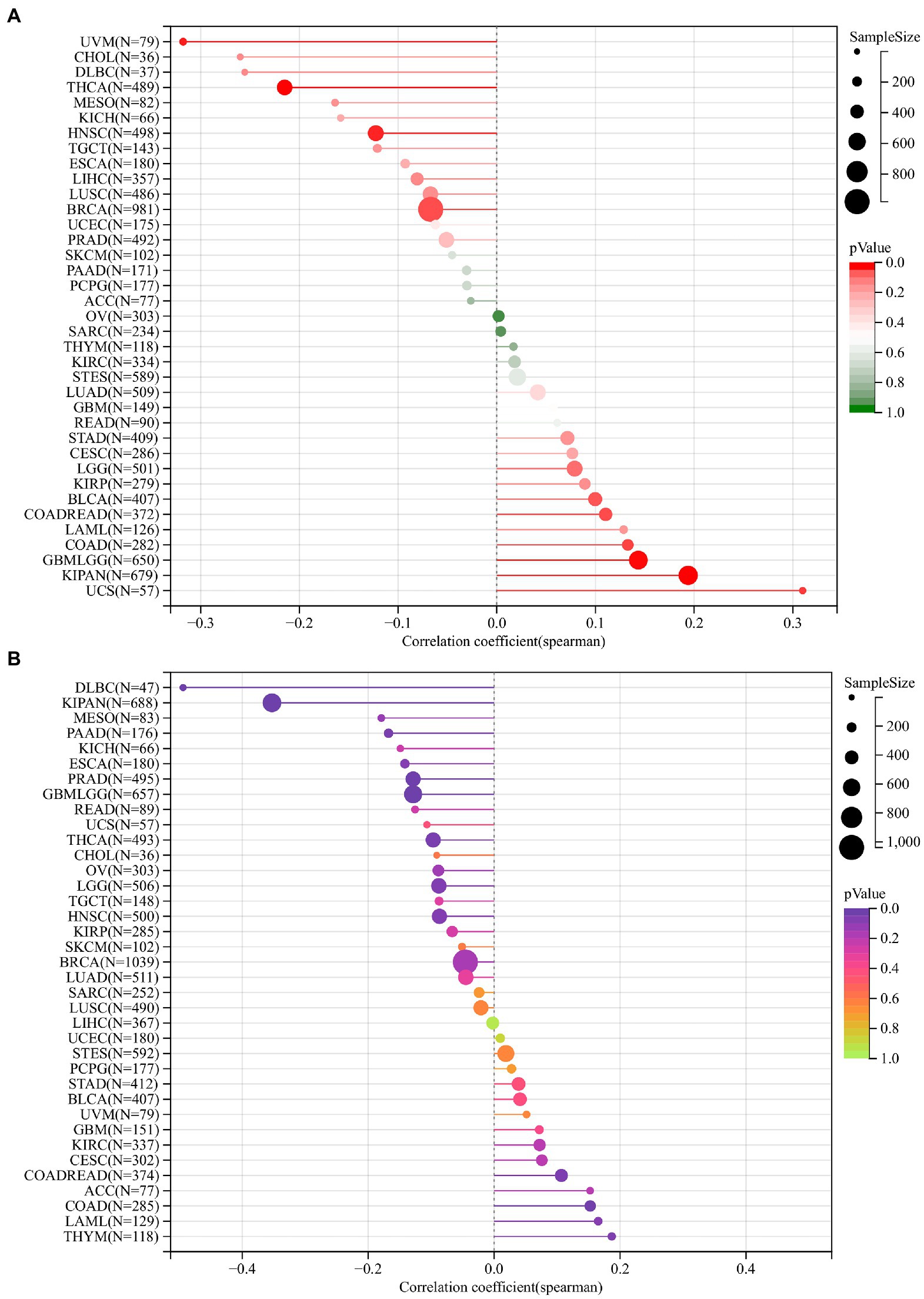
Figure 6. The relationship between the mRNA expression levels of TMB, MSI and DDX58 in various cancers found in TCGA database. TMB was calculated based on the total incidence of mutations per megabase pair in each tumor, and MSI was calculated based on the total incidence of deletions or insertions in repeated sequences per megabase pair. (A) Correlation between TMB and DDX58 expression. (B) Correlation between MSI and DDX58 expression. Spearman correlation test, p < 0.05 is significant.
DDX58 expression correlated with MSI in different types of cancer and we had calculated their spearman correlation in each tumor. A significant correlation was observed in 10 tumors, including significant positive correlation in 3 tumors, such as COAD (N = 285; R = 0.1526, p = 0.0098), COADREAD (N = 374; R = 0.1068, p = 0.0389), THYM (N = 118; R = 0.1868, p = 0.0428), and the significant negative correlation in 7 tumors, such as GBMLGG (N = 657; R = −0.1286, p = 0.0009) LGG (N = 506; R = −0.0878, p = 0.0483), KIPAN (N = 688; R = −0.3528,p = 1.351e-21), PRAD (N = 495; R = −0.1286, p = 0.0041), THCA (N = 493; R = −0.0967, p = 0.0317), PAAD (N = 176; R = −0.1677, p = 0.0260), DLBC (N = 47; R = −0.4937, p = 0.0004; Figure 6B).
It was worth noting that the absolute coefficients associated with TMB or MSI in the COAD cohort were relatively high compared with other cancer types, suggesting that the it may be sensitive to immunotherapy.
3.7. DDX58 might regulate tumor immune microenvironment by influencing immune invasion of various cancer types and expression of immune checkpoints
To determine whether this pathway affects the tumor immune microenvironment, we studied the expression of DDX58 with the degree of immune cell infiltration in each cancer type. Using the data collected from TCGA and the six types of immune cells available in TIMER database (B cells, CD4 + T cells, CD8 + T cells, neutrophils, macrophages and dendritic cells) for analysis, the results indicated that there was significant correlation in multiple tumors (Figure 7). It was worth noting that CD8 + T cells had the highest DLBC correlation coefficient. Their corresponding linear regression diagram showed that the high expression of DDX58 may be related to the increased level of immune cell infiltration. Similarly, DDX58 also affected the expression of immune checkpoints in different cancers (Supplementary Figure S3).
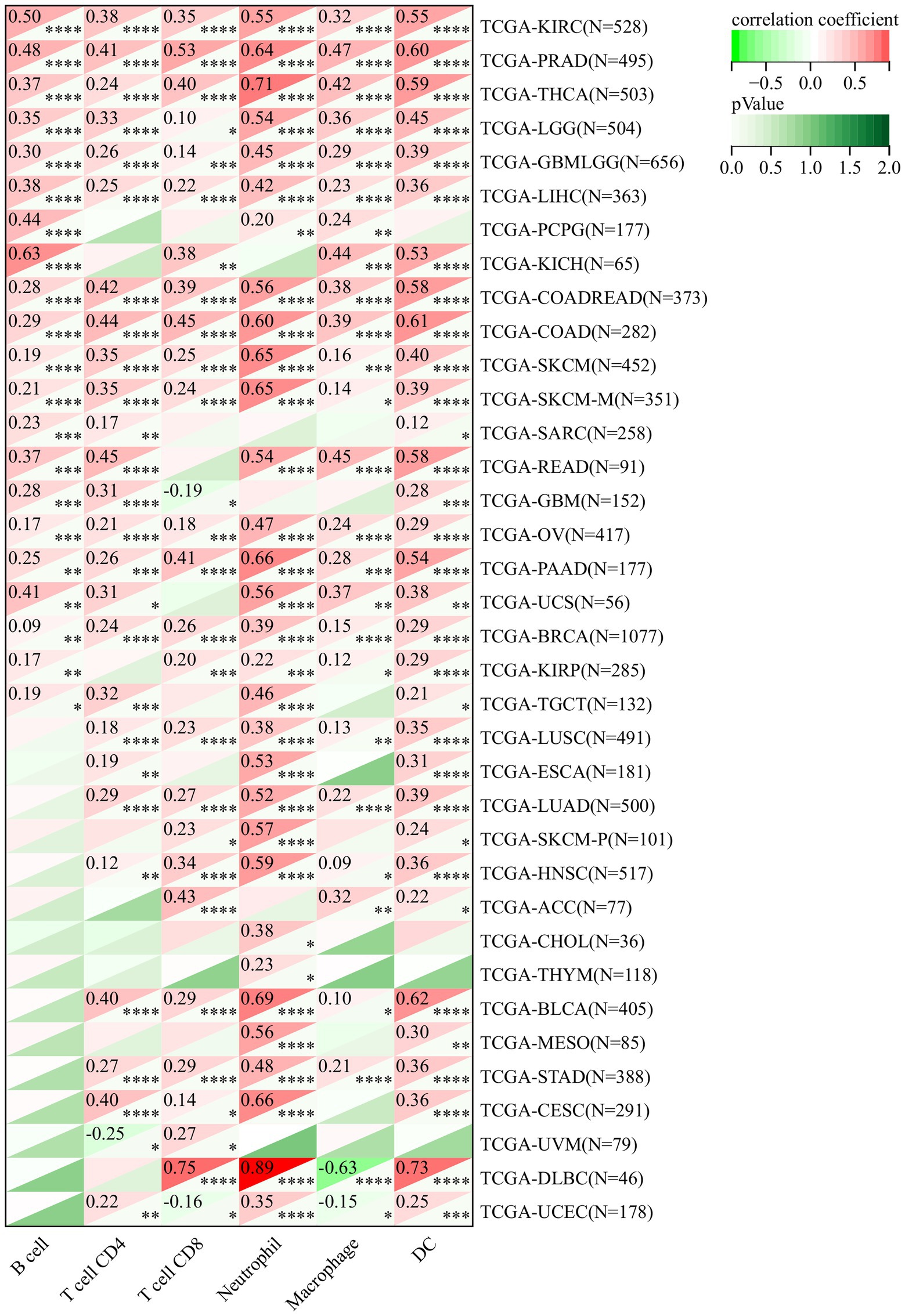
Figure 7. The expression level of DDX58 mRNA calculated by TCGA and TIMER in the database was significantly correlated with the infiltration score of six common immune cells (B cells, CD4 + T cells, CD8 + T cells, neutrophils, macrophages, dendritic cells). Spearman correlation test, p < 0.05 is significant.
3.8. DDX58 drug sensitivity analysis
The database was further analyzed to determine whether DDX58 expression was correlated with drugs using CellMiner™ (Figure 8). Our results indicated that the expression of DDX58 was positively correlated with the sensitivity to Cediranib, VE−821, Itraconazole, JNJ−42756493, IWR−1, Linsitinib. And the expression of DDX58 was negatively correlated with the drug sensitivity of geldanamycin analysis, Tanespimecin, TYROTHRICIN, Panobinostat, Alvespimycin, Quisinostat, XR−5944, Lapiphone, Paclitaxel,Tamoxifen.
4. Discussion
As of December 2019, COVID-19 had caused a worldwide pandemic and posed a serious threat to global public health (Talic et al., 2021). As a result of the COVID-19 pandemic, cancer patients were more likely to be infected with SARS CoV-2. According to these findings, COVID-19 might have an impact on cancer patients’ survival. RNA sensor RIG-I (DDX58) was a protein coding gene. The diseases related to RIG-I included Singleton Merten syndrome2 and Singleton Merten syndrome2. Signaling pathways that leaded to the production of type I interferon and proinflammatory cytokines in response to cytoplasmic viral nucleic acids (Bamming and Horvath, 2009; Shi et al., 2017; Zhao et al., 2017; Cadena et al., 2019). It formed ribonucleoprotein complex with viral RNA, on which homologous polymerization forms silk (Yoneyama et al., 2004; Sumpter et al., 2005). 3pRNA (RIG-1 agonist) treatment could increase cell death in melanoma cell lines and keep most melanoma cells in a non-proliferative state (Thier et al., 2022). In addition, RIG-1 activation inhibited STAT3/CSE pathway activity to restrain the proliferation of colon cancer cells (Deng et al., 2022).
Thus, to clarify how DDX58 contributes to the pathogenicity of COVID-19, we must examine its relation to DDX58, this study systematically analyzed the expression profile of DDX58 in the entire cancer type spectrum. Using TCGA pan-cancer database and related data resources, we analyzed the expression, survival analysis, methylation expression, mutation status, microsatellite instability (MSI), immune related microenvironment, gene related network, function and drug sensitivity of DDX58. Analysis of the relationship between DDX58 expression and cancer immune invasion, tumor mutation, microenvironment and drug sensitivity had been finished, in order to determine DDX58’s potential for cancer immunotherapy and anti-COVID-19 treatment. We also carried out the correlation analysis between DDX58 and AEC2 (SARS CoV-2 receptor; Supplementary Figure S2) to better understand the role of DDX58 in COVID-19 and cancers.
In this study, we found the changes of DDX58 mRNA in tumors. According to our research, pan-cancer was closely associated with the expression of DDX58 protein. DDX58 was highly expressed in BRCA, ESCA, STES, KIPAN, STAD, HNSC, KIRC, LIHC, CHOL, while it was low expressed in LUAD, COAD, READ, KIRP, LUSC, and KICH. DDX58 was significantly associated with poor prognosis of LGG, TGCT, PAAD, LUAD, but significantly associated with improved prognosis of KIRC, SKCM, MESO patients. It indicated that DDX58 might play different roles and functions in different cancers.
Eight genes were obtained by crossing the potential genes that interact with DDX58 in the two databases, and nine genes including DDX58 were analyzed by GO and KEGG. These 8 genes were ATG5, ATG12, RNF135, NLRC5, MAVS, ISG15, TRIM25, and CYLD, respectively. ATG5 usually combined with ATG12, catalyzed ATG7 and ATG10, played a role in autophagy, and regulates various functions of the body (Cui et al., 2022). It was known that RNF135 regulated the expression of IFN, and it participated in the RIG-I signal pathway by targeting RIG-I (Lai et al., 2019). NLRC5 could combine with LC3 to mediate MHC class I antigen presentation pathway (Zhan et al., 2022). MAVS mediated antiviral innate immunity (Zhang et al., 2022). The protein encoded by ISG15 gene was a ubiquitin like protein, when it was activated by interferon-αand-β, it binded to target proteins in cells. The encoded protein had a variety of functions, including chemotactic activity to neutrophils, orientation of junction target protein to intermediate filament, intercellular signal transduction and antiviral activity during viral infection (Jurczyszak et al., 2022). In responsd to ubiquitin E3 ligase and ISG15 E3 ligase (Zou and Zhang, 2006), TRIM25 played a role in the innate immune response to viruses by ubiquitinating DDX58 and IFIH1 (Chiang et al., 2021). CYLD was a ubiquitin free enzyme that participates in NFκB activation and TNF-α induced necrosis (Dobson-Stone et al., 2020). Through enrichment analysis, it was found that these genes were associated with interferon related pathways, phagosomes, ubiquitination, RIG-I, NFκB related pathway, suggesting that it may affect the development of cancer through regulating immunity (Overman et al., 2017; Yang et al., 2021).
Disease network analysis found that DDX58 was related to genetic, family or genetic disease, immune system disease, infectious disease, cancer or disease. This also showed that this gene was closely related to tumor and infectious diseases. Afterwards, we examined the relationship between DDX58 expression and immune cell infiltration, and found that DDX58 was significantly correlated with six types of immune cells (B cells, CD4 + T cells, CD8 + T cells, neutrophils, macrophages, and dendritic cells). In addition, abnormal DNA methylation was highly related to the occurrence, growth and carcinogenesis of tumors (Yang et al., 2021). Our study found that compared with their normal counterparts, cancer tissues were significantly hypermethylated, indicating that DDX58 might promote tumor development by altering DNA methylation. However, the exact mechanism was still unclear. TMB and MSI are effective biomarkers to predict the prognosis of various tumors and indicators of immune response. TMB and MSI had been shown to be indicators of drug response in previous studies, particularly those that target immune checkpoint inhibitors such as CTLA4 and PD-1/PD-L1 (Overman et al., 2017; Mariathasan et al., 2018; Shim et al., 2020). Subsequently, we used the CellMinerTM database to find that the expression of DDX58 was related to the sensitivity to many drugs, including Cediranib, VE−821, Itraconazole, JNJ−42,756,493, IWR−1, Linsitinib. These results are helpful to promote clinical drug guidance.
However, there were still some deficiencies in our research. First, based on bioinformatics analysis, there was a lack of relevant experimental or clinical data. In addition, although there was a correlation between the expression of DDX58 in some tumors and survival rates, and DDX58 changed the infiltration of immune cells, we were unable to establish a direct causal relationship. Future biological research needs to further clarify and confirm the role of DDX58 in cancer.
In conclusion, the expression level of DDX58 was significantly different in pan carcinoma.Turning RIG-I Sensor Activation Against Cancer had been used in clinical trails (Iurescia et al., 2020).And it had been proved that SARS CoV-2 M protein could inhibit the expression of IFNb and interferon stimulated genes induced by RIG-1(Sui et al., 2021). However, how DDX58 played a role in these two diseases had not been reported. As an immune related biomarker, DDX58 could be used to diagnose and predict the prognosis of COVID-19 cancer patients and their potential therapeutic targets.
5. Conclusion
We found that DDX58 expression, survival prognosis, methylation, MSI, TMB, tumor immune microenvironment and drug sensitivity were different in pan-cancer. It was expected that DDX58 might become a potential target for COVID-19 cancer therapy based on its abnormal expression in pan-cancer and significant differences in prognosis and immune environment. As a result, this study provided new insight into DDX58’s possible role in drug regulation as well as exploring its multiple roles in pan-cancer.
Data availability statement
The original contributions presented in the study are included in the article/Supplementary material, further inquiries can be directed to the corresponding authors.
Author contributions
LS, YZ, and YH conceived and designed the study. ZH, LY, and LJ performed the experiments. LY and JC analyzed the data. ZH and LY wrote the manuscript. All authors contributed to the article and approved the submitted version.
Funding
This work was supported by Joint Funds for the innovation of science, Technology, Fujian province (Grant number: 2020Y9039) and Medical Research Fund of Guangdong (No. 2021112015285821).
Acknowledgments
The authors thank reviewers for helpful comments on the manuscript.
Conflict of interest
The authors declare that the research was conducted in the absence of any commercial or financial relationships that could be construed as a potential conflict of interest.
Publisher’s note
All claims expressed in this article are solely those of the authors and do not necessarily represent those of their affiliated organizations, or those of the publisher, the editors and the reviewers. Any product that may be evaluated in this article, or claim that may be made by its manufacturer, is not guaranteed or endorsed by the publisher.
Supplementary material
The Supplementary material for this article can be found online at: https://www.frontiersin.org/articles/10.3389/fmicb.2022.1085086/full#supplementary-material
Footnotes
References
Al-Quteimat, O. M., and Amer, A. M. (2020). The impact of the COVID-19 pandemic on cancer patients. Am. J. Clin. Oncol. 43, 452–455. doi: 10.1097/COC.0000000000000712
Bamming, D., and Horvath, C. M. (2009). Regulation of signal transduction by enzymatically inactive antiviral RNA helicase proteins MDA5, RIG-I, and LGP2. J. Biol. Chem. 284, 9700–9712. doi: 10.1074/jbc.M807365200
Beroukhim, R., Mermel, C. H., Porter, D., Wei, G., Raychaudhuri, S., Donovan, J., et al. (2010). The landscape of somatic copy-number alteration across human cancers. Nature 463, 899–905. doi: 10.1038/nature08822
Cadena, C., Ahmad, S., Xavier, A., Willemsen, J., Park, S., Park, J. W., et al. (2019). Ubiquitin-dependent and-independent roles of E3 ligase RIPLET in innate immunity. Cells 177, 1187–1200.e16. doi: 10.1016/j.cell.2019.03.017
Chiang, C., Dvorkin, S., Chiang, J. J., Potter, R. B., and Gack, M. U. (2021). The small t antigen of JC virus antagonizes RIG-I-mediated innate immunity by inhibiting TRIM25's RNA binding ability. MBio 12, e00620–e00621. doi: 10.1128/mBio.00620-21
Cui, J., Ogasawara, Y., Kurata, I., Matoba, K., Fujioka, Y., Noda, N. N., et al. (2022). Targeting the ATG5-ATG16L1 protein-protein interaction with a hydrocarbon-stapled peptide derived from ATG16L1 for autophagy inhibition. J. Am. Chem. Soc. 144, 17671–17679. doi: 10.1021/jacs.2c07648
Deng, Y., Fu, H., Han, X., Li, Y., Zhao, W., Zhao, X., et al. (2022). Activation of DDX58/RIGI suppresses the growth of tumor cells by inhibiting STAT3/CSE signaling in colon cancer. Int. J. Oncol. 61, 1–13. doi: 10.3892/ijo.2022.5410
Dobson-Stone, C., Hallupp, M., Shahheydari, H., Ragagnin, A. M. G., Chatterton, Z., Carew-Jones, F., et al. (2020). CYLD is a causative gene for frontotemporal dementia - amyotrophic lateral sclerosis. Brain 143, 783–799. doi: 10.1093/brain/awaa039
Gounder, M. M., Agaram, N. P., Trabucco, S. E., Robinson, V., Ferraro, R. A., Millis, S. Z., et al. (2022). Clinical genomic profiling in the management of patients with soft tissue and bone sarcoma. Nat. Commun. 13:3406. doi: 10.1038/s41467-022-30496-0
Han, H. J., Nwagwu, C., Anyim, O., Ekweremadu, C., and Kim, S. (2021). COVID-19 and cancer: from basic mechanisms to vaccine development using nanotechnology. Int. Immunopharmacol. 90:107247. doi: 10.1016/j.intimp.2020.107247
Iurescia, S., Fioretti, D., and Rinaldi, M. (2020). The innate immune Signalling pathways: turning RIG-I sensor activation against cancer. Cancers 12:3158. doi: 10.3390/cancers12113158
Jurczyszak, D., Manganaro, L., Buta, S., Gruber, C., Martin-Fernandez, M., Taft, J., et al. (2022). ISG15 deficiency restricts HIV-1 infection. PLoS Pathog. 18:e1010405. doi: 10.1371/journal.ppat.1010405
Lai, Y., Liang, M., Hu, L., Zeng, Z., Lin, H., Yi, G., et al. (2019). RNF135 is a positive regulator of IFN expression and involved in RIG-I signaling pathway by targeting RIG-I. Fish Shellfish Immunol. 86, 474–479. doi: 10.1016/j.fsi.2018.11.070
Li, T., Fan, J., Wang, B., Traugh, N., Chen, Q., Liu, J. S., et al. (2017). TIMER: a web server for comprehensive analysis of tumor-infiltrating immune cells. Cancer Res. 77, e108–e110. doi: 10.1158/0008-5472.CAN-17-0307
Mariathasan, S., Turley, S. J., Nickles, D., Castiglioni, A., Yuen, K., Wang, Y., et al. (2018). TGFbeta attenuates tumour response to PD-L1 blockade by contributing to exclusion of T cells. Nature 554, 544–548. doi: 10.1038/nature25501
Morelli, M., Galluzzo, M., Madonna, S., Scarponi, C., Scaglione, G. L., Galluccio, T., et al. (2021). HLA-Cw6 and other HLA-C alleles, as well as MICB-DT, DDX58, and TYK2 genetic variants associate with optimal response to anti-IL-17A treatment in patients with psoriasis. Expert. Opin. Biol. Ther. 21, 259–270. doi: 10.1080/14712598.2021.1862082
Overman, M. J., McDermott, R., Leach, J. L., Lonardi, S., Lenz, H. J., Morse, M. A., et al. (2017). Nivolumab in patients with metastatic DNA mismatch repair-deficient or microsatellite instability-high colorectal cancer (CheckMate 142): an open-label, multicentre, phase 2 study. Lancet Oncol. 18, 1182–1191. doi: 10.1016/S1470-2045(17)30422-9
Reimer, N., Unberath, P., Busch, H., Börries, M., Metzger, P., Ustjanzew, A., et al. (2021). Challenges and experiences extending the cBioPortal for cancer genomics to a molecular tumor board platform. Stud. Health Technol. Inform. 287, 139–143. doi: 10.3233/SHTI210833
Shi, Y., Yuan, B., Zhu, W., Zhang, R., Li, L., Hao, X., et al. (2017). Ube2D3 and Ube2N are essential for RIG-I-mediated MAVS aggregation in antiviral innate immunity. Nat. Commun. 8:15138. doi: 10.1038/ncomms15138
Shim, J. H., Kim, H. S., Cha, H., Kim, S., Kim, T. M., Anagnostou, V., et al. (2020). HLA-corrected tumor mutation burden and homologous recombination deficiency for the prediction of response to PD-(L)1 blockade in advanced non-small-cell lung cancer patients. Ann. Oncol. 31, 902–911. doi: 10.1016/j.annonc.2020.04.004
Sui, L., Zhao, Y., Wang, W., Wu, P., Wang, Z., Yu, Y., et al. (2021). SARS-CoV-2 membrane protein inhibits type I interferon production through ubiquitin-mediated degradation of TBK1. Front. Immunol. 12:662989. doi: 10.3389/fimmu.2021.662989
Sumpter, R. Jr., Loo, Y. M., Foy, E., Li, K., Yoneyama, M., Fujita, T., et al. (2005). Regulating intracellular antiviral defense and permissiveness to hepatitis C virus RNA replication through a cellular RNA helicase, RIG-I. J. Virol. 79, 2689–2699. doi: 10.1128/JVI.79.5.2689-2699.2005
Talic, S., Shah, S., Wild, H., Gasevic, D., Maharaj, A., Ademi, Z., et al. (2021). Effectiveness of public health measures in reducing the incidence of covid-19, SARS-CoV-2 transmission, and covid-19 mortality: systematic review and meta-analysis. BMJ 375:e068302. doi: 10.1136/bmj-2021-068302
Thier, B., Zhao, F., Stupia, S., Brüggemann, A., Koch, J., Schulze, N., et al. (2022). Innate immune receptor signaling induces transient melanoma dedifferentiation while preserving immunogenicity. J. Immunother. Cancer 10:e003863. doi: 10.1136/jitc-2021-003863
Yamada, T., Sato, S., Sotoyama, Y., Orba, Y., Sawa, H., Yamauchi, H., et al. (2021). RIG-I triggers a signaling-abortive anti-SARS-CoV-2 defense in human lung cells. Nat. Immunol. 22, 820–828. doi: 10.1038/s41590-021-00942-0
Yang, B., Wang, J. Q., Tan, Y., Yuan, R., Chen, Z. S., and Zou, C. (2021). RNA methylation and cancer treatment. Pharmacol. Res. 174:105937. doi: 10.1016/j.phrs.2021.105937
Yoneyama, M., Kikuchi, M., Natsukawa, T., Shinobu, N., Imaizumi, T., Miyagishi, M., et al. (2004). The RNA helicase RIG-I has an essential function in double-stranded RNA-induced innate antiviral responses. Nat. Immunol. 5:730. doi: 10.1038/ni1087
Zhan, L., Zhang, J., Zhang, J., Liu, X., Zhu, S., Shi, Y., et al. (2022). LC3 and NLRC5 interaction inhibits NLRC5-mediated MHC class I antigen presentation pathway in endometrial cancer. Cancer Lett. 529, 37–52. doi: 10.1016/j.canlet.2021.12.031
Zhang, R., Hou, X., Wang, C., Li, J., Zhu, J., Jiang, Y., et al. (2022). The endoplasmic reticulum ATP13A1 is essential for MAVS-mediated antiviral innate immunity. Adv. Sci. 9:e2203831. doi: 10.1002/advs.202203831
Zhao, C., Jia, M., Song, H., Yu, Z., Wang, W., Li, Q., et al. (2017). The E3 ubiquitin ligase TRIM40 attenuates antiviral immune responses by targeting MDA5 and RIG-I. Cell Rep. 21, 1613–1623. doi: 10.1016/j.celrep.2017.10.020
Keywords: DDX58, pan-cancer, biomaker, immune infiltration, SARS-CoV-2
Citation: Huang Z, Yi L, Jin L, Chen J, Han Y, Zhang Y and Shi L (2022) Systematic analysis of virus nucleic acid sensor DDX58 in malignant tumor. Front. Microbiol. 13:1085086. doi: 10.3389/fmicb.2022.1085086
Edited by:
Lihong Peng, Hunan University of Technology, ChinaReviewed by:
Zheng Yan, Henan Provincial Cancer Hospital, ChinaTao Tan, Kunming University of Science and Technology, China
Copyright © 2022 Huang, Yi, Jin, Chen, Han, Zhang and Shi. This is an open-access article distributed under the terms of the Creative Commons Attribution License (CC BY). The use, distribution or reproduction in other forums is permitted, provided the original author(s) and the copyright owner(s) are credited and that the original publication in this journal is cited, in accordance with accepted academic practice. No use, distribution or reproduction is permitted which does not comply with these terms.
*Correspondence: Yuanyuan Han, aHl5QGltYmNhbXMuY29tLmNu; Yan Zhang, MjgxNzYyMUBxcS5jb20=; Libin Shi, MTk5MjI0Njc3NUBxcS5jb20=
†These authors have contributed equally to this work