- 1Cell and Molecular Biology Department, Ribeirão Preto Medical School, University of São Paulo, Ribeirão Preto, SP, Brazil
- 2Faculty of Philosophy, Sciences and Letters of Ribeirão Preto, University of São Paulo, Ribeirão Preto, SP, Brazil
- 3Faculty of Pharmaceutical Sciences of Ribeirão Preto, University of São Paulo, Ribeirão Preto, SP, Brazil
The demand for robust microbial cell factories that produce valuable biomaterials while resisting stresses imposed by current bioprocesses is rapidly growing. Rhodosporidium toruloides is an emerging host that presents desirable features for bioproduction, since it can grow in a wide range of substrates and tolerate a variety of toxic compounds. To explore R. toruloides suitability for application as a cell factory in biorefineries, we sought to understand the transcriptional responses of this yeast when growing under experimental settings that simulated those used in biofuels-related industries. Thus, we performed RNA sequencing of the oleaginous, carotenogenic yeast in different contexts. The first ones were stress-related: two conditions of high temperature (37 and 42°C) and two ethanol concentrations (2 and 4%), while the other used the inexpensive and abundant sugarcane juice as substrate. Differential expression and functional analysis were implemented using transcriptomic data to select differentially expressed genes and enriched pathways from each set-up. A reproducible bioinformatics workflow was developed for mining new regulatory elements. We then predicted, for the first time in this yeast, binding motifs for several transcription factors, including HAC1, ARG80, RPN4, ADR1, and DAL81. Most putative transcription factors uncovered here were involved in stress responses and found in the yeast genome. Our method for motif discovery provides a new realm of possibilities in studying gene regulatory networks, not only for the emerging host R. toruloides, but for other organisms of biotechnological importance.
1. Introduction
Microbial cell factories are in ever-growing demand due to the pursuit of more sustainable products supporting the concepts of green chemistry and biorefineries (Cherubini, 2010; Martins et al., 2021; Tan and Lamers, 2021). The benefits go beyond obtaining valuable products from low-cost raw materials, but include the independence from oil, a finite and extremely polluting material (Borodina and Nielsen, 2014; Liu et al., 2021). Innovations in obtaining products from renewable substrates are based on the genetic improvement and metabolic engineering of microorganisms that can degrade and ferment different biomasses (Martins-Santana et al., 2018). The main challenges the currently available cell factories face are not only breaking complex compounds into simpler chains, but also tolerating adverse conditions resulting from bioprocesses (Geiselman et al., 2020; Devi et al., 2022).
Rhodosporidium toruloides is emerging as a potential, robust host for completing both tasks. This oleaginous, carotenogenic yeast can flourish on a wide range of substrates, such as sugarcane juice and bagasse, crude glycerol, wheat straw, cassava starch, among others (Bonturi et al., 2017; Park et al., 2018; Lopes, 2020). It can also store high amounts of lipids—up to 60% of its total cell weight—and tolerate compounds commonly toxic to other yeast species (Saini et al., 2020). It performs better than the widely used baker’s yeast, Saccharomyces cerevisiae, for example, as it can degrade C5 sugars and lignin-derived compounds, which the latter cannot (Nora et al., 2019). The advantages go over other lipid-producing strains, such as Yarrowia lipolytica, since it can grow in more complex carbon sources and presents higher tolerance to several inhibitors like 5-hydroxy methyl ester, furfural, acetic acid and vanillin (Saini et al., 2020). R. toruloides has already been shown to produce several different bioproducts in a variety of conditions. Examples of these bioproducts include enzymes, carotenoids, sugar alcohols, such as arabitol and galactitol, and lipid-based compounds, such as linoleic acid, oleic acid and cocoa butter substitute (Jagtap and Rao, 2018; Park et al., 2018; Jagtap et al., 2019; Bonturi et al., 2022). Recently, terpenes that can be used as precursors of biodiesel and jet fuel alternatives were produced on a pilot scale, derived from corn stover hydrolysates (Yaegashi et al., 2017; Geiselman et al., 2020; Kirby et al., 2021). Hence, all these distinctive characteristics point to R. toruloides as a great candidate for improving the productivity of biorefineries.
There is great interest in using unconventional, more robust yeasts in detriment to the use of S. cerevisiae for ethanol production in bioreactors (Wehrs et al., 2019; Wu et al., 2020). Ethanol tolerance and thermotolerance, for instance, are desirable features. First, ethanol accumulation during fermentation can become a significant stress factor during the process (Stanley et al., 2010). Secondly, higher fermentation temperatures can lower the cost of cooling bioreactors during the process and reduce bacterial contamination, decreasing the need for antibiotics (Abdel-Banat et al., 2010; Huang et al., 2018). Thus, to explore if R. toruloides could tolerate and thrive in these stressful environments, we decided to investigate the transcriptional response of this yeast growing in high temperatures and in the presence of ethanol.
Furthermore, Soccol et al. (2017) developed a process for producing biofuels using R. toruloides grown in a simple media containing only sugarcane juice and urea. Sugarcane juice is an abundant and low-cost substrate, containing up to 15% of fermentable sugars. Based on their work, we additionally sought to understand the changes in transcripts of R. toruloides grown in media containing sugarcane juice and urea (Soccol et al., 2017).
Transcription factors (TF) are proteins that bind to specific DNA sequences modulating the rate of transcription of DNA to mRNA. These particular DNA sequences are collectively called transcription factor binding sites (TFBS) or binding motifs, and are present in the cis-regulatory region of DNA surrounding the transcription start sites. The activity of TFs can directly or indirectly transform physiological and environmental signals into gene expression patterns. Thus, identifying both the proteins and the position of their binding motifs is essential for understanding gene regulation in the cell and allowing better transcription control, which can serve as a basis for new bioengineering applications (Martins-Santana et al., 2018). However, finding new regulatory sequence motifs is a challenging procedure, especially in eukaryotic organisms, even when we have a large amount of available experimental data (Ambrosini et al., 2020). One of the reasons is that the primary nucleotide sequence is one of many characteristics that specify a target. Additional factors, such as genomic context, DNA binding, modification, and shape, can change nucleotide preferences (Inukai et al., 2017). Besides, it is only possible to test some environmental conditions to which a natural regulatory network can respond. Nevertheless, new tools and methodological approaches are developing to predict regulatory elements in different datasets. In the future, they might help overcome motif discovery limitations in non-model organisms (Ksouri et al., 2021; Novakovsky et al., 2021; Wetzel et al., 2022).
Here, we developed a bottom-up bioinformatic pipeline for cis-regulatory element discovery combining tools for motif prediction and sequence analysis. We applied it to the emergent microbial cell factory R. toruloides, whereas this workflow can be well suitable to other biotechnologically relevant organisms. To do that, we cultivated R. toruloides in the settings described above and extracted RNA samples from early time points of growth. Then, we performed RNA sequencing, functional analysis of the transcriptional responses, and discovery of new motifs from genes of the main pathways found to be enriched in each specific condition. In this sense, we are providing new insights regarding the regulation of R. toruloides genes in a transcriptomic approach and describing, for the first time, putative TFBS that can be used for transcriptional control in this yeast, offering the basis for building new genetic tools for this promising host.
2. Materials and methods
2.1. Strains, media and growth conditions
For RNA sequencing experiments using R. toruloides grown in a medium with sugarcane juice (SCJ), cultures were first grown in LB medium (1% yeast extract, 1% tryptone, and 0.5% NaCl) for 24 h at 30°C and 200 rpm. LB medium was used in the inoculum so that no other sugar influenced the yeast metabolism. The inoculum was centrifuged and transferred to media containing sugarcane juice. Considering the concentration of 120 g of sucrose per liter of juice, we standardized the final concentration of the media as 4% sucrose. In addition, 1% urea was added as a nitrogen source. The filtered sugarcane juice was pasteurized at 65°C for 30 min before being added to the autoclaved medium. SCJ comprises about 75% water, 13–15% of sucrose, and 10–15% of fibers, and pasteurization should not change overall composition. The cultures were grown for 8 h and then collected for RNA extraction. Aliquots of the inoculum in LB grown for 24 h were also collected to serve as internal control, and hereafter will be called time 0 h.
The experiments for the stress conditions were carried out as follows: the inoculum was grown in YPD medium (1% yeast extract, 2% peptone, and 2% glucose) at 200 rpm at 30°C for 24 h. These inoculants were centrifuged and transferred to the respective media, containing YPD and one of the respective stress conditions: 2% ethanol and 4% ethanol, which were grown in a shaker at 30°C, and the high-temperature conditions that were grown at 37°C and 42°C. The control condition was determined as the inoculum grown in YPD for 24 h. After being transferred to the respective media, the cultures were grown for another 16 h and collected for RNA extraction and sequencing. All inoculations were performed in a 1: 10 ratio, and all experiments were performed in triplicates. The strain R. toruloides IFO0880 was used in all experiments (Lin et al., 2014; Coradetti et al., 2018).
2.2. RNA extraction and sequencing
Cell lysis was performed by pelleting yeast cells from the culture by centrifugation and resuspending it with Trizol® (Thermo Fisher Scientific). Samples were transferred to tubes containing zirconium beads and lysed using a cell homogenizer. RNA extraction using Trizol® (Thermo Fisher Scientific) followed the manufacturer’s instructions. The resulting RNA samples were immediately purified using the Qiagen® Rneasy mini kit. Samples were submitted to analysis by Agilent Bioanalyzer RNA 6000 Nano kit for RNA integrity check, aiming for a RIN number higher than 8. After being extracted and purified, all RNA samples were kept in a –80°C freezer. The samples were sent for sequencing at the Genomics Center of the University of São Paulo in Piracicaba, São Paulo, Brazil. The facility prepared the sequencing library using Illumina TruSeq Stranded mRNA Sample Prep LT Protocol. RNA sequencing was performed using the HiSeq SBS v4 kit in Illumina HiSeq 2500, with paired reads of 100 bp (2 × 101). Raw data is available as Sequencing Read Archives (SRA) on the NCBI website under accession number PRJNA883675.
2.3. RNA-seq differential gene expression analysis
Read quality check was performed using FastQC (Andrews, 2018), and the trimming was made using the Trimmomatic software (Bolger et al., 2014). Henceforth, we used only the reads assigned by Trimmomatic as paired. Gene expression was quantified with kallisto (Bray et al., 2016) used for counting the R. toruloides IFO0880 JGI reference transcriptome (Coradetti et al., 2018) available at: https://mycocosm.jgi.doe.gov/Rhoto_IFO0880_4/Rhoto_IFO0880_4.home.html. The differential expression analysis was done using DESeq2 (Love et al., 2014) in the R platform (R Core Team, 2019). Genes whose adjusted value of ps (adjpval) were lower than 0.05 and whose log2-fold change values were lower than −1 or higher than 1 were selected as differentially expressed genes (DEGs).
2.4. Functional and pathway analysis
To analyze the DEGs function, we used the gene function annotation file created by KOG (also provided by JGI, as above). For pathway enrichment analysis, we used the GAGE R package (Luo et al., 2009). As input in this step, we used the DEGs from each condition with their function annotated by the KEGG Automatic Annotation Server,1 as this tool provides a compatible file for GAGE analysis. The enriched pathways were filtered by a value of p lower than 0.05. Bubble maps were plotted using the R package ggplot2 (Wickham, 2016). Path view R package (Luo and Brouwer, 2013) was used to visualize enriched pathways whenever needed.
2.5. Putative cis-regulatory elements discovery
For each condition, we selected the DEGs that belong to the KOG classes. We extracted the promoter sequence of these genes from the reference database. We applied the HOMER software (Heinz et al., 2010) in each promoter sequence set, with the following parameters: –len 10, −stat hypergeo, −olen 3, −strand both, –maxBack 0.3. The background file was the R. toruloides IFO0880 JGI reference promoter sequences. We performed a pairwise comparison between all motifs by applying the universal motif (Tremblay, 2020) function ‘compare_motifs’ using Pearson correlation coefficient (PCC) as the method and average mean as the scoring strategy. We also performed pairwise alignment using TOMTOM from MEME suite (Gupta et al., 2007), providing as input our motif file as both target and source, with the following arguments: –min-overlap 0, −evalue, −thresh 10.0 and –dist Pearson. Finally, we filtered the results by a value of p lower than 0.05, an e-value lower than 1 and a PCC higher than 0.8. All motifs from our experimental settings were found using HOMER and were aligned with the ones from JASPAR2018_CORE_fungi_non-redundant database using TOMTOM. Subsequently, they were plotted and analyzed as a graph, using Gephi (Bastian et al., 2009), applying Circle Pack Layout for representation with the variables ‘predicted or annotated,’ experimental condition and node degree as hierarchy.
2.6. Finding TF candidates
We got the amino acid sequence of the corresponding transcription factor protein for the overrepresented motifs found on the JASPAR alignment. Then we performed a BLAST on the JGI platform to search for similar proteins on the R. toruloides reference assembled genome (provided by JGI, as above). Amino acid sequences and GO functions of each predictive TF were extracted from UniProt.2
2.7. Bioinformatics workflow
All analyses performed on the R environment were organized in an easy-to-use Jupyter Notebook. The customized code and instructions to install and run the workflow are publicly available at: https://github.com/computational-chemical-biology/cis_reg.
3. Results
3.1. A new pipeline for the discovery of regulatory elements from transcriptomic data
Figure 1 summarizes the workflow developed in this work. The experimental design consisted of growing cells and extracting their RNA, as described in the Methods section. Then, the RNA sequencing data was used as input for the motif discovery pipeline. First, differential expression analysis was performed to select the differentially expressed genes (DEGs), and functional analysis was executed to find enriched pathways for each condition. We then extracted the promoter sequences from the genes of the specific enriched pathways and applied the HOMER software for motif prediction. Table 1 contains the number of promoter sequences and motifs found by HOMER for each case. We performed an internal pairwise comparison between all motifs by applying the universal motif package and the TOMTOM tool. Subsequently, we performed an external comparison against the Jaspar database using TOMTOM. The entire workflow will be described in detail throughout the “Results” section.
3.2. Differential expression analysis shows a significant transcriptional change in sugarcane and high-temperature conditions
The RNA sequencing data were analyzed through the RNA-seq differential analysis using DESeq2. For all analyses, there were two different condition settings: the first one was the sugarcane juice condition (SCJ), and the second one was the stress conditions (comprising 37 and 42°C, and ethanol 2 and 4%). The differential expression analysis for R. toruloides grown for 8 h on the SCJ condition was measured relative to its own control, which was the inoculum grown in LB for 24 h (also called time 0 h). The 8-h timepoint was selected for this condition because cells are still in exponential phase and we wanted to grasp gene expression as soon as transcription is adapting to the new medium. The differential expression analysis for R. toruloides grown for 16 h in stress conditions was measured using the inoculum grown in YPD for 24 h as internal control. The 16-h timepoint was chosen for a similar reason: when cells are still in exponential phase but already had time to grow accustomed to the each stress. Each experimental setting was always analyzed separately since they had different internal controls. Growth curves of R. toruloides in all aforementioned conditions can be visualized in Supplementary Figure S1.
The principal component analysis (PCA) was performed to check the quality of the sequencing samples, where we could observe the consistency of our sample replicates in a summarized representation. As shown in the PCA graphs in Supplementary Figure S2A, where the two first components explain 84% of the variance, the replicates of each condition are close to each other and distant from their respective controls, which indicates that the gene expression differences are consistent inside each condition, allowing the comparison between them. Nevertheless, in Supplementary Figure S2B, we can see that the replicates of the culture grown at 37°C are very close to the control condition. While the 42°C is separated by the first principal component, explaining 50.1% of the variance alone.
To further investigate the quality of the RNA-seq experiments, the distance between samples, based on the transcript expressions, was measured (Supplementary Figure S3). In the heatmap analysis, Euclidean distance was applied to the expression pattern of each sample, where darker blue means more proximity and lighter blue means the greater distance between samples. We can see the same trend in which the replicates of each condition are grouped, which confirms the PCA analysis. Similar to PCA, in Supplementary Figure S3A, we can see that the ethanol 2% and ethanol 4% conditions were not distant from each other. This shows that the changes in transcriptional behavior in R. toruloides were not divergent between the two conditions, albeit they are both distant from the control. In Supplementary Figure S3B, it is possible to see a similar trend to PCA, where the SCJ condition is distant from its internal control.
The Venn diagram depicted in Figure 2 summarizes the DEGs found for R. toruloides grown in each experimental condition. The conditions in which more genes presented transcriptional change were the SCJ and the temperature 42°C. More than 3,000 DEGs were found for those experimental settings, while there were around 1,000 DEGs for each of the other conditions: 37°C and ethanol 2 and 4%. These results show that the latter conditions, although causing a significant change in the transcriptional behavior of R. toruloides, did not cause as much impact as the change to a rich substrate caused by the addition of sugarcane in the media nor the increase in temperature by growing at 42°C. R. toruloides grown in SCJ had a more significant number of down-regulated genes versus up-regulated genes, while growing at 42°C resulted in the opposite: more genes were up-regulated (Supplementary Figure S4). The outermost numbers in the Venn diagram represent the number of genes exclusively differentially expressed for each condition. The exclusive DEGs can lead to a new inducible promoter library, given that the promoters will likely be induced by the same condition in which their genes were differentially expressed. Although still under development, the current promoter toolbox for R. toruloides is limited (Johns et al., 2016; Liu et al., 2016; Wang et al., 2016; Nora et al., 2019). Our dataset can be useful for developing inducible promoters in the future.
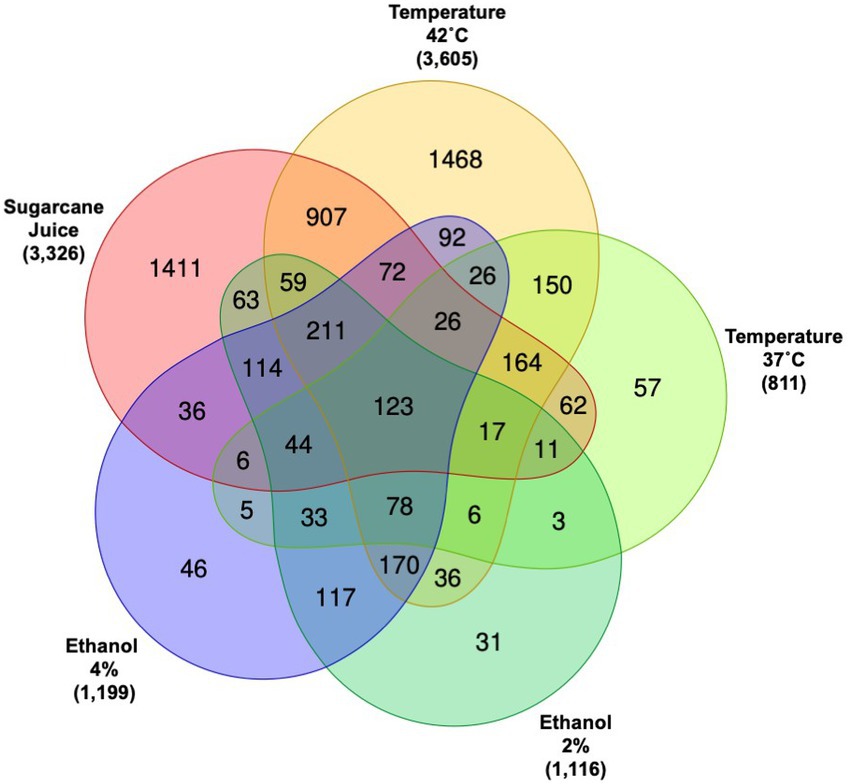
Figure 2. Venn diagram of differentially expressed genes (DEGs) for all conditions. The Venn diagram shows how many DEGs are shared between all conditions and how many are exclusive for each condition. Numbers outside of the Venn diagram represent the total of DEGs when compared to each respective control.
3.3. Functional analyses uncover KEGG pathway enrichment in each condition
We performed a pathway enrichment analysis to analyze the DEGs’ function. To do that, we first had the transcript function annotated by KEGG Orthology. Functional annotation by KEGG was the input required for the R package used for the analysis, called GAGE. Both the annotation and GAGE analysis are described in the methods section. The resulting pathways were filtered to a value of p of less than 0.05. Figure 3 shows the biochemical pathways of R. toruloides that are enriched when using SCJ as a substrate. The main up-regulated pathways enriched in this condition are those related to cell growth and metabolism, such as DNA metabolism and replication, meiosis and amino acid biosynthesis. Pathways associated with the biosynthesis of amino acids, ribosomes and cell cycle showed the greatest numbers of up-regulated genes.
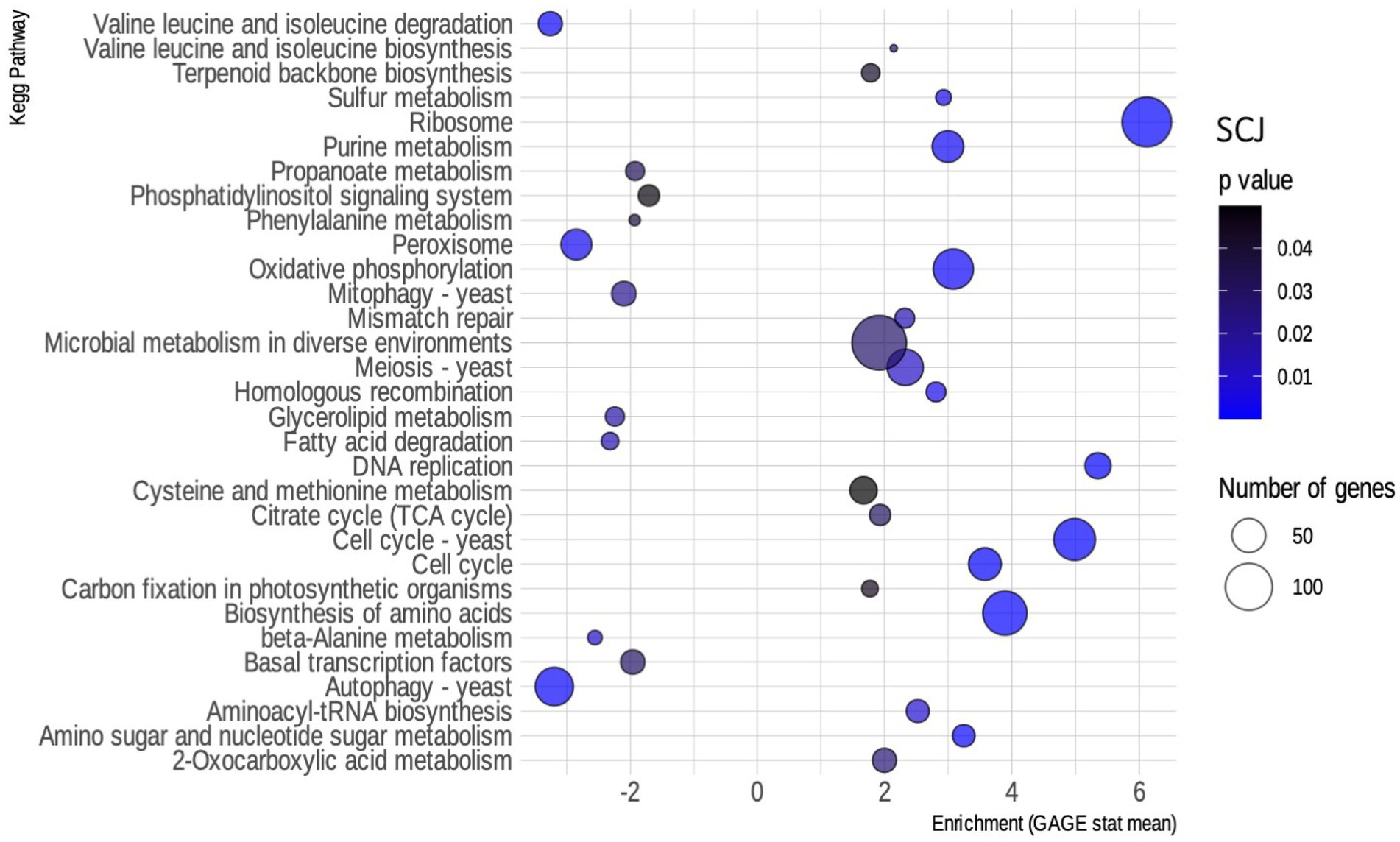
Figure 3. Enriched kyoto encyclopedia of genes and genomes (KEGG) pathways for Rhodosporidium toruloides grown on sugarcane juice (SCJ). Bubble map showing the biochemical pathways of R. toruloides noted by KEGG enriched in the SCJ condition, as obtained by the GAGE package. Pathways that have an enrichment value greater than 0 are up-regulated, while those that have a value less than 0 are down-regulated. Blue scale inside the bubbles represents the decreasing value of ps. The different sizes of the bubbles define the approximate number of DEGs in each biochemical pathway.
For the 42°C condition, although there was a higher number of up-regulated genes (Supplementary Figure S4), most of the enriched pathways were found to be down-regulated (Figure 4). Included on that list are several metabolism pathways, showing the exact opposite of what happens in SCJ—metabolism genes are being turned off. In contrast, the condition of 37°C resulted in a reduced number of differentially expressed transcripts compared to the control grown at 30°C (Supplementary Figure S5). In Supplementary Figure S6, the routes found to be enriched by GAGE by the presence of ethanol in the media can be seen. Most of the pathways are metabolism-related and down-regulated in both conditions.
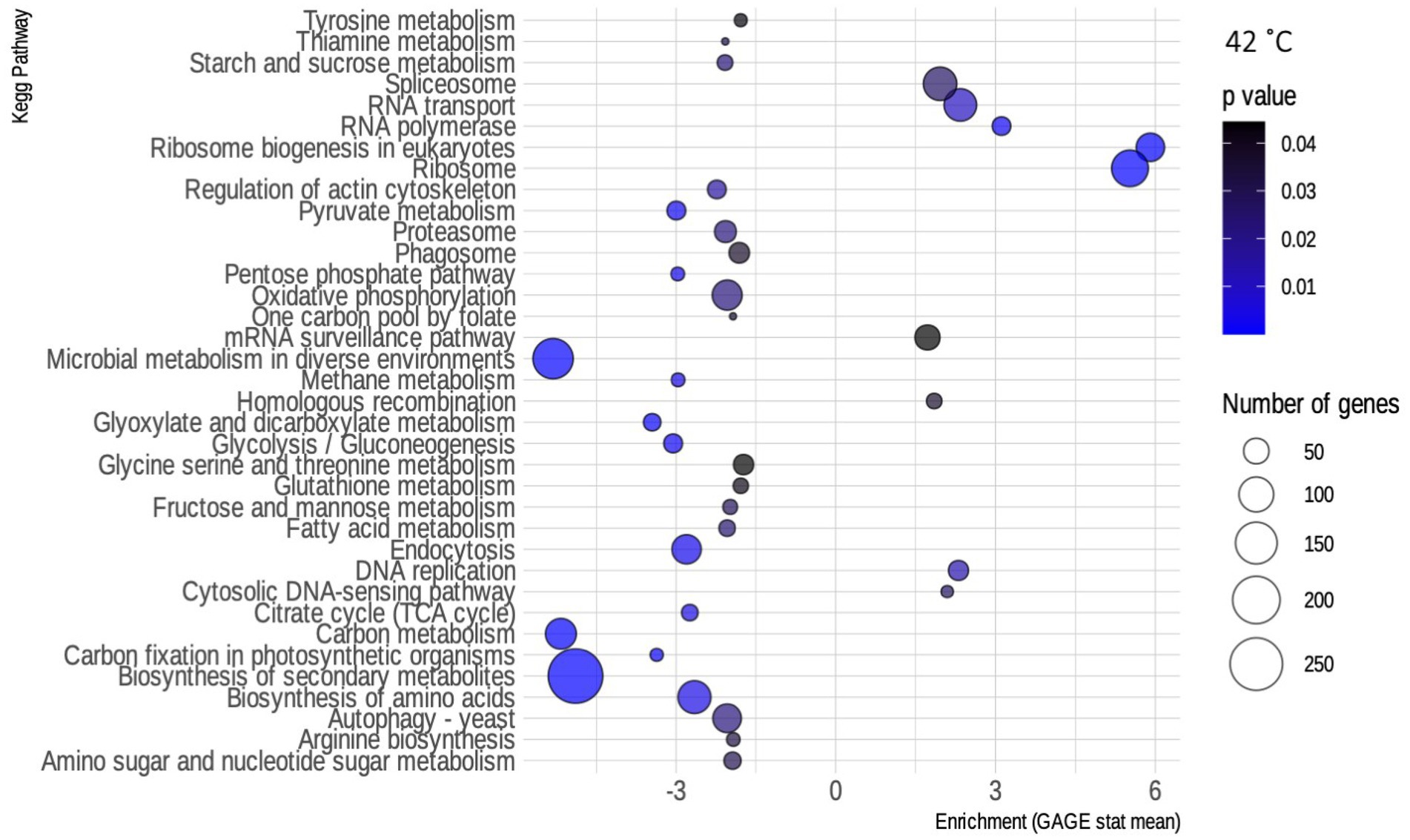
Figure 4. Enriched kyoto encyclopedia of genes and genomes (KEGG) pathways for R. toruloides grown at 42°C. Bubble map showing the biochemical pathways of R. toruloides noted by KEGG that are enriched in the 42°C condition, as obtained by the GAGE package. Pathways that have an enrichment value greater than 0 are up-regulated, while those that have a value less than 0 are down-regulated. Blue scale inside the bubbles represents the decreasing value of ps. The different sizes of the bubbles define the approximate number of DEGs in each biochemical pathway.
3.4. Identification of putative cis-regulatory elements and transcription factor candidates
Subsequently, we performed a new functional analysis using the function annotation file of the reference gene created by KOG (provided by the JGI website as described in “Materials and methods”). The new functional annotation using the KOG database was chosen due to a better correspondence with our transcript IDs resulting in a greater number of categorized genes and less redundancy in gene classes. The most significant classes of genes were selected for further analyses, which can be seen in Figure 5. All the remaining classes in which the DEGs of all conditions tested were classified according to KOG annotation are presented in Supplementary Figure S7. In both cases, data is shown as the percentage of DEGs in relation to the total number of genes in the R. toruloides genome.
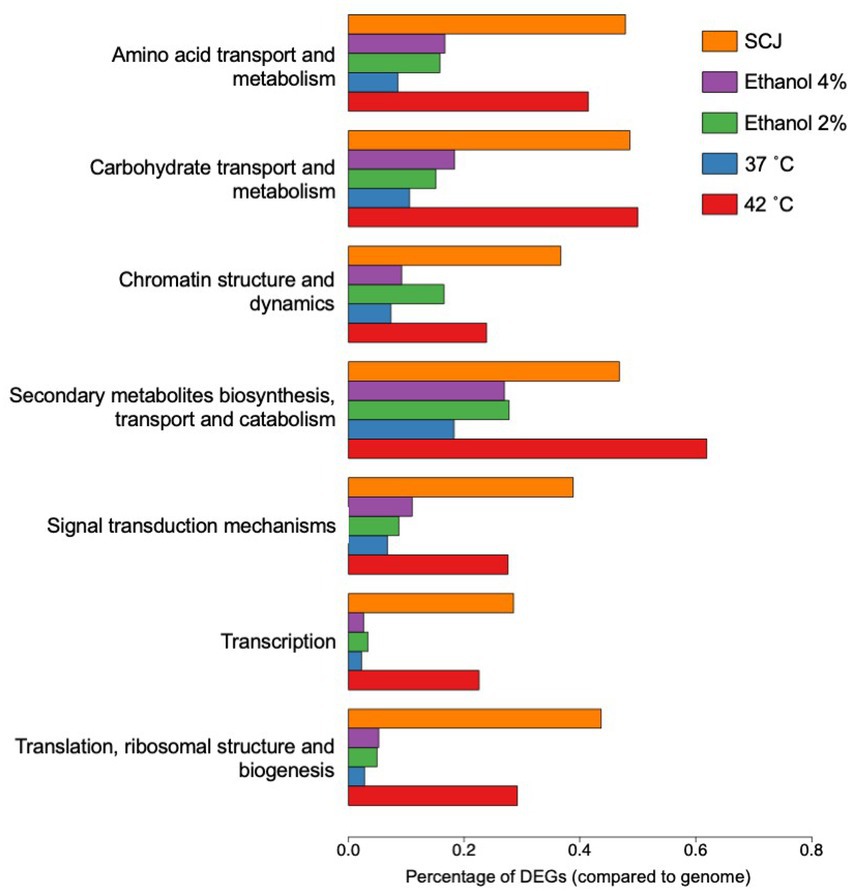
Figure 5. Eukaryotic orthologous groups (KOG) selected for TFBS finding. Percentage of DEGs compared to the total number of genes in the R. toruloides genome for each condition are shown as annotated using KOG. These are the most relevant conditions with the greatest amount of DEGs and were selected for further analysis. The remaining KOG annotations are shown in Supplementary Figure S5.
We used the transcriptomic data generated in this work to search for putative TFs and putative TFBS that might play a role in regulating both the stress response and the response to complex carbon sources. The pursuit of TF and TFBS was carried out in several stages to select suitable candidates. First, we extracted the sequence from the promoters corresponding to the genes of the KOG classes from Figure 5. By doing this, we both restricted the number of sequences to search motifs, which may reduce background noise, and we attempted to guarantee a biological relationship between these promoter sequences. Then, we used the HOMER software to obtain the putative motifs from those sequences. The motifs obtained were read on the R platform using the universal motif library. A pairwise comparison was performed between them, with Pearson’s correlation coefficient (PCC) as a method. The pairwise comparison was made following the rationale that if the motif is found in more than one gene, the chances of being a real motif increase. We also performed pairwise alignment using TOMTOM to complement the analysis. Finally, we filtered the results by a value of p less than 0.05, an e-value less than 1 and a PCC greater than 0.8. The resulting motifs were then compared to the Jaspar fungi 2018 (non-redundant DNA) database using TOMTOM, and some of these alignments can be seen in Figure 6. The motif candidates and motifs from JASPAR, aligned by TOMTOM, were plotted in a graph so that we could analyze the similarities between them (Figure 7). The main TFs corresponding to the putative TFBS found using TOMTOM are listed in Table 2. The percentage of identity of TF proteins found by this method against proteins from R. toruloides genome is also listed in Table 2, along with GO functions of the TF proteins.
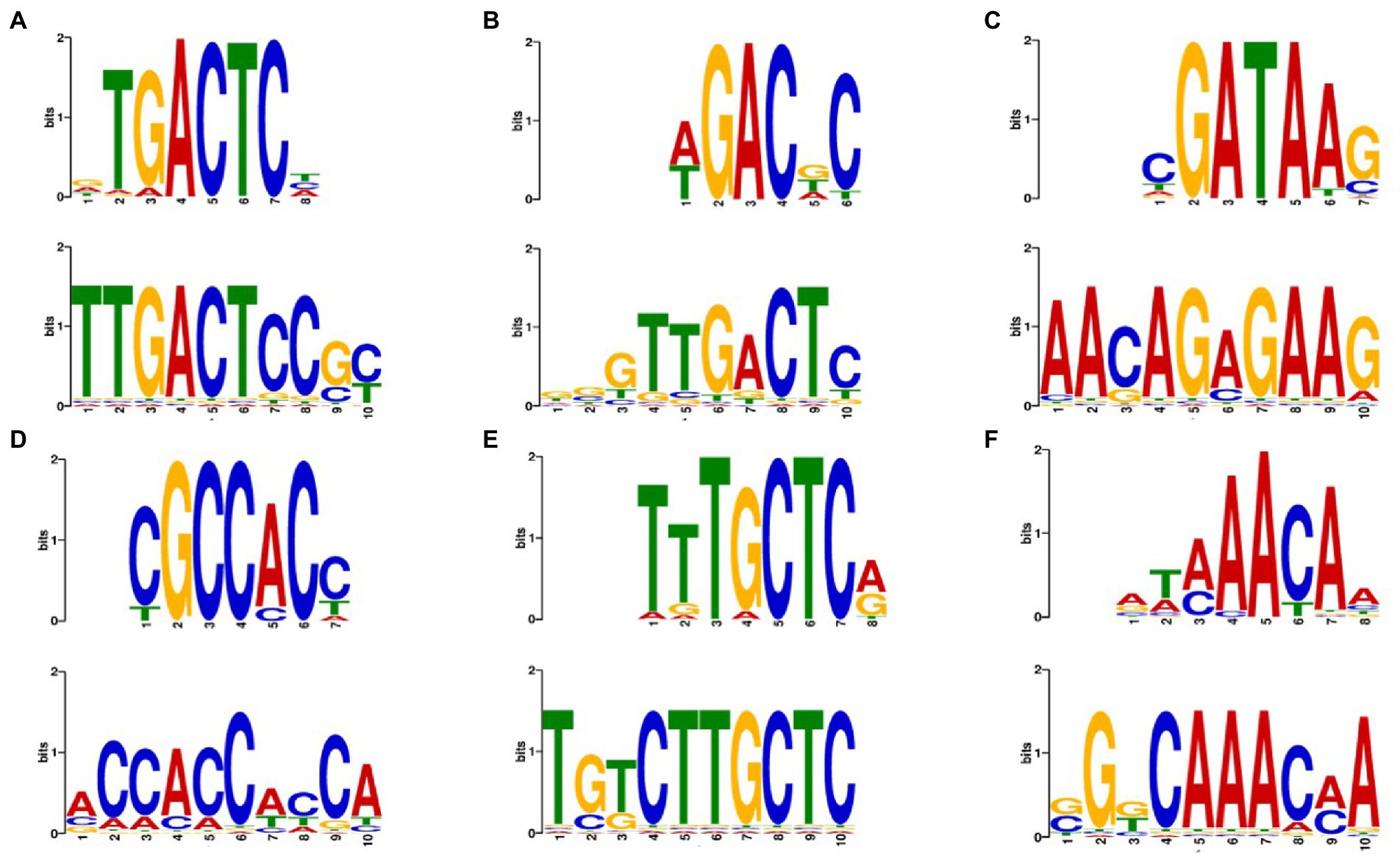
Figure 6. Examples of putative motifs aligned with transcription factors motifs from the JASPAR database using TOMTOM. (A) Top motif is the binding motif for ARG81 from Saccharomyces cerevisiae. Bottom motif is from SCJ condition. Eukaryotic orthologous groups (KOG) class: Amino acid transport and metabolism. Value of p: 1.08e–03. E-value: 1.51e + 00 (B) Top motif for AGR80 from S. cerevisiae. Bottom motif is from 42°C condition. KOG class: Amino acid transport and metabolism. Value of p: 4.98e–03. E-value: 8.76e–01. (C) Top motif for DAL80 from S. cerevisiae. Bottom motif is from ethanol 2% condition. KOG class: Amino acid transport and metabolism. value of p: 3.38e–02. E-value: 5.95e + 00. (D) Top for RPN4 from S. cerevisiae. Bottom motif is from 42°C condition. KOG class: Chromatin structure and dynamics. value of p: 4.15e–03. E-value: 7.31e–01. (E) Top motif for MAC1 from S. cerevisiae. Bottom motif is from ethanol 2% condition. KOG class: Secondary metabolites biosynthesis transport and catabolism. Value of p: 4.15e–03. E-value: 7.30e–01. (F) Top motif for HCM1 from S. cerevisiae. Bottom motif is from SCJ condition. KOG class: Chromatin structure and dynamics. Value of p: 3.44e–02. E-value: 6.05e + 00.
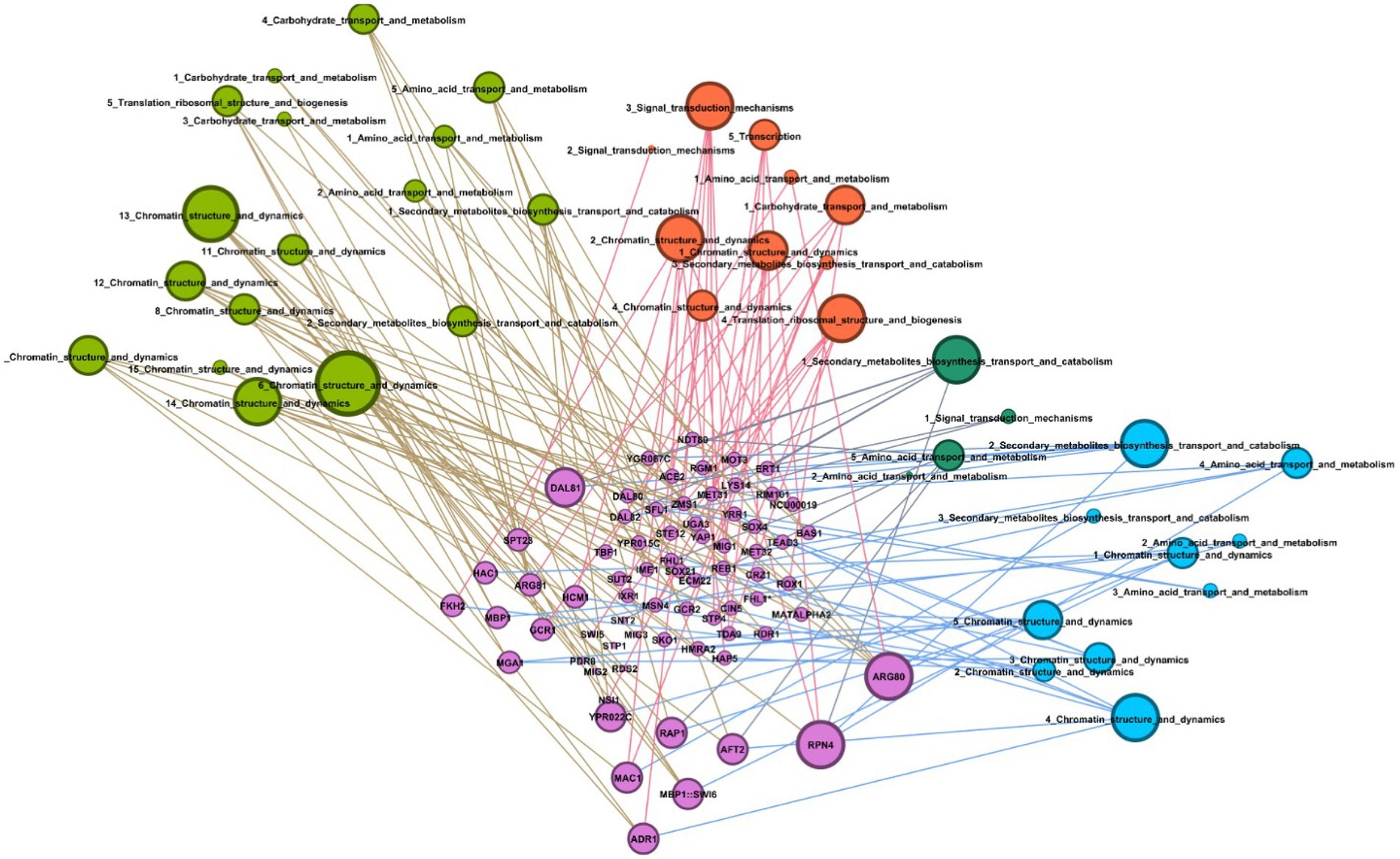
Figure 7. Graph of putative transcription factors and their putative binding sites. Graph showing interaction between putative transcription factors for R. toruloides with the transcription factors (TF) binding motifs predicted using HOMER. Green nodes represent the sugarcane juice condition, orange nodes represent the 42°C condition, dark green nodes are the 2% ethanol, and blue nodes are the 4% ethanol condition. Written inside nodes are the number of the TFBS and the name of the gene class annotated by eukaryotic orthologous groups (KOG) in which that motif was found. Pink nodes are the putative transcription FTs found using TOMTOM.
4. Discussion
In this work, we developed a reproducible bioinformatics workflow that can be used to discover regulatory elements using transcriptomic data as input. We applied to the emergent microbial cell factory R. toruloides. First, we analyzed the transcriptomic data and showed that R. toruloides presented different transcriptional responses to all the conditions tested. When using media containing SCJ and urea, functional analysis showed a predominance of metabolism pathways being up-regulated, while degradation pathways such as autophagy and peroxisome are down-regulated. This pattern is reasonable since the yeast adapted its metabolism from the inoculum containing no sugar to the rich and complex environment provided by SCJ.
Interestingly, one of the pathways found to be up-regulated by GAGE was the terpenoid backbone biosynthesis. This is a good indicator that we can use the abundant and inexpensive media composed of sugarcane and urea as a start point substrate to produce terpenes in R. toruloides, like previously done using corn stover hydrolysates (Kirby et al., 2021). Moreover, when growing the yeast at 42°C, cytosolic DNA sensing pathways and mRNA surveillance pathways were up-regulated and represent cell stress responses (Jamar et al., 2017; Meng et al., 2021). Similarly, in this condition, the homologous recombination pathway is up-regulated—possibly for dealing with cell damage (Kawale and Sung, 2020). We also hypothesized that the transcriptional responses to the two high-temperature conditions could be classified into two distinct types of stress responses. The 42°C condition caused the heat-shock response, when cells repress protein biosynthesis—as we can see by the decrease of the biosynthesis of amino acids—and protein misfolding and oxidative stress occur (Verghese et al., 2012).
In contrast, the condition of 37°C might have reached the other type of response: thermotolerance—given that most transcripts were not differentially expressed compared to the control grown at 30°C. The very few pathways found to be enriched in this condition, like cell cycle and oxidative phosphorylation, might suggest that R. toruloides is enduring the increase in temperature (Shui et al., 2015; Huang et al., 2018). Nevertheless, another study showed that cultivating R. toruloides at 37°C significantly impaired its growth (Wu et al., 2020).
Yet, the ethanol response in our yeast remarkably corresponds with what is described in the literature for ethanol stress and tolerance in S. cerevisiae (Stanley et al., 2010; Yoshida et al., 2021). It is interesting to note that cell cycle and DNA replication pathways are down-regulated, showing inhibition of cell growth, as expected for ethanol stress (Stanley et al., 2010). Inhibition of endocytosis was also reported as an ethanol stress response (Lucero et al., 2000). We can see that both ethanol concentrations decreased the expression of R. toruloides endocytosis genes. Additionally, the proteasome genes are up-regulated, demonstrating that cells are dealing with misfolded proteins, which is described in the literature as a sign of ethanol stress (Bubis et al., 2020). Hence, we hypothesized that the response to the two different concentrations of ethanol is still a stress response and that R. toruloides has not yet been able to tolerate the compound (Lucero et al., 2000; Stanley et al., 2010; Bubis et al., 2020).
Furthermore, we explored the cis-regulatory elements related to the DEGs in the transcriptomic analysis. The TFBS predicted for R. toruloides presented similarity mainly with binding sites for the following TFs: ARG80, RPN4, ADR1, and DAL81. Those TFs can be seen in the graph (Figure 7) as having a more significant number of similar putative motifs. As demonstrated in Table 2, HAC1 protein is involved in ER-unfolded protein response, and RPN4 protein is involved in response to stress. This could explain the enrichment of both their binding motifs in our industrial stress conditions. Studies showed that mutations in the RPN4 protein conferred ethanol resistance to S. cerevisiae (Bubis et al., 2020). However, no homologs of these proteins were found in our carotenogenic yeast genome. Some TFs were responsive to oxidative stress, such as AFT2 and HCM1, whereas only HCM1 was found in the R. toruloides genome.
Interestingly, the DAL81 protein was found to be mutated in response to high-temperature stress in a study by Huang et al. (2018). This could explain the enrichment of its binding motifs in our heat shock transcripts, although a homolog of this protein was not found. SWI6 binding motifs may be an exciting source for future studies, since this protein was found in R. toruloides genome with 39% identity, which regulates the heat stress response. Interestingly, binding motifs for SPT23, a protein that regulates cold response, were also found. This protein was found in the genome of R. toruloides with 45% identity.
Nevertheless, the binding of the TF proteins to their respective motifs discovered by our pipeline needs to be further tested to confirm their activity. Alternatively, in the case of the ones whose corresponding binding protein homologs were not found, the identified motifs could be recognized by yet-unknown TFs. As shown by Antonieto et al. (2019), a transcriptional regulator with low homology to AZF1 recognizes a well-conserved motif regulating the expression of cellulases genes.
The motifs found can be used as starting points for new transcriptional modulation studies. The ultimate goal would be to define a core promoter sequence for R. toruloides and then change the sequences of TFBS according to the desired behavior. For instance, one can study ethanol tolerance in this organism by adding the RPN4 consensus sequence to their respective constructs. Additionally, one can use our pipeline for other organisms with experimentally-defined functional promoter sequences, where scientists can compose motif sequences and their locations to engineer new transcriptional behaviors (D’Ambrosio and Jensen, 2017; Monteiro et al., 2020; Feng and Marchisio, 2021; Tominaga et al., 2022). Also, as mentioned in the Results section, our transcriptomic data can be used to create new promoter libraries for our yeast. Ultimately, anyone can resort to our tools to generate a better transcriptional understanding of their organism of interest.
5. Conclusion
Here, we characterized the transcriptional responses of R. toruloides when growing in industry-like conditions and discovered new regulatory elements enriched in each context. We showed how differential gene expression, followed by a custom, reproducible bioinformatics motif discovery workflow, could predict putative motifs for binding transcription factors in our strain, most of which involved stress-related responses. DNA motifs similar to the binding sites for ADR1, ARG80, DAL81, and RPN4 transcription factors were the most abundantly enriched in our dataset. While ADR1 and ARG80 proteins were found in the R. toruloides genome with 54 and 60% of identity, respectively, DAL81 and RPN4 were not found. The novel putative cis-regulatory elements described here offer a great initial point to optimize gene regulation in industrial conditions expanding the current knowledge on regulatory networks for this yeast. Furthermore, our pipeline for motif discovery can be easily applied to other unexplored hosts.
Data availability statement
The datasets presented in this study can be found in online repositories. The names of the repository/repositories and accession number(s) can be found at: https://www.ncbi.nlm.nih.gov/bioproject/PRJNA883675/, PRJNA883675.
Author contributions
RS-R, M-EG, LN, and MC designed the project. LN and ÍS performed RNA experiments. MC, LN, and ÍS performed the differential expression analysis. MC developed the motif discovery pipeline. RS contributed substantially to the analysis, interpretation of data, and availability of the developed workflow. LN wrote the manuscript. All authors contributed to the article and approved the submitted version.
Funding
This work was supported by the São Paulo Research Foundation (FAPESP), the National Council for Scientific and Technological Development (CNPq), and CAPES, all from Brazil. LN was funded by FAPESP grant number 2019/04942-7 and CNPq grant number 140212/2019-1. M-EG was funded by FAPESP grant number 2021/01748-5. RS-R was funded by FAPESP grant numbers 2019/15675-0. MC and ÍS were funded by CAPES. RS was funded by FAPESP grant numbers 2017/18922-2, 2019/05026-4 and 2020/02207-5.
Acknowledgments
The authors would like to thank all the collaborators from the Joint Bioenergy Institute (Emeryville, California).
Conflict of interest
The authors declare that the research was conducted in the absence of any commercial or financial relationships that could be construed as a potential conflict of interest.
Publisher’s note
All claims expressed in this article are solely those of the authors and do not necessarily represent those of their affiliated organizations, or those of the publisher, the editors and the reviewers. Any product that may be evaluated in this article, or claim that may be made by its manufacturer, is not guaranteed or endorsed by the publisher.
Supplementary material
The Supplementary material for this article can be found online at: https://www.frontiersin.org/articles/10.3389/fmicb.2022.1069443/full#supplementary-material
Abbreviations
DEG, differentially expressed genes; GO, gene ontology; KEGG, Kyoto Encyclopedia of genes and genomes; KOG, eukaryotic Orthologous Groups; PCC, Pearson’s correlation coefficient; SCJ, sugarcane juice; TF, transcription factor; TFBS, transcription factor binding site.
Footnotes
References
Abdel-Banat, B. M. A., Hoshida, H., Ano, A., Nonklang, S., and Akada, R. (2010). High-temperature fermentation: how can processes for ethanol production at high temperatures become superior to the traditional process using mesophilic yeast? Appl. Microbiol. Biotechnol. 85, 861–867. doi: 10.1007/s00253-009-2248-5
Ambrosini, G., Vorontsov, I., Penzar, D., Groux, R., Fornes, O., Nikolaeva, D. D., et al. (2020). Insights gained from a comprehensive all-against-all transcription factor binding motif benchmarking study. Genome Biol. 21:114. doi: 10.1186/s13059-020-01996-3
Andrews, S. (2018). FastQC: a quality control tool for high throughput sequence data, FastQC: a quality control tool for high throughput sequence data. Available at: http://www.bioinformatics.babraham.ac.uk/projects/fastqc/ (Accessed October 10, 2022).
Antonieto, A. C. C., Nogueira, K. M. V., de Paula, R. G., Nora, L. C., Cassiano, M. H. A., Guazzaroni, M. E., et al. (2019). A novel Cys2His2 zinc finger homolog of AZF1 modulates Holocellulase expression in Trichoderma reesei. mSystems 4, 1–14. doi: 10.1128/mSystems.00161-19
Bastian, M., Heymann, S., and Jacomy, M. (2009). ‘Gephi: an open source software for exploring and manipulating networks’. In Proceedings of the Third International ICWSM Conference, pp. 361–362.
Bolger, A. M., Lohse, M., and Usadel, B. (2014). Trimmomatic: a flexible trimmer for Illumina sequence data. Bioinformatics 30, 2114–2120. doi: 10.1093/bioinformatics/btu170
Bonturi, N., Crucello, A., Viana, A. J. C., and Miranda, E. A. (2017). Microbial oil production in sugarcane bagasse hemicellulosic hydrolysate without nutrient supplementation by a Rhodosporidium toruloides adapted strain. Process Biochem. 57, 16–25. doi: 10.1016/j.procbio.2017.03.007
Bonturi, N., Pinheiro, M. J., de Oliveira, P. M., Rusadze, E., Eichinger, T., Liudžiūtė, G., et al. (2022). ‘Development of a dedicated Golden Gate assembly platform (RtGGA) for Rhodotorula toruloides’, metabolic engineering. Communications 15, 1–10. doi: 10.1016/j.mec.2022.e00200
Borodina, I., and Nielsen, J. (2014). Advances in metabolic engineering of yeast Saccharomyces cerevisiae for production of chemicals. Biotechnol. J. 9, 609–620. doi: 10.1002/biot.201300445
Bray, N. L., Pimentel, H., Melsted, P., and Pachter, L. (2016). Near-optimal probabilistic RNA-seq quantification. Nat. Biotechnol. 34, 525–527. doi: 10.1038/nbt.3519
Bubis, J. A., Spasskaya, D. S., Gorshkov, V. A., Kjeldsen, F., Kofanova, A. M., Lekanov, D. S., et al. (2020). Rpn4 and proteasome-mediated yeast resistance to ethanol includes regulation of autophagy. Appl. Microbiol. Biotechnol. 104, 4027–4041. doi: 10.1007/s00253-020-10518-x
Cherubini, F. (2010). The biorefinery concept: using biomass instead of oil for producing energy and chemicals. Energy Convers. Manag. 51, 1412–1421. doi: 10.1016/j.enconman.2010.01.015
Coradetti, S. T., Pinel, D., Geiselman, G. M., Ito, M., Mondo, S. J., Reilly, M. C., et al. (2018). Functional genomics of lipid metabolism in the oleaginous yeast Rhodosporidium toruloides. elife 7:e32110. doi: 10.7554/eLife.32110
D’Ambrosio, V., and Jensen, M. K. (2017). Lighting up yeast cell factories by transcription factor-based biosensors. FEMS Yeast Res. 17, 1–12. doi: 10.1093/femsyr/fox076
Devi, A., Bajar, S., Kour, H., Kothari, R., Pant, D., and Singh, A. (2022). Lignocellulosic biomass valorization for bioethanol production: a circular bioeconomy approach. Bioenergy Res. 15, 1820–1841. doi: 10.1007/s12155-022-10401-9
Feng, X., and Marchisio, M. A. (2021). Saccharomyces cerevisiae promoter engineering before and during the synthetic biology era. Biology 10:504. doi: 10.3390/biology10060504
Geiselman, G. M., Zhuang, X., Kirby, J., Tran-Gyamfi, M. B., Prahl, J. P., Sundstrom, E. R., et al. (2020). Production of ent-kaurene from lignocellulosic hydrolysate in Rhodosporidium toruloides. Microb. Cell Factories 19, 24–12. doi: 10.1186/s12934-020-1293-8
Gupta, S., Stamatoyannopoulos, J. A., Bailey, T. L., and Noble, W. (2007). Quantifying similarity between motifs. Genome Biol. 8:R24. doi: 10.1186/gb-2007-8-2-r24
Heinz, S., Benner, C., Spann, N., Bertolino, E., Lin, Y. C., Laslo, P., et al. (2010). Simple combinations of lineage-determining transcription factors prime cis-regulatory elements required for macrophage and B cell identities. Mol. Cell 38, 576–589. doi: 10.1016/j.molcel.2010.05.004
Huang, C. J., Lu, M. Y., Chang, Y. W., and Li, W. H. (2018). Experimental evolution of yeast for high-temperature tolerance. Mol. Biol. Evol. 35, 1823–1839. doi: 10.1093/molbev/msy077
Inukai, S., Kock, K. H., and Bulyk, M. L. (2017). Transcription factor–DNA binding: beyond binding site motifs. Curr. Opin. Genet. Dev. 43, 110–119. doi: 10.1016/j.gde.2017.02.007
Jagtap, S. S., Bedekar, A. A., Liu, J. J., Jin, Y. S., and Rao, C. V. (2019). Production of galactitol from galactose by the oleaginous yeast Rhodosporidium toruloides IFO0880. Biotechnol. Biofuels 12, 250–213. doi: 10.1186/s13068-019-1586-5
Jagtap, S. S., and Rao, C. V. (2018). Production of d-arabitol from d-xylose by the oleaginous yeast Rhodosporidium toruloides IFO0880. Appl. Microbiol. Biotechnol. 102, 143–151. doi: 10.1007/s00253-017-8581-1
Jamar, N. H., Kritsiligkou, P., and Grant, C. M. (2017). The non-stop decay mRNA surveillance pathway is required for oxidative stress tolerance. Nucleic Acids Res. 45, 6881–6893. doi: 10.1093/nar/gkx306
Johns, A. M. B., Love, J., and Aves, S. J. (2016). Four inducible promoters for controlled gene expression in the oleaginous yeast Rhodotorula toruloides. Front. Microbiol. 7, 1–12. doi: 10.3389/fmicb.2016.01666
Kawale, A. S., and Sung, P. (2020). Mechanism and significance of chromosome damage repair by homologous recombination. Essays Biochem. 64, 779–790. doi: 10.1042/EBC20190093
Kirby, J., Geiselman, G. M., Yaegashi, J., Kim, J., Zhuang, X., Tran-Gyamfi, M. B., et al. (2021). Further engineering of R. toruloides for the production of terpenes from lignocellulosic biomass. Biotechnol. Biofuels 14, 101–116. doi: 10.1186/s13068-021-01950-w
Ksouri, N., Castro-Mondragón, J. A., Montardit-Tarda, F., van Helden, J., Contreras-Moreira, B., and Gogorcena, Y. (2021). Tuning promoter boundaries improves regulatory motif discovery in nonmodel plants: the peach example. Plant Physiol. 185, 1242–1258. doi: 10.1093/plphys/kiaa091
Lin, X., Wang, Y., Zhang, S., Zhu, Z., Zhou, Y. J., Yang, F., et al. (2014). Functional integration of multiple genes into the genome of the oleaginous yeast Rhodosporidium toruloides. FEMS Yeast Res. 14, 547–555. doi: 10.1111/1567-1364.12140
Liu, Y., Cruz-Morales, P., Zargar, A., Belcher, M. S., Pang, B., Englund, E., et al. (2021). Biofuels for a sustainable future. Cells 184, 1636–1647. doi: 10.1016/j.cell.2021.01.052
Liu, Y., Yap, S. A., Koh, C. M. J., and Ji, L. (2016). Developing a set of strong intronic promoters for robust metabolic engineering in oleaginous Rhodotorula (Rhodosporidium) yeast species. Microb. Cell Fact. 15, 200–209. doi: 10.1186/s12934-016-0600-x
Lopes, H. J. S. (2020). Estratégias de adaptação e de cultivo de Rhodotorula torloides visando produção de óleo microbiano a partir de hidrolisados hemicelulósicos, Portuguese: Universidade Estadual de Campinas, pp. 1–9.
Love, M. I., Huber, W., and Anders, S. (2014). Moderated estimation of fold change and dispersion for RNA-seq data with DESeq2. Genome Biol. 15, 550–521. doi: 10.1186/s13059-014-0550-8
Lucero, P., Peñalver, E., Moreno, E., and Lagunas, R. (2000). Internal trehalose protects endocytosis from inhibition by ethanol in Saccharomyces cerevisiae. Appl. Environ. Microbiol. 66, 4456–4461. doi: 10.1128/AEM.66.10.4456-4461.2000
Luo, W., and Brouwer, C. (2013). Pathview: an R/bioconductor package for pathway-based data integration and visualization. Bioinformatics 29, 1830–1831. doi: 10.1093/bioinformatics/btt285
Luo, W., Friedman, M. S., Shedden, K., Hankenson, K. D., and Woolf, P. J. (2009). GAGE: generally applicable gene set enrichment for pathway analysis. BMC Bioinform. 10, 1–17. doi: 10.1186/1471-2105-10-161
Martins, L. C., Palma, M., Angelov, A., Nevoigt, E., Liebl, W., and Sá-Correia, I. (2021). Complete utilization of the major carbon sources present in sugar beet pulp hydrolysates by the oleaginous red yeasts Rhodotorula toruloides and Mucilaginosa. J. Fungi 7, 1–21. doi: 10.3390/jof7030215
Martins-Santana, L., Nora, L. C., Sanches-Medeiros, A., Lovate, G. L., Cassiano, M. H. A., and Silva-Rocha, R. (2018). Systems and synthetic biology approaches to engineer fungi for fine chemical production. Front. Bioeng. Biotechnol. 6, 1–18. doi: 10.3389/fbioe.2018.00117
Meng, L., Yang, X., Lin, X., Jiang, H. Y., Hu, X. P., and Liu, S. X. (2021). Effect of overexpression of SNF1 on the transcriptional and metabolic landscape of baker’s yeast under freezing stress. Microb. Cell Factories 20, 10–13. doi: 10.1186/s12934-020-01503-0
Monteiro, L. M. O., Sanches-Medeiros, A., Westmann, C. A., and Silva-Rocha, R. (2020). Unraveling the complex interplay of Fis and IHF through synthetic promoter engineering. Front. Bioeng. Biotechnol. 8, 1–13. doi: 10.3389/fbioe.2020.00510
Nora, L. C., Wehrs, M., Kim, J., Cheng, J. F., Tarver, A., Simmons, B. A., et al. (2019). A toolset of constitutive promoters for metabolic engineering of Rhodosporidium toruloides. Microb. Cell Factories 18, 117–111. doi: 10.1186/s12934-019-1167-0
Nora, L. C., Westmann, C. A., Guazzaroni, M. E., Siddaiah, C., Gupta, V. K., and Silva-Rocha, R. (2019). Recent advances in plasmid-based tools for establishing novel microbial chassis. Biotechnol. Adv. 37:107433. doi: 10.1016/j.biotechadv.2019.107433
Novakovsky, G., Saraswat, M., Fornes, O., Mostafavi, S., and Wasserman, W. W. (2021). Biologically relevant transfer learning improves transcription factor binding prediction. Genome Biol. 22:280. doi: 10.1186/s13059-021-02499-5
Park, Y. K., Nicaud, J. M., and Ledesma-Amaro, R. (2018). The engineering potential of Rhodosporidium toruloides as a workhorse for biotechnological applications. Trends Biotechnol. 36, 304–317. doi: 10.1016/j.tibtech.2017.10.013
R Core Team (2019). A language and environment for statistical computing. R Foundation for Statistical Computings. Available at: http://www.r-project.org (Accessed October 10, 2022).
Saini, R., Hegde, K., Brar, S. K., and Vezina, P. (2020). Advanced biofuel production and road to commercialization: an insight into bioconversion potential of Rhodosporidium sp. Biomass Bioenergy 132:105439. doi: 10.1016/j.biombioe.2019.105439
Shui, W., Xiong, Y., Xiao, W., Qi, X., Zhang, Y., Lin, Y., et al. (2015). Understanding the mechanism of thermotolerance distinct from heat shock response through proteomic analysis of industrial strains of saccharomyces cerevisiae. Mol. Cell. Proteomics 14, 1885–1897. doi: 10.1074/mcp.M114.045781
Soccol, C. R., Dalmas Neto, C. J., Soccol, V. T., Sydney, E. B., da Costa, E. S. F., Medeiros, A. B. P., et al. (2017). Pilot scale biodiesel production from microbial oil of Rhodosporidium toruloides DEBB 5533 using sugarcane juice: performance in diesel engine and preliminary economic study. Bioresour. Technol. 223, 259–268. doi: 10.1016/j.biortech.2016.10.055
Stanley, D., Bandara, A., Fraser, S., Chambers, P. J., and Stanley, G. A. (2010). The ethanol stress response and ethanol tolerance of Saccharomyces cerevisiae. J. Appl. Microbiol. 109, 13–24. doi: 10.1111/j.1365-2672.2009.04657.x
Tan, E. C. D., and Lamers, P. (2021). Circular bioeconomy concepts—a perspective. Front. Sustain. 2, 1–8. doi: 10.3389/frsus.2021.701509
Tominaga, M., Kondo, A., and Ishii, J. (2022). Engineering of synthetic transcriptional switches in yeast. Life 12, 1–20. doi: 10.3390/life12040557
Tremblay, B. (2020). ‘Universalmotif: Import, modify, and export motifs with R.’. Bioconductor, R package. Available at: https://bioconductor.org/packages/universalmotif/ (Accessed October 10, 2022).
Verghese, J., Abrams, J., Wang, Y., and Morano, K. A. (2012). Biology of the heat shock response and protein chaperones: budding yeast (Saccharomyces cerevisiae) as a model system. Microbiol. Mol. Biol. Rev. 76, 115–158. doi: 10.1128/mmbr.05018-11
Wang, Y., Lin, X., Zhang, S., Sun, W., Ma, S., and Zhao, Z. K. (2016). Cloning and evaluation of different constitutive promoters in the oleaginous yeast Rhodosporidium toruloides. Yeast 33, 99–106. doi: 10.1002/yea.3145
Wehrs, M., Tanjore, D., Eng, T., Lievense, J., Pray, T. R., and Mukhopadhyay, A. (2019). Engineering robust production microbes for large-scale cultivation. Trends Microbiol. 27, 524–537. doi: 10.1016/J.TIM.2019.01.006
Wetzel, J. L., Zhang, K., and Singh, M. (2022). Learning probabilistic protein–DNA recognition codes from DNA-binding specificities using structural mappings. Genome Res. 32, 1776–1786. doi: 10.1101/gr.276606.122
Wu, C.-C., Ohashi, T., Misaki, R., Limtong, S., and Fujiyama, K. (2020). Ethanol and H2 O2 stresses enhance lipid production in an oleaginous Rhodotorula toruloides thermotolerant mutant L1-1. FEMS Yeast Res. 20, 1–14. doi: 10.1093/femsyr/foaa030
Yaegashi, J., Kirby, J., Ito, M., Sun, J., Dutta, T., Mirsiaghi, M., et al. (2017). Rhodosporidium toruloides: a new platform organism for conversion of lignocellulose into terpene biofuels and bioproducts. Biotechnol. Biofuels 10, 241–213. doi: 10.1186/s13068-017-0927-5
Keywords: transcription factor, motifs, RNA sequencing, sugarcane juice, industrial stress, cell factory, biorefineries
Citation: Nora LC, Cassiano MHA, Santana ÍP, Guazzaroni M-E, Silva-Rocha R and da Silva RR (2023) Mining novel cis-regulatory elements from the emergent host Rhodosporidium toruloides using transcriptomic data. Front. Microbiol. 13:1069443. doi: 10.3389/fmicb.2022.1069443
Edited by:
Sujit Jagtap, University of Illinois at Urbana-Champaign, United StatesReviewed by:
Ashwini Bedekar, University of Illinois at Urbana-Champaign, United StatesShashi Kant Bhatia, Konkuk University, South Korea
Copyright © 2023 Nora, Cassiano, Santana, Guazzaroni, Silva-Rocha and da Silva. This is an open-access article distributed under the terms of the Creative Commons Attribution License (CC BY). The use, distribution or reproduction in other forums is permitted, provided the original author(s) and the copyright owner(s) are credited and that the original publication in this journal is cited, in accordance with accepted academic practice. No use, distribution or reproduction is permitted which does not comply with these terms.
*Correspondence: Luísa Czamanski Nora, bGNub3JhQGdtYWlsLmNvbQ==; Ricardo Roberto da Silva, cmlkYXNpbHZhQHVzcC5icg==
†These authors have contributed equally to this work