- 1Delft Bioinformatics Lab, Delft University of Technology, Delft, Netherlands
- 2Department of Biotechnology, Delft University of Technology, Delft, Netherlands
- 3Infectious Disease and Microbiome Program, Broad Institute of MIT and Harvard, Cambridge, MA, United States
The success of antibiotics as a therapeutic agent has led to their ineffectiveness. The continuous use and misuse in clinical and non-clinical areas have led to the emergence and spread of antibiotic-resistant bacteria and its genetic determinants. This is a multi-dimensional problem that has now become a global health crisis. Antibiotic resistance research has primarily focused on the clinical healthcare sectors while overlooking the non-clinical sectors. The increasing antibiotic usage in the environment – including animals, plants, soil, and water – are drivers of antibiotic resistance and function as a transmission route for antibiotic resistant pathogens and is a source for resistance genes. These natural compartments are interconnected with each other and humans, allowing the spread of antibiotic resistance via horizontal gene transfer between commensal and pathogenic bacteria. Identifying and understanding genetic exchange within and between natural compartments can provide insight into the transmission, dissemination, and emergence mechanisms. The development of high-throughput DNA sequencing technologies has made antibiotic resistance research more accessible and feasible. In particular, the combination of metagenomics and powerful bioinformatic tools and platforms have facilitated the identification of microbial communities and has allowed access to genomic data by bypassing the need for isolating and culturing microorganisms. This review aimed to reflect on the different sequencing techniques, metagenomic approaches, and bioinformatics tools and pipelines with their respective advantages and limitations for antibiotic resistance research. These approaches can provide insight into resistance mechanisms, the microbial population, emerging pathogens, resistance genes, and their dissemination. This information can influence policies, develop preventative measures and alleviate the burden caused by antibiotic resistance.
Introduction
Antibiotic-resistant bacterial infections have contributed to 4.95 million deaths worldwide, with 1.27 million of those directly resulting from antimicrobial resistance (AMR; Gigante et al., 2022; Papavarnavas and Mendelson, 2022). It is the leading cause of death worldwide and affects high, low, and middle-income countries (Pokharel et al., 2019; Gupta and Gupta, 2021). Antibiotic-resistant bacterial infections are rising exponentially, making them harder to treat (Dušan et al., 2016; Robinson et al., 2016). This continuation will lead to an estimated 10 million deaths annually by 2050 if the burden of AMR is not alleviated (Van Boeckel et al., 2015).
For most of human history, bacteria have caused infectious diseases and contributed to high mortality rates (MacLean and San, 2019). To counteract this, antibiotics were routinely prescribed to treat and prevent such diseases. This saved millions of lives each year. Due to its success, antibiotics were used in animal husbandry and agricultural practices with the added benefits of growth promotion (Kang et al., 2018). Unfortunately, this success was limited to the emergence of antibiotic-resistant bacteria (ARB) and its effects on human health and longevity (Fair and Tor, 2014; MacLean and San, 2019; Kumar et al., 2020).
The continuous and unwarranted use of antibiotics in humans, animals, and agricultural areas exerts selection pressure on the bacteria found in these sectors. This gives rise to bacteria resistant to multiple antibiotics classes leading to untreatable infections (Davies and Davies, 2010; Cabello and Godfrey, 2016).
The ability of bacteria to resist antibiotics is embedded in their evolutionary history, which has led to various phenotypic and genotypic resistance mechanisms (Pornsukarom and Thakur, 2017; Power, 2019). These include the inactivation, modification, degradation, and expulsion of the antibiotic or its target site, therefore protecting the bacteria (Capita and Alonso-Calleja, 2013; Blair et al., 2015; Munita and Arias, 2016; Kumar, 2017; Kang et al., 2018). It is also possible that bacteria gain resistance through mutations, changing the nature of proteins expressed in the bacterial organism, or by horizontal gene transfer phenomena, which is the exchange of genetic material by the use of mobile genetic elements (MGEs) between bacterial strains (Pornsukarom and Thakur, 2017; MacLean and San, 2019). This allows bacteria to collect numerous resistant traits and ultimately become multi-drug resistant (MDR; Munita and Arias, 2016; von Wintersdorff et al., 2016; Kumar, 2017; Power, 2019).
The ineffectiveness of antibiotics threatens the ability to treat common bacterial infections and minor injuries. Since first-line antibiotics are deemed sub-par, more expensive treatment, alternative therapy and additional care is necessary. This can result in prolonged infections and an increase in morbidity and mortality rates. This puts an economic burden on families, society, and modern medicine (Gandra et al., 2014; Friedman et al., 2016; Aslam et al., 2018; Power, 2019).
For over a decade, antibiotic resistance has been studied using the traditional culture-dependent approach. This involves the use of artificial conditions to culture microorganisms. Unfortunately, some microorganisms cannot be cultured by this approach (Schmieder and Edwards, 2012; Nowrotek et al., 2019). Approximately 80% of bacterial species in the human gut and 99% of environmental bacterial species remain uncultured (Lagier et al., 2012; Nowrotek et al., 2019). Factors such as slow growth, microbial competition, specific growth requirements, and environmental stressors can affect the culturing process. This leaves limited information regarding gene transfer between these bacterial communities (Garmendia et al., 2012; Schmieder and Edwards, 2012; Suenaga, 2012; Nowrotek et al., 2019; Moralez et al., 2021).
Whole metagenome DNA sequencing can describe the genomes of the total microbial community found in nature. This approach enables the study of culturable and non-culturable bacteria by bypassing the need for isolation and laboratory cultivation of microorganisms. DNA directly isolated from the environmental sample can broaden the understanding of the structure, gene/species richness and distribution, and the functional and metabolic potential of a microbial community (Suenaga, 2012).
Applying this approach to antibiotic resistance in different microbial communities can identify known and novel resistance genes and mobile genetic elements, i.e., plasmids, integrons, transposons, and phage’s (Partridge et al., 2018; Stalder et al., 2019; Hall et al., 2022; Yao et al., 2022). This information is the stepping stone to making new policies for infection and prevention control measures, thereby reducing the incidence of infection and optimizing the use of antibiotics by health professionals, in the healthcare, animal, and agricultural industries. In addition, it will provide improved awareness and understanding of antibiotic resistance as a whole.
Unfortunately, AMR monitoring systems focus on clinical and the public health sectors as a representation of AMR as a whole. Organizations such as WHO and the implementation of the Global Antimicrobial Surveillance System (GLASS) have relied on the use of traditional culturable methods for clinical AMR research. This creates a biased outlook on AMR as non-clinical sectors, and non-culturable bacteria are excluded. This review will focus on AMR in non-clinical sectors and the use of bioinformatics as an aid in this research.
DNA sequencing technologies used in antibiotic resistance research
Microbiomes are complex and require sequencing technologies to be comprehensive enough to capture the present representative sequences from species. Several sequencing platforms can be used to study AMR in clinical and non-clinical sectors. They are useful in detecting antibiotic resistance genes (ARGs), virulence factors, MGEs, and diversity of the microbial community (Forbes et al., 2017). The advancements in metagenomics have been driven by next-generation sequencing technologies (NGS) i.e., second and third-generation sequencing technologies (Table 1).
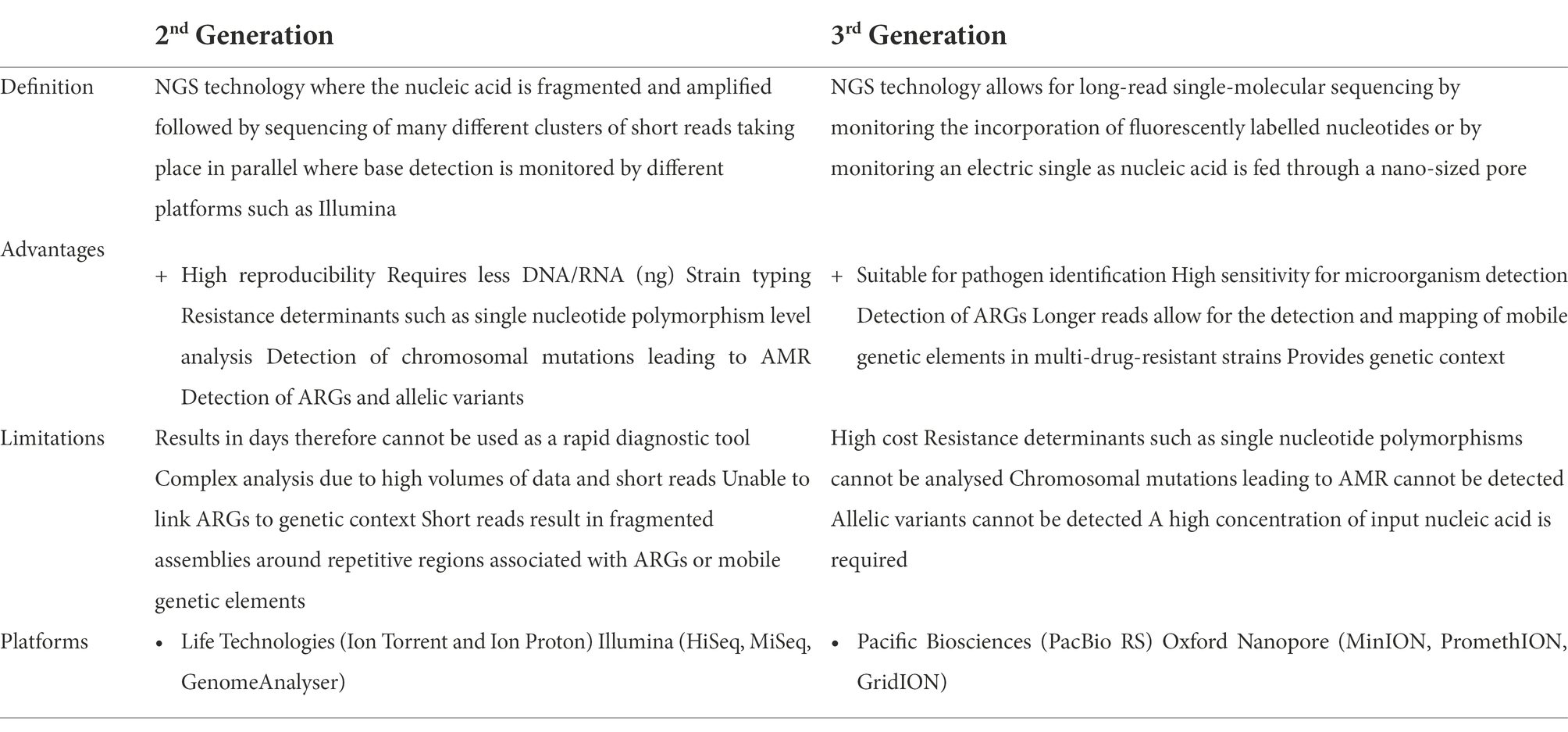
Table 1. Second-generation versus third-generation sequencing technologies for antibiotic susceptibility testing.
Second-generation sequencing has been the most widely used sequencing technology for microbial genomics. Second-generation sequencing technologies, i.e., Illumina and Ion Torrent, produce short reads from 100 up to 300 base pairs with high accuracy (~0.1% error rate; De Maio et al., 2019; Yee and Simner, 2019). These short reads provide information on species population, evolutionary relationships, allelic variations, SNPs, and the detection of specific genes, i.e., ARGs (Boolchandani et al., 2019; De Maio et al., 2019; Yee and Simner, 2019). Although short reads provide information on the genomic content of the bacterial isolates in a sample, it does not provide information on the genomic structure, i.e., which genes are present on chromosomes and MGEs such as plasmids which are crucial in understanding the dissemination of ARGs (George et al., 2017; De Maio et al., 2019; Yee and Simner, 2019; Maguire et al., 2020).
Third-generation technologies overcome this limitation by providing a better view of the genetic structure as longer reads (on average 1–100 Kbp) span most of the repetitive sequences, linking ARGs to MGEs, e.g., plasmids (Boolchandani et al., 2019; Yee and Simner, 2019). This long-read technology is advantageous for studying AMR in bacterial isolates, and metagenomic samples as the complexity of assembly is reduced (De Maio et al., 2019). Unfortunately, the high error rate makes it difficult to identify specific allelic variants or SNPs in chromosomal genes leading to AMR (Yee and Simner, 2019). On the other hand, Pacbio and ONT are easily accessible and able to produce raw data in real-time, making them a good tool for the rapid diagnostics (Che et al., 2019). Long reads are also a practical method to determine the species/gene richness, distribution, and functional potential of a microbial community (Garmendia et al., 2012; Crofts et al., 2017).
While third-generation technologies are successful in AMR research, it is limited by the high error rate and low accuracy (Yee and Simner, 2019). To overcome this, a combination of short-reads (i.e., Illumina) with long-reads (i.e., ONT) is a promising way to generate fully resolved and accurate bacterial genome assemblies which characterize antibiotic resistance genes on plasmids and genomes from environmental samples (Liu et al., 2020; Chen et al., 2021; Moralez et al., 2021). This combination allows for better assembly of complex genomes as long reads provide information on the structure of the genome, and short reads can be used to correct errors in long reads (De Maio et al., 2019).
These sequencing technologies directly characterize the microbiomes in humans, animals, and environmental samples, providing high-throughput sequencing of either whole genomes, targeted amplicons, or whole metagenomes (Di Bella et al., 2013).
Whole-genome sequencing (WGS) uses DNA sequencing technologies to sequence the entire genome of isolated pure organisms to characterize genomic variants (Table 2; Collineau et al., 2019). WGS overcomes the limitations of traditional culture-dependent approaches and phenotypic tests, i.e., disk diffusion for AMR (Balloux et al., 2018). By opting to use WGS, more information on the bacterial genetic determinants conveying resistance and their association with mobile genetic elements can be established (Balloux et al., 2018; Oniciuc et al., 2018). This contributes to outbreak detection, infection control, and epidemiological surveillance, all of which are an essential part of the AMR surveillance (Collineau et al., 2019).
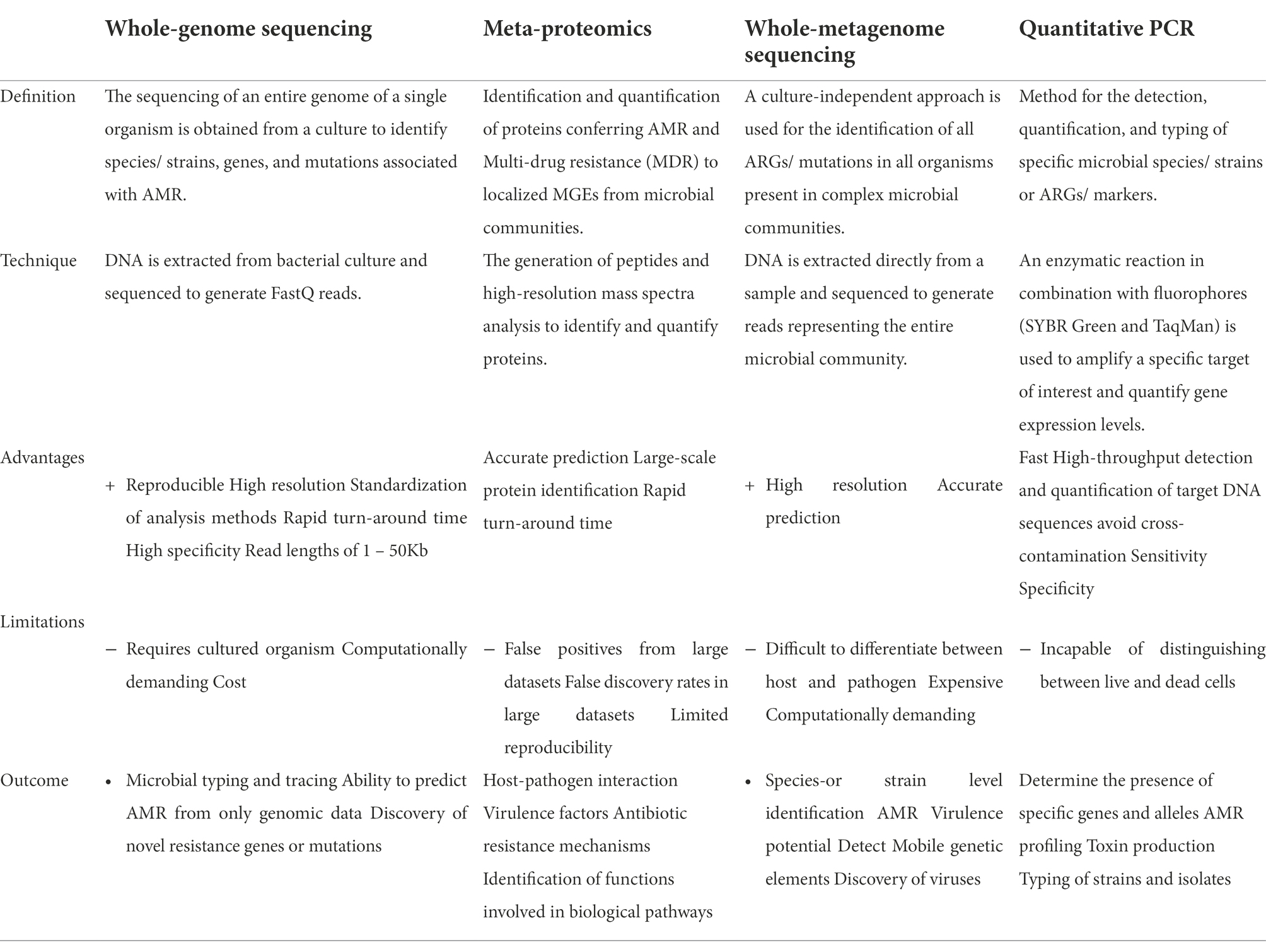
Table 2. Metagenomic sequencing vs. other techniques used to study qualitatively and quantitatively antibiotic resistance.
Amplicon sequencing uses marker genes such as 16S (prokaryotes) or 18S rRNA (eukaryotes) genes. Both approaches require the DNA extracted directly from the microbial community and are subjected to either direct sequencing or amplification via polymerase chain reaction (PCR; Table 2). In both instances, the sequences are queried against a reference database (Table 3). This is a reliable process to identify the composition of a microbial community down genus-level within a sample (Ranjan et al., 2016; Forbes et al., 2017; Oniciuc et al., 2018).
Metagenomic sequencing involves the fragmentation, sequencing, assembly, and annotation of the total genomic DNA isolated in a given sample (Oniciuc et al., 2018; Xu et al., 2021). Similar to WGS, whole metagenome sequencing (WMS) can provide information on the entire gene content of either prokaryotic and eukaryotic organisms as well as species or strain-level identification and virulence or resistance potential (Ranjan et al., 2016; Quainoo et al., 2017; Hendriksen et al., 2019). In addition, WMS makes it possible to predict the metabolic potential of the microbial community. This comprehensive view of all gene content allows access to information on AMR that is essential for setting up control and prevention strategies in all sectors (Xu et al., 2021).
Meta-proteomics focuses on functional change in a microbiome. Assisting in the control strategies and plays a key role in identifying molecular mechanisms of bacterial pathogenesis and disease outcome determinants. It can aid in developing pathogen-specific treatment strategies that can lower the spread of AMR. Meta-proteomics is reliable for reviewing bacteria in soil, sludge, food, and the ocean (Wilmes and Bond, 2004; Bastida et al., 2014; Williams and Cavicchioli, 2014; Maier et al., 2017). Unfortunately, there are inconsistent protocols for sample preparation, inefficient bioinformatic tools, and challenges in measuring low-abundance proteins within a complex protein sample (Zhang et al., 2018). This technique, while reliable, should be applied with other sequencing technologies to have an in-depth analysis of microbial communities, antibiotic resistance genes, and host-pathogen interaction (Zhang and Figeys, 2019; Khodadadi et al., 2020).
Bioinformatic analysis of metagenomic sequences for antibiotic resistance research
The constant development of new computational pipelines is advantageous in providing an accurate depiction of the resistome from metagenomic sequencing data. These analyses rely on a variety of algorithms: quality control of DNA sequencing data, genome and metagenome assembly algorithms, read-mapping, variant detection, phylogenetics, taxonomic databases, and visualization (McArthur and Tsang, 2017). AMR databases are discussed in more detail in the next section.
The typical process to identify antibiotic resistance genes from sequenced metagenomes is based on one of two general techniques: (i) read-mapping and (ii) assembly. Each of these could be followed by binning or an annotation step (Table 4). A bioinformatics pipeline for analysing metagenomic data for antibiotic resistance research has been presented in Figure 1.
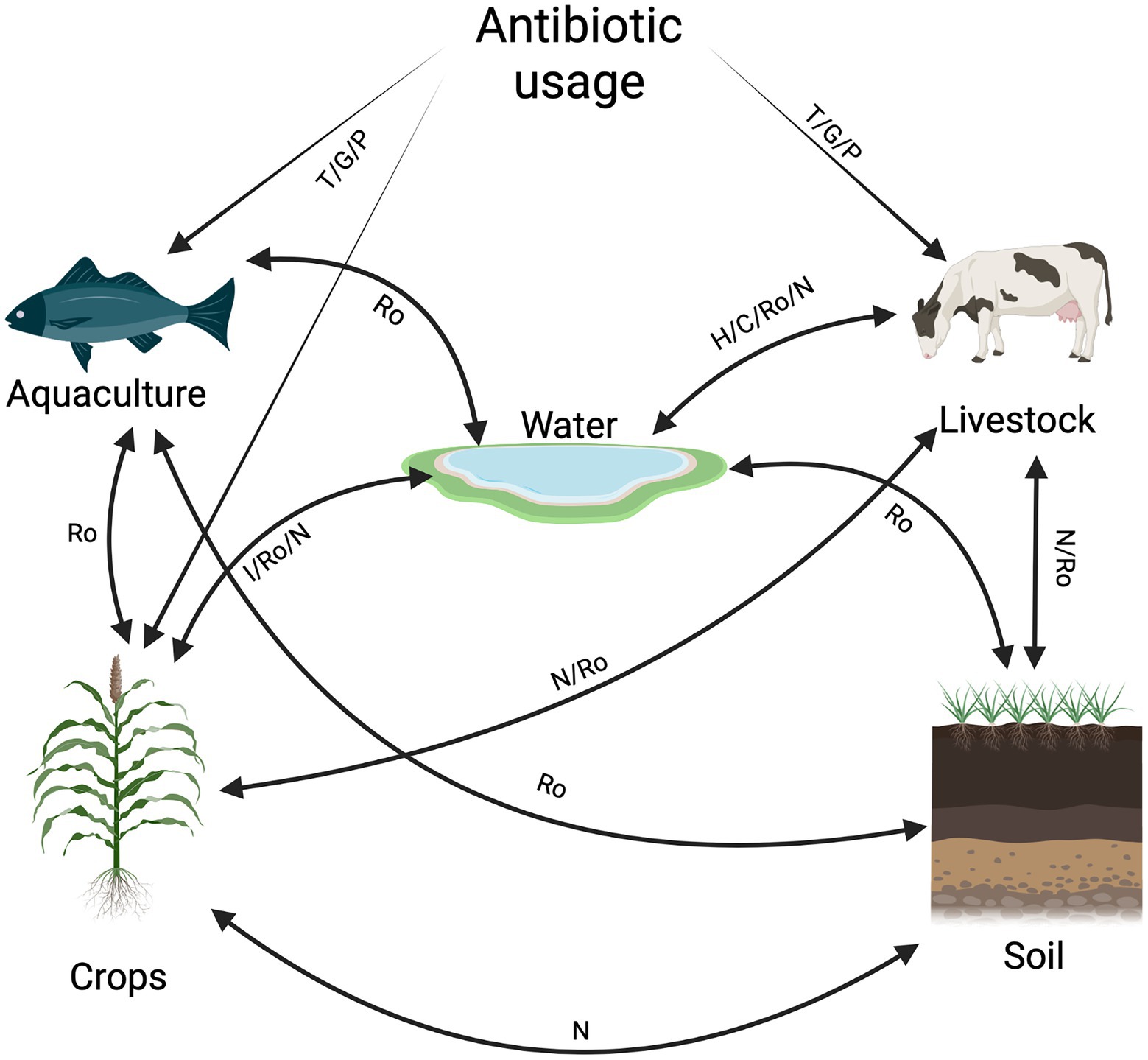
Figure 1. Pathway map of antibiotic agents and antibiotic resistance dissemination between livestock, agriculture, aquaculture, and water. The movement of antibiotic resistance or antibiotic agents is indicated by solid lines. The potential routes of transmission and exposure between non-clinical sectors and antibiotic usage is indicated by the abbreviations: Ro: run-off /leaching, T: treatment, P: prevention, GP: growth promoter, H: animal house, C: cleaning, N: nutrients, I: irrigation (Created with BioRender.com). Routes between non-clinical sectors (↔) and antibiotic usage in non-clinical sectors (→) have been described by the previous studies mentioned. Water ↔ crop: (Hu et al., 2010; Gatica and Cytryn, 2013; Meena et al., 2015; Bürgmann et al., 2018; Rodríguez-Molina et al., 2019), water ↔ soil: (Hu et al., 2010; Seiler and Berendonk, 2012; Michael et al., 2013; Meena et al., 2015; Tang, 2016), water ↔ livestock: (Miyata et al., 2011; Hao et al., 2014; Van Boeckel et al., 2015; Magouras et al., 2017; Madec and Haenni, 2018; McEwen and Collignon, 2018; Opatowski et al., 2021), water ↔ aquaculture: (Seiler and Berendonk, 2012; Shah et al., 2014; Grenni et al., 2018; Pérez-Sánchez et al., 2018; Yuan et al., 2019; Preena et al., 2020a,b; Reverter et al., 2020; Sonone et al., 2020), antibiotic use → aquaculture: (Shah et al., 2014; Pérez-Sánchez et al., 2018; Stärk et al., 2019; Preena et al., 2020b), antibiotic use → livestock: (Ahmed et al., 2007; Marshall and Levy, 2011; Landers et al., 2012; Hao et al., 2014; Speksnijder et al., 2015; Van Boeckel et al., 2017; McEwen and Collignon, 2018), antibiotic use → crops: (Hirniesen et al., 2012; Pan and Chu, 2017; Sundin and Wang, 2018; Zhu et al., 2019; Gao, 2021), livestock ↔ soil and crops: (Popowska et al., 2012; Wang et al., 2015; Lin et al., 2016; Chen et al., 2017; Xie et al., 2018; Pérez-Valera et al., 2019; Zhang et al., 2019).
Read mapping allows for raw read sequences to be directly aligned to reference databases (Table 3) using pairwise tools such as MiniMap2 (Li, 2018), Bowtie2 (Langmead and Salzberg, 2012), Burrows-wheeler aligner (BWA; Langmead and Salzberg, 2012), or by splitting reads into k-mers and mapping to a reference database using k-mer alignment (KMA; Clausen et al., 2016; Figure 2). This provides information on the similarity of one sequence to another by counting the number of alignments to quantify the abundance of similar sequence patterns (Clausen et al., 2016).
In the last decade, read-mapping approaches were deemed superior to assembly approaches for AMR gene detection, i.e., reads are first assembled and mapped to a database using BLAST (Johnson et al., 2008; Hunt et al., 2017; Clausen et al., 2018). The success of an assembly-based approach is highly dependent on the quality of the assembly (Inouye et al., 2014; Clausen et al., 2016). An approach as such is problematic as genes of interest, i.e., ARG, s can be split over two or more contigs and will not be identified if the assembly is poor quality (Clausen et al., 2016, 2018).
Read-mapping based methods overcome this by using the tools above, which allow for fast mapping and alignment of raw reads against large reference genomes and entire databases (Clausen et al., 2016). This can identify genes from low abundance organisms in a complex community with speed and ease of computation. Since epidemiological databases are constantly being updated with new sequences due to natural evolution, the read-mapping approach becomes difficult as results constantly change. There is no guarantee that the read will cover a unique part of the reference sequence and will result in a tie for the best match due to the random selection (Clausen et al., 2016). Fortunately, tools like SRST2 (Inouye et al., 2014) resolve the ties by pre-and post-processing of sequences for read-mapping, which can predict the presence of ARGs in a sample (Clausen et al., 2018; Taş et al., 2021).
Identification and characterization of ARGs can also be achieved using assembly-based approaches (Table 4; Figure 2; Breitwieser et al., 2019). This method allows reads to be assembled into contigs and then queried against reference databases (Table 3). The assembly of WMS data is complicated as there is an uneven or unknown abundance of different genomes (Ghurye et al., 2016).
Unrelated genomes may also contain nearly identical DNA repeats, which could represent MGEs (Boolchandani et al., 2019). In addition, multiple individual organisms could be from the same species but may harbour small genetic differences indicating strain variants (Ghurye et al., 2016). Technical factors, e.g., library preparation, sequencing depth, and sequencing platforms, affect the accuracy in assembling WMS data into larger contigs (Escobar-Zepeda et al., 2015). Metagenomic assemblers such as MEGAHIT (Li et al., 2015), MetaSPades (Nurk et al., 2017), IDBA-UD (Peng et al., 2012), and MetaVelvet (Namiki et al., 2012) have been developed to overcome these challenges by normalizing sequencing depth, correcting read errors, and detecting and reporting genomic variants and repeats (Ghurye et al., 2016; Vosloo et al., 2021). To date, no single assembler is the best at accurately reconstructing known genomes and capturing taxonomic diversity in a metagenomic sample. Multiple assemblers are recommended to be applied to a subset of samples to determine which has the best fit (Escobar-Zepeda et al., 2015; Ghurye et al., 2016; Boolchandani et al., 2019).
Binning classification can predict the taxonomical composition using information contained in reads (Figure 2). Reads are grouped to represent an individual genome or closely related genomes (Table 4). Binning can be done either with reads or assembled sequences and employs two strategies to obtain taxonomic assignment; (i) sequence composition classification and (ii) sequence alignment against references. The first is based on looking at genomic signatures using k-mers to identify evolutionary conservation among species and uses software such as TETRA (Teeling et al., 2004), MetaClusterTA (Wang et al., 2014), and PhylophytiaS (McHardy et al., 2007). Similarly, software like MaxBin (Wu et al., 2016) and Amphora2 (Wu and Scott, 2012) use k-mer signatures but also considers gene markers, GC content, and coverage information for binning on assembled sequences or reads. Another method based on reference read alignment is the Burrows-Wheeler Transform (Burrows and Wheeler, 1994) which indexes like BWA (Li and Durbin, 2009) or Bowtie (Langmead and Salzberg, 2012) and is fast and accurate in assessing species richness and abundance in WMS by aligning them to reference genomes. Genometa (Davenport and Tümmler, 2013) is a software that looks at OTUs in the WMS data and groups according to genomic islands and operons. Taxonomic classification in long reads using the potential coding regions to search in annotated protein databases using BLAST (Johnson et al., 2008) or Megan (Huson et al., 2007). Binning achieved after the assembly of contigs can lead to the generation of partial genomes of unknown or uncultured organisms. This can be used to perform similarity-based binning of other metagenomic datasets. If binning is achieved before assembly, it can reduce the complexity of an assembly-based approach and may reduce the computational demands. However, caution should be taken to ensure the validity of the genome bins as there is a chance of false assignments (Thomas et al., 2012; Bengtsson-Palme, 2018a). In this instance, CheckM (Parks et al., 2015) can be used to evaluate the quality of bins on metagenomic samples and assess the quality of assembled metagenomic genomes by estimation of completeness and contamination based on marker-gene validation (Du and Sun, 2022).
Taxonomic assignments of reads can also be done with genome annotation. This process identifies the coding regions and their location to determine gene function. Annotation of metagenomic sequences can be done in two ways, (i) existing pipelines, e.g., RAST (Aziz et al., 2008) and IMG (Markowitz et al., 2009), can be used for assembled genomes, and (ii) annotation can be performed on an entire community and relies on unassembled reads and short contigs. WMS data is annotated by identifying genes (feature prediction) and assigning putative gene functions and taxonomic neighbours (functional annotation; Thomas et al., 2012). Prediction tools such as MetaGeneAnnotator (Noguchi et al., 2008) or MetaGeneMark use internal information to classify sequences as either coding or non-coding and are done with a low error rate of 2% (Thomas et al., 2012).
Antibiotic resistance databases
The performance of antibiotic resistance gene prediction and taxonomic identification depends on the availability of accurate databases. Two types of public databases exist, generalized AMR databases, which include a wide range of ARGs and mechanistic information, and specialized databases, which provide extensive information on specific gene families, e.g., the β-lactamase family (Hendriksen et al., 2019).
Several bioinformatic tools and databases can be used in AMR research. They focus on fast and reliable predictions of ARB and their genetic determinants in complex communities (Van Camp et al., 2020). It is important to note that the quality and type of sequence play an essential role in AMR research. The bioinformatics tool’s robustness can differ when analysing sequences of low-quality (Hendriksen et al., 2019). Any database’s value depends on maintenance, curation, and continuous updates with detailed metadata. Correctly predicting ARGs from large-scale datasets with improved accuracy depends on input parameters such as e-values, bit scores, identity, and query coverage levels (Lal Gupta et al., 2020).
General antibiotic resistance databases
General antibiotic resistance databases incorporate a wide variety of ARGs and mechanistic information.
Established tools like ResFinder (Zankari et al., 2012) accept short reads and contigs to detect the presence of acquired ARGs. This can be done for complete or partial genome sequences and uses BLAST (Johnson et al., 2008), or KMA (Clausen et al., 2018) approaches (Zankari et al., 2012; Table 3). The specificity of using BLAST to detect antibiotic resistance genes depends on the selection criteria for gene length and percentage similarity. This method requires computational expertise and could be challenging to identify multiple copies of ARGs in de novo assembled genomes (Clausen et al., 2018; Hameed and Fatima, 2021). This can be solved by using longer reads which increases the cost.
In comparison, the KMA approach was developed to map the raw reads against redundant AMR databases as the traditional BLAST method remains too slow to map raw reads directly (Clausen et al., 2018; Hendriksen et al., 2019). This approach provides accurate bacterial genome analysis and can identify ARGs present in low abundance, which might be excluded in incomplete assemblies. There is also a chance of false positives due to sequencing errors which can be overcome by setting the minimum threshold for the number of reads needed for a positive outcome (Hendriksen et al., 2019; Hameed and Fatima, 2021).
The Comprehensive Antibiotic Resistance Database (CARD; Jia et al., 2016) for the identification of resistance genes, their products, and associated phenotypes. This database allows for the identification of chromosomal mutations that confer resistance to antibiotics. Similar to ResFinder (Zankari et al., 2012), the percentage coverage and identity can be adjusted to allow for the selection of new ARGs. The Resistance Gene Identifier (RGI), a tool for de novo annotation of genes, complete genomes, or genome assembled sequences for AMR, uses CARD’s curated database to predict ARGs in either DNA or amino acid format. This is because some sequences cannot be mapped due to divergence in the nucleotide sequences; however, amino acid sequences in this instance can be translated to proteins to characterize the resistome (Xavier et al., 2016; Lakin et al., 2019). CARD uses two prediction models: a protein homolog model based on functional resistance homologs and a protein variant model that detects mutations conferring resistance (Jia et al., 2016; Imchen et al., 2020). The application of ontologies such as CARD’s Antibiotic Resistance Ontology (ARO) has organized AMR information based on molecular determinants, resistance mechanisms, individual antimicrobials, antimicrobial targets, and drug classes, therefore, making this database favourable (McArthur and Tsang, 2017).
Other curated databases include; MEGARes (Lakin et al., 2017), ResFams (Gibson et al., 2015), NDARO (Sayers et al., 2021), FARME (Wallace et al., 2017), and MUSTARD (Ruppé et al., 2019), all of which are curated for the detection of AMR determinants in large metagenomic datasets (Arango Argoty, 2019; Arango-Argoty et al., 2020; Imchen et al., 2020; Lal Gupta et al., 2020). Interestingly, FARME, a functional antibiotic resistance metagenomic element database, focuses on AMR gene elements from environmental samples (soil, faecal matter, wastewater treatment plants, oral and aquatic biomes) rather than individual ARGs obtained from cultured clinical isolates, providing more access and analysis of non-clinical sectors. This database is compiled from publicly available DNA sequences from 30 functional metagenomic projects and their corresponding predicted proteins conferring AMR (Wallace et al., 2017). FARME contains over seven times the number of non-redundant protein sequences as compared to CARD (Wallace et al., 2017; Lal Gupta et al., 2020). It provides information on regulatory elements, MGEs, and predicted proteins flanking ARGs, which are conserved between functional metagenomic AMR sequences from soil biomes and pathogenic clinical isolates (Forsberg et al., 2012; Wallace et al., 2017). This allows for better insight into AMR in unculturable bacteria found in non-clinical settings (Wallace et al., 2017; Bengtsson-Palme, 2018b; Imchen et al., 2020).
ResFams is a curated database of protein families linked to their profile Hidden Markov Models (HMMs) associated with AMR function are trained using unique AMR protein sequences obtained from CARD, LaCED, and Lahey databases (Lal Gupta et al., 2020). This platform primarily focuses on pathogen-associated ARGs and provides a comprehensive view of resistomes in the environment and the evolution of resistant pathogens (Lal Gupta et al., 2020). ResFams were evaluated with functional metagenomic datasets and demonstrated improved sensitivity, and identified 64% more ARGs in soil and human gut microbiomes compared to BLAST-based searches of CARD and ARDB databases. This increased sensitivity is due to the HMM-based analysis. HMM, models are specific models constructed based on observed sequence variation across genes/protein families and capture possible variation for the families (Xavier et al., 2016). Although this approach is sensitive and can detect distant matches that BLAST-based approaches cannot, ResFams is computationally expensive and requires the user to provide local computational resources to run HMM-based searches (Xavier et al., 2016; Lakin et al., 2019).
Specific antibiotic resistance databases
Specific databases are created to meet specific needs and provide extensive information about a specific gene family. So far, only specialized databases are available for β-lactamase.
The β-lactamase database (BLAD) is a catalogue of resistance patterns from all classes of β-lactamases collected from NCBI and published data with crystal structures of proteins from the Protein Data Bank (PDB; Berman et al., 2000). Metagenomic sequences can be queried against BLAD to obtain basic information about β-lactamases, search for the gene of interest, identify resistance patterns and search and analyse the 3D structure of the β-lactamase (Danishuddin et al., 2013; Naas et al., 2017).
The CBMAR β-lactamase database (Srivastava et al., 2014) includes detailed biochemical and molecular information to understand known and unknown β-lactamase genes. This provides a cache of information on nucleotides, 3D protein structures, sequence alignments, and mutation profiles.
Similarly, LacED (Thai et al., 2009) is a specialized platform that contains mutational and structural data for TEM and SHV β-lactamases. This is extremely important to understand the evolution of multidrug-resistant pathogens as β-lactamase evolution, and the emergence of new enzymes affects the now available treatment for infections. Furthermore, these resistant genes are present on plasmids which help their spread to different biomes, challenging the treatment options in both clinical and non-clinical sectors (Dandachi et al., 2019).
Antibiotic resistance in non-clinical sectors
AMR is a natural phenomenon that predates the use of antibiotics. A wide array of novel and clinically characterized ARGs have been detected in environmental samples ranging from pristine environments to agricultural soil (Yang et al., 2019). The major driving force of AMR is the mis-use of antibiotics while other factors such as poor infrastructure, i.e., hygiene and sanitation play a role in maintaining AMR (Collignon et al., 2018; Essack and Sartorius, 2018). Approximately more than 60% of total antibiotic use occurs outside the field of human medicine (Prestinaci et al., 2015; Kumar et al., 2020; Polianciuc et al., 2020). The main non-clinical sectors that are involved in the development of AMR are animal production (food-animals and aquaculture), agriculture (plants), and the environmental (water and soil) compartment. These sectors are interconnected and facilitate the spread of pathogenic ARB within and between them and ultimately to humans. Table 5 summarizes the findings done on AMR in various non-clinical reservoirs through the use of metagenomics.
Food-animals
Each year, half of the antibiotics produced are used for industrial farm animal production (Schmieder and Edwards, 2012). The global demand for animal protein has directly driven the use of antibiotics in livestock. The food-animal industry is a hotspot for antibiotic usage, estimated to increase by 67% in highly populated countries (Van Boeckel et al., 2015). Antibiotics are administered as metaphylactic and prophylactic treatment for the whole flock or herd to prevent the spread of infectious diseases. Furthermore, it is used as a growth-promoting agent across the globe (Van Boeckel et al., 2015; Founou et al., 2016; Robinson et al., 2016).
Historically, the negative effects of antibiotic usage in livestock production have been overlooked because of the drive to keep up with consumer demand (Robinson et al., 2016; Ramtahal et al., 2022). This has led to the prolonged use and dosage, creating an ideal condition for the emergence of ARB and ARGs (Marshall and Levy, 2011; Madec and Haenni, 2018).
AMR can spread through direct and indirect contact, which was confirmed by finding the same ARBs in the poultry farm and the caretaker (Wee et al., 2020). Antibiotics and their residues can be found in most connecting areas such as soil and plants, and animal products which can lead to health risks when consumed due to the direct toxicity, allergic reactions, carcinogenic effects, and disturbance of the beneficial microbiota in children, those who are pregnant, the elderly, and people living with immunocompromised people (Oliveira et al., 2020).
Areas such as the EU and United States have made efforts to ban the use of antibiotics as growth enhancers. However, they are still widely used in South Africa, Brazil, India, China, and Russia (Van Boeckel et al., 2015; Robinson et al., 2016). This strategy is based on the assumption that susceptible strains can outnumber AMR microorganisms if the advantage of becoming resistant is decreased (Andersson and Hughes, 2011). This was supported by a post-ban study conducted in Denmark and Norway. Interestingly, even though ARB decreased, the broilers that were studied were still colonized by ARB years after the ban came into effect. It is such that the ban on antibiotics is still up for debate as decreasing antibiotic use could increase the frequency of bacterial infections (Holmes et al., 2016; Andersson and Hughes, 2019).
The World Health Organization has recommended that farmers and the food industry stop using antibiotics for the growth and prevention of disease in healthy animals. Farms should consider the employment of proper preventive measures and farming practices to limit the risk of bacterial infections instead of the routine antibiotic dosing of animals to prevent disease. If this is done correctly, we could see the decreased use of antibiotics and the alleviation of (Marshall and Levy, 2011; Robinson et al., 2016; Aslam et al., 2018; Van Gompel et al., 2020).
Water
Water represents one of the most important bacterial habitats and is a major pathway and reservoir for disseminating microorganisms and AMR. This is due to the spread of AMR by humans, and animals, or contamination of the environment by pathogens (Hutton and Chase, 2017; Miłobedzka et al., 2021).
Grey water
Wastewater treatment plants (WWTPs) collect chemical pollutants, including antibiotics, which are disposed of from households, hospitals, and factories. The WWTP aims to remove contaminants before disposing water in natural environments, e.g., streams, rivers, and lakes. While the reuse and reclamation of water through WWTPs reduce water shortage, they assist in the spread and emergence of AMR (Michael et al., 2013). This impacts the economy and society of countries affected by AMR (Bürgmann et al., 2018; Prüss-Ustün et al., 2019). It could be possible that proper sanitation and safe drinking water in the high-, middle-and low-income countries affect the emergence and spread of AMR. Although the removal of contaminants is successful, some ARB are not removed by the WWTP and are released with the effluent, which can spread to other natural environments (Meena et al., 2015).
Since WWTPs receive a diverse range of ARB and their genes from different sources. This ARB accumulates in the WWTP, and these high concentrations provide a selective pressure that facilitates the emergence of new ARB strains (Bürgmann et al., 2018). This is seen in wastewater treatment that uses biological treatment processes such as activated sludge, creating a favourable environment for ARB, ARGs, and their transmission (Guo et al., 2017). Contradictorily, it was hypothesized that the WWTP is efficient in removing ARBs which was seen in a study of the Dutch (Pallares-Vega et al., 2019). It could be argued that AMR in WWTP decreased since the Netherlands decreased antibiotic use as a whole. While this can be observed, antibiotic residues are not fully removed from the WWTP and persist in lower concentration when released with the effluent which serves as a selection pressure for ARBs (Keen and Fugère, 2017). WWTPs should revise their treatment options to manage AMR concerns while also providing sanitary water to communities. Policies about sludge and biosolid disposals are needed along with better infrastructure which will help decrease the spread of AMR to other sectors (Bürgmann et al., 2018; Kraemer et al., 2019).
Blue/green water
Freshwater environments are among the natural environments susceptible to contamination with antibiotics. These are released through different sources such as agricultural run-off, sewage discharge, and leaching from farms (Hutton et al., 2007; Hutton and Chase, 2017). The combination of antibiotics with a high density of bacteria provides a favourable environment for the development of ARGs (Marti et al., 2014). ARB carrying resistance genes can persist in freshwater and ultimately return to humans and animals by horizontal gene transfer (HGT; Chen et al., 2017; Mirza et al., 2020). Freshwater serves as a source of drinking water, recreational purposes and for agricultural practices, which use 70% of all freshwater, e.g., aquifers, streams, and lakes (Bürgmann et al., 2018). Continuous use of freshwater encourages the spread of AMR and the emergence of new pathogens, which leads to an increased risk of infection and prolonged and untreatable infections in humans, animals, and the environment (Nnadozie and Odume, 2019).
To date (Table 5), there is evidence showing that the consumption of contaminated water poses health risks (Jjemba et al., 2010; Shi et al., 2013; Sanganyado and Gwenzi, 2019) and that the WWTP has the potential to contribute to the dissemination of AMR in receiving rivers (Tang et al., 2016; Guo et al., 2017). Unfortunately, information on antibiotics in streams, lakes, beaches, pond water, surface and groundwater, and drinking water is scarce as it is presumed that antibiotic concentrations are naturally low in these areas. However, low antibiotic concentrations still select for ARB (Nnadozie and Odume, 2019). Studies also focus on ARB and genes at discharge points, i.e., receiving river or effluent, of the WWTP. At this point, the concentration of AMR is high but declines further away from the WWTP, downstream, which could be a result of degradation through biological or non-biological processes, uptake by aquatic microorganisms through HGT, diluted, absorption onto particulate matter, or transportation into different water systems (Anyaduba, 2016; Mirza et al., 2020). More focus should be on AMR within each freshwater environment, its link to different sectors, AMR after the WWTP discharge points and in potable water to gain a more holistic view (Mirza et al., 2020).
Aquaculture
Aquaculture refers to the breeding, rearing, and harvesting of aquatic organisms in different water environments. This term is commonly used to describe fish farming. Aquaculture contributes to more than half of the world’s seafood consumption and production and has increased by 6% a year since 2001 (Rocha et al., 2022). The aquacultural sector is a complex interconnected system influenced by the environment, cultural, human, and economic factors (Brunton et al., 2019; Schar et al., 2021).
Like livestock production, antibiotics are used in aquaculture as a therapeutic agent to prevent and treat bacterial outbreaks. High concentrations are used due to high stocking densities, and the lack of individual treatment (Yukgehnaish et al., 2020).
Antibiotics are mixed with feed before being administered to animals or can be directly applied to the aquatic environment. This leads to the dispersal and leaching of antibiotics into the environment (Brunton et al., 2019). The gut microbiota of fish is also affected by the continuous use of antibiotics which alter the benefit of host-microbiota relationships allowing the gut microbiota to become resistant to antibiotics that are administered (Tyagi et al., 2019). Aquaculture is also affected by toxic materials such as silver and mercury, which co-resist and co-regulate with antibiotics. These toxic materials accumulate in fish bodies and the aquatic food web. This toxicity can be passed to humans and animals through consumption. It can affect the nervous system leading to death, as discussed by (Sonone et al., 2020), who reviewed the various heavy metal sources, i.e., agricultural activities, electronic waste, mining, industrial effluents, power plants, and biomedical waste and its role in degrading the aquaculture population, causing physical deformities in organisms and polluting the aquatic environment.
Several studies (Table 5) have shown that the excess use of antibiotics has led to the emergence of AMR. It cannot be ignored that the aquaculture industry is integrated with sewage, industrial wastewater, and land agriculture as manure and other agricultural residues are used in fish food. Areas in Western Europe and North America have banned antibiotics as a growth promoter, but it is still used as a therapeutic agent in fish food. Since 70 to 80% of antibiotics given to fish are excreted into the water, the entire body of water is exposed. This leads to leaching into different water environments and sediments, which provide long-term selection pressure in the aquatic environment, favoring horizontal gene transfer and the spread of AMR, as discussed by (Taylor et al., 2011; Watts et al., 2017; Mirza et al., 2020). Such policies on aquacultural practices should be reviewed to decrease the incidence of ARB and infections (Marti et al., 2014; Pérez-Sánchez et al., 2018; Preena et al., 2020a; Zhao et al., 2020).
Soil
Microbes in soil are of great importance as they break down organic matter, recycle nutrients, bioremediation, and produce antibiotics (Chandra and Kumar, 2017). Variation in biotic, e.g., plants, animals and other bacteria, and abiotic, e.g., water, soil and atmosphere, conditions of soil cause the residing microbes to adapt and develop strategies for survival and successful reproduction. Antibiotic production is the most powerful adaptation strategy from soil microbes to inhibit the growth of competing microbes (Chandra and Kumar, 2017). This strategy has led to the natural development of AMR. Antibiotics and disinfectants used in medicine, agriculture, and aquaculture have been the driver of AMR through manure/fertiliser application on soil, irrigation, and run-off. Consequences of these are the emergence of new ARGs and the spread of pathogenic ARB to other environments, humans, and animals (Martínez, 2008; Wang et al., 2015; Chandra and Kumar, 2017).
Although AMR research has shown that WWTP effluents can still contain a high level of ARGs and bacteria after disinfection, there is not much research on the sludge and biosolids applied to soil and how this changes the bacterial community and resistome (Anyaduba, 2016; Nnadozie and Odume, 2019). The application of manure/fertiliser/sludge to soil should also be looked into to determine if there is any difference in soil and crop quality as they are used in agricultural and remediation practices. Studies have shown the prevalence of AMR in soil and how bioremediation and pollutants have facilitated its spread (Table 5).
Plants
The agricultural industry has been valued at an estimated US$ 3.2 trillion worldwide and accounts for the largest share of the GDP and employment in developing countries and underdeveloped nations. Unfortunately, the agriculture industry has been suffering due to population growth, pest resistance, and the burden of natural resources (Raman, 2017).
Manure is commonly used as fertilizer on vegetable farmlands to enhance crop yield. This enhances AMR as 30–90% of antibiotics consumed by animals are excreted in faeces which is used as manure. Using sewage sludge and water for irrigation contributes to rising AMR in agriculture (Minden et al., 2017; Grenni et al., 2018; Spielmeyer, 2018). Crops can take up antibiotics through water transport and passive absorption (Hu et al., 2010). Antibiotic-resistant bacteria found in soil can reach the interior of fruits and vegetables either through germination seeds in contaminated soil or the direct transmission of bacteria from the soil to the plant via irrigation. Both these routes can cause the bacteria to colonize in the roots and the edible parts of the plant (Hirniesen et al., 2012; Alegbeleye et al., 2018; Sundin and Wang, 2018).
These edible plants are consumed by animals or sold as ready-to-eat products such as bagged salads or pre-cut vegetables, which may enhance the proliferation and survival of ARB (Jung et al., 2014; Rajwar et al., 2016; Wadamori et al., 2017; Mir et al., 2018). This is due to many factors such as hygiene standards, irrigation water, temperature, storage, pH, soil, manure, and antimicrobial agents. Since these products rarely go through a heating step before consumption, it increases the risk of consumers being exposed to high numbers of different pathogenic ARB (Mir et al., 2018). This poses a threat to society as consumers who opt for ready-to-eat products are faced with the risk of acquiring foodborne illnesses which could be untreatable with antibiotics. Table 5 summarizes studies showing that manure, fertilizers, compost, and irrigation are all routes for the transmission and dissemination of AMR to plants.
Antibiotic resistance and its transmission
The use and misuse of antibiotics in humans, animals, and the environment have been linked to the emergence of ARB in each of these sectors. Antibiotic residues, ARB, and ARGs, are spread to different environments (Miłobedzka et al., 2021). Most antibiotics are excreted in animal urine and faeces (Jayalakshmi et al., 2017). This is introduced to the environment directly by manure amendment, which provides nutrients for crops, or through water which is the primary link between humans, animals, and nature (Miłobedzka et al., 2021).
Since antibiotics are used in the food animal industry, ARB can spread via run-off, which can go into lakes, streams, and rivers. Water from these areas is used for irrigation for agricultural practices. This leads to the spread of ARB from food animals to water, soil, and crops (Figure 1). This interconnectedness facilitates the exchange of AMR and allows for the emergence of new genetic determinants. This emergence and spread can occur by HGT (Schmieder and Edwards, 2012; Pan and Chu, 2017; Sarkar et al., 2018; Mahmood et al., 2019).
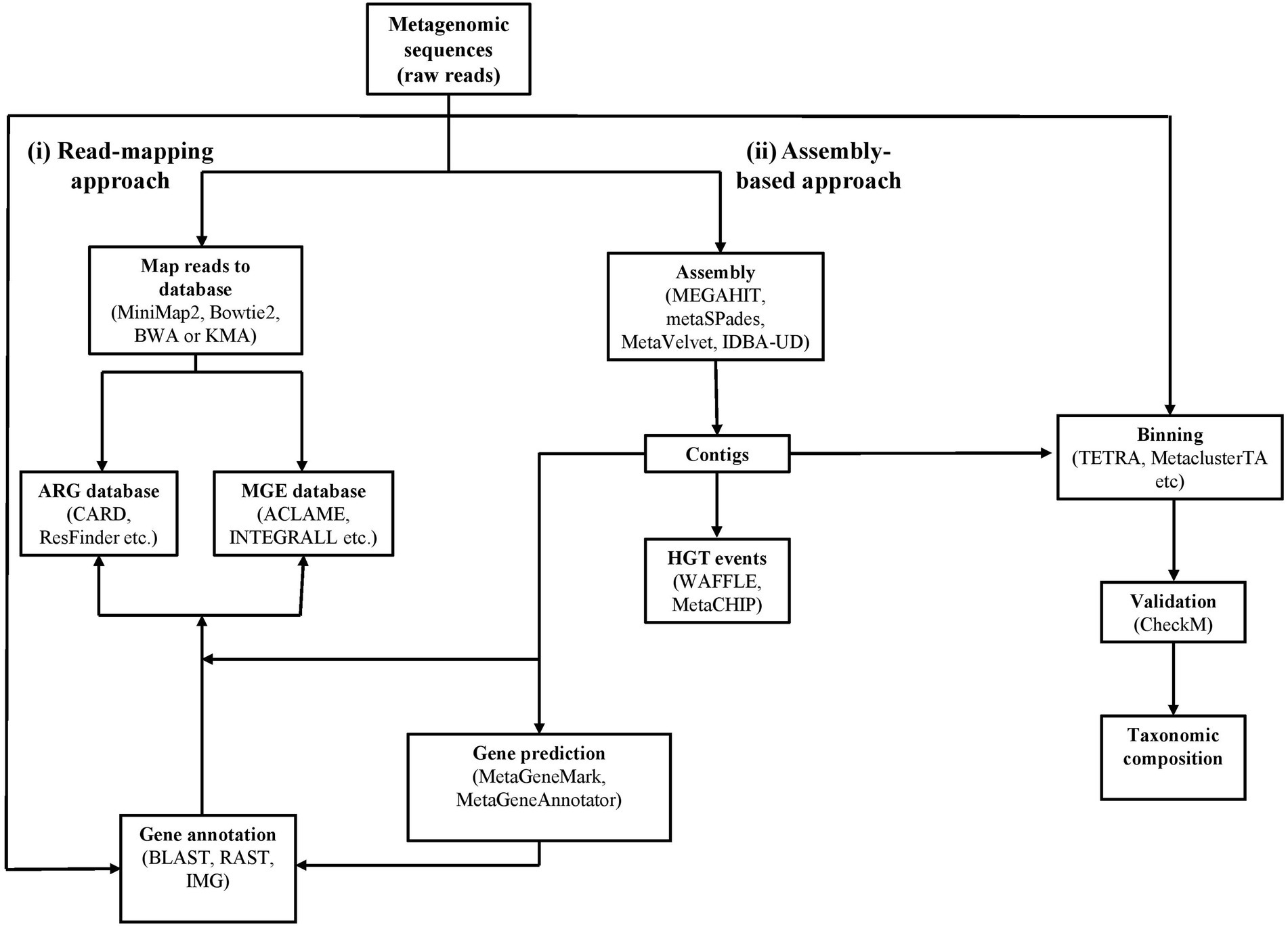
Figure 2. Workflow for determining antibiotic resistance genes, mobile genetic elements, horizontal gene transfer events and taxonomic composition using metagenomic data. The workflow is indicated by solid lines with example tools and databases annotated in the boxes. The complete lists are described in Tables 3, 4.
Horizontal gene transfer
ARB exists across animal, human and environmental sectors. The evolution of AMR and the dynamics of ARGs spread across these sectors are critical for predicting emerging pathogens and controlling AMR dissemination. HGT is primarily responsible for rapid AMR spread and dissemination of ARGs between bacteria and across species (Sun et al., 2020; Vinayamohan et al., 2022). AMR can be established either vertically by point mutations or horizontally by acquiring MGEs such as plasmids and transposons (Vinayamohan et al., 2022).
HGT is a process that introduces variation in the bacterial genome under natural selection. It is an essential process that allows bacteria to adapt to environmental changes such as exposure to antibiotics (Hall et al., 2020).
HGT predates the production and use of antibiotics. Unfortunately, the increased usage of antibiotics has put selective pressure on bacterial strains. This has sped up the process of bacteria becoming antibiotic-resistant by various genotypic and phenotypic resistance mechanisms. This diversification can be seen in the resistance to antibiotics of different classes (Power, 2019).
Genes that confer resistance can either be intrinsic or extrinsic (Ali et al., 2018). Intrinsic resistance is the bacterium’s innate ability to resist the antimicrobial agent’s activity. This occurs through structural or functional characteristics. Extrinsic or acquired resistance occurs when a previously susceptible bacterium obtains the ability to resist the activity of the antimicrobial agent. This can result from the mutation of genes involved in normal physiological processes, the acquisition of foreign resistance genes, or a combination of both (Blair et al., 2015; von Wintersdorff et al., 2016; Kumar, 2017).
Pathogenic bacteria can acquire resistance via HGT. This has caused AMR to spread from commensal and environmental bacteria to pathogenic ones. ARGs are often carried in MGEs, e.g., plasmids, transposons, or integrons which act as vectors for transferring genetic information between bacterial cells. The three classical pathways of HGT are conjugation, transformation, and transduction. These HGT mechanisms lead to increased bacteria fitness, which is essential for survival in the presence of antibiotics (Capita and Alonso-Calleja, 2013; Munita and Arias, 2016; von Wintersdorff et al., 2016; Kumar, 2017; Vinayamohan et al., 2022).
Recent studies have identified other mechanisms by which DNA can transfer between hosts. Gene transfer agents are DNA-containing particles, similar to phages, but cannot carry genes for particle production (Redfield and Soucy, 2018). Genes can also be transferred between bacteria that form intercellular connections by nanotubes or membrane fusion (Hall et al., 2017, 2020). Some bacteria can also release DNA-containing membrane-bound vesicles that carry genetic information to new hosts. Interestingly, some of these mechanisms are not under bacterial control but are controlled by semi-autonomous segments of DNA (Hall et al., 2017).
Bioinformatic approaches such as MetaCHIP (Song et al., 2019) and WAAFLE (WAAFLE, 2022) can be used to detect HGT events in metagenomic sequences. These processes involve an all-against-all BLASTN or BLASTX of genes within assembled contigs, and potential HGT events are determined based on genes with the best hits in other taxonomic groups (Douglas and Langille, 2019). These approaches are limited by sequencing technologies and assemblers, which often fail to assemble long regions with high sequence similarity (Song et al., 2019). It can be assumed that inferring HGT events will become easier as the assembly of metagenomic sequences improves (Douglas and Langille, 2019).
Mobile genetic elements
Specialized MGEs mediate HGT between bacteria. These play an important role in bacterial ecology and evolution. Several factors impact HGT in bacteria, such as gene expression, protein connectivity, and biochemical properties; however, the range of genes carried on MGEs remains unknown (Rodríguez-Beltrán et al., 2020).
MGEs are segments of DNA that encode enzymes and other proteins that mediate the movement of DNA within or between bacterial cells (Hall et al., 2017; Emamalipour et al., 2020). MGEs carry genes other than those necessary for transfer and replication (Hall et al., 2017). These accessory genes do not play a role in their vertical or horizontal transmission but affect the success of MGEs. The range of accessory genes encoded by MGEs and their ability to be phenotypically expressed in different genetic backgrounds are critical in its evolution (Rodríguez-Beltrán et al., 2020).
The most important elements that play a role in HGT are the conjugative and mobilized elements. Mobilized elements contain all genetic information required to transfer from one bacterium to another, while conjugative elements use conjugation functions of elements such as plasmids and transposons to transfer from one host to another. These MGEs can carry multi-drug resistant (MDR) plasmids and give rise to MDR bacteria via HGT (Vinayamohan et al., 2022). Bacteriophages also play a role in the spread of DNA by the transduction process. Some elements can translocate to new sites on a genome but cannot transfer to a new bacterial host. These include the transposons and mobile integrons (Van Hoek et al., 2011; Hall et al., 2017).
Detecting mobile genetic elements
The ability of MGEs containing ARGs to spread is controlled by the genetic elements and host factors. The genetic elements and host factors control the ability of MGEs containing ARG, but most important is the selective pressure in the environment. When antibiotics are present in the environment, there is strong selective pressure on the spread of resistance. Elements that promote resistance will be selected for, and those stopping the spread of mobile elements will be selected against (von Wintersdorff et al., 2016; Hall et al., 2017; Partridge et al., 2018). To control the spread of AMR, it is important to understand MGEs and the ecology of the environments in which they spread easily (Van Hoek et al., 2011; Slizovskiy et al., 2020).
Several databases such as ACLAME (Leplae et al., 2004, 2010), ISFinder (Siguier et al., 2006), INTEGRALL (Moura et al., 2009), and ICEberg (Liu et al., 2019) and web-based tools such as ISSaga2 (Varani et al., 2011), MobilomeFinder (Ou et al., 2007), oriTfinder (Li et al., 2018), TnpPred (Riadi et al., 2012) and MobileElementFinder (Johansson et al., 2021) were developed to identify MGEs in metagenomic datasets (Peng et al., 2021). These web-based tools and databases range from detecting plasmids, integrons, transposons, prophages, and insertion sequences.
Overall, there is no single solution for detecting MGEs as these databases, and web-based tools are incomplete and biased towards pathogens studied extensively. This makes it difficult and time-consuming as most web-based tools and databases need to be evaluated first and used in combination to provide an all-inclusive view on MGEs associated with AMR (Rankin et al., 2011; Brito, 2021). This information can be applied to clinical and non-clinical sectors to potentially influence policies and strategies which can help control the spread of AMR (Sun et al., 2020).
Outlook
Antibiotic resistance affects animals, plants, the environment and human health (Malagón-Rojas and Lagos, 2020). Monitoring and understanding the prevalence, mechanisms and spread of AMR is a priority in non-clinical sectors (Boolchandani et al., 2019). Since measures against AMR are focused on the clinical health sector, the environment is under-represented which allows for significant gaps in data, research and control strategies therefore failing to link the effects of AMR from one sector to the other (Malagón-Rojas and Lagos, 2020).
Metagenomic sequencing allows for the detection of known and novel ARGs, HGT events, and associated MGEs in complex communities providing in-depth information on their prevalence, distribution, and transmission in non-clinical sectors such as the animal industry, agriculture, and the environment (Forbes et al., 2017).
While metagenomics is promising, its implementation in non-clinical sectors is still in its early stages. Metagenomic sequencing creates a vast amount of data and requires specialized bioinformatic expertise, which makes this a costly approach (Forbes et al., 2017; Oniciuc et al., 2018). To date, only a few countries and laboratories have the resources and expertise to use metagenomic approaches as a surveillance system for AMR. In addition, bioinformatics methodologies need to be standardized and constantly updated and curated to allow for an accurate comparison between various samples, as the selection of bioinformatic tools and AMR databases does have a significant impact on the results (Hendriksen et al., 2019; Lal Gupta et al., 2020).
Nevertheless, using bioinformatic tools and databases can assist in identifying risky practices, effects of antibiotic usage, and hotspots of AMR. This can facilitate the design of new policies to control the spread of AMR between clinical and non-clinical sectors (Shen and Zhao, 2018; Nijsingh et al., 2019). Such knowledge and practices are urgent as these sectors, including the public health sector, are faced with a future of untreatable infections, increasingly costly medicinal treatment, a higher cost of living, and an increased mortality rate (Bengtsson and Greko, 2014; Littmann et al., 2015; Nijsingh et al., 2019).
Conclusion
The application of metagenomic and bioinformatic approaches to AMR research can provide fast and reliable predictions of AMR and antibiotic use in various non-clinical sectors. These hold a great promise for understanding AMR molecularly, predicting outbreaks and transmission, and emerging pathogens. This information can lead to better policymaking in each sector and decrease the incidence of infections as conditions for the animal industry, agriculture, and the environmental sectors are improved.
Author contributions
SP and TA conceived, designed and wrote the manuscript. AU and DC-F edited and proof-read the manuscript. All authors contributed to the article and approved the submitted version.
Funding
This work is based on the research supported by wholly/in part by the National Research Foundation of South Africa (Grant Numbers: 120192).
Conflict of interest
The authors declare that the research was conducted in the absence of any commercial or financial relationships that could be construed as a potential conflict of interest.
Publisher’s note
All claims expressed in this article are solely those of the authors and do not necessarily represent those of their affiliated organizations, or those of the publisher, the editors and the reviewers. Any product that may be evaluated in this article, or claim that may be made by its manufacturer, is not guaranteed or endorsed by the publisher.
References
Ahmed, A. M., Motoi, Y., Sato, M., Maruyama, A., Watanabe, H., Fukumoto, Y., et al. (2007). Zoo animals as reservoirs of gram-negative bacteria harboring integrons and antimicrobial resistance genes. Appl. Environ. Microbiol. 73, 6686–6690. doi: 10.1128/AEM.01054-07
Alegbeleye, O. O., Singleton, I., and Sant Ana, A. S. (2018). Sources and contamination routes of microbial pathogens to fresh produce during field cultivation: a review. Food Microbiol. 73, 177–208. doi: 10.1016/j.fm.2018.01.003
Ali, A. S., Al-Qahtani, A., Nabi, S., Altaf, K., Sonekhi, G. B., Ahmed, J., et al. (2018). A short review on antibiotics and ever-changing microbial resistance mechanisms. British Journal of Pharmacy. 3:1. doi: 10.5920/bjpharm.2018.XX
Alneberg, J., Bjarnason, B. S., De Bruijn, I., Schirmer, M., Quick, J., Ijaz, U. Z., et al. (2014). Binning metagenomic contigs by coverage and composition. Nat. Methods, 1144–1146. doi: 10.1038/nmeth.3103
Altschul, S. F., Gish, W., Miller, W., Myers, E. W., and Lipman, D. J. (1990). Basic local alignment search tool. J. Mol. Biol. 215, 403–410. doi: 10.1016/S0022-2836(05)80360-2
Andersson, D. I., and Hughes, D. (2011). Persistence of antibiotic resistance in bacterial populations. FEMS Microbiol. Rev. 35, 901–911. doi: 10.1111/j.1574-6976.2011.00289.x
Andersson, D. I., and Hughes, D. (2019). Selection and transmission of antibiotic-resistant bacteria. Microbial Transmission. 35, 117–137.
Arango Argoty, G. A. (2019). Computational tools for annotating antibiotic resistance in metagenomic United States: Data: Virginia Tech.
Arango-Argoty, G., Guron, G., Garner, E., Riquelme, M. V., Heath, L., Pruden, A., et al. (2020). ARGminer: a web platform for the crowdsourcing-based curation of antibiotic resistance genes. Bioinformatics 36, 2966–2973. doi: 10.1093/bioinformatics/btaa095
Aslam, B., Wang, W., Arshad, M. I., Khurshid, M., Muzammil, S., Rasool, M. H., et al. (2018). Antibiotic resistance: a rundown of a global crisis. Infection and drug resistance. 11:1645. doi: 10.2147/IDR.S173867
Aziz, R. K., Bartels, D., Best, A. A., DeJongh, M., Disz, T., Edwards, R. A., et al. (2008). The RAST server: rapid annotations using subsystems technology. BMC Genomics 9, 1–15. doi: 10.1186/1471-2164-9-75
Balloux, F., Brynildsrud, O. B., Van Dorp, L., Shaw, L. P., Chen, H., Harris, K. A., et al. (2018). From theory to practice: translating whole-genome sequencing (WGS) into the clinic. Trends Microbiol. 26, 1035–1048. doi: 10.1016/j.tim.2018.08.004
Bankevich, A., Nurk, S., Antipov, D., Gurevich, A. A., Dvorkin, M., Kulikov, A. S., et al. (2012). SPAdes: a new genome assembly algorithm and its applications to single-cell sequencing. J. Comput. Biol. 19, 455–477. doi: 10.1089/cmb.2012.0021
Bastida, F., Hernández, T., and García, C. (2014). Metaproteomics of soils from semiarid environment: functional and phylogenetic information obtained with different protein extraction methods. J. Proteome 101, 31–42. doi: 10.1016/j.jprot.2014.02.006
Bengtsson, B., and Greko, C. (2014). Antibiotic resistance—consequences for animal health, welfare, and food production. Ups. J. Med. Sci. 119, 96–102. doi: 10.3109/03009734.2014.901445
Bengtsson-Palme, J. (2018a). Strategies for taxonomic and functional annotation of metagenomes. Netherlands: Metagenomics: Elsevier. p. 55–79.
Bengtsson-Palme, J. (2018b). The diversity of uncharacterized antibiotic resistance genes can be predicted from known gene variants—but not always. Microbiome. 6:125. doi: 10.1016/B978-0-08-102268-9.00003-3
Berman, H. M., Westbrook, J., Feng, Z., Gilliland, G., Bhat, T. N., Weissig, H., et al. (2000). The protein data bank. Nucleic Acids Res. 28, 235–242. doi: 10.1093/nar/28.1.235
Blair, J. M., Webber, M. A., Baylay, A. J., Ogbolu, D. O., and Piddock, L. J. (2015). Molecular mechanisms of antibiotic resistance. Nat. Rev. Microbiol. 13, 42–51. doi: 10.1038/nrmicro3380
Boolchandani, M., D’Souza, A. W., and Dantas, G. (2019). Sequencing-based methods and resources to study antimicrobial resistance. Nat. Rev. Genet. 20, 356–370. doi: 10.1038/s41576-019-0108-4
Breitwieser, F. P., Lu, J., and Salzberg, S. L. (2019). A review of methods and databases for metagenomic classification and assembly. Brief. Bioinform. 20, 1125–1136. doi: 10.1093/bib/bbx120
Brito, I. L. (2021). Examining horizontal gene transfer in microbial communities. Nat. Rev. Microbiol. 19, 442–453. doi: 10.1038/s41579-021-00534-7
Brunton, L. A., Desbois, A. P., Garza, M., Wieland, B., Mohan, C. V., Häsler, B., et al. (2019). Identifying hotspots for antibiotic resistance emergence and selection, and elucidating pathways to human exposure: application of a systems-thinking approach to aquaculture systems. Sci. Total Environ. 687, 1344–1356. doi: 10.1016/j.scitotenv.2019.06.134
Bürgmann, H., Frigon, D., Gaze, H., W, M., Manaia, C., Pruden, A., et al. (2018). Water and sanitation: an essential battlefront in the war on antimicrobial resistance. FEMS Microbiol. Ecol. 94:fiy101. doi: 10.1093/femsec/fiy101
Burrows, M., and Wheeler, D. Technical report 124. Palo Alto, CA: Digital Equipment Corporation. (1994).
Cabello, F. C., and Godfrey, H. P. (2016). Even therapeutic antimicrobial use in animal husbandry may generate environmental hazards to human health. Environ. Microbiol. 18, 311–313. doi: 10.1111/1462-2920.13247
Capita, R., and Alonso-Calleja, C. (2013). Antibiotic-resistant bacteria: a challenge for the food industry. Crit. Rev. Food Sci. Nutr. 53, 11–48. doi: 10.1080/10408398.2010.519837
CDC. Gov. list of selected multistate foodborne outbreak investigations. (2021). Available from: http://www.cdc.gov/foodsafety/outbreaks/multistate-outbreaks/outbreaks-list.html.
Chandra, N., and Kumar, S. (2017). “Antibiotics producing soil microorganisms” in Antibiotics and antibiotics resistance genes in soils. eds. H. Z. Muhammad, S. Vladimir, and A. Varma (Berlin: Springer), 1–18.
Che, Y., Xia, Y., Liu, L., Li, A., Yang, Y., and Zhang, T. (2019). Mobile antibiotic resistome in wastewater treatment plants revealed by Nanopore metagenomic sequencing. Microbiome. 7:44. doi: 10.1186/s40168-019-0663-0
Chen, Q.-L., An, X.-L., Li, H., Zhu, Y.-G., Su, J.-Q., and Cui, L. (2017). Do manure-borne or indigenous soil microorganisms influence the spread of antibiotic resistance genes in manured soil? Soil Biol. Biochem. 114, 229–237. doi: 10.1016/j.soilbio.2017.07.022
Chen, Q.-L., An, X.-L., Zheng, B.-X., Ma, Y.-B., and Su, J.-Q. (2018). Long-term organic fertilization increased antibiotic resistome in phyllosphere of maize. Sci. Total Environ. 645, 1230–1237. doi: 10.1016/j.scitotenv.2018.07.260
Chen, Y.-H., Chiang, P.-W., Rogozin, D. Y., Degermendzhy, A. G., Chiu, H.-H., and Tang, S.-L. (2021). Salvaging high-quality genomes of microbial species from a meromictic lake using a hybrid sequencing approach. Communications Biology. 4:996. doi: 10.1038/s42003-021-02510-6
Chen, B., He, R., Yuan, K., Chen, E., Lin, L., Chen, X., et al. (2017). Polycyclic aromatic hydrocarbons (PAHs) enriching antibiotic resistance genes (ARGs) in the soils. Environ. Pollut. 220, 1005–1013. doi: 10.3389/fmicb.2017.01133
Chen, Z., Yu, D., He, S., Ye, H., Zhang, L., Wen, Y., et al. (2017). Prevalence of antibiotic-resistant Escherichia coli in drinking water sources in Hangzhou City. Front. Microbiol. 8:1133.
Clausen, P. T. L. C., Aarestrup, F. M., and Lund, O. (2018). Rapid and precise alignment of raw reads against redundant databases with KMA. BMC Bioinformatics. 19:307. doi: 10.1186/s12859-018-2336-6
Clausen, P. T. L. C., Zankari, E., Aarestrup, F. M., and Lund, O. (2016). Benchmarking of methods for identification of antimicrobial resistance genes in bacterial whole genome data. J. Antimicrob. Chemother. 71, 2484–2488. doi: 10.1093/jac/dkw184
Collignon, P., Beggs, J. J., Walsh, T. R., Gandra, S., and Laxminarayan, R. (2018). Anthropological and socioeconomic factors contributing to global antimicrobial resistance: a univariate and multivariable analysis. The Lancet Planetary Health. 2, e398–e405. doi: 10.1016/S2542-5196(18)30186-4
Collineau, L., Boerlin, P., Carson, C. A., Chapman, B., Fazil, A., Hetman, B., et al. (2019). Integrating whole-genome sequencing data into quantitative risk assessment of foodborne antimicrobial resistance: a review of opportunities and challenges. Front. Microbiol. 10:1107. doi: 10.3389/fmicb.2019.01107
Crofts, T. S., Gasparrini, A. J., and Dantas, G. (2017). Next-generation approaches to understand and combat the antibiotic resistome. Nat. Rev. Microbiol. 15:422. doi: 10.1038/nrmicro.2017.28
Dandachi, I., Chaddad, A., Hanna, J., Matta, J., and Daoud, Z. (2019). Understanding the epidemiology of multi-drug resistant gram-negative bacilli in the Middle East using a one health approach. Front. Microbiol. 10, 1–39. doi: 10.3389/fmicb.2019.01941
Danishuddin, M., Hassan Baig, M., Kaushal, L., and Khan, A. U. (2013). BLAD: a comprehensive database of widely circulated beta-lactamases. Bioinformatics 29, 2515–2516. doi: 10.1093/bioinformatics/btt417
Davenport, C. F., and Tümmler, B. (2013). Advances in computational analysis of metagenome sequences. Environ. Microbiol. 15, 1–5. doi: 10.1111/j.1462-2920.2012.02843.x
Davies, J., and Davies, D. (2010). Origins and evolution of antibiotic resistance. Microbiol. Mol. Biol. Rev. 74, 417–433. doi: 10.1128/MMBR.00016-10
De Maio, N., Shaw, L. P., Hubbard, A., George, S., Sanderson, N. D., Swann, J., et al. (2019). Comparison of long-read sequencing technologies in the hybrid assembly of complex bacterial genomes. Microbial genomics. 5:294. doi: 10.1099/mgen.0.000294
Di Bella, J. M., Bao, Y., Gloor, G. B., Burton, J. P., and Reid, G. (2013). High throughput sequencing methods and analysis for microbiome research. J. Microbiol. Methods 95, 401–414. doi: 10.1016/j.mimet.2013.08.011
Donato, J. J., Moe, L. A., Converse, B. J., Smart, K. D., Berklein, F. C., McManus, P. S., et al. (2010). Metagenomic analysis of apple orchard soil reveals antibiotic resistance genes encoding predicted bifunctional proteins. Appl. Environ. Microbiol. 76, 4396–4401. doi: 10.1128/AEM.01763-09
Douglas, G. M., and Langille, M. G. (2019). Current and promising approaches to identify horizontal gene transfer events in metagenomes. Genome Biol. Evol. 11, 2750–2766. doi: 10.1093/gbe/evz184
Du, Y., and Sun, F. (2022). HiCBin: binning metagenomic contigs and recovering metagenome-assembled genomes using hi-C contact maps. Genome Biol. 23:63. doi: 10.1186/s13059-022-02626-w
Dušan, J., Jasper, L., Anna, Z., and Otto, C. (2016). Antimicrobial resistance—a threat to the world’s sustainable development. Ups. J. Med. Sci. 121, 159–164. doi: 10.1080/03009734.2016.1195900
Eckstrom, K., Barlow, J. W., and Chang, Y.-F. (2019). Resistome metagenomics from plate to farm: the resistome and microbial composition during food waste feeding and composting on a Vermont poultry farm. PLoS One 14:e0219807-e. doi: 10.1371/journal.pone.0219807
Emamalipour, M., Seidi, K., Zununi Vahed, S., Jahanban-Esfahlan, A., Jaymand, M., Majdi, H., et al. (2020). Horizontal gene transfer: from evolutionary flexibility to disease progression. Frontiers in Cell and Developmental Biology. 8:229. doi: 10.3389/fcell.2020.00229
Escobar-Zepeda, A., Vera-Ponce de León, A., and Sanchez-Flores, A. (2015). The road to metagenomics: from microbiology to DNA sequencing technologies and bioinformatics. Front. Genet. 6:348. doi: 10.3389/fgene.2015.00348
Essack, S. Y., and Sartorius, B. (2018). Global antibiotic resistance: of contagion, confounders, and the COM-B model. The Lancet Planetary Health. 2, e376–e377. doi: 10.1016/S2542-5196(18)30187-6
Fair, R. J., and Tor, Y. (2014). Antibiotics and bacterial resistance in the 21st century. Perspectives in medicinal chemistry. 6:PMCS14459. doi: 10.4137/PMC.S14459
Forbes, J. D., Knox, N. C., Ronholm, J., Pagotto, F., and Reimer, A. (2017). Metagenomics: the next culture-independent game changer. Front. Microbiol. 8:1069. doi: 10.3389/fmicb.2017.01069
Forsberg, K. J., Reyes, A., Wang, B., Selleck, E. M., Sommer, M. O. A., and Dantas, G. (2012). The shared antibiotic Resistome of soil bacteria and human pathogens. Science 337, 1107–1111. doi: 10.1126/science.1220761
Founou, L. L., Founou, R. C., and Essack, S. Y. (2016). Antibiotic resistance in the food chain: a developing country-perspective. Front. Microbiol. 7:1881. doi: 10.3389/fmicb.2016.01881
Fresia, P., Antelo, V., Salazar, C., Giménez, M., D’Alessandro, B., Afshinnekoo, E., et al. (2019). Urban metagenomics uncover antibiotic resistance reservoirs in coastal beach and sewage waters. Microbiome. 7, 1–9. doi: 10.1186/s40168-019-0648-z
Friedman, N. D., Temkin, E., and Carmeli, Y. (2016). The negative impact of antibiotic resistance. Clin. Microbiol. Infect. 22, 416–422. doi: 10.1016/j.cmi.2015.12.002
Gandra, S., Barter, D., and Laxminarayan, R. (2014). Economic burden of antibiotic resistance: how much do we really know? Clin. Microbiol. Infect. 20, 973–980. doi: 10.1111/1469-0691.12798
Gao, C. (2021). Genome engineering for crop improvement and future agriculture. Cells 184, 1621–1635. doi: 10.1016/j.cell.2021.01.005
Garmendia, L., Hernandez, A., Sanchez, M., and Martinez, J. (2012). Metagenomics and antibiotics. Clin. Microbiol. Infect. 18, 27–31. doi: 10.1111/j.1469-0691.2012.03868.x
Garner, E., Chen, C., Xia, K., Bowers, J., Engelthaler, D. M., McLain, J., et al. (2018). Metagenomic characterization of antibiotic resistance genes in full-scale reclaimed water distribution systems and corresponding potable systems. Environ. Sci. Technol. 52, 6113–6125. doi: 10.1021/acs.est.7b05419
Gatica, J., and Cytryn, E. (2013). Impact of treated wastewater irrigation on antibiotic resistance in the soil microbiome. Environ. Sci. 20, 3529–3538. doi: 10.1007/s11356-013-1505-4
George, S., Pankhurst, L., Hubbard, A., Votintseva, A., Stoesser, N., Sheppard, A. E., et al. (2017). Resolving plasmid structures in Enterobacteriaceae using the MinION nanopore sequencer: assessment of MinION and MinION/Illumina hybrid data assembly approaches. Microbial genomics. 3:118. doi: 10.1099/mgen.0.000118
Ghurye, J. S., Cepeda-Espinoza, V., and Pop, M. (2016). Focus: microbiome: metagenomic assembly: overview, challenges and applications. Yale J. Biol. Med. 89:353.
Gibson, M. K., Forsberg, K. J., and Dantas, G. (2015). Improved annotation of antibiotic resistance determinants reveals microbial resistomes cluster by ecology. ISME J. 9, 207–216. doi: 10.1038/ismej.2014.106
Gigante, V., Sati, H., and Beyer, P. (2022). Recent advances and challenges in antibacterial drug development. ADMET and DMPK. 10, 147–151. doi: 10.5599/admet.1271
Glass, E. M., Wilkening, J., Wilke, A., Antonopoulos, D., and Meyer, F. (2010). Using the metagenomics RAST server (MG-RAST) for analyzing shotgun metagenomes. Cold Spring Harbor Protocols. 2010:pdb prot5368.
Grenni, P., Ancona, V., and Barra, C. A. (2018). Ecological effects of antibiotics on natural ecosystems: a review. Microchem. J. 136, 25–39. doi: 10.1016/j.microc.2017.02.006
Guo, J., Li, J., Chen, H., Bond, P., and Yuan, Z. (2017). Metagenomic analysis reveals wastewater treatment plants as hotspots of antibiotic resistance genes and mobile genetic elements. Water Res. 123, 468–478. doi: 10.1016/j.watres.2017.07.002
Gupta, A., and Gupta, B. (2021). Silent slow pandemic of antimicrobial resistance, vol. 8, 354–355. doi: 10.18231/j.ijca.2021.067
Hall, J. P., Brockhurst, M. A., and Harrison, E. (2017). Sampling the mobile gene pool: innovation via horizontal gene transfer in bacteria. Philosophical Transactions of the Royal Society B: Biological Sciences. 372:20160424. doi: 10.1098/rstb.2016.0424
Hall, J. P., Harrison, E., and Baltrus, D. A. (2022). Introduction: the secret lives of microbial mobile genetic elements. The Royal Society. 377:20200460. doi: 10.1098/rstb.2020.0460
Hall, R. J., Whelan, F. J., McInerney, J. O., Ou, Y., and Domingo-Sananes, M. R. (2020). Horizontal gene transfer as a source of conflict and cooperation in prokaryotes. Front. Microbiol. 11:1569. doi: 10.3389/fmicb.2020.01569
Hameed, S., and Fatima, Z. (2021). Integrated Omics approaches to infectious diseases. Berlin: Springer.
Hao, H., Cheng, G., Iqbal, Z., Ai, X., Hussain, H. I., Huang, L., et al. (2014). Benefits and risks of antimicrobial use in food-producing animals. Front. Microbiol. 5:288. doi: 10.3389/fmicb.2014.00288
Hendriksen, R. S., Bortolaia, V., Tate, H., Tyson, G. H., Aarestrup, F. M., and McDermott, P. F. (2019). Using genomics to track global antimicrobial resistance. Frontiers. Public Health 7, 1–17. doi: 10.3389/fpubh.2019.00242
Hirniesen, K., Sharma, M., and Kniel, K. (2012). Human enteric pathogen internalization by root uptake into food crops. Foodborne Pathog. Dis. 9, 396–405. doi: 10.1089/fpd.2011.1044
Holmes, A. H., Moore, L. S. P., Sundsfjord, A., Steinbakk, M., Regmi, S., Karkey, A., et al. (2016). Understanding the mechanisms and drivers of antimicrobial resistance. Lancet 387, 176–187. doi: 10.1016/S0140-6736(15)00473-0
Hu, X., Zhou, Q., and Luo, Y. (2010). Occurrence and source analysis of typical veterinary antibiotics in manure, soil, vegetables and groundwater from organic vegetable bases, northern China. Environ. Pollut. 158, 2992–2998. doi: 10.1016/j.envpol.2010.05.023
Hunt, M., Mather, A. E., Sánchez-Busó, L., Page, A. J., Parkhill, J., Keane, J. A., et al. (2017). ARIBA: rapid antimicrobial resistance genotyping directly from sequencing reads. Microbial genomics. 3:131. doi: 10.1099/mgen.0.000131
Huson, D. H., Auch, A. F., Qi, J., and Schuster, S. C. (2007). MEGAN analysis of metagenomic data. Genome Res. 17, 377–386. doi: 10.1101/gr.5969107
Hutton, G., Haller, L., and Bartram, J. (2007). Global cost-benefit analysis of water supply and sanitation interventions. J. Water Health 5, 481–502. doi: 10.2166/wh.2007.009
Imchen, M., Moopantakath, J., Kumavath, R., Barh, D., Tiwari, S., Ghosh, P., et al. (2020). Current trends in experimental and computational approaches to combat antimicrobial resistance. Front. Genet. 11:563975. doi: 10.3389/fgene.2020.563975
Imelfort, M., Parks, D., Woodcroft, B. J., Dennis, P., Hugenholtz, P., and Tyson, G. W. (2014). GroopM: an automated tool for the recovery of population genomes from related metagenomes. PeerJ. 2:e603. doi: 10.7717/peerj.603
Inouye, M., Dashnow, H., Raven, L.-A., Schultz, M. B., Pope, B. J., Tomita, T., et al. (2014). SRST2: rapid genomic surveillance for public health and hospital microbiology labs. Genome Med. 6:90. doi: 10.1186/s13073-014-0090-6
Jayalakshmi, K., Paramasivam, M., Sasikala, M., Tamilam, T., and Sumithra, A. (2017). Review on antibiotic residues in animal products and its impact on environments and human health. J Entomol Zool Stud. 5, 1446–1451.
Jia, B., Raphenya, A. R., Alcock, B., Waglechner, N., Guo, P., Tsang, K. K., et al. (2016). CARD 2017: expansion and model-centric curation of the comprehensive antibiotic resistance database. Nucleic Acids Res. 45:gkw1004. doi: 10.1093/nar/gkw1004
Jjemba, P. K., Weinrich, L. A., Cheng, W., Giraldo, E., and LeChevallier, M. W. (2010). Regrowth of potential opportunistic pathogens and algae in reclaimed-water distribution systems. Appl. Environ. Microbiol. 76, 4169–4178. doi: 10.1128/AEM.03147-09
Johansson, M. H., Bortolaia, V., Tansirichaiya, S., Aarestrup, F. M., Roberts, A. P., and Petersen, T. N. (2021). Detection of mobile genetic elements associated with antibiotic resistance in salmonella enterica using a newly developed web tool: MobileElementFinder. J. Antimicrob. Chemother. 76, 101–109. doi: 10.1093/jac/dkaa390
Johnson, M., Zaretskaya, I., Raytselis, Y., Merezhuk, Y., McGinnis, S., and Madden, T. L. (2008). NCBI BLAST: a better web interface. Nucleic Acids Res. 36, W5–W9. doi: 10.1093/nar/gkn201
Jung, Y., Jang, H., and Matthews, K. R. (2014). Effect of the food production chain from farm practices to vegetable processing on outbreak incidence. Microb. Biotechnol. 7, 517–527. doi: 10.1111/1751-7915.12178
Kang, D. D., Li, F., Kirton, E., Thomas, A., Egan, R., An, H., et al. (2019). MetaBAT 2: an adaptive binning algorithm for robust and efficient genome reconstruction from metagenome assemblies. PeerJ. 7:e7359. doi: 10.7717/peerj.7359
Kang, Y., Li, Q., Yin, Z., Shen, M., Zhao, H., Bai, Y., et al. (2018). High diversity and abundance of cultivable tetracycline-resistant bacteria in soil following pig manure application. Sci. Rep. 8:1489. doi: 10.1038/s41598-018-20050-8
Keen, P. L., and Fugère, R. (2017). Antimicrobial resistance in wastewater treatment processes. New York: John Wiley & Sons.
Khodadadi, E., Zeinalzadeh, E., Taghizadeh, S., Mehramouz, B., Kamounah, F. S., Khodadadi, E., et al. (2020). Proteomic applications in antimicrobial resistance and clinical microbiology studies. Infection and Drug Resistance. 13:1785. doi: 10.2147/IDR.S238446
Kraemer, S. A., Ramachandran, A., and Perron, G. G. (2019). Antibiotic pollution in the environment: from microbial ecology to public policy. Microorganisms. 7:180. doi: 10.3390/microorganisms7060180
Kumar, C. S. (2017). Molecular mechanism of drug resistance: common themes. Drug Resistance in Bacteria, Fungi, Malaria, and Cancer., 25–46. doi: 10.1007/978-3-319-48683-3_2
Kumar, S. B., Arnipalli, S. R., and Ziouzenkova, O. (2020). Antibiotics in food chain: the consequences for antibiotic resistance. Antibiotics. 9:688. doi: 10.3390/antibiotics9100688
Lagier, J. C., Armougom, F., Million, M., Hugon, P., Pagnier, I., Robert, C., et al. (2012). Microbial culturomics: paradigm shift in the human gut microbiome study. Clin. Microbiol. Infect. 18, 1185–1193. doi: 10.1111/1469-0691.12023
Lakin, S. M., Dean, C., Noyes, N. R., Dettenwanger, A., Ross, A. S., Doster, E., et al. (2017). MEGARes: an antimicrobial resistance database for high throughput sequencing. Nucleic Acids Res. 45, D574–D580. doi: 10.1093/nar/gkw1009
Lakin, S. M., Kuhnle, A., Alipanahi, B., Noyes, N. R., Dean, C., Muggli, M., et al. (2019). Hierarchical hidden Markov models enable accurate and diverse detection of antimicrobial resistance sequences. Communications Biology. 2:294. doi: 10.1038/s42003-019-0545-9
Lal Gupta, C., Kumar Tiwari, R., and Cytryn, E. (2020). Platforms for elucidating antibiotic resistance in single genomes and complex metagenomes. Environ. Int. 138:105667. doi: 10.1016/j.envint.2020.105667
Landers, T. F., Cohen, B., Wittum, T. E., and Larson, E. L. (2012). A review of antibiotic use in food animals: perspective, policy, and potential. Public Health Rep. 127, 4–22. doi: 10.1177/003335491212700103
Langmead, B., and Salzberg, S. L. (2012). Fast gapped-read alignment with bowtie 2. Nat. Methods 9, 357–359. doi: 10.1038/nmeth.1923
Leplae, R., Hebrant, A., Wodak, S. J., and Toussaint, A. (2004). ACLAME: a CLAssification of Mobile genetic elements. Nucleic Acids Res. 32, D45–D49. doi: 10.1093/nar/gkh084
Leplae, R., Lima-Mendez, G., and Toussaint, A. (2010). ACLAME: a CLAssification of Mobile genetic elements, update 2010. Nucleic Acids Res. 38, D57–D61. doi: 10.1093/nar/gkp938
Leung, H. C., Yiu, S.-M., Yang, B., Peng, Y., Wang, Y., Liu, Z., et al. (2011). A robust and accurate binning algorithm for metagenomic sequences with arbitrary species abundance ratio. Bioinformatics 27, 1489–1495. doi: 10.1093/bioinformatics/btr186
Li, H. (2018). Minimap2: pairwise alignment for nucleotide sequences. Bioinformatics 34, 3094–3100. doi: 10.1093/bioinformatics/bty191
Li, H., and Durbin, R. (2009). Fast and accurate short read alignment with Burrows–Wheeler transform. Bioinformatics 25, 1754–1760. doi: 10.1093/bioinformatics/btp324
Li, D., Liu, C.-M., Luo, R., Sadakane, K., and Lam, T.-W. (2015). MEGAHIT: an ultra-fast single-node solution for large and complex metagenomics assembly via succinct de Bruijn graph. Bioinformatics 31, 1674–1676. doi: 10.1093/bioinformatics/btv033
Li, X., Xie, Y., Liu, M., Tai, C., Sun, J., Deng, Z., et al. (2018). oriTfinder: a web-based tool for the identification of origin of transfers in DNA sequences of bacterial mobile genetic elements. Nucleic Acids Res. 46, W229–W234. doi: 10.1093/nar/gky352
Lin, H., Sun, W., Zhang, Z., Chapman, S. J., Freitag, T. E., Fu, J., et al. (2016). Effects of manure and mineral fertilization strategies on soil antibiotic resistance gene levels and microbial community in a paddy–upland rotation system. Environ. Pollut. 211, 332–337. doi: 10.1016/j.envpol.2016.01.007
Littmann, J., Buyx, A., and Cars, O. (2015). Antibiotic resistance: an ethical challenge. Int. J. Antimicrob. Agents 46, 359–361. doi: 10.1016/j.ijantimicag.2015.06.010
Liu, M., Li, X., Xie, Y., Bi, D., Sun, J., Li, J., et al. (2019). ICEberg 2.0: an updated database of bacterial integrative and conjugative elements. Nucleic Acids Res. 47, D660–D665. doi: 10.1093/nar/gky1123
Liu, L., Wang, Y., Che, Y., Chen, Y., Xia, Y., Luo, R., et al. (2020). High-quality bacterial genomes of a partial-nitritation/anammox system by an iterative hybrid assembly method. Microbiome. 8:155. doi: 10.1186/s40168-020-00937-3
Luo, R., Liu, B., Xie, Y., Li, Z., Huang, W., Yuan, J., et al. (2012). SOAPdenovo2: an empirically improved memory-efficient short-read de novo assembler. Gigascience. 1:2047. doi: 10.1186/2047-217X-1-18
MacLean, R. C., and San, M. A. (2019). The evolution of antibiotic resistance. Science 365, 1082–1083. doi: 10.1126/science.aax3879
Madec, J.-Y., and Haenni, M. (2018). Antimicrobial resistance plasmid reservoir in food and food-producing animals. Plasmid 99, 72–81. doi: 10.1016/j.plasmid.2018.09.001
Magouras, I., Carmo, L. P., Stärk, K. D., and Schüpbach-Regula, G. (2017). Antimicrobial usage and-resistance in livestock: where should we focus? Frontiers in veterinary science. 4:148. doi: 10.3389/fvets.2017.00148
Maguire, F., Jia, B., Gray, K. L., Lau, W. Y. V., Beiko, R. G., and Brinkman, F. S. (2020). Metagenome-assembled genome binning methods with short reads disproportionately fail for plasmids and genomic islands. Microbial genomics. 6:436. doi: 10.1099/mgen.0.000436
Mahmood, A. R., Al-Haideri, H. H., and Hassan, F. M. (2019). Detection of antibiotics in drinking water treatment plants in Baghdad City, Iraq. Advances. Public Health 2019, 1–11. doi: 10.1155/2019/7851354
Maier, T. V., Lucio, M., Lee, L. H., VerBerkmoes, N. C., Brislawn, C. J., Bernhardt, J., et al. (2017). Impact of dietary resistant starch on the human gut microbiome, metaproteome, and metabolome. MBio 8, e01343–e01317. doi: 10.1128/mBio.01343-17
Malagón-Rojas, J., and Lagos, L. (2020). From environment to clinic: the role of pesticides in antimicrobial resistance. Revista Panamericana de Salud Publica= Pan. Am. J. Public Health 44:e44-e. doi: 10.26633/RPSP.2020.44
Markowitz, V. M., Mavromatis, K., Ivanova, N. N., Chen, I.-M. A., Chu, K., and Kyrpides, N. C. (2009). IMG ER: a system for microbial genome annotation expert review and curation. Bioinformatics 25, 2271–2278. doi: 10.1093/bioinformatics/btp393
Marshall, B. M., and Levy, S. B. (2011). Food animals and antimicrobials: impacts on human health. Clin. Microbiol. Rev. 24, 718–733. doi: 10.1128/CMR.00002-11
Marti, E., Variatza, E., and Balcazar, J. L. (2014). The role of aquatic ecosystems as reservoirs of antibiotic resistance. Trends Microbiol. 22, 36–41. doi: 10.1016/j.tim.2013.11.001
Martínez, J. L. (2008). Antibiotics and antibiotic resistance genes in natural environments. Science 321, 365–367. doi: 10.1126/science.1159483
McArthur, A. G., and Tsang, K. K. (2017). Antimicrobial resistance surveillance in the genomic age. Ann. N. Y. Acad. Sci. 1388, 78–91. doi: 10.1111/nyas.13289
McEwen, S. A., and Collignon, P. J. (2018). Antimicrobial resistance: a one health perspective. Antimicrobial resistance in bacteria from livestock and companion animals. 6, 521–547. doi: 10.1128/microbiolspec.ARBA-0009-2
McHardy, A. C., Martín, H. G., Tsirigos, A., Hugenholtz, P., and Rigoutsos, I. (2007). Accurate phylogenetic classification of variable-length DNA fragments. Nat. Methods 4, 63–72. doi: 10.1038/nmeth976
Meena, V., Dotaniya, M., Saha, J., and Patra, A. (2015). Antibiotics and antibiotic resistant bacteria in wastewater: impact on environment, soil microbial activity and human health. Afr. J. Microbiol. Res. 9, 965–978. doi: 10.5897/AJMR2015.7195
Michael, I., Rizzo, L., McArdell, C., Manaia, C., Merlin, C., Schwartz, T., et al. (2013). Urban wastewater treatment plants as hotspots for the release of antibiotics in the environment: a review. Water Res. 47, 957–995. doi: 10.1016/j.watres.2012.11.027
Miłobedzka, A., Ferreira, C., Vaz-Moreira, I., Calderón-Franco, D., Gorecki, A., Purkrtova, S., et al. (2021). Monitoring antibiotic resistance genes in wastewater environments: the challenges of filling a gap in the one-health cycle. J. Hazard. Mater. 424:127407. doi: 10.1016/j.jhazmat.2021.127407
Minden, V., Deloy, A., Volkert, A. M., Leonhardt, S. D., and Pufal, G. (2017). Antibiotics impact plant traits, even at small concentrations. AoB Plants. 9:010. doi: 10.1093/aobpla/plx010
Mir, S. A., Shah, M. A., Mir, M. M., Dar, B. N., Greiner, R., and Roohinejad, S. (2018). Microbiological contamination of ready-to-eat vegetable salads in developing countries and potential solutions in the supply chain to control microbial pathogens. Food Control 85, 235–244. doi: 10.1016/j.foodcont.2017.10.006
Mirza, S. A., Liaqat, I., Awan, M. U. F., and Afzaal, M. (2020). Long-range transport of antibiotics and AMR/ARGs. Antibiotics and Antimicrobial Resistance Genes in the Environment: Elsevier 1, 117–125. doi: 10.1016/B978-0-12-818882-8.00007-3
Miyata, M., Ihara, I., Yoshid, G., Toyod, K., and Umetsu, K. (2011). Electrochemical oxidation of tetracycline antibiotics using a Ti/IrO2 anode for wastewater treatment of animal husbandry. Water Sci. Technol. 63, 456–461. doi: 10.2166/wst.2011.243
Moralez, J., Szenkiel, K., Hamilton, K., Pruden, A., and Lopatkin, A. J. (2021). Quantitative analysis of horizontal gene transfer in complex systems. Curr. Opin. Microbiol. 62, 103–109. doi: 10.1016/j.mib.2021.05.001
Moura, A., Soares, M., Pereira, C., Leitão, N., Henriques, I., and Correia, A. (2009). INTEGRALL: a database and search engine for integrons, integrases and gene cassettes. Bioinformatics 25, 1096–1098. doi: 10.1093/bioinformatics/btp105
Munita, J. M., and Arias, C. A. (2016). Mechanisms of antibiotic resistance. Virulence mechanisms of bacterial pathogens. 4, 481–511. doi: 10.1128/microbiolspec.VMBF-0016-2015
Naas, T., Oueslati, S., Bonnin, R. A., Dabos, M. L., Zavala, A., Dortet, L., et al. (2017). Beta-lactamase database (BLDB)–structure and function. J. Enzyme Inhib. Med. Chem. 32, 917–919. doi: 10.1080/14756366.2017.1344235
Namiki, T., Hachiya, T., Tanaka, H., and Sakakibara, Y. (2012). MetaVelvet: an extension of velvet assembler to de novo metagenome assembly from short sequence reads. Nucleic Acids Res. 40:e155-e. doi: 10.1093/nar/gks678
Nijsingh, N., Munthe, C., and Larsson, D. J. (2019). Managing pollution from antibiotics manufacturing: charting actors, incentives and disincentives. Environ. Health 18, 1–17. doi: 10.1186/s12940-019-0531-1
Nnadozie, C. F., and Odume, O. N. (2019). Freshwater environments as reservoirs of antibiotic resistant bacteria and their role in the dissemination of antibiotic resistance genes. Environ. Pollut. 254:113067. doi: 10.1016/j.envpol.2019.113067
Noguchi, H., Taniguchi, T., and Itoh, T. (2008). MetaGeneAnnotator: detecting species-specific patterns of ribosomal binding site for precise gene prediction in anonymous prokaryotic and phage genomes. DNA Res. 15, 387–396. doi: 10.1093/dnares/dsn027
Nowrotek, M., Jałowiecki, Ł., Harnisz, M., and Płaza, G. A. (2019). Culturomics and metagenomics: in understanding of environmental resistome. Front. Environ. Sci. Eng. 13:40. doi: 10.1007/s11783-019-1121-8
Nurk, S., Meleshko, D., Korobeynikov, A., and Pevzner, P. A. (2017). metaSPAdes: a new versatile metagenomic assembler. Genome Res. 27, 824–834. doi: 10.1101/gr.213959.116
Oliveira, N., Gonçalves, B., Lee, S., Oliveira, C., and Corassin, C. (2020). Use of antibiotics in animal production and its impact on human health. J Food Chem Nanotechnol. 6, 40–47. doi: 10.17756/jfcn.2020-082
Oniciuc, E. A., Likotrafiti, E., Alvarez-Molina, A., Prieto, M., Santos, J. A., and Alvarez-Ordóñez, A. (2018). The present and future of whole genome sequencing (WGS) and whole metagenome sequencing (WMS) for surveillance of antimicrobial resistant microorganisms and antimicrobial resistance genes across the food chain. Genes. 9:268. doi: 10.3390/genes9050268
Opatowski, L., Opatowski, M., Vong, S., and Temime, L. (2021). A one-health quantitative model to assess the risk of antibiotic resistance Acquisition in Asian Populations: impact of exposure through food, water. Livestock and Humans. Risk Analysis. 41, 1427–1446. doi: 10.1111/risa.13618
Ou, H.-Y., He, X., Harrison, E. M., Kulasekara, B. R., Thani, A. B., Kadioglu, A., et al. (2007). MobilomeFINDER: web-based tools for in silico and experimental discovery of bacterial genomic islands. Nucleic Acids Res. 35, W97–W104. doi: 10.1093/nar/gkm380
Pallares-Vega, R., Blaak, H., van der Plaats, R., de Roda Husman, A. M., Hernandez Leal, L., van Loosdrecht, M. C. M., et al. (2019). Determinants of presence and removal of antibiotic resistance genes during WWTP treatment: a cross-sectional study. Water Res. 161, 319–328. doi: 10.1016/j.watres.2019.05.100
Pan, M., and Chu, L. (2017). Fate of antibiotics in soil and their uptake by edible crops. Sci. Total Environ. 599, 599–600.
Papavarnavas, N. S., and Mendelson, M. (2022). Optimising blood cultures: the interplay between diagnostic and antimicrobial stewardship. S. Afr. Med. J. 112, 395–396. doi: 10.7196/SAMJ.2022.v112i6.16557
Parks, D. H., Imelfort, M., Skennerton, C. T., Hugenholtz, P., and Tyson, G. W. (2015). CheckM: assessing the quality of microbial genomes recovered from isolates, single cells, and metagenomes. Genome Res. 25:1043. doi: 10.1101/gr.186072.114
Partridge, S. R., Kwong, S. M., Firth, N., and Jensen, S. O. (2018). Mobile genetic elements associated with antimicrobial resistance. Clin. Microbiol. Rev. 31, 1–61. doi: 10.1128/CMR.00088-17
Peng, Y., Leung, H. C., Yiu, S., and Chin, F. Y. (2012). IDBA-UD: A de novo assembler for single-cell and metagenomic sequencing data with highly uneven depth. Bioinformatics 28, 1420–1428. doi: 10.1093/bioinformatics/bts174
Peng, Z., Mao, Y., Zhang, N., Zhang, L., Wang, Z., and Han, M. (2021). Utilizing metagenomic data and Bioinformatic tools for elucidating antibiotic resistance genes in environment. Front. Environ. Sci. 9:757365. doi: 10.3389/fenvs.2021.757365
Pérez-Sánchez, T., Mora-Sánchez, B., and Balcázar, J. L. (2018). Biological approaches for disease control in aquaculture: advantages, limitations and challenges. Trends Microbiol. 26, 896–903. doi: 10.1016/j.tim.2018.05.002
Pérez-Valera, E., Kyselková, M., Ahmed, E., Sladecek, F. X. J., Goberna, M., and Elhottová, D. (2019). Native soil microorganisms hinder the soil enrichment with antibiotic resistance genes following manure applications. Sci. Rep. 9:6760. doi: 10.1016/j.watres.2019.05.100
Pokharel, S., Raut, S., and Adhikari, B. (2019). Tackling antimicrobial resistance in low-income and middle-income countries. BMJ Specialist Journals. e002104. doi: 10.1136/bmjgh-2019-002104
Polianciuc, S. I., Gurzău, A. E., Kiss, B., Ştefan, M. G., and Loghin, F. (2020). Antibiotics in the environment: causes and consequences. Medicine and pharmacy reports. 93:231. doi: 10.15386/mpr-1742
Popowska, M., Rzeczycka, M., Miernik, A., Krawczyk-Balska, A., Walsh, F., and Duffy, B. (2012). Influence of soil use on prevalence of tetracycline, streptomycin, and erythromycin resistance and associated resistance genes. Antimicrob. Agents Chemother. 56, 1434–1443. doi: 10.1128/AAC.05766-11
Pornsukarom, S., and Thakur, S. (2017). Horizontal dissemination of antimicrobial resistance determinants in multiple salmonella serotypes following isolation from the commercial swine operation environment after manure application. Appl. Environ. Microbiol. 83, e01503–e01517. doi: 10.1128/AEM.01503-17
Power, M. (2019). 17 Antimicrobial resistance. In Current Therapy in Medicine of Australian Mammals. (2nd ed.). eds. L. Vogelnest and T. Portas (Australia: CSIRO Publishing), 285–292.
Preena, P. G., Swaminathan, T. R., Kumar, V. J. R., and Singh, I. S. B. (2020b). Antimicrobial resistance in aquaculture: a crisis for concern. Biologia 75, 1497–1517. doi: 10.2478/s11756-020-00456-4
Preena, P., Swaminathan, T. R., Rejish Kumar, V., and Bright, S. I. (2020a). Unravelling the menace: detection of antimicrobial resistance in aquaculture. Lett. Appl. Microbiol. 71, 26–38. doi: 10.1111/lam.13292
Prestinaci, F., Pezzotti, P., and Pantosti, A. (2015). Antimicrobial resistance: a global multifaceted phenomenon. Pathogens and global health. 109, 309–318. doi: 10.1179/2047773215Y.0000000030
Prüss-Ustün, A., Wolf, J., Bartram, J., Clasen, T., Cumming, O., Freeman, M. C., et al. (2019). Burden of disease from inadequate water, sanitation and hygiene for selected adverse health outcomes: an updated analysis with a focus on low-and middle-income countries. Int. J. Hyg. Environ. Health 222, 765–777. doi: 10.1016/j.ijheh.2019.05.004
Quainoo, S., Coolen, J. P., van Hijum, S. A., Huynen, M. A., Melchers, W. J., van Schaik, W., et al. (2017). Whole-genome sequencing of bacterial pathogens: the future of nosocomial outbreak analysis. Clin. Microbiol. Rev. 30, 1015–1063. doi: 10.1128/CMR.00016-17
Rajwar, A., Srivastava, P., and Sahgal, M. (2016). Microbiology of fresh produce: route of contamination, detection methods, and remedy. Crit. Rev. Food Sci. Nutr. 56, 2383–2390. doi: 10.1080/10408398.2013.841119
Raman, R. (2017). The impact of genetically modified (GM) crops in modern agriculture: a review. GM Crops & Food. 8, 195–208. doi: 10.1080/21645698.2017.1413522
Ramtahal, M. A., Amoako, D. G., Akebe, A. L., Somboro, A. M., Bester, L. A., and Essack, S. Y. (2022). A public health insight into salmonella in poultry in Africa: a review of the past decade: 2010–2020. Microb. Drug Resist. 28, 710–733. doi: 10.1089/mdr.2021.0384
Ranjan, R., Rani, A., Metwally, A., McGee, H. S., and Perkins, D. L. (2016). Analysis of the microbiome: advantages of whole genome shotgun versus 16S amplicon sequencing. Biochem. Biophys. Res. Commun. 469, 967–977. doi: 10.1016/j.bbrc.2015.12.083
Rankin, D., Rocha, E., and Brown, S. (2011). What traits are carried on mobile genetic elements, and why? Heredity 106, 1–10. doi: 10.1038/hdy.2010.24
Redfield, R. J., and Soucy, S. M. (2018). Evolution of bacterial gene transfer agents. Front. Microbiol. 9:2527. doi: 10.3389/fmicb.2018.02527
Reverter, M., Sarter, S., Caruso, D., Avarre, J.-C., Combe, M., Pepey, E., et al. (2020). Aquaculture at the crossroads of global warming and antimicrobial resistance. Nat. Commun. 11, 1–8. doi: 10.1038/s41467-020-15735-6
Riadi, G., Medina-Moenne, C., and Holmes, D. S. (2012). TnpPred: a web service for the robust prediction of prokaryotic transposases. Comparative and functional genomics. 2012:761. doi: 10.1155/2012/678761
Robinson, T. P., Bu, D., Carrique-Mas, J., Fèvre, E. M., Gilbert, M., Grace, D., et al. (2016). Antibiotic resistance is the quintessential one health issue. Trans. R. Soc. Trop. Med. Hyg. 110, 377–380. doi: 10.1093/trstmh/trw048
Robinson, T. P., Wertheim, H. F. L., Kakkar, M., Kariuki, S., Bu, D., and Price, L. B. (2016). Animal production and antimicrobial resistance in the clinic. Lancet 387, e1–e3. doi: 10.1016/S0140-6736(15)00730-8
Rocha, C. P., Cabral, H. N., Marques, J. C., and Gonçalves, A. M. (2022). A global overview of aquaculture food production with a focus on the Activity’s development in transitional systems—the case study of a south European country (Portugal). Journal of Marine Science and Engineering. 10:417. doi: 10.3390/jmse10030417
Rodríguez-Beltrán, J., Sørum, V., Toll-Riera, M., de la Vega, C., Peña-Miller, R., and San, M. Á. (2020). Genetic dominance governs the evolution and spread of mobile genetic elements in bacteria. Proc. Natl. Acad. Sci. 117, 15755–15762. doi: 10.1073/pnas.2001240117
Rodríguez-Molina, D., Mang, P., Schmitt, H., Chifiriuc, M. C., Radon, K., and Wengenroth, L. (2019). Do wastewater treatment plants increase antibiotic resistant bacteria or genes in the environment? Protocol for a systematic review. Syst. Rev. 8:304. doi: 10.1186/s13643-019-1236-9
Ruppé, E., Ghozlane, A., Tap, J., Pons, N., Alvarez, A.-S., Maziers, N., et al. (2019). Prediction of the intestinal resistome by a three-dimensional structure-based method. Nat. Microbiol. 4, 112–123. doi: 10.1038/s41564-018-0292-6
Sanganyado, E., and Gwenzi, W. (2019). Antibiotic resistance in drinking water systems: occurrence, removal, and human health risks. Sci. Total Environ. 669, 785–797. doi: 10.1016/j.scitotenv.2019.03.162
Sarkar, D. J., Mukherjee, I., Shakil, N. A., Rana, V. S., Kaushik, P., and Debnath, S. (2018). Antibiotics in agriculture: Use and impact. 4–19.
Sayers, E. W., Beck, J., Bolton, E. E., Bourexis, D., Brister, J. R., Canese, K., et al. (2021). Database resources of the national center for biotechnology information. Nucleic Acids Res. 49:D10. doi: 10.1093/nar/gkaa892
Schar, D., Zhao, C., Wang, Y., Larsson, D., Gilbert, M., and Van Boeckel, T. P. (2021). Twenty-year trends in antimicrobial resistance from aquaculture and fisheries in Asia. Nat. Commun. 12, 1–10. doi: 10.1038/s41467-021-25655-8
Schmieder, R., and Edwards, R. (2012). Insights into antibiotic resistance through metagenomic approaches. Future Microbiol. 7, 73–89. doi: 10.2217/fmb.11.135
Seiler, C., and Berendonk, T. U. (2012). Heavy metal driven co-selection of antibiotic resistance in soil and water bodies impacted by agriculture and aquaculture. Front. Microbiol. 3:399. doi: 10.3389/fmicb.2012.00399
Shah, S. Q., Cabello, F. C., L'Abée-Lund, T. M., Tomova, A., Godfrey, H. P., Buschmann, A. H., et al. (2014). Antimicrobial resistance and antimicrobial resistance genes in marine bacteria from salmon aquaculture and non-aquaculture sites. Environ. Microbiol. 16, 1310–1320. doi: 10.1111/1462-2920.12421
She, Y., Cai, H., and Liu, G. (2018). Effects of antibiotic on microflora in ileum and cecum for broilers by 16S rRNA sequence analysis. 1680–1691.
Shen, Q., and Zhao, F. (2018). Antibiotics and antibiotic resistance from animal manures to soil: a review. Eur. J. Soil Sci. 69, 181–195.
Shi, P., Jia, S., Zhang, X.-X., Zhang, T., Cheng, S., and Li, A. (2013). Metagenomic insights into chlorination effects on microbial antibiotic resistance in drinking water. Water Res. 47, 111–120. doi: 10.1016/j.watres.2012.09.046
Sieber, C. M., Probst, A. J., Sharrar, A., Thomas, B. C., Hess, M., Tringe, S. G., et al. (2018). Recovery of genomes from metagenomes via a dereplication, aggregation and scoring strategy. Nat. Microbiol. 3, 836–843. doi: 10.1038/s41564-018-0171-1
Siguier, P., Pérochon, J., Lestrade, L., Mahillon, J., and Chandler, M. (2006). ISfinder: the reference Centre for bacterial insertion sequences. Nucleic Acids Res. 34, D32–D36. doi: 10.1093/nar/gkj014
Simpson, J. T., Wong, K., Jackman, S. D., Schein, J. E., Jones, S. J., and Birol, I. (2009). ABySS: a parallel assembler for short read sequence data. Genome Res. 19, 1117–1123. doi: 10.1101/gr.089532.108
Slizovskiy, I. B., Mukherjee, K., Dean, C. J., Boucher, C., and Noyes, N. R. (2020). Mobilization of antibiotic resistance: are current approaches for colocalizing resistomes and mobilomes useful? Front. Microbiol. 11:1376.
Song, W., Wemheuer, B., Zhang, S., Steensen, K., and Thomas, T. (2019). MetaCHIP: community-level horizontal gene transfer identification through the combination of best-match and phylogenetic approaches. Microbiome. 7, 1–14. doi: 10.1186/s40168-019-0649-y
Sonone, S. S., Jadhav, S., Sankhla, M. S., and Kumar, R. (2020). Water contamination by heavy metals and their toxic effect on aquaculture and human health through food chain. Letters in applied NanoBioScience. 10, 2148–2166. doi: 10.33263/LIANBS102.21482166
Speksnijder, D., Jaarsma, A., Van Der Gugten, A., Verheij, T. J., and Wagenaar, J. (2015). Determinants associated with veterinary antimicrobial prescribing in farm animals in the Netherlands: a qualitative study. Zoonoses Public Health 62, 39–51. doi: 10.1111/zph.12168
Spielmeyer, A. (2018). Occurrence and fate of antibiotics in manure during manure treatments: a short review. Sustain. Chem. Pharm. 9, 76–86. doi: 10.1016/j.scp.2018.06.004
Srivastava, A., Singhal, N., Goel, M., Virdi, J. S., and Kumar, M. (2014). CBMAR: a comprehensive β-lactamase molecular annotation resource. Database 2014:111. doi: 10.1093/database/bau111
Stalder, T., Press, M. O., Sullivan, S., Liachko, I., and Top, E. M. (2019). Linking the resistome and plasmidome to the microbiome. ISME J. 13, 2437–2446. doi: 10.1038/s41396-019-0446-4
Stärk, K. D., Pękala, A., and Muellner, P. (2019). Use of molecular and genomic data for disease surveillance in aquaculture: towards improved evidence for decision making. Prev. Vet. Med. 167, 190–195. doi: 10.1016/j.prevetmed.2018.04.011
Su, J. Q., Wei, B., Xu, C. Y., Qiao, M., and Zhu, Y. G. (2014). Functional metagenomic characterization of antibiotic resistance genes in agricultural soils from China. Environ. Int. 65, 9–15. doi: 10.1016/j.envint.2013.12.010
Suenaga, H. (2012). Targeted metagenomics: a high-resolution metagenomics approach for specific gene clusters in complex microbial communities. Environ. Microbiol. 14, 13–22. doi: 10.1111/j.1462-2920.2011.02438.x
Sun, D., Jeannot, K., Xiao, Y., and Knapp, C. W. (2020). Horizontal gene transfer mediated bacterial antibiotic resistance Horizontal Gene Transfer Mediated Multidrug Resistance: A Global Crisis, Published in Frontiers in microbiology 6.
Sundin, G. W., and Wang, N. (2018). Antibiotic resistance in plant-pathogenic bacteria. Annu. Rev. Phytopathol. 56, 161–180. doi: 10.1146/annurev-phyto-080417-045946
Tang, P.-C. (2016). A metagenomic insight into the role of wastewater treatment plants as potential hotspot for antibiotic resistant bacteria and antibiotic resistance genes. 1–378.
Tang, J., Bu, Y., Zhang, X.-X., Huang, K., He, X., Ye, L., et al. (2016). Metagenomic analysis of bacterial community composition and antibiotic resistance genes in a wastewater treatment plant and its receiving surface water. Ecotoxicol. Environ. Saf. 132, 260–269. doi: 10.1016/j.ecoenv.2016.06.016
Taş, N., de Jong, A. E. E., Li, Y., Trubl, G., Xue, Y., and Dove, N. C. (2021). Metagenomic tools in microbial ecology research. Curr. Opin. Biotechnol. 67, 184–191. doi: 10.1016/j.copbio.2021.01.019
Taylor, N. G., Verner-Jeffreys, D. W., and Baker-Austin, C. (2011). Aquatic systems: maintaining, mixing and mobilising antimicrobial resistance? Trends Ecol. Evol. 26, 278–284. doi: 10.1016/j.tree.2011.03.004
Teeling, H., Waldmann, J., Lombardot, T., et al. (2004). TETRA: a web-service and a stand-alone program for the analysis and comparison of tetranucleotide usage patterns in DNA sequences. BMC Bioinformatics. 5:163. doi: 10.1186/1471-2105-5-163
Thai, Q. K., Bös, F., and Pleiss, J. (2009). The lactamase engineering database: a critical survey of TEM sequences in public databases. BMC Genomics 10, 1–9. doi: 10.1186/1471-2164-10-390
Thomas, T., Gilbert, J., and Meyer, F. (2012). Metagenomics-a guide from sampling to data analysis. Microbial informatics and experimentation. 2, 1–12. doi: 10.1186/2042-5783-2-3
Torres-Cortés, G., Millán, V., Ramírez-Saad, H. C., Nisa-Martínez, R., Toro, N., and Martínez-Abarca, F. (2011). Characterization of novel antibiotic resistance genes identified by functional metagenomics on soil samples. Environ. Microbiol. 13, 1101–1114. doi: 10.1111/j.1462-2920.2010.02422.x
Tyagi, A., Singh, B., Thammegowda, N. K. B., and Singh, N. K. (2019). Shotgun metagenomics offers novel insights into taxonomic compositions, metabolic pathways and antibiotic resistance genes in fish gut microbiome. Arch. Microbiol. 201, 295–303. doi: 10.1007/s00203-018-1615-y
Van Boeckel, T. P., Brower, C., Gilbert, M., Grenfell, B. T., Levin, S. A., Robinson, T. P., et al. (2015). Global trends in antimicrobial use in food animals. Proc. Natl. Acad. Sci. 112, 5649–5654. doi: 10.1073/pnas.1503141112
Van Boeckel, T. P., Glennon, E. E., Chen, D., Gilbert, M., Robinson, T. P., Grenfell, B. T., et al. (2017). Reducing antimicrobial use in food animals. Science 357, 1350–1352. doi: 10.1126/science.aao1495
Van Camp, P.-J., Haslam, D. B., and Porollo, A. (2020). Bioinformatics approaches to the understanding of molecular mechanisms in antimicrobial resistance. Int. J. Mol. Sci. 21:1363. doi: 10.3390/ijms21041363
Van Gompel, L., Luiken, R. E. C., Hansen, R. B., Munk, P., Bouwknegt, M., Heres, L., et al. (2020). Description and determinants of the faecal resistome and microbiome of farmers and slaughterhouse workers: a metagenome-wide cross-sectional study. Environ. Int. 143:105939. doi: 10.1016/j.envint.2020.105939
Van Hoek, A. H., Mevius, D., Guerra, B., Mullany, P., Roberts, A. P., and Aarts, H. J. (2011). Acquired antibiotic resistance genes: an overview. Front. Microbiol. 2:203. doi: 10.3389/fmicb.2011.00203
Varani, A. M., Siguier, P., Gourbeyre, E., Charneau, V., and Chandler, M. (2011). ISsaga is an ensemble of web-based methods for high throughput identification and semi-automatic annotation of insertion sequences in prokaryotic genomes. Genome Biol. 12, 1–9. doi: 10.1186/gb-2011-12-3-r30
Vinayamohan, P. G., Pellissery, A. J., and Venkitanarayanan, K. (2022). Role of horizontal gene transfer in the dissemination of antimicrobial resistance in food animal production. Curr. Opin. Food Sci. 47:100882. doi: 10.1016/j.cofs.2022.100882
von Wintersdorff, C. J. H., Penders, J., van Niekerk, J. M., Mills, N. D., Majumder, S., van Alphen, L. B., et al. (2016). Dissemination of antimicrobial resistance in microbial ecosystems through horizontal gene transfer. Front. Microbiol. 7. doi: 10.3389/fmicb.2016.00173
Vosloo, S., Huo, L., Anderson, C. L., Dai, Z., Sevillano, M., Pinto, A., et al. (2021). Evaluating de novo assembly and binning strategies for time series drinking water Metagenomes. Microbiology Spectrum. 9, e01434–e01421. doi: 10.1128/Spectrum.01434-21
Wadamori, Y., Gooneratne, R., and Hussain, M. A. (2017). Outbreaks and factors influencing microbiological contamination of fresh produce. J. Sci. Food Agric. 97, 1396–1403. doi: 10.1002/jsfa.8125
Wallace, J. C., Port, J. A., Smith, M. N., and Faustman, E. M. (2017). FARME DB: a functional antibiotic resistance element database. Database 2017:165. doi: 10.1093/database/baw165
Wang, Y., Leung, H. C. M., Yiu, S. M., and Chin, F. Y. L. (2014). MetaCluster-TA: taxonomic annotation for metagenomic data based on assembly-assisted binning. BMC genomics 15, 1–9. doi: 10.1186/1471-2164-15-S1-S12
Wang, F.-H., Qiao, M., Chen, Z., Su, J.-Q., and Zhu, Y.-G. (2015). Antibiotic resistance genes in manure-amended soil and vegetables at harvest. J. Hazard. Mater. 299, 215–221. doi: 10.1016/j.jhazmat.2015.05.028
Watts, J. E., Schreier, H. J., Lanska, L., and Hale, M. S. (2017). The rising tide of antimicrobial resistance in aquaculture: sources, sinks and solutions. Mar. Drugs 15:158. doi: 10.3390/md15060158
Wee, B. A., Muloi, D. M., and van Bunnik, B. A. D. (2020). Quantifying the transmission of antimicrobial resistance at the human and livestock interface with genomics. Clin. Microbiol. Infect. 26, 1612–1616. doi: 10.1016/j.cmi.2020.09.019
Williams, T. J., and Cavicchioli, R. (2014). Marine metaproteomics: deciphering the microbial metabolic food web. Trends Microbiol. 22, 248–260. doi: 10.1016/j.tim.2014.03.004
Willms, I. M., Kamran, A., Aßmann, N. F., Krone, D., Bolz, S. H., Fiedler, F., et al. (2019). Discovery of novel antibiotic resistance determinants in Forest and grassland soil Metagenomes. Front. Microbiol. 10, 1–11. doi: 10.3389/fmicb.2019.00460
Wilmes, P., and Bond, P. L. (2004). The application of two-dimensional polyacrylamide gel electrophoresis and downstream analyses to a mixed community of prokaryotic microorganisms. Environ. Microbiol. 6, 911–920. doi: 10.1111/j.1462-2920.2004.00687.x
Wu, M., and Scott, A. J. (2012). Phylogenomic analysis of bacterial and archaeal sequences with AMPHORA2. Bioinformatics 28, 1033–1034. doi: 10.1093/bioinformatics/bts079
Wu, Y.-W., Simmons, B. A., and Singer, S. W. (2016). MaxBin 2.0: an automated binning algorithm to recover genomes from multiple metagenomic datasets. Bioinformatics 32, 605–607. doi: 10.1093/bioinformatics/btv638
Xavier, B. B., Das, A. J., Cochrane, G., De Ganck, S., Kumar-Singh, S., Aarestrup, F. M., et al. (2016). Consolidating and exploring antibiotic resistance gene data resources. J. Clin. Microbiol. 54, 851–859. doi: 10.1128/JCM.02717-15
Xie, W. Y., Shen, Q., and Zhao, F. (2018). Antibiotics and antibiotic resistance from animal manures to soil: a review. Eur. J. Soil Sci. 69, 181–195. doi: 10.1111/ejss.12494
Xu, W., Chen, T., Pei, Y., Guo, H., Li, Z., Yang, Y., et al. (2021). Characterization of shallow whole-Metagenome shotgun sequencing as a high-accuracy and low-cost method by complicated mock microbiomes. Front. Microbiol. 12:380. doi: 10.3389/fmicb.2021.820380
Yang, Y., Ashworth, A. J., Willett, C., Cook, K., Upadhyay, A., Owens, P. R., et al. (2019). Review of antibiotic resistance, ecology, dissemination, and mitigation in US broiler poultry systems. Front. Microbiol. 10:2639. doi: 10.3389/fmicb.2019.02639
Yang, X., Noyes, N. R., Doster, E., Martin, J. N., Linke, L. M., Magnuson, R. J., et al. (2016). Use of metagenomic shotgun sequencing technology to detect foodborne pathogens within the microbiome of the beef production chain. Appl. Environ. Microbiol. 82, 2433–2443. doi: 10.1128/AEM.00078-16
Yao, Y., Maddamsetti, R., Weiss, A., Ha, Y., Wang, T., Wang, S., et al. (2022). Intra-and interpopulation transposition of mobile genetic elements driven by antibiotic selection. Nature Ecology & Evolution. 6, 555–564. doi: 10.1038/s41559-022-01705-2
Yee, R., and Simner, P. J. (2019). Next-generation sequencing approaches to predicting antimicrobial susceptibility testing results. Advances in Molecular Pathology. 2, 99–110. doi: 10.1016/j.yamp.2019.07.008
Yin, X., Jiang, X.-T., Chai, B., Li, L., Yang, Y., Cole, J. R., et al. (2018). ARGs-OAP v2. 0 with an expanded SARG database and hidden Markov models for enhancement characterization and quantification of antibiotic resistance genes in environmental metagenomes. Bioinformatics 34, 2263–2270. doi: 10.1093/bioinformatics/bty053
Yuan, L., Wang, L., Li, Z.-H., Zhang, M.-Q., Shao, W., and Sheng, G.-P. (2019). Antibiotic resistance and microbiota in the gut of Chinese four major freshwater carp from retail markets. Environ. Pollut. 255:113327. doi: 10.1016/j.envpol.2019.113327
Yukgehnaish, K., Kumar, P., Sivachandran, P., Marimuthu, K., Arshad, A., Paray, B. A., et al. (2020). Gut microbiota metagenomics in aquaculture: factors influencing gut microbiome and its physiological role in fish. Rev. Aquac. 3, 1903–1927. doi: 10.1111/raq.12416
Zankari, E., Hasman, H., Cosentino, S., Vestergaard, M., Rasmussen, S., Lund, O., et al. (2012). Identification of acquired antimicrobial resistance genes. J. Antimicrob. Chemother. 67, 2640–2644. doi: 10.1093/jac/dks261
Zhang, X., and Figeys, D. (2019). Perspective and guidelines for Metaproteomics in microbiome studies. J. Proteome Res. 18:054. doi: 10.1021/acs.jproteome.9b00054
Zhang, Y.-J., Hu, H.-W., Chen, Q.-L., Singh, B. K., Yan, H., Chen, D., et al. (2019). Transfer of antibiotic resistance from manure-amended soils to vegetable microbiomes. Environ. Int. 130:104912. doi: 10.1016/j.envint.2019.104912
Zhang, X., Li, L., Mayne, J., Ning, Z., Stintzi, A., and Figeys, D. (2018). Assessing the impact of protein extraction methods for human gut metaproteomics. J. Proteome 180, 120–127. doi: 10.1016/j.jprot.2017.07.001
Zhao, Y., Su, J.-Q., An, X.-L., Huang, F.-Y., Rensing, C., Brandt, K. K., et al. (2018). Feed additives shift gut microbiota and enrich antibiotic resistance in swine gut. Sci. Total Environ. 621, 1224–1232. doi: 10.1016/j.scitotenv.2017.10.106
Zhao, Y., Yang, Q. E., Zhou, X., Wang, F.-H., Muurinen, J., Virta, M. P., et al. (2020). Antibiotic resistome in the livestock and aquaculture industries: status and solutions. Crit. Rev. Environ. Sci. Technol. 1, 2159–2196. doi: 10.1080/10643389.2020.1777815
Zhu, B., Chen, Q., Chen, S., and Zhu, Y.-G. (2017). Does organically produced lettuce harbor higher abundance of antibiotic resistance genes than conventionally produced? Environ. Int. 98, 152–159. doi: 10.1016/j.envint.2016.11.001
Keywords: antibiotic resistance, metagenomics, bioinformatics, microbiomes, gene transfer
Citation: Pillay S, Calderón-Franco D, Urhan A and Abeel T (2022) Metagenomic-based surveillance systems for antibiotic resistance in non-clinical settings. Front. Microbiol. 13:1066995. doi: 10.3389/fmicb.2022.1066995
Edited by:
Muhammad Yasir, King Abdulaziz University, Saudi ArabiaReviewed by:
Lateef Salam, Elizade University, NigeriaVarsha Rani Gajamer, Darjeeling Government College, India
Copyright © 2022 Pillay, Calderón-Franco, Urhan and Abeel. This is an open-access article distributed under the terms of the Creative Commons Attribution License (CC BY). The use, distribution or reproduction in other forums is permitted, provided the original author(s) and the copyright owner(s) are credited and that the original publication in this journal is cited, in accordance with accepted academic practice. No use, distribution or reproduction is permitted which does not comply with these terms.
*Correspondence: Thomas Abeel, VC5BYmVlbEB0dWRlbGZ0Lm5s