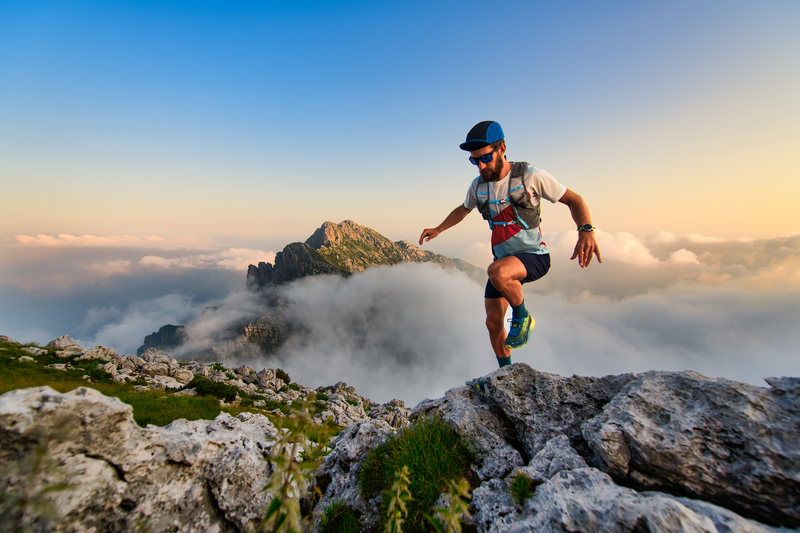
94% of researchers rate our articles as excellent or good
Learn more about the work of our research integrity team to safeguard the quality of each article we publish.
Find out more
ORIGINAL RESEARCH article
Front. Microbiol. , 24 November 2022
Sec. Food Microbiology
Volume 13 - 2022 | https://doi.org/10.3389/fmicb.2022.1060160
This article is part of the Research Topic Acetic Acid Bacteria View all 14 articles
Acetobacter species play an import role during cocoa fermentation. However, Acetobacter ghanensis and Acetobacter senegalensis are outcompeted during fermentation of the cocoa pulp-bean mass, whereas Acetobacter pasteurianus prevails. In this paper, an in silico approach aimed at delivering some insights into the possible metabolic adaptations of A. ghanensis LMG 23848T and A. senegalensis 108B, two candidate starter culture strains for cocoa fermentation processes, by reconstructing genome-scale metabolic models (GEMs). Therefore, genome sequence data of a selection of strains of Acetobacter species were used to perform a comparative genomic analysis. Combining the predicted orthologous groups of protein-encoding genes from the Acetobacter genomes with gene-reaction rules of GEMs from two reference bacteria, namely a previously manually curated model of A. pasteurianus 386B (iAp386B454) and two manually curated models of Escherichia coli (EcoCyc and iJO1366), allowed to predict the set of reactions present in A. ghanensis LMG 23848T and A. senegalensis 108B. The predicted metabolic network was manually curated using genome re-annotation data, followed by the reconstruction of species-specific GEMs. This approach additionally revealed possible differences concerning the carbon core metabolism and redox metabolism among Acetobacter species, pointing to a hitherto unexplored metabolic diversity. More specifically, the presence or absence of reactions related to citrate catabolism and the glyoxylate cycle for assimilation of C2 compounds provided not only new insights into cocoa fermentation but also interesting guidelines for future research. In general, the A. ghanensis LMG 23848T and A. senegalensis 108B GEMs, reconstructed in a semi-automated way, provided a proof-of-concept toward accelerated formation of GEMs of candidate functional starter cultures for food fermentation processes.
Acetic acid bacteria (AAB) are Gram-negative, obligately aerobic bacteria that incompletely oxidize a range of alcohols and reducing sugars for energy production. The region-and stereo-selectivity of these oxidation reactions has led to numerous biotechnological applications, most notably the conversion of D-sorbitol into L-sorbose (Shinjoh and Toyama, 2016). The genus Acetobacter comprises tens of species and is common to food fermentation processes, among which traditional vinegar production, kombucha fermentation, and cocoa fermentation (De Vuyst and Weckx, 2016; Yamada, 2016; Coton et al., 2017; Lu et al., 2018; De Vuyst and Leroy, 2020). For the latter fermentation process, several Acetobacter species have been isolated and selected as candidate functional starter cultures, including Acetobacter ghanensis LMG 23848T and Acetobacter senegalensis 108B (Camu et al., 2007; Moens et al., 2014; Illeghems et al., 2016). To gain more insights into their contribution to cocoa fermentation processes, their genomes have been sequenced and functionally annotated, revealing a possible role in citrate assimilation during fermentation of the cocoa pulp-bean mass (Illeghems et al., 2016). However, A. ghanensis and A. senegalensis are outcompeted during cocoa fermentation, whereas Acetobacter pasteurianus prevails (Lefeber et al., 2010, 2011, 2012; Papalexandratou et al., 2013; Díaz-Muñoz et al., 2021).
The gold standard in the taxonomic analysis of AAB are polyphasic approaches, combining phenotypic, chemotaxonomic, and genotypic data (Cleenwerck and De Vos, 2008; De Vuyst et al., 2008; Papalexandratou et al., 2009; Li et al., 2017). One specific metabolic feature that is typically tested for differentiating Acetobacter species is growth on ethanol as the sole carbon source. Therefore, a defined medium containing ethanol as the sole carbon source and ammonium as the sole nitrogen source is often used (Cleenwerck et al., 2002). However, carbon source usage may also be deduced through genomic analysis, since carbon assimilation pathways consist of a defined sequence of biochemical reactions, for which the respective enzyme-encoding genes must be present in the genome (Serres and Riley, 2006).
Growth of Acetobacter species on C2 compounds, such as ethanol and acetate, has so far been related to the presence of the glyoxylate cycle enzymes (Saeki et al., 1997; Sakurai et al., 2013). The glyoxylate cycle is a shunt of the tricarboxylic acid (TCA) cycle and involves only two enzymes, namely isocitrate lyase (EC 4.1.3.1), which converts isocitrate into glyoxylate and succinate, and malate synthase (EC 2.3.3.9), which binds acetyl-CoA onto glyoxylate to form malate. The glyoxylate cycle circumvents the carbon dioxide-producing reactions of the TCA cycle, thus leading to a net succinate production. The presence of genes encoding the glyoxylate cycle enzymes in Acetobacter species genomes and their relationship to the growth of these species in a medium containing ethanol as the sole carbon source has not been thoroughly investigated.
A genome-scale metabolic model (GEM) provides a well-defined data structure of the metabolic network inferred from a bacterial genome (Thiele and Palsson, 2010). Next to the use of a GEM as knowledge base of the biochemical reaction potential of a microbial strain, a GEM is also useful to perform simulations of the metabolism of a microbial strain, for instance using constraint-based modeling methods. GEMs have been used to compare the reaction potential of different microorganisms, and led to the concept of a pan-reactome (Prigent et al., 2018; Seif et al., 2018; Gu et al., 2019). In this case, the GEM reconstruction process is performed for different strains in parallel using different metabolic resources, for instance GEMs of reference species and metabolic databases.
In the present study, the approach mentioned above was applied to reconstruct GEMs for A. ghanensis LMG 23848T and A. senegalensis 108B, taking advantage of a comparative genomic analysis that encompassed a selection of 36 strains belonging to 27 species of the Acetobacter genus and a previously reconstructed and manually curated GEM of A. pasteurianus 386B (Pelicaen et al., 2019, 2020). These GEMs will be useful to gain more insights into the metabolism and hence metabolic adaptations of A. ghanensis LMG 23848T and A. senegalensis 108B to cocoa fermentation conditions by applying in silico flux balance analysis.
Three strains of Acetobacter species, originating from spontaneous cocoa fermentation processes carried out in heaps in Ghana in 2004, namely A. pasteurianus 386B, A. ghanensis LMG 23848T, and A. senegalensis 108B, were used throughout this study (Camu et al., 2007; Illeghems et al., 2013, 2016; Moens et al., 2014).
Growth experiments were performed in a modified defined medium (Verduyn et al., 1992). This medium contained: (NH4)2SO4, 5.0 g/l; KH2PO4, 1.375 g/l; MgSO4.7H2O, 0.5 g/l; ethylenediaminetetraacetic acid (EDTA), 15.0 mg/l; ZnSO4.7H2O, 4.5 mg/l; CoSO4.7H2O, 0.35 mg/l; MnCl2.4H2O, 1.0 mg/l; CuSO4.5H2O, 0.3 mg/l; CaCl2.2H2O, 4.5 mg/l; FeSO4.7H2O, 3.0 mg/l; MoO3, 0.24 mg/l; H3BO3, 1.0 mg/l; and KI, 0.1 mg/l, dissolved in 30 mM citrate buffer (pH 6.0). The final pH of the medium was adjusted to 5.0 with 0.1 M NaOH. A filter-sterilised vitamin mixture was added after heat sterilisation of the medium at 121°C for 20 min. The final vitamin concentrations were: biotin, 0.0005 mg/l; calcium pantothenate, 0.01 mg/l; nicotinic acid, 0.01 mg/l; myo-inositol, 0.25 mg/l; thiamine-HCl, 0.01 mg/l; pyridoxine-HCl, 0.01 mg/l; and para-aminobenzoic acid, 0.002 mg/l. Eight different carbon sources, namely glucose, fructose, mannitol, citric acid, glycerol, lactic acid, ethanol, and acetic acid (as sodium acetate), were used at a final concentration of 30 mM to assess if they could sustain growth of the three Acetobacter strains investigated. The pH of the citric acid, lactic acid, and sodium acetate stock solutions was set to 5.0.
For inoculum build-up, the three Acetobacter strains were grown overnight at 30°C in a standard incubator (160 rpm) in a modified yeast extract-glucose-mannitol (mYGM) medium (Lefeber et al., 2012), which contained: D-glucose, 20.0 g/l; D-mannitol, 20.0 g/l; lactic acid, 10.0 g/l; granulated yeast extract, 10.0 g/l; soy peptone, 5.0 g/l; MgSO4.7H2O, 1.0 g/l; (NH4)2HPO4, 1.0 g/l; KH2PO4, 1.0 g/l; and Na3C6H5O7, 1.0 g/l. The final pH of the medium was adjusted to 5.5 with 0.1 M NaOH. The overnight culture was centrifuged (4,000 x g, 20 min, 4°C) and washed with a filter-sterilized saline solution (0.85%, m/v, NaCl). Then, the cells were resuspended in sterile saline solution and inoculated in 2 ml of the defined medium mentioned above at an optical density at 600 nm (OD600) of 0.01, in duplicate, in test tubes with a total volume of 20 ml. The three Acetobacter strains were allowed to grow at 30°C for 48 h and 120 h. A threshold value for the OD600 of 0.1 was used to identify whether or not the strains had grown.
A similar growth experiment was performed in a modified defined medium with 30 mM phosphate buffer (pH 6.0) in triplicate. In this case, for inoculum build-up, the three Acetobacter strains were grown overnight at 30°C in a semi-defined medium (pH 5.5), which contained: lactic acid, 5.0 g/l; sodium acetate, 10.0 g/l; granulated yeast extract, 5.0 g/l; MgSO4.7H2O, 1.0 g/l; NH4H2PO4, 20.0 g/l; and K2HPO4, 10.0 g/l (Pelicaen et al., 2019). A threshold value for the OD600 of 0.1 was used to identify whether or not the strains had grown.
The protein-encoding genes of the A. ghanensis LMG 23848T and A. senegalensis 108B genomes were originally annotated using the bacterial genome annotation system GenDB v2.2 (Meyer, 2003; Illeghems et al., 2016). In the present study, these genomes were re-annotated using a previously in-house developed bioinformatics workflow (Pelicaen et al., 2019). The National Center for Biotechnology Information (NCBI, Bethesda, Madison, United States) RefSeq genome annotation version was taken as a basis for re-annotation of the genome of A. ghanensis LMG 23848T (accessed in April 2017; 2,454 protein-encoding genes) and A. senegalensis 108B (accessed in October 2017; 3,444 protein-encoding genes). The amino acid sequences of each set of protein-encoding genes were annotated using a combination of tools, namely BlastKOALA from the KEGG database (Kanehisa et al., 2016, 2017), the TransAAP tool to predict transport proteins (Elbourne et al., 2017), the subcellular localization predictor CELLO (Yu et al., 2004), eggNOG-mapper (Huerta-Cepas et al., 2017), the enzyme annotation tool PRIAM (Claudel-Renard et al., 2003), the RAST annotation pipeline from the KBase software (Overbeek et al., 2014; Arkin et al., 2018), and the tools embedded in InterProScan 5.22–61.0 (Jones et al., 2014). Furthermore, since the publication of the genome sequences of A. ghanensis LMG 23848T and A. senegalensis 108B (Illeghems et al., 2016), these genomes have been re-annotated by several annotation pipelines and the functional annotation data known at the time of analysis were stored in dedicated databases related to those pipelines. As such, these additional functional annotations were retrieved from the Carbohydrate-Active enZYmes (CAZy) database (Lombard et al., 2014), the Pathosystems Resource Integration Center (PATRIC) database (Wattam et al., 2017), and the Universal Protein Resource (UniProt) database (The UniProt Consortium, 2019).
In addition, the genome annotation pipelines used by GenDB, NCBI RefSeq (NCBI prokaryotic genome annotation pipeline; Tatusova et al., 2016) and PATRIC (RASTtk; Brettin et al., 2015) represent independent methods combining gene prediction and gene annotation. Therefore, functional annotation data of these sources were combined in an in-house MySQL database specific for A. ghanensis LMG 23848T and A. senegalensis 108B.
From all Acetobacter species mentioned in the NCBI Taxonomy database (accessed May 2019), the genome sequence of 96 strains of Acetobacter species was available in the NCBI RefSeq database. All those genome sequences were retrieved, as well as the genome sequences of Gluconobacter oxydans 621H, Komagataeibacter nataicola RZS01, and Escherichia coli str. K-12 substr. MG1655 (further referred to as E. coli). The latter three strains were used as outgroup. Next, the Acetobacter genomes were ranked according to their assembly quality level, based on information in the NCBI RefSeq database. For each Acetobacter species, the genome with the highest assembly quality level was selected, and in the case that the genome was not from the type strain, the type strain genome was also selected. Next, OrthoFinder (Emms and Kelly, 2015) was used to predict orthogroups from the protein-encoding genes of the selected Acetobacter genomes and from the three outgroup genomes. Protein identifiers from the NCBI RefSeq database were linked to gene identifiers based on the Genbank files of each genome. Subsequently, OrthoFinder was used to infer a rooted species tree based on the predicted orthogroups (Emms and Kelly, 2017, 2018).
The relationship between genes, proteins, and reactions can be described using gene-protein-reaction (GPR) associations, linking the different entities, possibly with their stoichiometry (Thiele and Palsson, 2010). However, in practice, GPR associations are typically implemented as Boolean rules that define reaction presence based on gene presence in an annotated genome (Machado et al., 2016). Albeit potentially oversimplifying the actual GPR associations, these Boolean rules (gene-reaction rules) allow to quickly evaluate the presence of a reaction in the GEM through in silico gene deletions (Ebrahim et al., 2013). In the present study, this concept was exploited to predict the reaction presence based on gene presence in the annotated genomes. To convert the text Boolean expression into a symbolic rule that allows its computational assessment, the Python library SymPy was used (Meurer et al., 2017).
As a first step in the reconstruction of the GEMs for A. ghanensis LMG 23848T and A. senegalensis 108B, the manually curated GEM of A. pasteurianus 386B (iAp386B454; Pelicaen et al., 2019) was used as a reference to evaluate which of the reactions of this GEM were present in the Acetobacter genomes considered. To minimize the number of false negative predictions of reaction presence, a separate analysis was performed, whereby only high-quality Acetobacter genomes with an NCBI assembly level corresponding to a complete genome were considered.
Next, the predictions obtained were used for the reconstruction of GEMs of A. ghanensis LMG 23848T, further referred to as iAg23848, and of A. senegalensis 108B, further referred to as iAs108B. New gene-reaction rules were associated to the transferred iAp386B454 reactions. For multi-copy orthogroups, i.e., orthogroups containing multiple genes for each genome, the logic ‘OR’ assumption was made, expressing that these genes are co-orthologs of the A. pasteurianus 386B genes. Cases for which A. pasteurianus 386B genes occurred in the same orthogroup as the ones of A. ghanensis LMG 23848T and A. senegalensis 108B and had a logic ‘AND’ relation in the iAp386B454 gene-reaction rules, were systematically checked and manually curated in the iAg23848 and iAs108B GEMs. Reactions without GPR, including spontaneous reactions as well as reactions for macromolecular biosynthesis and the iAp386B454 biomass reaction, were transferred as such to the iAg23848 and iAs108B GEMs.
Further manual curation was performed for the iAg23848 and iAs108B GEMs in a semi-automated way. First, GEM gap filling was performed using iAp386B454 as a template. Hereto, the GEM gap filling method of CobraPy was used (Ebrahim et al., 2013), which computes the minimal amount of reactions that need to be added from the iAp386B454 template to obtain in silico growth. Then, gap filling results were manually curated by combining functional annotation information of A. ghanensis LMG 23848T and A. senegalensis 108B, stored in their respective MySQL databases and in the GEMs. Only the reactions for which genome annotation evidence was found were added to the GEMs. This also included reactions necessary to reconcile in silico and in vitro growth experiments. Finally, flux balance analysis (FBA) and parsimonious FBA with biomass formation as the objective function were performed to examine the flux distributions of the newly constructed GEMs under simulated in vitro growth conditions. The total allowable carbon consumption flux was set to 60 c-mmol per gram of cell dry mass per h.
To predict the presence/absence of reactions of the glyoxylate cycle and the aerobic respiratory chain, a similar analysis as described in Section 2.4 was performed but now with an E. coli GEM as reference. For E. coli, two curated reconstruction sources were leveraged, namely the EcoCyc Pathway/Genome Database embedded in Pathway Tools version 21.5, and the iJO1366 GEM of the BIGG database (Orth et al., 2011; Keseler et al., 2017).
Growth experiments (Table 1) under defined medium conditions, using a citrate buffer, indicated that A. pasteurianus 386B showed the same growth phenotype as in defined medium with a phosphate buffer. Only the addition of lactic acid as a sole carbon source could sustain the growth of A. pasteurianus 386B under these conditions. For A. ghanensis LMG 23848T, increasing the incubation time from 48 h to 120 h allowed for growth of the bacterial population only on glucose as the sole carbon source, using a citrate buffer. In contrast, the growth phenotype of A. senegalensis 108B markedly differed when using a phosphate buffer or citrate buffer. Under growth conditions with a phosphate buffer, this strain only showed growth on lactic acid and citric acid as the sole carbon sources, but exchanging the phosphate buffer for a citrate buffer led to growth on all single carbon sources tested, at least after 120 h of incubation. These results indicated the influence of the medium buffer used, and the possibility of citrate co-consumption in the case that a citrate buffer was applied.
Table 1. Indication of bacterial population growth of strains of three different Acetobacter species in a modified defined medium with phosphate buffer or citrate buffer, supplemented with different carbon sources, after 48 h and 48 and 120 h of incubation, respectively.
Functional annotation information of A. ghanensis LMG 23848T and A. senegalensis 108B was stored in their respective MySQL databases. For A. ghanensis LMG 23848T, it comprised the functional annotations of 2,698 protein-encoding genes originally annotated with GenDB, extended with 160 unique protein-encoding genes from NCBI RefSeq, and 306 unique protein-encoding genes from PATRIC. For A. senegalensis 108B, it comprised the functional annotations of 3,605 protein-encoding genes originally annotated with GenDB, extended with 340 unique protein-encoding genes from NCBI RefSeq, and 625 unique protein-encoding genes from PATRIC.
The genome selection procedure resulted in 36 genomes (Table 2). Sixteen of these genomes had only a contig level quality, of which eight were type strain genomes. Based on the protein-encoding genes in these genomes, OrthoFinder predicted the presence of 6,149 orthogroups, of which 155 were orthogroups that contained at least one protein-encoding gene for each genome considered, and 98 of these orthogroups contained exactly one protein-encoding gene for each genome (so-called single-copy orthogroups).
Based on all predicted orthogroups, OrthoFinder inferred a rooted species tree (Figure 1). The outgroup genomes chosen, namely E. coli, G. oxydans 621H, and K. nataicola RZS01, were successfully placed outside the Acetobacter species subtree by OrthoFinder. Moreover, the tree locations of genomes of hereto unidentified Acetobacter species provided a first indication to which Acetobacter species they were most related to. It concerned Acetobacter sp. DsW_063 (isolated from a fruit fly) as A. nitrogenifigens, Acetobacter sp. DsW_54 (fruit fly) as A. fabarum, Acetobacter sp. JWB (fruit fly) as A. pomorum, Acetobacter sp. BCRC 14118 (African vinegar) as A. ascendens (formerly identified as A. pasteurianus), and Acetobacter sp. B6 (South Korean vinegar) as A. pasteurianus.
Figure 1. Rooted species tree inference by OrthoFinder. Branch lengths represent the average number of substitutions per site. Type strain genomes are indicated with an asterisk (*). For genomes for which the strain name is not indicated, the reader is referred to Table 2.
The predicted orthogroups were used in combination with the A. pasteurianus iAp386B454 GEM reference to evaluate the iAp386B454 GEM reaction presence or absence in 11 high-quality Acetobacter genomes. The most frequent missing reactions in the central metabolism, which has been published before for A. pasteurianus 386B (Illeghems et al., 2013; Pelicaen et al., 2019), were the ones catalyzed by proton-translocating NAD(P)+ transhydrogenase (EC 7.1.1.1; missing in A. aceti TMW2.1153, A. tropicalis BDGP1, A. pasteurianus Ab3, and A. senegalensis 108B), phosphoglucomutase (EC 5.4.2.2; missing in A. aceti TMW2.1153, A. tropicalis BDGP1, A. pasteurianus Ab3, and A. senegalensis 108B), ornithine cyclodeaminase (EC 4.3.1.12; missing in A. persici TMW2.1084, A. aceti TMW2.1153, and A. ghanensis LMG 23848T), a putative periplasmic mannitol oxidation reaction (missing in A. ascendens LMG 1590T, A. aceti TMW2.1153, and A. pasteurianus Ab3), ribose 5-phosphate isomerase (EC 5.3.1.6; missing in A. persici TMW2.1084, A. tropicalis BDGP1, and A. senegalensis 108B), and fructokinase (EC 2.7.1.4; missing in A. ascendens LMG 1590T and A. ghanensis LMG 23848T). Both phosphate acetyltransferase (EC 2.3.1.8) and acetate kinase (EC 2.7.2.1) were missing in A. aceti TMW2.1153.
Although the lactate:H+ symporter was missing in A. aceti TMW2.1153, the genome contained genes encoding a quinone-dependent (EC 1.1.5.12) and cytochrome c-dependent (EC 1.1.2.4) D-lactate dehydrogenase. This strain was originally isolated from a water kefir and other A. aceti strains have been typically found in an ethanol-rich habitat, examples including vinegar. The absence of the lactate:H+ symporter in the A. aceti TMW2.1153 genome might indicate genome evolution toward excluding lactate as a substrate, other means of lactate transport in the cell, or, possibly, the erroneous prediction of absence of a gene encoding this function. In contrast, characteristic reactions for the Acetobacter genus, including the membrane-bound pyrroloquinoline quinone (PQQ)-dependent ethanol dehydrogenase (EC 1.1.5.5) and succinyl-CoA:acetate CoA-transferase (EC 2.8.3.18) were found in all high-quality Acetobacter genomes considered.
Combining the predicted orthogroups and the iAp386B454 GEM allowed to reconstruct GEMs for A. ghanensis LMG 23848T and A. senegalensis 108B. GEM compositions before and after curation-based gap filling are shown in Table 3. The concomitant increase of the number of reactions and decrease of the number of dead-end metabolites indicate a successful gap filling process. A complication arose in this analysis due to the occurrence of twelve A. pasteurianus 386B gene identifiers in the iAp386B454 GEM, which were not present in the A. pasteurianus 386B NCBI RefSeq genome version used for the OrthoFinder analysis. The 10 reactions associated to these genes were computationally filtered and manually checked to see whether the presumed orthologs were present in the A. ghanensis LMG 23848T and A. senegalensis 108B genomes, and if so, the reactions and associated gene-reaction rules were manually added to their respective GEMs. This was the case for D-glucose:ubiquinone oxidoreductase (EC 1.1.5.2), phosphoenolpyruvate carboxylase (EC 4.1.1.31), and 3-isopropylmalate dehydratase (EC 4.2.1.33), both for A. ghanensis LMG 23848T and A. senegalensis 108B.
Table 3. Composition of genome-scale metabolic models (GEMs) of Acetobacter ghanensis LMG 23848T and Acetobacter senegalensis 108B after automated reconstruction and curation-based gap filling.
For A. ghanensis LMG 23848T, a quinone-dependent D-lactate dehydrogenase (EC 1.1.5.12) was absent in the genome, but a cytochrome c-dependent enzyme (EC 1.1.2.4) was identified. No acetolactate decarboxylase (EC 4.1.1.5) was found in the A. ghanensis LMG 23848T genome. Instead, a gene encoding diacetyl reductase (EC 1.1.1.303) was present. Finally, no quinone-dependent glycerol 3-phosphate dehydrogenase (EC 1.1.5.3) was found. Whereas for A. senegalensis 108B no proton-translocating NAD(P)+ transhydrogenase (EC 7.1.1.1) was found in the genome, a soluble NAD(P)+ transhydrogenase (EC 1.6.1.1) was present. Additionally, no gene could be found encoding gluconokinase (EC 2.7.1.12), possibly explaining why A. senegalensis 108B could not grow under defined medium conditions with glucose as the sole carbon source. The fact that citrate could sustain growth of this strain paved the way to find a genomic cause. Indeed, a putative citrate:H+ symporter and a gene cluster encoding citrate lyase was found in the genome, as previously reported (Illeghems et al., 2016). The gene cluster encoding citrate lyase was also present in two Acetobacter species isolated from fruit or fruit-derived fermented foods, namely A. persici JCM 25330T (peach fruit) and A. malorum CECT 7742 (strawberry vinegar). Finally, the A. senegalensis 108B genome encoded a phosphoenolpyruvate carboxykinase (EC 4.1.1.49), which was not found in A. pasteurianus 386B, thus providing another anaplerotic link between the TCA cycle and the gluconeogenesis pathway in A. senegalensis 108B.
The results of the growth experiments for A. ghanensis LMG 23848T and A. senegalensis 108B were used to fill the reaction gaps in these models using the iAp386B454 GEM as a reaction repository. The FBA gap filling results indicated that 17 iAp386B454 GEM reactions were needed to obtain in silico growth of A. ghanensis LMG 23848T with glucose as the sole carbon source. For A. senegalensis 108B, 10 iAp386B454 GEM reactions were necessary when growth was required with lactic acid as the sole carbon source. Manual curation allowed the addition of all but two reactions for A. ghanensis LMG 23848T, for which no genomic evidence could be found. These reactions were the ones catalyzed by asparagine synthase (EC 6.3.5.4) and ornithine cyclodeaminase (EC 4.3.1.12). Both are involved in amino acid biosynthesis, the first being necessary for L-asparagine biosynthesis and the latter responsible for L-proline biosynthesis. The gene encoding the alternative L-proline biosynthesis enzyme NAD(P)-dependent pyrroline-5-carboxylate reductase (EC 1.5.1.2) was annotated with an internal stop codon in the NCBI RefSeq annotation. Other biosynthesis pathways for these amino acids may exist in A. ghanensis LMG 23848T, since in vitro growth was obtained under defined medium conditions without the addition of amino acids. Conversely, for A. senegalensis 108B, genomic evidence was found for all gap filling results. However, this evidence had to be retrieved from genes in the NCBI RefSeq annotation, for which no amino acid sequence was available (e.g., due to a predicted frameshift) or genes only annotated in the GenDB or PATRIC genome annotation versions.
Flux balance analysis with the iAg23848 GEM on glucose as the sole carbon source did not result in growth, unless L-proline and L-asparagine were additionally available, in which case the predicted specific growth rate was 0.70 h−1 and the consumption of both amino acids was balanced by the protein biosynthesis reaction. The flux distribution revealed obligatory NADPH oxidation by the proton-translocating NAD(P)+ transhydrogenase. However, genome annotation analysis did not support the presence of this reaction, since the A. ghanensis LMG 23848T genome missed the 𝛽-subunit of this enzyme. Growth of A. ghanensis LMG 23848T under defined medium conditions with glucose was only found when a citrate buffer was used. Allowing additional in silico consumption of citrate showed that constraining the proton-translocating NAD(P)+ transhydrogenase did not prevent growth, resulting in the prediction of a specific growth rate of 0.58 h−1 with the glutamate synthase reaction (EC 1.4.1.13) as the largest NADPH consumer.
The iAs108B GEM was solved with FBA under two growth conditions, namely with lactic acid or with citric acid as the sole carbon sources. No additional nutrients had to be added to obtain in silico growth, suggesting that this model contained all reactions necessary for biomass formation. FBA with lactic acid resulted in a specific growth rate of 0.86 h−1. The soluble NAD(P)+ transhydrogenase produced NADPH, whereas phosphoenolpyruvate carboxykinase supplied all necessary phosphoenolpyruvate. FBA with citric acid resulted in a specific growth rate of 0.75 h−1. Most of the citric acid consumed was directly used in the TCA cycle, whereas only 12% was consumed by citrate lyase. No acetate was produced by the model; all intracellular acetate produced was consumed by succinyl-CoA:acetate CoA-transferase.
The presence of genes encoding the glyoxylate cycle enzymes was assessed in all 36 Acetobacter genomes examined. Presence/absence was compared to experimental data related to the growth of the strains in a defined medium containing ethanol as the sole carbon source and obtained for the type strains of a number of Acetobacter species (Table 4; Cleenwerck et al., 2008). For strains of eight out of 19 Acetobacter species that were able to grow, three species (A. estunensis, A. peroxydans, and A. lovaniensis) had at the time of analysis no genome sequenced, two species for which a genome sequence was available (A. aceti and A. nitrogenifigens) encoded the glyoxylate cycle, and finally, three species with a genome sequence available (A. senegalensis, A. cibinongensis, and A. fabarum) did not encode the glyoxylate cycle. The latter three species could thus correspond to false positive experimental results based on growth with ethanol as the sole carbon source that were obtained formerly (Table 4) and should hence be repeated, albeit that the genome sequence available for A. fabarum is not that from the type strain.
Table 4. Overview of the presence of the glyoxylate cycle enzymes in genomes of strains of Acetobacter species.
The enzymes involved in the aerobic respiratory chain as known for E. coli were present in all Acetobacter genomes considered, comprising NADH dehydrogenase type I (EC 7.1.1.2), NADH dehydrogenase type II (EC 1.6.5.9), cytochrome bo3 oxidase (EC 7.1.1.3), and cytochrome bd oxidase (EC 7.1.1.7). Concerning NADPH metabolism, E. coli contains both a proton-translocating NAD(P)+ transhydrogenase and a soluble NAD(P)+ transhydrogenase. From the comparative genomic analysis, it was apparent that, at least for the high-quality Acetobacter genomes, the presence of these enzymes was mutually exclusive. An interesting exception was A. aceti, for which both reactions were predicted to be present.
The reconstruction of a GEM for A. ghanensis LMG 23848T and A. senegalensis 108B, two candidate starter culture strains for cocoa fermentation (Illeghems et al., 2016), provided in silico tools to gain insights into the metabolic properties of these strains. In the current study, the GEM reconstruction process was accelerated using a semi-automated approach with experimental support. Growth experiments under defined medium conditions identified individual carbon sources that allowed the growth of A. ghanensis LMG 23848T and A. senegalensis 108B. These growth conditions could then be used in simulations using FBA with the newly reconstructed iAg23848 and iAs108B GEMs, providing insights into the metabolic flux distributions of the models.
For A. senegalensis 108B, the prediction of citrate assimilation based on a previous genome analysis (Illeghems et al., 2016) was confirmed. In general, conversion of citrate present in the cocoa pulp-bean mass is ascribed to lactic acid bacteria that proliferate at the start of a cocoa fermentation process (De Vuyst and Weckx, 2016; De Vuyst and Leroy, 2020). Citrate consumption by AAB species may contribute to their survival during this more or less anaerobic stage of a cocoa fermentation process (Illeghems et al., 2016). For A. ghanensis LMG 23848T, the absence of a quinone-dependent D-lactate dehydrogenase (EC 1.1.5.12) was probably compensated by the presence of a cytochrome c-dependent enzyme (EC 1.1.2.4), which probably allowed lactate consumption. However, this may be an explanation for the lower lactate consumption rate of A. ghanensis LMG 23848T, compared to A. pasteurianus 386B, under cocoa pulp-simulating conditions (Moens et al., 2014). In general, both lactate and acetate are overoxidized during a late stage of a cocoa fermentation process (Moens et al., 2014; De Vuyst and Weckx, 2016; De Vuyst and Leroy, 2020). Additionally, no acetolactate decarboxylase (EC 4.1.1.5) was found in the A. ghanensis LMG 23848T genome, although acetoin was formed (Moens et al., 2014). In A. pasteurianus, it has been shown that acetoin production solely depends on acetolactate decarboxylase, in contrast to the theoretically proposed acetaldehyde carboligation pathway, as found in Saccharomyces cerevisiae (De Ley, 1959; Gunawan et al., 2007). However, in A. ghanensis LMG 23848T as well as in A. senegalensis 108B, yet another pathway may be responsible for acetoin formation, since in both genomes a gene encoding diacetyl reductase (EC 1.1.1.303) was present, as is the case in lactic acid bacteria (Keenan and Lindsay, 1968). Finally, no quinone-dependent glycerol 3-phosphate dehydrogenase (EC 1.1.5.3) could be found, possibly explaining why A. ghanensis LMG 23848T could not grow under defined medium conditions with glycerol as the sole carbon source.
Re-annotation of the genomes of A. ghanensis LMG 23848T and A. senegalensis 108B proved to be critical to reconstruct their GEMs. Reaction presence predictions using the iAp386B454 GEM as a reference were successful, but led to metabolic network gaps in essential biosynthesis reactions. Without the current re-annotation effort, it would be impossible to speculate about their presence. Keeping this in mind, the mutual occurrence of genome sequence errors in query and reference genomes blurs the outcome of this functional comparative genomic analysis, since it is ultimately based on the prediction of the presence or absence of genes in the genomes considered. However, considering that genomic data are the only well-structured and publicly available data source at hand to compare the metabolic properties of Acetobacter species, this analysis may provide interesting insights for future research. The predicted differences in the carbon and redox metabolic potential of the Acetobacter species investigated revealed the possible metabolic diversity in this genus. Specifically, the presence or absence of the lactate:H+ symporter, fructokinase, gluconokinase, ribose 5-phosphate isomerase, and citrate lyase may represent adaptations to different habitats and niches. Also, the presence or absence of a proton-translocating and soluble NAD(P)+ transhydrogenase may represent an important and hitherto not sufficiently explored adaptation to the use of different carbon sources and their influence on the redox metabolism of Acetobacter species (Pelicaen et al., 2020). In E. coli, both enzymes are present, each having a specific functional role, whereby the first enzyme produces NADPH and the latter re-oxidizes excess NADPH (Sauer et al., 2004). The complete coverage of the key reactions of the aerobic respiratory chain among the Acetobacter genomes is a strong indication that this metabolic feature is a defining physiological factor of this genus.
The predicted presence (e.g., A. aceti and A. nitrogenifigens) or absence (e.g., A. ghanensis, A. senegalensis, and A. pasteurianus) of the glyoxylate cycle enzymes represented an interesting case where genomic and experimental evidence go hand in hand. Alternative pathways have been described for the assimilation of C2 compounds in microorganisms, namely the ethylmalonyl-CoA pathway and the methylaspartate cycle (Alber et al., 2006; Khomyakova et al., 2011). However, these pathways have not been found in AAB, and no genomic evidence for their presence could be found in the Acetobacter genomes considered. If the glyoxylate cycle is the only C2 assimilation pathway present in species of the genus Acetobacter, then the phenotypic test concerning the growth of an Acetobacter species in a medium with ethanol as the sole carbon source, commonly used to infer the taxonomy of an unknown Acetobacter strain, should be backed by genomic evidence. The results presented here indicate that this was indeed not the case for some Acetobacter species, thus questioning experimental data (Cleenwerck et al., 2008). Acetobacter senegalensis 108B was not able to grow under defined medium conditions with ethanol as the sole carbon source. In addition, no genomic evidence was found for the glyoxylate cycle in the re-annotated A. senegalensis 108B genome. The experimental results presented here are in contrast to previous results obtained for A. senegalensis 108B and for the type strain of A. senegalensis (Camu et al., 2007; Ndoye et al., 2007). Thus, this is an argument that genomic evidence should be taken into account for future species descriptions of members of the Acetobacter genus.
From a practical viewpoint, in fermentation processes such as vinegar production, the use of strains that can solely oxidize ethanol to acetic acid, but not assimilate it into biomass components, may be favorable (Mullins and Kappock, 2013; Sakurai et al., 2013). Similarly, for the fermentation of cocoa pulp-bean mass, the absence of the glyoxylate cycle in the genomes of A. ghanensis LMG 23848T, A. senegalensis 108B, and A. pasteurianus 386B (Illeghems et al., 2013; Pelicaen et al., 2019), may represent a competitive advantage of these strains to quickly oxidize the available ethanol, produced by yeast species, to acetic acid. Genomic screening of strains may provide a more straightforward strategy to select those strains for which no evidence of the glyoxylate cycle can be found, to use them as starter cultures in such fermentation processes.
In the present study, the possibility of integrating two different data structures based on the same genomic data source was explored. A first data structure comprises GEMs, allowing to catalogue the entire biochemical reaction potential of a microbial strain and in silico metabolic flux simulations. Also, gene-reaction rules, manually curated or not, allow to explore the causal links between the genome and the reactome. A second data structure, the orthogroups, predicts the phylogenetic relatedness between protein-encoding genes from different species genomes, from which estimates of their shared function can be made. Combining the manually curated gene-reaction rules of a GEM of A. pasteurianus 386B and E. coli and a set of predicted orthogroups of a selection of Acetobacter genomes allowed to predict the presence of reactions that characterized the metabolism of A. pasteurianus 386B and E. coli for these Acetobacter species. Evaluation of biochemical reaction presence in bacterial genomes represents a new frontier in genome annotation, since this evaluation is a step closer to the actual biological functionalities that the genome encodes for. The results obtained stress the need for proper data curation and species description.
The datasets presented in this study can be found in online repositories. The genome-scale metabolic models in xml format can be found at https://zenodo.org under submission number 6320681 or under DOI at https://doi.org/10.5281/zenodo.6320681.
RP carried out the research and drafted the manuscript. DG, SW, and LDV supervised the research and revised and edited the manuscript. All authors approved the manuscript.
The authors acknowledge financial support from the Research Council of the Vrije Universiteit Brussel (SRP7 and IOF342 projects). RP was the recipient of a PhD Fellowship strategic basic research of the Research Foundation Flanders (FWO-Vlaanderen; 1S27316N).
The authors declare that the research was conducted in the absence of any commercial or financial relationships that could be construed as a potential conflict of interest.
All claims expressed in this article are solely those of the authors and do not necessarily represent those of their affiliated organizations, or those of the publisher, the editors and the reviewers. Any product that may be evaluated in this article, or claim that may be made by its manufacturer, is not guaranteed or endorsed by the publisher.
Alber, B. E., Spanheimer, R., Ebenau-Jehle, C., and Fuchs, G. (2006). Study of an alternate glyoxylate cycle for acetate assimilation by Rhodobacter sphaeroides. Mol. Microbiol. 61, 297–309. doi: 10.1111/j.1365-2958.2006.05238.x
Arkin, A. P., Cottingham, R. W., Henry, C. S., Harris, N. L., Stevens, R. L., Maslov, S., et al. (2018). KBase: the United States Department of Energy Systems Biology Knowledgebase. Nat. Biotechnol. 36, 566–569. doi: 10.1038/nbt.4163
Brettin, T., Davis, J. J., Disz, T., Edwards, R. A., Gerdes, S., Olsen, G. J., et al. (2015). RASTtk: a modular and extensible implementation of the RAST algorithm for building custom annotation pipelines and annotating batches of genomes. Sci. Rep. 5:8365. doi: 10.1038/srep08365
Camu, N., De Winter, T., Verbrugghe, K., Cleenwerck, I., Vandamme, P., Takrama, J. S., et al. (2007). Dynamics and biodiversity of populations of lactic acid bacteria and acetic acid bacteria involved in spontaneous heap fermentation of cocoa beans in Ghana. Appl. Environ. Microbiol. 73, 1809–1824. doi: 10.1128/AEM.02189-06
Claudel-Renard, C., Chevalet, C., Faraut, T., and Kahn, D. (2003). Enzyme-specific profiles for genome annotation: PRIAM. Nucleic Acids Res. 31, 6633–6639. doi: 10.1093/nar/gkg847
Cleenwerck, I., and De Vos, P. (2008). Polyphasic taxonomy of acetic acid bacteria: an overview of the currently applied methodology. Int. J. Food Microbiol. 125, 2–14. doi: 10.1016/j.ijfoodmicro.2007.04.017
Cleenwerck, I., Gonzalez, A., Camu, N., Engelbeen, K., De Vos, P., and De Vuyst, L. (2008). Acetobacter fabarum sp. nov., an acetic acid bacterium from a Ghanaian cocoa bean heap fermentation. Int. J. Syst. Evol. Microbiol. 58, 2180–2185. doi: 10.1099/ijs.0.65778-0
Cleenwerck, I., Vandemeulebroecke, K., Janssens, D., and Swings, J. (2002). Re-examination of the genus Acetobacter, with descriptions of Acetobacter cerevisiae sp. nov. and Acetobacter malorum sp. nov. Int. J. Syst. Evol. Microbiol. 52, 1551–1558. doi: 10.1099/00207713-52-5-1551
Coton, M., Pawtowski, A., Taminiau, B., Burgaud, G., Deniel, F., Coulloumme-Labarthe, L., et al. (2017). Unraveling microbial ecology of industrial-scale kombucha fermentations by metabarcoding and culture-based methods. FEMS Microbiol. Ecol. 93:5. doi: 10.1093/femsec/fix048
De Ley, J. (1959). On the formation of acetoin by Acetobacter. J. Gen. Microbiol. 21, 352–365. doi: 10.1099/00221287-21-2-352
De Vuyst, L., Camu, N., De Winter, T., Vandemeulebroecke, K., Van de Perre, V., Vancanneyt, M., et al. (2008). Validation of the (GTG)5-rep-PCR fingerprinting technique for rapid classification and identification of acetic acid bacteria, with a focus on isolates from Ghanaian fermented cocoa beans. Int. J. Food Microbiol. 125, 79–90. doi: 10.1016/j.ijfoodmicro.2007.02.030
De Vuyst, L., and Leroy, F. (2020). Functional role of yeasts, lactic acid bacteria, and acetic acid bacteria in cocoa fermentation processes. FEMS Microbiol. Rev. 44, 432–453. doi: 10.1093/femsre/fuaa014
De Vuyst, L., and Weckx, S. (2016). The cocoa bean fermentation process: from ecosystem analysis to starter culture development. J. Appl. Microbiol. 121, 5–17. doi: 10.1111/jam.13045
Díaz-Muñoz, C., Van de Voorde, D., Comasio, A., Verce, M., Hernandez, C. E., Weckx, S., et al. (2021). Curing of cocoa beans: fine-scale monitoring of the starter cultures applied and metabolomics of the fermentation and drying steps. Front. Microbiol. 11:616875. doi: 10.3389/fmicb.2020.616875
Ebrahim, A., Lerman, J. A., Palsson, B. Ø., and Hyduke, D. R. (2013). COBRApy: COnstraints-based reconstruction and analysis for python. BMC Syst. Biol. 7:74. doi: 10.1186/1752-0509-7-74
Elbourne, L. D. H., Tetu, S. G., Hassan, K. A., and Paulsen, I. T. (2017). TransportDB 2.0: a database for exploring membrane transporters in sequenced genomes from all domains of life. Nucleic Acids Res. 45, D320–D324. doi: 10.1093/nar/gkw1068
Emms, D. M., and Kelly, S. (2015). OrthoFinder: solving fundamental biases in whole genome comparisons dramatically improves orthogroup inference accuracy. Genome Biol. 16:157. doi: 10.1186/s13059-015-0721-2
Emms, D. M., and Kelly, S. (2017). STRIDE: species tree root inference from gene duplication events. Mol. Biol. Evol. 34, 3267–3278. doi: 10.1093/molbev/msx259
Emms, D. M., and Kelly, S. (2018). STAG: species tree inference from all genes. bioRxiv. doi: 10.1101/267914
Gu, C., Kim, G. B., Kim, W. J., Kim, H. U., and Lee, S. Y. (2019). Current status and applications of genome-scale metabolic models. Genome Biol. 20:121. doi: 10.1186/s13059-019-1730-3
Gunawan, C., Satianegara, G., Chen, A. K., Breuer, M., Hauer, B., Rogers, P. L., et al. (2007). Yeast pyruvate decarboxylases: variation in biocatalytic characteristics for (R)-phenylacetylcarbinol production. FEMS Yeast Res. 7, 33–39. doi: 10.1111/j.1567-1364.2006.00138.x
Huerta-Cepas, J., Forslund, K., Coelho, L. P., Szklarczyk, D., Jensen, L. J., von Mering, C., et al. (2017). Fast genome-wide functional annotation through orthology assignment by eggNOG-mapper. Mol. Biol. Evol. 34, 2115–2122. doi: 10.1093/molbev/msx148
Illeghems, K., De Vuyst, L., and Weckx, S. (2013). Complete genome sequence and comparative analysis of Acetobacter pasteurianus 386B, a strain well-adapted to the cocoa bean fermentation ecosystem. BMC Genomics 14:526. doi: 10.1186/1471-2164-14-526
Illeghems, K., Pelicaen, R., De Vuyst, L., and Weckx, S. (2016). Assessment of the contribution of cocoa-derived strains of Acetobacter ghanensis and Acetobacter senegalensis to the cocoa bean fermentation process through a genomic approach. Food Microbiol. 58, 68–78. doi: 10.1016/j.fm.2016.03.013
Jones, P., Binns, D., Chang, H.-Y., Fraser, M., Li, W., McAnulla, C., et al. (2014). InterProScan 5: genome-scale protein function classification. Bioinformatics 30, 1236–1240. doi: 10.1093/bioinformatics/btu031
Kanehisa, M., Furumichi, M., Tanabe, M., Sato, Y., and Morishima, K. (2017). KEGG: new perspectives on genomes, pathways, diseases and drugs. Nucleic Acids Res. 45, D353–D361. doi: 10.1093/nar/gkw1092
Kanehisa, M., Sato, Y., and Morishima, K. (2016). BlastKOALA and GhostKOALA: KEGG tools for functional characterization of genome and metagenome sequences. J. Mol. Biol. 428, 726–731. doi: 10.1016/j.jmb.2015.11.006
Keenan, T. W., and Lindsay, R. C. (1968). Diacetyl production and utilization by lactobacillus species. J. Dairy Sci. 51, 188–191. doi: 10.3168/jds.S0022-0302(68)86948-6
Keseler, I. M., Mackie, A., Santos-Zavaleta, A., Billington, R., Bonavides-Martínez, C., Caspi, R., et al. (2017). The EcoCyc database: reflecting new knowledge about Escherichia coli K-12. Nucleic Acids Res. 45, D543–D550. doi: 10.1093/nar/gkw1003
Khomyakova, M., Bukmez, O., Thomas, L. K., Erb, T. J., and Berg, I. A. (2011). A methylaspartate cycle in Haloarchaea. Science 331, 334–337. doi: 10.1126/science.1196544
Lefeber, T., Janssens, M., Camu, N., and De Vuyst, L. (2010). Kinetic analysis of strains of lactic acid bacteria and acetic acid bacteria in cocoa pulp simulation media toward development of a starter culture for cocoa bean fermentation. Appl. Environ. Microbiol. 76, 7708–7716. doi: 10.1128/AEM.01206-10
Lefeber, T., Janssens, M., Moens, F., Gobert, W., and De Vuyst, L. (2011). Interesting starter culture strains for controlled cocoa bean fermentation revealed by simulated cocoa pulp fermentations of cocoa-specific lactic acid bacteria. Appl. Environ. Microbiol. 77, 6694–6698. doi: 10.1128/AEM.00594-11
Lefeber, T., Papalexandratou, Z., Gobert, W., Camu, N., and De Vuyst, L. (2012). On-farm implementation of a starter culture for improved cocoa bean fermentation and its influence on the flavour of chocolates produced thereof. Food Microbiol. 30, 379–392. doi: 10.1016/j.fm.2011.12.021
Li, L., Cleenwerck, I., De Vuyst, L., and Vandamme, P. (2017). Identification of acetic acid bacteria through matrix-assisted laser desorption/ionization time-of-flight mass spectrometry and report of Gluconobacter nephelii Kommanee et al. 2011 and Gluconobacter uchimurae Tanasupawat et al. 2012 as later heterotypic synonyms of Gluconobacter japonicus Malimas et al. 2009 and Gluconobacter oxydans (Henneberg 1897) De Ley 1961 (approved lists 1980) emend. Gosselé et al. 1983, respectively. Syst. Appl. Microbiol. 40, 123–134. doi: 10.1016/j.syapm.2017.01.003
Lombard, V., Golaconda Ramulu, H., Drula, E., Coutinho, P. M., and Henrissat, B. (2014). The carbohydrate-active enzymes database (CAZy) in 2013. Nucleic Acids Res. 42, D490–D495. doi: 10.1093/nar/gkt1178
Lu, Z.-M., Wang, Z.-M., Zhang, X.-J., Mao, J., Shi, J.-S., and Xu, Z.-H. (2018). Microbial ecology of cereal vinegar fermentation: insights for driving the ecosystem function. Curr. Opin. Biotechnol. 49, 88–93. doi: 10.1016/j.copbio.2017.07.006
Machado, D., Herrgård, M. J., and Rocha, I. (2016). Stoichiometric representation of gene-protein-reaction associations leverages constraint-based analysis from reaction to gene-level phenotype prediction. PLoS Comput. Biol. 12:e1005140. doi: 10.1371/journal.pcbi.1005140
Meurer, A., Smith, C. P., Paprocki, M., Čertík, O., Kirpichev, S. B., Rocklin, M., et al. (2017). SymPy: symbolic computing in python. Peer J. Comp. Sci. 3:e103. doi: 10.7717/peerj-cs.103
Meyer, F. (2003). GenDB - an open source genome annotation system for prokaryote genomes. Nucleic Acids Res. 31, 2187–2195. doi: 10.1093/nar/gkg312
Moens, F., Lefeber, T., and De Vuyst, L. (2014). Oxidation of metabolites highlights the microbial interactions and role of Acetobacter pasteurianus during cocoa bean fermentation. Appl. Environ. Microbiol. 80, 1848–1857. doi: 10.1128/AEM.03344-13
Mullins, E. A., and Kappock, T. J. (2013). Functional analysis of the acetic acid resistance (aar) gene cluster in Acetobacter aceti strain 1023. Acetic Acid Bacteria 2:e3. doi: 10.4081/aab.2013.s1.e3
Ndoye, B., Cleenwerck, I., Engelbeen, K., Dubois-Dauphin, R., Guiro, A. T., Van Trappen, S., et al. (2007). Acetobacter senegalensis sp. nov., a thermotolerant acetic acid bacterium isolated in Senegal (sub-Saharan Africa) from mango fruit (Mangifera indica L.). Int. J. Syst. Evol. Microbiol. 57, 1576–1581. doi: 10.1099/ijs.0.64678-0
Orth, J. D., Conrad, T. M., Na, J., Lerman, J. A., Nam, H., Feist, A. M., et al. (2011). A comprehensive genome-scale reconstruction of Escherichia coli metabolism. Mol. Syst. Biol. 7:535. doi: 10.1038/msb.2011.65
Overbeek, R., Olson, R., Pusch, G. D., Olsen, G. J., Davis, J. J., Disz, T., et al. (2014). The SEED and the rapid annotation of microbial genomes using subsystems technology (RAST). Nucleic Acids Res. 42, D206–D214. doi: 10.1093/nar/gkt1226
Papalexandratou, Z., Cleenwerck, I., De Vos, P., and De Vuyst, L. (2009). (GTG)5-PCR reference framework for acetic acid bacteria. FEMS Microbiol. Lett. 301, 44–49. doi: 10.1111/j.1574-6968.2009.01792.x
Papalexandratou, Z., Lefeber, T., Bahrim, B., Lee, O. S., Daniel, H.-M., and De Vuyst, L. (2013). Hanseniaspora opuntiae, Saccharomyces cerevisiae, lactobacillus fermentum, and Acetobacter pasteurianus predominate during well-performed Malaysian cocoa bean box fermentations, underlining the importance of these microbial species for a successful cocoa bean fermentation process. Food Microbiol. 35, 73–85. doi: 10.1016/j.fm.2013.02.015
Pelicaen, R., Gonze, D., De Vuyst, L., and Weckx, S. (2020). Genome-scale metabolic modeling of Acetobacter pasteurianus 386B reveals its metabolic adaptation to cocoa fermentation conditions. Food Microbiol. 92:103597. doi: 10.1016/j.fm.2020.103597
Pelicaen, R., Gonze, D., Teusink, B., De Vuyst, L., and Weckx, S. (2019). Genome-scale metabolic reconstruction of Acetobacter pasteurianus 386B, a candidate functional starter culture for cocoa bean fermentation. Front. Microbiol. 10:2801. doi: 10.3389/fmicb.2019.02801
Prigent, S., Nielsen, J. C., Frisvad, J. C., and Nielsen, J. (2018). Reconstruction of 24 Penicillium genome-scale metabolic models shows diversity based on their secondary metabolism. Biotechnol. Bioeng. 115, 2604–2612. doi: 10.1002/bit.26739
Saeki, A., Taniguchi, M., Matsushita, K., Toyama, H., Theeragool, G., Lotong, N., et al. (1997). Microbiological aspects of acetate oxidation by acetic acid bacteria, unfavorable phenomena in vinegar fermentation. Biosci. Biotech. Bioch. 61, 317–323. doi: 10.1271/bbb.61.317
Sakurai, K., Yamazaki, S., Ishii, M., Igarashi, Y., and Arai, H. (2013). Role of the glyoxylate pathway in acetic acid production by Acetobacter aceti. J. Biosci. Bioeng. 115, 32–36. doi: 10.1016/j.jbiosc.2012.07.017
Sauer, U., Canonaco, F., Heri, S., Perrenoud, A., and Fischer, E. (2004). The soluble and membrane-bound transhydrogenases UdhA and PntAB have divergent functions in NADPH metabolism of Escherichia coli. J. Biol. Chem. 279, 6613–6619. doi: 10.1074/jbc.M311657200
Seif, Y., Kavvas, E., Lachance, J.-C., Yurkovich, J. T., Nuccio, S.-P., Fang, X., et al. (2018). Genome-scale metabolic reconstructions of multiple salmonella strains reveal serovar-specific metabolic traits. Nat. Commun. 9:3771. doi: 10.1038/s41467-018-06112-5
Serres, M. H., and Riley, M. (2006). Genomic analysis of carbon source metabolism of Shewanella oneidensis MR-1: predictions versus experiments. J. Bacteriol. 188, 4601–4609. doi: 10.1128/JB.01787-05
Shinjoh, M., and Toyama, H. (2016). “Industrial applications of acetic acid bacteria (vitamin C and others)” in Acetic Acid Bacteria Ecology and Physiology. eds. K. Matsushita, H. Toyama, N. Tonouchi, and A. Okamoto-Kainuma (Tokyo, Japan: Springer)
Tatusova, T., DiCuccio, M., Badretdin, A., Chetvernin, V., Nawrocki, E. P., Zaslavsky, L., et al. (2016). NCBI prokaryotic genome annotation pipeline. Nucleic Acids Res. 44, 6614–6624. doi: 10.1093/nar/gkw569
The UniProt Consortium (2019). UniProt: a worldwide hub of protein knowledge. Nucleic Acids Res. 47, D506–D515. doi: 10.1093/nar/gky1049
Thiele, I., and Palsson, B. Ø. (2010). A protocol for generating a high-quality genome-scale metabolic reconstruction. Nat. Protoc. 5, 93–121. doi: 10.1038/nprot.2009.203
Verduyn, C., Postma, E., Scheffers, W. A., and Van Dijken, J. P. (1992). Effect of benzoic acid on metabolic fluxes in yeasts: a continuous-culture study on the regulation of respiration and alcoholic fermentation. Yeast 8, 501–517. doi: 10.1002/yea.320080703
Wattam, A. R., Davis, J. J., Assaf, R., Boisvert, S., Brettin, T., Bun, C., et al. (2017). Improvements to PATRIC, the all-bacterial bioinformatics database and analysis resource center. Nucleic Acids Res. 45, D535–D542. doi: 10.1093/nar/gkw1017
Yamada, Y. (2016). “Systematics of acetic acid bacteria” in Acetic Acid Bacteria Ecology and Physiology. eds. K. Matsushita, H. Toyama, N. Tonouchi, and A. Okamoto-Kainuma (Tokyo, Japan: Springer)
Keywords: cocoa fermentation, Acetobacter metabolism, comparative genomics, genome-scale metabolic modelling, flux balance analysis
Citation: Pelicaen R, Weckx S, Gonze D and De Vuyst L (2022) Application of comparative genomics of Acetobacter species facilitates genome-scale metabolic reconstruction of the Acetobacter ghanensis LMG 23848T and Acetobacter senegalensis 108B cocoa strains. Front. Microbiol. 13:1060160. doi: 10.3389/fmicb.2022.1060160
Received: 02 October 2022; Accepted: 04 November 2022;
Published: 24 November 2022.
Edited by:
Maria Gullo, University of Modena and Reggio Emilia, ItalyReviewed by:
Salvatore La China, University of Modena and Reggio Emilia, ItalyCopyright © 2022 Pelicaen, Weckx, Gonze and De Vuyst. This is an open-access article distributed under the terms of the Creative Commons Attribution License (CC BY). The use, distribution or reproduction in other forums is permitted, provided the original author(s) and the copyright owner(s) are credited and that the original publication in this journal is cited, in accordance with accepted academic practice. No use, distribution or reproduction is permitted which does not comply with these terms.
*Correspondence: Luc De Vuyst, bHVjLmRlLnZ1eXN0QHZ1Yi5iZQ==
Disclaimer: All claims expressed in this article are solely those of the authors and do not necessarily represent those of their affiliated organizations, or those of the publisher, the editors and the reviewers. Any product that may be evaluated in this article or claim that may be made by its manufacturer is not guaranteed or endorsed by the publisher.
Research integrity at Frontiers
Learn more about the work of our research integrity team to safeguard the quality of each article we publish.