- 1National Institute for Infectious Diseases, INMI “Lazzaro Spallanzani”, IRCCS, Rome, Italy
- 2Biostatistic Unit, Fondazione-IRCCS “Casa Sollievo della Sofferenza” Hospital, San Giovanni Rotondo, FG, Italy
- 3Cancer Stem Cells Unit, Institute for Stem Cell Biologyl, Regenerative Medicine and Innovative Therapeutics (ISBReMIT), Fondazione-IRCCS “Casa Sollievo della Sofferenza” Hospital, Opera di San Pio da Pietrelcina, San Giovanni Rotondo, FG, Italy
- 4Division of Gastroenterology, Fondazione-IRCCS “Casa Sollievo della Sofferenza” Hospital, San Giovanni Rotondo, FG, Italy
Background and aim: COVID-19, the infectious disease caused by SARS-CoV-2 virus that has been causing a severe pandemic worldwide for more than 2 years, is characterized by a high heterogeneity of clinical presentations and evolution and, particularly, by a varying severity of respiratory involvement. This study aimed to analyze the diversity and taxonomic composition of the gut microbiota at hospital admission, in order to evaluate its association with COVID-19 outcome. In particular, the association between gut microbiota and a combination of several clinical covariates was analyzed in order to characterize the bacterial signature associate to mild or severe symptoms during the SARS-CoV-2 infection.
Materials and methods: V3–V4 hypervariable region of 16S rRNA gene sequencing of 97 rectal swabs from a retrospective cohort of COVID-19 hospitalized patients was employed to study the gut microbiota composition. Patients were divided in two groups according to their outcome considering the respiratory supports they needed during hospital stay: (i) group “mild,” including 47 patients with a good prognosis and (ii) group “severe,” including 50 patients who experienced a more severe disease due to severe respiratory distress that required non-invasive or invasive ventilation. Identification of the clusters of bacterial population between patients with mild or severe outcome was assessed by PEnalized LOgistic Regression Analysis (PELORA).
Results: Although no changes for Chao1 and Shannon index were observed between the two groups a significant greater proportion of Campylobacterota and Actinobacteriota at phylum level was found in patients affected by SARS-CoV-2 infection who developed a more severe disease characterized by respiratory distress requiring invasive or non-invasive ventilation. Clusters have been identified with a useful early potential prognostic marker of the disease evolution.
Discussion: Microorganisms residing within the gut of the patients at hospital admission, were able to significantly discriminate the clinical evolution of COVID-19 patients, in particular who will develop mild or severe respiratory involvement. Our data show that patients affected by SARS-CoV-2 with mild or severe symptoms display different gut microbiota profiles which can be exploited as potential prognostic biomarkers paving also the way to new integrative therapeutic approaches.
Introduction
COVID-19 is an infectious disease caused by the Severe Acute Respiratory Syndrome Coronavirus-2 (SARS-CoV-2). It is mainly a respiratory infection characterized by a high heterogeneity in clinical evolution, with very different clinical presentations, ranging from asymptomatic or paucisymptomatic forms in most cases, to severe forms with fatal respiratory compromise (Ochani et al., 2021).
Up to date, COVID-19 severity have been associated with several risk or prognostic factors, such as comorbidities, age, sex, genetic factors, and geographical location but, predicting whether an individual will require hospitalization or respiratory support, or can recover at home, still represents a hard challenge (Yao et al., 2022). Clearly, there are likely several other factors that determine how COVID-19 evolves in the individual and they may play a role in determining disease manifestations, such as the need for respiratory support. Among these factors gut microbiota could be a valid factor to consider (Dhar and Mohanty, 2020).
Although several studies have demonstrated that respiratory infections caused by viral or bacterial pathogens are linked with variations of the gut microbiota composition (Lv et al., 2021; Mizutani et al., 2022), the role of the intestinal microbiota in the evolution of the COVID-19 is still poorly known (Zuo et al., 2020).
Pulmonary health is also affected by the gut microbiota through cross-talk between the gut microbiota and the lungs, which is designated as the “gut-lung axis” (Dang and Marsland, 2019). The gut-lung axis is supposed to be bidirectional, since microbial associated molecular patterns (MAMPs) and endotoxins can influence the lung health through circulation. Once inflammation is established within the lungs, in turns it affects the gut microbiota (Zhang et al., 2020; Qu et al., 2022). Regarding the role of gut microbiota composition on COVID-19, most of the studies studies have focused their attention on the differences between COVID-19 and non-COVID-19 patients in order to investigate the possible role of the gut microbiota in susceptibility to SARS-CoV-2 infection (Marcialis and Bardanzellu, 2019; Yamamoto et al., 2021).
In addition, other published studies have unveiled the relationship between the gut microbiota and the severity of the disease in hospitalized patients underlining how the microbiota alteration could be associated with the clinical evolution (Yeoh et al., 2021). This relationship is based on the ability of the microbiota to modulate the immune response, either through modification of the gut-lung axis (Din et al., 2021), or altering the expression of angiotensin-converting enzyme 2 (ACE2) receptors which allow SARS-CoV-2 to enter host cells (Koester et al., 2021).
To date, few studies evaluated the gut microbiota as a prognostic factor for disease progression and severity in hospitalized patients (Yeoh et al., 2021). The potential role of gut microbiota composition at the hospital admission to be proposed as one of the predictors of clinical evolution in response to respiratory viruses such as SARS-CoV-2 needs to be explored. This study aimed to investigate whether gut microbiota in rectal swabs from hospitalized COVID-19 patients along with other clinical variables, including age, comorbidities and obesity, was able to discriminate the clinical outcome during the SARS-CoV-2 early infection. In particular, we investigated the gut microbiota profiles of patients affected by SARS-CoV2 with mild or severe symptoms. In the next future the analysis of the intestinal microbiota could lead to useful potential markers of severe prognosis in order to predict if the patient will require intensive care and ventilatory support.
Materials and methods
Ethics statement
Informed consent from participants was not required as per the REGULATION (EU) 2016/679 OF THE EUROPEAN PARLIAMENT 138 AND OF THE COUNCIL of 27 April 2016 (concerning the protection of natural persons with regard to the processing of personal data and on the free movement of such data), and repealing Directive 95/46/EC 140 (General Data Protection Regulation), on account of the public health emergency during an infectious disease outbreak. Approval by the National Institute for Infectious Diseases Spallanzani’s Institutional Review Board was not required for the same reasons. All interventions carried out on patients were based on their needs according to clinical judgment, and were not performed for the purposes of this study. Data were analyzed anonymously.
Study design
From May 2020 to January 2021, 600 rectal swabs were collected from patients with COVID-19 at the admission at National Institute for Infectious Diseases “Lazzaro Spallanzani” in Rome. The diagnosis has been confirmed by a SARS-CoV-2 RT-PCR positive on nasopharingeal swabs. Patients who had SARS-CoV-2 positivity with Cycle Threshold (Ct value) between 7 to 35 cycles were included into the study. Among the 600 patients hospitalized during the study period, 97 patients, admitted to the ordinary ward of Infectious Disease, were enrolled and classified in two groups according to their outcome considering the respiratory supports they needed during hospital stay: i) group “mild,” including 47 patients with a good prognosis and who never required non-invasive or invasive ventilation and ii) group “severe,” including 50 patients who experienced a more severe disease due to severe respiratory distress that required non-invasive or invasive ventilation.
No subjects had been vaccinated against COVID-19. Rectal swabs were collected at hospital admission and processed within 4 h and then stored at −80°C until analysis. All samples were collected during standard of care rounds using all the necessary precautions. Clinical records and Laboratory Information Systems (LIS) were used to retrieve patient data, including laboratory test results and clinical manifestations. 16 s rRNA sequencing was performed at Division of Gastroenterology, Fondazione-IRCCS “Casa Sollievo della Sofferenza” Hospital in San Giovanni Rotondo (Foggia).
DNA extraction
Microbial DNA was extracted starting from 500 μl of sample using Nimbus automatic extractor (Seegene Technologies, Korea) according to the manufacturer’s protocol after treatment with 500 μl of Lysis Buffer ATL (QIAGEN, Hilden, Germany) at 56°C for 10 min with 20 μl Proteinase K (Darmstadt, Germany). Protocol used for the extraction/purification was the STARMag 96 × 4 Universal Cartige kit (Seegene Technologies, Korea). The kit utilized is applied to automatic nucleic acid purification system with the convenient handling of magnetic beads and it is intended to be used for DNA isolation from different matrix (for example serum, swabs, stool, biopsy). The purification procedure comprises four steps: sample lysis, nucleic acid bind to magnetic beads, wash debris and purified nucleic acid elution. The kit provides reagents for the purification of up 20 ug of pure nucleic acid from sample with an A260/280 ratio > 1.6–1.9 and typical concentration of 20–50 ug/ul. The elution volume was 60 ng/ul.
Sequencing and bioinformatic analysis
The obtained DNA was quantified using a Qubit dsDNA HS Assay Kit (ThermoFisher Scientific, Massachusetts, United States) with a Qubit 4 Fluorometer. Microbiota amplicon sequencing was performed following the protocol recommended by Illumina. Briefly, amplification of the V3 and V4 region from 16S rRNA gene were obtained by PCRs using the suggested Illumina 16S Metagenomics Sequencing Workflow.1 The obtained fragments were sequenced with the MiSeq instrument (Illumina) with V3 reagents (600 cycle, 2 × 300 bp). Fastq raw sequencing data (deposited in ArrayExpress under the accession code E-MTAB-12236) were imported into qiime2 v.2021.2 (Bolyen et al., 2019; Estaki et al., 2020) using default parameters and then Illumina primers (forward: TCGTCGGCAGCGTCAGATGTGTATAAGAGAC AGCCTACGGGNGGCWGCAG, reverse: GTCTCGTGGGC TCGGAGATGTGTATAAGAGACAGGACTACHVGGGTATC TAATCC) were removed using q2-cutadapt plugin in trim-paired mode (Martin, 2011). Q2-dada2 plugin was utilized for trimming, denoising and filtering (Callahan et al., 2016) while q2-feature-classifier plugin (Bokulich et al., 2018) was employed for the assignment of taxonomy to amplicon sequence variants (ASVs) against the pre-trained Naïve Bayes classifier SILVA 138 99% operational taxonomic units (OTUs) full-length sequence dataset (Quast et al., 2013; Bokulich et al., 2021). Alpha rarefaction was produced with q2-diversity plugin in alpha-rarefaction, minimal sequence c ount between samples as max depth. q2-fragment-insertion plugin was used in sepp mode to produce the phylogenetic tree, against sepp-refs-silva-128, necessary in q2-diversity core-metrics-phylogenetic (executed with sampling-depth: minimal sequences count between samples). The Bray-curtis matrix from core-metrics analysis was tested with q2-diversity beta-group-significance, PERMANOVA test, p-values<0.05 as significant. Predictions of the functional profile of a microbial community based on 16S rRNA sequence data in R environment (Wemheuer et al., 2020) was obtained with Tax4Fun2 Pairwise comparisons of differentially abundant Kyoto Encyclopedia of Genes and Genomes (KEGG) pathways among different groups were performed using Mann–Whitney test, p-values <0.05 were considered significative, and visualized with STAMP v2.1.3 (Parks et al., 2014).
Statistical analysis
Demographic and clinical-pathological features of COVID-19 patients with good (group “mild”) or severe (group “severe”) outcome were reported as mean ± standard deviation (SD), median along with interquartile range (i.e., first-third quartiles) and observed frequencies (and percentages) for continuous and categorical variables, respectively. The PEnalized LOgistic Regression Analysis (PELORA) was performed in order to identify clusters of bacterial populations, such that the linear combination of their abundances is differential between patients with good or severe outcome (see details in Supplementary materials and methods). All statistical analyses and plots were performed by the computing environment R (R Development Core Team 2008, version 4.2, packages: ComplexHeatmaps, supclust, ggplot2, gridExtra).
Results
Study population
Demographic and clinical-pathological data of the patients enrolled in this study are presented in Table 1. We enrolled 97 patients with a SARS-CoV-2 qRT-PCR positive nasopharingeal swab from a retrospective cohort of 600 rectal swabs from COVID-19 hospitalized patients. In order to evaluate the correlation between gut microbiota composition at hospital admission and clinical outcome, we divided our study population into two groups: group “mild,” with a good evolution (47/97), who never needed non-invasive or invasive ventilation, and group “severe,” who experimented a severe evolution of the disease with respiratory distress (50/97) and required non-invasive or invasive ventilation. As showed in Table 1, in group “severe” pneumonia and hypertension rate was statistically significantly higher (p = 0.005 and p = 0.015 respectively) as compared to group “mild.” Furthermore the use of ACE inhibitors was more frequent in patients with severe outcome (p = 0.035). Additionally, in patients of group “severe” the PaO2/FiO2 (ratio) resulted significantly lower (p < 0.001), as expected, as well as lymphocytes (p < 0.001), while WBC (p = 0.033), neutrophils (p = 0.004), LDH (p = 0.001), CRP (p = 0.018), ferritin (p < 0.001) and fibrinogen (p = 0.013) were all significantly higher than patients with mild symptoms (Table 1). Considering the clinical evolution, the intensive care admission (p < 0.001), the proportion of patients requiring ventilation (p < 0.001) and the number of deaths (p = 0.013) were significantly higher in “severe” group (Table 1).
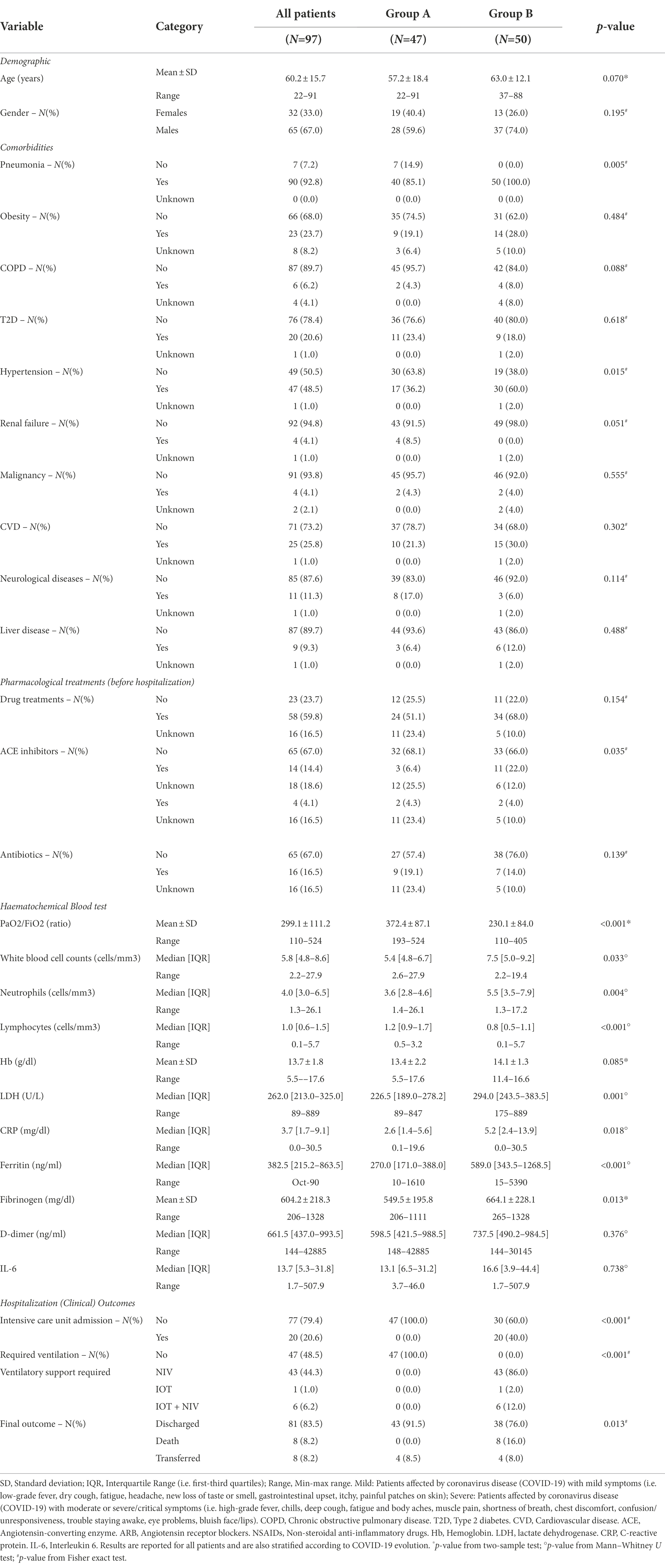
Table 1. Demographic and clinical characteristics (i.e. comorbidities, pharmacological treatments and haematochemical test results) of COVID-19 patients evaluated at their hospitalization.
Comparison of gut microbiota composition between patients with mild or severe disease evolution.
While no changes in Chao1 and Shannon index were observed between group “mild” and group “severe” (Supplementary Figure 1), the different fecal microbiota composition of patients with mild and severe outcome of COVID-19 are reported in Figure 1, with major changes displayed at lower taxonomic levels. Worth of note, at phylum level, Campylobacterota and Actinobacteriota were increased in patients with the worse outcome (group “severe”). The phylum of Campylobacterota includes several pathogens causing diarrhea, cramps, fever and pain. At family level, Peptostreptococcale, Corynebacteriaceae and Campylobacteriaceae increased in group “severe,” while Ruminoccoccaceae (involved in producing short-chain fatty acids) and Enterobacteriaceae decreased. Conversely, in patients with mild disease, Enterobacteriaceae, Veillonellaceae and Streptococcaceae increased. Furthermore, at genus and species level an increase of Corynebacterium, Campylobacter and Finegoldia in group “severe” and Escherichia-Shigella and Faecalibacterium in group “mild” was observed. PCoA analysis of gut microbiota based on Bray–Curtis dissimilarity (Supplementary Figure 2) plots the difference in the community of fecal microbiota across the two groups (mild and severe) while a rarefaction curve is reported in Supplementary Figure 3.
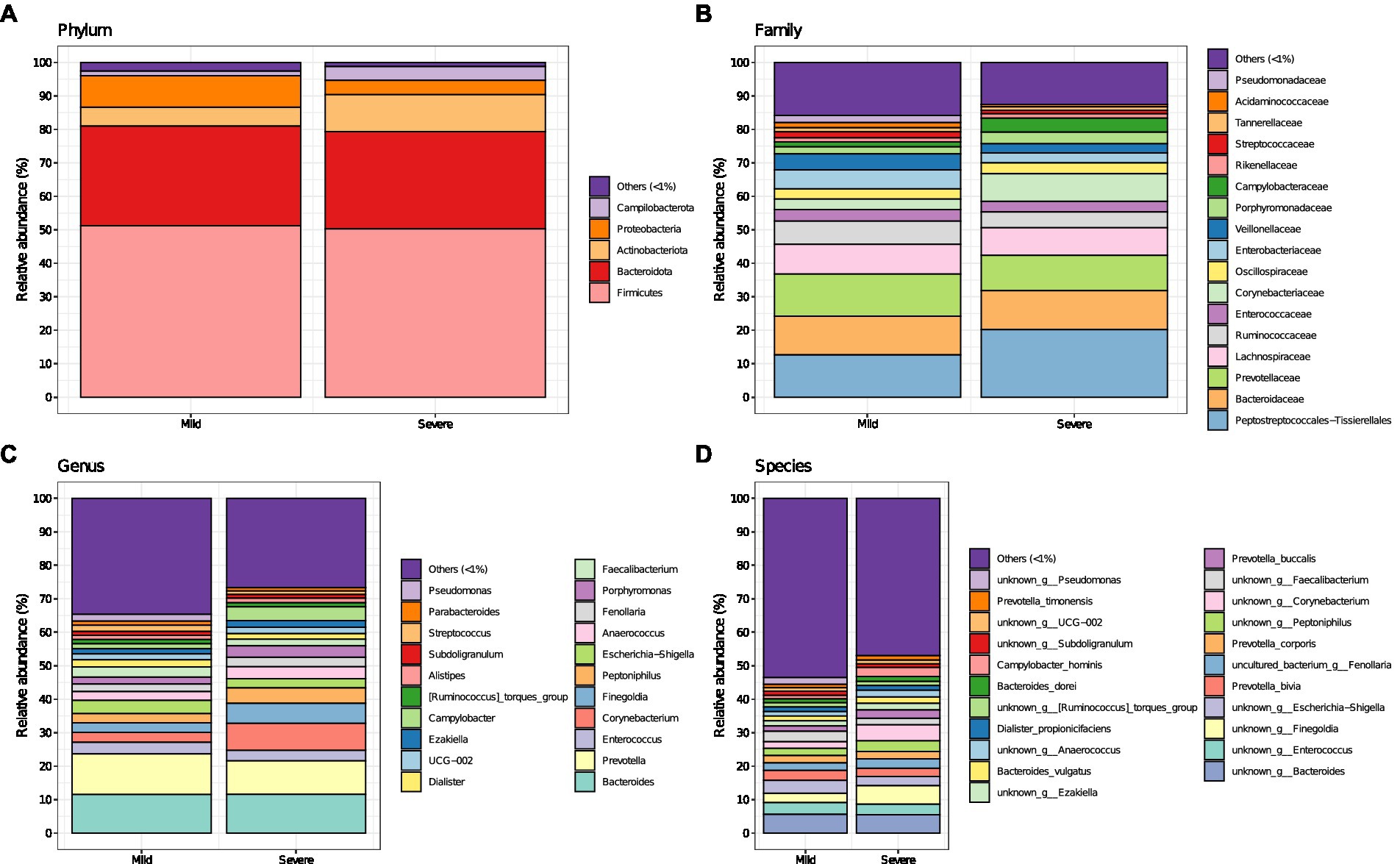
Figure 1. Fecal microbiota composition (i.e., mean relative abundance %) at Phylum (A), Family (B), Genus (C), and Species (D) levels in patients with coronavirus disease (COVID-19) at their hospitalization, for mild and severe symptoms separately. “Others (<1%)” category includes bacteria with mean relative abundance less than 1%.
PELORA algorithm identified bacterial populations associated to disease evolution
Taking advanteage of the PELORA algorithm we identified clusters of bacterial populations that better discriminate between patients with mild from those with severe COVID-19 evolution based on the relative abundances generated by taxonomic analyses. Table 2 reports the list of the bacteria detected by the algorithm within each cluster. Although every taxon reported concurred to the distinctive cluster which better discriminate patients with mild or severe respiratory failure, we have observed that at Phylum level Proteobacteria, Desulfobacterota, Patescibacteria, Spirochaetota in cluster 1 discriminate the outcome between the two groups and that at Family level Enterobacteriaceae, Elusimicrobiaceae, Sedimentibacteraceae, Atopobiaceae, Sphingomonadaceae, Acidaminococcaceae, Spirochaetaceae, Lachnospiraceae had a greater discriminatory power. At Genus level an increase of Escherichia-Shigella, Megasphaera, Succiniclasticum, Clostridium_sensu_stricto_13, Sphingomonas, Parvimonas, Candidatus_Stoquefichus, Alloscardovia, Succinivibrio, Klebsiella, Gordonibacter, Proteiniphilum, Catonella, Acinetobacter, Flavonifractor, Gardnerella, Halomonas, Lachnospiraceae_UCG-001, Lachnospiraceae_UCG-003, uncultured_f__Desulfovibrionaceae, uncultured_f__Prevotellaceae, unknown_f__Hungateiclostridiaceae was observed in cluster 1 and an increase of Finegoldia, [Clostridium]_methylpentosum_group, Facklamia, Butyrivibrio, Prevotellaceae_NK3B31_group, Corynebacterium, Prevotellaceae_UCG-001, Hymenobacter, Lactobacillus, Actinobaculum Eubacterium, Cutibacterium, Holdemania, Pseudoflavonifractor, Epulopiscium, Saccharimonadales, unknown_f__Corynebacteriaceae was observed in cluster 2.
Figure 2 graphically represents the distribution of Z-scores computed at clusters centroids at different taxa levels, showing that two clusters, composed by the linear combination of definite microorganisms residing within the gut of the patients at hospital admission, were able to significantly discriminate the clinical evolution of COVID-19 patients, in particular who will develop mild or severe respiratory involvement.
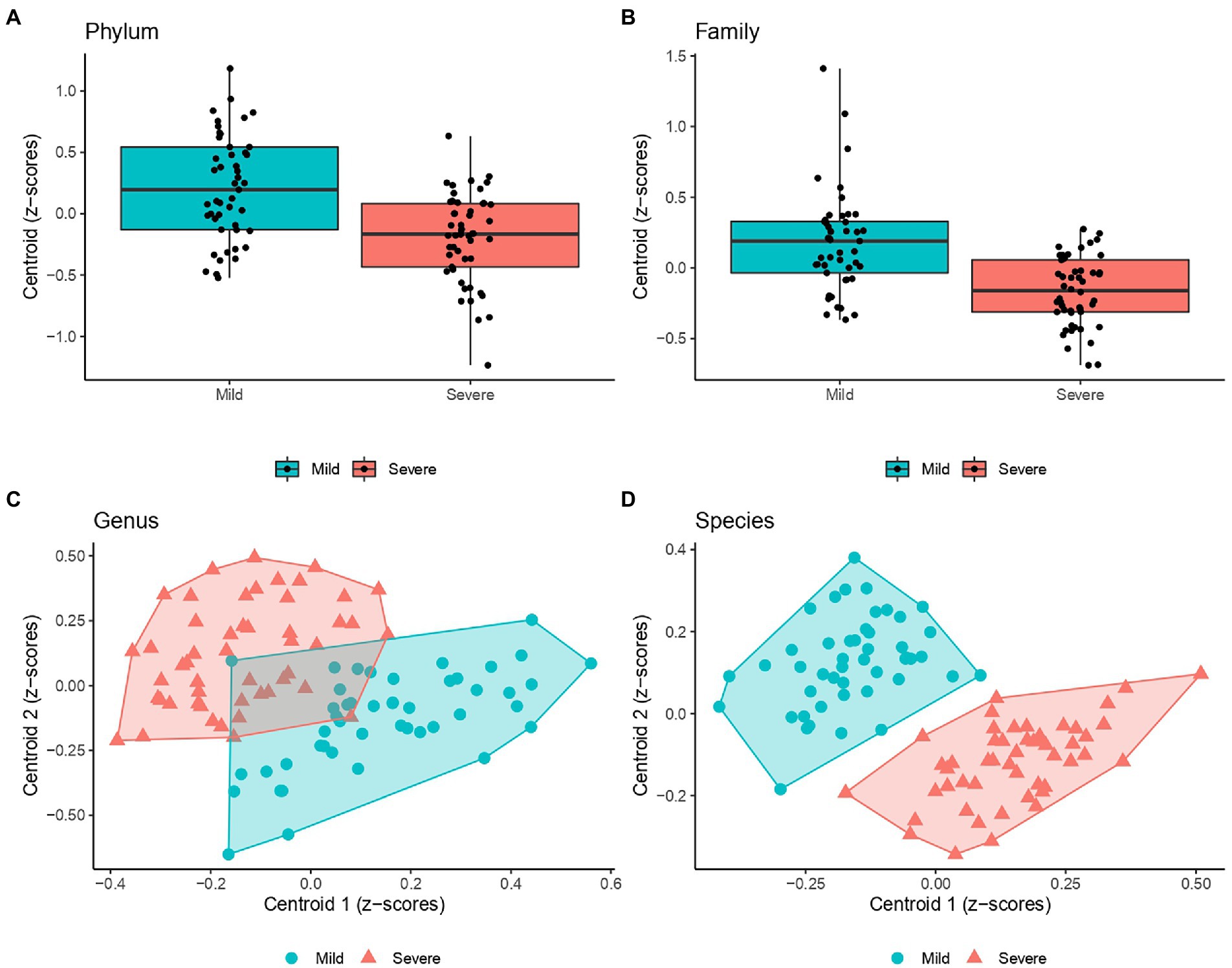
Figure 2. Scatter plots of the Z-scores computed within each cluster (i.e., centroid) detected by PEnalized LOgistic Regression Analysis at Genus (C), and Species (D). Each point represents the Z-scores pair computed at each individual and were filled with blue and red colors to designate COVID-19 patients with milder or more severe evolution. Moreover, a polygon connecting the outermost data points is shown for both group. As a single cluster of bacteria population was detected at both Phylum (A) and Family levels (B), box plots were presented (instead of scatter plots).
At species levels, patients with mild symptoms were characterized by lower Z-scores from cluster 1 and higher Z-scores from cluster 2. Conversely, patients with “severe” outcome were categorized by higher Z-scores from cluster 1 and lower Z-scores from cluster 2. Moreover, Figure 3 reports the heatmaps shwing the relative abundance of each microorganism detected within each cluster at the phylum (Figure 3A), family (Figure 3B), genus (Figure 3C) and species (Figure 3D) level for each recruited subject in the good and severe group of outcome.
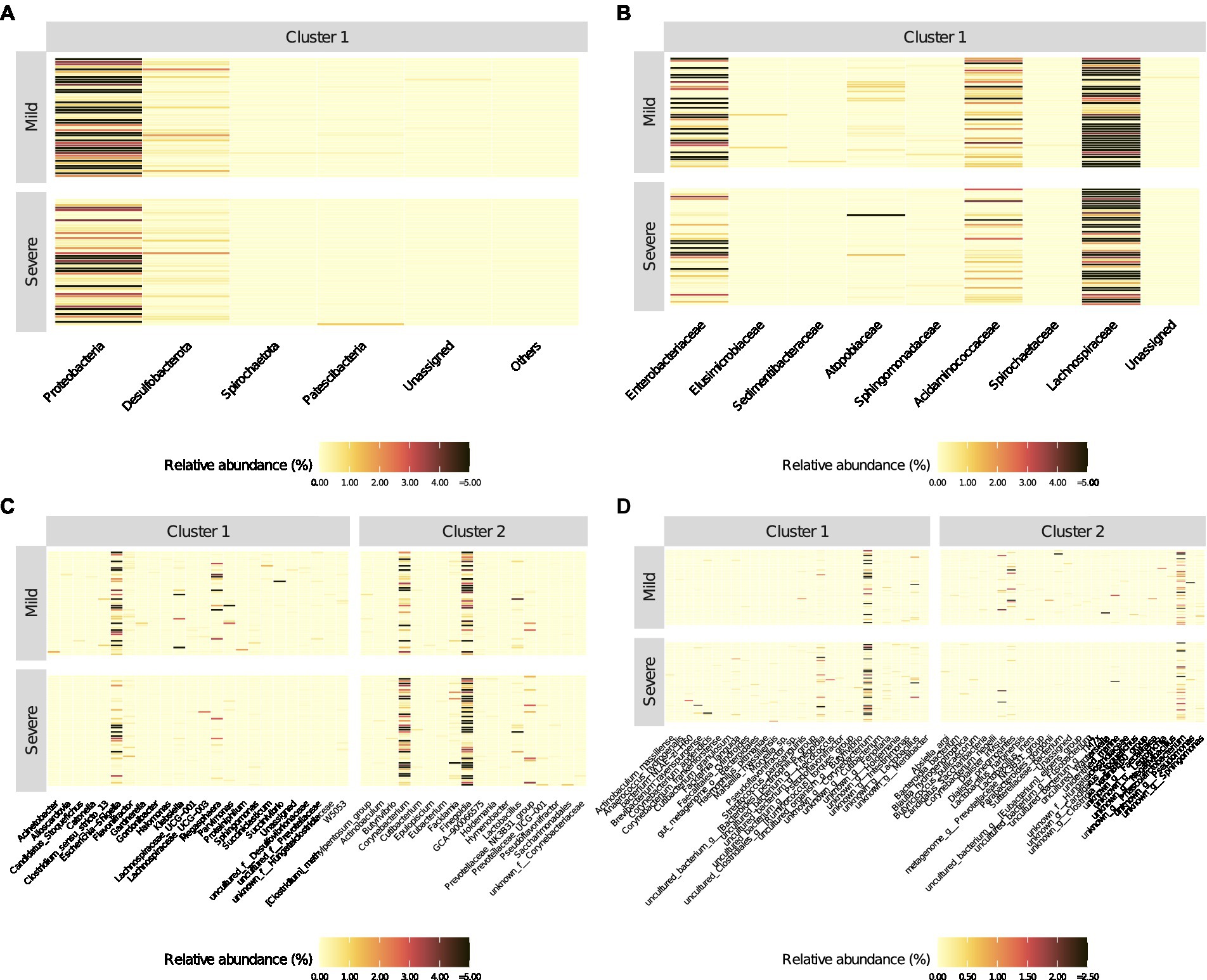
Figure 3. Heatmaps of relative abundance (%) of bacterial populations identified (into different clusters) by the PEnalized LOgistic Regression Analysis at different taxonomic hierarchy, grouped by COVID-19 patients with mild and severe symptoms, respectively. The order of the rows (patients) was determined by the main heatmap, which has been defined at the Phylum level. All other heatmaps are automatically adjusted according to the settings in the main heatmap.
Predicting the functional capabilities of microbial communities based on 16S datasets
To explore the possible functional contributions of the gut microbiome on patients with mild or severe clinical evolution, we performed Tax4Fun2 prediction analyses based on 16S rRNA gene abundance profiles. As shown in Figure 4, functional profiling unveiled significant changes in the metabolic pathways between the two groups. At level 1 of analysis, we identified 25 the predicted differentially expressed metabolic pathways (p-values <0.05, Mann–Whitney test) that lead to the functional divergence in patients with mild or severe clinical evolution. Compared with the “severe” group, 8 functional categories were enriched in the gut microbiomes profiles of patients belonging to the “mild” group, including Arginine and Proline metabolism, beta_Lactam resistance, butanoate metabolism, degradation of aromatic compounds, fatty acid degradation, flagellar assembly, Glycolysis/Gluconogenesis, Sulfur metabolism. In contrast, in the gut microbiomes of severe group, 17 pathways were more abundant (i.e., Tubercolosis, Legionellosis, D-Glutamine and D-glutamate metabolism, DNA replication, folate biosinthesis, methane metabolism). Although these results show that in addition to microbial profile differences, there may be differences to functionalities of microbiome between mild or severe clinical evolution, it should be underlined that that functional prediction is only speculative and not necessarily indicative of actual observed differences.
Discussion
Gut microbiota is a complex ecosystem which has been estimated to exceed 1014 microorganisms including bacteria, virus and fungi living in symbiosis with the host (Belkaid and Hand, 2014). This microbe community is useful in maintaining the host’s homeostasis since it influence several physiological functions, such as maintenance of the intestinal integrity, protection against pathogenic organisms, energy production and regulation of host’s immunity (Li et al., 2019). However, this status can become compromised following alterations in the core gut microbiota composition or functions, a condition known as dysbiosis (Hou et al., 2022). This change in intestinal microbiota profiles may compromise the host’s functions in which it is involved, including the immune system’s response against infections (Belkaid and Hand, 2014). In contrast, there is evidence that bacterial and viral infections can cause alterations in the gut microbiota profile, predisposing the host to secondary infections (not reported for the current cohort) or worsening clinical status (Harper et al., 2021; Kassa et al., 2021; Mizutani et al., 2022). In this context, an increasing importance is attributed to the role played by the intestinal microbiota in COVID-19 infection (Zuo et al., 2021). The bacterial dysbiosis in respiratory and intestinal tract is related to inflammatory response and although, mechanistically, this phenomenon remains poorly defined, the existence of the gut–lung axis and its implications in both health and disease may play a key role in both disease etiology and treatment. No significant differences were observed in the bacterial diversity of this study’s cohort, although previous studies have demonstrated that COVID-19 patients show a significantly reduced bacterial diversity (Mazzarelli et al., 2021; Hazan et al., 2022). This discrepancy may be explained because in the study by Hazan et al. (2022) and in our previous report (Mazzarelli et al., 2021) symptomatic patients were compared to healthy control, whereas in the current cohort, severely versus mild symptomatic hospitalized infected patients were compared.
Ferreira et al. (2020), reported a higher abundance of opportunistic bacteria such as Streptococcus, Rothia, Veilonella, and Actinomyces; and reduced levels of beneficial symbionts, including Agathobacter, Fusicatenibacter, Roseburia, and Ruminococcaceae. Accordingly, an increase in families containing potential pathogens such as Peptostreptococcaceae, Enterobacteriaceae, Staphylococcaceae, Vibrionaceae, Aerococcaceae, Dermabacteraceae, was observed in our previous paper when comparing the microbiota profiles of COVID-19 patients admitted to ordinary infectious ward to that of healthy controls (Mazzarelli et al., 2021).
Identifying prognostic markers is important in the management of COVID-19 patients to predict clinical evolution and it could also impact therapies (He et al., 2020). However, few studies evaluated the gut microbiota as a prognostic factor for disease progression in hospitalized patients. The existence of a correlation between the COVID-19 severity and fecal microbiota dysbiosis (including depletion of commensal bacteria) has been reported (De and Dutta, 2022). As shown by Zuo et al. (2020), twenty-three bacterial taxa were significantly correlated with disease severity, with the main bacteria belonging to the phylum Firmicutes and the genus Coprobacillus, as well as the Clostridium ramosum and Clostridium hathewayi species. In our cohort, the abundance of Firmicutes and of Coprobacillus genus was not significantly different between the two groups, whereas the species Clostridium ramosum and Clostridum hatewayi, which Zuo et al. found associated with COVID-19 severity, were not detected at all.
In the present study, analyzing gut microbiota composition in an association with other variables through multivariate analysis, we tried to identify a putative prognostic marker of clinical evolution in terms of severity of respiratory distress and need of ventilation support, useful to use early when the patient enters the hospital ward to predict the outcome. We grouped within the “severe” group patients requiring either non-invasive or invasive ventilation because they were all hospitalized in Intensive Care Unit, because other severe symptoms were diagnosed and considered and also because, although it would have been interesting to analyze the gut microbiota profiles separately in the two groups with invasive or non-invasive, the sample size would have been smaller, with the risk to mislead conclusions. We did not find significant differences in Chao1 and Shannon indices between the two groups of patients with different clinical evolution, and this evidence indicates that at baseline there is a uniformity of the microbiota in the patients admitted to the ordinary ward and is in agreement with our previous observation. However, fecal microbiota composition showed that, at phylum level, Campilobacterota and Actinobacteriota were increased in patients who had a more aggressive disease. Concerning Campylobacterota, they mainly include the genera Campylobacter, which are leading food-borne pathogens causing gastrointestinal infections, and Helicobacter, responsible of both gastric (such as gastritis, peptic ulcer, gastric cancer,) and extra-gastric (such as idiopathic thrombocytopenic purpura, anemias, MALT lymphoma, pancreatitis and pancreatic cancers) diseases (Nordestgaard et al., 2018). While for Helicobacter the standard clinical practice implies antibiotics-based therapies for bacterial eradication (Goderska et al., 2018), the Campylobacter infections, which in our study makes the greatest contribution to Campylobacterota increase, are often self-limiting and rarely require antibiotic treatment (Butzler, 2004).
Concerning Actinobacteriota, the family of Corynebacteriaceae was the main contributor to their increase in our study. Members of this family have been reported to cause alveolitis (Rintala, 2011), pneumonia (Yang et al., 2018), chronic respiratory diseases (Renom et al., 2014). Antibiotic therapy is recommended also in this case, although many species show multi-drug resistance, hence the need of selecting the appropriate antibiotic (Olender, 2013). Gradel et al. (2009), showed a possible association between the presence of Campylobacter spp. and inflammatory bowel disease and development of intestinal inflammation, so the finding of Campylobacter spp. in the gut microbiota of “severe” group of SARS-CoV-2 patients could explain the worst clinical outcome. Although finding genera containing pathogenic bacteria is not necessarily a good indicator of there being pathogenic species in the samples, all the six Campylobacter species identified in our samples have been described as involved in a number of inflammatory diseases. In detail: (i) C. concisus has been described as a pathogen associated with diseases of the gastrointestinal tract, such as Barrett’s esophagus, prolonged diarrhoea, and inflammatory bowel disease (Kirk et al., 2018); (ii) C. gracilis has been associated with periodontal diseases and pleuropulmonary infections (Shinha, 2015); (iii) C. hominis has been associated to ulcerative colitis and Crohn’s disease (Igwaran and Okoh, 2019); (iv) C. showae has been linked to gingivitis and periodontitis, has recently been associated with inflammatory bowel disease and colorectal cancer (Hsu et al., 2019); (v) C. sputorum has been described to cause diarrhoea in children (Lindblom et al., 1995); (vi) C. ureolyticus has been identified as a gastrointestinal pathogen, isolated from patients with Crohn’s disease and many other pathogenic conditions (Burgos-Portugal et al., 2012). The same is true for the Corynebacterium genus, which, with its 2.7 fold increase in the “severe” groups with respect to “mild,” gave the major contribution to Actinobacteriota increase. A number of Corynebacterium species identified in our cohort are known to be respiratory airways pathogens. In detail, just to cite few examples: (i) C. argentoratense has been detected in the upper respiratory tract of patients suffering from tonsillitis (Bomholt et al., 2013); (ii) C. durum is a pathogen of the respiratory tract (Riegel et al., 1997); (iii) C.imitans was first isolated in from a child suffering from acute respiratory disease that was initially diagnosed as pharyngeal diphtheria (Funke et al., 1997); (iv) C. jeikeium was reported to cause different forms of infections, including endocarditis, especially in immunocompromised subjects (Rezaei Bookani et al., 2017); (v) C. propinquum has been described in bronchopneumonia (Malkoçoğlu et al., 2016) and as the causative agent of respiratory tract infections worldwide (Jangda et al., 2016).
Furthermore, our results were in accordance to Farsi et al. (2022), who reported that alterations in gut microbiome were associated with COVID-19 severity and severe prognosis; such alterations included the increment of Bacteroidota, Parabacteroides, Clostridium, Bifidobacterium, Ruminococcus, Campylobacter, Rothia, Corynebacterium, Megasphaera, Enterococcus, Aspergillus spp. and the decrement of Roseburia, Eubacterium, Lachnospira and Faecalibacterium. In agreement with the findings by Farsi et al. (2022) Campylobacter and Corynebacterium were significantly increased while Roseburia and Lachnospira were under-represented in our COVID patients with severe disease compared to mild ones. On the contrary, Bacteroidota, Parabacteroides, Bifidobacterium, Ruminococcus, Rothia, Enterococcus, Eubacterium, Faecalibacterium were not differently represented between the two groups, while Megasphaera was decreased in the “severe” group. Finally, Aspergillus was not detected in our cohort. Several factor could explain such differences between our study and the review by Farsi et al. (2022), first of all the heterogeneity in the cohorts compositions, including different genetic backgrounds and lifestyles; in addition, methodological differences including DNA extraction matrix (stool sample rather than rectal swab), DNA isolation and sequencing procedure, data processing and statistical analysis.
In addition, a reduction at Family level of Ruminoccoccaceae was observed in patients with severe symptoms. A decreased abundance of Ruminococcaceae has been involved in several inflammatory bowel diseases and playing a main role in the maintenance of gut health due to their ability to produce butyrate and other Short Chain Fatty Acids (SCFAs) (Parada Venegas et al., 2019). Among SCFAs, butyrate owes antinflammatory properties that could explain why patients had a more severe disease. It should be noted that, unexpectedly, in our study, Enterobacteriaceae increased in the group of patients with a less severe outcome than in patients with a worse one. It could be argued that this phenomenon could be due to the higher exposure to active antibiotics (e.g., cephalosporins) more easily prescribed to most seriously ill patients at emergency department (76% of “severe” patients vs. 57% of “mild” patients were administered with antibiotics). Unfortunately data regarding antibiotic therapy before hospitalization were not avaible for all patients and it could have affected the different detection of these bacteria in the two groups of study patients. In the current study, Proteobacteria was more prevalent in less severe (mild) patients in the same line to what was observed by Yeoh et al. (2021) who described an increase in Proteobacteria (compared to COVID-19 negative control and COVID-19 patients during hospitalization) in the gut microbiota of COVID-19 patients discharged after negative RT-qPCR for viral RNA in nasopharyngeal swabs.
Furthermore, we took advantage of the Penalized Logistic Regression Analysis (PELORA) algorithm to identify the bacterial populations that best discriminate between the two groups and these changes in microbiota compositions differently affect the functional and metabolic profiles influenced by microbial communities belonging to patients with mild or severe clinical evolution.
Concerning the functional prediction of pathways affected by changes in gut microbial community, worth of note was among others, the increase in severely affected COVID-19 patients in Legionellosis and Tubercolosis, which both involves a pulmonary compromission, as COVID-19.
Being an observational, retrospective, single-centre study, our study has some limitations, including the enrollment of patients with different clinical management. This study require further testing and longitudinal data for them to be proposed as prognostic markers. Moreover, multiple factors can cause changes in microbiota, including the use of antibiotics. Noteworthy, that rectal swab was collected on the first day after patients’ hospitalization allowing us to limit this bias.
In conclusion, our study provides a list of bacterial markers more prevalent in severe patients compared to mild patients which may contribute to prognosis evaluation, paving the way to new interventional approaches.
Data availability statement
Fastq raw sequencing data (deposited in ArrayExpress under the accession code E-MTAB-12236).
Ethics statement
Ethical review and approval was not required for the study on human participants in accordance with the local legislation and institutional requirements. Written informed consent for participation was not required for this study in accordance with the national legislation and the institutional requirements.
Author contributions
AM, MG, CP, LM, AVu, CG, and CF: sample and clinical collection. VP, CP, AVi, EB, GM, and NT: metagenomic experiments and analysis. AF, MC, and PP: statistical analysis. EN, FP, and VP: provision of study materials. VP, FP, EN, AM, and MG: writing – original draft. All authors: writing – review and editing. All authors contributed to the article and approved the submitted version.
Funding
This study was supported by funds to the Istituto Nazionale per le Malattie Infettive (INMI) Lazzaro Spallanzani IRCCS, Rome, Italy, from the Ministero della Salute (Ricerca Corrente, linea 1; COVID-2020-12371817), the European Commission – Horizon 2020 (EU project 101003544 – CoNVat; EU project 101003551 – EXSCALATE4CoV; EU project 101005111 DECISION; EU project 101005075-KRONO) and the European Virus Archive – GLOBAL (grant nos. 653316 and 871029). SHARP: 848096. VP is supported by Italian Association for Cancer Research (AIRC) under IG 2019 – ID. 23006 project – P.I. and by Italian Ministry of Health Ricerca Corrente program 2022–2024.
Conflict of interest
The authors declare that the research was conducted in the absence of any commercial or financial relationships that could be construed as a potential conflict of interest.
Publisher’s note
All claims expressed in this article are solely those of the authors and do not necessarily represent those of their affiliated organizations, or those of the publisher, the editors and the reviewers. Any product that may be evaluated in this article, or claim that may be made by its manufacturer, is not guaranteed or endorsed by the publisher.
Supplementary material
The Supplementary material for this article can be found online at: https://www.frontiersin.org/articles/10.3389/fmicb.2022.1049215/full#supplementary-material
Footnotes
1. ^https://www.illumina.com/content/dam/illumina-marketing/documents/products/other/16s-metagenomics-faq-1270-2014-003.pdf
References
Belkaid, Y., and Hand, T. W. (2014). Role of the microbiota in immunity and inflammation. Cells 157, 121–141. doi: 10.1016/j.cell.2014.03.011
Bokulich, Nicholas, Dillon, Matthew, Robeson, Mike, Ziemski, Michal, Kaehler, Ben, and O'Rourke, Devon. (2021). bokulich-lab/RESCRIPt: 2021.2.0 (2021.2.0). Zenodo. doi: 10.5281/zenodo.4569379.
Bokulich, N. A., Kaehler, B. D., Rideout, J. R., Dillon, M., Bolyen, E., Knight, R., et al. (2018). Optimizing taxonomic classification of marker-gene amplicon sequences with QIIME 2’s q2-feature-classifier plugin. Microbiome 6:90. doi: 10.1186/s40168-018-0470-z
Bolyen, E., Rideout, J. R., Dillon, M. R., Al-Ghalith, G. A., et al. (2019). Reproducible, interactive, scalable and extensible microbiome data science using QIIME 2. Nat. Biotechnol. 37, 852–857. doi: 10.1038/s41587-019-0209-9
Bomholt, C., Glaub, A., Gravermann, K., Albersmeier, A., Brinkrolf, K., Rückert, C., et al. (2013). Whole-genome sequence of the clinical strain Corynebacterium argentoratense DSM 44202, isolated from a human throat specimen. Genome Announc. 1, e00793–e00713. doi: 10.1128/genomeA.00793-13
Burgos-Portugal, J. A., Kaakoush, N. O., Raftery, M. J., and Mitchell, H. M. (2012). Pathogenic potential of campylobacter ureolyticus. Infect. Immun. 80, 883–890. doi: 10.1128/IAI.06031-11
Butzler, J. P. (2004). Campylobacter, from obscurity to celebrity. Clin. Microbiol. Infect. 10, 868–876. doi: 10.1111/j.1469-0691.2004.00983.x
Callahan, B. J., McMurdie, P. J., Rosen, M. J., Han, A. W., Johnson, A. J., and Holmes, S. P. (2016). DADA2: high-resolution sample inference from Illumina amplicon data. Nat. Methods 13, 581–583. doi: 10.1038/nmeth.3869
Dang, A. T., and Marsland, B. J. (2019). Microbes, metabolites, and the gut-lung axis. Mucosal Immunol. 12, 843–850. doi: 10.1038/s41385-019-0160-6
De, R., and Dutta, S. (2022). Role of the microbiome in the pathogenesis of COVID-19. Front. Cell. Infect. Microbiol. 31:736397. doi: 10.3389/fcimb.2022.736397
Dhar, D., and Mohanty, A. (2020). Gut microbiota and Covid-19- possible link and implications. Virus Res. 285:198018. doi: 10.1016/j.virusres.2020.198018
Din, A. U., Mazhar, M., Wasim, M., Ahmad, W., Bibi, A., Hassan, A., et al. (2021). SARS-CoV-2 microbiome dysbiosis linked disorders and possible probiotics role. BiomedPharmacother :133. doi: 10.1016/j.biopha.2020.110947
Estaki, M., Jiang, L., Bokulich, N. A., McDonald, D., González, A., Kosciolek, T., et al. (2020). QIIME 2 enables comprehensive end-to-end analysis of diverse microbiome data and comparative studies with publicly available data. Curr. Protoc. Bioinformatics 70:e100. doi: 10.1002/cpbi.100
Farsi, Y., Tahvildari, A., Arbabi, M., Vazife, F., Sechi, L. A., Shahidi Bonjar, A. H., et al. (2022). Diagnostic, prognostic, and therapeutic roles of gut microbiota in COVID-19: a comprehensive systematic review. Front. Cell. Infect. Microbiol. 4:804644. doi: 10.3389/fcimb.2022.804644
Ferreira, C., Viana, S. D., and Reis, F. (2020). Is gut microbiota dysbiosis a predictor of increased susceptibility to poor outcome of COVID-19 patients? An update. Microorganisms. 9:53. doi: 10.3390/microorganisms9010053
Funke, G., Efstratiou, A., Kuklinska, D., Hutson, R. A., De Zoysa, A., Engler, K. H., et al. (1997). Corynebacterium imitans sp. nov. isolated from patients with suspected diphtheria. J. Clin. Microbiol. 35, 1978–1983. doi: 10.1128/jcm.35.8.1978-1983.1997
Goderska, K., Pena, S. A., and Alarcon, T. (2018). Helicobacter pylori treatment: antibiotics or probiotics. Appl. Microbiol. Biotechnol. 102, 1–7. doi: 10.1007/s00253-017-8535-7
Gradel, K. O., Nielsen, H. L., Schønheyder, H. C., Ejlertsen, T., Kristensen, B., and Nielsen, H. (2009). Increased short- and long-term risk of inflammatory bowel disease after salmonella or campylobacter gastroenteritis. Gastroenterology 137, 495–501. doi: 10.1053/j.gastro.2009.04.001
Harper, A., Vijayakumar, V., Ouwehand, A. C., Ter Haar, J., Obis, D., Espadaler, J., et al. (2021). Viral infections, the microbiome, and probiotics. Front. Cell. Infect. Microbiol. 12:596166. doi: 10.3389/fcimb.2020.596166
Hazan, S., Stollman, N., Bozkurt, H. S., Dave, S., Papoutsis, A. J., Daniels, J., et al. (2022). Lost microbes of COVID-19: Bifidobacterium, Faecalibacterium depletion and decreased microbiome diversity associated with SARS-CoV-2 infection severity. BMJ Open Gastroenterol. 9:e000871. doi: 10.1136/bmjgast-2022-000871
He, Y., Wang, J., Li, F., and Shi, Y. (2020). Main clinical features of COVID-19 and potential prognostic and therapeutic value of the microbiota in SARS-CoV-2 infections. Front. Microbiol. 5:1302. doi: 10.3389/fmicb.2020.01302
Hou, K., Wu, Z. X., Chen, X. Y., Wang, J. Q., Zhang, D., Xiao, C., et al. (2022). Microbiota in health and diseases. Signal Transduct. Target. Ther. 7:135. doi: 10.1038/s41392-022-00974-4
Hsu, T., Gemmell, M. R., Franzosa, E. A., Berry, S., Mukhopadhya, I., Hansen, R., et al. (2019). Comparative genomics and genome biology of campylobacter showae. Emerg. Microbes Infect. 8, 827–840. doi: 10.1080/22221751.2019.1622455
Igwaran, A., and Okoh, A. I. (2019). Human campylobacteriosis: a public health concern of global importance. Heliyon. 5:e02814. doi: 10.1016/j.heliyon.2019.e02814
Jangda, U., Upadhyay, A., Bagheri, F., Patel, N. R., and Mendelson, R. I. (2016). Corynebacterium propinquum: a rare cause of prosthetic valve endocarditis. Case Rep. Med. :1391789. doi: 10.1155/2016/1391789
Kassa, Y., Million, Y., Gedefie, A., and Moges, F. (2021). Alteration of gut microbiota and its impact on immune response in patients with chronic HBV infection: a review. Infect Drug Resist. 6, 2571–2578. doi: 10.2147/IDR.S305901
Kirk, K. F., Méric, G., Nielsen, H. L., Pascoe, B., Sheppard, S. K., Thorlacius-Ussing, O., et al. (2018). Molecular epidemiology and comparative genomics of campylobacter concisus strains from saliva, faeces and gut mucosal biopsies in inflammatory bowel disease. Sci. Rep. 8:1902. doi: 10.1038/s41598-018-20135-4
Koester, S. T., Li, N., Lachance, D. M., Morella, N. M., and Dey, N. (2021). Variability in digestive and respiratory tract Ace2 expression is associated with the microbiome. PLoS One 16:e0248730. doi: 10.1371/journal.pone.0248730
Li, N., Ma, W. T., Pang, M., Fan, Q. L., and Hua, J. L. (2019). The commensal microbiota and viral infection: a comprehensive review. Front. Immunol. 4:1551. doi: 10.3389/fimmu.2019.01551
Lindblom, G. B., Sjögren, E., Hansson-Westerberg, J., and Kaijser, B. (1995). Campylobacter upsaliensis, C. sputorum sputorum and C. concisus as common causes of diarrhoea in Swedish children. Scand. J. Infect. Dis. 27, 187–188. doi: 10.3109/00365549509019006
Lv, Z., Xiong, D., Shi, J., Long, M., and Chen, Z. (2021). The interaction between viruses and intestinal microbiota: a review. Curr. Microbiol. 78, 3597–3608. doi: 10.1007/s00284-021-02623-5
Malkoçoğlu, G., Gencer, H., Kaya, A., Dalgıç, N., Bulut, M. E., and Aktaş, E. (2016). Corynebacterium propinquum bronchopneumonia in a child with ataxia telangiectasia. Turk. J. Pediatr. 58, 558–561. doi: 10.24953/turkjped.2016.05.018
Marcialis, M. A., and Bardanzellu, F. (2019). Fanos VMicrobiota and coronavirus DiseaseWhich came first, the chicken or the egg? Clin. Infect. Dis. 2020, 1–3. doi: 10.1093/cid/ciaa965
Martin, M. (2011). Cutadapt removes adapter sequences from high-throughput sequencing reads. EMBnet.journal 17, 10–12. doi: 10.14806/ej.17.1.200
Mazzarelli, A., Giancola, M. L., Farina, A., Marchioni, L., Rueca, M., Gruber, C. E. M., et al. (2021). INMI COVID-19 study group. 16S rRNA gene sequencing of rectal swab in patients affected by COVID-19. PLoS One 16:e0247041. doi: 10.1371/journal.pone.0247041
Mizutani, T., Ishizaka, A., Koga, M., Tsutsumi, T., and Yotsuyanagi, H. (2022). Role of microbiota in viral infections and pathological progression. Viruses 14:950. doi: 10.3390/v14050950
Nordestgaard, R. L. M., Spiegelhauer, M. R., Frandsen, T. H., Gren, C., Stauning, A. T., and Andersen, L. P. (2018). “Clinical manifestations of the epsilonproteobacteria (helicobacter pylori)” in Helicobacter Pylori - New Approaches of an Old Human Microorganism [Internet]. ed. B. M. Roesler (London: IntechOpen)
Ochani, R., Asad, A., Yasmin, F., Shaikh, S., Khalid, H., Batra, S., et al. (2021). COVID-19 pandemic: from origins to outcome. A comprehensive review of viral pathogenesis, clinical manifestation, diagnostic evaluation, and management. Infez. Med. 29, 20–36.
Olender, A. (2013). Antibiotic resistance and detection of the Most common mechanism of resistance (MLSB) of opportunistic Corynebacterium. Chemotherapy 59, 294–306. doi: 10.1159/000357467
Parada Venegas, D., De la Fuente, M. K., Landskron, G., González, M. J., Quera, R., Dijkstra, G., et al. (2019). Short chain fatty acids (SCFAs)-mediated gut epithelial and immune regulation and its relevance for inflammatory bowel diseases. Front. Immunol. 10:277. doi: 10.3389/fimmu.2019.00277, Erratum in: Front. Immunol. 2019 June 28;10:1486
Parks, D. H., Tyson, G. W., Hugenholtz, P., and Beiko, R. G. (2014). STAMP: statistical analysis of taxonomic and functional profiles. Bioinformatics 30, 3123–3124. doi: 10.1093/bioinformatics/btu494
Qu, L., Cheng, Q., Wang, Y., Mu, H., and Zhang, Y. (2022). COPD and gut-lung Axis: how microbiota and host inflammasome influence COPD and related therapeutics. Front. Microbiol. 1:868086. doi: 10.3389/fmicb.2022.868086
Quast, C., Pruesse, E., Yilmaz, P., Gerken, J., Schweer, T., Yarza, P., et al. (2013). The SILVA ribosomal RNA gene database project: improved data processing and web-based tools. Nucleic Acids Res. 41, D590–D596. doi: 10.1093/nar/gks1219
Renom, F., Gomila, M., Garau, M., Gallegos, M. C., Guerrero, D., Lalucat, J., et al. (2014). Respiratory infection by Corynebacterium striatum: epidemiological and clinical determinants. New Microbes New Infect. 2, 106–114. doi: 10.1002/nmi2.48
Rezaei Bookani, K., Marcus, R., Cheikh, E., Parish, M., and Salahuddin, U. (2017). Corynebacterium jeikeium endocarditis: a case report and comprehensive review of an underestimated infection. IDCases 11, 26–30. doi: 10.1016/j.idcr.2017.11.004
Riegel, P., Heller, R., Prevost, G., and Jehl, F. (1997). Monteil H.52 Mollmann S, 2014. Corynebacterium durum sp. nov., from human clinical specimens. Int. J. Syst. Bacteriol. 47, 1107–1111. doi: 10.1099/00207713-47-4-1107
Rintala, H. (2011). Actinobacteria in indoor environments: exposures and respiratory health effects. Front. Biosci. S3, 1273–1284. doi: 10.2741/225
Shinha, T. (2015). Fatal bacteremia caused by campylobacter gracilis, United States. Emerg. Infect. Dis. 21, 1084–1085. doi: 10.3201/eid2106.142043
Wemheuer, F., Taylor, J. A., Daniel, R., Johnston, E., Meinicke, P., Thomas, T., et al. (2020). Tax4Fun2: prediction of habitat-specific functional profiles and functional redundancy based on 16S rRNA gene sequences. Environ. Microbiome 15:11. doi: 10.1186/s40793-020-00358-7
Yamamoto, S., Saito, M., Tamura, A., Prawisuda, D., Mizutani, T., and Yotsuyanagi, H. (2021). The human microbiome and COVID-19: a systematic review. PLoS One 16:e0253293. doi: 10.1371/JOURNAL.PONE.0253293
Yang, K., Kruse, R. L., Lin, W. V., and Musher, D. M. (2018). Corynebacteria as a cause of pulmonary infection: a case series and literature review. Pneumonia 10:10. doi: 10.1186/s41479-018-0054-5
Yao, Y., Tian, J., Meng, X., Kan, H., Zhou, L., and Wang, W. (2022). Progression of severity in coronavirus disease 2019 patients before treatment and a self-assessment scale to predict disease severity. BMC Infect. Dis. 22:409. doi: 10.1186/s12879-022-07386-3
Yeoh, Y. K., Zuo, T., Lui, G. C., Zhang, F., Liu, Q., Li, A. Y., et al. (2021). Gut microbiota composition reflects disease severity and dysfunctional immune responses in patients with COVID-19. Gut 70, 698–706. doi: 10.1136/gutjnl-2020-323020
Zhang, D., Li, S., Wang, N., Tan, H. Y., Zhang, Z., and Feng, Y. (2020). The cross-talk between gut microbiota and lungs in common lung diseases. Front. Microbiol. 11:301. doi: 10.3389/fmicb.2020.00301
Zuo, T., Wu, X., Wen, W., and Lan, P. (2021). Gut microbiome alterations in COVID-19. Genomics Proteomics Bioinformatics 19, 679–688. doi: 10.1016/j.gpb.2021.09.004
Keywords: microbiota (16S rRNA), COVID-19, biomarkers, SARS-CoV-2, intensive and critical care
Citation: Mazzarelli A, Giancola ML, Fontana A, Piselli P, Binda E, Trivieri N, Mencarelli G, Marchioni L, Vulcano A, De Giuli C, Panebianco C, Villani A, Copetti M, Perri F, Fontana C, Nicastri E and Pazienza V (2022) Gut microbiota composition in COVID-19 hospitalized patients with mild or severe symptoms. Front. Microbiol. 13:1049215. doi: 10.3389/fmicb.2022.1049215
Edited by:
Guoliang Zhang, Shenzhen Third People’s Hospital, ChinaReviewed by:
Abhishek Asthana, Cleveland Clinic, United StatesRodrigo Garcia-Lopez, National Autonomous University of Mexico, Mexico
Copyright © 2022 Mazzarelli, Giancola, Fontana, Piselli, Binda, Trivieri, Mencarelli, Marchioni, Vulcano, De Giuli, Panebianco, Villani, Copetti, Perri, Fontana, Nicastri and Pazienza. This is an open-access article distributed under the terms of the Creative Commons Attribution License (CC BY). The use, distribution or reproduction in other forums is permitted, provided the original author(s) and the copyright owner(s) are credited and that the original publication in this journal is cited, in accordance with accepted academic practice. No use, distribution or reproduction is permitted which does not comply with these terms.
*Correspondence: Emanuele Nicastri, emanuele.nicastri@inmi.it; Valerio Pazienza, pazienza_valerio@yahoo.it
†These authors have contributed equally to this work
‡These authors have contributed equally to this work and share senior authorship