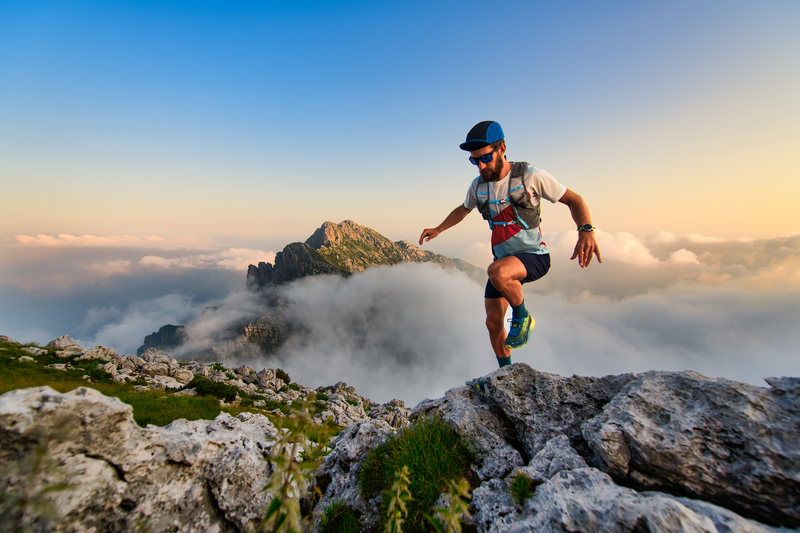
95% of researchers rate our articles as excellent or good
Learn more about the work of our research integrity team to safeguard the quality of each article we publish.
Find out more
ORIGINAL RESEARCH article
Front. Microbiol. , 02 December 2022
Sec. Aquatic Microbiology
Volume 13 - 2022 | https://doi.org/10.3389/fmicb.2022.1037626
Lake Cajititlán is a subtropical and endorheic lake, which is heavily impacted by nutrient pollution. Agricultural runoff and poorly treated wastewater have entered this reservoir at alarming rates during past rainy seasons, causing the cultural eutrophication of this body of water and resulting in several massive fish kill events. In this study, shotgun metagenomic sequencing was used to examine the taxonomic and functional structure of microbial communities in Lake Cajititlán during the rainy season. Several water quality features and their interactions with microbial communities were also assessed to identify the major factors affecting the water quality and biota, specifically fish species. According to current water quality regulations, most of the physicochemical variables analyzed (dissolved oxygen, pH, Secchi disk, NH4+, NO3−, blue-green algae, total phosphorus, and chlorophyll-a) were outside of the permissible limits. Planktothrix agardhii and Microcystis aeruginosa were the most abundant phytoplankton species, and the dominant bacterial genera were Pseudomonas, Streptomyces, and Flavobacterium, with Pseudomonas fluorescens, Stenotrophomonas maltophilia, and Aeromonas veronii representing the most abundant bacterial species. All of these microorganisms have been reported to be potentially harmful to fish, and the latter three (P. fluorescens, S. maltophilia, A. veronii) also contain genes associated with pathogenicity in fish mortality (fur, luxS, aer, act, aha, exu, lip, ser). Genetic evidence from the microbial communities analyzed herein reveals that anthropogenic sources of nutrients in the lake altered genes involved in nitrogen, phosphorus, sulfur, and carbon metabolism, mainly at the beginning of the rainy season. These findings suggest that abiotic factors influence the structure of the microbial communities, along with the major biogeochemical cycles of Lake Cajititlán, resulting in temporal variations and an excess of microorganisms that can thrive in high-nutrient and low-oxygen environments. After reviewing the literature, this appears to be the first study that focuses on characterizing the water quality of a subtropical hypereutrophic lake through associations between physicochemical variables and shotgun metagenomic data. In addition, there are few studies that have coupled the metabolism of aquatic ecosystems with nutrient cycles.
Anthropogenic activities and processes negatively impact water sources around the world, degrading the health of these systems, along with the ecological services they support and the biodiversity they harbor (Dokulil et al., 2000; de Anda and Shear, 2001; Skoulikidis et al., 2006; Skoulikidis, 2009; Kagalou et al., 2012; Kalogianni et al., 2017; Abell et al., 2019; Katsuki et al., 2019; Yu et al., 2020; Díaz-Torres et al., 2021).
In freshwater environments, phytoplankton make up the autotrophic component of the planktonic community and are thus the base of the food web. Massive phytoplankton blooms impact aquatic habitats by blocking light penetration and depleting oxygen at night (Ssebiyonga et al., 2013). In addition, certain genera of cyanobacteria (Microcystis, Anabaena, Planktothrix, Oscillatoria, Anabaenopsis, Nostoc) can be toxic to fish because they produce microcystins, a class of peptide toxins that can cause cell damage and organismal death by inhibiting phosphatases (Fu et al., 2005). Furthermore, some of the bacterial species can cause serious disease in fish. For instance, Aeromonas veronii can affect freshwater fish, amphibians, birds, and mammals (D’Aloia et al., 1996; Ghenghesh et al., 1999; Zhang et al., 2019). Moreover, Pseudomonas fluorescens is the most common culprit of fish illness and is frequently associated with skin and fin diseases (Pękala-Safińska, 2018). There have likewise been reports of health disorders and mortality in freshwater fish caused by Shewanella putrefaciens, Acinetobacter spp., and Stenotrophomonas maltophilia (Pękala-Safińska, 2018).
Changes in lake water quality impact microbial communities and their metabolic activities, altering biogeochemical processes such as sulfur, nitrogen, phosphorus, and carbon metabolism (Hupfer and Lewandowski, 2008; Liu et al., 2018; Wang et al., 2018; Yao et al., 2018). However, there is still limited knowledge on the interactions between water quality and biogeochemical processes within tropical and subtropical lake ecosystems (Díaz-Torres et al., 2021, 2022). Therefore, it is crucial to understand how microbial metabolism drives biogeochemical processes in these types of lakes that have high levels of nutrient pollution (Newton et al., 2011; Graham et al., 2016). Microbial metagenomics has proven to be quite valuable in informing remediation strategies for deteriorated ecosystems (Bowker, 2007; Steven et al., 2012). This technique allows for a fuller understand of the microbial community and what drives its structure, knowledge which is necessary for informed decision making (Guisan et al., 2013). Most of the reported metagenomic studies in lakes have been performed using amplicon sequencing, which is the most widely used approach for characterizing microbial diversity (Sharpton, 2014; Parulekar et al., 2017; Zhang et al., 2018; Nakatsu et al., 2019; Oyewusi et al., 2020). Amplicon sequencing studies in bacteria and archaea usually focus on the small 16S rRNA subunit, which is a phylogenetically and taxonomically informative marker (Hassler et al., 2022). Alternatively, in shotgun metagenomic sequencing, the entire genome is divided into small fragments and individually sequenced. This produces DNA sequences (reads) that align with multiple genomic regions (contigs) (Quince et al., 2017). As a result, metagenomic data allows researchers to investigate the following two elements of a microbial community simultaneously: 1) which organisms are present (bacteria, viruses, eukaryotes, archaea), and 2) what can they do (Hamady and Knight, 2009).
Lake Cajititlán is a small endorheic, subtropical, shallow lake located in the municipality of Tlajomulco de Zúñiga in the Mexican state of Jalisco (Díaz-Torres et al., 2021). This lake is both a symbol of identity and an important source of income for the inhabitants of Tlajomulco and the surrounding area, as the main economic activities of the region (e.g., tourism and fishing) depend on it. However, Lake Cajititlán is currently at risk of severe degradation due to the construction of new housing developments, the overexploitation of aquifers, and because of local, municipal, and agricultural wastewater that is being directly discharged to the lake (Caro-Becerra et al., 2017). Agriculture is the main economic activity in the Lake’s basin. Nevertheless, due to the excessive use of fertilizers, agricultural activities are one of the primary sources of nutrient pollution, resulting in cultural eutrophication process that is exacerbated by the endorreic nature of this reservoir (de Anda et al., 2019). Furthermore, the water quality of this lake is also affected by the lack of tertiary treatments to remove nutrients from wastewater, which is discharged into the lake from three municipal wastewater treatment plants (WWTPs) (de Anda et al., 2019). As a result, the lake has become hypereutrophic, triggering phytoplankton blooms, which have caused several massive fish kills between 2013 and 2022. All of these fish kill events occurred either during or immediately following the rainy season (Gradilla-Hernández et al., 2018; de Anda et al., 2019) due to the high rates of dissolved oxygen (DO) consumption by primary consumers during the night cycle, resulting in oxygen depletion (anoxia/hypoxia) (Gradilla-Hernández et al., 2018, 2020). Understanding the structure and function of microbial communities in freshwater lakes with severe anthropogenic pollution problems, like Lake Cajititlán, would greatly benefit remediation plans. Therefore, the objective of the present study was to assess the associations between water quality and microbial communities within Lake Cajititlán by analyzing several physicochemical variables along with shotgun metagenomic data during the rainy season. After reviewing the literature, this appears to be the first study that focuses on understanding the water quality of a subtropical hypereutrophic lake through associations between physicochemical variables and the structure and function of microbial communities using shotgun metagenomic sequencing.
Lake Cajititlán is a subtropical endorheic lake in the state of Jalisco, located about 25 kilometers from Guadalajara, Mexico’s second largest city (Limón-Macías et al., 1983; Caro-Becerra et al., 2017, p. 21). The storage volume is 70.89 hm3, the maximum depth is 5.4 m, and the surface area is 1,744 ha (de Anda et al., 2019). As reported by Gradilla-Hernández et al. (2019), the hot-dry season in the area lasts from February to May, the rainy season is from June to September, and the cold-dry season is from October to January. According to monthly historical trends (1998–2018) in precipitation for Lake Cajititlán, July receives the most precipitation (7.60 mm), followed by August (5.93 mm) and then September (5.93 mm) (5.47 mm) (CONAGUA, 2021). Annual historical behavior for the TN:TP ratio and the ecosystem-specific water quality index (ES-WQI) for Lake Cajititlán has also been reported, with the lowest ES-WQI values in July and the highest fluctuations in the TN:TP ratio during the rainy season (Díaz-Torres et al., 2021).
Physicochemical data were measured in situ once a month from March to September 2018 at three depths (80 cm, intermediate depth, and interstitial) and at five sampling stations (CEA-1, CEA-2, CEA-3, CEA-4, and CEA-5; Figure 1). Dissolved oxygen (DO), electrical conductivity (EC), pH, water temperature (WT), nitrate (NO3−), ammonium (NH4+), turbidity, oxidation–reduction potential (ORP), phycocyanin-containing blue-green algae (BGA-PC), and chlorophyll-a were measured using two multiparameter probes (6,600 and 6,829 V2 YSI® at xylem brand, OH, United States) (YSI, 2009). Furthermore, during the rainy season (July–September) of 2018, water samples were taken once a month for shotgun metagenomic analysis, as these months have displayed the lowest water quality index values recorded in Lake Cajititlán and because the massive fish kill events were observed during these same months (Gradilla-Hernández et al., 2018; de Anda et al., 2019; Díaz-Torres et al., 2021). Water samples were collected using a Van Dorn water sampler at the same sites and depths that the physicochemical parameters were measured and were placed in disinfected 1 l plastic containers. Two duplicates of each sample (2 l each) were taken, for a total of 60 samples. Total nitrogen (TN) and total phosphorus (TP) measurements were provided by the State Water Commission (CEA by its Spanish abbreviation). These measurements were taken at a depth of 0.8 meters at the same sites and sampling months as the other physicochemical parameters.
Each water sample replicate was first filtered using a membrane with a 20–25 μM pore diameter. The filtrate was subsequently run through a second membrane with a 0.45 μM pore size. The FastDNA Spin Kit for Soil (MP Biomedicals, OH, United States) was then used to extract and purify the DNA from the samples, by adding 100 mg of each filter piece to a lysis matrix according to the manufacturer’s instructions. Quantification of DNA was conducted using a NanoDrop ND-1000 UV–Vis spectrophotometer (NanoDrop Technologies, Wilmington, DE). The DNA samples were then pooled by the depth of each site (CEA-1 to CEA-5) and sampling month (July to September; e.g., sample 1: CEA-1/July/80 cm and interstitial), to ultimately obtain 30 total samples (five samples per sampling month and its replica, July = 10, August = 10, and September = 10). The samples were then cleaned using the AM Pure XP kit (Beckman Coulter, IN, United States), and their DNA was quantified using the Qubit 2.0 fluorometer (Invitrogen, Carlsbad, CA, United States). Additionally, absorbance ratios at 260/280 and 260/230 nm were measured to analyze the purity of the samples. Finally, the samples were subjected to shotgun metagenomic sequencing on the Illumina® Novaseq6000 platform (Illumina, San Diego, CA, United States), yielding paired-end read lengths of 2 × 150 bp and 5 Gb of clean data per sample. Sequencing was carried out at the National Genomic Sequencing Laboratory Tec-BASE of Tecnologico de Monterrey.
OmicsBox 2.0.36 bioinformatics software was used to analyze and process the raw reads from the Lake Cajititlán samples (BioBam Bioinformatics, 2019). The reads were preprocessed using the Trimmomatic tool, which includes removing adapters and contaminant sequences, trimming bases, and filtering short, low-quality reads (Bolger et al., 2014). Subsequently, the samples were subjected to a quality control check using the FASTQ tool (Andrews, 2010). The final clean paired-end reads for each sample were individually subjected to metagenomic assembly using metaSPAdes, which is an assembly toolkit containing several assembly pipelines based on the de Bruijn graph (Nurk et al., 2017). The assemblages were then compared to the RefSeq v.2021_04 database using Kraken2 to obtain the microbial composition of Lake Cajititlán (Wood et al., 2019). Metagenomic gene prediction was performed using FragGeneScan, which is an application to find (fragmented) genes in short reads (Rho et al., 2010). The samples were then functionally annotated using the EggNOG mapper, which employs precomputed EggNOG-based orthology assignments (Huerta-Cepas et al., 2018). Finally, using the “Sample comparison GO chart” function, a comparative analysis of genetic ontology annotations was performed between the different samples of each month.
All statistical analyses were carried out using the R (R version 4.1.2) software unless otherwise stated (R Core Team, 2021). All bar graphs and line plots were constructed using the ggplot2 package (Wickham, 2016). The heatmap was developed using the dist() function and the pheatmap package (Kolde, 2019). Mean values and standard deviations (SD) were calculated for each physicochemical parameter by sampling month with the mean() and sd() functions. One-way analyses of variance (ANOVA) (α = 0.05) were performed to test for temporal differences in water quality features. The mean physicochemical parameter values were compared to different national standards and international recommendations (Vollenweider and Kerekes, 1981, 1982; Salas, 2003; WHO, 2003; MRCCC, 2013; LFD, 2019; NOM-001, 2021).
A box plot was created to depict the relative read abundances of Lake Cajititlán’s microbial communities at the domain-level (archaea, bacteria, eukaryotes, and viruses). The relative read abundance of each of the 30 samples was calculated individually, and the results were plotted by sampling month. All boxplots were constructed to incorporate the results of one-way ANOVA (α = 0.05) and Tukey’s HSD tests, which were performed using the stats package’s TukeyHSD function (R Core Team, 2021). Phytoplankton species and bacterial genera were examined using bar graphs of relative read abundance over the sampling month. Bar charts were created using the scales packages. Taxa of phytoplankton species and bacterial genera that were found in proportions of less than 0.01%, were categorized as “others” (Wickham and Seidel, 2022).
Principal coordinate analysis (PCoA) was also performed to investigate differences in phytoplankton species and bacterial genera across the sampling months. The vegan package and the cmdscale function were used to calculate distances based on Bray-Curtis differences (Oksanen et al., 2022). Furthermore, a pairwise analysis of variance (ADONIS) was also conducted using the vegan package and the adonis2() function to test the significance of temporal changes in the composition of Lake Cajititlán’s bacterial and phytoplankton communities (Clarke, 1993; Anderson, 2001; Anderson et al., 2006). Additionally, a redundancy analysis (RDA) was performed to determine the relationship between these communities and the physicochemical parameters using the vegan and ggord packages (Beck, 2021; Oksanen et al., 2022).
A literature search was conducted to analyze which bacterial species have been reported as pathogenic to fish using different terms or keywords such as pathogenic bacteria, fish pathogens, fish pathogenic bacteria, fish bacterial disease, fish diseases, and fish pathogens, among others. This search was performed using PubMed, PubMed Central, ScienceDirect, JSTOR and Scopus databases, resulting in 22 articles relevant to the objective (Almodóvar et al., 2004; Ellermeier and Slauch, 2007; Geng et al., 2007; Swain et al., 2007; Ilardi et al., 2009; Wang et al., 2009; Geng et al.,2010a,b; Loch and Faisal, 2010; Troxell et al., 2010; Dang et al., 2011; Johnson et al., 2011; Humbert et al., 2013; Ngwa et al., 2014; Liu et al., 2015; Harke et al., 2016; Menanteau-Ledouble et al., 2016; Bukowska et al., 2017; Pękala-Safińska, 2018; Basri et al., 2020; Li et al., 2020; Shabana et al., 2022). These species were then compared with the taxonomic data obtained herein and were displayed using a relative read abundance on a chord diagram created with the circlize package (Gu et al., 2014). In addition, a heatmap was built using the virulence factors that play an important role in the development of diseases caused by the most abundant species by sampling month. These analyses were carried out to infer potential synergistic factors (i.e., bacterial diseases, changes in the physicochemical parameters of the water, harmful cyanobacteria, eutrophication, and anthropogenic activities) that could have triggered the massive fish kill events in Lake Cajititlán.
Genes involved in the metabolism of carbon, nitrogen, and sulfur, as well as in the phosphorus cycle were searched for in the KEGG database1, and the abundance of the group of genes by metabolic pathway was shown through bar charts of relative read abundance per sampling month using the phyloseq and vegan packages (Kanehisa et al., 2015; McMurdie and Holmes, 2013; Oksanen et al., 2022). Finally, the metabolic pathways that were statistically significant for each sampling month were thoroughly analyzed, and a graphic representation of the metabolic pathways, their stages, and the genes involved was created, followed by a Euclidean distance heatmap to visualize gene abundance per sampling month (Kolde, 2019).
All physicochemical parameters, except for TN and TP, showed significant temporal fluctuations when comparing the values reported for the seven sampling months (Table 1). Most of these parameters were outside the permissible limits. However, March (which is in the hot-dry season) showed the fewest parameters outside the limits. WT and EC were the only variables that were within acceptable limits for all the months analyzed. The lowest ORP values and highest turbidity values were observed in July, whereas the highest TN values were observed in June, the onset of the rainy season (Gradilla-Hernández et al., 2019). Compared with the limits established by the applicable Mexican regulation, the TP, DO, and pH were outside of the permissible limits for all months, except for the DO, which was found to be within these guidelines for March and June (Table 1).
The sequencing of all samples resulted in a total of 748,721,392 raw reads and 748,721,392 contigs (Supplementary Table S1). Kraken2 was used to compare the reads to the RefSeq v.2021_04 reference database, resulting in 29,544,581 total reads (49.87%) that were classified in one of the following taxonomic domains: bacteria (46.45%), eukaryotes (3.17%), archaea (0.27%) and viruses (0.07%) (Supplementary Table S1). Regarding EggNOG Mapper results, a predicted gene annotation of 12,491,565 (> 18%) was obtained (Supplementary Table S2). Classified reads per month ranged from 49.98 to 51.72% (Supplementary Table S2). Statistical analyses revealed no significant differences in the abundance of archaea and viruses per sampling month (Figure 2). However, temporal differences were detected in the bacteria and eukaryotes. Furthermore, an inverse trend was observed between these two domains in the first 2 months (July and August); while the relative read abundance of bacteria decreased, the abundance of eukaryotes increased (Figure 2).
Figure 2. Relative read abundance of Lake Cajititlán’s microbial communities at the domain level by sampling month. (A) Archaea. (B) Bacteria. (C) Eukaryota. (D) Viruses. Relative read abundances were calculated separately for each domain.
The diversity and abundance of phytoplankton (Figure 3) and bacterial communities (Figure 4) were assessed at different sampling months using relative read abundance bar plots. The temporal differences were examined using PCoA. RDAs were then used to evaluate the relationship between the presence and prevalence of these communities and the physicochemical variables of Lake Cajititlán. Within the phytoplankton communities, Planktothrix agardhii (17.70%) and Microcystis aeruginosa (14.00%) were consistently the most abundant species during all sampled months (Figure 3A). The Pseudomonas (8.95%), Streptomyces (5.32%), and Flavobacterium (3.36%) bacterial genera were the most abundant in all sampled months (Figure 4A). Significant temporal variations were only detected in the composition of the phytoplankton (p < 0.05) and bacterial (p < 0.05) communities between July and September according to the ADONIS analysis (but not observed with the PCoA analysis) (Figure 3B, 4B; Supplementary Table S3, S4).
Figure 3. (A) Phytoplankton species relative read abundance by sampling month. (B) PCoA of phytoplankton communities by sampling month. (C) RDA of phytoplankton composition and physicochemical variables by sampling month. Dissolved oxygen (DO), pH, water temperature (WT), oxidation–reduction potential (ORP), ammonium (NH4+), nitrate (NO3−), blue-green algae (BGA-PC), and chlorophyll-a and electrical conductivity (EC) total nitrogen (TN), total phosphorus (TP), and Turbidity.
Figure 4. (A) Relative read abundance of bacteria genera by sampling month. (B) PCoA of bacterial communities by sampling month. (C) RDA of bacterial composition and physicochemical variables by sampling month. Dissolved oxygen (DO), pH, water temperature (WT), oxidation–reduction potential (ORP), ammonium (NH4+), nitrate (NO3−), blue-green algae (BGA-PC), and chlorophyll-a and electrical conductivity (EC) total nitrogen (TN), total phosphorus (TP), and Turbidity.
According to the RDA, the physicochemical factors that were most correlated with the taxonomic composition of the phytoplankton communities during July were TP and pH. Similarly, NH4+, NO3−, and TP were most correlated with the communities in August and EC and turbidity were most correlated with the taxonomic composition in September (Figure 3C). The first three redundancy components explained 55% of the variability (RDA1: 25%; RDA2: 17%; RDA3: 13%; Supplementary Table S5). The first component was positively correlated with ORP. The second component was negatively correlated with NH4+ and TN, but positively correlated with BGA-PC. Turbidity, ORP, pH, and NO3-were the most highly (positively) correlated parameters with the third component (Supplementary Table S6). Likewise, WT, NH4+, chlorophyll-a and BGA-PC were most correlated with the taxonomic composition of the bacterial communities in July. NH4+, TP, and TN were most correlated with the communities in August, whereas TP, ORP, and DO were more correlated with the taxonomic composition in September (Figure 4C). The first three components explained 61% of the variability (RDA1: 39%; RDA2: 14%; RDA3: 8%; Supplementary Table S7). DO, BGA-PC, and TN were most (positively) correlated with the first component. The second component was most correlated with TN (positive correlation), along with BGA-PC and chlorophyll-a (both with negative correlations). DO and TP were the most highly (positively) correlated with the third component (Supplementary Table S8).
Parameters with positive correlations may imply that microorganisms thrive in environments with higher values of these physicochemical factors. Conversely, negative correlations indicate that higher values of these parameters negatively influence the predominance of microbial populations during the study period (Liu et al., 2014).
A literature search was carried out to determine which bacterial species have been reported as fish pathogens in other freshwater bodies to compare with this study. Pseudomonas fluorescens (21.37%), Stenotrophomonas maltophilia (18.86%), and Aeromonas veronii (12.58%) were the most abundant species, as shown in in Figure 5. The monthly abundance of P. fluorescens (50.03%) was higher in July, and its abundance decreased throughout the sampling months. The relative abundance of S. maltophilia remained constant during the three-month investigation. The highest abundance of A. veronii was observed in August (50.73%), followed by July (38.74%) and September (10.53%). The remaining pathogenic bacteria in the chord diagram were found to be less abundant for all 3 months of study.
Figure 5. Chord diagram showing the distribution of bacterial species that have been reported as pathogenic for fish. The numbers around the circumference represent the percentage of relative abundance coverage of the bacterial species by sampling month in Lake Cajititlán.
P. fluorescens is one of the main causes of septicemic diseases in freshwater fish, causing severe economic losses in aquaculture (Swain et al., 2007; Wang et al., 2009; Shabana et al., 2022). This species is usually associated with pathologies of the skin and fins of fish and has also been reported to cause sudden mortality in fish (Pękala-Safińska, 2018). A. veronii is a major pathogen causing sepsis and ulcer syndrome in freshwater fish (Li et al., 2020). S. maltophilia has been reported to cause infectious intussusception syndrome in these freshwater animals (Geng et al., 2007, 2010a,b). The virulence factors that play an essential role in the development of diseases caused by P. fluorescens and A. veronii in fish are depicted in a heatmap (Supplementary Figure S1). So far, no information has been found on virulence genes associated with S. maltophilia fish diseases. For P. fluorescens to be infectious to the host, the acquisition of iron is essential, which is related to the pathogenic fur gene (Ellermeier and Slauch, 2007; Troxell et al., 2010; Johnson et al., 2011; Liu et al., 2015). The highest relative abundance of the fur gene occurred in July, followed by September, while the fewest were observed in August (Supplementary Figure S1). The genes responsible for diseases (sepsis and ulcer syndrome) caused by A. veronii are as follows: aer, act, aha, ser, exu, lip, and luxS (Li et al., 2020). The heatmap shows a trend like that of the fur gene, with all virulence genes most abundant in July, followed by September, and then August. The exu (nuclease) gene was the most abundant gene, while luxS (quorum sensing controlled gene) was the least abundant (Li et al., 2020; Supplementary Figure S1).
The relative read abundances of functional genes involved in the biogeochemical cycles for nitrogen, phosphorus, carbon, and sulfur were calculated based on annotated reads (Figure 6). In general, all of the biogeochemical cycle pathways were more abundant in July, then decreased in August, and slightly increased in September. The genes associated with sulfur metabolism only displayed significant differences between July and September (value of p = 0.0352; Figure 6A) for the sulfur oxidation system (SOX system). No significant differences were identified regarding central carbon metabolism (Figure 6B). The genes associated with nitrogen metabolism showed significant differences for all of the following pathways: the denitrification pathway (July vs. September: value of p = 0.0169), the comammox pathway (July vs. September: value of p = 0.0257), the dissimilatory nitrate reduction to ammonia (DNRA) pathway (July vs. August: value of p = 0.0017; July vs. September: value of p = 0.0016), the assimilatory nitrate reduction to ammonia pathway (ANRA) (July vs. August: value of p = 0.0007 July vs. September: value of p = 0.0001), and the nitrification pathway (July vs. September: value of p = 0.0317). There were no temporal variations in the nitrogen fixation or the anammox pathways (Figure 6C). Regarding the phosphorus cycle, the relative read abundance of genes involved in microbial regulation of organic P-mineralization (OPM) was significantly different between July and September (value of p = 0.0412). There were no significant temporal variations in the genes involved in inorganic P solubilization or P uptake and transport (IPS-PUT), nor in the genes related to P-starvation response regulation (PSRR; Figure 6D).
Figure 6. Relative read abundance of genes associated with major pathways in (A) Sulfur metabolism–ASR, assimilatory sulfate reduction; DSR, dissimilatory sulfate reduction; SOX system, sulfur oxidation system. (B) Central carbon metabolism–CFPP, carbon fixation pathways in prokaryotes; CFPO, carbon fixation in photosynthetic organisms. (C) Nitrogen metabolism–DNRA, dissimilatory nitrate reduction to ammonia; ANRA, assimilatory nitrate reduction to ammonia. (D) Phosphorus cycle–IPS-PUT, Inorganic P solubilization and P uptake and transport; OPM, organic P-mineralization; PSRR, P-starvation response regulation. Different letters indicate significant differences within a single pathway.
The metabolic pathways that were statistically different between sampling month for relative read abundances were further analyzed using an Euclidean distance heatmap and a graphical representation. (Figure 7A). All pathways for the nitrogen cycle are depicted since the majority displayed significant differences between sampling months (Figure 7B). The SOX system and the OPM pathways (Figure 7C) represent sulfur and phosphorus metabolism, respectively (Figure 7D). The pathways involved in central carbon metabolism are not displayed because no temporal variations were detected.
Figure 7. (A) Heatmap of significant metabolic pathways and their genes. (B) Nitrogen metabolism. (C) Sulfur pathway; sulfur oxidation system (SOX system). (D) Phosphorus pathway; organic P-mineralization (OPM). Genes with asterisks indicate that they were detected in this study.
The heatmap reveals that most of the studied genes were overrepresented in July, then declined in August, and then some slightly surged again in September, particularly those of the SOX system pathway (Figure 7D). However, notable exceptions were detected for some genes, such as the nosZ gene of the denitrification pathway, which is required to obtain N2 from N2O, where nosZ was more highly represented in July and August and displayed the lowest annotated reads in September. Reads for the amoCAB genes, which are necessary to produce hydroxylamine (NH2OH) from NH4+, decreased throughout the months of the study (Figure 7B). The soxZ gene, which is required to produce S, SO3 and SH in the SOX system pathway of sulfur metabolism, was most abundant in September, followed by July, and August (Figure 7C). Finally, the phn gene, which is required for the mineralization of organic phosphorus in the OPM pathway of the phosphorus cycle, was most abundant in August, followed by July and September (Figure 7D).
Most physicochemical parameters (except for WT and EC) from March to September 2018 were outside of the permissible limits defined by the applicable Mexican regulation and international water quality recommendations (Table 1). This is concerning because the reservoir’s main activity is commercial fishing as well as weekend recreational boat trips, implying an impact on the local economy, i.e., loss of tourism, aesthetic value, fisheries, and seriously affecting the food web dynamics and nutrient balance (de Anda et al., 2019).
The rapid changes in water quality parameters during the wet season have been attributed to runoff caused by heavy rainfall (Díaz-Torres et al., 2021, 2022). Furthermore, significant temporal changes in physicochemical parameters can be observed even during the dry-hot season (March–May; Table 1), indicating that these alterations could be due mainly to climate change impacts on the hydrological cycle of Lake Cajititlán (Inglezakis et al., 2016). Consequently, the physicochemical and biological properties of this body of water are concerning throughout the year. Other research in lakes and estuaries have focused on the influence of climate change on water temperature, nutrient loads, and eutrophication (Kirilova et al., 2011; Chaturvedi et al., 2021). An analysis of the potential effects of climate change in the basin region of Lake Cajititlán would allow for a better understanding of the seasonal dynamics in the reservoir’s physicochemical parameters.
Eutrophication is assessed using the OECD’s trophic status classification, which considers three parameters: TP, chlorophyll-a, and secchi disc transparency (Caspers, 1984). According to the findings of this study, Lake Cajititlán is classified as hypereutrophic because the values of these three parameters fell into that category during all 7 months of evaluation (Table 1). This is the worst OECD classification in terms of trophic status and water quality in bodies of water. According to Ram et al. (2014) and Kangur et al. (2005), the main causes for massive fish kills in different bodies of water include natural and anthropogenic hypoxia as well as the proliferation of harmful algae. Accordingly, the presence of phytoplankton biomass as well as fish killed or fish gasping for air on the surface of Lake Cajititlán were observed during the monitoring activities of this study. Moreover, cyanobacteria species that are capable of producing toxins that harm fish were identified through shotgun metagenomics. Five of the seven months studied (Table 1) displayed DO levels below the acceptable limit for aquatic life (5 mg/L; LFD, 2019), which could be driving the mass fish kill episodes in Lake Cajititlán [Smith and Schindler, 2009; Organisation for Economic Co-operatin and Development (OECD)].
There are very few studies on the analysis of microbial communities of a freshwater system, and even fewer a eutrophic state and are being analyzed with shotgun metagenomics. In this have study, approximately 50% of the total reads were classified taxonomically (Table S1). These findings were compared with a similar study, which examined water samples from soda lakes with and without cyanobacterial blooms and using the same metagenomic pipeline as this study. The authors analyzed 24 water samples and obtained about 14 million reads, of which 5,385,139 reads were taxonomically assigned (less than 50%; Andreote et al., 2018). Therefore, it is possible to infer that current genomic databases have limitations and are not representative of water microbiomes. Most of the information added to existing databases comes from pharmaceutical and industrial biotechnology, and human health research efforts, which can mislead genetic annotations of water microbiomes (for example, annotations that are obviously incompatible with water ecosystems), or there is not enough information on water microbial communities to annotate metagenomic studies (Wu et al., 2009; Segata et al., 2012; Huttenhower et al., 2012; Choi et al., 2016). To address the demands in water-related research topics, which have also risen significantly in recent years, it is important to curate databases using genomic data derived from cultured representatives originating from water.
Significant temporal differences were observed in the composition of phytoplankton and bacterial communities between July and September (Supplementary Tables S3, S4). This suggests that the abundance and diversity of these two communities are related, as has been shown in other lakes where changes in environmental factors and phytoplankton communities have influenced the composition of bacterial communities and ecosystem-wide changes (Woodhouse et al., 2015; Ramanan et al., 2016). Planktothrix agardhii and Microcystis aeruginosa were the most abundant species observed in this study (Figure 3A). These findings are consistent with a study carried out in Lake Cajititlán that analyzed the 16S and 18S rRNA genes to study the phytoplankton communities of this reservoir, in which the genera Planktothrix spp. and Microcystis spp. were identified. However, the target metagenomic analysis used in that study did not allow for the classification of these phytoplankton groups at the species level (Díaz-Torres et al., 2021). Other studies have found that these two cyanobacterial species are the most dominant in phytoplankton communities, as well as in the harmful algae blooms in other shallow, subtropical, and eutrophic water bodies, such as Lake Cajititlán using other methods (e.g., Malassez counting chamber with a microscope; Kruk et al., 2002; Suda et al., 2002; Bouchamma et al., 2004; Briand et al., 2008; Gągała et al., 2010). This suggests that Lake Cajititlán provides favorable conditions for the development of P. agardhii and M. aeruginosa. Additionally, the genes involved in the production of microcystins (mcy-genes) have been investigated, revealing that Planktothrix and Microcystis predominate in blooms of toxigenic cyanobacteria in freshwater bodies (Dang et al., 2011; Humbert et al., 2013; Ngwa et al., 2014; Harke et al., 2016; Bukowska et al., 2017). Thus, the production of microcystins could be involved in the massive fish kill events that have occurred in Lake Cajititlán. This toxin has already been detected in the water of Lake Cajititlán in a previous study (Díaz-Torres et al., 2021), but toxicological studies regarding bioaccumulation in fish tissues are still needed to confirm this theory.
The most abundant bacterial genera were Pseudomonas, Streptomyces, and Flavobacterium (Figure 4A). This is consistent with other studies that have found these taxa to be among the most abundant genera in freshwater systems, as well as eutrophic and hypereutrophic water bodies (Choi et al., 2005; Michaud et al., 2012; Shao et al., 2021). This suggests that these bacteria thrive in subtropical and eutrophic environments, like Lake Cajititlán. Moreover, they coincide with the most abundant bacterial genera reported by Díaz-Torres et al. (2021) using the 16S rRNA gene, except for Streptomyces, which was not even identified as one of the most abundant genera (> 0.01%). Additionally certain genera that were detected herein (Acidovorax, Acinetobacter, Aeromonas, Agrobacterium, Devosia, Microbacterium, Mycobacterium, Mycolicibacterium, Nocardioides, Rhizobium, Rhodococcus, and Streptomyces) were not detected in that previous study, and vice versa (Aromatoleum, Chthoniobacter, CL500 and Cylindrospermopsis). However, this is expected, as shotgun metagenomic sequencing analysis has potential advantages over meta-barcoding sequencing, such as more genetic information per sample (higher sequencing depth) and the possibility for a better quantitative match (Bell et al., 2021) Furthermore, shotgun metagenomic sequencing covers all genetic information in a sample, so the data may be utilized for metabolic function profiling, metagenomic assembly and binning, antibiotic resistance gene profiling, among other things (Segata et al., 2012; Sunagawa et al., 2013; Laudadio et al., 2019).
NH4+, TP, TN, ORP, DO, EC, BGA-PC, and turbidity were the most correlated factors with the prevalence of phytoplankton and bacterial communities throughout the research period according to the RDA. The TN and NH4+ parameters are important water quality factors that influence the distribution of bacterial and phytoplankton communities. These organisms need a substantial quantity of nitrogen for the synthesis of their primary constituents, which include NAD, purines and pyrimidines, amino acids, and amino sugars. Furthermore, both these organisms and archaea are key drivers in the transformation of diverse types of N into chemical forms used by plants (NH4+ and NO3−) (Reitzer, 1996; Wang et al., 2018). In Lake Cajititlán, rains have been reported to cause significant fluctuations in nutrient concentrations, which increase during the wet season due to surface runoff containing large amounts of nitrogenous and phosphorous fertilizers (Gradilla-Hernández et al., 2020; Díaz-Torres et al., 2021, 2022). This is consistent with the were higher values of NH4+ observed in the wet season (June–September; mean = 3.52 mg/L) compared to the dry season (March–May; mean = 2.50 mg/L). In terms of TP, no significant differences were detected. Because microorganisms and plants require P in the form of phosphates, which were not evaluated in this study, the direct influence of this element on bacterial and phytoplankton populations in Lake Cajititlán cannot be correlated (CEDRSSA, 2019; Zheng et al., 2019).
The most abundant species during the three months of this study were Pseudomonas fluorescens, Stenotrophomonas maltophilia, and Aeromonas veronii (Figure 5). The most important species in fish pathology is P. fluorescens, which is frequently associated with skin problems and fin diseases. Furthermore, infections with this species have been associated with sudden fish kills (Pękala-Safińska, 2018). The ferric uptake regulator gene (fur) in the P. fluorescens pathogen is essential for optimum infection to occur in fish, as this gene regulates the expression of several proteins (Liu et al., 2015). In this study, this species relative read abundance was greatest in July, which matched with the highest abundance of fur gene reads. However, no direct association was observed in August and September (Figure 5, Supplementary Figure S1). S. maltophilia is commonly isolated from freshwater fish (Juhnke and des Jardin, 1989; Dungan et al., 2003), causing lethargy, skin depigmentation, focal hemorrhages, petechiae, and edema in the body cavity, and even fish mortality (Geng et al., 2010a).
A. veronii has been reported to infect freshwater fish, amphibians, birds, and mammals, causing significant losses in the aquaculture sector and threatening food safety (D’Aloia et al., 1996; Ghenghesh et al., 1999; Zhang et al., 2019). Moreover, A. veronii can cause infections in humans, particularly the elderly and immunocompromised children, resulting in sepsis, gastroenteritis, and other diseases (Mencacci, 2003; Roberts et al., 2006; Chen et al., 2015). Because this species was the third most abundant in this research, it is imperative that aquatic products from Lake Cajititlán undergo proper food safety inspection before consumption (Figure 5). Previous research shows that the pathogenicity of A. veronii in fish is multifactorial. In this study, seven important virulence genes (aer, act, aha, ser, exu, lip, and luxS) were detected (Supplementary Figure S1; Li et al., 2020). These findings are consistent with an investigation conducted in China, where 203 freshwater fish were collected, and 87 strains of A. veronii isolated, 50% of which carried at least four or more of the virulence genes evaluated in this work (Li et al., 2020). These results are of great importance for future decision-making with the objective of conserving the ecosystem as well as the food safety of aquatic products from Lake Cajititlán. The infection process of P. fluorescens, S. maltophilia, A. veronii and other pathogenic microorganisms, together with stressful factors for the fish, could be the cause of the massive kill events that have been reported at this reservoir (Townsend et al., 1992; Glibert et al., 2002; Kangur et al., 2005). However, toxicological and pathological analyses in fish and proteomic analyses are require to determine whether the genes detected in this study are being expressed. Previous studies of Lake Cajititlán evaluated the 16S rRNA gene at the genus level and made theoretical inferences about which species of pathogenic bacteria could be present according to the genera found (Díaz-Torres et al., 2021, 2022). In the present research, use of the shotgun sequencing approach allowed for the characterization of bacteria in this reservoir at the species level, enabling the identification of previously described species, as can be observed in the chord diagram (Figure 5).
Significant temporal changes in several pathways of nitrogen, phosphorus, and sulfur metabolism were observed in this study (Figure 6C). Regarding nitrogen metabolism, the narGHI, napAB, and nirK genes were the most abundant in the denitrification pathway, which intensified in July (Figure 7A). The first step of the denitrification pathway (NO3− to NO2−) is governed by the first two genes (narGHI and napAB). The second step (NO2− to NO) is mainly regulated by nirK, because its abundance was higher than nirS. The third step (NO to N2O) is controlled by norBC and the last step (N2O to N2) by nosZ. However, this last gene was more abundant in August and its lowest abundance was observed in September (Figures 7A,B). This might imply that each stage of this metabolic pathway does not occur sequentially (Howarth et al., 2011). On the other hand, anaerobic conditions with DO values less than 1.7 mg/L are required for nitrogen removal, and thus for conventional biological denitrification (Daigger, 2014). In this study, DO values lower than required were reported at different sites and sampling depths, mainly in April and May (Supplementary Table S9). However, it is well known that the DO in eutrophic water bodies reaches its peak during the day due to the accelerated photosynthetic process carried out by the excessive amount of phytoplankton (monitoring time of this study 8:00-13:00), and it is depleted during the night, attributed to the respiration metabolism, and perhaps as a result, the genes of microorganisms capable of denitrification are present (Smith and Schindler, 2009; Figures 6C, 7A). On the other hand, these results also suggest that facultative anaerobic microorganisms capable of performing complete denitrification, such as P. aeruginosa, P. fluorescens, P. stutzeri, and P. denitrificans, could carry out the denitrification process at higher concentrations of DO, as demonstrated in a previous study, in which the authors showed that P. stutzeri isolated from a wastewater treatment system, can carry out nitrification and denitrification simultaneously in the presence of high levels of oxygen (bottles containing an atmosphere of 92% oxygen; Su et al., 2001; Liou and Madsen, 2008; Arat et al., 2015; Tang et al., 2020). This information coincides with the fact that Pseudomonas was the most abundant genus in this study (Figure 6C). In addition, the genes (narGHI, norBC, napAB, nirK, nirS, nosZ) involved in the denitrification process were found annotated in the species P. flourescens, P. stutzeri, and P. aeruginosa (Supplementary Table S10); this information suggests that it is worth investigating more about facultative anaerobic microorganisms in water ecosystems since it could be very useful for water remediation processes.
The denitrification process, as well as the anammox, are important in the removal of nitrogen from aquatic ecosystems. However, the anammox pathway showed no temporal variations and was substantially less abundant than the denitrification process (Ward et al., 2009; Bernhard, 2010). Therefore, these results suggest that denitrification is the most important nitrogen removal mechanism in Lake Cajititlán, as previously suggested by Díaz-Torres et al. (2022) using the PICRUSt functional inference approach.
Similar to the denitrification pathway, all genes involved in comammox metabolism were found to be more abundant in July (Figures 6C, 7A). Because this pathway involves the direct conversion of NH3 into NO3-by a single Nitrospira bacterium, also known as comammox Nitrospira, these results correspond to the greatest NH4+ levels shown in July compared to the other months (Lawson and Lücker, 2018). Comammox bacteria are distinguished from other canonical nitrifiers due to their unique metabolic mechanism (Annavajhala et al., 2018; Palomo et al., 2018). While this genus was not classified as the most abundant bacteria in this study, its presence was identified in the metadata, and it became more abundant in July. Furthermore, the genes involved in the comammox process (pmoABC-amoABC, hao, narGH) were found to be annotated in the phylum Nitrospirae, indicating that this bacterium may be carrying out this metabolism in Lake Cajititlán (Supplementary Table S10).
A greater relative read abundance of DNRA-associated genes was identified throughout the research compared to other nitrogen pathways (Figure 6C), indicating that microbial communities in the water column had a sustained capability to convert NO3− to NH3. A greater abundance of all the genes (narGHI, napAB, nirBD, and nrfAH) involved in this metabolic pathway was observed in July, similar to the ANRA pathway. Although the final product of both pathways is the same, ANRA is produced under aerobic conditions when reduced nitrogen is limited, and DNRA occurs when oxygen is limited (anaerobic conditions) and their respective reduced products (NH4+ and N2 + N2O, respectively) are produced in greater amounts (Teje et al., 1981; Henson et al., 2017). Based on the OD measurements (Table 1), the genes of the DNRA pathway are more functional in Lake Cajititlán than the genes of ANRA (Figure 6C). This suggests that the massive bloom of phytoplankton in Lake Cajititlán is mostly attributable to the use of nitrogen from chemical fertilizers that reach this reservoir through surface drag, rather than a symbiotic interaction with atmospheric nitrogen-fixing bacteria (Aasfar et al., 2021).
Nitrification is an aerobic nitrogen oxidation pathway that produces NO3− from NH3 (EPA, 2002). This pathway was the least abundant in the sampled months (Figure 6C), which might be due to the Nitrospira species enhancing comamox metabolism more than nitrifying bacteria conducted nitrification; although Nitrospira bacteria participate in the nitrification pathway, the whole genetic repertory for ammonia and nitrate oxidation (pmoABC-amoABC), hydroxylamine (hao), and nitrate oxidoreductase (narGH) are required for commamox metabolism (Camejo et al., 2017; Palomo et al., 2018). According to genes associated with nitrification metabolism (hao, amoCAB, nrxAB), these were more abundant in July, as were the other nitrogen pathways (Figure 7A). Nitrifying bacteria include species of genera such as Nitrosomonas, Nitrosococcus, Nitrobacter, Nitrosolobus, Nitrosovibrio, Nitrospina, Nitrospira, and Nitrococcus (Hagopian and Riley, 1998). In this study, only the Nitrobacter and Nitrospira genera were detected in the database; for the set of read abundance from these two genera was more abundant in July, followed by September and August, which corresponds with the dynamics of this metabolism (Figure 7A).
All phosphorus metabolic pathways were more abundant in July than in August and September (Ren et al., 2019). The most abundant pathway was organic OPM, followed by IPS-PUT, and PSRR (Figure 6D). Temporal changes were only detected in genes implicated in the OPM pathway (phoAD, upgQ, appA, and phn). The increased representation of these genes in July suggests an increase in the P concentration of the water. However, phosphates were not measured in this study, which is how microorganisms and plants utilize P, and no temporal variations were observed for TP (Table 1; Karl, 2000). All genes, except for phn, were more abundant in July. The highest abundance of phn, which codes for phosphonoacetate hydrolase and is involved in the pathway’s final step, was observed in August (Figures 7A,B). The phn gene was more abundant in August, which indicates that there could be a greater organic P mineralization during that month. All the genes involved in the OPM pathway had the lowest abundance in September, which might imply that there was less phosphorus available for the microbial communities in this reservoir.
Regarding sulfur metabolism, the genes associated with the ASR pathway were more abundant than the genes of other metabolic pathways (Figure 6A). Fungi, prokaryotes, and photosynthetic organisms use ASR to convert inorganic SO42− to S2−, which is then incorporated into amino acid carbon skeletons to produce Cys or homo-Cys (Brunold, 1993). This process occurs in both anaerobic and aerobic conditions and has advantages over the DSR pathway, which only occurs in anaerobic environments (Kushkevych et al., 2020). These findings suggest that the ASR pathway was the most abundant in this study due to the capacity of facultative anaerobic microbes to function both in the presence of DO (i.e., on the surface of Lake Cajititlán), as well as at low DO concentrations (near the sediments of the lake). However, only the SOX system pathway displayed significant temporal variations (Figure 6A). This system, which is present in many sulfur oxidizing bacteria and certain archaea, completely oxidizes reduced sulfur (S) species to SO42− (Quentmeier and Friedrich, 2001; Sauvé et al., 2007; Grabarczyk et al., 2015). The heatmap data shows that all the genes involved in the SOX system pathway were more abundant in July, except for the soxZ gene, which was most abundant in September (Figure 7A). Microbial redox reactions of inorganic sulfur compounds are one of the vital processes responsible for the environmental balance of sulfur, mainly sulfur anions. These reactions are mostly mediated by alpha-Proteobacteria sulfur oxidizing (sox) genes. SoxZ is a sulfur compound chelating protein that binds to soxY and forms a complex with soxB, a sulfate thiolesterase, eventually cleaving the sulfur adduct (Bagchi and Ghosh, 2005). As a result, our findings show that the involvement of the sulfur genes is critical since crucial unions are formed to produce the ultimate product, thiosulfate.
Although there were no temporal differences in the carbon cycle pathways, carbohydrate metabolism was the most abundant (Figure 6B). Surface runoff and untreated or partially treated wastewater discharged into Lake Cajititlán by three treatment plants provide an important source of energy for the reservoir’s microbial communities. Furthermore, organic matter pollution increases during the rainy season when wastewater mixes with rain and when treatment plants are over capacity, releasing a mixture of sewage and rainwater into the lake (de Anda et al., 2019; Gradilla-Hernández et al., 2019).
This research discusses the interactions between abiotic and biotic elements of a subtropical and hypereutrophic lake. Most physicochemical variables (DO, pH, Secchi disk, NH4+, NO3−, BGA-PC, TP, and chlorophyll-a) were outside of the permissible limits. Likewise, significant temporal variations were observed for most of the physicochemical parameters (DO, pH, WT, Secchi disk, ORP, NH4+, NO3−, BGA-PC, chlorophyll-a, and EC). These abiotic factors influence the structure of microbial communities, as well as the major nutrient-associated biogeochemical cycles of Lake Cajititlán. The most abundant phytoplankton species identified were Planktothrix agardhii and Microcystis aeruginosa, both of which are potentially toxic to fish due to their capacity to produce microcystins. Pseudomonas, Streptomyces, and Flavobacterium were the most abundant bacterial genera. Pseudomonas fluorescens, Stenotrophomonas maltophilia, and Aeromonas veronii were the most abundant bacterial species reported as harmful to fish, and some of their genes (fur, luxS, aer, act, aha, exu, lip, ser) are associated with fish infection. The physicochemical factors most associated with the prevalence of phytoplankton and bacterial communities were NH4+, TP, TN, ORP, DO, EC, BGA-PC, and turbidity. The genes associated with carbohydrate metabolism, CFPP, DNRA, denitrification, IPS-PUT, OPM, and ASR were found in greater abundance in the microbial communities. Denitrification, comammox, DNRA, ANRA, nitrification, the SOX system, and OPM were the metabolic pathways that displayed significant temporal variations. The genetic evidence presented in this study suggests that the genes for the metabolism of nitrogen, phosphorus, sulfur, and carbon were regulated by anthropogenic nutrient sources reaching Lake Cajititlán, since all metabolic pathways were more abundant in July. This was presumably induced by surface drags produced by rainfall and/or organic matter that enters the lake through the effluents of the WWTPs. Alterations in the biogeochemical cycles have endangered the biodiversity of Lake Cajititlán in recent years. Additional research, such as genome-wide transcriptional profiling of the bacteria directly involved in biogeochemical cycles and associated proteomic analyses, might aid in elucidating the connection between gene transcript abundance, enzyme activity, and its regulation by different nutrient sources. This, in turn, will contribute to a better understanding of the metabolic and ecological niches of microbial communities in aquatic environments.
The datasets presented in this study can be found in online repositories. The names of the repository/repositories and accession number(s) can be found at: https://www.ncbi.nlm.nih.gov/sra/PRJNA851822.
OD-T, MG-H, and CS-G conceived the study and designed the methodology. MG-H, DO-N, and CS-G supervised the manuscript’s preparation. OD-T, JA, and MG-H collected the data. OD-T analyzed the data. MG-H, CS-G, and OL-M were responsible for funding acquisition. All authors contributed to the article and approved the submitted version.
Sample sequencing was financed through the 2022 Core Lab Genomics Tec-BASE Seed Fund for Research Projects from Tecnologico de Monterrey.
OD-T gratefully acknowledges the Mexican Council for Science and Technology (CONACyT, Mexico) and the Centro de Investigacion y Asistencia en Tecnologia y Diseño del Estado de Jalisco (CIATEJ) for providing both financial (in the form of a scholarship) and academic support.
The authors declare that the research was conducted in the absence of any commercial or financial relationships that could be construed as a potential conflict of interest.
All claims expressed in this article are solely those of the authors and do not necessarily represent those of their affiliated organizations, or those of the publisher, the editors and the reviewers. Any product that may be evaluated in this article, or claim that may be made by its manufacturer, is not guaranteed or endorsed by the publisher.
The Supplementary material for this article can be found online at: https://www.frontiersin.org/articles/10.3389/fmicb.2022.1037626/full#supplementary-material
Aasfar, A., Bargaz, A., Yaakoubi, K., Hilali, A., Bennis, I., Zeroual, Y., et al. (2021). Nitrogen fixing Azotobacter species as potential soil biological enhancers for crop nutrition and yield stability. Front. Microbiol. 12:628379. doi: 10.3389/fmicb.2021.628379
Abell, J. M., Özkundakci, D., Hamilton, D. P., van Dam-Bates, P., and Mcdowell, R. W. (2019). Quantifying the extent of anthropogenic eutrophication of lakes at a National Scale in New Zealand. Environ. Sci. Technol. 53, 9439–9452. doi: 10.1021/acs.est.9b03120
Almodóvar, A., Nicola, G., and Nuevo, M. (2004). Effects of a bloom of Planktothrix rubescens on the fish community of a Spanish reservoir. Limnetica. 23. doi: 10.23818/limn.23.15
Anderson, M. J. (2001). A new method for non-parametric multivariate analysis of variance. Austral Ecol. 26, 32–46. doi: 10.1111/j.1442-9993.2001.01070.pp.x
Anderson, M. J., Ellingsen, K. E., and McArdle, B. H. (2006). Multivariate dispersion as a measure of beta diversity. Ecol. Lett. 9, 683–693. doi: 10.1111/j.1461-0248.2006.00926.x
Andreote, A. P. D., Dini-Andreote, F., Rigonato, J., Machineski, G. S., Souza, B. C. E., Barbiero, L., et al. (2018). Contrasting the genetic patterns of microbial communities in Soda Lakes with and without cyanobacterial bloom. Front. Microbiol. 9:244. doi: 10.3389/fmicb.2018.00244
Andrews, S. (2010). FastQC: A quality control tool for high throughput sequence data packege of R. Available online at: https://www.bioinformatics.babraham.ac.uk/projects/fastqc/
Annavajhala, M. K., Kapoor, V., Santo-Domingo, J., and Chandran, K. (2018). Comammox functionality identified in diverse engineered biological wastewater treatment systems. Environ. Sci. Technol. Lett. 5, 110–116. doi: 10.1021/acs.estlett.7b00577
Arat, S., Bullerjahn, G. S., and Laubenbacher, R. (2015). A network biology approach to denitrification in Pseudomonas aeruginosa. PLoS One 10(2:e0118235. doi: 10.1371/journal.pone.0118235
Bagchi, A., and Ghosh, T. C. (2005). A structural study towards the understanding of the interactions of SoxY, SoxZ, and SoxB, leading to the oxidation of sulfur anions via the novel global sulfur oxidizing (sox) operon. Biochem. Biophys. Res. Commun. 335, 609–615. doi: 10.1016/j.bbrc.2005.07.115
Basri, L., Nor, R. M., Salleh, A., Yasin I.S., M., Saad, M. Z., Abd Rahaman, N. Y., et al. (2020). Co-Infections of Tilapia Lake Virus, Aeromonas hydrophila and Streptococcus agalactiae in Farmed Red Hybrid Tilapia. Animals. 10:2141. doi: 10.3390/ani10112141
Beck, M. W. (2021). Ggord: ordination plots with ggplot2. R package versión 1:6. doi: 10.5281/zenodo.842225
Bell, K. L., Petit, R. A. 3rd, Cutler, A., Dobbs, E. K., Macpherson, J. M., Read, T. D., et al. (2021). Comparing whole-genome shotgun sequencing and DNA metabarcoding approaches for species identification and quantification of pollen species mixtures. Ecol. Evol. 11, 16082–16098. doi: 10.1002/ece3.8281
Bernhard, A. (2010). The nitrogen cycle: processes, players, and human impact. Nat. Educ. Knowl. 3:25.
BioBam Bioinformatics (2019). OmicsBox-bioinformatics made easy (version 2.0.36). Available online: https://www.biobam.com/omicsbox
Bolger, A. M., Lohse, M., and Usadel, B. (2014). Trimmomatic: a flexible trimmer for Illumina sequence data. Bioinformatics 30, 2114–2120. doi: 10.1093/bioinformatics/btu170
Bouchamma, E. O., Derraz, M., Naji, B., and Dauta, A. (2004). Influence des conditions nutritionnelles sur la croissance et les capacités de stockage intracellulaire (azote et phosphore) chez Planktothrix agardhiiisolée des eaux de la retenue eutrophe El Kansera (Maroc). Acta Bot. Gall. 151, 381–392. doi: 10.1080/12538078.2004.1051544
Bowker, M. A. (2007). Biological soil crust rehabilitation in theory and practice: an underexploited opportunity. Restor. Ecol. 15, 13–23. doi: 10.1111/j.1526-100X.2006.00185.x
Briand, E., Gugger, M., Francois, J.-C., Bernard, C., Humbert, J.-F., and Quiblier, C. (2008). Temporal variations in the dynamics of potentially microcystin-producing strains in a bloom-forming Planktothrix agardhii (cyanobacterium) population. Appl. Environ. Microbiol. 74, 3839–3848. doi: 10.1128/aem.02343-07
Brunold, C. (1993). Regulatory interactions between sulfate and nitrate assimilation in: LJ KokDe, I. Stulen, H. Rennenberg, C. Brunold, and W. E. Rauser, eds. Sulfur nutrition and sulfur assimilation in higher plants. The Hague, The Netherlands: SPB Academic Publishing, 61–75.
Bukowska, A., Kaliński, T., Koper, M., Kostrzewska-Szlakowska, I., Kwiatowski, J., Mazur-Marzec, H., et al. (2017). Predicting blooms of toxic cyanobacteria in eutrophic lakes with diverse cyanobacterial communities. Sci. Rep. 7. doi: 10.1038/s41598-017-08701-8
Camejo, P. Y., Santo Domingo, J., McMahon, K. D., and Noguera, D. R (2017). Genome-enabled insights into the ecophysiology of the comammox bacterium “Candidatus Nitrospira nitrosa”. ASM journals mSystems. 2 e00059–e00017. doi: 10.1128/mSystems.00059-17
Caro-Becerra, J. L., Vizcaíno-Rodríguez, L. A., Mayoral-Ruiz, P. A., and Lujan-Godínez, R. (2017). El Humedal de la Laguna de Cajititlán Visto desde el manejo de Cuencas Para Evaluar Riesgos y Desastres. Reaxion, Ciencia y Universidad Tecnológica de León. Available online at: http://reaxion.utleon.edu.mx/Art_Impr_Art_El_humedal_de_la_Laguna_de_Cajititlan_visto_desde_el_manejo_de_cuencas_para_evaluar_riesgos_y_desastres.html
Caspers, H. (1984). OECD: Eutrophication of Waters. Monitoring, Assessment and Control. — 154 pp. Paris: Organisation for Economic Co-Operation and Development 1982. (Publié en français sous le titre Eutrophication des Eaux. Méthodes de Surveillance, d’Evaluation et de Lutte). Int. Rev. Gesamten Hydrobiol. 69, 200–200. doi: 10.1002/iroh.19840690206
Centro de Estudios para el Desarrollo Rural Sustentable y la Soberanía Alimentaria (CEDRSSA). (2019). Fertilizantes. Available online: http://www.cedrssa.gob.mx/post_n-fertilizantes-n.html
Chaturvedi, A., Pandey, B., Yadav, A. K., and Saroj, S. (2021). Chapter 5—An overview of the potential impacts of global climate change on water resources in water conservation in the era of global climate change. Amsterdam, Netherlands: Elsevier. 99–120
Chen, P.-L., Tsai, P.-J., Chen, C.-S., Lu, Y.-C., Chen, H.-M., Lee, N.-Y., et al. (2015). Aeromonas stool isolates from individuals with or without diarrhea in southern Taiwan: predominance of Aeromonas veronii. J. Microbiol. Immunol. Infect. 48, 618–624. doi: 10.1016/j.jmii.2014.08.007
Choi, H., Kim, B., Kim, J., and Han, M. (2005). Streptomyces neyagawaensis as a control for the hazardous biomass of Microcystis aeruginosa (cyanobacteria) in eutrophic freshwaters. Biol. Control 33, 335–343. doi: 10.1016/j.biocontrol.2005.03
Choi, J., Yang, F., Stepanauskas, R., Cardenas, E., Garoutte, A., Williams, R., et al. (2016). Strategies to improve reference databases for soil microbiomes. ISME J. 11, 829–834. doi: 10.1038/ismej.2016.168
Clarke, K. R. (1993). Non-parametric multivariate analyses of changes in community structure. Austral Ecol. 18, 117–143. doi: 10.1111/j.1442-9993.1993.tb00438.x
CONAGUA Gobierno de México (2021). Información Estadística Climatológica. Resource Document. Servicio Meteorológico Nacional. Available online at: https://smn.conagua.gob.mx/es/climatologia/informacion-climatologica/informacion-estadistica-climatologica
Daigger, G. T. (2014). Oxygen and Carbon Requirements for Biological Nitrogen Removal Processes Accomplishing Nitrification, Nitritation, and Anammox. Water Environment Research. 86, 204–209. doi: 10.2175/106143013x13807328849
D’Aloia, M., Bailey, T. A., Samour, J. H., Naldo, J., and Howlett, J. C. (1996). Bacterial flora of captive houbara (Chlamydotis undulata), kori (Ardeotis kori) and rufous-crested (Eupodotis ruficrista) bustards. Avian Pathol. 25, 459–468. doi: 10.1080/03079459608419155
Dang, T. C., Fujii, M., Rose, A. L., Bligh, M., and Waite, T. D. (2011). Characteristics of the freshwater cyanobacterium Microcystis aeruginosa grown in iron-limited continuous culture. Appl. Environ. Microbiol. 78, 1574–1583. doi: 10.1128/aem.06908-11
de Anda, J., de Díaz-Torres, J. J., Gradilla-Hernández, M. S., and de la Torre-Castro, L. M. (2019). Morphometric and water quality features of Lake Cajititlán, Mexico. Environ. Monit. Assess. 191. doi: 10.1007/s10661-018-7163-8
de Anda, J., and Shear, H. (2001). Nutrients and eutrophication in Lake Chapala. Lerma-Chapala Watershed 183–198. doi: 10.1007/978-1-4615-0545-7_8
Díaz-Torres, O., de Anda, J., Lugo-Melchor, O. Y., Pacheco, A., Orozco-Nunnelly, D. A., Shear, H., et al. (2021). Rapid changes in the phytoplankton community of a subtropical, shallow, hypereutrophic lake during the rainy season. Front. Microbiol. 12:617151. doi: 10.3389/fmicb.2021.617151
Díaz-Torres, O., Lugo-Melchor, O. Y., de Anda, J., Pacheco, A., Yebra-Montes, C., Gradilla-Hernández, M. S., et al. (2022). Bacterial dynamics and their influence on the biogeochemical cycles in a subtropical hypereutrophic Lake during the rainy season. Front. Microbiol. 13:832477. doi: 10.3389/fmicb.2022.832477
Dokulil, M., Chen, W., and Cai, Q. (2000). Anthropogenic impacts to large lakes in China: the tai Hu example. Aquat. Ecosyst. Health Manag. 3, 81–94. doi: 10.1080/14634980008656993
Dungan, R. S., Yates, S. R., and Frankenberger, W. T. (2003). Transformations of selenate and selenite by Stenotrophomonas maltophilia isolated from a seleniferous agricultural drainage pond sediment. Environ. Microbiol. 5, 287–295. doi: 10.1046/j.1462-2920.2003.00410.x
Ellermeier, J. R., and Slauch, J. M. (2007). Fur regulates expression of the salmonella Pathogenicity Island 1 type III secretion system through HilD. J. Bacteriol. 190, 476–486. doi: 10.1128/jb.00926-07
Fu, W. Y., Chen, J. P., Wang, X. M., and Xu, L. H. (2005). Altered expression of P53, Bcl-2 and bax induced by microcystin-LR in vivo and in vitro. Toxicon 46, 171–177. doi: 10.1016/j.toxicon.2005.03.021
Gągała, I., Izydorczyk, K., Skowron, A., Kamecka-Plaskota, D., Stefaniak, K., Kokociński, M., et al. (2010). Appearance of toxigenic cyanobacteria in two polish lakes dominated by Microcystis aeruginosa and Planktothrix agardhii and environmental factors influence. Ecohydrol. Hydrobiol. 10, 25–34. doi: 10.2478/v10104-009-0045-5
Geng, Y., Wang, K., Chen, D., and Huang, X. (2007). Isolation, identification and phylogenetic analysis of Stenotrophomonas maltophilia in channel catfish. Chin. J. Vet. Sci. 27, 330–335.
Geng, Y., Wang, K., Chen, D., Huang, X., He, M., and Yin, Z. (2010a). Stenotrophomonas maltophilia, an emerging opportunist pathogen for cultured channel catfish, Ictalurus punctatus, China. Aquaculture. 308, 132–135. doi: 10.1016/j.aquaculture.2010.08
Geng, Y., Wang, K., Xiao, D., Chen, D., and Huang, J. (2010b). Pathological studies on channel catfish induced by extracellular products of Stenotrophomonas maltophilia. Acta Hydrobiol. Sin. 34, 345–352. doi: 10.3724/Sp.J.1035.2009.00345
Ghenghesh, K. S., Abeid, S. S., Jaber, M. M., and Ben-Taher, S. A. (1999). Isolation and haemolytic activity of Aeromonas species from domestic dogs and cats. Comp. Immunol. Microbiol. Infect. Dis. 22, 175–179. doi: 10.1016/s0147-9571(98)00134-9
Glibert, P. M., Landsberg, J. H., Evans, J. J., Al-Sarawi, M. A., Faraj, M., Al-Jarallah, M. A., et al. (2002). A fish kill of massive proportion in Kuwait Bay, Arabian gulf, 2001: the roles of bacterial disease, harmful algae, and eutrophication. Harmful Algae 1, 215–231. doi: 10.1016/s1568-9883(02)00013-6
Grabarczyk, D. B., Chappell, P. E., Johnson, S., Stelzl, L. S., Lea, S. M., and Berks, B. C. (2015). Structural basis for specificity and promiscuity in a carrier protein/enzyme system from the sulfur cycle. Proc. Natl. Acad. Sci. 112, E7166–E7175. doi: 10.1073/pnas.1506386112
Gradilla-Hernández, M. S., de Anda, J., Garcia-Gonzalez, A., Meza-Rodríguez, D., Yebra Montes, C., and Perfecto-Avalos, Y. (2019). Multivariate water quality analysis of Lake Cajititlán. Mexico. Environ. Monit. Assess. 192:5. doi: 10.1007/s10661-019-7972-4
Gradilla-Hernández, M. S., de Anda, J., Garcia-Gonzalez, A., Montes, C. Y., Barrios-Piña, H., Ruiz-Palomino, P., et al. (2020). Assessment of the water quality of a subtropical lake using the NSF-WQI and a newly proposed ecosystem specific water quality index. Environ. Monit. Assess. 192:296. doi: 10.1007/s10661-020-08265-7
Gradilla-Hernández, M. S., de Anda-Sanchez, J., Ruiz-Palomino, P., Barrios-Piña, H., Senés-Guerrero, C., Del Toro Barbosa, M., et al. (2018). “Estudio preliminar del Índice de calidad de agua en el lago de cajitilán y su potencial predictivo de la mortandad masiva de peces” in Memorias del Congreso Nacional de Hidráulica 2018 (Mexico: Academia Mexicana de Hidráulica)
Gu, Z., Gu, L., Eils, R., Schlesner, M., and Brors, B. (2014). circlize implements and enhances circular visualization in R. Bioinformatics 30, 2811–2812. doi: 10.1093/bioinformatics/btu393
Graham, E. B., Knelman, J. E., Schindlbacher, A., Siciliano, S., Breulmann, M., Yannarell, A., et al. (2016). Microbes as engines of ecosystem function: when does community structure enhance predictions of ecosystem processes? Front. Microbiol. 7:214. doi: 10.3389/fmicb.2016.00214
Guisan, A., Tingley, R., Baumgartner, J. B., Naujokaitis-Lewis, I., Sutcliffe, P. R., Tulloch, A. I., et al. (2013). Predicting species distributions for conservation decisions. Ecol. Lett. 16, 1424–1435. doi: 10.1111/ele.12189
Hagopian, D. S., and Riley, J. G. (1998). A closer look at the bacteriology of nitrification. Aquac. Eng. 18, 223–244. doi: 10.1016/s0144-8609(98)00032-6
Hamady, M., and Knight, R. (2009). Microbial community profiling for human microbiome projects: tools, techniques, and challenges. Genome Res. 19, 1141–1152. doi: 10.1101/gr.085464.108
Harke, M. J., Steffen, M. M., Gobler, C. J., Otten, T. G., Wilhelm, S. W., Wood, S. A., et al. (2016). A review of the global ecology, genomics, and biogeography of the toxic cyanobacterium. Microcystis spp. Harmful Alga. 54, 4–20. doi: 10.1016/j.hal.2015.12.007
Hassler, H. B., Probert, B., Moore, C., Lawson, E., Jackson, R. W., Russell, B. T., et al. (2022). Phylogenies of the 16S rRNA gene and its hypervariable regions lack concordance with core genome phylogenies. Microbiome. 10:104. doi: 10.1186/s40168-022-01295-y
Henson, W. R., Huang, L., Graham, W. D., and Ogram, A. (2017). Nitrate reduction mechanisms and rates in an unconfined eogenetic karst aquifer in two sites with different redox potential. J. Geophys. Res. Biogeosci. 122, 1062–1077. doi: 10.1002/2016jg003463
Howarth, R., Chan, F., Conley, D. J., Garnier, J., Doney, S. C., Marino, R., et al. (2011). Coupled biogeochemical cycles: eutrophication and hypoxia in temperate estuaries and coastal marine ecosystems. Front. Ecol. Environ. 9, 18–26. doi: 10.1890/100008
Huerta-Cepas, J., Szklarczyk, D., Heller, D., Hernández-Plaza, A., Forslund, S. K., Cook, H., et al. (2018). eggNOG 5.0: a hierarchical, functionally and phylogenetically annotated orthology resource based on 5090 organisms and 2502 viruses. Nucleic Acids Res. 47, D309–D314. doi: 10.1093/nar/gky1085
Humbert, J.-F., Barbe, V., Latifi, A., Gugger, M., Calteau, A., Coursin, T., et al. (2013). A tribute to disorder in the genome of the bloom-forming freshwater cyanobacterium Microcystis aeruginosa. PLoS One 8:e70747. doi: 10.1371/journal.pone.0070747
Hupfer, M., and Lewandowski, J. (2008). Oxygen controls the phosphorus release from lake sediments - a long-lasting paradigm in limnology. Int. Rev. Hydrobiol. 93, 415–432. doi: 10.1002/iroh.200711054
Huttenhower, C., Gevers, D., Knight, R., Abubucker, S., Badger, J. H., Chinwalla, A. T., et al. (2012). Structure, function and diversity of the healthy human microbiome. Nature 486, 207–214. doi: 10.1038/nature11234
Ilardi, P., Fernandez, J., and Avendano-Herrera, R. (2009). Chryseobacterium piscicola sp. nov., isolated from diseased salmonid fish. International journal of systematic and evolutionary microbiology. 59, 3001–3005. doi: 10.1099/ijs.0.007021-0
Inglezakis, V. J., Poulopoulos, S. G., Arkhangelsky, E., Zorpas, A. A., and Menegaki, A. N. (2016). Aquatic Environment. Environ. Dev. 137–212. doi: 10.1016/b978-0-444-62733-9.00003-4
Johnson, M., Sengupta, M., Purves, J., Tarrant, E., Williams, P. H., Cockayne, A., et al. (2011). Fur is required for the activation of virulence gene expression through the induction of the sae regulatory system in staphylococcus aureus. Int. J. Med. Microbiol. 301, 44–52. doi: 10.1016/j.ijmm.2010.05.003
Juhnke, M. E., and des Jardin, E., (1989). Selective medium for isolation of Xanthomonas maltophilia from soil and rhizosphere environments. Appl. Environ. Microbiol. 55, 747–750. doi: 10.1128/aem.55.3.747-750.1989
Kagalou, I., Leonardos, I., Anastasiadou, C., and Neofytou, C. (2012). The DPSIR approach for an Integrated River management framework. A preliminary application on a Mediterranean site (Kalamas River-NW Greece). Water Resour. Manag. 26, 1677–1692. doi: 10.1007/s11269-012-9980-9
Kalogianni, E., Vourka, A., Karaouzas, I., Vardakas, L., Laschou, S., and Skoulikidis, N. T. (2017). Combined effects of water stress and pollution on macroinvertebrate and fish assemblages in a Mediterranean intermittent river. Sci. Total Environ. 603-604, 639–650. doi: 10.1016/j.scitotenv.2017.06.0
Kanehisa, M., Sato, Y., Kawashima, M., Furumichi, M., and Tanabe, M. (2015). KEGG as a reference resource for gene and protein annotation. Nucleic Acids Res. 44, D457–D462. doi: 10.1093/nar/gkv1070
Kangur, K., Kangur, A., Kangur, P., and Laugaste, R. (2005). Fish kill in Lake Peipsi in summer 2002 as a synergistic effect of a cyanobacterial bloom, high temperature, and low water level. Proc. Est. Acad. Sci. Biol. Ecol. 54, 67–80.
Katsuki, K., Seto, K., Tsujimoto, A., Takata, H., and Sonoda, T. (2019). Relationship between regional climate change and primary ecosystem characteristics in a lagoon undergoing anthropogenic eutrophication, Lake Mokoto, Japan. Estuar. Coast. Shelf Sci. 222, 205–213. doi: 10.1016/j.ecss.2019.04.016
Kirilova, E. P., Heiri, O., Bluszcz, P., Zolitschka, P., and Lotter, A. F. (2011). Climate-driven shifts in diatom assemblages recorded in annually laminated sediments of Sacrower (see NE Germany). Aquat. Sci. 73, 201–210. doi: 10.1007/s00027-010-0169-0
Kolde, R. (2019). Pheatmap: Pretty Heatmaps. R package version 1.0.12. cran package. Available online at: https://cran.r-project.org/web/packages/pheatmap/index.html
Kruk, C., Mazzeo, N., Lagerot, G., and Reynolds, C. (2002). Classification schemes for phytoplankton: a local validation of a functional approach to the analysis of species temporal replacement. J. Plankton Res. 24, 901–912. doi: 10.1093/plankt/24.9.901
Kushkevych, I., Cejnar, J., Treml, J., Dordević, D., Kollar, P., and Vítězová, M. (2020). Recent advances in metabolic pathways of sulfate reduction in intestinal bacteria. Cells 9:698. doi: 10.3390/cells9030698
Laudadio, I., Fulci, V., Stronati, L., and Carissimi, C. (2019). Next-generation metagenomics: Methodological challenges and opportunities. OMICS 23, 327–333. doi:doi: 10.1089/omi.2019.0073
Lawson, C. E., and Lücker, S. (2018). Complete ammonia oxidation: an important control on nitrification in engineered ecosystems? Curr. Opin. Biotechnol. 50, 158–165. doi: 10.1016/j.copbio.2018.01.015
Ley Federal de Derechos (LFD) (2019). Disposiciones Aplicables en Materia de Aguas Nacionales. Comisión Nacional del Agua (CONAGUA). Available online at: https://files.conagua.gob.mx/conagua/publicaciones/Publicaciones/CGRF-1-19%20LFD.pdf.
Li, T., Raza, S. H. A., Yang, B., Sun, Y., Wang, G., Sun, W., et al. (2020). Aeromonas veronii infection in commercial freshwater fish: a potential threat to public health. Animals 10:608. doi: 10.3390/ani10040608
Limón-Macías, J. G., Amezcua-Cerda, J. J., and Bastidas, R. V. (1983). “Rehabilitation plan for Lake Cajititlán: an endangered shallow lake. In lake restoration, protection, and management,” in Proceedings of the 2nd Annual Conference. North American Lake management society. (Washington, DC: U.S. Environmental Protection Agency), 69–72.
Loch, T. P., and Faisal, M. (2010). Isolation of Aeromonas salmonicida subspecies salmonicida from Lake Whitefish (Coregonus clupeaformis) inhabiting Lakes Michigan and Huron. J. Great Lakes Res. 36, 13–17. doi: 10.1016/j.jglr.2009.07.002
Liou, J. S.-C., and Madsen, E. L. (2008). Microbial Ecological Processes: Aerobic/Anaerobic. Encyclopedia of Ecology. 2348–2357. doi: 10.1016/b978-008045405-4.00254-8
Liu, L., Chi, H., and Sun, L. (2015). Pseudomonas fluorescens: identification of fur-regulated proteins and evaluation of their contribution to pathogenesis. Dis. Aquat. Org. 115, 67–80. doi: 10.3354/dao02874
Liu, L., Yang, J., Lv, H., and Yu, Z. (2014). Synchronous dynamics and correlations between bacteria and phytoplankton in a subtropical drinking water reservoir. FEMS Microbiol. Ecol. 90, 126–138. doi: 10.1111/1574-6941.12378
Liu, W., Yao, L., Jiang, X., Guo, L., Cheng, X., and Liu, G. (2018). Sediment denitrification in Yangtze lakes is mainly influenced by environmental conditions but not biological communities. Sci. Total Environ. 616-617, 978–987. doi: 10.1016/j.scitotenv.2017.10.221
Mary River Catchment Coordinating Committee (MRCCC) (2013). Water quality standards . Integrated catchment management. Available online at: https://mrccc.org.au/wp-content/uploads/2013/10/Water-Quality-Salinity-Standards.pdf
McMurdie, P. J., and Holmes, S. (2013). Phyloseq: an R package for reproducible interactive analysis and graphics of microbiome census data. PLoS One 8:e61217. doi: 10.1371/journal.pone.0061217
Mencacci, A. (2003). Aeromonas veronii biovar veronii septicaemia and acute suppurative cholangitis in a patient with hepatitis B. J. Med. Microbiol. 52, 727–730. doi: 10.1099/jmm.0.05214-0
Menanteau-Ledouble, S., Kumar, G., Saleh, M., and El-Matbouli, M. (2016). Aeromonas salmonicida: updates on an old acquaintance. Dis. Aquat. Org. 120, 49–68. doi: 10.3354/dao03006
Michaud, L., Caruso, C., Mangano, S., Interdonato, F., Bruni, V., and Lo Giudice, A. (2012). Predominance of Flavobacterium, pseudomonas, and Polaromonas with in the prokaryotic community of freshwater shallow lakes in the northern Victoria land East Antarctica. FEMS Microbiol. Ecol. 82, 391–404. doi: 10.1111/j.1574-6941.2012.01394.x
Nakatsu, C. H., Byappanahalli, M. N., and Nevers, M. B. (2019). Bacterial community 16S rRNA gene sequencing characterizes riverine microbial impact on Lake Michigan. Front. Microbiol. 10:996. doi: 10.3389/fmicb.2019.00996
Newton, R. J., Jones, S. E., Eiler, A., McMahon, K. D., and Bertilsson, S. (2011). A guide to the natural history of freshwater lake bacteria. Microbiol. Mol. Biol. Rev. 75, 14–49. doi: 10.1128/mmbr.00028-10
Ngwa, F. F., Madramootoo, C. A., and Jabaji, S. (2014). Comparison of cyanobacterial microcystin synthetase (mcy)Egene transcript levels,mcy Egene copies, and biomass as indicators of microcystin risk under laboratory and field conditions. Microbiol. Open. 3, 411–425. doi: 10.1002/mbo3.173
NORMA Oficial Mexicana (NOM-001-SEMARNAT-2021) (2021). Que establece los límites permisibles de contaminantes en las descargas de aguas residuales en cuerpos receptores propiedad de la nación. Diario Oficial de la Federación. Mexico city, Mexico. Available online at: https://www.dof.gob.mx/nota_detalle.php?codigo=5645374&fecha=11/03/2022(accessed Mach 16, 2022).
Nurk, S., Meleshko, D., Korobeynikov, A., and Pevzner, P. A. (2017). metaSPAdes: a new versatile metagenomic assembler. Genome Res. 27, 824–834. doi: 10.1101/gr.213959.116
Oksanen, J., Simpson, G. L., Blanchet, F. G., Kindt, R., Legendre, P., and Weedon, J. (2022). Vegan: community ecology package. R package version 2, 6–2. https://CRAN.R-project.org/package=vegan
Organisation for Economic Co-operatin and Development (OECD)Díaz, R. J. (2010). “OECD: Agriculture’s impact on aquaculture: Hypoxia and eutrophication in marine waters” in Advancing the Aquaculture Agenda: Workshop Proceedings (Paris: OECD Publishing) doi: 10.1787/9789264088726-20-en
Oyewusi, H. A., Abdul Wahab, R., Edbeib, M. F., Mohamad, M. A. N., Abdul Hamid, A. A., Kaya, Y., et al. (2020). Functional profiling of bacterial communities in Lake Tuz using 16S rRNA gene sequences. Biotechnol. Biotechnol. Equip. 35, 1–10. doi: 10.1080/13102818.2020.1840437
Palomo, A., Pedersen, A. G., Fowler, S. J., Dechesne, A., Sicheritz-Pontén, T., and Smets, B. F. (2018). Comparative genomics sheds light on niche differentiation and the evolutionary history of comammox Nitrospira. ISME J. 12, 1779–1793. doi: 10.1038/s41396-018-0083-3
Parulekar, N. N., Kolekar, P., Jenkins, A., Kleiven, S., Utkilen, H., Johansen, A., et al. (2017). Characterization of bacterial community associated with phytoplankton bloom in a eutrophic lake in South Norway using 16S rRNA gene amplicon sequence analysis. PLoS One 12:e0173408. doi: 10.1371/journal.pone.0173408
Pękala-Safińska, A. (2018). Contemporary threats of bacterial infections in freshwater fish. J. Vet. Res. 62, 261–267. doi: 10.2478/jvetres-2018-0037
Quentmeier, A., and Friedrich, C. G. (2001). The cysteine residue of the SoxY protein as the active site of protein-bound sulfur oxidation ofParacoccus pantotrophusGB17. FEBS Lett. 503, 168–172. doi: 10.1016/s0014-5793(01)02727-2
Quince, C., Walker, A. W., Simpson, J. T., Loman, N. J., and Segata, N. (2017). Shotgun metagenomics, from sampling to analysis. Nat. Biotechnol. 35, 833–844. doi: 10.1038/nbt.3935
R Core Team (2021). R: A language and environment for statistical computing. R Foundation for Statistical Computing, Vienna, Austria. Available online at: https://www.R-project.org/
Ram, A., Jaiswar, J. R. M., Rokade, M. A., Bharti, S., Vishwasrao, C., and Majithiya, D. (2014). Nutrients, hypoxia and mass Fishkill events in Tapi estuary India. Estuar. Coast. Shelf Sci. 148, 48–58. doi: 10.1016/j.ecss.2014.06.013
Ramanan, R., Kim, B.-H., Cho, D.-H., Oh, H.-M., and Kim, H.-S. (2016). Algae–bacteria interactions: evolution, ecology and emerging applications. Biotechnol. Adv. 34, 14–29. doi: 10.1016/j.biotechadv.2015.12
Reitzer, L. J. (1996). “Ammonia assimilation and the biosynthesis of glutamine, glutamate, aspartate, asparagine, l-alanine, and d-alanine” in Escherichia coli and salmonella: Cellular and molecular biology. eds. F. C. Neidhardt, R. Curtiss III, J. L. Ingraham, E. C. C. Lin, K. B. Low, and B. Magasanik, et al. 2nd ed (Washington, DC: ASM Press), 391–407.
Ren, Z., Qu, X., Peng, W., Yu, Y., and Zhang, M. (2019). Functional properties of bacterial communities in water and sediment of the eutrophic river-Lake system of Poyang Lake China. PeerJ. 12:e7318. doi: 10.7717/peerj.7318.31338262
Rho, M., Tang, H., and Ye, Y. (2010). FragGeneScan: predicting genes in short and error-prone reads. Nucleic Acids Res. 38, e191–e191. doi: 10.1093/nar/gkq747
Roberts, M. T. M., Enoch, D. A., Harris, K. A., and Karas, J. A. (2006). Aeromonas veronii biovar sobria bacteraemia with septic arthritis confirmed by 16S rDNA PCR in an immunocompetent adult. J. Med. Microbiol. 55, 241–243. doi: 10.1099/jmm.0.46295-0
Salas, H. J. (2003). “A Simplified Phosphorus Trophic State Model for Warm-Water Tropical Lakes” in 2003 AWWA Source Water Protection Symposium. January 19-22 (Albuquerque, NM, USA)
Sauvé, V., Bruno, S., Berks, B. C., and Hemmings, A. M. (2007). The SoxYZ complex carries sulfur cycle intermediates on a peptide swinging arm. J. Biol. Chem. 282, 23194–23204. doi: 10.1074/jbc.m701602200
Segata, N., Waldron, L., Ballarini, A., Narasimhan, V., Jousson, O., and Huttenhower, C. (2012). Metagenomic microbial community profiling using unique clade-specific marker genes. Nat. Methods 9, 811–814. doi: 10.1038/nmeth.2066
Seitzinger, S., Harrison, J. A., Böhlke, J. K., Bouwman, A. F., Lowrance, R., Peterson, B., et al. (2006). Denitrification across landscapes and waterscapes: a synthesis. Ecol. Appl. 16, 2064–2090. doi: 10.1890/1051-0761(2006)016[2064:dalawa]2.0.co
Shabana, B. M., Elkenany, R. M., and Younis, G. (2022). Sequencing and multiple antimicrobial resistance of Pseudomonas fluorescens isolated from Nile tilapia fish in Egypt. Braz. J. Biol. 84:e257144. doi: 10.1590/1519-6984.257144
Shao, K., Yao, X., Wu, Z., and Gao, G. (2021). The bacterial community composition and its environmental drivers in the rivers around eutrophic Chaohu Lake, China. BMC Microbiol. 21:179. doi: 10.1186/s12866-021-02252-9
Sharpton, T. J. (2014). An introduction to the analysis of shotgun metagenomic data. Front. Plant Sci. 5. doi: 10.3389/fpls.2014.00209
Skoulikidis, N. T. (2009). The environmental state of rivers in the Balkans—a review within the DPSIR framework. Sci. Total Environ. 407, 2501–2516. doi: 10.1016/j.scitotenv.2009.01.0
Skoulikidis, N. T., Amaxidis, Y., Bertahas, I., Laschou, S., and Gritzalis, K. (2006). Analysis of factors driving stream water composition and synthesis of management tools—a case study on small/medium Greek catchments. Sci. Total Environ. 362, 205–241. doi: 10.1016/j.scitotenv.2005.05.0
Smith, V. H., and Schindler, D. W. (2009). Eutrophication science: where do we go from here? Trends Ecol. Evol. 24, 201–207. doi: 10.1016/j.tree.2008.11.009
Ssebiyonga, N., Erga, S. R., Hamre, B., Stamnes, J. J., and Frette, Ø. (2013). Light conditions and photosynthetic efficiency of phytoplankton in Murchison Bay, Lake Victoria Uganda. Limnologica. 43, 185–193. doi: 10.1016/j.limno.2012.09.005
Steven, B., Gallegos-Graves, L. V., Starkenburg, S. R., Chain, P. S., and Kuske, C. R. (2012). Targeted and shotgun metagenomic approaches provide different descriptions of dryland soil microbial communities in a manipulated field study. Environ. Microbiol. Rep. 4, 248–256. doi: 10.1111/j.1758-2229.2012.00328.x
Su, J.-J., Liu, B.-Y., and Liu, C.-Y. (2001). Comparison of aerobic denitrification under high oxygen atmosphere by Thiosphaera pantotropha ATCC 35512 and Pseudomonas stutzeri SU2 newly isolated from the activated sludge of a piggery wastewater treatment system. J. Appl. Microbiol. 90, 457–462. doi: 10.1046/j.1365-2672.2001.01265.x
Suda, S., Yongmanitchai, W., Nopartnaraporn, N., Watanabe, M. M., Liu, Y., Day, J. G., et al. (2002). Taxonomic revision of water-bloom-forming species of oscillatorioid cyanobacteria. Int. J. Syst. Evol. Microbiol. 52, 1577–1595. doi: 10.1099/00207713-52-5-1577
Sunagawa, S., Mende, D. R., Zeller, G., Izquierdo-Carrasco, F., Berger, S. A., Kultima, J. R., et al. (2013). Metagenomic species profiling using universal phylogenetic marker genes. Nat. Methods 10, 1196–1199. doi: 10.1038/nmeth.2693
Swain, P., Behura, A., Dash, S., and Nayak, S. K. (2007). Serum antibody response of Indian major carp, Labeo rohita to three species of pathogenic bacteria; Aeromonas hydrophila, Edwardsiella tarda and Pseudomonas fluorescens. Vet. Immunol. Immunopathol. 117, 137–141. doi: 10.1016/j.vetimm.2007.02.010
Tang, M., Jiang, J., Lv, Q., Yang, B., Zheng, M., Gao, X., et al. (2020). Denitrification performance of Pseudomonas fluorescens Z03 immobilized by graphene oxide-modified polyvinyl-alcohol and sodium alginate gel beads at low temperature. Royal Soc. Open Sci. 7:191542. doi: 10.1098/rsos.191
Teje, J., Sørensen, J., and Chang, Y. (1981). Assimilatory and dissimilatory nitrate reduction: perspectives and methodology for measurement of several nitrogen cycle processes. Ecol. Bull. 33, 331–342.
Townsend, S. A., Boland, K., and Wrigley, T. J. (1992). Factors contributing to a fish kill in the Australian wet/dry tropics. Water Res. 26, 1039–1044.
Troxell, B., Sikes, M. L., Fink, R. C., Vazquez-Torres, A., Jones-Carson, J., and Hassan, H. M. (2010). Fur negatively regulates hns and is required for the expression of HilA and virulence in salmonella enterica Serovar typhimurium. J. Bacteriol. 193, 497–505. doi: 10.1128/jb.00942-10
United States Environmental Protection Agency (EPA) (2002). Nitrification. Environmental Protectio Agency. Available online at: https://www.epa.gov/sites/default/files/2015-09/documents/nitrification_1.pdf
Vollenweider, R. A., and Kerekes, J. J. (1981). “Background and summary results of the OECD cooperative program on eutrophication” in Restoration of lakes and inland waters. Environmental Protection Agency. EPA/440/5-81-010, 25–36.
Vollenweider, R. A., and Kerekes, J. J. (1982). Eutrophication of waters. Monitoring, assessment and control. Organization for Economic co-Operation and Development (OECD), Paris:156.
Wang, H. H., Chu, H. L., Dou, Q., Xie, Q. Z., Tang, M., Sung, C. K., et al. (2018). Phosphorus and nitrogen drive the seasonal dynamics of bacterial communities in pinus forest rhizospheric soil of the qinling mountains. Front. Microbiol. 9:1930. doi: 10.3389/fmicb.2018.01930
Wang, H. R., Hu, Y. H., Zhang, W. W., and Sun, L. (2009). Construction of an attenuated Pseudomonas fluorescens strain and evaluation of its potential as a cross-protective vaccine. Vaccine 27, 4047–4055. doi: 10.1016/j.vaccine.2009.04.023
Ward, B. B., Devol, A. H., Rich, J. J., Chang, B. X., Bulow, S. E., Naik, H., et al. (2009). Denitrification as the dominant nitrogen loss process in the Arabian Sea. Nature 461, 78–81. doi: 10.1038/nature08276
Wickham, H. (2016). gplot2: Elegant graphics for data analysis. Springer-Verlag New York, Second Edition. ISBN: 978-3-319-24275-0
Wickham, H., and Seidel, D. (2022). Scale functions for visualization. R package version 1.2.0. Available online at: https://cran.r-project.org/web/packages/scales/scales.pdf
Wood, D. E., Lu, J., and Langmead, B. (2019). Improved metagenomic analysis with kraken 2. Genome Biol. 20:257. doi: 10.1186/s13059-019-1891-0
Woodhouse, J. N., Kinsela, A. S., Collins, R. N., Bowling, L. C., Honeyman, G. L., Holliday, J. K., et al. (2015). Microbial communities reflect temporal changes in cyanobacterial composition in a shallow ephemeral freshwater lake. ISME J. 10, 1337–1351. doi: 10.1038/ismej.2015.218
World Health Organization (WHO). (2003). Cyanobacterial toxins: Microcystin-LR in drinking-water. Background document for preparation of WHO guidelines for drinking water quality. Geneva: World Health Organization (WHO/SDE/WSH/03.04/57).
Wu, D., Hugenholtz, P., Mavromatis, K., Pukall, R., Dalin, E., Ivanova, N. N., et al. (2009). A phylogeny-driven genomic encyclopaedia of bacteria and archaea. Nature 462, 1056–1060. doi: 10.1038/nature08656
Yao, L., Chen, C., Liu, G., and Liu, W. (2018). Sediment nitrogen cycling rates and microbial abundance along a submerged vegetation gradient in a eutrophic lake. Sci. Total Environ. 616, 899–907. doi: 10.1016/j.scitotenv.2017.10.2
YSI (2009). 6-series multiparameter water quality Sondes, user manual. Available online at: https://www.ysi.com/File%20Library/Documents/Manuals/069300-YSI-6-Series-Manual-RevJ.pdf
Yu, C., Li, Z., Xu, Z., and Yang, Z. (2020). Lake recovery from eutrophication: quantitative response of trophic states to anthropogenic influences. Ecol. Eng. 143:105697. doi: 10.1016/j.ecoleng.2019.105697
Zhang, J., Ding, X., Guan, R., Zhu, C., Xu, C., Zhu, B., et al. (2018). Evaluation of different 16S rRNA gene V regions for exploring bacterial diversity in a eutrophic freshwater lake. Sci. Total Environ. 618, 1254–1267. doi: 10.1016/j.scitotenv.2017.09.2
Zhang, D., Kang, Y., Song, M., Shu, H., Guo, S., Jia, J., et al. (2019). Identity and virulence properties of Aeromonas isolates from healthy northern snakehead (Channa argus) in China. Lett. Appl. Microbiol. 69, 100–109. doi: 10.1111/lam.13172
Keywords: subtropical lake, hypereutrophic lake, physicochemical parameters, shotgun metagenomic sequencing, pathogenic bacteria, biogeochemical cycling
Citation: Díaz-Torres O, Lugo-Melchor OY, de Anda J, Orozco-Nunnelly DA, Gradilla-Hernández MS and Senés-Guerrero C (2022) Characterizing a subtropical hypereutrophic lake: From physicochemical variables to shotgun metagenomic data. Front. Microbiol. 13:1037626. doi: 10.3389/fmicb.2022.1037626
Received: 06 September 2022; Accepted: 31 October 2022;
Published: 02 December 2022.
Edited by:
Danny Ionescu, Leibniz-Institute of Freshwater Ecology and Inland Fisheries (IGB), GermanyReviewed by:
J. Michael Beman, University of California, United StatesCopyright © 2022 Díaz-Torres, Lugo-Melchor, de Anda, Orozco-Nunnelly, Gradilla-Hernández and Senés-Guerrero. This is an open-access article distributed under the terms of the Creative Commons Attribution License (CC BY). The use, distribution or reproduction in other forums is permitted, provided the original author(s) and the copyright owner(s) are credited and that the original publication in this journal is cited, in accordance with accepted academic practice. No use, distribution or reproduction is permitted which does not comply with these terms.
*Correspondence: Misael Sebastián Gradilla-Hernández, bXNncmFkaWxsYUB0ZWMubXg=; Carolina Senés-Guerrero, Y2Fyb2xpbmEuc2VuZXNAdGVjLm14
Disclaimer: All claims expressed in this article are solely those of the authors and do not necessarily represent those of their affiliated organizations, or those of the publisher, the editors and the reviewers. Any product that may be evaluated in this article or claim that may be made by its manufacturer is not guaranteed or endorsed by the publisher.
Research integrity at Frontiers
Learn more about the work of our research integrity team to safeguard the quality of each article we publish.