- 1Jiangsu Key Laboratory of Atmospheric Environment Monitoring and Pollution Control, Collaborative Innovation Center of Atmospheric Environment and Equipment Technology, School of Environmental Science and Engineering, Nanjing University of Information Science and Technology, Nanjing, China
- 2Shenzhen Key Laboratory of Water Resource Utilization and Environmental Pollution Control, School of Civil and Environmental Engineering, Harbin Institute of Technology, Shenzhen, China
- 3State Key Laboratory of Urban Water Resource and Environment, School of Environment, Harbin Institute of Technology, Harbin, China
- 4China Municipal Engineering Central South Design and Research Institute Co., Ltd., Wuhan, China
- 5CAS Key Laboratory of Marine Ecology and Environmental Sciences, Institute of Oceanology, Chinese Academy of Sciences, Qingdao, China
This study aimed to explore the performance of denitrification deep-bed filter (DN-DBF) to treat municipal sewage for meeting a more stringent discharge standard of total nitrogen (TN) (10.0 mg L–1). A lab-scale DN-DBF was conducted to optimize operation parameters and reveal the microbiological mechanism for TN removal. The results showed that more than 12.7% TN removal was obtained by adding methanol compared with sodium acetate. The effluent TN concentration reached 6.0–7.0 mg L–1 with the optimal influent carbon and nitrogen ratio (C/N) and hydraulic retention time (HRT) (3:1 and 0.25 h). For the nitrogen removal mechanism, Blastocatellaceae_Subgroup_4 and norank_o_JG30-KF-CM45 were dominant denitrification floras with an abundance of 6–10%. Though large TN was removed at the top layer of DN-DBF, microbial richness and diversity at the middle layer were higher than both ends. However, the relative abundance of nitrite reductase enzymes (EC1.7.2.1) gradually increases as the depth increases; conversely, the relative abundance of nitrous oxide reductase gradually decreased.
Introduction
Eutrophication, caused by excessive discharged nutrients (nitrogen and phosphorus) from wastewater treatment plants, has become one of the most urgent problems and gained significant attention in recent years (Piao and Kim, 2016). In some regions with sensitive aquatic ecology in China, the total nitrogen (TN) concentration is limited to less than 10.0 mg L–1, which is superior to Chinese integrated wastewater discharge standard first-A (TN ≤ 15 mg L–1). However, the traditional secondary biological treatment was difficult to meet due to the strict TN discharge standard (Li et al., 2014). Hence, more and more advanced treatment technologies are needed to control TN discharge and protect the limited water sources.
Tertiary denitrification was required to further remove the nitrate nitrogen (NO3–-N) so as to achieve a high TN discharging standard since NO3–-N is the major component of TN in a secondary effluent. Denitrification deep-bed filter (DN-DBF) could transform NO3–-N to N2 and be considered to be an effective means to improve TN removal efficiency (Husband et al., 2014; Löwenberg et al., 2016; Shi et al., 2016). However, previous studies on DN-DBF just to meet one-class A discharge standard, for the parameters [such as carbon source type, chemical oxygen demand (COD) and TN (C/N) ratio, and hydraulic retention time (HRT)] of further advanced treatment were not yet clear (Cao et al., 2016; Xu et al., 2016).
Carbon source type and dosage play important roles that need to be optimized during the tertiary denitrification process for meeting a higher discharge standard. Many materials could be utilized as carbon sources, such as waste paper (Bao et al., 2016; Haske-Cornelius et al., 2021), biodegradable polymer (Wang et al., 2021), and wheat straw (Khokhar et al., 2014; Liu et al., 2021). Readily biodegradable organic matters are the optimal electron donors compared with refractory organic and other electron donors (Cao et al., 2016; Luo et al., 2020). Meanwhile, an insufficient dose would result in a low denitrification rate, while COD concentration would not meet the discharge standard when excessive dosage carbon sources are added (Ge et al., 2012). On the other hand, a conflict exists between TN removal efficiency and construction cost, which results from too long or too short HRT. Hence, appropriate C/N ratios and HRT should be optimized to achieve the optimum denitrification effect.
Meanwhile, microbial action in DN-DBF restricts the performance of TN removal, which indicates that it is necessary to analyze the characteristics of the microbial community in DN-DBF to reveal the mechanism of TN removal. Microbial communities and functional microorganisms in wastewater treatment are closely related to environmental and operational conditions (Adrados et al., 2014; Feng and Tong, 2015). Previous studies reported that the dominant denitrifying bacterial genera were Dechloromonas, Acidovorax, Bosea, Polaromonas, and Chryseobacterium, and particle sizes and packing type affected the community composition diversity (Tian and Wang, 2021; Yang et al., 2021). However, current studies only focused on the changes of microbial communities, but few on metabolic pathways.
Therefore, this study optimized operating parameters (carbon source type, C/N, and HRT) and investigated the performance of DN-DBF to ensure effluent compliance with the TN discharge standard (10.0 mg L–1). Besides, high-throughput sequencing technology was applied to reveal the degradation mechanism of pollutants.
Materials and Methods
Experimental Systems
The tertiary DN-DBF (Figure 1) was made from a plexiglass column with a diameter of 100 mm and a height of 1.8 m, and packed with 2–3 mm sizes of quartz sand. The packed height was 1.1 m, and a 0.3 m support gravel stone layer was set under that. The porosity and bulk density were 0.42 and 1.18 kg m3, respectively. The effluent of micro-coagulated was pumped to the top of DN-DBF and the effluent was discharged at the bottom. Besides, the sampling points and piezometers were installed on both sides of the DN-DBF and the intervals were 100 and 200 mm, respectively. The filtering media were backwashed every 24 h for 16 min by combined air and water for 8 min and water backwashing for 8 min. During backwashing, the air flow rate was 95.5 m h–1 and the water flow rate was 31.8 m h–1.
The secondary effluent was collected from an anaerobic-multistage anaerobic/oxic (A-MAO) process to a micro-flocculation tank, in which phosphorus was removed by polyaluminium chloride (PAC). The flocs were subsided in the settling tank, and the effluent was pumped into DN-DBF with external organic carbon. The main characteristics of the secondary effluent from the A-MAO process and the influent of DN-DBF are summarized in Table 1.
System Performance and Batch Experiments
Operating conditions of the DN-DBF are shown in Table 2. Carbon source type [methanol (CH3OH) and sodium acetate (NaAC)], C/N (7.0–8.0, 5.0–6.0, 4.0–5.0, 3.0–4.0, and 1.5–3.0), and HRT (0.5, 0.25, and 0.1 h) were investigated by long-time batch experiments at different periods. Under steady state, samples were taken at 0, 10, 30, 50, 70, 90, and 110 cm along the DN-DBF depth, and TN and COD concentrations were tested so as to investigate denitrification performance. Besides, the biomass attached on quartz sand samples and suspended biofilm were taken and measured from the DN-DBF regularly.
Chemical Analysis Methods
Samples were collected from the micro-flocculation setting tank and effluent of DN-DBF once a day. Besides, samples in different depths were collected when the system was in stable operation. COD, TN, ammonia nitrogen (NH4+-N), NO3–-N, and total phosphorus (TP) were analyzed according to standard methods (APHA, 2005). Biofilm biomass was determined using the weighted method. pH and temperature were monitored online by using WTW pH/Oxi 340i meter with dissolved oxygen (DO) and pH probes (WTW, Germany).
Microbiological Analysis Methods
In order to evaluate the diversity of microbial community structure of the DN-DBF system, three biofilm samples were collected at the 95th day from three parts (10, 50, and 100 cm). The total DNA was extracted with the Fast DNA Spin Kit for Soil (MP Biomedicals, Santa Ana, CA, United States). The purified DNA was applied in polymerase chain reaction (PCR) analysis by PCR instrument (9700, GeneAmp® ABI, Foster City, CA, United States) with the primer set of 338F (50-ACTCCTACGGGAGGCAGCA-30) and 806R (50-GGACTACCAGGGTATCTAAT-30). Besides, the PCR programs were as follows: initial denaturation at 95°C for 3 min; followed by 30 cycles of denaturation at 95°C for 30 s, annealing at 45°C for 30 s, elongation at 72°C for 45 s; and finally at 72°C for 10 min and 10°C until halted by user. The amplicons were sequenced on an Illumina MiSeq platform by Majorbio company (Shanghai, China). The original image data files were transformed into original sequencing sequence by CASAVA base recognition analysis, and the results were stored in FASTQ file format.
Paired-end reads of the original DNA fragments were merged using Trimmomatic and FLASH softwares (V1.2.71) (Magoc and Salzberg, 2011), and sequencing reads were assigned to each sample based on a unique barcode. Mothur version v.1.30.1 was used to calculate microbial richness and diversity (ACE index, Chao 1 index, Simpson index, and Shannon index) (Schloss et al., 2011). The operational taxonomic units (OTUs) were assigned with Usearch software (version 7.12), and all sequence column similarities within a stationary threshold (>97%) were combined together to be considered as one of the OTUs. Microbial abundance at the phylum and genus levels was counted depending on taxonomic data.
PICRUSt software3 was used to remove the 16S marker gene, and Non-supervised Orthologous Groups (eggNOG)4 databases and Kyoto Encyclopedia of Genes and Genomes (KEGG)5 were used to conduct the 16S rRNA functional prediction.
Results and Discussion
Optimization of Denitrification Deep-Bed Filter Operating Conditions
Carbon Source Types
CH3OH and NaAC were added to DN-DBF. The effect of carbon source types on denitrification was compared, as shown in Figure 2A. The average TN removal efficiency was 41.11 and 51.04% with an effluent concentration of 8.09 and 6.80 mg L–1, respectively, when equal COD was dosed by CH3OH and NaAC. More 10% removal efficiency and 1.29 mg L–1 TN were removed by CH3OH than NaAC with 0.598 and 0.750 kg (m3 d)–1 TN translated into N2, respectively. Therefore, CH3OH was the optimal carbon source for denitrification of DN-DBF. The denitrification rate of using CH3OH as carbon source was higher than NaAC, even compared to previous studies in other bioreactor configurations (Table 3).
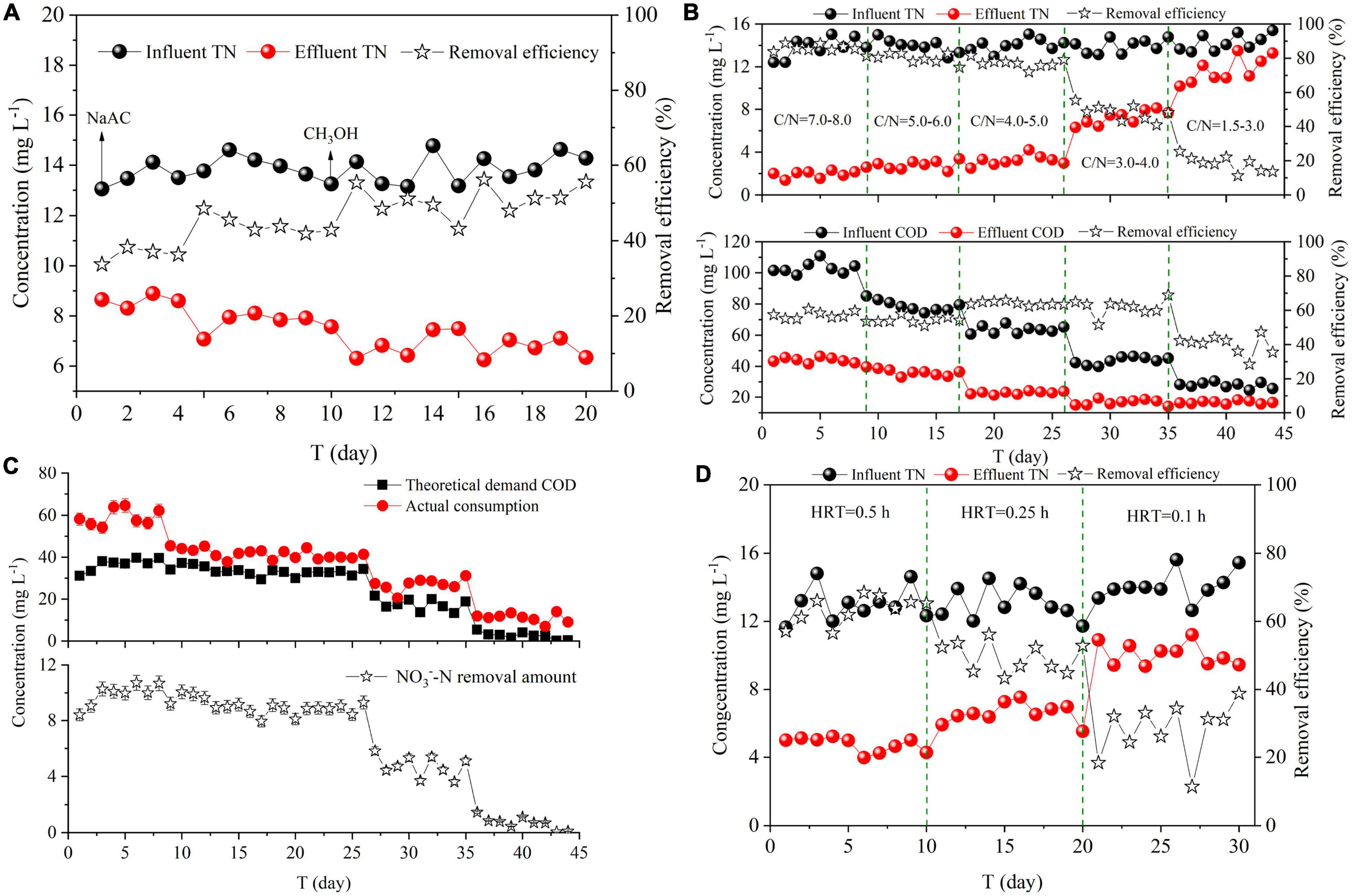
Figure 2. Effect of operating conditions on TN removal (A) Carbon source types, (B,C) C/N ratios, (D) HRT.
Due to the low cost and high efficiency of CH3OH and NaAC, they have been widely used by many sorts of denitrification process (Wei et al., 2016). CH3OH and NaAC requirement correlated with the removal of NO3–-N could be estimated by Eqs 1, 2; 2.47 g CH3OH (about 3.7 g COD) and 5.60 g CH3OONa (about 4.37 g COD) were consumed to transform 1 g NO3– to N2. Therefore, more NaAC was consumed to achieve the denitrification process. Previous studies have shown that carbon source switchover resulted to the change of microbial community structure (Liang et al., 2014). Therefore, the difference in denitrification efficiency between methanol and sodium acetate may be caused by the difference in microbial community structure.
C/N Ratio
Five C/N ratios (7.0–8.0, 5.0–6.0, 4.0–5.0, 3.0–4.0, and 1.5–3.0) were examined to study the effect of their nitrogen removing performance on DN-DBF. As shown in Figure 2B, TN removal efficiency gradually decreased and the concentration in effluent rose as the C/N radio reduced. The removal efficiency of TN maintained stable vibration that ranged from 72.05 to 88.89% and effluent concentration was 1.38–3.54 mg L–1 when C/N ratio exceeded 4. With further reduction of C/N, stable TN removal performance was destroyed and the efficiency dropped to 13.65–25.33% with high TN concentration (10.20–15.39 mg L–1) in the effluent.
In addition, it should be noted that large-dosage carbon sources resulted in less TN concentration in the effluent, and excessive COD concentration would not meet the discharge standard, while an insufficient dose would lead to a low denitrification rate. COD in effluent was 21.36–46.32 mg L–1 at the first three periods (C/N was 7.0–8.0, 5.0–6.0, and 4.0–5.0). Though the COD value was inferior to discharge standard, the utilization of carbon source was low and more COD was wasted (Figure 2B). However, TN was over 10 mg L–1 with a low-concentration COD (15.51–18.32 mg L–1) when C/N was below 3.0. Hence, the optimal and economical influent C/N was 3.0–4.0, which could meet the TN discharge standard (10.0 mg L–1).
The theoretical demand of COD and actual consumption value is demonstrated in Figure 2C. The theoretical demand of COD was correlated by Eq. 1 and its less than actual consumption was due to the fact that partial carbon source was consumed or degraded by other processes instead of denitrification. Meanwhile, part of NO3–-N was absorbed for assimilation in the biological reactor (Constantin and Fick, 1997; Zhang et al., 2021). Cheng and Lin (1993) utilized methanol as a carbon source for denitrification and established the theoretical C/N ratio of 0.71. Wang et al. (2009) found that low C/N (C/N = 1) was not sufficient for the denitrification bacteria to grow. Nevertheless, Gómez et al. (2000), Fan et al. (2001), and Wei et al. (2016) concluded that the optimal C/N ratio was 1.25, 1.1, and 2.2 for denitrification with methanol as the electron donor, which was inconsistent with this study.
Hydraulic Retention Time
Hydraulic retention time, as another important parameter for DN-DBF, restricts the denitrification performance and the occupied area of structure. Figure 2D showed the effect of different HRT (0.5, 0.25, and 0.1 h) on TN removal. TN removal efficiency decreased and effluent concentration increased with the tightening of HRT, and the average TN removal efficiency was 63.37 and 49.47% with the effluent concentration of 4.75 and 6.59 mg L–1 when HRT was 0.5 and 0.25 h, respectively, while the steep increase of TN concentration in the effluent (average concentration was 10.08 mg L–1) reflected the fact that 0.1 h could not meet the discharge standard and the optimal and economical HRT was 0.25 h.
Denitrification is a rapid process of nitrogen conversion compared with the ammonia oxidation process. Farabegoli et al. (2003) drew a conclusion that 55% TN removal efficiency was obtained when influent TN concentration was 15 mg L–1 and HRT was 7 min. The above conclusion was similar to this study. Nevertheless, Shen and Wang (2011) used cross-linked starch/polycaprolactone blends as solid carbon source and concluded that the 26.86 mg NO3–-N (L h)–1 and 90% NO3–-N were removed at HRT 1 h, which was obviously more than those in this study. The phenomenon was inferred that carbon source style was the main factor that restricted HRT.
Nutrient Removal and Head Loss Along the Filter Depth
Nutrient removal along the filter depth reflected the pollutants’ removal features of DN-DBF (Figure 3), the concentration of TN and COD in the influent were 14.14 and 42.37 mg L–1, and 55.37 and 64.81% removal efficiency were achieved with effluent concentration of 6.31 and 14.91 mg L–1. It is interesting that the denitrification rates of both pollutants were higher at the top of the filter than the bottom part; 48.85% TN and 47.93% COD were removed at the top 40 cm, while 5.52% TN and 16.87% COD were removed at the bottom 70 cm. The study of Dong et al. (2012) found that the specific denitrification rate along the bio-filter depth was 3.80, 1.21, 0.66, and 0.09 kg NO3–-N (m3 d)–1, respectively, and the highest specific denitrification rate appeared at 0–20 cm. It might be because more biomass accumulated on top of head loss; this is another standard to evaluate the performance of DN-DBF, and its change along the filter depth is shown in Table 4. As the increase of filter depth and operation time is extending, the head loss presented a significant increasing trend. The head loss changed by low increasing rates before the 12th hour, but the stable station was broken and the head loss jumped from 0.083–0.147 to 0.152–0.394 mH2O after the 12th hour. The specific incremental rate of head loss before the 12th hour along the filter depth was 0.007, 0.009, 0.010, 0.011, and 0.012 mH2O, respectively. However, the specific incremental rate after the 12th hour was 0.009–0.031 mH2O. Thus, the optimal backwash time was 12 h. Simate (2015) found that the head loss of the granular filter bed ran up suddenly at the 18th hour, which is similar to this study.
Biofilm Biomass Along the Filter Depth
Biomass yield is an important indicator to be taken into account for explaining the denitrification performance of DN-DBF. Biomass yields in different filter depths are summarized in Figure 4; the total biomass and attached biomass increased at the first 30 cm of DN-DBF, and reduced as filter depth continued to increase. However, the suspended biomass was reduced along with the filter depth. The maximum quality of attached biomass, suspended biomass, and total biomass was 21.03, 20.01, and 33.10 mg SS (g quartz sand)–1, respectively, and appeared at 30, 10, and 30 cm. On the other hand, a similar rule was discovered for volatile suspended solids (VSS). The ratio of suspended solids (SS) and VSS was about 1.5–2.0, which indicated that approximately 40–50% inorganic particles exited and contributed to the head loss of DN-DBF.
At the top layer of this filter, the suspended biomass was more than the attached biomass, but it was opposite the other sampling point. The reason for this phenomenon may be that the SS was intercepted on the first 10 cm and hydraulic scour affected the formation of biofilms (Li et al., 2011; Wu et al., 2016). This result is consistent with Dong et al. (2012). There was a high correlation between biofilm biomass and effluent quality. Large TN and COD were removed at the top of the filter due to the accumulation of biofilm biomass. Meanwhile, the higher the biomass yield rates resulted from more COD and NO3–-N that were needed for complete denitrification and cell growth (Christensson et al., 1994).
Microbial Mechanism of Nitrogen Removal
Microbial Richness and Diversity
The microbial biochemistry processes play an important role in the treatment of wastewater by DN-DBF. Therefore, analysis of the microbial process could help to characterize the mechanism and approach. In this section, 16S rRNA gene high-throughput sequencing technology was utilized to analyze the microbial community of the samples and assess the diversity and richness of the bacterial community, which was utilized to explain the denitrification mechanism of DN-DBF.
From 10 to 100 cm, the ACE and Chao index showed a tendency to rise and then fall, which indicated that the system owned the maximum microbial abundance when the filter depth was 50 cm (Table 5). Meanwhile, the variation of Shannon and Simpson diversity index from 10 to 100 cm illustrated that the diversity of microbial community also reached peak value at 50 cm. Community diversity was directly determined by species richness and species evenness. This phenomenon reflected that the backwashing process led to impacting on the stability of microorganisms and contributed to the lower diversity of microbial community than the middle part. Sun et al. (2016) and Huang et al. (2020) reported the maximal diversity index appeared at the middle part in the denitrification filler by comparing the diversity index (Shannon) along a denitrification filler, which was consistent with this study.
Microbial Community Composition
During the process of operation, the bacteria with higher relative abundance of microbial community mainly affiliated to four phyla, i.e., Proteobacteria, Chloroflexi, Actinobacteria, and Saccharibacteria. All of them were substantiated to be the dominant denitrifying bacteria (Figure 5A). The results explained that the relative abundance of dominant denitrifying bacteria directly affected the denitrification efficiency. Previous researches have indicated that multiple types of Proteobacteria microorganisms were in several wastewater treatment bioreactors, which was in accord with the study of Ma et al. (2015). When the depth of filter was between 10 and 50 cm, Blastobacter was dominant and abundant, which was affiliated to α-Proteobacteria; it was a crucial participant in nitrogen removal and COD degradation in wastewater treatment.
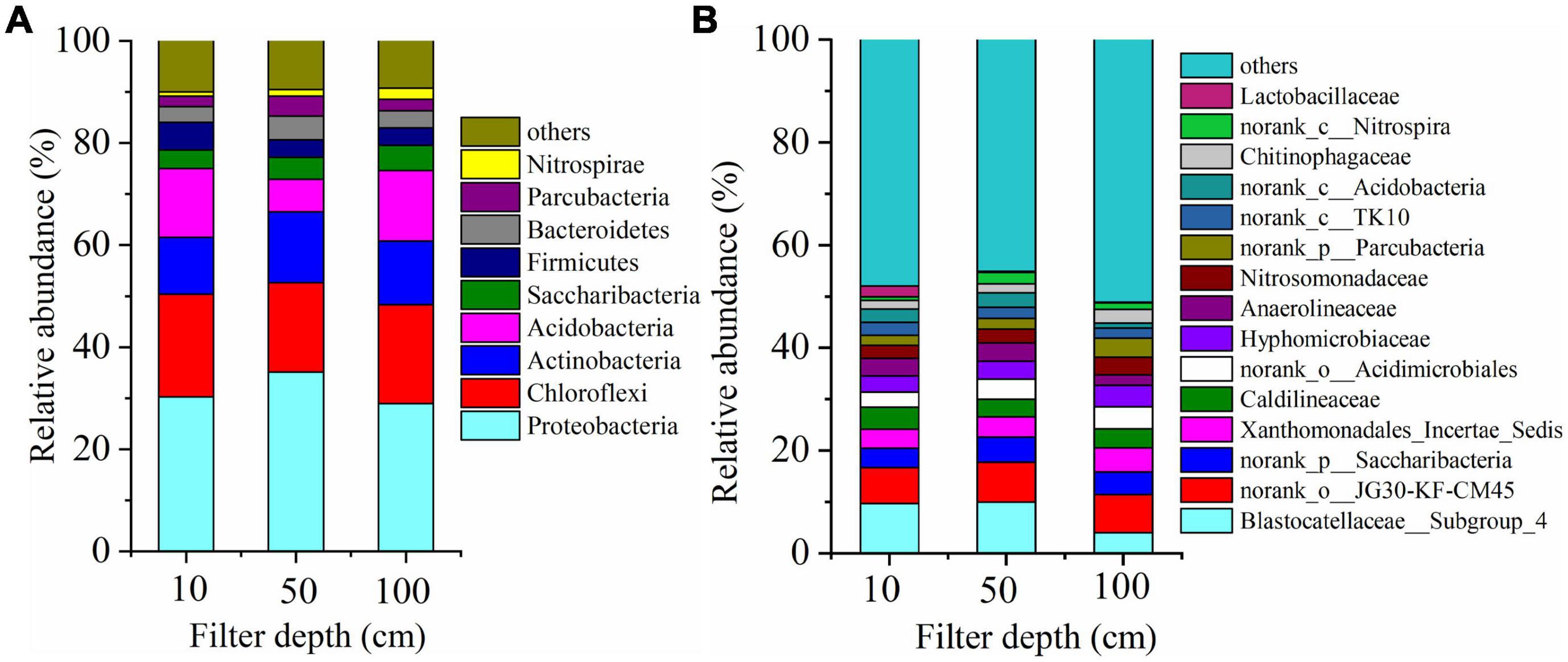
Figure 5. Change of microbial community composition along the DN-DBF depth (A) at phylum level and (B) at genus level.
Figure 5B demonstrates the dominant microbial flora abundance at family level. The abundance of microbial communities was evenly distributed, except for the Blastocatellaceae_Subgroup_4 and norank_o_JG30-KF-CM45 abundance of 6–10%, and the remaining flora abundance fluctuates between 2 and 5%. Chang et al. (2019) used a denitrification filter to remove polluted river, and results showed that Blastocatellaceae was the dominant heterotrophic denitrifying bacteria with a proportion of 2.3%. JG30-KF-CM45 was also a typical denitrifying bacterium and proportional to the organic load in an urban sewage treatment process (Remmas et al., 2017). Meanwhile, the norank_p_Saccharibacteria under the Spirulina was reported as a denitrification group participating in organic matter removal (Sun et al., 2018). Caldilineaceae was with an abundance of about 4%, which was similar to previous reports such as Wu et al. (2018) who found that its share in short-range denitrification was 3.72%. Besides, Xanthomonadales_Incertal_Sidis, Hyphomicrobiaceae, and norank_p_Parcubacteria are typical heterotrophic anti-nitrifying bacteria. norank_c_Acidobacteria, Lactobacilliaceae, and norank_o_Acidimicrobiales were organic degradation bacteria belonging to Acidobacteria, which was related to the addition of carbon sources in the influent. Nitrosomonadaceae and norank_c_Nitrospira are nitrifying bacteria, whose appearance indicated that nitrification occurred at the upper and end of this filter.
Pathway of Nitrogen Metabolism
Figure 6 and Table 6 demonstrate the abundance of key enzymes related to nitrogen cycle depending on functional prediction. Specific enzymes, such as nitrate reductase (EC1.7.2.1), chlorophyllide reductase iron protein subunit X (EC1.18.6.1), nitronate monooxygenase (EC1.13.12.16), and nitrilase (EC3.5.5.1), are involved in the denitrification process of DN-DBF. With the augmentation of the depth of the filter, the reduction of chlorophyllide reductase iron protein subunit X (EC1.18.6.1), nitrilase (EC3.5.5.1), nitrate reductase 2 (EC1.7.99.4), nitric oxide reductase (EC1.7.2.5), and chlorophyllide reductase iron protein subunit X (EC1.18.6.1) took on an upward and then downward tendency. Huang et al. (2020) discussed the abundance of key enzymes related to nitrogen cycle in an iron carbon-based constructed wetland (CW), and chlorophyllide reductase iron protein subunit X (EC1.18.6.1) in CW with Fe0-C filter demonstrated the lowest abundance at the middle layer and the highest value appeared in CW with ceramsite filler, which was consistent with this study.
At the filter depth of 50 cm, the abundance of relative enzyme reached the maximum, and the number and denitrifying activity of corresponding denitrifying bacteria was the highest; therefore, the denitrification was the strongest with the highest nitrogen removal rate in this part. The results coincided with the changes of microbial community composition and diversity. During the denitrification process, nitrite reductase controlled the reduction of NO3–-N and the production of N2O, and the process of transforming N2O to NO was commanded by nitrous oxide reductase. The relative abundance of nitrite reductase gradually increases as the depth increases, and conversely, the relative abundance of nitrous oxide reductase gradually decreased. It indicated that more N2O is converted to NO with the deepening of the filter. It is worth noting that the relative abundance of nitronate monooxygenase demonstrated a decrease first and then ascended. In view of the effect of influent and backwashing, DO was enriched on both ends of the filter. Hence, the abundance of aerobic bacteria was relatively large and resulted in high nitronate monooxygenase abundance in the center of this filter.
Conclusion
The DN-DBF parameters were optimized for the advanced treatment of TN from the secondary effluent. More than 12.7% of TN removal efficiency was obtained by adding methanol compared with sodium acetate, and the optimal influent C/N ratio and HRT were 3:1 and 0.25 h, respectively. The backwash time was 12 h, and the total and attached biomass reached the maximum at 30 cm of DN-DBF, where the diversity and richness of microbial community was higher than that in both ends. High-throughput sequencing technology showed that Proteobacteria, Chloroflexi, Actinobacteria, and Saccharibacteria were dominant flora at phylum level, and Blastocatellaceae_Subgroup_4 and norank_o_JG30-KF-CM45 were dominant denitrification floras with an abundance of 6–10%. Meanwhile, the abundance of nitrite reductase enzymes (EC1.7.2.1) reached the maximum at 50 cm.
Data Availability Statement
The original contributions presented in the study are included in the article/supplementary material, further inquiries can be directed to the corresponding authors.
Author Contributions
ZD and TC made substantial contributions to the conception or design of the work and the acquisition, analysis, or interpretation of data for the work, drafted the work or revised it critically for important intellectual content, gave final approval of the version to be published, and agreed to be accountable for all aspects of the work in ensuring that questions related to the accuracy or integrity of any part of the work are appropriately investigated and resolved. All authors listed have made a substantial, direct, and intellectual contribution to the work, and approved it for publication.
Funding
This work was supported by a project grant from the National Natural Science Foundation of China (421078064), Project of Shenzhen Science and Technology Plan (Grant Nos. KCXFZ202001011006362, KJYY20180718094802190, and JCYJ20200109113006046), Project of China Municipal Engineering Central South Design and Research Institute (Theory and Application: High Efficiency, Energy Saving and Intelligent Biological Enhanced Denitrification Process), and Doctor Program of Entrepreneurship and Innovation in Jiangsu Province (Grant No. R2020SCB10).
Conflict of Interest
The authors declare that the research was conducted in the absence of any commercial or financial relationships that could be construed as a potential conflict of interest.
Publisher’s Note
All claims expressed in this article are solely those of the authors and do not necessarily represent those of their affiliated organizations, or those of the publisher, the editors and the reviewers. Any product that may be evaluated in this article, or claim that may be made by its manufacturer, is not guaranteed or endorsed by the publisher.
Footnotes
- ^ http://ccb.jhu.edu/software/FLASH/
- ^ http://drive5.com/uparse/
- ^ http://huttenhower.sph.harvard.edu/galaxy/root?tool_id=PICRUSt_normalize/
- ^ http://eggnog.embl.de/
- ^ http://www.genome.jp/kegg/
References
Adrados, B., Sanchez, O., Arias, C. A., Becares, E., Garrido, L., Mas, J., et al. (2014). Microbial communities from different types of natural wastewater treatment systems: vertical and horizontal flow constructed wet-lands and biofilters. Water Res. 55, 304–312. doi: 10.1016/j.watres.2014.02.011
APHA (2005). Standard Methods for the Examination of Water and Wastewater, 21th Edn. Washington, DC: American Public Health Association.
Bao, T., Chen, T., Wille, M. L., Chen, D., Yu, S., Bian, J., et al. (2016). Production of zeolite composite filters using waste paper pulp as slow release carbon source and performance investigation in a biological aerated filter. J. Water Process Eng. 9, 38–46. doi: 10.1016/j.jwpe.2015.11.009
Cao, X., Li, Y., Jiang, X., Zhou, P., Zhang, J., and Zheng, Z. (2016). Treatment of artificial secondary effluent for effective nitrogen removal using a combination of corncob carbon source and bamboo charcoal filter. Int. Biodeter. Biodegr. 115, 164–170. doi: 10.1016/j.ibiod.2016.08.018
Chang, J., Mei, J., Jia, W., Chen, J., Li, X., Ji, B., et al. (2019). Treatment of heavily polluted river water by tidal-operated biofilters with organic/inorganic media: evaluation of performance and bacterial community. Bioresour. Technol. 279, 34–42. doi: 10.1016/j.biortech.2019.01.060
Cheng, K. C., and Lin, Y. F. (1993). Relationship between denitrifying bacteria and methanogenic bacteria in a mixed culture system of acclimated sludges. Water Res. 27, 1749–1759. doi: 10.1016/0043-1354(93)90113-V
Christensson, M., Lie, E., and Welander, T. (1994). A comparison between ethanol and methanol as carbon sources for denitrification. Water Sci. Technol. 30, 83–90. doi: 10.2166/wst.1994.0255
Constantin, H., and Fick, M. (1997). Influence of c-sources on the denitrification rate of a high-nitrate concentrated industrial wastewater. Water Res. 31, 583–589. doi: 10.1016/S0043-1354(96)00268-0
Dong, W. Y., Zhang, X. B., Wang, H. J., Sun, F. Y., and Liu, T. Z. (2012). Enhanced denitrification with external carbon sources in a biological anoxic filter. Water Sci. Technol. 66, 2243–2250. doi: 10.2166/wst.2012.460
Fan, B., Qu, J. H., Lei, P. J., and Li, D. P. (2001). Studies on nitrate removal by a combined heterotrophy electrode biofilm reactor. Acta Sci. Circum. 21, 257–262. doi: 10.1016/j.jhazmat.2011.06.008
Farabegoli, G., Gavasci, R., Lombardi, F., and Romani, F. (2003). Denitrification in tertiary filtration: application of an up-flow filter. J. Environ. Sci. Health Part A 38, 2169–2177. doi: 10.1081/ese-120023349
Feng, J., and Tong, Z. (2015). Bacterial assembly and temporal dynamics in activated sludge of a full-scale municipal wastewater treatment plant. ISME J. 9:683. doi: 10.1038/ismej.2014.162
Ge, S., Peng, Y., Wang, S., Lu, C., Cao, X., and Zhu, Y. (2012). Nitrite accumulation under constant temperature in anoxic denitrification process: the effects of carbon sources and COD/NO3–N. Bioresour. Technol. 114, 137–143. doi: 10.1016/j.biortech.2012.03.016
Gómez, M. A., González-López, J., and Hontoria-Garc ′ıa, E. (2000). Influence of carbon source on nitrate removal of contaminated groundwater in a denitrifying submerged filter. Hazard Mater. J. 80, 69–80. doi: 10.1016/S0304-3894(00)00282-X
Haske-Cornelius, O., Gierlinger, S., Vielnascher, R., Gabauer, W., Prall, K., Pellis, A., et al. (2021). Cultivation of heterotrophic algae on paper waste material and digestate. Algal. Res. 54:102193. doi: 10.1016/j.algal.2021.102193
Huang, X., Yang, X. M., Zhu, J., and Yu, J. H. (2020). Microbial interspecific interaction and nitrogen metabolism pathway for the treatment of municipal wastewater by iron carbon based constructed wetland. Bioresour. Technol. 315:123814. doi: 10.1016/j.biortech.2020.123814
Husband, J. A., Slattery, L., Corsoro, F., and Antwi, J. (2014). Full-scale operating experience of deep bed denitrification filter achieving<1.5 mg/l total nitrogen and<0.05 mg/l total phosphorus. Proc. Water Environ. Fed. 12, 3185–3197. doi: 10.2166/wst.2012.874
Karanasios, K. A., Vasiliadou, I. A., Tekerlekopoulou, A. G., Akratos, C. S., Pavlou, S., and Vayenas, D. V. (2016). Effect of C/N ratio and support material on heterotrophic denitrification of potable water in bio-filters using sugar as carbon source. Int. Biodeter. Biodegr. 111, 62–73. doi: 10.1016/j.ibiod.2016.04.020
Khokhar, Z., Syed, Q., Nadeem, M., Irfan, M., Wu, J., Samra, Z., et al. (2014). Enhanced production of cellulase by Trichoderma reesei using wheat straw as a carbon source. World Appl. Sci. J. 30, 1095–1104. doi: 10.5829/idosi.wasj.2014.30.09.14138
Li, W. W., Sheng, G. P., Wang, Y. K., Liu, X. W., Xu, J., and Yu, H. Q. (2011). Filtration behaviors and biocake formation mechanism of mesh filters used in membrane bioreactors. Sep. Purif. Technol. 81, 472–479. doi: 10.1016/j.seppur.2011.08.026
Li, Y., Wang, Q., Wu, Y., Li, W., and Liu, Z. (2014). Application of micro-flocculation and sand filtration as advanced wastewater treatment technique. Desalin. Water Treat. 52, 1299–1306. doi: 10.1080/19443994.2013.787029
Liang, B., Cheng, H., Van Nostrand, J. D., Ma, J., Yu, H., Kong, D., et al. (2014). Microbial community structure and function of nitrobenzene reduction biocathode in response to carbon source switchover. Water Res. 54, 137–148. doi: 10.1016/j.watres.2014.01.052
Liu, C., Wang, X., Yang, H., Liu, C., Zhang, Z., and Chen, G. (2021). Biodegradable polyhydroxyalkanoates production from wheat straw by recombinant Halomonas elongata A1. Int. J. Biol. Macromol. 187, 675–682. doi: 10.1016/j.ijbiomac.2021.07.137
Löwenberg, J., Zenker, A., Krahnstover, T., Boehler, M., Baggenstos, M., Koch, G., et al. (2016). Upgrade of deep bed filtration with activated carbon dosage for compact micropollutant removal from wastewater in technical scale. Water Res. 94, 246–256. doi: 10.1016/j.watres.2016.02.033
Luo, G., Hou, Z., Tian, L., and Tan, H. (2020). Comparison of nitrate-removal efficiency and bacterial properties using PCL and PHBV polymers as a carbon source to treat aquaculture water. Aquac. Fish. 5, 92–98. doi: 10.1016/j.aaf.2019.04.002
Ma, Q., Qu, Y., Shen, W., Zhang, Z., Wang, J., Liu, Z., et al. (2015). Bacterial community compositions of coking wastewater treatment plants in steel industry revealed by Illumina high-throughput sequencing. Bioresour. Technol. 179, 436–443. doi: 10.1016/j.biortech.2014.12.041
Magoc, T. J., and Salzberg, S. L. (2011). FLASH: fast length adjustment of short reads to improve genome assemblies. Bioinformatics 27, 2957–2963. doi: 10.1093/bioinformatics/btr507
Piao, W., and Kim, Y. (2016). Evaluation of monthly environmental loads from municipal wastewater treatment plants operation using life cycle assessment. Environ. Eng. Res. 21, 284–290. doi: 10.4491/eer.2015.124
Remmas, N., Melidis, P., Zerva, I., Kristoffersen, J. B., Nikolaki, S., Tsiamis, G., et al. (2017). Dominance of candidate Saccharibacteria in a membrane bioreactor treating medium age landfill leachate: effects of organic load on microbial communities, hydrolytic potential and extracellular polymeric substances. Bioresour. Technol. 238, 48–56. doi: 10.1016/j.biortech.2017.04.019
Schloss, P. D., Gevers, D., and Westcott, S. L. (2011). Reducing the effects of PCR amplification and sequencing artifacts on 16S rRNA-based studies. PLoS One 12:e27310. doi: 10.1371/journal.pone.0027310
Shen, Z., and Wang, J. (2011). Biological denitrification using cross-linked starch/PCL blends as solid carbon source and biofilm carrier. Bioresour. Technol. 102, 8835–8838. doi: 10.1016/j.biortech.2011.06.090
Shi, Y., Wei, N., and Wu, G. (2016). Tertiary denitrification of the secondary effluent in biofilters packed with composite carriers under different carbon to nitrogen ratios. Environ. Eng. Res. 21, 311–317. doi: 10.4491/eer.2015.134
Simate, G. S. (2015). The treatment of brewery wastewater for reuse by integration of coagulation/flocculation and sedimentation with carbon nanotubes ‘sandwiched’ in a granular filter bed. J. Ind. Eng. Chem. 21, 1277–1285. doi: 10.1016/j.jiec.2014.06.001
Sun, Y. X., Shen, D., Zhou, X., Shi, N., and Tian, Y. (2016). Microbial diversity and community structure of denitrifying biological filters operated with different carbon sources. SpringerPlus 5:1752. doi: 10.1186/s40064-016-3451-3
Sun, Z., Xie, D. G., Jiang, X. R., Fu, G. H., Xiao, D. X., and Zheng, L. (2018). Effect of eco-remediation and microbial community using multilayer solar planted floating island (MS-PFI) in the drainage channel. bioRxiv [Preprint] bioRxiv: 327965, doi: 10.1101/327965
Tian, L., and Wang, L. (2021). Multi-omics analysis reveals structure and function of biofilm microbial communities in a pre-denitrification biofilter. Sci. Total Environ. 757:143908. doi: 10.1016/j.scitotenv.2020.143908
Wang, H., Chen, N., Feng, C., and Deng, Y. (2021). Insights into heterotrophic denitrification diversity in wastewater treatment systems: progress and future prospects based on different carbon sources. Sci. Total Environ. 780:146521. doi: 10.1016/j.scitotenv.2021.146521
Wang, Q., Feng, C., Zhao, Y., and Hao, C. (2009). Denitrification of nitrate contaminated groundwater with a fiber-based biofilm reactor. Bioresour. Technol. 100, 2223–2227. doi: 10.1016/j.biortech.2008.07.057
Wang, Z., Fei, X., He, S., Huang, J., and Zhou, W. (2016). Application of light-weight filtration media in an anoxic biofilter for nitrate removal from micro-polluted surface water. Water Sci. Technol. 74, 1016–1024. doi: 10.2166/wst.2016.299
Wei, N., Shi, Y., Wu, G., Hu, H., Guo, Y., Wu, Y., et al. (2016). Removal of nitrogen and phosphorus from the secondary effluent in tertiary denitrifying biofilters combined with micro-coagulation. Water Sci. Technol. 73, 2754–2760. doi: 10.2166/wst.2016.130
Wu, C., Wang, Y., Zhou, Y., and Zhu, C. (2016). Pretreatment of petrochemical secondary effluent by micro-flocculation and dynasand filtration: performance and DOM removal characteristics. Water Air Soil Pollut. 227:415. doi: 10.1007/s11270-016-3118-2
Wu, L., Li, Z., Zhao, C., Liang, D., and Peng, Y. (2018). A novel partial-denitrification strategy for post-anammox to effectively remove nitrogen from landfill leachate. Sci. Total Environ. 633, 745–751. doi: 10.1016/j.scitotenv.2018.03.213
Wu, X. H., and Li, Y. M. (2017). Effect of C/N ratio on denitrification of denitrification filters with different filter materials. Chinese J. Environ. Eng. 11, 55–62.
Xu, J. H., He, S. B., Wu, S. Q., Huang, J. C., Zhou, W. L., and Chen, X. C. (2016). Effects of HRT and water temperature on nitrogen removal in autotrophic gravel filter. Chemosphere 147, 203–209. doi: 10.1016/j.chemosphere.2015.12.136
Yang, L., Guo, L. K., Ren, Y. X., Dou, J. W., Zhu, P. T., Cui, S., et al. (2021). Denitrification performance, biofilm formation and microbial diversity during startup of slow sand filter using powdery polycaprolactone as solid carbon source. J. Environ. Chem. Eng. 9:105561. doi: 10.1016/j.jece.2021.105561
Keywords: advanced treatment, denitrification deep-bed filter, conditions optimization, total nitrogen, metabolic pathway
Citation: Huang X, Xing Y, Wang H, Dai Z and Chen T (2022) Nitrogen Advanced Treatment of Urban Sewage by Denitrification Deep-Bed Filter: Removal Performance and Metabolic Pathway. Front. Microbiol. 12:811697. doi: 10.3389/fmicb.2021.811697
Received: 09 November 2021; Accepted: 10 December 2021;
Published: 26 January 2022.
Edited by:
Tian Li, Nankai University, ChinaReviewed by:
Junfeng Chen, Qufu Normal University, ChinaWentao Shang, The University of Hong Kong, Hong Kong SAR, China
Copyright © 2022 Huang, Xing, Wang, Dai and Chen. This is an open-access article distributed under the terms of the Creative Commons Attribution License (CC BY). The use, distribution or reproduction in other forums is permitted, provided the original author(s) and the copyright owner(s) are credited and that the original publication in this journal is cited, in accordance with accepted academic practice. No use, distribution or reproduction is permitted which does not comply with these terms.
*Correspondence: Hongjie Wang, whj1533@qq.com; Tiantian Chen, chentian0819@163.com