- Guangdong Provincial Key Laboratory of Microbial Safety and Health, State Key Laboratory of Applied Microbiology Southern China, Institute of Microbiology, Guangdong Academy of Sciences, Guangzhou, China
The contamination of antibiotic resistance genes (ARGs) may directly threaten human health. This study used a metagenomic approach to investigate the ARG profile in a drinking water treatment system (DWTS) in south China. In total, 317 ARG subtypes were detected; specifically, genes encoding bacitracin, multidrug, and sulfonamide were widely detected in the DWTS. Putative ARG hosts included Acidovorax (6.0%), Polynucleobacter (4.3%), Pseudomonas (3.4%), Escherichia (1.7%), and Klebsiella (1.5%) as the enriched biomarkers in the DWTS, which mainly carried bacitracin, beta-lactam, and aminoglycoside ARGs. From a further analysis of ARG-carrying contigs (ACCs), Stenotrophomonas maltophilia and Pseudomonas aeruginosa were the most common pathogens among the 49 ACC pathogens in the DWTS. The metagenomic binning results demonstrated that 33 high-quality metagenome-assembled genomes (MAGs) were discovered in the DWTS; particularly, the MAG identified as S. maltophilia-like (bin.195) harbored the greatest number of ARG subtypes (n = 8), namely, multidrug (n = 6; smeD, semE, multidrug_transporter, mexE, semB, and smeC), beta-lactam (n = 1; metallo-beta-lactamase), and aminoglycoside [n = 1; aph(3’)-IIb]. The strong positive correlation between MGEs and ARG subtypes revealed a high ARG dissemination risk in the DWTS. Based on the pure-culture method, 93 isolates that belong to 30 genera were recovered from the DWTS. Specifically, multidrug-resistant pathogens and opportunistic pathogens such as P. aeruginosa, Bacillus cereus, and S. maltophilia were detected in the DWTS. These insights into the DWTS’s antibiotic resistome indicated the need for more comprehensive ARG monitoring and management in the DWTS. Furthermore, more effective disinfection methods need to be developed to remove ARGs in DWTSs, and these findings could assist governing bodies in the surveillance of antibiotic resistance in DWTSs.
Introduction
Antibiotic resistance genes (ARGs) are emerging environmental pollutants (Pruden et al., 2006). The excessive use and abuse of antibiotics in medicine and agriculture have promoted the emergence, spread, and accumulation of ARGs in various environmental matrices (Jia et al., 2019), including natural and man-impacted environments, such as soil (Fang et al., 2015), sediment (Jiang et al., 2018), surface water (Zhang et al., 2020b), wastewater (Wang et al., 2020), groundwater (Wu et al., 2020), drinking water (Wan et al., 2020), and tap water (Ma et al., 2019). Thus, antimicrobial resistance has become a global issue and is regarded as a medical concern by both researchers and the public.
Additionally, antibiotic-resistant bacteria (ARB) have become a serious threat to human health. In particular, pathogenic bacteria might directly affect the treatability of patients with infectious diseases (Kumar et al., 2019). There is some literature on the occurrence of ARB in drinking water and the evaluation of the impact of ARB on human health. However, most of the research has only investigated one species of bacteria or a group of resistant bacteria, such as Pseudomonas aeruginosa strains (Mombini et al., 2019), aminoglycoside-resistant bacteria (Ullmann et al., 2019), Escherichia coli, and Salmonella spp. (Perez-Vidal et al., 2019), and the presence of β-lactamase-producing Gram-negative bacteria (Torkar and Drazetic, 2017). There has been limited comprehensive research on the antibiotic resistance of different species of bacteria or multigroup-resistant bacteria in a drinking water treatment system (DWTS). Thus, a comprehensive risk identification of ARB in DWTSs has not been conducted.
Thus, it is necessary to fully understand the occurrence and abundance of ARGs and ARB in DWTSs. However, very few studies have reported the occurrence and distribution of ARGs and ARB in the whole drinking water treatment process. Bergeron et al. investigated the ARB and ARGs in raw source water and treated drinking water and found that the ARGs of sulfonamides and tetracycline antibiotics and ARB were frequently observed in the source water (Bergeron et al., 2015). Moreover, Bergeron et al. surveyed the presence of ARGs and ARB in a rural drinking water plant in Louisiana, United States, and demonstrated that ARGs and ARB were present in the raw intake water but not in the treated and distributed water (Bergeron et al., 2017). With the development of sequencing technology and high-throughput DNA testing techniques, more ARGs have been discovered in finished water and tap water, which arouses great concern as a public health issue. For example, Su et al. used the quantitative PCR (qPCR) method to investigate the occurrence and diversity of ARGs in the DWTS and found that most ARGs, including sul1, ermB, tetQ, tetW, cfr, cmlA, fexA, fexB, floR, and qnrS, significantly increased from finished water in the distribution system to tap water, and Pseudomonas might be involved in the proliferation and spread of ARGs in the DWTS (Su et al., 2018). Furthermore, Xu et al. used high-throughput qPCR to detect ARGs in the DWTS, and the results demonstrated that 285 ARGs were discovered, which provided comprehensive information on the prevalence of ARGs in the DWTS (Xu et al., 2016).
Compared to qPCR and high-throughput qPCR approaches, a high-throughput sequencing-based metagenomic analysis can overcome the drawbacks of amplification-based methods, including the limited availability of primers, the possible bias in the amplification process, and the false-negative results caused by an enzyme inhibitor in the environmental samples. Moreover, a metagenomic approach could obtain more comprehensive profiles of ARGs. For example, Bai et al. applied metagenomic sequencing to investigate the prevalence of ARGs in drinking water sources, and the results indicated that 71 ARG subtypes within 14 ARG types were present (Bai et al., 2019). Jia et al. also applied metagenomic sequencing to investigate the changing profiles of ARGs and bacterial community in a DWTS and demonstrated that 151 ARG subtypes within 15 ARG types were detected, and chlorination increased their total relative abundance and reduced their diversity in the opportunistic bacteria (Jia et al., 2015). Therefore, a metagenomic approach could assist in investigating the full profile of ARGs in the DWTS comprehensively and determining ARB biomarkers as putative ARG carriers.
Mobile genetic elements (MGEs) play a key role in transferring ARGs among different microorganisms in the environments (Zhu et al., 2019). MGEs (e.g., plasmids, transposons, and integrons) are widely distributed in environments, and they help the dissemination of ARGs via horizontal gene transfer (Zhang et al., 2019). Statistics and network analyses have demonstrated a close positive correlation between ARG subtypes and MGEs (Qian et al., 2018; Zeng et al., 2019; Pu et al., 2020). Moreover, recently, a metagenomic analysis provided direct evidence on the co-occurrence patterns of ARGs and MGEs, which accurately indicated the possible transfer potential of ARGs in environmental compartments such as feces, soils, and water (Zhang et al., 2020c).
The Pearl River Delta (PRD) is a densely populated area, and the three major tributaries of the Pearl River Basin—Dongjiang, Xijiang, and Beijiang—are the main drinking water sources, serving more than 1.4 million people in Guangzhou, China (Su et al., 2018). However, information about the presence and abundance of ARGs, MGEs, and ARB in the DWTS in this area is still limited. Although some researchers have investigated the ARG profiles of drinking water sources in the PRD region using a metagenomic approach, the occurrence and abundance of ARGs in different phases of the DWTS in the PRD region have not been determined (Jiang et al., 2018). Additionally, researchers have investigated the limited target ARGs in the DWTS in the PRD region, but there has been no comprehensive research on MGEs and ARB in the studied area (Su et al., 2018). Subsequently, the results of the present study can provide a better understanding of the diversity, abundance, and dissemination of ARGs and ARB in the DWTS in the PRD region.
Materials and Methods
Sample Collection and DNA Extraction
The raw water from the selected drinking water treatment plant (DWTP) (Guangzhou, China) originates in the North Rivers of Pearl River, and its phased treatment includes flocculation, sedimentation, filtration, and chlorination (Figure 1). Water samples were collected along the treatment processes of the DWTP and distribution pipeline, including samples for raw water (A), flocculation tank effluent (B), sedimentation tank effluent (C), quartz sand (D), sand filter effluent (E), chlorine-disinfected water (F), and tap water (G). They were respectively collected from a water pumping station (5 L), flocculation tank (18 L), sedimentation tank (18 L), sand filter (36 L), clear water tank (54 L), and tap water (about 2,000 L) (Figure 1). Additionally, quartz sand samples (D) were obtained from the upper part of the quartz-sand layer in the sand filter (50 g). To minimize the temporal variation, three replicate water samples were simultaneously collected from each location (21 samples in total) in July 2019. All of the samples were immediately transported to a laboratory for the experiments. The water sampling and pre-treatment methods were performed as previously described27. DNA for each sample was extracted using a FastDNA SPIN Kit for Soil (MP Biomedicals, CA, United States), and the DNA concentration and purity were measured using a microspectrophotometry (Nano DropND-2000, NanoDrop Technologies, Wilmington, DE, United States).
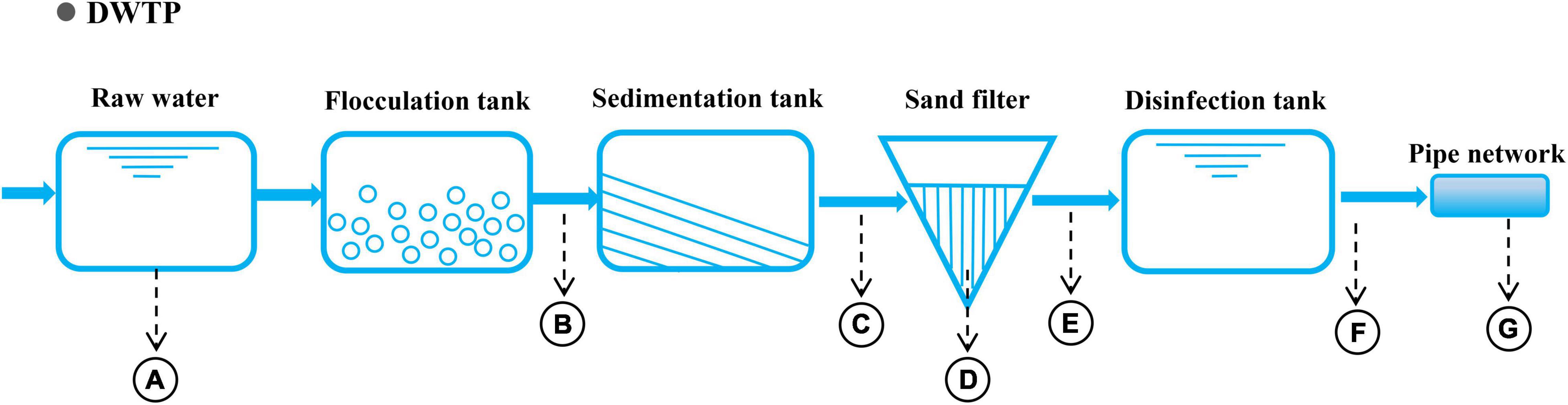
Figure 1. Process diagram of the DWTP and sampling sites. Sampling points: A: raw water; B: flocculation tank effluent; C: sedimentation tank effluent; D: quartz sand; E: sand filter effluent; F: chlorine-disinfected water; and G: tap water.
Water Quality Analyses
For each sampling site, temperature, pH, and dissolved oxygen (DO) level were measured in situ using an HQ40D portable multi-parameter water quality analyzer (HACH, Loveland, CO, United States). Turbidity was measured using a 2100Q portable turbidimeter (HACH, Loveland, CO, United States). Ammonia-nitrogen, chemical oxygen demand (COD), and total organic carbon (TOC) were measured using a DR1900 portable spectrophotometer (HACH, Loveland, CO, United States). Adenosine triphosphate (ATP) level was measured with an ATP assay kit (Beyotime, Shanghai, China). All determinations were performed in triplicate.
Cultivation and Molecular Identification of Bacteria
Bacteria were isolated from the water samples of the different sampling sites. Briefly, 0.2 ml of water samples or proper dilution of the water samples was spread on R2A agar plates with an L-type coating rod. Then, all the plates were incubated at 22°C for 7 days. Subsequently, total heterotrophic bacterial counts (HPCs) were determined by counting the number of colonies on the R2A agar plates. Next, colonies with different morphologies were picked and purified using the streaking method (Massa et al., 1998). The process was repeated until a pure bacterial strain was obtained. To identify the obtained bacteria, all pure isolates were first incubated in R2A broth for 24 h, and then, genomic DNA for each pure isolate was extracted using a DNA extraction kit (Magen Biotech, Guangzhou, China). Subsequently, the 16S rRNA gene was amplified in a 25-μl volume using universal primers 27 F and1492 R (Mas-Ud et al., 2020), and amplification was performed as previously described (Gu et al., 2018). Then, PCR products were sequenced by BGI Tech Solutions Co., Ltd (Guangzhou, China), and 16S rRNA gene sequences were submitted to the NCBI public library of bioinformatics for comparison and identification.1
Antimicrobial Susceptibility Testing
Antibiotic resistance of the representative bacterial isolates from the DWTS was determined using the standard Kirby–Bauer disc diffusion method according to Clinical and Laboratory Standards Institute Guidelines (CLSI, 2020). The antibiotics and concentrations of the disks (Oxoid, Basingstoke, United Kingdom) used in this study were as follows: piperacillin-tazobactam (TZP, 100/10 μg), norfloxacin (NOR, 10 μg), clindamycin (DA, 2 μg), cefepime (FEP, 3 μg), ceftriaxone (CRO, 30 μg), ciprofloxacin (CIP, 5 μg), penicillin (P, 10 μg), cefotaxime (CTX, 30 μg), chloramphenicol (C, 30 μg), ampicillin (AMP, 10 μg), trimethoprim-sulfamethoxazole (SXT, 1.25/23.75 μg), erythromycin (E, 15 μg), aztreonam (ATM, 30 μg), meropenem (MEM, 10 μg), cefoxitin (FOX, 30 μg), amikacin (AK, 30 μg), kanamycin (K, 30 μg), tobramycin (TOB, 10 μg), amoxicillin-clavulanate (AMC, 30 μg), tetracycline (TE, 30 μg), gentamicin (CN, 10 μg), ceftazidime (CAZ, 30 μg), and levofloxacia (LEV, 5 μg). Additionally, multiple antibiotic resistance index (MARI) values were calculated for all the isolates to evaluate whether they originated from high-risk antibiotic pollution sources (Labella et al., 2021).
Metagenomic Sequencing and Metagenomic Analysis
The extracted DNA from each sample was sequenced using the Illumina HiSeq 2500 platform (Illumina Inc., San Diego, CA, United States). Over 10 Gb of raw data was generated for each sample (Supplementary Table 1). Quality control and assembly were conducted as described previously (Bai et al., 2021). To obtain low-abundance species, the obtained clean data were mapped to its own Scaftigs to obtain un-using reads, and then all the un-using reads were collated for hybrid packaging. The open reading frames (ORFs) within the contigs of each sample were subsequently predicted using Prodigal.2 The gene prediction results were used for gene clustering by Linclust.3 The longest sequence in each cluster was taken as the representative sequence to obtain the non-redundant gene catalogue (Unigenes). Clean reads were mapped to the gene catalogue by BBmap,4 and the relative abundance of each gene in each sample was calculated using the number of reads mapped and gene length with the following formula:
where k stands for any identified gene, Gk is the relative abundance of each identified gene, rk is the number of mapped metagenomic reads for each identified gene, and Lk is the length of each identified gene. ARGs were detected against a structured ARG reference database (SARG version 2.0) with the following parameters: under a cutoff of ≥80% similarity and ≥70% query coverage (Yin et al., 2018). Furthermore, an online analysis pipeline (ARGs-OAP) based on the SARG database was used for the ARG-like sequence annotation from the metagenomic sequencing reads with default settings (Yin et al., 2018). Subsequently, the abundance of ARGs was normalized and expressed as the number of copies of ARGs per cell (copy/cell) (Ma et al., 2017). A contig was retrieved as an ARG-carrying contig (ACC) if it contained ≥ one ARG-ORF (Zhang et al., 2019). The contigs that harbored ARGs were selected, and the protein sequences on the contigs were used to make a comparison against the local NCBI NR database using BLASTP with a cutoff of E-value ≤ 10–5 (Mao et al., 2014). Subsequently, the MEtaGenome ANalyzer (MEGAN, version 6) was used to align the profiles. If ≥ 50% of ORFs on a contig were attributed to the same taxon, these ARG-carrying contigs (ACCs) were assigned to this taxon (Ishii et al., 2013). ARG-carrying pathogens were determined according to a previous study (Li et al., 2015).
The plasmids, integrons, and insert sequences were identified based on aclame, integration, and ISfinder databases with the following parameters: under a cutoff of identity ≥80% and query cover ≥70%. Metagenome binning, bin checking, and taxonomic annotation were performed as previously described (Poghosyan et al., 2020). The metagenome bins that were >50% complete with <10% contamination were retained for building the phylogenomic tree using GToTree (https://github.com/AstrobioMike/GToTree/wiki). The abundance [reported as reads per kilobase per million (RPKM)] of genes was conducted as previously described (Potgieter et al., 2020). The occurrence of ARGs in chromosomes or plasmids was also determined as previously described (Camacho et al., 2009), and MAFFT (Katoh and Standley, 2013) was used to align the amino acid sequences of IntI genes recovered from the metagenomes together with the reference sequences of class 1 (IntI1, AAQ16665.1) integrases. Raw data have been submitted to the NCBI Sequence Read Archive (SRA) under accession number SRR17063149-17063169.
Results and Discussion
Water Quality
The results of the physicochemical and microbiological parameters of water samples are illustrated in Table 1. The water temperature was 27–28°C, and the water pH was 7.63–8.16. The turbidity (NTU) decreased significantly from 69.47 to 2.62 NTU after the settling process. Water DO maintained a steady state during the water treatment process. However, the ATP and HPC gradually decreased. After chlorination, no cultivable bacteria were detected. However, in the tap water, 10 CFU/ml HPC were detected. CODMn decreased gradually with the treatment process and increased slightly after entering the pipe network. TOC was always kept at a very low level. Other parameters, such as TN, also demonstrated a downward trend. They remained at a low concentration in the chlorine-disinfected water, even in the tap water. All the physicochemical qualities of the chlorine-disinfected water met the drinking water standards.
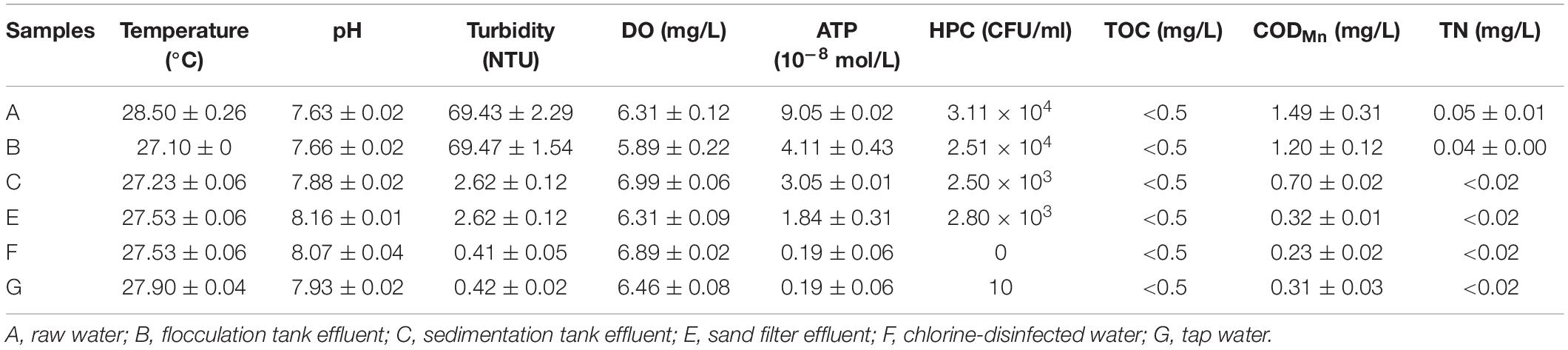
Table 1. Physicochemical and microbiological parameters measured at different treatment units for drinking water.
Bacterial Taxa Particularly Enriched in the Drinking Water Treatment System
The dominant bacterial phyla and genera were identified based on the metagenomic data in every sampling site (Figure 2). A total of 95 bacterial phyla were identified. The relative abundances of the top nine bacteria at the phyla level in all samples are illustrated in Figure 2A. Proteobacteria and Actinobacteria were the dominant phyla in the DWTP, and they shifted largely during the water treatment process. After flocculation, the relative abundance of Proteobacteria was greatly improved and then remained at a certain ratio until chlorination, whereas the proportion of Actinobacteria decreased sharply after flocculation, followed by a slight increase after the settling process. However, the fate of the Actinobacteria was the same as the Proteobacteria; it decreased greatly after chlorination but maintained a certain ratio.
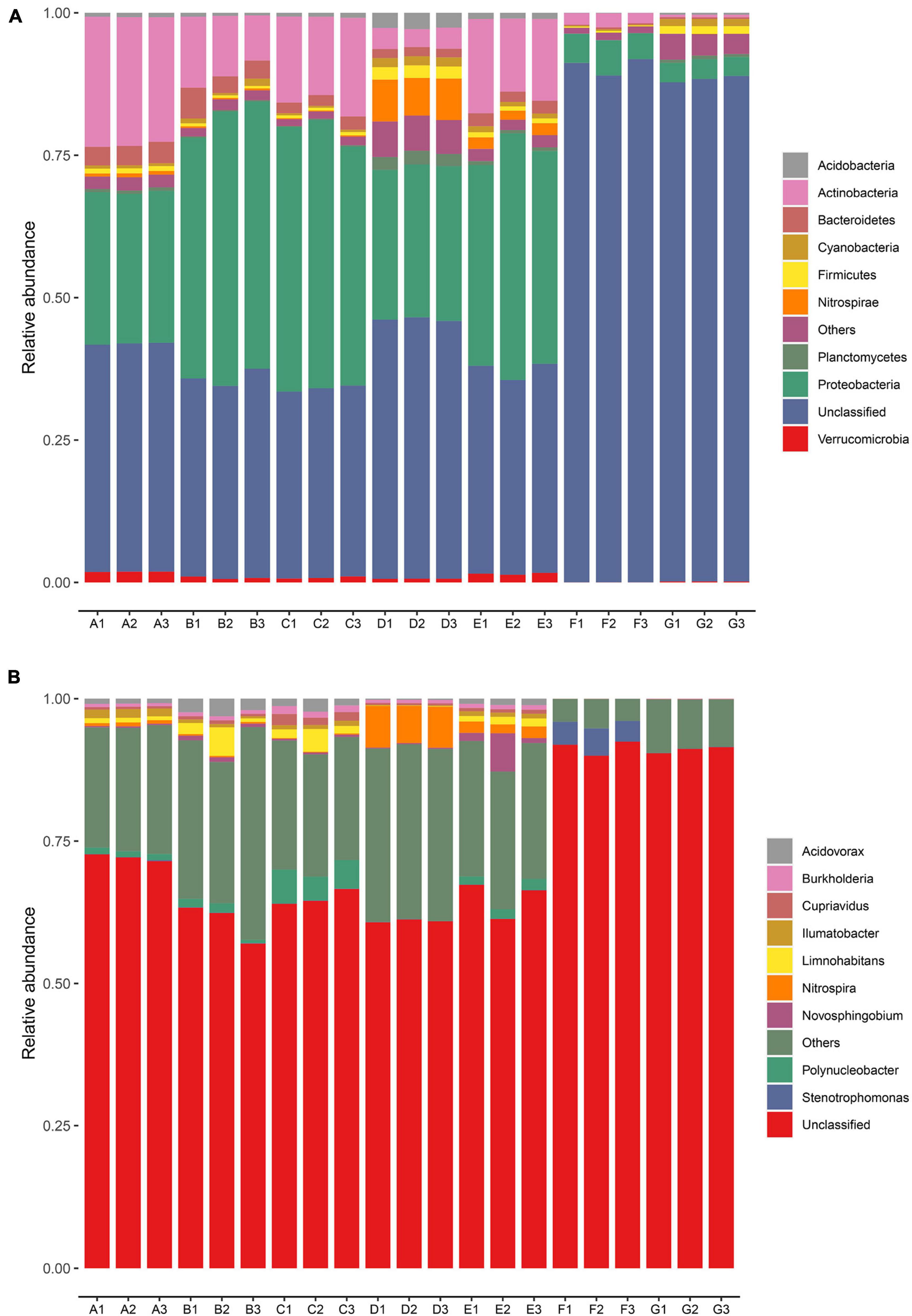
Figure 2. Relative abundance of bacterial community in the DWTS bulk water and sand biofilm at the phylum level (A) and genus level (B). Sequences that could not be classified into any known phylum or genus are assigned as unclassified bacteria. The rare species with relative abundance <0.1% are assigned to others. A1–A3: raw water; B1–B3: flocculation tank effluent; C1–C3: sedimentation tank effluent; D1–D3: quartz sand; E1–E3: sand filter effluent; F1–F3: chlorine-disinfected water; G1–G3: tap water. Three samples were taken from each sampling site.
Previous studies have indicated that δ-Proteobacteria could be used as a biomarker to distinguish source water and tap water (Han et al., 2020). In other words, δ-Proteobacteria exhibit relatively low chlorine resistance, but α-Proteobacteria, β-Proteobacteria, γ-Proteobacteria, and Actinobacteria demonstrate strong resistance to the water treatment processes, including chlorination. Similarly, another author found that α-Proteobacteria and Actinobacteria were dominant in all the chlorine-disinfection samples (Li et al., 2020). Thus, in this study, we observed that the phyla of Proteobacteria and Actinobacteria were still predominant in the finished water. Moreover, the microbial community structure in the sand biofilm was significantly different from the water samples. The relative abundance of Nitrospirae, Planctomycetes, Chloroflexi, and Acidobacteria in the sand biofilm was higher than in the water samples. We also observed that the microbial community in the sand biofilm shaped the sand filter effluent. This finding is consistent with our previous study (Hou et al., 2018) and others (Oh et al., 2018; Zhou et al., 2021). Eustigmatophyceae, Firmicutes, and Planctomycetes in the finished water increased when they passed through the pipe network into the tap water, which might be involved in the regrowth and secondary contamination of bacteria in the pipe network (Douterelo et al., 2016). Some previous studies have demonstrated that the bacterial community is strongly correlated with the resistance profile (Jia et al., 2015; Zhang L. et al., 2018; Chen et al., 2019). In particular, Proteobacteria and Acidobacteria are important hosts of ARGs (Ma et al., 2019), and some studies have detected significant positive correlations between them and ARGs (Su et al., 2015; Xu et al., 2020). Thus, the changes in the relative abundance of Proteobacteria and Acidobacteria might explain the variations in the abundances of ARGs during the water treatment processes.
As evident in Figure 2B, at the genus level, the dominant genera were Polynucleobacter, Acidovorax, Limnohabitans, and Nitrospira, of which Acidovorax has been reported to be the host of ARGs in the DWTP (Jia et al., 2019). After chlorine disinfection, the relative abundance of Stenotrophomonas greatly increased and became the predominant bacteria. Stenotrophomonas has been reported to be typical chlorine-resistant bacteria (Luo et al., 2021), and it harbors many ARGs (Gil-Gil et al., 2020). Some studies reported that chlorine disinfection leads to an increase in the abundance of antibiotic-resistant bacteria (Khan et al., 2016), and chlorine disinfection affects the horizontal transfer of ARG through various pathways, including increasing the relative abundance of MGEs (Zhang et al., 2019). Moreover, some researchers have demonstrated that bacteria that survived chlorine disinfection might have higher levels of antibiotic resistance than other bacteria (Jia et al., 2015). Furthermore, the increased ARG abundance of chlorine-resistant bacteria might increase antibiotic resistance in pathogenic bacteria. Therefore, chlorine-resistant bacteria deserve attention.
Antibiotic Resistome in the Drinking Water Treatment System
Metagenomic data based on the structured SARG database were used to profile the antibiotic resistome in the DWTS. All the identified ARG-like sequences were classified into 13 ARG types (e.g., aminoglycoside and bacitracin resistance gene, as illustrated in Supplementary Material 1) according to the set script. As illustrated in Figure 3A, the abundance of 13 ARG types detected in all samples was normalized as the number of copies of ARGs per cell (copy/cell). Gene-encoded bacitracin (0.3 × 10–2 to 0.40 copies/cell, mean value = 0.23 ± 0.11 copies/cell), multidrug (0.02 to 0.23 copies/cell, mean value = 0.13 ± 0.05 copies/cell), and sulfonamide (0.2 × 10–3 to 0.7 × 10–1 copies/cell, mean value = 0.05 ± 0.02 copies/cell) ARGs were detected at the highest levels of abundance in the A–E samples. Previous studies have also reported that bacitracin, multidrug, and sulfonamide ARGs were predominant in drinking water samples (Ma et al., 2019; Zhang et al., 2020d).
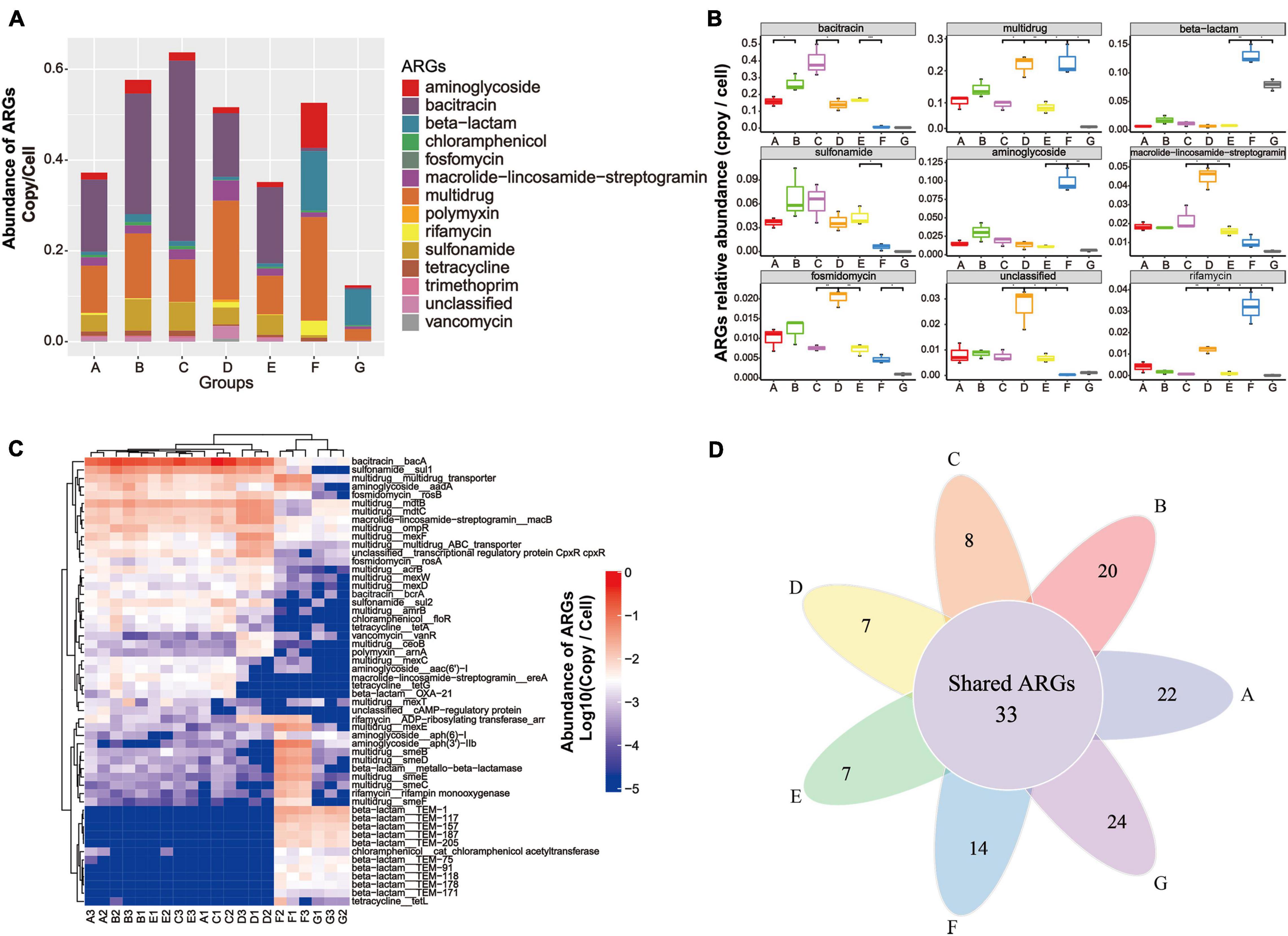
Figure 3. Occurrence and abundance of ARG types and ARG subtypes in the DWTS bulk water and sand biofilm. (A) Characterization of ARG types and abundance. (B) Boxplots showing the number of detected ARG types in the DWTS bulk water and sand biofilm. (C) Occurrence and fate of the top 20 ARG subtypes in the DWTS bulk water and sand biofilm. (D) Venn diagram of ARGs subtypes in the DWTS bulk water and sand biofilm. A1–A3: raw water; B1–B3: flocculation tank effluent; C1–C3: sedimentation tank effluent; D1–D3: quartz sand; E1–E3: sand filter effluent; F1–F3: chlorine-disinfected water; G1–G3: tap water. Three samples were taken from each sampling site.
However, aminoglycoside, beta-lactam, and rifamycin ARGs were notably enriched after chlorination. Moreover, in tap water, beta-lactam and multidrug ARGs retained a high abundance when they passed through the drinking water distribution system, which might threaten human health. From another perspective, the bacitracin ARG was mainly distributed in the B and C samples, which account for about 36 and 30%, respectively (Supplementary Figure 1 and Supplementary Material 2). The multidrug ARGs were mainly distributed in the B and F samples, which account for 20 and 26%, respectively (Supplementary Figure 1), while the sulfonamide ARGs were distributed in the A, B, C, and E samples. Similarly, as evident from Figure 3B, bacitracin and sulfonamide ARGs can be significantly removed by sand filters and chlorination. In other words, the DWTS effectively removed these ARGs, while the other ARGs cannot be eliminated very well. Some ARG types increased through the DWTS, such as multidrug, beta-lactam, aminoglycoside, and rifamycin (Figure 3B).
In total, 464 ARG subtypes were annotated (e.g., bacA, sul1 and multidrug_transporter, illustrated in Supplementary Material 1). Figure 3C illustrates the profile of the top 20 ARG subtypes during the drinking water treatment processes. Bacitracin_bacA (0.1 to 0.5 copies/cell) had the highest levels of abundance in the A–E samples, followed by sulfonamide_sul1 (0.2 × 10–1 to 0.9 × 10–1 copies/cell) and multidrug_mdtB (0.2 × 10–1 to 0.5 × 10–1 copies/cell). After chlorination, gene-encoded multidrug (acrB, amrB, and ceoB), bacitracin (bcrA), sulfonamide (sul1 and sul2), chloramphenicol (floR), tetracycline (tetA and tetG), polymyxin (arnA), macrolide-lincosamide-streptogramin (ereA), and beta-lactam (OXA-21) decreased sharply. Previous studies have reported that tetA could be removed using free chlorine, and this is similar to our results (Amarasiri et al., 2020). However, some researchers have demonstrated that the abundance of sul1 and sul2 increased after chlorination (Liu et al., 2018; Zhu et al., 2021). Subsequently, the shift of these ARGs remains controversial when chlorine is used.
Additionally, multidrug (multidrug_transporter, mexE, smeB, smeC, smeD, smeE, and smeF), aminoglycoside [aadA, aph (6)-1, aph (3’)-IIb], beta-lactam (metallo-beta-lactamase, TEM-1, TEM-117, TEM-157, TEM-187, TEM-205, TEM-75, TEM-91, and TEM-118), and rifamycin (rifampin monooxygenase) were enriched. With respect to the phenomenon that some ARGs increased after chlorine disinfection, some researchers have pointed out that free chlorine could promote the transformation of ARGs through a series of cell responses, for example, raising levels of reactive oxygen species (ROS), ROS-mediated DNA damage, and bacterial membrane damage (Zhang et al., 2021). Our results indicated that chlorination reduced the diversity of the ARG types. Additionally, the Venn diagrams for the different drinking water treatment processes display the number of unique and shared ARG subtypes at each sampling site (Figure 3D). The shared 33 ARG subtypes, which constituted 10% of the total ARG subtypes, were universally present from the raw water to tap water (Supplementary Material 3). The shared ARG subtypes were distributed in aminoglycoside (n = 1), aminoglycoside (n = 2), bacitracin (n = 2), beta-lactam (n = 2), fosmidomycin (n = 2), macrolide-lincosamide-streptogramin (n = 2), multidrug (n = 18), rifamycin (n = 1), tetracycline (n = 1), vancomycin (n = 1), and unclassified (n = 1), respectively. Thus, the largest variety of shared ARG subtypes belonged to the multidrug ARGs. These shared ARG subtypes persistent in the DWTS will lead to continuous pollution. It is also worth noting that the unique ARG subtypes in process F (n = 14) that escaped from chlorine disinfection were assigned to beta-lactam (n = 11), followed by macrolide-lincosamide-streptogramin (n = 2) and chloramphenicol (n = 1), respectively. Furthermore, the unique ARG subtypes in process G (n = 24) were assigned to aminoglycoside (n = 1), beta-lactam (n = 18), bleomycin (n = 1), macrolide-lincosamide-streptogramin (n = 2), multidrug (n = 1), and vancomycin (n = 1), respectively. Therefore, it is evident that the unique ARG subtypes in tap water rebounded when passed through the distribution system, which requires further attention. Shared ARG subtypes or unique ARG subtypes in the processes F and G pose a risk to human health when people drink the water. Additionally, these ARG subtypes might be transmitted to human living areas; for example, when people use water to wash food, such as fruit and vegetables, the ARGs will contaminate the fruit and vegetable surfaces and affect health through the food chain. Recently, scholars have discovered that ARGs in vegetables or fruit pose risks to ecological environment health (Sun et al., 2021). Furthermore, when people use the water to take a bath, the ARG subtypes might also remain on the skin (Nielsen and Jiang, 2019).
Antibiotic Resistance Genes Host Pattern in the Drinking Water Treatment System
As evident from Figure 4A, most of the ACCs were annotated as fragments of Proteobacteria (46.6%). Additionally, these contig-harbored ARGs were mainly resistant to bacitracin (26.08%), aminoglycoside (4.74%), multidrug (4.31%), and beta-lactam (3.45%). Similarly, the Proteobacteria phylum was identified as the most abundant bacterial host of ARGs (25.3–94.6%) in DWTPs (Zhao et al., 2022). Proteobacteria phylum was also regarded as a major pool of ARGs in sewage sludge (Xu et al., 2018), industrial mariculture systems (Wang et al., 2018), and the human gut (Hu et al., 2016). This is supported by the findings of our research. As illustrated in Figure 4B, before chlorine disinfection (processes A–E), the main putative ARG hosts were Acidovorax and Polynucleobacter, which were also the dominant bacteria in the raw water (Figure 2B and Supplementary Table 2). In the flocculation tank effluent, we found that Acidovorax and Stenotrophomonas were the main putative ARG hosts, while, in the sedimentation effluent, the main putative ARG carriers were Acidovorax, Polynucleobacter, and Stenotrophomonas. The main ARG carrier in the quartz sand sample was Pseudomonas, whereas Nitrospira, the predominant bacteria in the quartz sand biofilm, was not the dominant ARG host. Therefore, sand filters might be low risk for the transmission of ARGs. In the sand filter effluent, the main ARG carrier was Limnohabitans. Therefore, in general, we can see that Acidovorax and Stenotrophomonas were the most abundant ARG carriers of bacteria before chlorine disinfection.
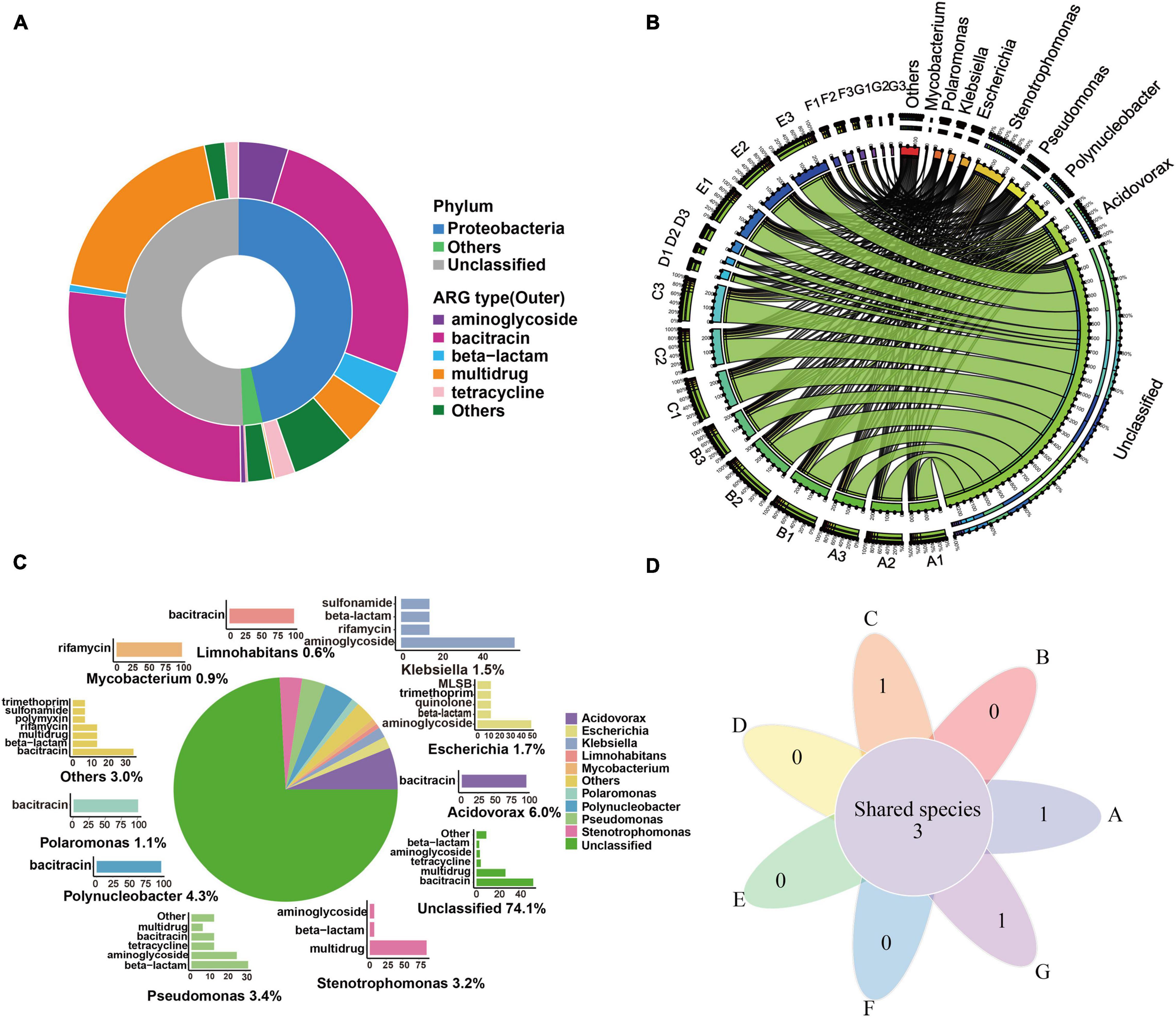
Figure 4. ARG hosts analysis in the DWTS. (A) Annotation of ARG-carrying contigs at the phylum level. (B) The circle diagram shows the taxonomy and proportion of putative ARG hosts in DWTS. (C) The taxonomy of ARG-carrying contigs (in the genus level) and the percentages of ARG types these contigs carried. a: Pie chart shows the taxonomy and percentage of ARG-carrying contigs. For example, Acidovorax (6.0%) denotes that 6.0% of ARG-carrying contigs were annotated as Acidovorax. b: Bar chart shows the percentages of ARGs types that were carried by the annotated ARG-carrying contigs. The percentage of these annotated ARG-carrying contigs was set as 100%. For example, 100% of the ARG-carrying contigs originating from Acidovorax spp. carried bacitracin resistance genes. (D) Venn diagrams showing the number of shared species that carried ARG subtypes in DWTS bulk water and sand biofilm. A1–A3: raw water; B1–B3: flocculation tank effluent; C1–C3: sedimentation tank effluent; D1–D3:quartz sand; E1–E3: sand filter effluent; F1–F3: chlorine-disinfected water; G1–G3: tap water. Three samples were taken from each sampling site.
However, after chlorine disinfection (processes F and G), the main ARG carriers were Stenotrophomonas and Escherichia. Interestingly, after the chlorine disinfection, the relative abundance of Stenotrophomonas also greatly increased and became the predominant bacteria (Figure 2B). Stenotrophomonas has been reported to be a highly chlorine-resistant Gram-negative bacterium that can regrow post disinfection (Shekhawat et al., 2020, 2021), and it is regarded as a global emerging Gram-negative multiple drug-resistant organism (Brooke, 2012). Similarly, Escherichia was widely reported to be an indicator of drug-resistant genes (Zhang et al., 2020e) and easily escaped the oxidation by disinfectants (Mounaouer and Abdennaceur, 2016). We assumed that these bacteria might regrow in the drinking water distribution system, or there was secondary pollution in the pipe network. Therefore, these bacteria are of high concern for public health safety in drinking water environments.
From all the water samples, as illustrated in Figure 4C, the dominant putative ARG hosts include Acidovorax (6.0%), Polynucleobacter (4.3%), Pseudomonas (3.4%), Escherichia (1.7%), Klebsiella (1.5%), Polaromonas (1.1%), Mycobacterium (0.9%), and Limnohabitans (0.6%). Acidovorax was the most abundant bacterium that carried bacitracin ARGs as Polynucleobacter, Polaromonas, and Limnohabitans. A previous study also reported that Acidovorax was the main carrier of bacitracin_bacA (Asante and Sekyere, 2019), and Polynucleobacter was an abundant ARG host in drinking water sources and frequently carried bacitracin ARGs (Bai et al., 2019). It was reported that the putative ARG host distribution pattern might be most likely responsible for the overall ARG profile (Bai et al., 2019). Therefore, these bacteria were most likely to drive the shift of bacitracin ARGs. Mycobacterium drove the shift of rifamycin ARGs, and Pseudomonas, Escherichia, and Klebsiella carried the largest variety of ARGs. According to the further analysis of ARG-carrying contigs (ACCs), we found that 49 ACCs were annotated as pathogens (Supplementary Material 4). They were mainly classified as Stenotrophomonas maltophilia (n = 15), P. aeruginosa (n = 12), E. coli (n = 7), Klebsiella pneumoniae (n = 6), Acinetobacter baumannii (n = 3), Rhodococcus erythropolis (n = 2), Pseudomonas putida (n = 2), Mycobacterium smegmatis (n = 1), and Enterobacter aerogenes (n = 1). Thus, we can see that the most common pathogens were S. maltophilia and P. aeruginosa in the DWTS. Moreover, Escherichia spp. mainly carried tetracycline and sulfonamide ARGs (Figure 4C), while Stenotrophomonas spp. mainly carried aminoglycoside, beta-lactam, and multidrug ARGs (Figure 4C). Additionally, pathogens were distributed in processes A (n = 41), B (n = 40), C (n = 40), D (n = 12), E (n = 41), F (n = 26), and G (n = 20). In particular, S. maltophilia was persistent in every process (Figure 4D and Supplementary Material 5), and the sustained survival of these bacteria in the DWTS could lead to the horizontal propagation of ARGs and even increase risks to human health.
Distribution of Antibiotic Resistance Genes in Mobile Elements
As illustrated in Figure 5A, ARGs present in the DWTS were more prevalent in bacterial chromosomes than in plasmids. Specifically, ARGs for bacitracin, rifamycin, vancomycin, quinolone, polymyxin, kasugamycin, and fosmidomycin were just prevalent in chromosomes. These ARGs might not contribute to horizontal gene transfer. While ARGs for multidrug, beta-lactam, aminoglycoside, tetracycline, sulfonamide, trimethoprim, and chloramphenicol were prevalent mainly in plasmids. Significantly, chloramphenicol was only presented in plasmids. Therefore, we must focus on these kinds of ARGs with a higher risk of transmission. Additionally, the phylum of Proteobacteria harbored the highest abundance of plasmids (Figure 5B), followed by Firmicutes. Meanwhile, the abundance of plasmids was higher than that of insert sequence (IS) and integron (Figure 5C). The most abundant MGE plasmids appeared in the sand filter effluent, followed by the flocculation tank effluent.
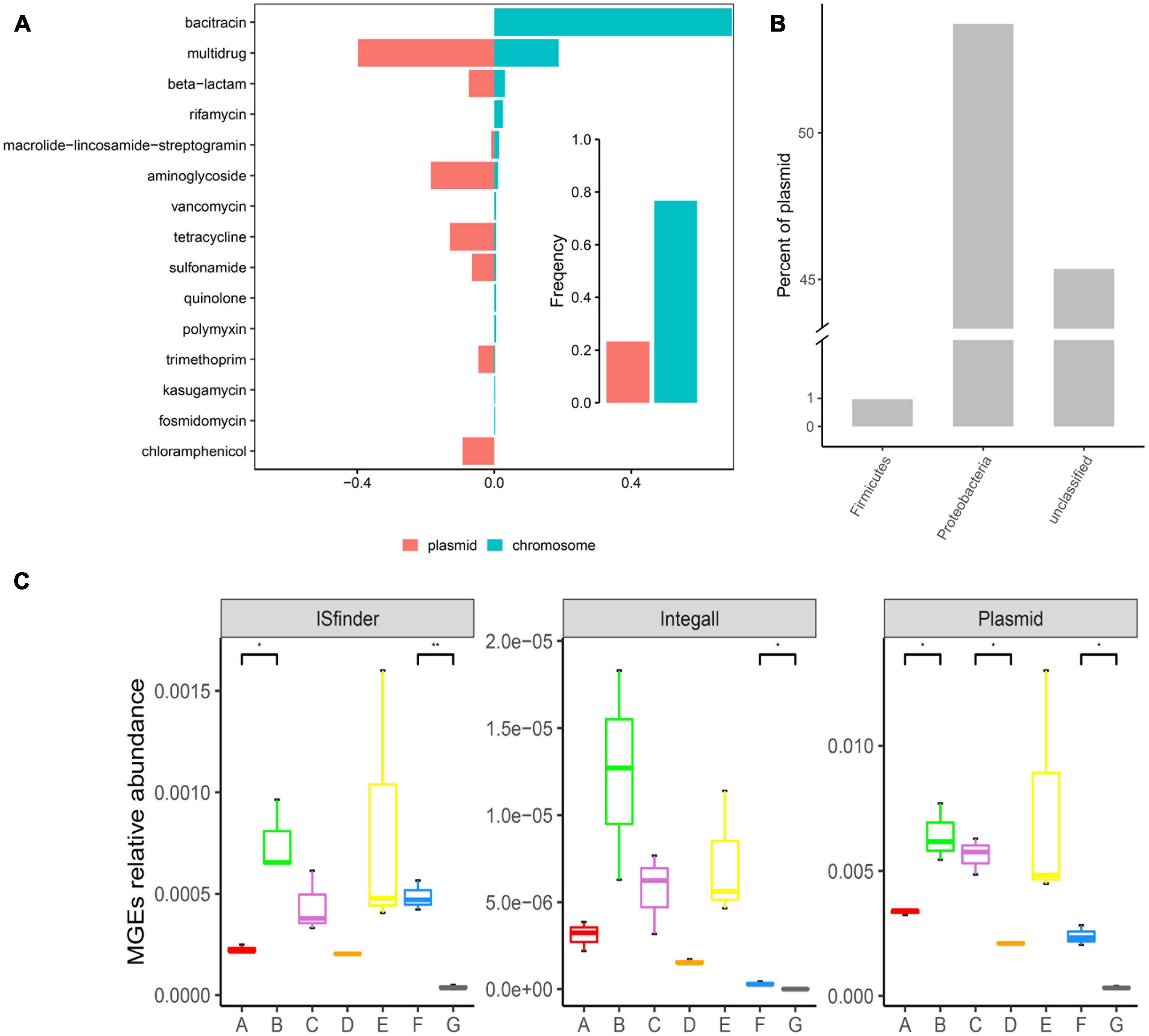
Figure 5. Distribution of ARGs in mobile genetic elements (MGEs) and distribution of MGEs in the DWTS. (A) Occurrence and abundance of ARG types in MGEs. Bar plot showing the frequency of ARGs in bacterial chromosomes (blue) or plasmids (red) summarized by antibiotic class. (B) Distribution of plasmids in bacteria (at the phylum level). (C) Distribution of MGEs in the DWTS. A: raw water; B: grid reaction tank effluent; C: settling pond effluent; D: quartz sand; E: sand filter effluent; F: chlorine-disinfected water; G: tap water.
However, in the quartz sand biofilm, the abundance of MGE plasmids was the lowest, and the distribution trend of MGE ISfinder in drinking water treatment processes was similar to the MGE plasmids. Interestingly, ARG abundance in the quartz sand biofilm was also the lowest (Supplementary Figure 2). At the same time, the Shannon index indicated that microbial diversity was the most abundant in the quartz sand biofilm. These results demonstrate that the probability of horizontal transmission of ARGs was the least in the quartz sand filters and the highest in the flocculation tank effluent. Integron class1 is a major player in disseminating antibiotic resistance genes across pathogens and commensals. Therefore, in this study, we also looked for integron class1 and found a higher prevalence in the DWTS; the abundance of integron class1 in the flocculation tank effluent water was the highest than the other samples (Supplementary Figure 3). Additionally, it decreased after chlorine disinfection and significantly decreased in the process of drinking water transmission, as well as MGE plasmids and MGE ISfinder. Plasmids and integrons are prone to recombination (Mazel, 2006); thus, chlorine disinfection and residual chlorine in the distribution system could prevent the dissemination of ARGs.
Co-occurrence Patterns Between Antibiotic Resistance Gene Subtypes
A network analysis based on the metagenomic approach was used to discern the co-occurrence patterns between ARG subtypes and demonstrated a good advantage (Ju et al., 2016; Zhang et al., 2020a). As illustrated in Figure 6 and Supplementary Table 3, a total of 90 pairs of ARG subtypes under a cutoff of p < 0.01 and ρ > 0.8 were discovered from 29 ARG subtypes assigned to 12 ARG types. ARG subtypes assigned to the same ARG type (i.e., intra-types) tend to be co-occurring. Overall, ten out of the 90 pairs were intra-type co-occurrence correlations. For instance, emrE and qacEdelta1 for multidrug resistance correlated (r = 0.85), as well as the sul1 and sul2 (r = 0.93) for sulfonamide resistance and tetA and tetG (r = 0.88; Supplementary Table 3) for tetracycline resistance. Co-occurrence patterns were also observed between different ARGs subtypes (i.e., inter-types). For example, aac (3)-I for aminoglycoside resistance strongly correlated with ksgA (r = 1) for kasugamycin resistance, bacA for bacitracin resistance correlated with sul1 for sulfonamide resistance (r = 0.96), floR for chloramphenicol resistance correlated with tetG for tetracycline resistance (0.95), and qacEdelta1 for multidrug resistance correlated with sul1 for sulfonamide resistance (0.93). Recent research also addressed the significant positive correlations among these ARG types and subtypes (Zhang S. et al., 2018; Zhang et al., 2020a).
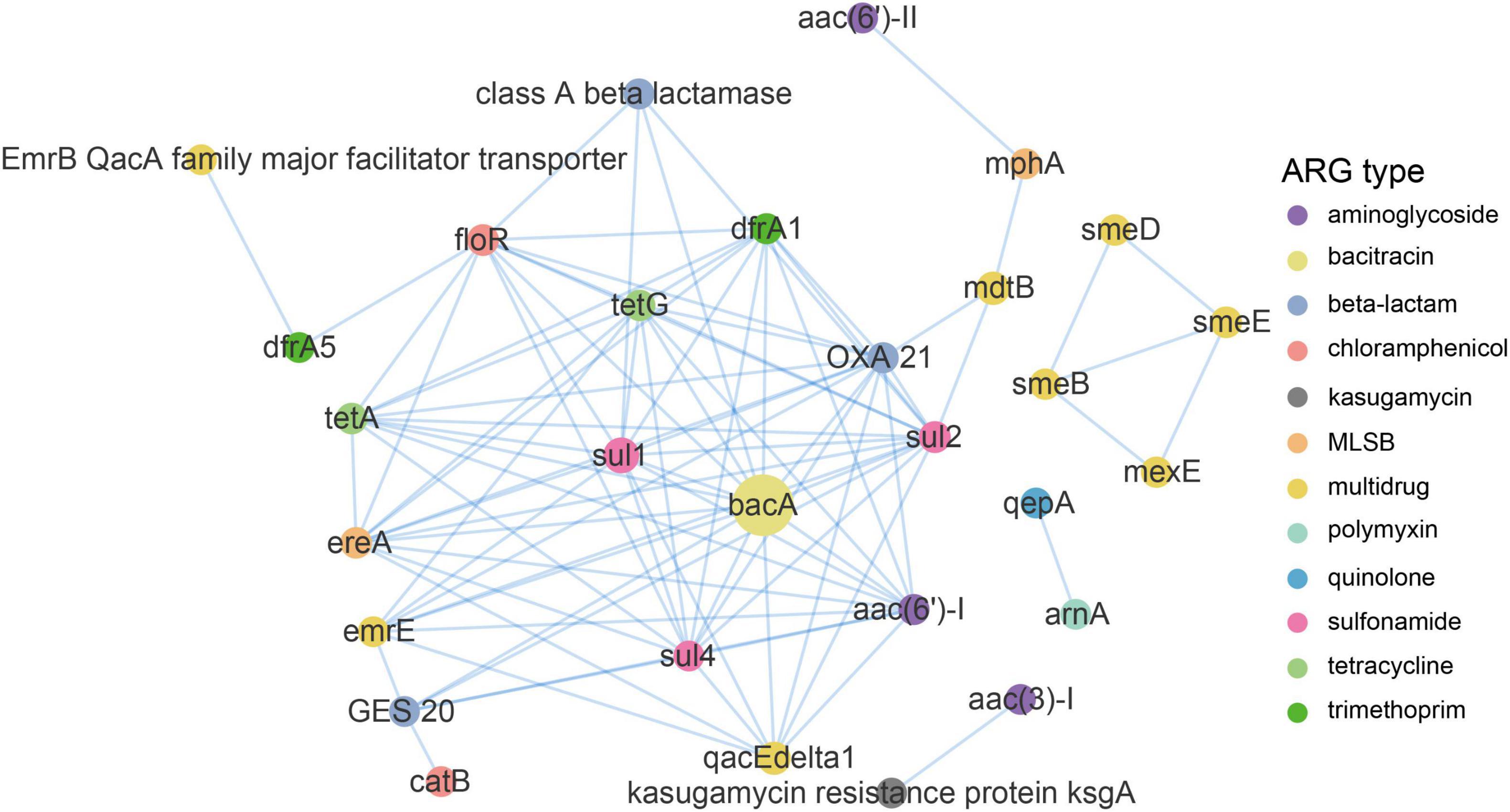
Figure 6. Network analysis demonstrating the co-occurrence patterns of ARG subtypes in the DWTS. The nodes were colored according to network modularity, and the node size is proportional to the number of connections (degree). An edge is a strong (ρ > 0.8) and significant (p-value <0.01) connection between nodes.
Co-occurrence Patterns Among Antibiotic Resistance Genes Subtypes, Mobile Genetic Elements, and Bacterial Taxa
A network analysis was performed to explore the co-occurrence patterns among ARG subtypes, MGEs, and bacterial genera. Co-occurrence patterns between ARG subtypes and bacterial genera could be a good way to track the potential hosts of ARGs in their environment. We hypothesized that the ARGs and bacterial taxa had a strong and significant positive correlation under a cutoff of p < 0.01 and ρ > 0.8. As illustrated in Supplementary Figure 4 and Supplementary Material 6, the co-occurrence results indicated that 42 genera might be potential hosts for 27 ARG subtypes. Among the 42 bacterial genera, Acidovorax, Curvibacter, Variovorax, Comamonas, and Hydrogenophaga carried more diverse ARG subtypes (14, 14, 13, 12, and 12, respectively) than other genera. For example, Acidovorax was identified as the possible host of the multidrug-resistance gene (emrE and qacEdeltal), sulfonamide-resistance gene (e.g., sul1 and sul2), tetracycline-resistance gene (tetA and tetG), trimethoprim-resistance gene (dfrA1), beta-lactam-resistance gene (OXA-21 and class-A-beta-lactamase), chloramphenicol-resistance gene (floR), bacitracin-resistance gene (bacA), macrolide-lincosamide-streptogramin-resistance gene (ereA), and aminoglycoside-resistance gene [aac(6_)-I] (Supplementary Material 6). Likewise, Curvibacter was the possible host of the bacitracin-resistance gene (bacA), beta-lactam-resistance gene (OXA-21 and class-A-beta-lactamase), multidrug-resistance gene (emrE and qacEdeltal), sulfonamide-resistance genes (e.g., sul1 and sul2), tetracycline-resistance gene (tetA and tetG), and trimethoprim-resistance gene (dfrA1).
Additionally, a total of 57 pairs with p < 0.01 and ρ > 0.8 were observed between the MGEs and bacterial genera. For instance, intI1 had a significant and positive correlation with ten bacterial genera (Supplementary Material 6). IS21 correlated positively and significantly with 15 bacterial genera, and IS3 had a strong positive collection with 10 bacterial genera. Furthermore, a total of 31 pairs with p < 0.01 and ρ > 0.8 were observed between the MGE and ARG subtypes. IntI1 had a significantly positive correlation with eight ARG subtypes, and IS21 had a significantly positive correlation with nine ARG subtypes. These results implied the important role of IntI1 and IS21 in antibiotic resistance dissemination. It has been reported that MGE-associated ARGs tend to persist in the treatment at DWTPs and might pose a higher risk of resistance dispersal (Zhao et al., 2022). Similarly, recent literature has also reported the increase in the proportion of MGE-associated ARGs in wastewater treatment plants (Che et al., 2019).
Bins
In this study, after metagenomic binning, 33 high-quality metagenome-assembled genomes (MAGs) were obtained, which mainly included Gammaproteobacteria (n = 13), Alphaproteobacteria (n = 5), Betaproteobacteria (n = 2), Planctomycetota (n = 2), Bacteroidota (n = 1), Actinobacteriota (n = 2), and Nitrospirota (n = 1) (Figure 7). The general characteristics of these 33 MAGs are illustrated in Supplementary Table 4. Of the 33 MAGs, the phylum Proteobacteria (n = 20) dominated the microbial community in the DWTS, followed by Planctomycetota (n = 2) and Actinobacteriota (n = 2). The MAG identified as S. maltophilia-like (bin.195) harbored the greatest number of ARG subtypes (n = 8), including multidrug (n = 6; smeD, semE, multidrug_transporter, mexE, semB, and smeC), beta-lactam (n = 1; metallo-beta-lactamase), and aminoglycoside [n = 1; aph(3’)-IIb] (Supplementary Table 5). Meanwhile, Burkholderiaceae-like (bin.379) also contained bacitracin (n = 1; bacA). Additionally, the MAG (bin.84) identified as R. erythropolis-like contained aminoglycoside [n = 2; ant (3”)-Ih-aac (6’)-Iid and ant (2”)-I] and rifamycin (n = 1; rifampin monooxygenase). Interestingly, based on our genome data of strain F2 identified to the genus of Stenotrophomonas sp., which also harbored smeD, semE, multidrug_transporter, semB, and smeC (Supplementary Material 7 and Supplementary Figure 5), these results can be verified against each other.
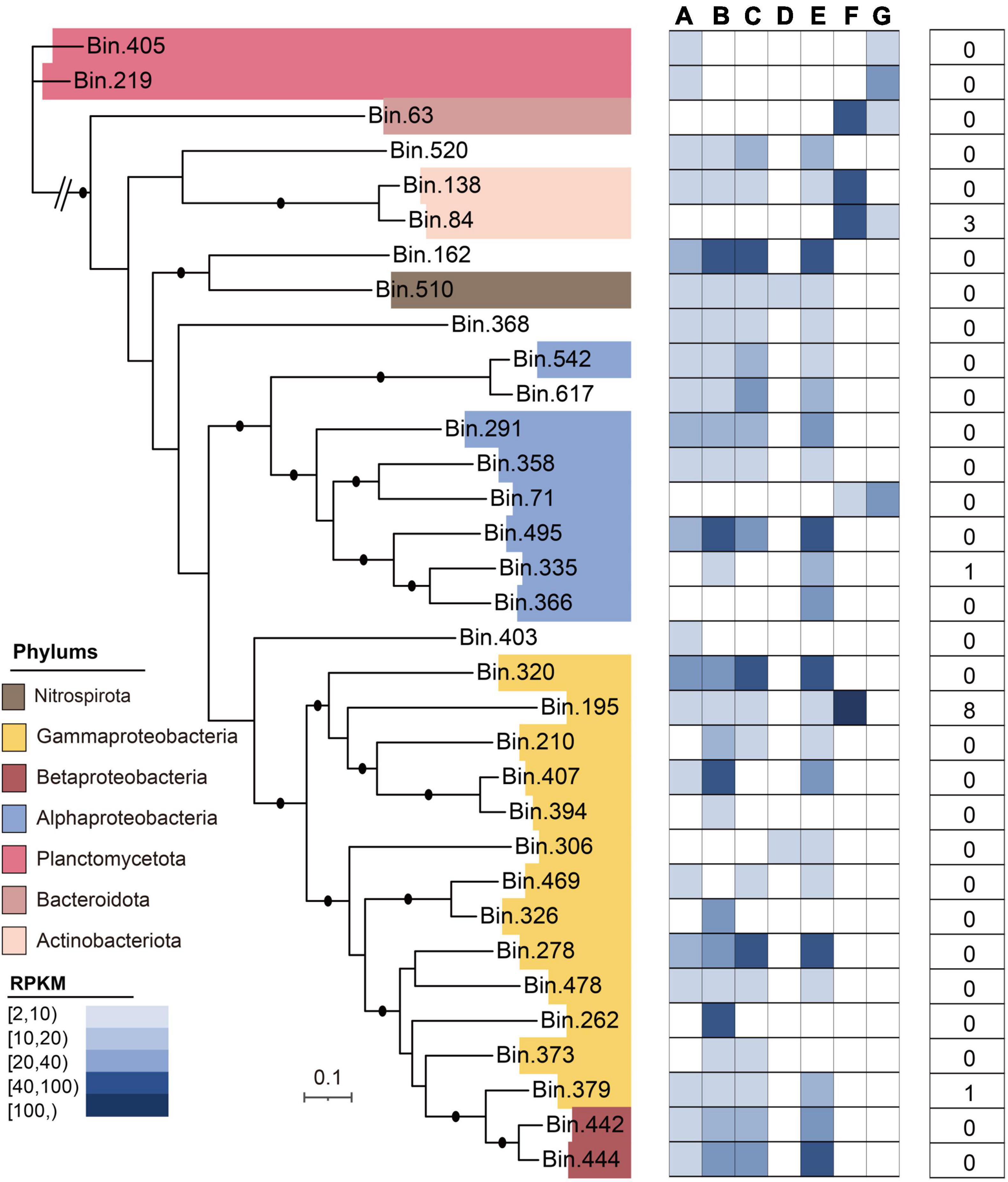
Figure 7. Taxonomic assignment of 33 MAGs reconstructed from the DWTS. The phylogenomic tree was constructed using 31 commonly conserved protein-coding genes. The average relative abundances [in reads per kilobase per million (RPKM)] of all MAGs in drinking water production processes are shown as a heat map, followed by data indicating the presence of the total ARG subtype numbers in MAGs.
Isolation and Identification of Bacteria From the Drinking Water Treatment System
A total of 93 isolates were recovered from the DWTS using traditional culture technology (Supplementary Tables 6, 7). The isolates belong to 30 genera based on a 16S rRNA gene sequencing analysis, and they were found to belong to five phyla: Proteobacteria, Firmicutes, Actinobacteria, Deinococcus-Thermus, and Bacterioidetes. Proteobacteria (42%) and Firmicutes (38%) were the dominant phyla. As illustrated in Supplementary Table 7, at the genus level, Bacillus was the most abundant genus (20%), followed by Pseudomonas (11%) and Acidovorax (9%), respectively. Moreover, 47 isolates within 20 genera were recovered from the sand filter, representing the maximum microbial diversity in all the sampling sites. Bacteria isolated from this process accounted for 51% of all the isolates, meaning that bacteria in the sand filter were the most abundant. These results were consistent with the microbial diversity analysis based on the metagenomic data in this study.
It is worth noting that some pathogens and opportunistic pathogens such as P. aeruginosa, Bacillus cereus, Staphylococcus hominis, E. coli, and Aeromonas enteropelogenes were detected in water treatment processes. Fortunately, only one bacterium was detected after chlorination, and it was not a pathogen or opportunistic pathogen, which indicated that chlorination effectively removed high-risk bacteria in the DWST. However, it does not demonstrate that the tap water was completely microbial safe. In our study, five isolates were obtained from the tap water, indicating secondary pollution in the drinking water pipe network. Previous literature has reported that disinfectant could induce culturable bacteria and various pathogenic bacteria into a viable but non-culturable (VBNC) state (Lin et al., 2017) in the DWTS, which would result in these bacteria not being detected using traditional culture methods. Therefore, a comprehensive microbiological safety assessment of the drinking water could combine molecular methods with culture-based methods.
Antimicrobial Susceptibility Testing
The antimicrobial susceptibility testing results (Table 2) demonstrated that selected isolates had high resistance to FOX (56%), 48% of the selected isolates were resistant to P, and 28% were resistant to AMC. However, the resistance to TOB, DA, and E was lower (4, 8, and 8%, respectively). In the Proteobacteria, 88% of the isolates were resistant to AMC and 63% were resistant to AMP. However, the incidence of resistance to TE, TOB, CIP, CAZ, and MEM was very low. In the Firmicutes, 71% of the isolates were resistant to FOX, 59% to P, and only 12% to TE, DA, and E. Bacteria were regarded as multidrug resistant (MDR) if they were resistant to three or more groups of antibiotics. In this study, 28% of the selected isolates belong to MDR, and the occurrence of MDR phenotypes was mainly related to the bacterial species. The MARI was applied to verify the origin of the antibiotic resistance in the isolates. The MARI value of the selected isolates recovered from the DWTS was 0.09–0.50. Compared to other environments, the MARI value of the isolates that recovered from the DWTS was lower. For example, the MARI value of Salmonella enterica that recovered from broiler chickens and retail shops was 0.2–0.6 (Elkenany et al., 2019), and E. coli that isolated from clinical specimens, including eye and blood cells, had higher MARI values of 0.82 and 0.74, respectively (Masoud et al., 2021). Additionally, according to the MARI value, the B3, B11, D38, D20, and F2 isolates might be from a source where antibiotics were used frequently.
Conclusion
In this study, metagenomic approaches were applied to comprehensively profile the pattern of the microbial community structure, ARGs, and MGEs in the DWTS. The results indicated that Proteobacteria, Acidobacteria, Bacteroidetes, and Firmicutes were the dominant phyla in the DWTS and shifted largely during the water treatment process. Additionally, gene-encoded bacitracin (0.3 × 10–2 to 0.40 copies/cell), multidrug (0.02–0.23 copies/cell), and sulfonamide (0.2 × 10–3 to 0.7 × 10–1 copies/cell) were detected at the highest levels of abundance before chlorination, while the aminoglycoside, beta-lactam, and rifamycin ARGs were notably enriched after chlorination. Among the drinking water treatment processes, chlorination profoundly influenced the ARG composition. A total of 317 ARG subtypes were annotated, and bacitracin_bacA (0.1–0.5 copies/cell) was generally the most prevalent subtype in all samples. The taxonomy results demonstrated that the dominant putative ARG hosts included Acidovorax (6.0%), Polynucleobacter (4.3%), Pseudomonas (3.4%), Escherichia (1.7%), and Klebsiella (1.5%). A further analysis indicated that 49 ACCs were annotated as pathogens. The most common pathogens were S. maltophilia and P. aeruginosa in the DWTS. Additionally, the network analyses suggested that 42 genera were deduced as the potential hosts of the 27 ARG subtypes. The metagenomic binning results demonstrated that 33 high-quality MAGs were recovered from the DWTS and bin.195 harbored the greatest number of ARG subtypes (n = 8), including multidrug (n = 6; smeD, semE, multidrug_transporter, mexE, semB, and smeC), beta-lactam (n = 1; metallo-beta-lactamase), and aminoglycoside [n = 1; aph(3’)-IIb]. In total, ninety-three isolates were obtained from the DWTS, and 28% of the selected isolates were MDR. Additionally, some pathogens and opportunistic pathogens such as P. aeruginosa, B. cereus, S. hominis, E. coli, and A. enteropelogenes were isolated from the DWTS. The occurrence of these pathogenic bacteria demonstrates that higher requirements for disinfection technology are necessary and might threaten the safety of drinking water. Our findings provide comprehensive insights into the occurrence and dissemination of ARGs and ARB in the DWTS.
Data Availability Statement
Raw data have been submitted to the NCBI Sequence Read Archive (SRA) under accession numbers SRR17063149-17063169.
Author Contributions
QW and QG conceived and designed the experiments. QG, TL, and MS performed the experiments. QG, SW, SZ, YZ, RP, and XW analyzed the data. QW, YD, JW, ZL, LC, WC, XL, and JZ contributed reagents, materials, and analysis tools. QG wrote the manuscript. MC, LX, and QG reviewed the manuscript. All authors contributed to the article and approved the submitted version.
Funding
This work was supported by the National Natural Science Foundation of China for Youth (31901663), the China Postdoctoral Science Foundation (2019M662829), the Guangdong Pearl River Talent Plan local innovation team (2017BT01S174), the Key Laboratory of Guangdong Province (2020B121201009), and the GDAS Project of Science and Technology Development (2019GDASYL-0103008).
Conflict of Interest
The authors declare that the research was conducted in the absence of any commercial or financial relationships that could be construed as a potential conflict of interest.
Publisher’s Note
All claims expressed in this article are solely those of the authors and do not necessarily represent those of their affiliated organizations, or those of the publisher, the editors and the reviewers. Any product that may be evaluated in this article, or claim that may be made by its manufacturer, is not guaranteed or endorsed by the publisher.
Supplementary Material
The Supplementary Material for this article can be found online at: https://www.frontiersin.org/articles/10.3389/fmicb.2021.798442/full#supplementary-material
Footnotes
- ^ https://www.ncbi.nlm.nih.gov/
- ^ https://github.com/hyattpd/Prodigal
- ^ https://www.nature.com/articles/s41467-018-04964-5
- ^ http://sourceforge.net/projects/bbmap/
References
Amarasiri, M., Sano, D., and Suzuki, S. (2020). Understanding human health risks caused by antibiotic resistant bacteria (ARB) and antibiotic resistance genes (ARG) in water environments: current knowledge and questions to be answered. Crit. Rev. Environ. Sci. Technol. 50, 2016–2059. doi: 10.1080/10643389.2019.1692611
Asante, J., and Sekyere, J. O. (2019). Understanding antimicrobial discovery and resistance from a metagenomic and metatranscriptomic perspective: advances and applications. Environ. Microbiol. Rep. 11, 62–86. doi: 10.1111/1758-2229.12735
Bai, Y., Ruan, X. H., Li, R. F., Zhang, Y. P., and Wang, Z. Z. (2021). Metagenomics-based antibiotic resistance genes diversity and prevalence risk revealed by pathogenic bacterial host in Taihu Lake, China. Environ. Geochem. Health doi: 10.1007/s10653-021-01021-x
Bai, Y., Ruan, X., Xie, X., and Yan, Z. (2019). Antibiotic resistome profile based on metagenomics in raw surface drinking water source and the influence of environmental factor: a case study in Huaihe River Basin, China. Environ. Pollut. 248, 438–447. doi: 10.1016/j.envpol.2019.02.057
Bergeron, S., Boopathy, R., Nathaniel, R., Corbin, A., and Lafleur, G. (2015). Presence of antibiotic resistant bacteria and antibiotic resistance genes in raw source water and treated drinking water. Int. Biodeterior. Biodegrad. 102, 370–374. doi: 10.1016/j.ibiod.2015.04.017
Bergeron, S., Raj, B., Nathaniel, R., Corbin, A., and Lafleur, G. (2017). Presence of of antibiotic resistance genes in raw source water of a drinking water treatment plant in a rural community of USA. Int. Biodeterior. Biodegrad. 124, 3–9. doi: 10.1016/j.ibiod.2017.05.024
Brooke, J. S. (2012). Stenotrophomonas maltophilia: an emerging global opportunistic pathogen. Clin. Microbiol. Rev. 25, 2–41. doi: 10.1128/CMR.00019-11
Camacho, C., Coulouris, G., Avagyan, V., Ma, N., Papadopoulos, J., Bealer, K., et al. (2009). BLAST plus : architecture and applications. BMC Bioinform. 10:421. doi: 10.1186/1471-2105-10-421
Che, Y., Xia, Y., Liu, L., Li, A. D., Yang, Y., and Zhang, T. (2019). Mobile antibiotic resistome in wastewater treatment plants revealed by Nanopore metagenomic sequencing. Microbiome 7:44. doi: 10.1186/s40168-019-0663-0
Chen, Y.-R., Guo, X.-P., Feng, J.-N., Lu, D.-P., Niu, Z.-S., Tou, F.-Y., et al. (2019). Impact of ZnO nanoparticles on the antibiotic resistance genes (ARGs) in estuarine water: ARG variations and their association with the microbial community. Environ. Sci. Nano 6, 2405–2419. doi: 10.1039/C9EN00338J
CLSI (2020). “Performance Standards for Antimicrobial Susceptibility Testing; Twenty-Fifth Informational Supplement. Approved Standard-M02-A12”. Malvern, PA: The Clinical and Laboratory Standards Institute.
Douterelo, I., Husband, S., Loza, V., and Boxall, J. (2016). Dynamics of biofilm regrowth in drinking water distribution systems. Appl. Environ. Microbiol. 82, 4155–4168. doi: 10.1128/AEM.00109-16
Elkenany, R., Elsayed, M. M., Zakaria, A. I., El-Sayed, S. A., and Rizk, M. A. (2019). Antimicrobial resistance profiles and virulence genotyping of Salmonella enterica serovars recovered from broiler chickens and chicken carcasses in Egypt. BMC Vet. Res. 15:1. doi: 10.1186/s12917-019-1867-z
Fang, H., Wang, H., Cai, L., and Yu, Y. (2015). Prevalence of antibiotic resistance genes and bacterial pathogens in long-term manured greenhouse soils as revealed by metagenomic survey. Environ. Sci. Technol. 49, 1095–1104. doi: 10.1021/es504157v
Gil-Gil, T., Martinez, J. L., and Blanco, P. (2020). Mechanisms of antimicrobial resistance in Stenotrophomonas maltophilia: a review of current knowledge. Exp. Rev. Anti Infect. Therapy 18, 335–347. doi: 10.1080/14787210.2020.1730178
Gu, Q., Wu, Q., Zhang, J., Guo, W., Ding, Y., Wang, J., et al. (2018). Isolation and transcriptome analysis of phenol-degrading bacterium from carbon-sand filters in a full-scale drinking water treatment plant. Front. Microbiol. 9:2162. doi: 10.3389/fmicb.2018.02162
Han, Z., An, W., Yang, M., and Zhang, Y. (2020). Assessing the impact of source water on tap water bacterial communities in 46 drinking water supply systems in China. Water Res. 172:115469. doi: 10.1016/j.watres.2020.115469
Hou, L., Zhou, Q., Wu, Q., Gu, Q., Sun, M., and Zhang, J. (2018). Spatiotemporal changes in bacterial community and microbial activity in a full-scale drinking water treatment plant. Sci. Total Environ. 625, 449–459. doi: 10.1016/j.scitotenv.2017.12.301
Hu, Y., Yang, X., Li, J., Lv, N., Liu, F., Wu, J., et al. (2016). The bacterial mobile resistome transfer network connecting the animal and human microbiomes. Appl. Environ. Microbiol. 82, 6672–6681. doi: 10.1128/AEM.01802-16
Ishii, S. I., Suzuki, S., Norden-Krichmar, T. M., Tenney, A., Chain, P. S. G., Scholz, M. B., et al. (2013). A novel metatranscriptomic approach to identify gene expression dynamics during extracellular electron transfer. Nat. Commun. 4:1601. doi: 10.1038/ncomms2615
Jia, S., Shi, P., Hu, Q., Li, B., Zhang, T., and Zhang, X.-X. (2015). Bacterial community shift drives antibiotic resistance promotion during drinking water chlorination. Environ. Sci. Technol. 49, 12271–12279. doi: 10.1021/acs.est.5b03521
Jia, S., Wu, J., Ye, L., Zhao, F., Li, T., and Zhang, X.-X. (2019). Metagenomic assembly provides a deep insight into the antibiotic resistome alteration induced by drinking water chlorination and its correlations with bacterial host changes. J. Haz. Mater. 379:120841. doi: 10.1016/j.jhazmat.2019.120841
Jiang, H., Zhou, R., Zhang, M., Cheng, Z., Li, J., Zhang, G., et al. (2018). Exploring the differences of antibiotic resistance genes profiles between river surface water and sediments using metagenomic approach. Ecotoxicol. Environ. Saf. 161, 64–69. doi: 10.1016/j.ecoenv.2018.05.044
Ju, F., Li, B., Ma, L., Wang, Y., Huang, D., and Zhang, T. (2016). Antibiotic resistance genes and human bacterial pathogens: co-occurrence, removal, and enrichment in municipal sewage sludge digesters. Water Res. 91, 1–10. doi: 10.1016/j.watres.2015.11.071
Katoh, K., and Standley, D. M. (2013). MAFFT multiple sequence alignment software version 7: improvements in performance and usability. Mol. Biol. Evol. 30, 772–780. doi: 10.1093/molbev/mst010
Khan, S., Beattie, T. K., and Knapp, C. W. (2016). Relationship between antibiotic- and disinfectant-resistance profiles in bacteria harvested from tap water. Chemosphere 152, 132–141. doi: 10.1016/j.chemosphere.2016.02.086
Kumar, M., Ram, B., Honda, R., Poopipattana, C., Vu Duc, C., Chaminda, T., et al. (2019). Concurrence of antibiotic resistant bacteria (ARB), viruses, pharmaceuticals and personal care products (PPCPs) in ambient waters of Guwahati, India: Urban vulnerability and resilience perspective. Sci. Total Environ. 693:133640. doi: 10.1016/j.scitotenv.2019.133640
Labella, A., Molero, R., Leiva-Rebollo, R., Perez-Recuerda, R., and Borrego, J. J. (2021). Identification, resistance to antibiotics and biofilm formation of bacterial strains isolated from a reverse osmosis system of a drinking water treatment plant. Sci. Total Environ. 774:145718. doi: 10.1016/j.scitotenv.2021.145718
Li, B., Ju, F., Cai, L., and Zhang, T. (2015). Profile and fate of bacterial pathogens in sewage treatment plants revealed by high-throughput metagenomic approach. Environ. Sci. Technol. 49, 10492–10502. doi: 10.1021/acs.est.5b02345
Li, W. Y., Tan, Q. W., Zhou, W., Chen, J. P., Li, Y., Wang, F., et al. (2020). Impact of substrate material and chlorine/chloramine on the composition and function of a young biofilm microbial community as revealed by high-throughput 16S rRNA sequencing. Chemosphere 242:10. doi: 10.1016/j.chemosphere.2019.125310
Lin, H., Ye, C., Chen, S., Zhang, S., and Yu, X. (2017). Viable but non-culturable E. coli induced by low level chlorination have higher persistence to antibiotics than their culturable counterparts. Environ. Pollut. 230, 242–249. doi: 10.1016/j.envpol.2017.06.047
Liu, S.-S., Qu, H.-M., Yang, D., Hu, H., Liu, W.-L., Qiu, Z.-G., et al. (2018). Chlorine disinfection increases both intracellular and extracellular antibiotic resistance genes in a full-scale wastewater treatment plant. Water Res. 136, 131–136. doi: 10.1016/j.watres.2018.02.036
Luo, L.-W., Wu, Y.-H., Yu, T., Wang, Y.-H., Chen, G.-Q., Tong, X., et al. (2021). Evaluating method and potential risks of chlorine-resistant bacteria (CRB): a review. Water Res. 188:116474. doi: 10.1016/j.watres.2020.116474
Ma, L., Li, B., and Zhang, T. (2019). New insights into antibiotic resistome in drinking water and management perspectives: a metagenomic based study of small-sized microbes. Water Res. 152, 191–201. doi: 10.1016/j.watres.2018.12.069
Ma, L., Li, B., Jiang, X.-T., Wang, Y.-L., Xia, Y., Li, A.-D., et al. (2017). Catalogue of antibiotic resistome and host-tracking in drinking water deciphered by a large scale survey. Microbiome 5:154. doi: 10.1186/s40168-017-0369-0
Mao, Y., Xia, Y., Wang, Z., and Zhang, T. (2014). Reconstructing a thauera genome from a hydrogenotrophic-denitrifying consortium using metagenomic sequence data. Appl. Microbiol. Biotechnol. 98, 6885–6895. doi: 10.1007/s00253-014-5756-x
Masoud, S. M., Abd El-Baky, R. M., Aly, S. A., and Ibrahem, R. A. (2021). Co-Existence of certain ESBLs, MBLs and plasmid mediated quinolone resistance genes among MDR E. coli isolated from different clinical specimens in egypt. Antibiot. Basel 10:835. doi: 10.3390/antibiotics10070835
Massa, S., Caruso, M., Trovatelli, F., and Tosques, M. (1998). Comparison of plate count agar and R2A medium for enumeration of heterotrophic bacteria in natural mineral water. World J. Microbiol. Biotechnol. 14, 727–730. doi: 10.1023/A:1008893627877
Mas-Ud, M. A., Ali, R., and Hasan, Z. (2020). Molecular detection and biological control of human hair dandruff causing microorganism Staphylococcus aureus. J. Pure Appl. Microbiol. 14, 147–156. doi: 10.22207/JPAM.14.1.16
Mazel, D. (2006). Integrons: agents of bacterial evolution. Nat. Rev. Microbiol. 4, 608–620. doi: 10.1038/nrmicro1462
Mombini, S., Rezatofighi, S. E., Kiyani, L., and Motamedi, H. (2019). Diversity and metallo-beta-lactamase-producing genes in Pseudomonas aeruginosa strains isolated from filters of household water treatment systems. J. Environ. Manag. 231, 413–418. doi: 10.1016/j.jenvman.2018.10.068
Mounaouer, B., and Abdennaceur, H. (2016). Modeling and kinetic characterization of wastewater disinfection using chlorine and UV irradiation. Environ. Sci. Pollut. Res. 23, 19861–19875. doi: 10.1007/s11356-016-7173-4
Nielsen, M. C., and Jiang, S. C. (2019). Alterations of the human skin microbiome after ocean water exposure. Mar. Pollut. Bull. 145, 595–603. doi: 10.1016/j.marpolbul.2019.06.047
Oh, S., Hammes, F., and Liu, W. T. (2018). Metagenomic characterization of biofilter microbial communities in a full-scale drinking water treatment plant. Water Res. 128, 278–285. doi: 10.1016/j.watres.2017.10.054
Perez-Vidal, A., Patricia Rivera-Sanchez, S., Janeth Florez-Elvira, L., Antonio Silva-Leal, J., Diaz-Gomez, J., Fernanda Herrera-Cuero, L., et al. (2019). Removal of E. coli and Salmonella in pot ceramic filters operating at different filtration rates. Water Res. 159, 358–364. doi: 10.1016/j.watres.2019.05.028
Poghosyan, L., Koch, H., Frank, J., Van Kessel, M. a. H. J., Cremers, G., Van Alen, T., et al. (2020). Metagenomic profiling of ammonia- and methane-oxidizing microorganisms in two sequential rapid sand filters. Water Res. 185:116288. doi: 10.1016/j.watres.2020.116288
Potgieter, S. C., Dai, Z., Venter, S. N., Sigudu, M., and Pinto, A. J. (2020). Microbial nitrogen metabolism in chloraminated drinking water reservoirs. Msphere 5:2. doi: 10.1128/mSphere.00274-20
Pruden, A., Pei, R., Storteboom, H., and Carlson, K. H. (2006). Antibiotic resistance genes as emerging contaminants: studies in northern colorado. Environ. Sci. Technol. 40, 7445–7450. doi: 10.1021/es060413l
Pu, Q., Zhao, L.-X., Li, Y.-T., and Su, J.-Q. (2020). Manure fertilization increase antibiotic resistance in soils from typical greenhouse vegetable production bases, China. J. Hazard. Mater. 391:122267. doi: 10.1016/j.jhazmat.2020.122267
Qian, X., Gu, J., Sun, W., Wang, X.-J., Su, J.-Q., and Stedfeld, R. (2018). Diversity, abundance, and persistence of antibiotic resistance genes in various types of animal manure following industrial composting. J. Hazard. Mater. 344, 716–722. doi: 10.1016/j.jhazmat.2017.11.020
Shekhawat, S. S., Gupta, A. B., Kulshreshtha, N. M., and Prakash, R. (2021). UV disinfection studies on chlorine tolerant bacteria recovered from treated sewage. J. Environ. Chem. Eng. 9:105253. doi: 10.1016/j.jece.2021.105253
Shekhawat, S. S., Kulshreshtha, N. M., and Gupta, A. B. (2020). Investigation of chlorine tolerance profile of dominant gram negative bacteria recovered from secondary treated wastewater in Jaipur, India. J. Environ. Manag. 255:109827. doi: 10.1016/j.jenvman.2019.109827
Su, H.-C., Liu, Y.-S., Pan, C.-G., Chen, J., He, L.-Y., and Ying, G.-G. (2018). Persistence of antibiotic resistance genes and bacterial community changes in drinking water treatment system: from drinking water source to tap water. Sci. Total Environ. 616, 453–461. doi: 10.1016/j.scitotenv.2017.10.318
Su, J.-Q., Wei, B., Ou-Yang, W.-Y., Huang, F.-Y., Zhao, Y., Xu, H.-J., et al. (2015). Antibiotic resistome and its association with bacterial communities during sewage sludge composting. Environ. Sci. Technol. 49, 7356–7363. doi: 10.1021/acs.est.5b01012
Sun, Y. M., Guo, G., Tian, F., Chen, H. H., Liu, W. J., Li, M., et al. (2021). Antibiotic resistance genes and bacterial community on the surfaces of five cultivars of fresh tomatoes. Ecotoxicology 30, 1550–1558. doi: 10.1007/s10646-020-02303-3
Torkar, K. G., and Drazetic, M. (2017). The microbial contamination and the presence of beta-lactamase producing Gram-negative bacteria in the water and on the surfaces of public recreation water facilities. Int. J. Environ. Health Res. 27, 293–305. doi: 10.1080/09603123.2017.1342227
Ullmann, I. F., Tunsjo, H. S., Andreassen, M., Nielsen, K. M., Lund, V., and Charnock, C. (2019). Detection of aminoglycoside resistant bacteria in sludge samples from norwegian drinking water treatment plants. Front. Microbiol. 10:487. doi: 10.3389/fmicb.2019.00487
Wan, K., Lin, W., Zhu, S., Zhang, S., and Yu, X. (2020). Biofiltration and disinfection codetermine the bacterial antibiotic resistome in drinking water: a review and meta-analysis. Front. Environ. Sci. Eng. 14:10. doi: 10.1007/s11783-019-1189-1
Wang, J. H., Lu, J., Zhang, Y. X., Wu, J., Luo, Y. M., and Liu, H. (2018). Metagenomic analysis of antibiotic resistance genes in coastal industrial mariculture systems. Biores. Technol. 253, 235–243. doi: 10.1016/j.biortech.2018.01.035
Wang, S., Ma, X., Liu, Y., Yi, X., Du, G., and Li, J. (2020). Fate of antibiotics, antibiotic-resistant bacteria, and cell-free antibiotic-resistant genes in full-scale membrane bioreactor wastewater treatment plants. Bioresour. Technol. 302:122825. doi: 10.1016/j.biortech.2020.122825
Wu, D.-L., Zhang, M., He, L.-X., Zou, H.-Y., Liu, Y.-S., Li, B.-B., et al. (2020). Contamination profile of antibiotic resistance genes in ground water in comparison with surface water. Sci. Total Environ. 715:136975. doi: 10.1016/j.scitotenv.2020.136975
Xu, L., Campos, L. C., Canales, M., and Ciric, L. (2020). Drinking water biofiltration: Behaviour of antibiotic resistance genes and the association with bacterial community. Water Res. 182:115954. doi: 10.1016/j.watres.2020.115954
Xu, L., Ouyang, W., Qian, Y., Su, C., Su, J., and Chen, H. (2016). High-throughput profiling of antibiotic resistance genes in drinking water treatment plants and distribution systems. Environ. Pollut. 213, 119–126. doi: 10.1016/j.envpol.2016.02.013
Xu, R., Yang, Z. H., Wang, Q. P., Bai, Y., Liu, J. B., Zheng, Y., et al. (2018). Rapid startup of thermophilic anaerobic digester to remove tetracycline and sulfonamides resistance genes from sewage sludge. Sci. Total Environ. 612, 788–798. doi: 10.1016/j.scitotenv.2017.08.295
Yin, X., Jiang, X.-T., Chai, B., Li, L., Yang, Y., Cole, J. R., et al. (2018). ARGs-OAP v2.0 with an expanded SARG database and Hidden Markov Models for enhancement characterization and quantification of antibiotic resistance genes in environmental metagenomes. Bioinformatics 34, 2263–2270. doi: 10.1093/bioinformatics/bty053
Zeng, Q., Sun, J., and Zhu, L. (2019). Occurrence and distribution of antibiotics and resistance genes in greenhouse and open-field agricultural soils in China. Chemosphere 224, 900–909. doi: 10.1016/j.chemosphere.2019.02.167
Zhang, G., Lu, S., Wang, Y., Liu, X., Liu, Y., Xu, J., et al. (2020b). Occurrence of antibiotics and antibiotic resistance genes and their correlations in lower Yangtze River, China. Environ. Pollut. 257:113365. doi: 10.1016/j.envpol.2019.113365
Zhang, H., Zhang, Q., Song, J., Zhang, Z., Chen, S., Long, Z., et al. (2020c). Tracking resistomes, virulence genes, and bacterial pathogens in long-term manure-amended greenhouse soils. J. Hazard. Mater. 396:122618. doi: 10.1016/j.jhazmat.2020.122618
Zhang, K., Xin, R., Zhao, Z., Ma, Y., Zhang, Y., and Niu, Z. (2020d). Antibiotic resistance genes in drinking water of China: occurrence, distribution and influencing factors. Ecotoxicol. Environ. Safety 188:109837. doi: 10.1016/j.ecoenv.2019.109837
Zhang, S., Abbas, M., Rehman, M. U., Huang, Y., Zhou, R., Gong, S., et al. (2020e). Dissemination of antibiotic resistance genes (ARGs) via integrons in Escherichia coli: a risk to human health. Environ. Pollut. 266:115260. doi: 10.1016/j.envpol.2020.115260
Zhang, G., Guan, Y., Zhao, R., Feng, J., Huang, J., Ma, L., et al. (2020a). Metagenomic and network analyses decipher profiles and co-occurrence patterns of antibiotic resistome and bacterial taxa in the reclaimed wastewater distribution system. J. Hazard. Mater. 400:123170. doi: 10.1016/j.jhazmat.2020.123170
Zhang, H. C., Chang, F. Y., Shi, P., Ye, L., Zhou, Q., Pan, Y., et al. (2019). Antibiotic Resistome alteration by different disinfection strategies in a full-scale drinking water treatment plant deciphered by metagenomic assembly. Environ. Sci. Technol. 53, 2141–2150. doi: 10.1021/acs.est.8b05907
Zhang, L., Gu, J., Wang, X., Zhang, R., Tuo, X., Guo, A., et al. (2018). Fate of antibiotic resistance genes and mobile genetic elements during anaerobic co-digestion of Chinese medicinal herbal residues and swine manure. Bioresour. Technol. 250, 799–805. doi: 10.1016/j.biortech.2017.10.100
Zhang, S., Yang, G., Hou, S., Zhang, T., Li, Z., and Liang, F. (2018). Distribution of ARGs and MGEs among glacial soil, permafrost, and sediment using metagenomic analysis. Environ. Pollut. 234, 339–346. doi: 10.1016/j.envpol.2017.11.031
Zhang, S., Wang, Y., Lu, J., Yu, Z., Song, H., Bond, P. L., et al. (2021). Chlorine disinfection facilitates natural transformation through ROS-mediated oxidative stress. Isme J.15, 2969–2985. doi: 10.1038/s41396-021-00980-4
Zhao, Q. Q., He, H., Gao, K., Li, T., and Dong, B. Z. (2022). Fate, mobility, and pathogenicity of drinking water treatment plant resistomes deciphered by metagenomic assembly and network analyses. Sci. Total Environ. 804:150095. doi: 10.1016/j.scitotenv.2021.150095
Zhou, Z., Xu, L., Zhu, L., Liu, Y., Shuai, X., Lin, Z., et al. (2021). Metagenomic analysis of microbiota and antibiotic resistome in household activated carbon drinking water purifiers. Environ. Int. 148, 106394–106394. doi: 10.1016/j.envint.2021.106394
Zhu, L., Zhao, Y., Yang, K., Chen, J., Zhou, H., Chen, X., et al. (2019). Host bacterial community of MGEs determines the risk of horizontal gene transfer during composting of different animal manures. Environ. Pollut. 250, 166–174. doi: 10.1016/j.envpol.2019.04.037
Zhu, N. J., Ghosh, S., Edwards, M. A., and Pruden, A. (2021). Interplay of biologically active carbon filtration and chlorine-based disinfection in mitigating the dissemination of antibiotic resistance genes in water reuse distribution systems. Environ. Sci. Technol. 55, 8329–8340. doi: 10.1021/acs.est.1c01199
Keywords: antibiotic resistance genes, antibiotic resistant bacteria, metagenomic approach, metagenome-assembled genomes, pathogens, DWTS
Citation: Gu Q, Sun M, Lin T, Zhang Y, Wei X, Wu S, Zhang S, Pang R, Wang J, Ding Y, Liu Z, Chen L, Chen W, Lin X, Zhang J, Chen M, Xue L and Wu Q (2022) Characteristics of Antibiotic Resistance Genes and Antibiotic-Resistant Bacteria in Full-Scale Drinking Water Treatment System Using Metagenomics and Culturing. Front. Microbiol. 12:798442. doi: 10.3389/fmicb.2021.798442
Received: 20 October 2021; Accepted: 20 December 2021;
Published: 22 February 2022.
Edited by:
Catherine D. Carrillo, Canadian Food Inspection Agency, CanadaReviewed by:
Yu Xia, Southern University of Science and Technology, ChinaOlutayo Falodun, University of Ibadan, Nigeria
Copyright © 2022 Gu, Sun, Lin, Zhang, Wei, Wu, Zhang, Pang, Wang, Ding, Liu, Chen, Chen, Lin, Zhang, Chen, Xue and Wu. This is an open-access article distributed under the terms of the Creative Commons Attribution License (CC BY). The use, distribution or reproduction in other forums is permitted, provided the original author(s) and the copyright owner(s) are credited and that the original publication in this journal is cited, in accordance with accepted academic practice. No use, distribution or reproduction is permitted which does not comply with these terms.
*Correspondence: Qingping Wu, wuqp203@163.com
†These authors have contributed equally to this work