- 1Oklahoma Biological Survey and Department of Biology, University of Oklahoma, Norman, OK, United States
- 2National Park Service, Great Lakes Inventory and Monitoring Network, Ashland, WI, United States
Microbiomes are increasingly recognized as widespread regulators of function from individual organism to ecosystem scales. However, the manner in which animals influence the structure and function of environmental microbiomes has received considerably less attention. Using a comparative field study, we investigated the relationship between freshwater mussel microbiomes and environmental microbiomes. We used two focal species of unionid mussels, Amblema plicata and Actinonaias ligamentina, with distinct behavioral and physiological characteristics. Mussel microbiomes, those of the shell and biodeposits, were less diverse than both surface and subsurface sediment microbiomes. Mussel abundance was a significant predictor of sediment microbial community composition, but mussel species richness was not. Our data suggest that local habitat conditions which change dynamically along streams, such as discharge, water turnover, and canopy cover, work in tandem to influence environmental microbial community assemblages at discreet rather than landscape scales. Further, mussel burrowing activity and mussel shells may provide habitat for microbial communities critical to nutrient cycling in these systems.
Introduction
Key ecosystem processes are carried out by both microbes and animals, but microbial communities are particularly important to evaluate in tandem with animal influences on ecosystem function as microbiome data combined with environmental data improve our understanding of ecosystem processes (Graham et al., 2016). Further, effects of animals on microbial communities are important and underexplored (Skelton et al., 2017; Fitzpatrick et al., 2018; Thoemmes and Cove, 2020). As more systems are investigated, the implications of animal-microbial interactions and their impact on ecosystem function become more apparent. For example, marine birds translocate nutrients from the ocean to islands, where those nutrients increase organic matter decomposition rates by soil bacteria (Fukami et al., 2006); earthworms affect the function, but not community composition, of methanotrophic bacteria in landfills (Héry et al., 2008); and marsupial burrowing activity causes successional shifts in microbial community composition and increases nitrogen availability in soils (Eldridge et al., 2015). It is particularly important to understand baseline interactions between animals and microbes in the wake of climate and land use change. In this context, streams are a good study system because they are globally threatened by pollution and climate change (Jury and Vaux, 2005).
Within these systems, freshwater mussels (bivalve mollusks in the order Unionida) are large (∼10–100 mm adult shell length), long-lived (∼10 to over 100 years) benthic animals that perform important ecosystem functions in streams, such as filtering the water and recycling and storing nutrients (Vaughn and Hoellein, 2018). Freshwater mussels are globally imperiled and as mussel communities shift and populations decline (Spooner and Vaughn, 2008; Vaughn et al., 2015), evaluating mussel-microbiome interactions is critical to predicting changes in ecosystem function. Mussels often occur as dense (∼10–100 mussels/m2), multispecies aggregations called mussel beds and can comprise a significant portion of benthic biomass (Vaughn and Spooner, 2006). These communities can have large impacts on both biotic and abiotic factors in streams (Vaughn and Hoellein, 2018).
Of importance to stream microbial function, filter-feeding mussels burrow in the sediment and transform as well as transport organic matter from the water column into the sediment via excretion and biodeposition of feces and pseudofeces (rejected particles encapsulated in mucus and expelled before ingestion). We know mussel beds can significantly influence nutrient cycling in the sediment on an ecosystem-wide scale (Hoellein et al., 2017; Nickerson et al., 2019) indicating interactions with sediment microbiomes. Aquatic sediment is a unique environment in which the interface between an oxygenated surface and an anoxic subsurface microhabitat is relatively shallow and mussel burrowing activity can directly influence the microhabitats of both layers, often introducing oxic microniches into anoxic habitats (Brune et al., 2000). Levels of oxygenation can affect microbial community composition and function (Sinsabaugh et al., 2009) and benthic organisms can couple microbially driven biogeochemical processes (nitrification-denitrification, elemental sulfur cycling, etc.; Nickerson et al., 2019). However, incorporating drivers of benthic microbial community structure and diversity into riverine ecosystem function requires further research (Zeglin, 2015).
Here we consider the mussel microbiome to be comprised of the microbial communities on mussel shells and in their biodeposits. How these communities interact with the sediment microbial communities, and potential differences between mussel species in how this occurs, is a key research gap. Host physiology and diet are known to impact hosted microbiomes in a variety of organisms (Turnbaugh and Gordon, 2009; Phillips et al., 2012; Ye et al., 2014; Pierce et al., 2016) and freshwater mussels have species-specific physiological and behavioral traits (Haag, 2012). Interspecific differences may influence the mussel microbiome and therefore interactions with the sediment microbiome. For example, mussel species investigated in southeastern Oklahoma U.S. are either thermally sensitive (e.g., Actinonaias ligamentina) or thermally tolerant (e.g., Amblema plicata), and thermally sensitive species excrete nutrients at higher rates at warm temperatures, with different stoichiometric ratios when stressed (Spooner and Vaughn, 2008). Additionally, Allen and Vaughn (2009) found that mussels exhibit species-specific differences in burrowing activity with thermally sensitive species demonstrating higher activity.
Here we asked, how similar are the freshwater mussel microbiome and the sediment microbiome, and how do these relationships change with mussel abundance, species composition, and environmental conditions? We addressed these questions with a field study comparing benthic microbiomes in three mussel beds in a small river in the southern US focusing on two dominant mussel species. We sampled microbial communities from four microhabitats: the surface layer of sediment, sediment from 6 to 10 cm below the surface, mussel shells, and mussel biodeposits. Microbes were identified using 16S rRNA analysis. We predicted that environmental conditions among microhabitats would be sufficiently distinct to host unique assemblages of microbes. We expected that differences in mussel species’ behavior and nutrient excretion would produce species-specific host-associated microbial community composition. We also expected that microbial community structure within the sediment would reflect mussel-associated changes in biogeochemical cycling.
Materials and Methods
Study Area and Focal Mussel Species
We conducted our study in the Kiamichi River, Oklahoma, a well-studied stream in the southcentral U.S. known for its high freshwater mussel biodiversity (Matthews et al., 2005). Mussel assemblages in this river are typically dominated by two species, Actinonaias ligamentina and Amblema plicata, that make up ∼70% of mussel biomass in this region but differ morphologically, behaviorally, and physiologically (Vaughn, 2010; Hopper et al., 2019). Amblema plicata has a ridged shell and tends to be sedentary, while A. ligamentina is an active burrower with a smooth shell (Allen and Vaughn, 2009). The two species also differ in their thermal preferences, which influences filtration rates as well as nutrient excretion rates and stoichiometry (Spooner and Vaughn, 2008; Trentman et al., 2018).
Field Study
In July 2018, we collected microbial samples from mussel beds in the Kiamichi River. We selected three sites (Figure 1) with previously documented abundant, diverse mussel assemblages and data on mussel roles in nutrient recycling and storage (Atkinson et al., 2013; Atkinson and Vaughn, 2015; Hopper et al., 2018). Sites varied in abiotic characteristics that may influence environmental microbiomes such as flow, substrate, and shade. Thus, we characterized sites by measuring flow (with a Hach LDO meter), sediment particle sizes (with Wolman pebble counts), and shading (using a densiometer) in summers 2015–2016 as part of a larger study (Hopper et al., 2018; Vaughn et al., 2021).
At each of the three selected sites, we sampled four individuals of both A. plicata and A. ligamentina. For each mussel bed, we first conducted tactile searches on the sediment surface to locate mussels. Searches were conducted from downstream to upstream to minimize disturbance of the sediment. We then placed a 0.25 m2 quadrat around locations that contained at least one individual of each species. Although quadrats could contain multiple individuals of each species, they all contained both focal species, and we only sampled one individual of each species per quadrat. We had a total of 12 sampling locations and 24 total mussels (12 A. plicata and 12 A. ligamentina) across all sites. We used a custom 4.9 cm diameter, clear acrylic sediment corer to collect one sediment core from each quadrat, for a total of 12 sediment cores. To prevent cross-contamination between samples, we used 90% ethanol to rinse the interior of our corer between samples. Mussels have been shown to affect bacterial growth and metabolism at depths of 6 cm or greater below the surface (McCall et al., 1986; Black and Just, 2018) so we extruded the sediment from the corer with a rubber stopper and took subsamples from the surface layer (n = 12) and 6–10 cm below (n = 12) using an ethanol rinsed, flame sterilized spatula.
We removed mussel individuals from the sediment and used sterile razor blades to collect a single biofilm sample from the shell of each mussel. Blades were rinsed with 90% ethanol, wiped with a sterile kimwipe, and flame sterilized for 1 min between samples (Horton et al., 2019; Miller-ter Kuile et al., 2021). Five shell samples did not successfully sequence (three from A. ligamentina and two from A. plicata) resulting in a final n of 19. We then gently scrubbed mussel shells using sterile nylon mesh to remove the remaining biofilm and left them in containers with 1 L of filtered river water for 4–6 h to allow time for mussels to biodeposit sufficient material for collection. Biodeposits were collected using an ethanol rinsed, flame-sterilized spatula. Similarly, not all biodeposits samples sequenced successfully (2 from each species) for a final n of 20. After mussels were removed from the sediment, quadrats were excavated to a depth of 15 cm and any additional mussels were identified to species and counted (Vaughn et al., 1997). While storage at –80°C is considered optimal for microbial community samples, short term cold storage demonstrates little change in fecal and soil microbiome community structure (Rubin et al., 2013; Choo et al., 2015), and so all microbiome samples were placed in sterile cryovials, stored on ice in coolers on the shaded riverbank for no more than 5 h, and then placed in liquid nitrogen until transfer to a –20°C freezer within 4 days.
Amplicon Library Construction and Sequencing
All samples were thawed, spun at 10,000 × gravity for 2 min, and water was removed via pipette. DNA was extracted using DNeasy PowerSoil® kits (Qiagen, Hilden, Germany). We amplified the v4 region of the 16s rRNA gene using primers and PCR protocols from Kozich et al. (2013). We purified post PCR samples with Ampure XP beads (BeckmanCoulter, Indianapolis, IL, United States) at 1 × concentration, quantified with a Qubit Fluorometer (Thermo Fisher Scientific, Waltham, Massachusetts, United States), diluted with lab grade water to 4 nM equimolar concentrations, and pooled. Library preparation was performed at the Sam Noble Oklahoma Museum of Natural History and library sequencing was performed at the University of Oklahoma Consolidated Core Lab using 2 × 250 bp paired-end sequencing on an Illumina MiSeq.
Bioinformatics and Data Analyses
Sequencing reads were merged and filtered using the program “AdapterRemoval” (Lindgreen, 2012). We performed closed reference OTU picking using “uParse” (Edgar, 2013) at 97% sequence similarity and assigned taxonomy with the SILVA reference database (v.32, Quast et al., 2013). After filtering out read abundances less than 0.1% of the average sequencing depth, we quantified richness and evenness of our samples with the number of unique OTUs and the Berger-Parker Dominance Index, respectively, using Quantitative Insights into Microbial Ecology (QIIME; Berger and Parker, 1970; Bolyen et al., 2019). We used Kruskal-Wallis tests to determine statistical differences in richness and evenness using the base R software (R Core Team, 2020). Significant results were further examined using Holm adjusted pairwise-Wilcoxon Rank Tests between microhabitats (Wright, 1992).
To quantify differences in beta diversity we calculated an Aitchison distance matrix (Euclidean distance of centered log-ratio transformed OTU counts) in R using the Compositions and Vegan packages (van den Boogaart and Tolosana-Delgado, 2008; Filzmoser et al., 2010; Gloor et al., 2017; Quinn et al., 2018; Oksanen et al., 2020). We used PERMANOVA to determine differences in bacterial community structure and permdisp to determine differences in dispersion among all microhabitats using Vegan’s “adonis” and “betadisper” functions, respectively. We conducted post hoc, pairwise PERMANOVA to evaluate differences among mussel and sediment microhabitats using the “pairwise.adonis” test from the pairwiseAdonis package (Wright, 1992; Martinez, 2020). We then tested the effects of site, sediment layer, mussel abundance and mussel richness on sediment microbial community structure as well as the effects of site and mussel species on shell and biodeposit microbial community structure. For both models, we used the “adonis2” function in Vegan for which the relative importance of each term is indicated by an R2 value (McArdle and Anderson, 2001; Oksanen et al., 2020). Differences in dispersion for these models were tested with Holm adjusted “betadipser” calculations. Environmental variables measured at each site (Ratio of D60 to D10 Wolman pebble counts, average discharge, average canopy cover, and average turnover) were correlated with bacterial communities using the “envfit” program in vegan.
To visualize differences in community structure, we performed principal coordinates analyses (PCA) using Aitchison distance matrices (Gloor et al., 2017). We were interested in microbial community patterns in each microhabitat, so in addition to our entire dataset we generated individual PCAs for sediment, shell, and biodeposit communities. Then to examine taxa contributing to differences in microbial community structure, we calculated axis loadings of each PCA by calculating Pearson rank-sum correlations between axis scores and CLR transformed abundances using R (Comrey and Lee, 1992; Quinn et al., 2018). Loadings with absolute r-values ≥ 0.70 were considered sufficiently correlated to evaluate (Comrey and Lee, 1992; Curry and Patten, 2014; Weingarten et al., 2019). We interpreted correlations on both the first and second PC axis. With this method, significant correlations with an r-value above 0.70 are interpreted such that higher r-values indicated a given taxa had higher abundances as PC values increase and r-values below –0.70 are interpreted as taxa demonstrating higher abundances as PC values decrease. Further, we were interested in functional differences between microbial communities and so we only interpreted taxa identified to family as higher classifications tended to encompass taxa with broad metabolic and niche preferences.
Results
Microhabitats displayed significant differences in microbial richness (χ2 = 24.65, p < 0.001) and evenness (χ2 = 40.23, p < 0.001; Figure 2). Post hoc Wilcoxon rank-sum tests showed that these differences were likely driven by mussel biodeposit and shell samples that were 44% less rich (W = 117.5, p < 0.001) and 8% less even (W = 811, p < 0.001) than sediment samples. Pairwise comparisons further showed that mussel shell and mussel biodeposit microbial communities were not significantly different in richness (p = 0.31), but biodeposit samples were significantly less even (p < 0.001). The top layer of sediment had no significant differences in richness from lower layers (p = 0.32) or evenness (p = 0.31). Bacterial community structure (F = 13.71, p = 0.001) and dispersion (F = 3.74, p = 0.016) were significantly distinct among the four microhabitats (Figure 3). Pairwise PERMANOVA demonstrated that all microhabitats were significantly different from each other (Table 1). Axis loading calculations resulted in 15 taxa with identified genera and 28 unique families significantly correlated with either the first or second axis (Table 2).
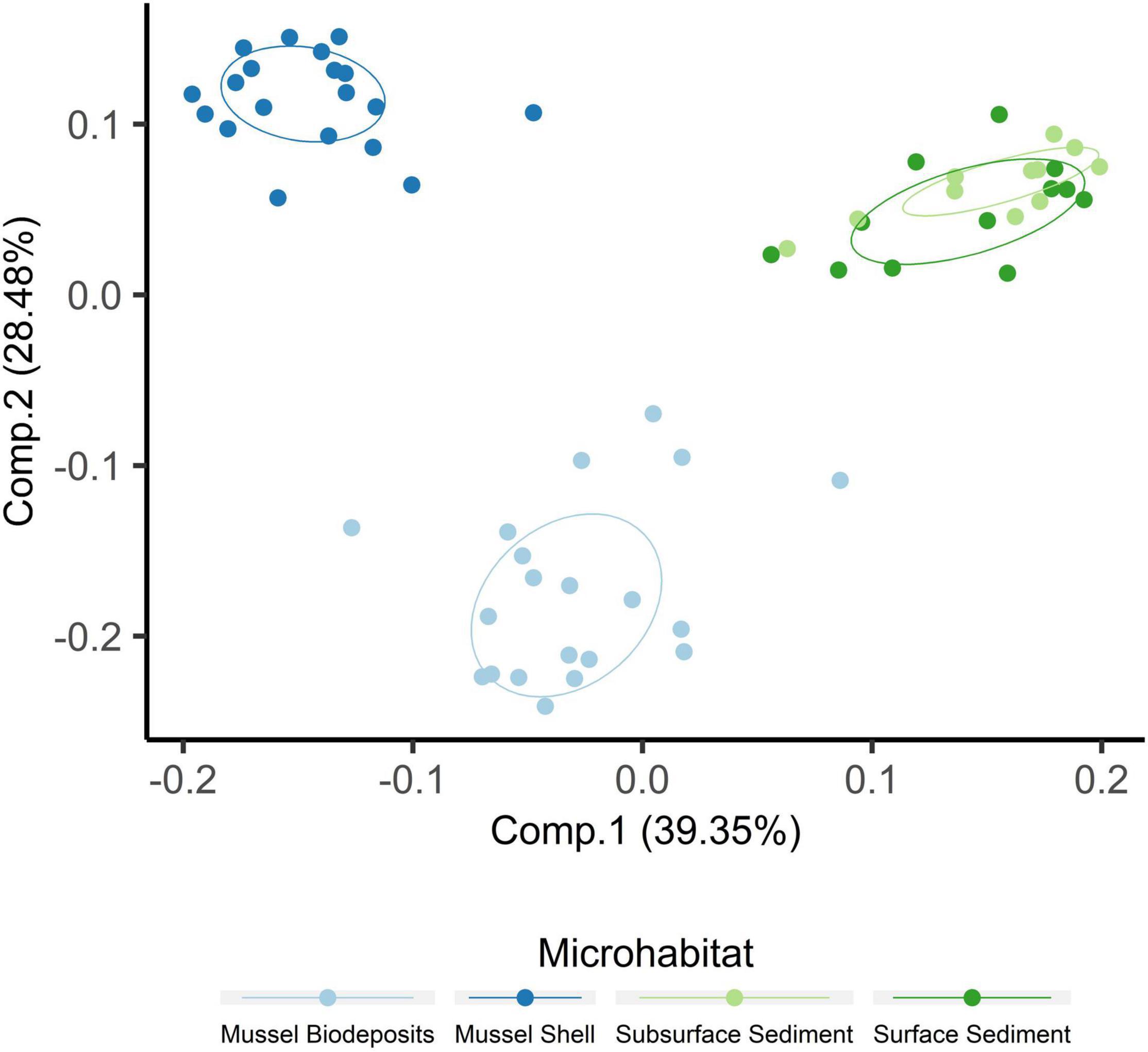
Figure 2. Principal coordinates analysis including samples from all microhabitats. Ellipses are drawn around centroids with a 50% confidence interval.
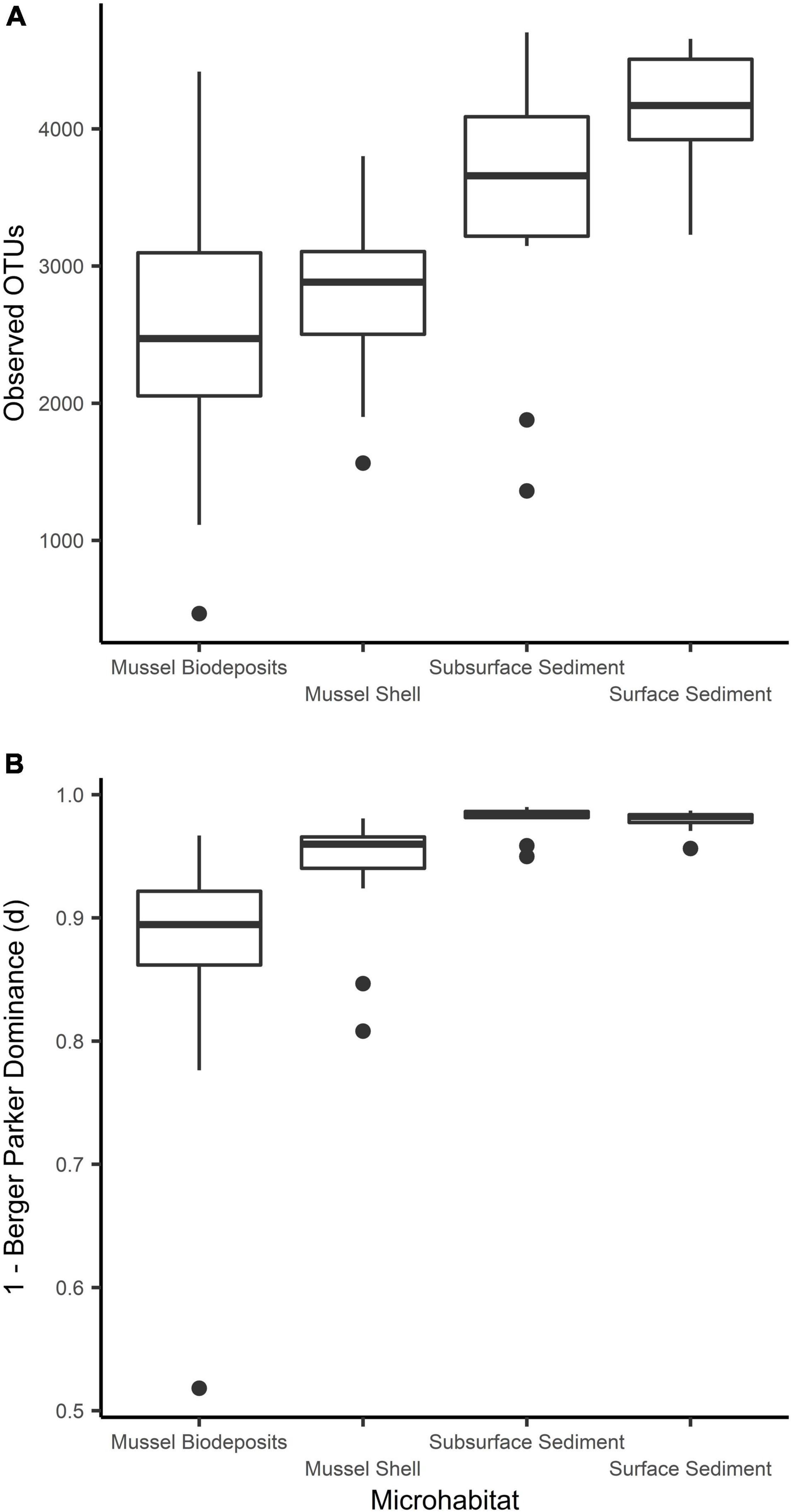
Figure 3. Comparisons of microbial community (A) richness and (B) evenness among sampled microhabitats.
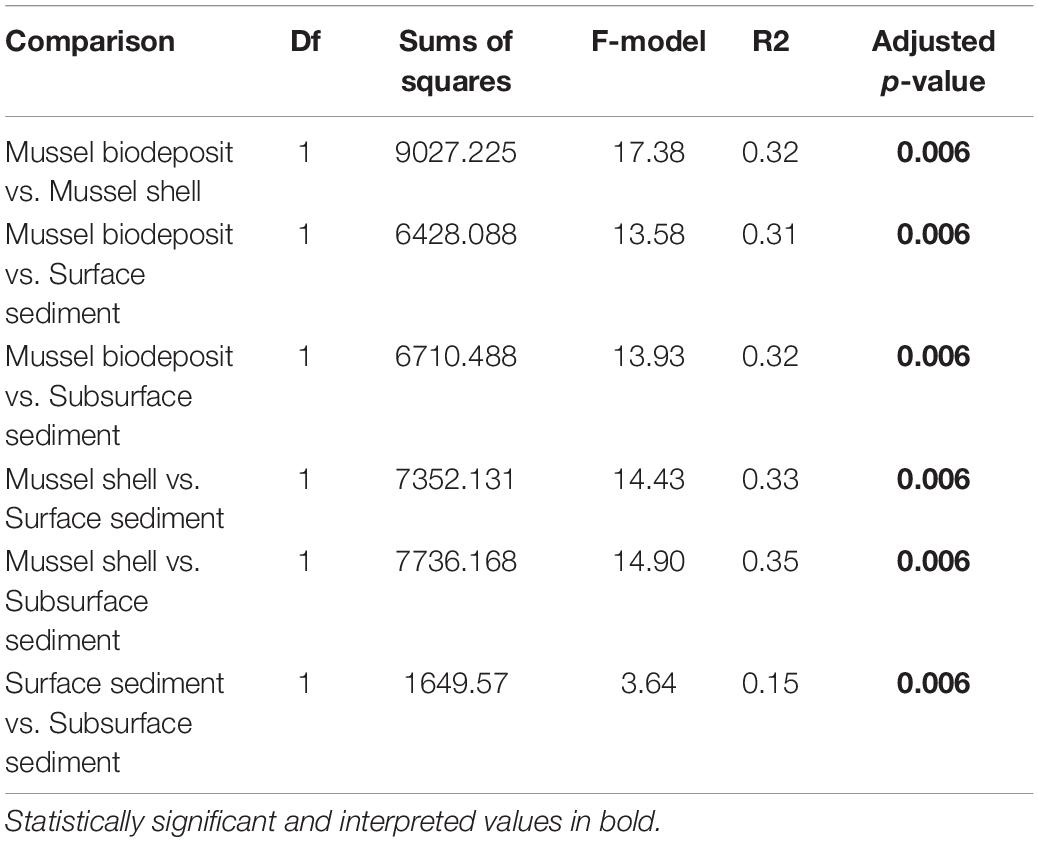
Table 1. Pairwise PERMANOVA results comparing microbial communities among microhabitats with Holm adjusted p-values.
Within quadrats, mussel abundance ranged from 3 to 13 mussels while richness ranged from 1 to 6 species. The PERMANOVA model testing the effects of site, sediment layer, mussel richness, and mussel abundance on sediment community structure was a statistically significant fit to these data (F = 2.52, p < 0.001). Of these variables, the strongest driver of sediment microbial community structure was sediment layer, followed by site, and then mussel abundance (Table 3). Mussel richness was not a significant predictor of sediment community structure (F = 1.144, p = 0.11). Sediment layer (F = 0.030, p = 0.86), mussel abundance (F = 1.65, p = 0.58), and mussel richness (F = 1.69, p = 0.58) did not show significant differences in dispersion and sites were only marginally significantly different in dispersion (F = 4.76, p = 0.07; Figure 4A). Sediment axis loadings resulted in 7 identified genera and 17 unique families (Table 4).
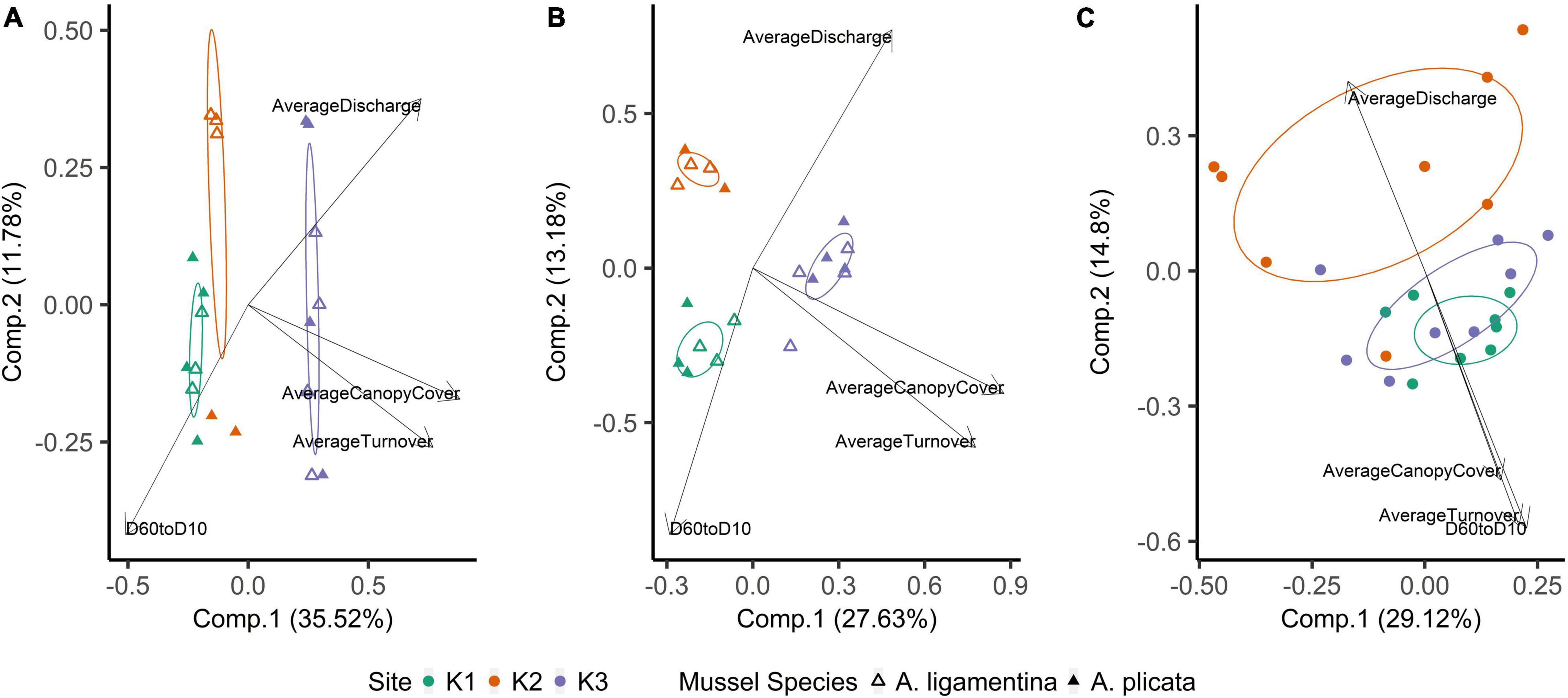
Figure 4. Principal coordinates analysis of (A) mussel biodeposit microbial communities, (B) mussel shell microbial communities, and (C) sediment microbial communities. Communities are colored based on the site from which they were sampled. Ellipses are drawn around centroids with a 50% confidence interval. Vectors were added post hoc and are based on envfit analysis.
Overall, the mussel biodeposit (F = 3.01, p = 0.001) and shell (F = 2.87, p = 0.001) models were statistically significant fits to these data. Mussel biodeposit microbial community structure seems to be driven by site, but mussel species was not significant (Table 3 and Figure 4B). Differences in dispersion were significant based on site (F = 5.75, p = 0.0248) but not mussel species (F = 0.201, p = 0.659). Similar to biodeposits, shell microbial communities seem driven by site, but not species (Table 3 and Figure 4C). There were no significant differences in dispersion by either site (F = 2.16, p = 0.296) or mussel species (F = 0.059, p = 0.811). Axis loadings for shell communities resulted in 8 identified genera and 18 unique families while biodeposit communities resulted in 4 identified genera and 16 unique families (Tables 5, 6).
The K2 mussel bed had larger substrates and pebble sizes that were evenly distributed indicated by a low D60/10. In comparison, the substrates of K1 and K3 were smaller but less evenly distributed. Discharge measurements suggest that the mussel bed at K3 typically had longer water turnover times (Table 7).
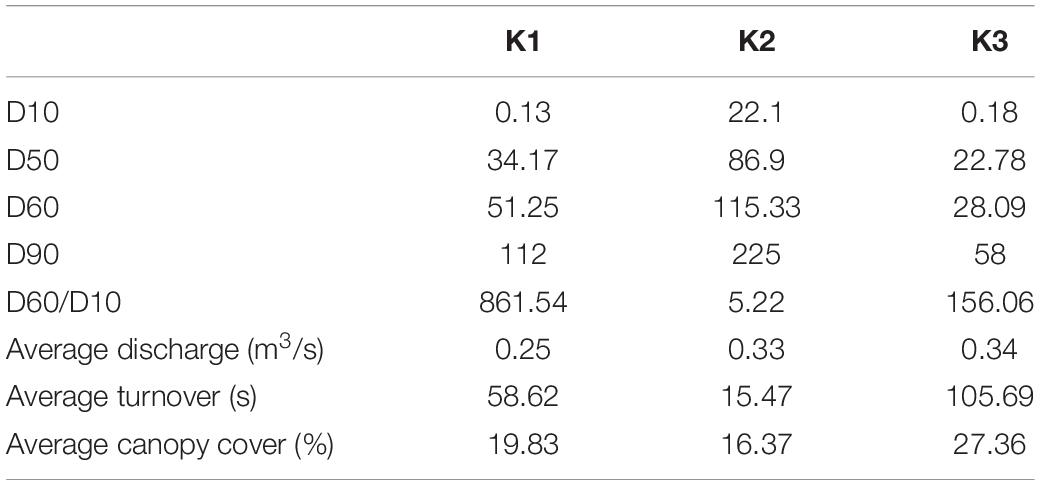
Table 7. Wolman pebble counts, substratum heterogeneity (D60/D10), flow measurements, and canopy cover collected in 2016, from Vaughn et al. (2021).
Discussion
Our investigation revealed that the microbial communities hosted by freshwater mussels are distinct from those of the surrounding sediment. These microhabitats are in constant contact; mussels deposit feces and pseudofeces directly into the sediment and we collected biofilm from shells that were exposed to surface and subsurface sediment. Yet, sediment communities demonstrated higher alpha diversity than those that were mussel-associated, and we also found low overlap in microbial community composition among animal-associated and environmental microhabitats. Our data indicate that both environmental conditions specific to locations along the river and animal activity shape these microbial communities. Interactions relevant to critical ecological function between these microhabitats can be inferred by examining the ecology of taxa that distinguish these distinct communities.
Site Characteristics Supersede Mussel Species Identity, Abundance, and Richness as a Driver of Microbial Community Composition Within Mussel Beds
While Weingarten et al. (2019) found that microbial communities retained by freshwater mussels were influenced by species as well as site, our study found that microbes on the shell and in material passed through the gut, did not differ between our focal species. These results are complementary. Much of the phytoplankton, detritus, and bacteria filtered by mussels survives gut passage alive and undamaged (Vaughn et al., 2008) and so it is possible that taxa retained in the gut may be influenced by mussel species, but taxa that pass through the gut are not. Mussel biodeposits, regardless of species, may reflect the same background food sources and differences in biodeposit community composition may be minimal when occupying the same site. The algae and bacteria able to colonize shells may similarly be site specific and this signal may overwhelm any differences in community on the basis of different shell characteristics between species.
In contrast to species, site was a strong predictor of microbial community composition in every model tested. K1 and K3 sediment communities are more similar to each other than to K2 (Figure 4C) and mussel shell and biodeposit communities at K2 and K1 are more similar to each other than to K3 (Figures 4A,B). These patterns are not entirely expected based on scale and hydrology. If increasing spatial scale were to predict our microbial community assemblages as it can in soil (Averill et al., 2021), then we would expect sites closer together to show greater overlap in community composition yet our most distant sites (K1 and K3) are more similar to each other than to K2 (Figure 1). Additionally, K1 is located upstream of a tributary impoundment, while K2 and K3 are downstream. Lack of releases from this impoundment during recent severe drought years has led to patchy drying of the lower river and increased water temperatures in shallow areas which has led to mussel declines and changes in mussel community composition (Atkinson et al., 2014; Vaughn et al., 2015). Based on these changes in flow regimes and mussel communities, these results are similarly unexpected.
Local characteristics at the stream reach level may offer insight into differences in these microbial communities. Sediment depth and particle size are both significant predictors of microbial community structure in streams (Sliva and Williams, 2005). K2’s relatively larger but more evenly distributed sediment particle sizes may drive distinct microbial communities from K1 and K3. We see this reflected in our envfit results which indicate higher values as community compositions grow more distinct from those of K2 (Figure 4 and Table 6). Similarly, differences in canopy cover govern shading and thus influence photosynthetic organisms on shells. K3 has the highest canopy cover and contains shell microbial communities that are most distinct from K2 and K1. K3 also has the greatest water turnover time which may partially explain its distinction in biodeposit community composition. Slower turnover in the water column will increase the duration of seston delivery which impacts what seston mussels filter (Byllaardt and Ackerman, 2014; Mistry and Ackerman, 2018), and therefore egest as biodeposits, as well as impact which bacterial taxa can colonize shells.
Mussel abundance also significantly affected sediment microbial communities. This result is supported by the findings of Black et al. (2017). They investigated relationships between sediment microbial communities and the presence or absence of mussels in the upper Mississippi River and found that sediment below mussels hosted distinct microbial communities. However, our results may underestimate the impact of mussel beds on sediment community structure. Our sampling resulted in a range of 12–56 mussels per m2 and unionid mussel abundances in this system can reach up to 100 mussels per m2. Investigating mussel impacts on sediment communities when present at higher densities may demonstrate greater significance.
Interactions Between the Distinct Microbial Communities Found Within Benthic Microhabitats May Be a Driver of Ecosystem Function
Our data suggests that as a system, mussel shells, biodeposits, and the surrounding sediment contain microbial communities that work synergistically across microhabitats to cycle sulfur and nitrogen in aquatic environments. Interactions between mussels and the surrounding sediment is particularly relevant to ongoing investigations of the impact of freshwater mussels on ecosystem function (Vaughn and Hoellein, 2018). Studies on mussel-driven changes in nutrient cycling often focus either on the nutrients mussels cycle themselves (Atkinson and Vaughn, 2015; Trentman et al., 2018) or on ecosystem processes carried out by sediment microbial heterotrophs (Black and Just, 2018; Nickerson et al., 2019).
Across microhabitats, there are microbes that are important to sulfur cycling. Typically in anaerobic subsurface sediments, sulfate reducing bacteria (SRB) use sulfate as a terminal electron acceptor instead of oxygen, resulting in sulfide compounds (Hansen, 1994). We found multiple SRB taxa within the family Thermodesulfovibrionaceae and the genus Desulfococcus that distinguish sediment from other microhabitats (Table 2; Galushko and Kuever, 2019; Umezawa et al., 2021). Then, much of the sulfide formed by SRB in sediments is oxidized back to sulfate by sulfur-oxidizing bacteria (SOB; Jørgensen and Nelson, 2004). Among the bacteria that distinguish shell bacterial communities from other microhabitats, we find SOB in the genera Novosphingobium and Rhodobacter (Table 2; Imhoff et al., 1984; Imhoff, 2015; Haosagul et al., 2020). We also see the family Comamonadaceae associated more strongly with mussel biodeposits than other microhabitats and this family has also been shown to oxidize sulfur compounds (Zhang et al., 2017). While we detect evidence of both SRB and SOB within the sediment, these SOB distinguish mussel communities from those of the sediment and their differential abundances may be facilitated by mussel activity. It is not unusual in marine sediments for sulfur bacteria to form symbiotic relationships with bivalves that depend on their primary production (Vaughn and Hoellein, 2018). If mussel activity expands the oxic-anoxic interface and delivers SOB to anoxic regions to respire sulfide produced by SRB, this may be an additional and critical mechanism by which mussels influence nutrient dynamics and primary production in freshwater environments. The presence of both SOB and SRB in these environments may prove especially interesting considering the interactions between sulfide produced by SRB and nitrogen removal in streams.
One of the primary mechanisms by which excess nitrogen (N) is removed as N2 gas from aquatic ecosystems rather than assimilated into microbial and algal biomass, is through dissimilatory N respiration by microbes in the sediment. However, under laboratory conditions, sulfide has been demonstrated to inhibit enzyme pathways required for both nitrification (Joye and Hollibaugh, 1995) and denitrification (Brunet and Garcia-Gil, 1996; Burgin and Hamilton, 2007). Yet, mussels have been shown to increase the potential for nitrogen removal in sediments proven to have relatively high amounts of sulfur deposition (Newton et al., 2013; Nickerson et al., 2019). When adjusting the ratio of carbon and nitrogen in a bioreactor, dos Santos et al. (2021) found that microbial communities with significantly higher N removal demonstrated increased abundances of six families of bacteria: Saprospiraceae, Chitinophagaceae, Xanthomonadaceae, Comamonadaceae, Bacillaceae, and Planctomycetaceae. We found five of these six families associated with different microhabitats across mussel beds (Tables 2–6) indicating that it may not simply be mussel driven changes in sediment communities that alter nutrient cycling in these systems, but rather interactions between mussel-associated and environmental microbes.
Nitrification and sulfide oxidation occur in oxic sediments while denitrification and sulfate reduction occur in anoxic environments. Mussels often traverse the boundary between oxic and anoxic layers of the sediment and so it is not surprising that we find microbes that encompass these diverse modes of respiration associated with them in the sediment. However, these modes of respiration are complementary, and we find key players in these cycles across both mussels and the sediment. These patterns suggest that the role of burrowing bivalves in facilitating interactions between these microbes may be underestimated. Further, delivery of both S contaminants and nitrates to anaerobic sediments can enhance both sulfide oxidation and denitrification (Cardoso et al., 1996) and sulfur cycling by microbes has been shown to account for a large portion of nitrate removal in streams, lakes, and wetlands (Burgin and Hamilton, 2008). Mussels may provide a niche for microbes with a wide array of respiratory functions that in summation serve to remove contaminants of ecological concern (Turner and Rabalais, 2003; Burgin and Hamilton, 2007).
Conclusion
In our exploratory study of the microbiomes of mussels (shell and biodeposits) and the environment they inhabitat (sediment) we found that mussel microbiomes were less diverse than those of the sediment, mussel abundance was a significant predictor of sediment microbial community composition, and local habitat influenced microbial assemblage composition more than site spatial location along the river. Our findings indicate that rather than a continuous shift in beta diversity along the river, microbial communities further away from each other are more similar than communities next to each other. In our system, a regional pool of bacterial taxa may thus be filtered by site-specific environmental conditions along the river continuum. Further, the presence of macro-organisms may be an additional mechanism that shapes the microbiome of benthic communities. Although synergistic communities of microbes are likely to persist in the sediment, interactions may be limited. Animal-facilitated interactions between freshwater microhabitats have implications for the removal of environmentally impactful metabolites such as nitrates and sulfides. We suggest more thorough testing of the impact of mussels and other burrowing organisms on microbial community diversity and function. Mussel aggregations may provide a niche for microbial communities that undoubtedly play a role in the complex cycling of multiple nutrients critical to primary production. Alterations to nitrogen and sulfide removal become especially important when considering anthropogenic inputs into freshwater systems.
Data Availability Statement
Data are available through Open Science Framework: Vaughn et al. (2021).
Author Contributions
EH, TP, and CV designed the comparative field study. EH performed analyses and wrote the initial manuscript with subsequent input from all authors.
Funding
The funding was provided by NSF DEB-1457542 to CV, the University of Oklahoma Research Council, the Oklahoma Biological Survey, and The University of Oklahoma Graduate Student Senate.
Conflict of Interest
The authors declare that the research was conducted in the absence of any commercial or financial relationships that could be construed as a potential conflict of interest.
Publisher’s Note
All claims expressed in this article are solely those of the authors and do not necessarily represent those of their affiliated organizations, or those of the publisher, the editors and the reviewers. Any product that may be evaluated in this article, or claim that may be made by its manufacturer, is not guaranteed or endorsed by the publisher.
Acknowledgments
We thank T. DuBose, N. Ferreira-Rodríguez, J. Hartwell, G. Hopper, J. Lopez, and B. Tweedy for assistance with field work. We thank C. Siler for use of molecular facilities and K. Sankaranarayanan for bioinformatic pipeline and aid in analyses. We thank C. Atkinson, K. Sankaranarayanan, C. Siler, and L. Souza for their input to study design and comments on the manuscript. This article is part of a dissertation at the University of Oklahoma and is a contribution to the program of the Oklahoma Biological Survey.
References
Allen, D. C., and Vaughn, C. C. (2009). Burrowing behavior of freshwater mussels in experimentally manipulated communities. J. North Am. Benthol. Soc. 28, 93–100. doi: 10.1899/07-170.1
Atkinson, C. L., and Vaughn, C. C. (2015). Biogeochemical hotspots: temporal and spatial scaling of the impact of freshwater mussels on ecosystem function. Freshw. Biol. 60, 563–574. doi: 10.1111/fwb.12498
Atkinson, C. L., Julian, J. P., and Vaughn, C. C. (2014). Species and function lost: role of drought in structuring stream communities. Biol. Conserv. 176, 30–38. doi: 10.1016/j.biocon.2014.04.029
Atkinson, C. L., Vaughn, C. C., Forshay, K. J., and Cooper, J. T. (2013). Aggregated filter-feeding consumers alter nutrient limitation: consequences for ecosystem and community dynamics. Ecology 94, 1359–1369. doi: 10.1890/12-1531.1
Averill, C., Werbin, Z. R., Atherton, K. F., Bhatnagar, J. M., and Dietze, M. C. (2021). Soil microbiome predictability increases with spatial and taxonomic scale. Nat. Ecol. Evol. 5, 747–756. doi: 10.1038/s41559-021-01445-9
Berger, W. H., and Parker, F. L. (1970). Diversity of planktonic foraminifera in deep-sea sediments. Science 168, 1345–1347. doi: 10.1126/science.168.3937.1345
Black, E. M., Chimenti, M. S., and Just, C. L. (2017). Effect of freshwater mussels on the vertical distribution of anaerobic ammonia oxidizers and other nitrogen-transforming microorganisms in upper Mississippi river sediment. PeerJ 5:e3536. doi: 10.7717/peerj.3536
Black, E. M., and Just, C. L. (2018). The genomic potentials of NOB and comammox nitrospira in river sediment are impacted by native freshwater mussels. Front. Microbiol. 9:2061. doi: 10.3389/fmicb.2018.02061
Bolyen, E., Rideout, J. R., Dillon, M. R., Bokulich, N. A., Abnet, C. C., Al-Ghalith, G. A., et al. (2019). Reproducible, interactive, scalable and extensible microbiome data science using QIIME 2. Nat. Biotechnol. 37, 852–857. doi: 10.1038/s41587-019-0209-9
Brune, A., Frenzel, P., and Cypionka, H. (2000). Life at the oxic-anoxic interface: microbial activities and adaptations. FEMS Microbiol. Rev. 24, 691–710. doi: 10.1016/S0168-6445(00)00054-1
Brunet, R. C., and Garcia-Gil, L. J. (1996). Sulfide-induced dissimilatory nitrate reduction to ammonia in anaerobic freshwater sediments. FEMS Microbiol. Ecol. 21, 131–138. doi: 10.1016/0168-6496(96)00051-7
Burgin, A. J., and Hamilton, S. K. (2007). Have we overemphasized the role of denitrification in aquatic ecosystems? A review of nitrate removal pathways. Front. Ecol. Environ. 5:89–96. doi: 10.1890/1540-929520075[89:HWOTRO]2.0.CO;2
Burgin, A. J., and Hamilton, S. K. (2008). NO3–Driven SO42- production in freshwater ecosystems: implications for N and S cycling. Ecosystems 11, 908–922. doi: 10.1007/s10021-008-9169-5
Byllaardt, J. V., and Ackerman, J. D. (2014). Hydrodynamic habitat influences suspension feeding by unionid mussels in freshwater ecosystems. Freshw. Biol. 59, 1187–1196. doi: 10.1111/fwb.12339
Cardoso, R. B., Sierra-Alvarez, R., Rowlette, P., Razo Flores, E., Go’mez, J., and Field, J. A. (1996). Sulfide oxidation under chemolithoautotrophic denitrifying conditions. J. Anat. 189, 503–505. doi: 10.1002/bit
Choo, J. M., Leong, L. E., and Rogers, G. B. (2015). Sample storage conditions significantly influence faecal microbiome profiles. Sci. Rep. 5:16350. doi: 10.1038/srep16350
Comrey, A. L., and Lee, H. B. (1992). A First Course in Factor Analysis, 2nd Edn. Hillsdale, MI: Lawrence Erlbaum Associates, Inc.
Curry, C. M., and Patten, M. A. (2014). Current and historical extent of phenotypic variation in the tufted and black-crested titmouse (paridae) hybrid zone in the southern great plains. Am. Midl. Nat. 171, 271–300. doi: 10.1674/0003-0031-171.2.271
dos Santos, C. E. D., Costa, R. B., Rabelo, C. A. B. S., Ferraz Júnior, A. D. N., Persinoti, G. F., Pozzi, E., et al. (2021). Hacking biofilm developed in a structured-bed reactor (SBRRIA) with integrated processes of nitrogen and organic matter removal. Bioprocess Biosyst. Eng. 44, 1841–1851. doi: 10.1007/s00449-021-02564-0
Edgar, R. C. (2013). UPARSE: highly accurate OTU sequences from microbial amplicon reads. Nat. Methods 10, 996–998. doi: 10.1038/nmeth.2604
Eldridge, D. J., Woodhouse, J. N., Curlevski, N. J. A., Hayward, M., Brown, M. V., and Neilan, B. A. (2015). Soil-foraging animals alter the composition and co-occurrence of microbial communities in a desert shrubland. ISME J. 9, 2671–2681. doi: 10.1038/ismej.2015.70
Filzmoser, P., Hron, K., and Reimann, C. (2010). The bivariate statistical analysis of environmental (compositional) data. Sci. Total Environ. 408, 4230–4238. doi: 10.1016/j.scitotenv.2010.05.011
Fitzpatrick, C. R., Copeland, J., Wang, P. W., Guttman, D. S., Kotanen, P. M., and Johnson, M. T. J. (2018). Assembly and ecological function of the root microbiome across angiosperm plant species. Proc. Natl. Acad. Sci. U.S.A. 115, E1157–E1165. doi: 10.1073/pnas.1717617115
Fukami, T., Wardle, D. A., Bellingham, P. J., Mulder, C. P. H., Towns, D. R., Yeates, G. W., et al. (2006). Above- and below-ground impacts of introduced predators in seabird-dominated island ecosystems. Ecol. Lett. 9, 1299–1307. doi: 10.1111/j.1461-0248.2006.00983.x
Galushko, A., and Kuever, J. (2019). “Desulfococcus,” in Bergey’s Manual of Systematics of Archaea and Bacteria, ed. W. B. Whitman (Atlanta: American Cancer Society), 1–5.
Gloor, G. B., Macklaim, J. M., Pawlowsky-Glahn, V., and Egozcue, J. J. (2017). Microbiome datasets are compositional: and this is not optional. Front. Microbiol. 8:2224. doi: 10.3389/fmicb.2017.02224
Graham, E. B., Knelman, J. E., Schindlbacher, A., Siciliano, S., Breulmann, M., Yannarell, A., et al. (2016). Microbes as engines of ecosystem function: when does community structure enhance predictions of ecosystem processes? Front. Microbiol. 7:214. doi: 10.3389/fmicb.2016.00214
Haag, W. R. (2012). North American Freshwater Mussels: Natural History, Ecology, and Conservation. Available online at: https://www.srs.fs.usda.gov/pubs/49874 (accessed October 14, 2019).
Hansen, T. A. (1994). Metabolism of sulfate-reducing prokaryotes. Antonie Van Leeuwenhoek 66, 165–185. doi: 10.1007/BF00871638
Haosagul, S., Prommeenate, P., Hobbs, G., and Pisutpaisal, N. (2020). Sulfur-oxidizing bacteria in full-scale biogas cleanup system of ethanol industry. Renew. Energy 150, 965–972. doi: 10.1016/j.renene.2019.11.140
Héry, M., Singer, A. C., Kumaresan, D., Bodrossy, L., Stralis-Pavese, N., Prosser, J. I., et al. (2008). Effect of earthworms on the community structure of active methanotrophic bacteria in a landfill cover soil. ISME J. 2, 92–104. doi: 10.1038/ismej.2007.66
Hoellein, T. J., Zarnoch, C. B., Bruesewitz, D. A., and DeMartini, J. (2017). Contributions of freshwater mussels (Unionidae) to nutrient cycling in an urban river: filtration, recycling, storage, and removal. Biogeochemistry 135, 307–324. doi: 10.1007/s10533-017-0376-z
Hopper, G. W., DuBose, T. P., Gido, K. B., and Vaughn, C. C. (2019). Freshwater mussels alter fish distributions through habitat modifications at fine spatial scales. Freshw. Sci. 38, 702–712. doi: 10.1086/705666
Hopper, G. W., Gido, K. B., Vaughn, C. C., Parr, T. B., Popejoy, T. G., Atkinson, C. L., et al. (2018). Biomass distribution of fishes and mussels mediates spatial and temporal heterogeneity in nutrient cycling in streams. Oecologia 188, 1133–1144. doi: 10.1007/s00442-018-4277-1
Horton, D. J., Theis, K. R., Uzarski, D. G., and Learman, D. R. (2019). Microbial community structure and microbial networks correspond to nutrient gradients within coastal wetlands of the Laurentian Great Lakes. FEMS Microbiol. Ecol. 95:fiz033. doi: 10.1093/femsec/fiz033
Imhoff, J. F. (2015). “Rhodobacter,” in Bergey’s Manual of Systematics of Archaea and Bacteria, eds D. J. Brenner, N. Kreig, J. T. Staley, and G. M. Garrity (Berlin: Springer Verlag), 1–12.
Imhoff, J. F., TRuPER, H. G., and Pfennig, N. (1984). Rearrangement of the species and genera of the phototrophic “purple nonsulfur bacteria.” Int. J. Syst. Bacteriol. 34, 340–343. doi: 10.1099/00207713-34-3-340
Jørgensen, B. B., and Nelson, D. C. (2004). “Sulfide oxidation in marine sediments: geochemistry meets microbiology,” in Sulfur Biogeochemistry – Past and Present, eds J. P. Amend, K. J. Edwards, and T. W. Lyons (Boulder, CO: Geological Society of America), 63–81.
Joye, S. B., and Hollibaugh, J. T. (1995). Influence of sulfide inhibition of nitrification on nitrogen regeneration in sediments. Science 270, 623–625. doi: 10.1126/science.270.5236.623
Jury, W. A., and Vaux, H. (2005). The role of science in solving the world’s emerging water problems. Proc. Natl. Acad. Sci. U.S.A. 102, 15715–15720. doi: 10.1073/pnas.0506467102
Kozich, J. J., Westcott, S. L., Baxter, N. T., Highlander, S. K., and Schloss, P. D. (2013). Development of a dual-index sequencing strategy and curation pipeline for analyzing amplicon sequence data on the miseq illumina sequencing platform. Appl. Environ. Microbiol. 79, 5112–5120. doi: 10.1128/aem.01043-13
Lindgreen, S. (2012). AdapterRemoval: easy cleaning of next-generation sequencing reads. BMC Res. Notes 5:337. doi: 10.1186/1756-0500-5-337
Martinez, A. (2020). Pairwiseadonis : Pairwise Multilevel Comparison using Adonis. Available online at: https://github.com/pmartinezarbizu/pairwiseAdonis (accessed September 23, 2021).
Matthews, W. J., Vaughn, C. C., Gido, K. B., and Marsh-Matthews, E. (2005). “Southern plains rivers,” in North American Rivers, eds A. Benke and C. Cushings (Amsterdam: Elsevier), 283–325.
McArdle, B. H., and Anderson, M. J. (2001). Fitting multivariate models to community data: a comment on distance-based redundancy analysis. Ecology 82, 290–297. doi: 10.2307/2680104
McCall, P. L., Matisoff, G., and Tevesz, M. J. S. (1986). The effects of a unionid bivalve on the physical, chemical, and microbial properties of cohesive sediments from Lake Erie. Am. J. Sci. 286, 127–159. doi: 10.2475/ajs.286.2.127
Miller-ter Kuile, A., Apigo, A., and Young, H. S. (2021). Effects of consumer surface sterilization on diet DNA metabarcoding data of terrestrial invertebrates in natural environments and feeding trials. Ecol. Evol. 11, 12025–12034. doi: 10.1002/ece3.7968
Mistry, R., and Ackerman, J. D. (2018). Flow, flux, and feeding in freshwater mussels. Water Resourc. Res. 54, 7619–7630. doi: 10.1029/2018WR023112
Newton, T. J., Vaughn, C. C., Spooner, D. E., Nichols, S. J., Michael, T., and Arts, M. T. (2013). Profiles of biochemical tracers in unionid mussels across a broad geographical range. J. Shellf. Res. 32, 497–507. doi: 10.2983/035.032.0229
Nickerson, Z. L., Mortazavi, B., and Atkinson, C. L. (2019). Using functional traits to assess the influence of burrowing bivalves on nitrogen-removal in streams. Biogeochemistry 146, 125–143. doi: 10.1007/s10533-019-00612-2
Oksanen, J., Blanchet, F. G., Friendly, M., Kindt, R., Legendre, P., McGlinn, D., et al. (2020). Vegan: Community Ecology Package. Available online at: https://CRAN.R-project.org/package=vegan (accessed June 29, 2021).
Phillips, C. D., Phelan, G., Dowd, S. E., McDonough, M. M., Ferguson, A. W., Delton Hanson, J., et al. (2012). Microbiome analysis among bats describes influences of host phylogeny, life history, physiology and geography. Mol. Ecol. 21, 2617–2627. doi: 10.1111/j.1365-294X.2012.05568.x
Pierce, M. L., Ward, J. E., Holohan, B. A., Zhao, X., and Hicks, R. E. (2016). The influence of site and season on the gut and pallial fluid microbial communities of the eastern oyster, Crassostrea virginica (Bivalvia, Ostreidae): community-level physiological profiling and genetic structure. Hydrobiologia 765, 97–113. doi: 10.1007/s10750-015-2405-z
Quast, C., Pruesse, E., Yilmaz, P., Gerken, J., Schweer, T., Yarza, P., et al. (2013). The SILVA ribosomal RNA gene database project: improved data processing and web-based tools. Nucleic Acids Res. 41, D590–D596. doi: 10.1093/nar/gks1219
Quinn, T. P., Erb, I., Richardson, M. F., and Crowley, T. M. (2018). Understanding sequencing data as compositions: an outlook and review. Bioinformatics 34, 2870–2878. doi: 10.1093/bioinformatics/bty175
Rubin, B. E. R., Gibbons, S. M., Kennedy, S., Hampton-Marcell, J., Owens, S., and Gilbert, J. A. (2013). Investigating the impact of storage conditions on microbial community composition in soil samples. PLoS One 8:e70460. doi: 10.1371/journal.pone.0070460
Sinsabaugh, R. L., Hill, B. H., and Follstad Shah, J. J. (2009). Ecoenzymatic stoichiometry of microbial organic nutrient acquisition in soil and sediment. Nature 462, 795–798. doi: 10.1038/nature08632
Skelton, J., Geyer, K. M., Lennon, J. T., Creed, R. P., and Brown, B. L. (2017). Multi-scale ecological filters shape the crayfish microbiome. Symbiosis 72, 159–170. doi: 10.1007/s13199-016-0469-9
Sliva, L., and Williams, D. D. (2005). Exploration of riffle-scale interactions between abiotic variables and microbial assemblages in the hyporheic zone. Can. J. Fish. Aquat. Sci. 62, 276–290. doi: 10.1139/f04-190
Spooner, D. E., and Vaughn, C. C. (2008). A trait-based approach to species’ roles in stream ecosystems: climate change, community structure, and material cycling. Oecologia 158, 307–317. doi: 10.1007/s00442-008-1132-9
Thoemmes, M. S., and Cove, M. V. (2020). Bacterial communities in the natural and supplemental nests of an endangered ecosystem engineer. Ecosphere 11:e03239. doi: 10.1002/ecs2.3239
Trentman, M. T., Atkinson, C. L., and Brant, J. D. (2018). Native freshwater mussel effects on nitrogen cycling: impacts of nutrient limitation and biomass dependency. Freshw. Sci. 37:2. doi: 10.1086/697293
Turnbaugh, P. J., and Gordon, J. I. (2009). The core gut microbiome, energy balance and obesity. J. Physiol. 587, 4153–4158.
Turner, R. E., and Rabalais, N. N. (2003). Linking landscape and water quality in the Mississippi River Basin for 200 years. Bioscience 53, 563–572.
Umezawa, K., Kojima, H., Kato, Y., and Fukui, M. (2021). Dissulfurispira thermophila gen. nov., sp. nov., a thermophilic chemolithoautotroph growing by sulfur disproportionation, and proposal of novel taxa in the phylum Nitrospirota to reclassify the genus Thermodesulfovibrio. Syst. Appl. Microbiol. 44:126184. doi: 10.1016/j.syapm.2021.126184
van den Boogaart, K. G., and Tolosana-Delgado, R. (2008). “compositions”: a unified R package to analyze compositional data. Comput. Geosci. 34, 320–338. doi: 10.1016/j.cageo.2006.11.017
Vaughn, C. C. (2010). Biodiversity losses and ecosystem function in freshwaters: emerging conclusions and research directions. Bioscience 60, 25–35. doi: 10.1525/bio.2010.60.1.7
Vaughn, C. C., and Hoellein, T. J. (2018). Bivalve impacts in freshwater and marine ecosystems. Annu. Rev. Ecol. Evol. Syst. 49, 183–208. doi: 10.1146/annurev-ecolsys-110617-062703
Vaughn, C. C., and Spooner, D. E. (2006). Unionid mussels influence macroinvertebrate assemblage structure in streams. J. North Am. Benthol. Soc. 25, 691–700.
Vaughn, C. C., Atkinson, C. L., and Julian, J. P. (2015). Drought-induced changes in flow regimes lead to long-term losses in mussel-provided ecosystem services. Ecol. Evol. 5, 1291–1305. doi: 10.1002/ece3.1442
Vaughn, C. C., Gido, K. B., and Parr, T. B. (2021). Overlapping Fish and Mussel Hotspots Data Archive. doi: 10.17605/OSF.IO/FWJH9
Vaughn, C. C., Nichols, S. J., and Spooner, D. E. (2008). Community and foodweb ecology of freshwater mussels. J. North Am. Benthol. Soc. 27, 409–423. doi: 10.1899/07-058.1
Vaughn, C. C., Taylor, C. M., and Eberhard, K. J. (1997). “A comparison of the effectiveness of timed searches vs. quadrat sampling in mussel surveys,” in Conservation and Management of Freshwater Mussels II: Initiatives for the Future, eds K. S. Cummings, A. C. Buchanan, C. A. Mayer, and T. J. Naimo (Islans, IL: Upper Mississippi River Conservation Committee Rock), 157–162.
Weingarten, E. A., Atkinson, C. L., and Jackson, C. R. (2019). The gut microbiome of freshwater unionidae mussels is determined by host species and is selectively retained from filtered seston. PLoS One 14:e0224796. doi: 10.1371/journal.pone.0224796
Wright, S. P. (1992). Adjusted P-values for simultaneous inference. Biometrics 48, 1005–1013. doi: 10.2307/2532694
Ye, L., Amberg, J., Chapman, D., Gaikowski, M., and Liu, W. T. (2014). Fish gut microbiota analysis differentiates physiology and behavior of invasive Asian carp and indigenous American fish. ISME J. 8, 541–551.
Zeglin, L. H. (2015). Stream microbial diversity in response to environmental changes: review and synthesis of existing research. Front. Microbiol. 6:454. doi: 10.3389/fmicb.2015.00454
Keywords: freshwater mussel, microbiome, nutrient cycling, sulfur, ecosystem function, nitrogen
Citation: Higgins E, Parr TB and Vaughn CC (2022) Mussels and Local Conditions Interact to Influence Microbial Communities in Mussel Beds. Front. Microbiol. 12:790554. doi: 10.3389/fmicb.2021.790554
Received: 06 October 2021; Accepted: 24 November 2021;
Published: 13 January 2022.
Edited by:
Daniel P. R. Herlemann, Estonian University of Life Sciences, EstoniaReviewed by:
Robert W. Thacker, Stony Brook University, United StatesEric Leis, United States Fish and Wildlife Service, United States
Copyright © 2022 Higgins, Parr and Vaughn. This is an open-access article distributed under the terms of the Creative Commons Attribution License (CC BY). The use, distribution or reproduction in other forums is permitted, provided the original author(s) and the copyright owner(s) are credited and that the original publication in this journal is cited, in accordance with accepted academic practice. No use, distribution or reproduction is permitted which does not comply with these terms.
*Correspondence: Edward Higgins, aGlnZ2luc2VAb3UuZWR1