- 1RNA-based Immunotherapy, Hautklinik, Comprehensive Cancer Center Erlangen European Metropolitan Area of Nuremberg, Deutsches Zentrum Immuntherapie, Universitätsklinikum Erlangen, Friedrich-Alexander-Universität Erlangen-Nürnberg, Erlangen, Germany
- 2Systems Tumor Immunology, Hautklinik, Universitätsklinikum Erlangen, Friedrich-Alexander-Universität Erlangen-Nürnberg, Comprehensive Cancer Center Erlangen European Metropolitan Area of Nuremberg, Deutsches Zentrum Immuntherapie, Erlangen, Germany
- 3Hautklinik, Universitätsklinikum Erlangen, Friedrich-Alexander-Universität Erlangen-Nürnberg, Comprehensive Cancer Center Erlangen European Metropolitan Area of Nuremberg, Deutsches Zentrum Immuntherapie, Erlangen, Germany
Merkel cell carcinoma (MCC) is a rare and highly aggressive cancer, which is mainly caused by genomic integration of the Merkel cell polyomavirus and subsequent expression of a truncated form of its large T antigen. The resulting primary tumor is known to be immunogenic and under constant pressure to escape immune surveillance. Because interferon gamma (IFNγ), a key player of immune response, is secreted by many immune effector cells and has been shown to exert both anti-tumoral and pro-tumoral effects, we studied the transcriptomic response of MCC cells to IFNγ. In particular, immune modulatory effects that may help the tumor evade immune surveillance were of high interest to our investigation. The effect of IFNγ treatment on the transcriptomic program of three MCC cell lines (WaGa, MKL-1, and MKL-2) was analyzed using single-molecule sequencing via the Oxford Nanopore platform. A significant differential expression of several genes was detected across all three cell lines. Subsequent pathway analysis and manual annotation showed a clear upregulation of genes involved in the immune escape of tumor due to IFNγ treatment. The analysis of selected genes on protein level underlined our sequencing results. These findings contribute to a better understanding of immune escape of MCC and may help in clinical treatment of MCC patients. Furthermore, we demonstrate that single-molecule sequencing can be used to assess characteristics of large eukaryotic transcriptomes and thus contribute to a broader access to sequencing data in the community due to its low cost of entry.
Introduction
Merkel cell carcinoma (MCC) is a rare and frequently metastatic neuroendocrine skin cancer with increasing incidence and high mortality (Fitzgerald et al., 2015; Paulson et al., 2018). Risk factors are increased age, UV light exposure, immunosuppression, and infection with the human Merkel cell polyomavirus (MCPyV; Heath et al., 2008). About 80% of all MCC tumors contain a mutated MCPyV genome that expresses viral oncoproteins (T antigens) (Feng H. et al., 2008; Houben et al., 2010). For malignant transformation, the MCPyV DNA is clonally integrated into the MCC genome in a replication-deficient form and expresses a truncated oncoprotein, called the large T (LT) antigen (Shuda et al., 2008). As this protein contains several tumor suppressors targeting motifs such as the retinoblastoma binding site, it promotes cell cycle progression that leads to uncontrolled cell proliferation (Borchert et al., 2014; Hesbacher et al., 2016). MCC is also characterized by an increased expression of programmed cell death protein 1 (PD-1), which allows the tumor cells to escape the control of the immune system (Afanasiev et al., 2013). Since 2018, checkpoint inhibitors (targeting PD-1 and its ligand PD-L1) have been approved by the Food and Drug Administration for the treatment of advanced MCC and show impressive clinical results, albeit not all patients respond to this treatment (Kaufman et al., 2016; Nghiem et al., 2016). It is suggested that MCC, as an immunogenic tumor, exerts different strategies of immune escape because it is capable of disturbing cellular immune responses by upregulation of PD-1 and PD-L1 and reducing the human leukocyte antigen (HLA) class I expression, leading to decreased or absent T cell infiltration (Ritter et al., 2017). The exact mechanism of immune escape is of high clinical relevance especially regarding immunotherapy resistance; however, it is not fully understood yet.
Interferons (IFNs) are suggested to beneficially influence antiviral immune responses and possess anti-polyomavirus (Co et al., 2007; Willmes et al., 2012) and anti-MCC activity (Krasagakis et al., 2008; Willmes et al., 2012), which makes them an interesting study subject in MCC. The type II IFN gamma (IFNγ) is of special interest, as it is well known for its ambiguous effects: Both anti-tumoral and pro-tumoral functions of IFNγ have been reported.
A closer investigation of the anti-tumoral effects shows that IFNγ is produced by activated lymphocytes including CD4+ and CD8+ T cells (Kasahara et al., 1983; Matsushita et al., 2015), gamma delta T cells (Gao et al., 2003), natural killer (NK) cells (Cooper et al., 2001; Yu et al., 2006), and NK T cells (Leite-De-Moraes et al., 1998). IFNγ plays a key role in modulating the immune response toward these effector cells in the tumor microenvironment. Its anti-tumor activity has been shown for fibrosarcoma and melanoma by the induction of HLA-I and signal transducer and activator of transcription 1 (STAT1)–associated cyclin-dependent kinase, leading to apoptosis of the tumor cells and recognition by the immune system (Ikeda et al., 2002; Gao et al., 2016). For MCC cell lines, it was shown that incubation with IFNγ slightly inhibited cell viability, reduced the expression of the MCPyV LT antigen (Willmes et al., 2012), and increased HLA-I expression (Paulson et al., 2014).
In contrast, pro-tumoral effects of IFNγ have been observed in colon carcinoma and melanoma, leading to a loss of IFNγ signaling sensitivity, reduced antigen expression (Beatty and Paterson, 2001), and induction of key immune suppressive molecules such as indoleamine-2,3-dioxygenase (IDO1) (Spranger et al., 2013) and immune checkpoint proteins (Benci et al., 2016). Hence, the same IFNγ signaling processes that promote anti-tumor immunity can achieve the opposite effect by inducing feedback inhibition and tumor immune escape (Bald et al., 2014).
To better understand the biology of a tumor and improve targeted therapies, high-throughput sequencing has been highly beneficial. The analysis of gene expression profiles of melanoma patients with checkpoint inhibitor treatment detected IFNγ-related mRNA profiles, which predicted clinical response to PD-1 treatment, underlining the huge clinical benefit of transcriptome sequencing studies (Ayers et al., 2017). Nanopore sequencing by Oxford Nanopore Technologies (ONT) belongs to the third-generation sequencing methods and enables real-time DNA and RNA sequencing using small nanopores (Jain et al., 2015, 2016). In this system, the DNA or RNA strand is guided through the nanopore creating nucleotide sequence-specific disturbance of the flow of an electrical current (Ip et al., 2015). In this process, up to 2 × 106 bases can be read continuously (Payne et al., 2019). Nanopore sequencing shows increasing popularity in the field of cancer biology (Euskirchen et al., 2017) and virology (Quick et al., 2016). It is characterized by relatively low establishment and maintenance costs as well as rapid sequencing and real-time data acquisition for further analysis.
As the comprehensive investigation of the transcriptomic response of MCC to IFNγ is of high interest, we used the Nanopore sequencing platform to study differentially expressed genes in MCC. We hypothesized that IFNγ stimulation of MCC cell lines would lead to transcriptomic alterations that elucidate the ambiguous pro- and anti-tumoral activity of this cytokine and hence examined the effect of IFNγ on the transcriptomes of three MCPyV-positive cell lines (WaGa, MKL-1, and MKL-2). Our results confirm the Nanopore sequencing technology as a suitable tool to study these alterations and show a clear upregulation of genes involved in tumor immune escape mechanisms, suggesting that these factors could contribute to the immune escape of MCC cells.
Materials and Methods
Cell Culture
The MCC cell lines WaGa (RRID:CVCL_E998) (Houben et al., 2010), MKL-1(RRID:CVCL_EQ70) (Rosen et al., 1987), and MKL-2 (RRID:CVCL_D027) (Martin et al., 1991) were grown in Roswell Park Memorial Institute 1640 medium (Lonza, Verviers, Belgium) supplemented with 10% fetal bovine serum (FBS) (Merck KGaA, Darmstadt, Germany), 2 mM L-glutamine (Lonza), gentamycin (20 mg/L; Lonza), 10 mM hydroxyethyl piperazineethanesulfonic acid (HEPES; PAA Labortechnik, Pasching, Austria), and 20 mM beta-mercaptoethanol (Gibco) and maintained at 37°C in 5% CO2. All cells were kindly provided by Prof. Jürgen Becker (DKTK, Essen, Germany), and mycoplasma testing was performed immediately after arrival of the cells. Cells were frozen and then thawed for further experiments, whereby they were cultured for 1 to 3 weeks before the experiments were performed.
Interferon Gamma Incubation and RNA Isolation of Cell Lines
For RNA extraction, 5 × 106 cells of each cell lines were seeded at six-well plates, and IFNγ (3,000 U/ml; PeproTech, Hamburg, Germany) was added in each cell suspension. Cells incubated without IFNγ served as control. After 24 h of incubation, cell pellets were stored at −80°C until further analysis. For each cell line, the IFNγ incubation was performed three times on different days to obtain three replicates and exclude any daily fluctuation.
From frozen cell pellets, RNA isolation was performed using the AllPrep DNA/RNA micro kit (Qiagen, Venlo, Netherlands). RNA concentration was measured via Nanodrop and stored at −20°C until library preparation.
Flow Cytometry Detection of Intracellular and Extracellular Markers
For antibody staining, 1 × 106 cells of each MCC cell line were cultured in a six-well plate in the presence or absence of IFNγ (3,000 U/ml) for 72 h. Cells were washed with fluorescence-activated cell sorting (FACS) buffer (dulbecco’s phosphate-buffered saline with 1% FBS and 0.2% sodium azide) and stained for the following markers: STAT1α/β, Indoleamine-2,3-dioxygenase (IDO), C-X-C motif chemokine 10 (CXCL10), PD-L1, bone marrow stromal antigen 2 (BST2), HLA class II histocompatibility antigen gamma chain (CD74), and HLA A, B, and C (HLA-ABC) with the following monoclonal antibodies: anti-human STAT1 (phycoerythine; PE, clone REA272, Miltenyi Biotec), anti-IDO1 (PE, clone eyedio, Invitrogen), anti-human CXCL10 (PE, clone REA334, Miltenyi Biotec), anti-CD274 (Pe-Cy7, clone MIH1, BD Biosciences), anti-human BST2 (PE, clone REA202, Miltenyi Biotec), anti-CD74 (PE, clone 5-329, Miltenyi Biotec), and anti–HLA-ABC (fluorescein-5-isothiocyanat, BD Biosciences). STAT1, IDO, CXCL10, and CD74 were stained intracellularly using the Cytofix/Cytoperm™ Fixation/Permeabilization Solution Kit (Thermo Fisher Scientific, Waltham, MA, United States). PD-L1, BST2, HLA-ABC, and CD74 were stained as surface molecule. Stained cells were analyzed using a FACSCanto II flow cytometer (BD Biosciences) and FlowJo software, version 10.5.3 (BD Biosciences). Geometric mean of the mean fluorescence intensity was compared between ± IFNγ-treated cell lines.
Preparation of Cell Lysates and Immunoblotting of the Merkel Cell Polyomavirus–Large T Antigen
Cell lysates were prepared out of 1 × 106 cells for each cell line (WaGa, MKL-1, and MKL-2), incubated with or without IFNγ (3,000 U/ml) for 72 h. Cell pellets were frozen at −80°C, thawed, and resuspended in 35 μl of lysis buffer [10% glycerol, 2 mM ethylenediaminetetraacetic acid (EDTA), 137 mM NaCl, 50 mM Tris (pH 8.0) (all from Carl Roth GmbH, Germany), 0.5% sodium deoxycholate (Merck KGaA, Darmstadt, Germany)] freshly supplemented with 2 mM phenylmethylsulfonyl fluoride (Carl Roth GmbH, Germany), 2 mM sodium orthovanadate (Merck KGaA, Darmstadt, Germany), 20 mM sodium fluoride (Merck KGaA, Darmstadt, Germany), 0.1 M MgCl2 (Carl Roth GmbH, Germany), and 0.1 μl of benzonase (250 U/μl, Merck KGaA, Darmstadt, Germany) and lysed on ice for 20 min. Cells were then centrifuged at 13,500 × g at 4°C for 20 min, supernatants were harvested, and the protein concentration in each lysate was determined using Bradford protein determination. Protein lysates were mixed with 4× Roti-load 1 (Carl Roth GmbH, Germany), followed by a denaturation step at 95°C for 10 min.
Per lane, 20 μg of protein lysate of the three MCC cell lines were loaded onto 12% sodium dodecylsulfate (SDS) polyacrylamide gels and separated using SDS-polyacrylamide gel electrophoresis (PAGE). Afterward, proteins were transferred onto a nitrocellulose membrane by semi-dry blot transfer. After blocking the membrane in 1× Roti-block (Carl Roth GmbH, Germany) for 1 h at room temperature, the membrane was incubated with anti-MCPyV LT antigen (clone CM2B4, Merck KGaA) diluted 1:1,000 in 1× Roti-block overnight at 4°C and with the horseradish peroxidase-conjugated secondary antibody (polyclonal anti-mouse immunoglobulin G; Cell Signaling, 1:2,500) for 1 h. The antibodies were detected via enhanced chemoluminescence (ECL) using Amersham ECL Prime Western blotting detection reagent (GE Healthcare, Solingen, Germany) and documented and quantified via the Amersham ImageQuant system (GE Healthcare). glyceraldehyde-3-phosphate dehydrogenase (GAPDH) (anti-GAPDH mouse monoclonal Ab, clone 6C5, Merck KGaA, Darmstadt, Germany) was used as a loading control.
Library Preparation and Nanopore Sequencing
Reagents and equipment for preparation and sequencing were obtained from ONT, Oxford, United Kingdom, unless stated otherwise. Library preparation was performed according to the instructions of the manufacturer using the PCR-cDNA Barcoding Kit (SQK-PCB109, ONT). Polyadenylated mRNA was reversely transcribed into cDNA and fused to barcodes (BCs) to allow sample-specific attribution. Of each RNA sample, 50 ng were used for library preparation. In total, six samples were loaded onto one flow cell with three biological replicates for each condition. Assigned BCs used were 1 through 6 for MKL-1 and 1, 2, 4, 5, 6, and 7 for both MKL-2 and WaGa.
Libraries were run for 72 h according to the guidelines of the manufacturer using the MK1B-MinION sequencer and R9.4 flow cells (FLO-MIN106, ONT). For each cell line, six samples (three replicates incubated with IFNγ and three replicates incubated without IFNγ) were loaded on one flow cell. Sequencing run monitoring and real-time data acquisition were performed using the MinKNOW software suite (v19.2.5). After real-time data were gathered, a high-accuracy base calling was performed using the CPU version of Guppy (v.4.2.2) deployed on a dedicated server, using the following command:
guppy_basecaller -r -i “fast5” -s
“sample” —num-callers \
1 –trim-barcodes –cpu_threads_per_caller 64 \
––c dna_r9.4.1_450bps_hac.cfg \
––barcode-kits SQK-PCB109 -q 0
Data Processing
After a high-accuracy base calling was performed, generated FASTQ files were aggregated per sample and filtered for average Phred score quality above 7 (Q>7). In addition, primary quality assessment was done with the package pycoQC (Leger and Leonardi, 2019). For raw data processing, we made use of the snakemake pipeline provided by Oxford Nanopore (available at1). The pipeline performs read alignment, quantification, and differential expression analysis as well as abundance estimation in transcripts per million. For the detailed version of the environment used, see “Supplementary Material 1 versions.txt”. As a reference genome, we used the transcriptome cDNA reference provided by ensemble (Homo_sapiens.GRCh38.cdna.all.fa.gz, release 103). To quantify LT antigen expression, we added the sequence of the LT cDNA (GenBank: LC148302.1) to the assembly. As annotation, we used the corresponding GTF file where the LT antigen was added as an additional entry. For detailed sequence information, see Supplementary Figure 1 for the sequence.
Differential Expression and Pathway Enrichment Analysis
Raw counts of the expressed transcripts were collected from the output of the snakemake pipeline. Further analysis was performed using the Bioconductor Package DESeq2 in R (Love et al., 2014); the code and data to perform the analysis were deposited at Zenodo under the DOI: 10.5281/zenodo.5031363. Because an exploratory approach was taken, we choose to set significance threshold to adjusted p-value < 0.1. P-values were adjusted using false discovery rate correction. Pairwise comparisons were performed between conditions (untreated and per flow cell and once across flow cell and cell lines). Gene set enrichment analysis was performed using the Bioconductor package fgsea (Sergushichev, 2016) with the C7 immune signature hallmarks, gene set version 7.2, by the Molecular Signatures Database (Subramanian et al., 2005; Liberzon et al., 2015).
Mapping of Different Human Leukocyte Antigen Alleles
To quantify expression of the six known HLA alleles of each cell line, we implemented a two-pass quantification procedure, which quantifies transcriptome wide expression in the first pass and then uses prior knowledge to estimate allele specific HLA expression in the second pass. The data were then aggregated and analyzed. This allowed us to leverage the allele specific reference sequences (Robinson et al., 2019) and to mitigate the high error rates in nanopore data. In the first step, the known HLA alleles of each cell line were used separately to create a reference FASTA file, encompassing all described sequence variants of these alleles. We then mapped generated FASTQ files against this reference while also only counting primary alignments of a read. Afterward, read counting was performed for all reads with a sequence identity of 80% to the reference. This was done to achieve a conservative expression estimate and reduce false positive mappings. We further aggregated all reads mapping to the variants to a four-digit resolution, because higher genotyping resolutions are not known for the cell lines. In a second step, we gathered count data from previous mapping runs against the human genome reference and recalculated transcripts per million (TPM) with the counts generated in the first step. We thus arrive at a conservative estimate of the expression of HLA alleles in the investigated cell lines.
Statistical Analysis
All graphs and statistical analysis for flow cytometry experiments were created by GraphPad Prism, version 8 (GraphPad Software, La Jolla, United States). The paired student’s t-test was used to compare intracellular and surface markers between cell lines treated with or without IFNγ as well as for the analysis of Western blot data. Data are reported as the median with standard error of the mean (SEM). Significance was determined as p-value ≤ 0.05.
Results
Interferon Gamma Increases Human Leukocyte Antigen Class I and PD-L1 in all Cell Lines, but Not Indoleamine-2,3-dioxygenase
To investigate the effect of IFNγ on important key players of the immune response, we measured the expression of (i) the HLA class I, whose loss can be a primary immune escape mechanism; (ii) PD-L1 as an important target for checkpoint inhibitors; and (iii) IDO as a key immunosuppressive enzyme. Antibody staining and FACS analysis of all three MCC cell lines were performed after 72-h incubation with IFNγ and as control without IFNγ. HLA class I was significantly upregulated in all three cell lines, whereas WaGa and MKL-2 cells displayed a stronger increase of HLA-ABC than MKL-1 cells (Figure 1). An increased expression of PD-L1 due to IFNγ was observed in all cell lines, but only for MKL-1 and MKL-2 with statistical significance. Staining for IDO showed no changes of the IDO status between the cell lines with and without IFNγ treatment. This indicates that all three cell lines are responsive to the cytokine but react differently to the treatment.
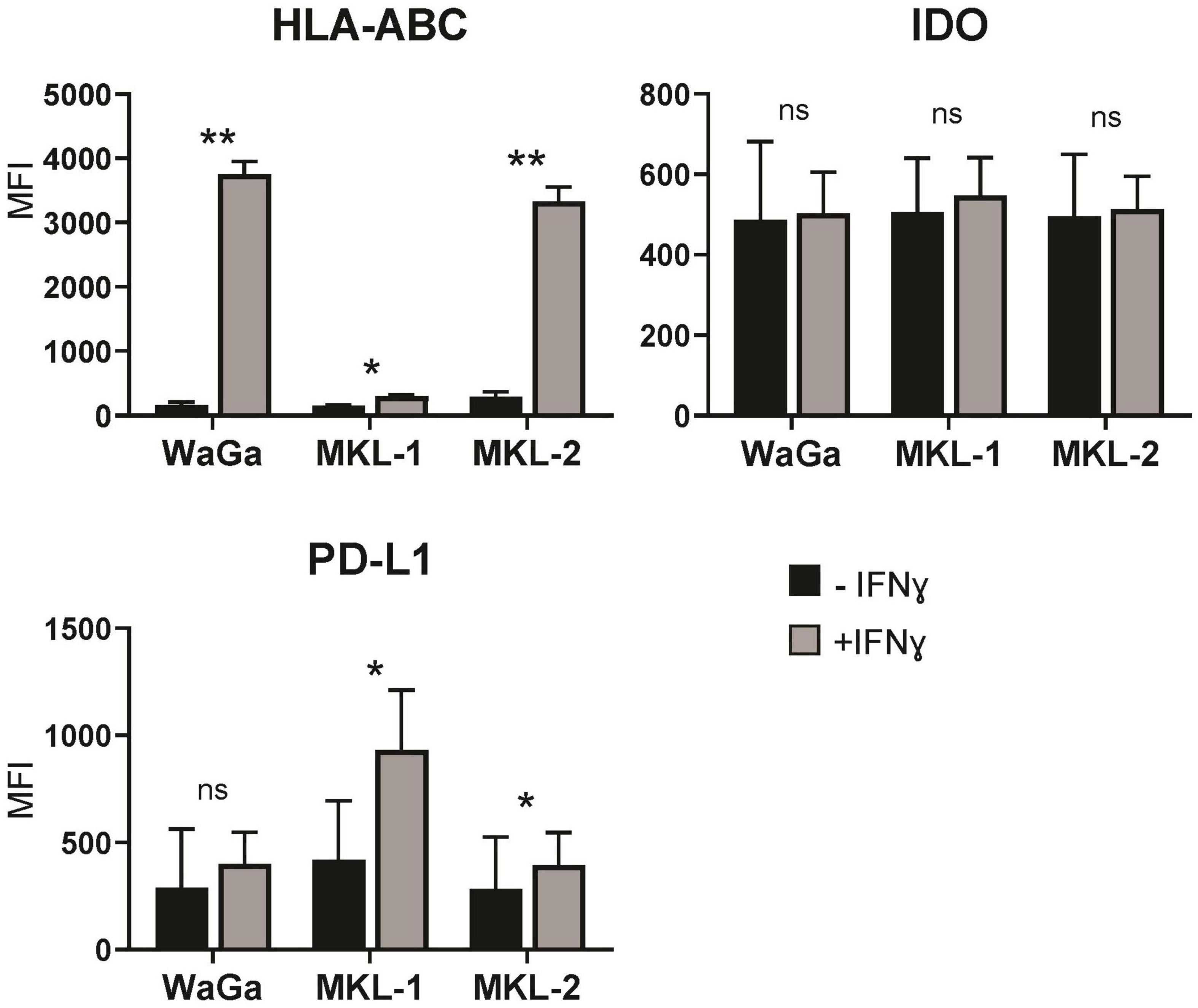
Figure 1. Merkel cell carcinoma cell lines respond to IFNγ by upregulating different proteins. After 72 h of treatment with or without IFNγ (3,000 U/ml), the Merkel cell carcinoma cell lines MKL-1, MKL-2, and WaGa were stained for human leukocyte antigen A, B, and C (HLA-ABC), indoleamine-2,3-dioxygenase (IDO), and programmed cell death 1 ligand 1 (PD-L1) and analyzed by flow cytometry. The average mean fluorescent intensity (MFI) of three to four independent experiments ± standard error is indicated. p-values were determined using the paired student’s t-test. *p < 0.05; **p < 0.01; ns, not significant.
Sequencing Runs
To comprehend the different transcriptional programs induced in the different cell lines by IFNγ, we sequenced RNA of the three MCC cell lines (MKL-1, MKL-2, and WaGa) via the Nanopore sequencing technology. Before RNA isolation, the cells were incubated with or without IFNγ for 24 h. During the sequencing runs, MKL-1 and WaGa produced comparable base pair (bp) outputs with 11.68 Gigabases (Gb) and 9.40 Gb after high-accuracy base calling. The experiments had similar pore counts available, at sequencing start with 1,446 for MKL-1 and 1,489 for WaGa. The flow cell dedicated for MKL-2 performed worse with only producing 3.29 Gb in total although similar pores were available for sequencing (1,480) and all experimental parameters equal but age of flow cell (Figure 2A). Overall, all three experiments generated high-quality Phred scores and high read lengths over their runtime, with MKL-1 producing a median read length of 1,217 bp and a median Phred(Q) of 11.98, and MKL-2 producing median read length of 630 bp and a median Q of 11.59. Finally, WaGa produced median read lengths of 683 bp and a median Q of 11.49. BC read yield showed biases in efficiency of each multiplexing BC. As for MKL-1, we observed a failure of BC 3 with no reads being generated from this BC. Of the remaining BCs, BC 6 performed best in total output. In further runs, we skipped BC 3 and assigned no sample to it. For MKL-2, BCs 1, 2, and 4, all assigned to the control group, showed good performance with BC 1, producing the best yield. In the treated group (BCs 5, 6, and 7), however, BC 6 again produced most reads with BC 5 and BC 7 almost failing. WaGa showed similar results, with the first three BCs (1, 2, and 4) performing equally well with BC 5 and BC 7, producing only 1% of reads. In addition, in this experiment, we had about 0.3% of erroneously assigned BC 8 detected. Unclassified counts were consistently low for all samples ranging from 0.8 to 2.0% of reads passing principal quality control (Figure 2B). Overall, Nanopore sequencing allowed for the quick and robust gathering of transcriptomics data from our samples. However, it is of note that some aspects like differentially BC efficacy and flow cell sensitivity are still a considerable factor.
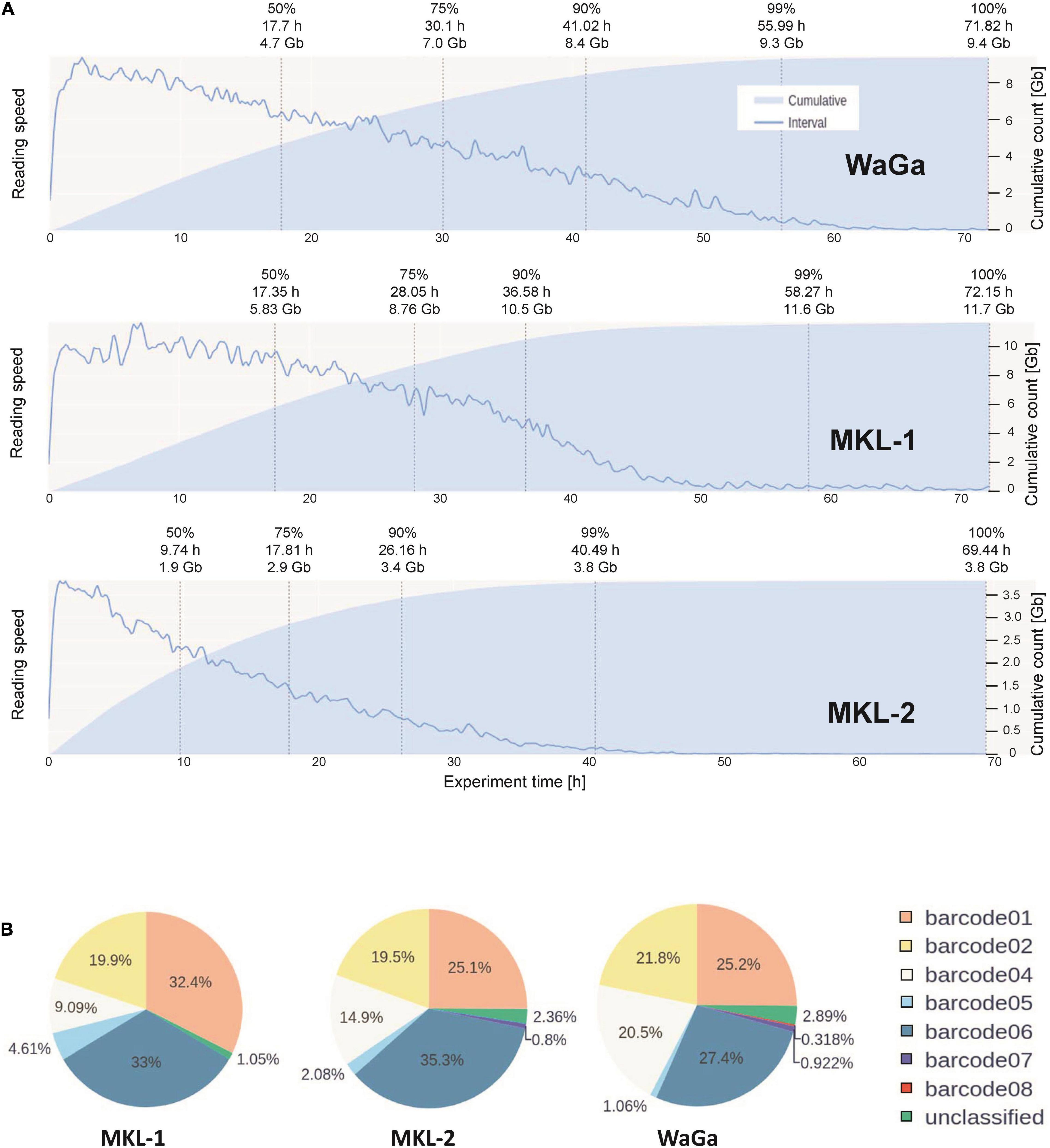
Figure 2. Functional performance of the Nanopore flowcells and barcodes. (A) Depiction of output over time of MKL-1, MKL-2, and WaGa cells. For each cell line, a new flow cell was loaded with the multiplexed library. The sequencing run lasted 72 h. The blue line indicates relative reading speed, i.e., bases per time, the light blue area represents cumulative reading output. (B) Barcode distribution of detected barcodes during the sequencing run of the three cell lines MKL-1, MKL-2, and WaGa. Barcode 3 is not displayed as it failed in the first run of MKL-1 and was skipped for further experiments. In the run of WaGa, we additionally tested barcode 8, which failed similar as barcode 3. The other barcodes (1, 2, 4, 5, 6, and 7) could be used according to their performance for multiplexing studies.
Differential Gene Expression
Our primary goal was to detect differentially expressed genes in the MCC cell lines after treatment with IFNγ. Therefore, we performed a differential expression analysis of the Nanopore sequencing data using raw counts produced by our referenced pipeline. First, we pooled counts of all three cell lines, treating them as biological replicates, and corrected for batch effects. At an adjusted p-value < 0.1 and an absolute fold change >2, we could detect 61 genes that were differentially expressed (Supplementary Figure 1). To check for plausibility and to further investigate the role of the differentially expressed genes in terms of cancer biology, we performed a pathway enrichment analysis. Several pathways such as the inflammatory response pathway and the interleukine 6-janus kinase-signal transducer and activator of transcription (IL6-JAK-STAT3) pathway were enriched in the presence of IFNγ. Seven hallmark pathways were slightly downregulated, but most importantly, genes involved in IFNγ and IFNα pathways were induced (Figure 3A). Because these two pathways overlap to a large degree, this indicates that the data are sound and plausible.
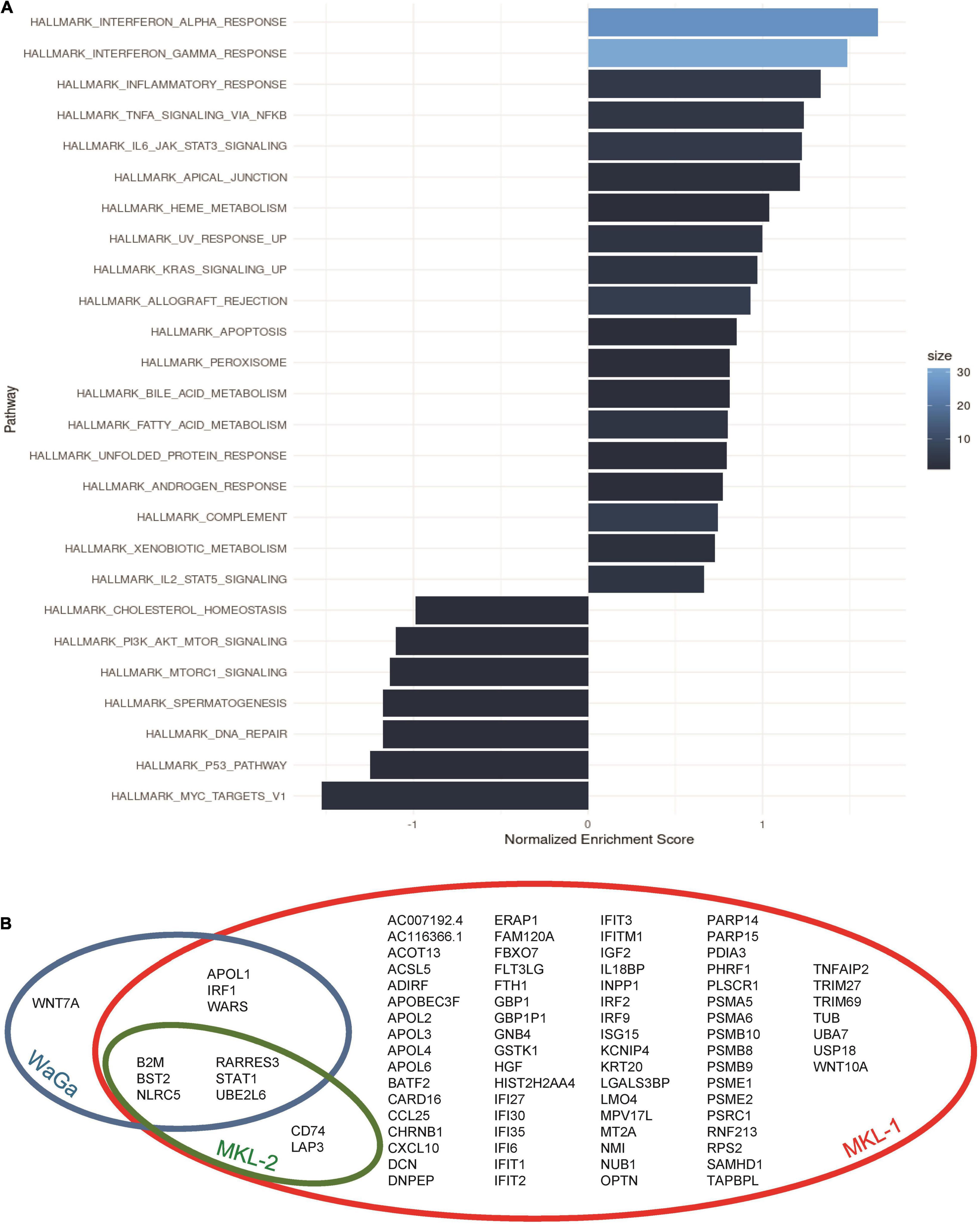
Figure 3. Pathway annotation and distribution of differentially expressed genes. (A) Hallmark pathway enrichment analysis of all used MCC cell lines (MKL-1, MKL-2, and WaGa): Data were pooled for the three cell lines in one plot. Gene set enrichment analysis was performed using the Bioconductor package fgsea with the immune signature hallmarks by Molecular Signatures Database. Size corresponds to gene count of how many expressed genes from the input overlap with the pathway. (B) The Venn diagram of the three cell lines gives an overview over shared differentially expressed genes between the cell lines MKL-1 (red circle), MKL-2 (green circle), and WaGa (blue circle).
To differentiate between the common IFNγ-induced program and a cell line–specific program, we investigated the cell lines individually and found 86 differentially expressed genes in cell line MKL-1, eight genes in MKL-2, and 10 genes in WaGa (Figure 2B). Six of these genes (UBE2L6, BST2, NLRC5, RARRES3, B2M, and STAT1) were differentially regulated in all three cell lines (Figure 3B). Furthermore, there was an overlap of two genes (CD74 and LAP3) between MKL-1 and MKL-2, whereas WaGA and MKL-1 showed differential expression for three genes (IRF1, WARS, and APOL1). Between WaGa and MKL-2, no individual overlap of differential gene expression could be detected. One gene (WNT7A) was differentially expressed uniquely in WaGa, whereas 74 of all detected genes were unique in MKL-1 and none for MKL-2. Obviously, the regulation (up- or downregulation) of each differentially expressed gene was of special interest. Thus, we performed a detailed examination of gene expression for each cell line in form of heatmaps (Figure 4) and volcano plots (Supplementary Figure 2). Most but not all differentially expressed genes were upregulated; WNT7A, HGF, PARP15, FAM120A, and TUB were significantly reduced in the presence of IFNγ.
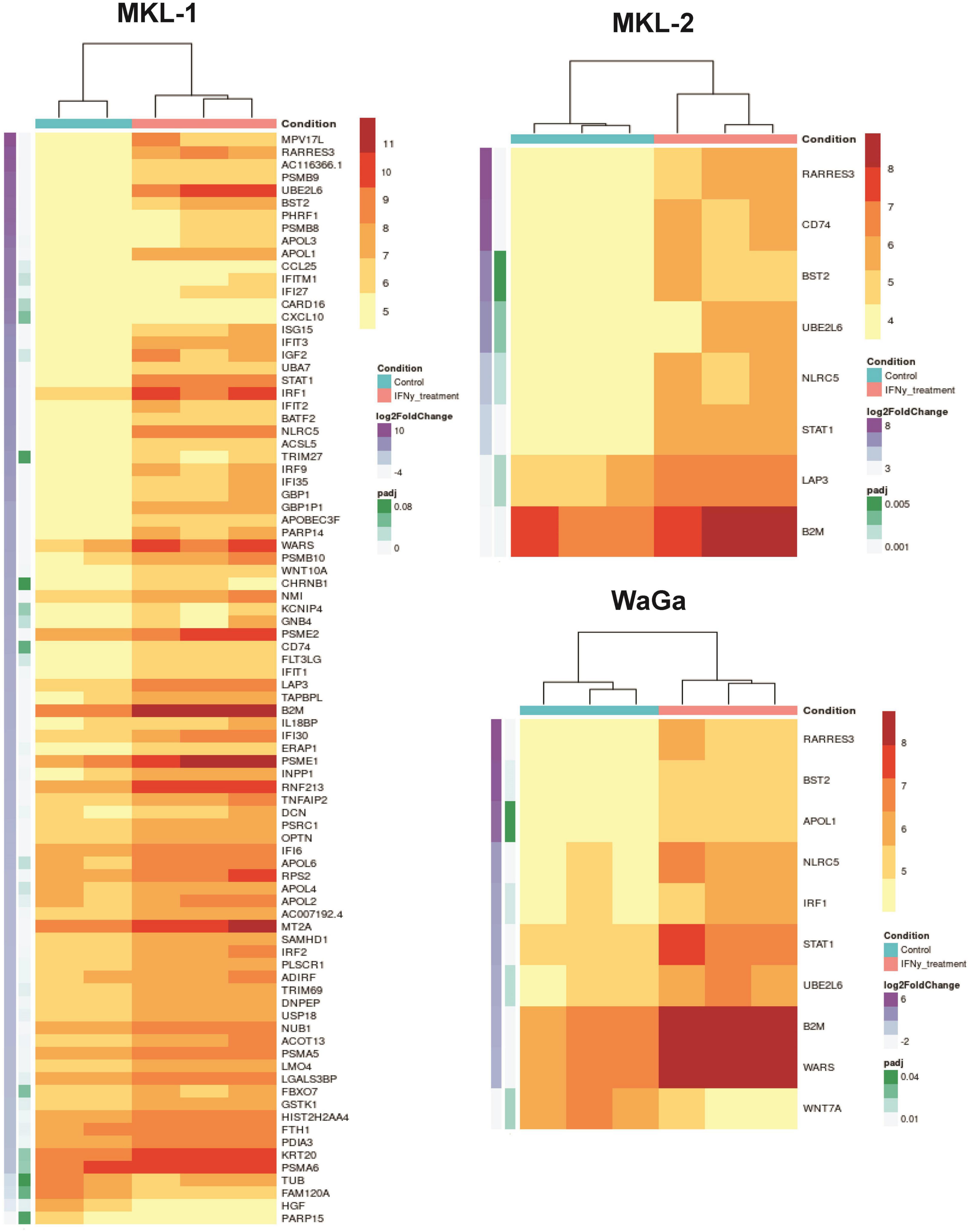
Figure 4. Differentially expressed genes of the cell lines MKL-1, MKL-2, and WaGa illustrated in heatmaps. Cell lines were incubated with IFNγ (blue top labels) or without IFNγ (pink top labels). Adjusted p-values are indicated in green and log2 fold change in purple on the left side. Listed genes were differentially expressed with a false discovery rate-adjusted p-value < 0.1 and an absolute fold change >2. A total of 86 genes was detected in MKL-1, eight genes in MKL-2, and 10 genes in WaGa cells. Heat scale represents vst-normalized counts using DESeq2.
The next step was to examine the role of the identified genes in the context of MCC and tumor biology. By manual annotation, we categorized the genes in the following groups according to their regulation, which we observed in the sequencing data: anti-tumoral genes, pro-tumoral genes, immune escape genes, and MCC-related genes (see Table 1).
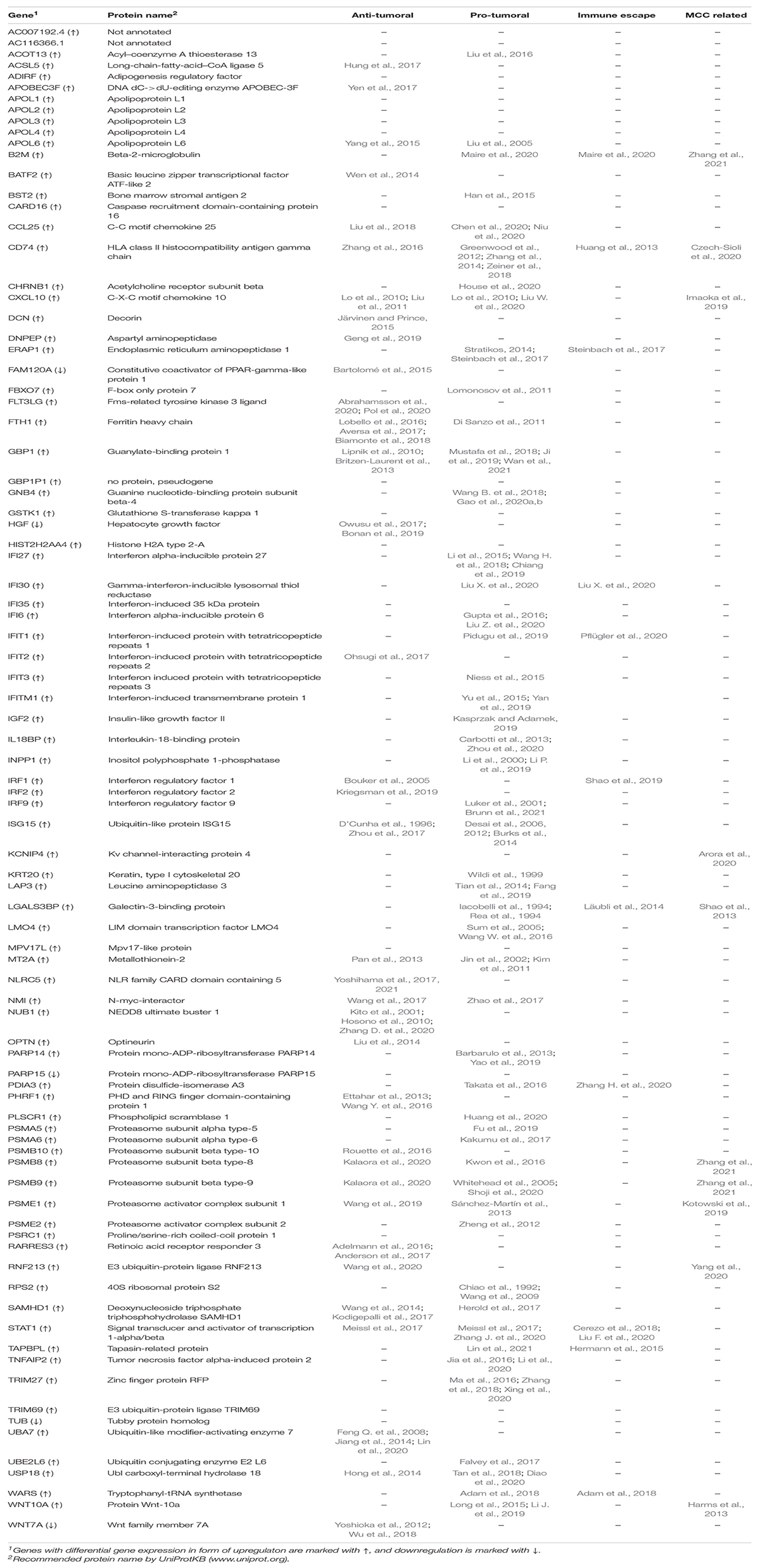
Table 1. Overview of all differentially expressed genes categorized according to their activity in cancer biology.
According to this classification, we detected 35 genes associated with anti-tumoral effects (e.g., resulting in an increase in the numbers of infiltrating lymphocytes or inhibition of tumor cell growth). In contrast, we found 49 genes associated with pro-tumoral effects (e.g., genes supporting tumor growth or increasing survival and migration of cancer cells). A lot of these pro-tumoral genes overlapped with the group of potential tumor immune escape genes, of which 11 genes showed significantly increased gene expression under IFNγ treatment: CD74, STAT1, B2M, IRF1, WARS, IFI30, TAPBPL, IFIT1, PDIA3, ERAP1, and LGALS3BP. Of note, in some cases, anti-tumoral genes were downregulated resulting in a pro-tumoral effect or, on the contrary, the expression of pro-tumoral genes was decreased leading to anti-tumoral activity. In addition, we found 14 genes, which could have both anti- and pro-tumoral activity. Which kind of effect these genes actually had was often cell-type or cancer-type dependent. With respect to the MCC-related genes, we identified 10 genes that were already known to play a role in MCC, such as genes involved in the integration site of the MCPyV or acting as predictive biomarker. Of these genes, B2M was the only one that was differentially expressed in all three cell lines while CD74 expression was altered in MKL-1 and MKL-2, whereas all other genes related to MCC were regulated by IFNγ to MKL-1 alone.
Human Leukocyte Antigen Expression Is Increased on mRNA Level by Interferon Gamma
The relatively high error rate of Nanopore sequencing results in poor mapping of the individual reads in the HLA regions of the reference genomes. Hence, we implemented a two-pass quantification procedure to exploit the up-front knowledge about the HLA-haplotypes of the used cell lines. With this two-pass quantification step, we were able to verify the upregulation of HLA-specific expression following an IFNγ treatment in the three MCC cell lines (Figure 5). The calculated TPM values of the six different HLA alleles were considerably higher for the IFNγ-treated group across all three cell lines, despite slight differences in single replicates or subtypes. However, because the different approaches on how read counts were generated, the data were not included in the statistical analysis of the whole transcriptomes described above. Instead, we decided to provide a separate estimate of the allele expression to make clear that these are two different avenues of analysis.
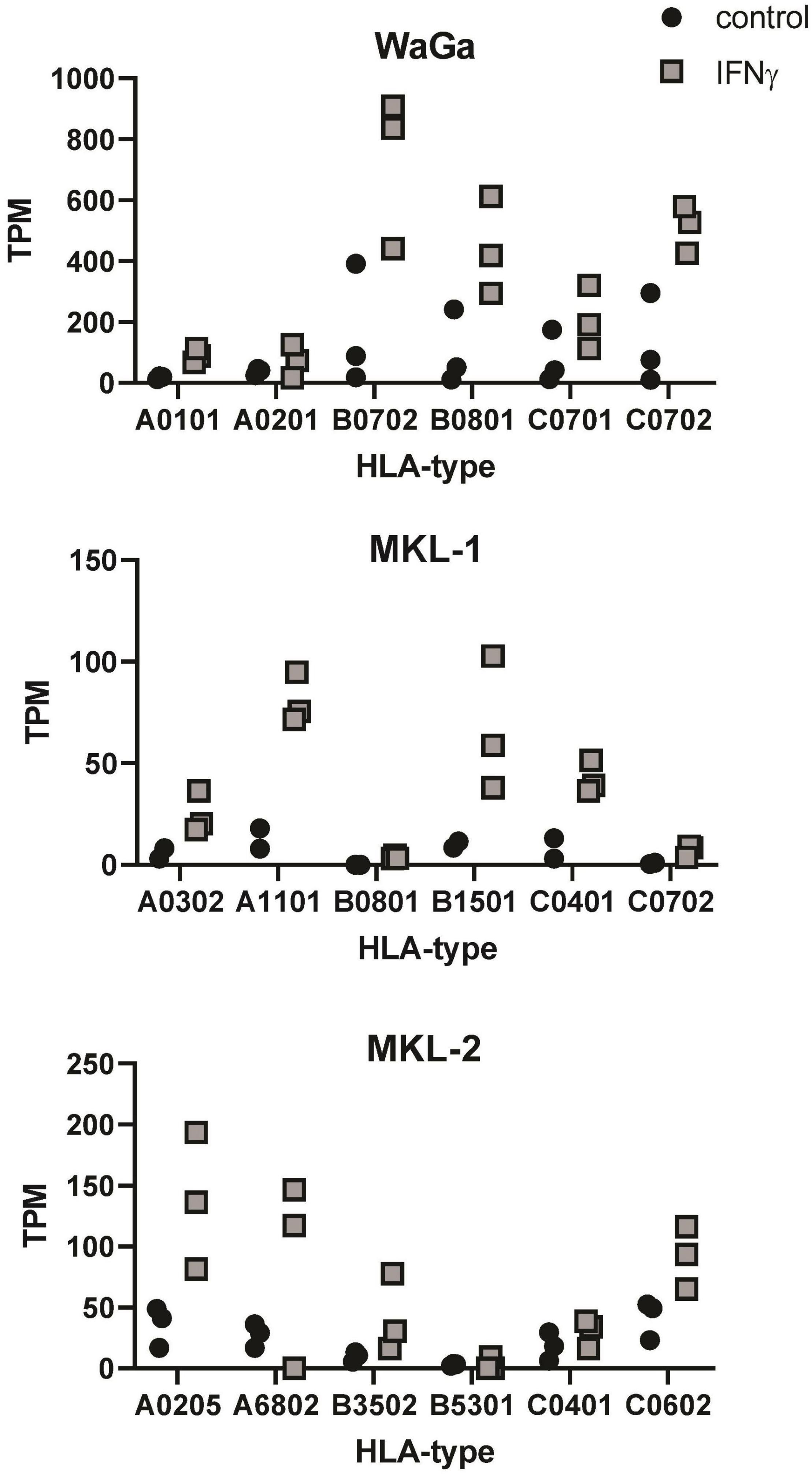
Figure 5. Detection of HLA expression after IFNγ incubation on mRNA level. Transcripts per million (TPM) values of six different HLA alleles after IFNγ treatment (gray squares) or without IFNγ incubation (black dots) are depicted for each MCC cell line. TPM values were acquired from our sequencing output using a new two-pass quantification method. For each cell line, three independent experiments were performed (two for MKL-1 control), so that each symbol represents one replicate of the respective cell line.
The relatively high error rate of Nanopore sequencing reads results in ubiquitous mapping of reads between loci that share a high degree of sequence similarity. This especially presents an issue with the HLA loci, because there is considerable sequence homology between the alleles and supertypes (HLA-A, B, and C). We found that, by using standard alignment methods, it is hard to allocate reads to the correct allele and thus decided to implement our two-pass quantification procedure, making use of the prior knowledge of the alleles present in our cell lines of interest.
Validation of Differential Gene Expression on Protein Level
To confirm our transcriptomic findings (Figure 6A) on protein level, we exemplarily studied four differentially expressed genes (STAT1, BST2, CXCL10, and CD74) using flow cytometry (Figure 6B). Therefore, the three MCC cell lines were incubated with IFNγ (3,000 U/ml) for 72 h, and, afterward, antibody staining of the selected genes was performed. We chose genes that were differentially expressed on mRNA level in all three cell lines (STAT1 and BST2) and genes that were upregulated in only one (CXCL10 in MKL-1) or two (CD74 in MKL-1 and MKL-2) of the MCC cell lines. Our findings in flow cytometry did mainly match with our transcriptomic data: We observed significantly increased STAT1 and BST2 level in all three cell lines. The molecule CXCL10 showed no significant differences between ± IFNγ in all tested cell lines. CD74 was stained as surface molecule but did not show any differences between ± IFNγ on protein level. Nevertheless, intracellular staining of MCC cells incubated with IFNγ led to an increase of CD74 in all three cell lines. These data indicate that the differences on mRNA-expression level translate to correspondingly different amounts of protein for most, but not all gene products.
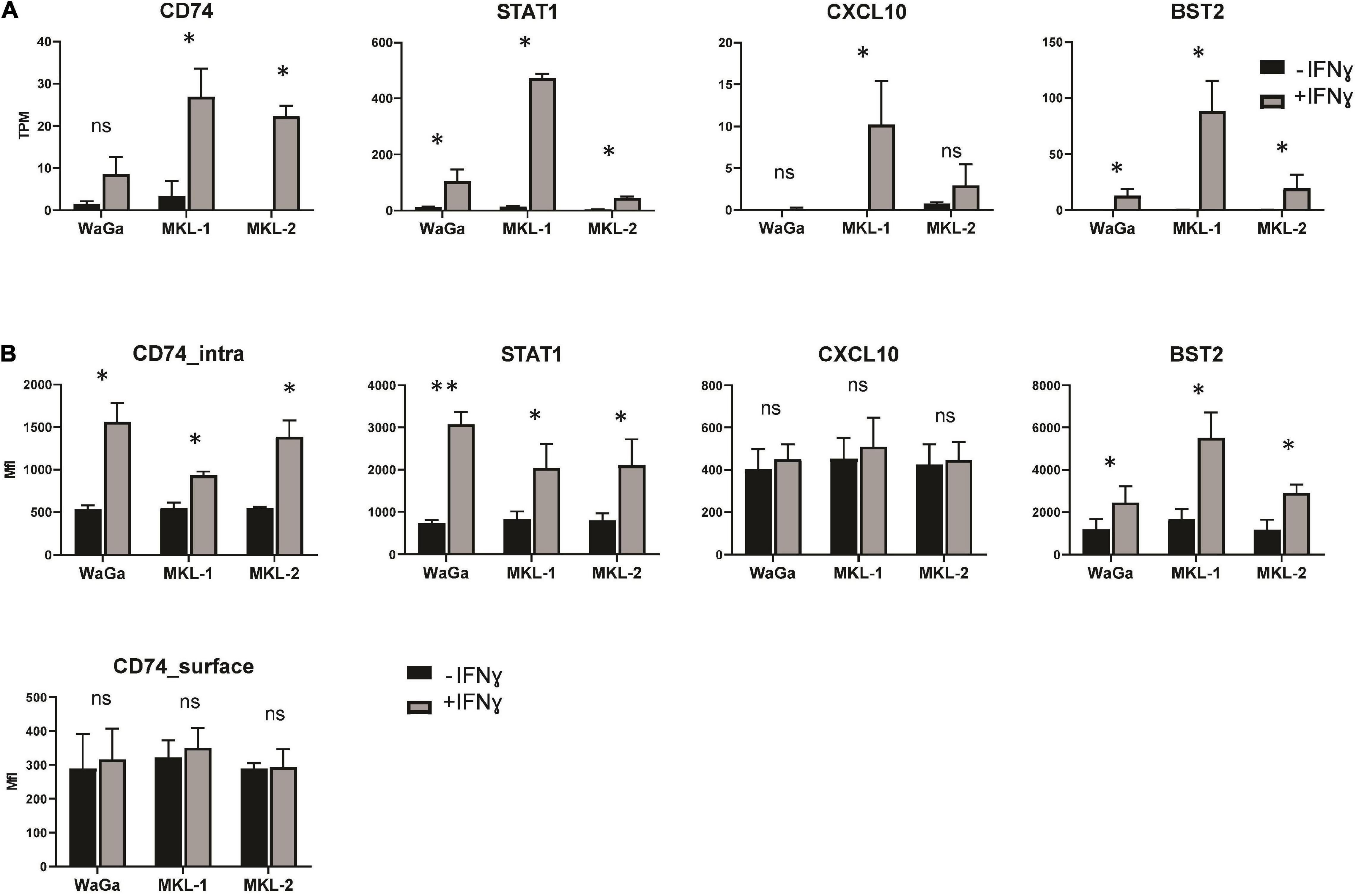
Figure 6. Validation of differential gene expression in MCC cell lines on protein level and mRNA level. (A) TPM values were acquired from our sequencing output. The data represent mean values ± SEM from three independent experiments (two for MKL-1 control). (B) After 72 h of treatment with or without IFNγ (3,000 U/ml), the Merkel cell carcinoma cell lines MKL-1, MKL-2, and WaGa were stained for signal transducer and activator of transcription 1-alpha/beta (STAT1), bone marrow stromal antigen 2 (BST2), C-X-C motif chemokine 10 (CXCL10), and HLA class II histocompatibility antigen gamma chain (CD74) and analyzed by flow cytometry. The average MFI of three independent experiments ± standard error is indicated. p-values were determined using the paired student’s t-test. *p < 0.05; **p < 0.01; ns, not significant.
Large T Antigen Expression Under Interferon Gamma Treatment
Expression of the LT antigen is a crucial factor for MCC development. LT antigen was shown to be regulated by IFNγ on protein level (Willmes et al., 2012); hence, we tested whether this regulation also takes place on mRNA level. TPM values were acquired from our sequencing output. The expression of the LT antigen was not substantially altered by IFNγ on mRNA level (Figure 7A). To study this result on protein level, we performed a Western Blot analysis with cell lysates treated with or without IFNγ for 72 h (Figures 7B,C). We detected a small but significant decrease of LT antigen after IFNγ incubation in the cell lines WaGa and MKL-1, whereas LT antigen expression was not significantly altered in MKL-2.
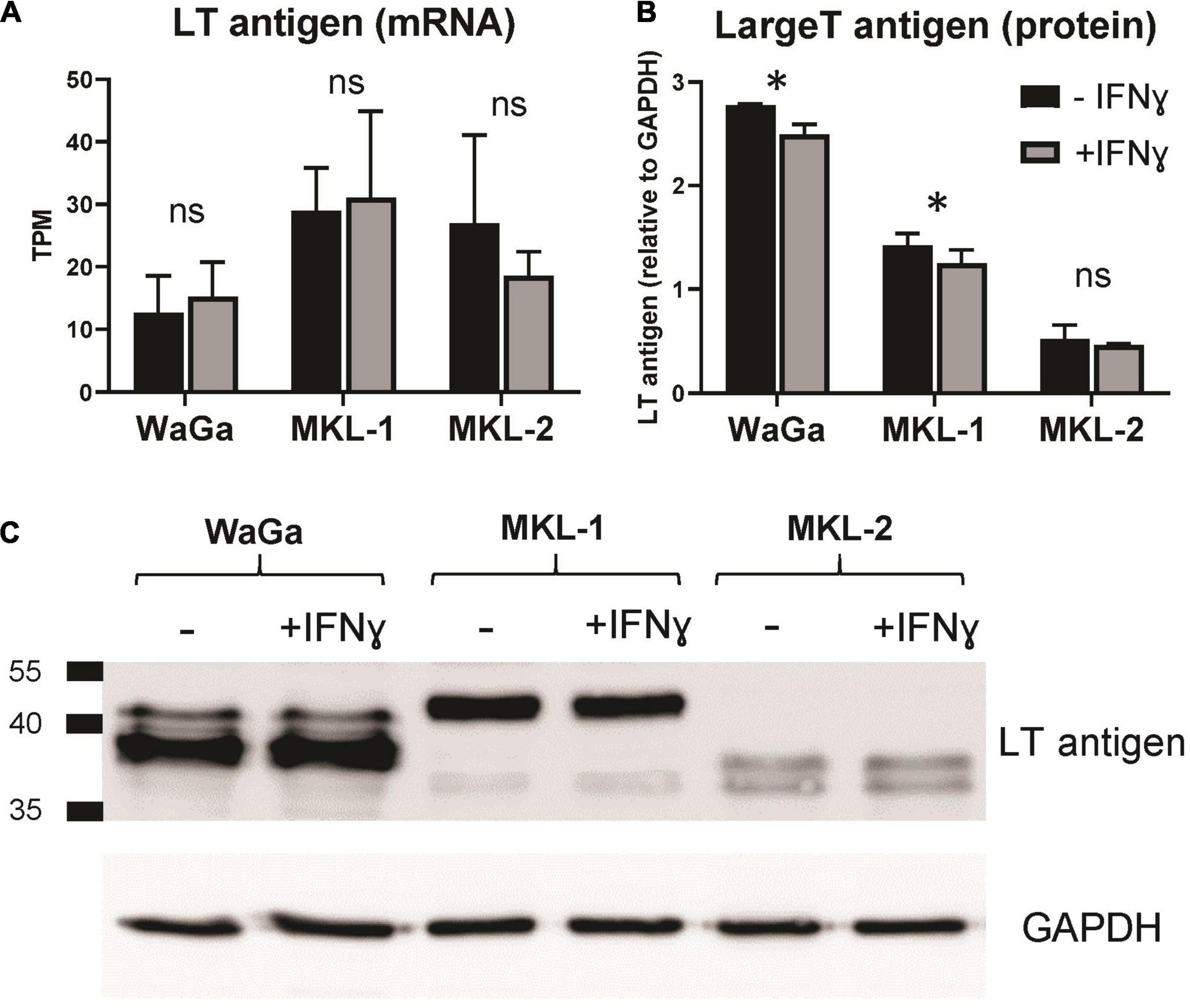
Figure 7. Large T antigen expression before and after IFNγ treatment. (A) TPM values were acquired from our sequencing output. The data represent mean values ± SEM from three independent experiments (two for MKL-1 control). (B) LT antigen expression was analyzed on protein level via Western blot analysis. Expression levels are depicted relative to the loading control GAPDH. The average of three independent experiments ± standard error is indicated. p-values were determined using the paired student’s t-test. *p < 0.05; **p < 0.01; ns, not significant. (C) Representative exposure of one western blot. Protein size standard is indicated in kilodalton.
Discussion
In this study, single-molecule Nanopore sequencing has been used to analyze the transcriptome of MCC cells. Previously, whole transcriptome and genome studies of MCC using the second-generation sequencing could demonstrate distinct mutation spectra and expression profiles between virus-positive and virus-negative MCC (Starrett et al., 2017). Czech-Sioli et al. used genome sequencing via the Nanopore platform to perform high-resolution analysis of the genomic structure of the integration site of the MCPyV into the host genome of MCC cell lines and primary tumors. In addition, they employed the technology to reveal copy numbers and integration pattern of the MCPyV (Czech-Sioli et al., 2020), underlining the increasing popularity of Nanopore sequencing in cancer research. However, no studies on the transcriptome-wide response to key cytokines had been conducted so far.
Immune Escape Genes and Human Leukocyte Antigen Class I Upregulation
As shown for IFNγ in various other settings, our sequencing studies found numerous differentially expressed genes that have been associated with both anti-tumoral and pro-tumoral effects. These data indicate the different modes of action of IFNγ: On the one hand, it acts highly tumor suppressive; on the other hand, it dampens the immune response by synthesis of inhibitory molecules (John and Darcy, 2016). Furthermore, we detected 11 immune escape genes that were significantly increased by IFNγ, suggesting that these are involved in the formation of an immunosuppressive tumor microenvironment counteracting the inflammatory effect of IFNγ (Mojic et al., 2017). Targeting these genes could improve the responsiveness to checkpoint inhibitor therapy and increase immunosurveillance and survival of MCC patients.
Downregulation of HLA class I and upregulation of immune checkpoint proteins (PD-1, CTLA-4, and PD-L1) are known to help the tumor to escape the control of the immune system. Paulson et al. reported reduced expression of HLA class I in MCC cell lines, which was reversible by IFNγ treatment (Paulson et al., 2014). In our flow cytometry experiments, we could also detect a clear upregulation of HLA class I surface expression in the presence of IFNγ in MKL-2 and WaGa cells, whereas MKL-1 cells showed only a slight increase of HLA class I. This is noteworthy because MKL-1 had the highest number of differentially expressed genes after IFNγ treatment. Expression levels of HLA have previously been shown to be low in MKL-1 cells, intermediate in WaGa cells, and high in MKL-2 cells (Ritter et al., 2017). In our flow cytometry experiments, without the addition of IFNγ, we could detect only low levels of HLA in WaGa and MKL-1 cells and higher levels in MKL-2 cells. Increased HLA induced by IFNγ, which counteracts HLA downregulation by the tumor, could contribute to higher effectiveness of immunotherapies in MCC patients. In awareness of the high error rates of Nanopore sequencing and the variability of the HLA allele–specific expression, we were able to verify the changes in HLA expression behavior, previously detected by flow cytometry, in the sequencing data. This strengthens the usability of Nanopore sequencing for highly variable alleles and the measurement of transcriptome-wide response to key cytokines. However, without prior HLA-typing of the subject, we consider that the third-generation sequencing still has difficulties to identify and quantify expression of highly polymorphic HLA loci.
Immune Checkpoints Are Influenced by Interferon Gamma Treatment
We studied the effect of IFNγ on the immune checkpoint PD-L1 and the key immune suppressive molecule IDO. IDO is of special interest for MCC as it has been shown that IDO expression is associated with MCPyV status and prognosis (Wardhani et al., 2019). To date, the effect of IFNγ on IDO has not been studied in MCC. We could not detect any alteration of IDO in all cell lines neither on mRNA nor on protein level. In contrast, IDO has been reported to be upregulated by IFNγ in the tumor microenvironment of melanoma (Spranger et al., 2013) and several other cell types, such as dendritic cells (Jürgens et al., 2009). Interestingly, our sequencing data show that WARS, which can protect cancer cells from IDO-mediated tryptophan depletion (Adam et al., 2018), is highly upregulated on mRNA level in the presence of IFNγ in all cell lines, underlining the pro-inflammatory activity of IFNγ in this context.
Interferon gamma is known to contribute to tumor immune resistance by inducing the expression of immune checkpoint receptors and their ligands (Benci et al., 2016). We could demonstrate a slight upregulation of PD-L1 in MKL-1 and MKL-2 cells by flow cytometry analysis. Another interesting protein associated with inhibitory effects on T cells is Tapasin-related protein, encoded by the gene TAPBPL. TAPBPL was highly upregulated in IFNγ-exposed MKL-1 cells. Tapasin-related protein is a T cell co-inhibitory molecule identified in 2021 (Lin et al., 2021), which is expressed on cancer cells and antigen-presenting cells as well as the corresponding receptor on activated CD4 and CD8 T cells. As a new immune checkpoint molecule, it might be an interesting target for cancer therapy.
Alteration in Large T Antigen Expression on Protein but Not on mRNA Level
Large T antigen mRNA expression was not significantly altered by IFNγ treatment in all three MCC cell lines. However, our studies of the LT antigen on protein level showed small but significant decreased expression in WaGa and MKL-1, whereas no alteration could be detected in MKL-2. We hypothesize that IFNγ might have a destabilization effect only on protein level in WaGa and MKL-1 cells, which is not detectable in the mRNA profile. Interestingly, Willmes et al. described a clear reduction of the LT antigen on protein level in WaGa cells, whereas MKL-1 and MKL-2 cells showed no change in LT protein expression, when analyzed after 7 days of IFNγ treatment (Willmes et al., 2012). This discrepancy may be attributed to the kinetics of LT antigen regulation, which may yield different results at different time points.
Oxford Nanopore Sequencing for Transcriptomics
In this study, we strived to elucidate the biological response of MCC cell lines to IFNγ stimulation while also trying to ascertain whether the expression studies of large eukaryotic transcriptomes are sensible and feasible using the Nanopore platform. We especially were interested to produce reliable and reproducible data at the current stage of the yet relatively new Nanopore system. There have been several benchmarking studies on Nanopore sequencing in the context of cDNA or direct RNA sequencing that discuss the system in a benchmark-type situation (Workman et al., 2019; Chen et al., 2021). Our goal, however, was to test practicability, robustness, and reproducibility in an applied setting. We found that the Nanopore system offers great potential for researchers to get access to in-house–produced sequencing data with relatively low entry costs and virtually no maintenance costs. These advantages come, however, at the price of high hardware requirements, a necessity of sufficient bioinformatics infrastructure and a fast-evolving system, in which laboratory protocols, software versions, and specifications as well as the recommendations of the manufacturer change with higher frequency as compared to firmly established sequencing environments as Illumina. We especially had issues pinpointing specific points of failure in experimental procedures that were virtually identical but for the flow cell used. Documentation on troubleshooting was sparse and hard to find at the time of our experiments. It is, however, noteworthy that, since then, Oxford Nanopore has greatly improved its available training resources.
Pore count at the beginning of an experiment appeared to be a good indicator of the possible total sequencing output for us; however, this was not true for flow cells that had been stored for longer periods and were approaching expiration date. In the experiment with MKL-2, we observed a rapid drop off of sequencing output after 24 h (Figure 2A) although the flow cell was older but still above warranty level and had ample pores available. In addition, BC performance widely varied. The good performance of BC 1 in terms of yield could be replicated across all three experiments, similar to BC 6. BC 3 failed completely—an issue the broader community was aware of, and we became aware of during troubleshooting. However, BC 5 and BC 7 bordered on failure as well over all three flow cells.
The MinION Sequencing platform by Oxford Nanopore is a rapidly evolving technology. Troubleshooting was challenging for us at times. The biological nature of embedded pores creates a necessity for stringent experiment planning, because extended storage times appeared to have a negative effect on yield. However, the access to sequencing data within 3 days from library prep to finished data is very attractive. In addition, long reads allow the accurate quantification of gene expression even at low sequencing depth because even in an cDNA assay, each reverse-transcribed molecule came from a native poly(A)-mRNA molecule with no fragmentation bias. Overall, the system is under active development and Q 20 chemistry (99% base call accuracy) has already been announced while constant improvements are made to hardware and protocols. The system offers a great entry point to sequencing, even for smaller laboratories, and allows rapid quantification of the expression landscape of a sample.
Validation of Transcriptomic Data on Protein Level
To validate our Nanopore sequencing data, we further analyzed the expression of STAT1, CXCL10, BST2, CD74, and the LT antigen on protein level. We detected a significant increase of STAT1 and BST2 in all three cell lines, as we had observed on mRNA level. STAT1 is itself involved in the IFNγ signaling pathway and both STAT1 and BST2 are known to be IFN inducible (Soler et al., 2003; Blasius et al., 2006; Krause et al., 2006; Yoo et al., 2011). In addition, CD74 significantly increased on protein level for all three cell lines. In our sequencing findings, increased CD74 was only significant for MKL-1 and MKL-2, whereas the observed upregulation in WaGa did not meet significance. Interestingly, CD74 showed significant regulation only when stained intracellularly; surface expression of CD74 on the MCC cell lines was, in general, lower and completely unaltered by IFNγ. The transcript of the CD74 gene forms invariant chain of the immature HLA class II complex. The cell surface form, in contrast, acts as receptor for macrophage migration inhibitory factor (Imaoka et al., 2019) and is involved in tumorigenesis (Liu et al., 2016). This complex post-translational processing is probably the reason for the discrepancy between mRNA and intracellular expression, on the one hand, and surface expression, on the other hand.
C-X-C motif chemokine 10 was strongly increased on mRNA level in one MCC cell line incubated with IFNγ (MKL-1); nevertheless, we could not detect any significant differences on protein level, and the signal was relatively low. Because CXCL10 is a chemokine, we speculate that it is rapidly secreted and does not accumulate to detectable levels intracellularly, especially because no secretion-blocking substances like brefeldin A or monensin were added.
In summary, we could demonstrate the complexity of IFNγ responses mirrored by numerous differentially expressed genes induced by IFNγ, mainly with anti- or pro-tumoral effects or even both. In this context, it would be interesting to sequence primary MCC cell cultures and to examine their response to IFNγ because this could better represent in vivo conditions. The identified immune escape genes are of special interest for further studies as these could deliver valuable information about how the tumor circumvents the control of the immune system. Targeting the genes involved in immune evasion and tumor progression could be beneficial for the clinical outcome of MCC patients.
Data Availability Statement
The datasets presented in this study can be found in online repositories. The names of the repository/repositories and accession number(s) can be found below: https://zenodo.org/record/5031363#.YVQtx31CSUk, DOI: 10.5281/zenodo.5031363; https://zenodo.org/record/5645039#.YYPJNrso9H4, DOI: 10.5281/zenodo.5645039.
Author Contributions
JD: conceptualization. TS and JD: experimental procedures. CL, AW, and JV: data processing and analysis. JD and JV: funding acquisition and supervision. TS and CL: investigation and writing—original draft. CL, TS, AW, CB, JD, and JV: writing—review and editing. All authors have read and agreed to the published version of the manuscript.
Funding
This work was supported by the Deutsche Forschungsgemeinschaft (DFG, German Research Foundation) via the Research Training Group GRK2504/1 (project number 401821119), research project B4 to JD. Further funding was by the German Federal Ministry of Education and Research (BMBF) (e:Med-MelAutim 01ZX1905A to JV); Manfred-Roth Stiftung to JV and JD and the Matthias Lackas Stiftung to JV.
Conflict of Interest
The authors declare that the research was conducted in the absence of any commercial or financial relationships that could be construed as a potential conflict of interest.
Publisher’s Note
All claims expressed in this article are solely those of the authors and do not necessarily represent those of their affiliated organizations, or those of the publisher, the editors and the reviewers. Any product that may be evaluated in this article, or claim that may be made by its manufacturer, is not guaranteed or endorsed by the publisher.
Acknowledgments
We thank Jürgen Becker for providing us the Merkel cell carcinoma cell lines. Furthermore, we thank Petra Mühl-Zürbes and Christina Draßner for their technical assistance in Western Blot preparation.
Supplementary Material
The Supplementary Material for this article can be found online at: https://www.frontiersin.org/articles/10.3389/fmicb.2021.785662/full#supplementary-material
Footnote
- ^ https://github.com/nanoporetech/pipeline-transcriptome-de/commit/47c850bd320031ed1e2b36cd631f287e0d98e464
References
Abrahamsson, H., Jensen, B. V., Berven, L. L., Nielsen, D. L., Šaltytė Benth, J., Johansen, J. S., et al. (2020). Antitumour immunity invoked by hepatic arterial infusion of first-line oxaliplatin predicts durable colorectal cancer control after liver metastasis ablation: 8-12 years of follow-up. Int. J. Cancer 146, 2019–2026. doi: 10.1002/ijc.32847
Adam, I., Dewi, D. L., Mooiweer, J., Sadik, A., Mohapatra, S. R., Berdel, B., et al. (2018). Upregulation of tryptophanyl-tRNA synthethase adapts human cancer cells to nutritional stress caused by tryptophan degradation. Oncoimmunology 7, e1486353–e1486353. doi: 10.1080/2162402X.2018.1486353
Adelmann, C. H., Ching, G., Du, L., Saporito, R. C., Bansal, V., Pence, L. J., et al. (2016). Comparative profiles of BRAF inhibitors: the paradox index as a predictor of clinical toxicity. Oncotarget 7, 30453–30460. doi: 10.18632/oncotarget.8351
Afanasiev, O. K., Yelistratova, L., Miller, N., Nagase, K., Paulson, K., Iyer, J. G., et al. (2013). Merkel polyomavirus-specific T cells fluctuate with Merkel cell carcinoma burden and express therapeutically targetable PD-1 and Tim-3 exhaustion markers. Clin. Cancer Res. 19, 5351–5360. doi: 10.1158/1078-0432.CCR-13-0035
Anderson, A. M., Kalimutho, M., Harten, S., Nanayakkara, D. M., Khanna, K. K., and Ragan, M. A. (2017). The metastasis suppressor RARRES3 as an endogenous inhibitor of the immunoproteasome expression in breast cancer cells. Sci. Rep. 7:39873. doi: 10.1038/srep39873
Arora, R., Choi, J. E., Harms, P. W., and Chandrani, P. (2020). Merkel cell polyomavirus in Merkel cell carcinoma: integration sites and involvement of the KMT2D tumor suppressor gene. Viruses 12:966. doi: 10.3390/v12090966
Aversa, I., Zolea, F., Ieranò, C., Bulotta, S., Trotta, A. M., Faniello, M. C., et al. (2017). Epithelial-to-mesenchymal transition in FHC-silenced cells: the role of CXCR4/CXCL12 axis. J. Exp. Clin. Cancer Res. 36:104. doi: 10.1186/s13046-017-0571-8
Ayers, M., Lunceford, J., Nebozhyn, M., Murphy, E., Loboda, A., Kaufman, D. R., et al. (2017). IFN-γ-related mRNA profile predicts clinical response to PD-1 blockade. J. Clin. Invest. 127, 2930–2940. doi: 10.1172/JCI91190
Bald, T., Landsberg, J., Lopez-Ramos, D., Renn, M., Glodde, N., Jansen, P., et al. (2014). Immune cell-poor melanomas benefit from PD-1 blockade after targeted type I IFN activation. Cancer Discov. 4, 674–687. doi: 10.1158/2159-8290.CD-13-0458
Barbarulo, A., Iansante, V., Chaidos, A., Naresh, K., Rahemtulla, A., Franzoso, G., et al. (2013). Poly(ADP-ribose) polymerase family member 14 (PARP14) is a novel effector of the JNK2-dependent pro-survival signal in multiple myeloma. Oncogene 32, 4231–4242. doi: 10.1038/onc.2012.448
Bartolomé, R. A., García-Palmero, I., Torres, S., López-Lucendo, M., Balyasnikova, I. V., and Casal, J. I. (2015). IL13 receptor α2 signaling requires a scaffold protein, FAM120A, to activate the FAK and PI3K pathways in colon cancer metastasis. Cancer Res. 75, 2434–2444. doi: 10.1158/0008-5472.CAN-14-3650
Beatty, G., and Paterson, Y. (2001). IFN-gamma-dependent inhibition of tumor angiogenesis by tumor-infiltrating CD4+ T cells requires tumor responsiveness to IFN-gamma. J. Immunol. 166, 2276–2282. doi: 10.4049/jimmunol.166.4.2276
Benci, J. L., Xu, B., Qiu, Y., Wu, T. J., Dada, H., Twyman-Saint Victor, C., et al. (2016). Tumor interferon signaling regulates a multigenic resistance program to immune checkpoint blockade. Cell 167, 1540–1554.e12. doi: 10.1016/j.cell.2016.11.022
Biamonte, F., Battaglia, A. M., Zolea, F., Oliveira, D. M., Aversa, I., Santamaria, G., et al. (2018). Ferritin heavy subunit enhances apoptosis of non-small cell lung cancer cells through modulation of miR-125b/p53 axis. Cell Death Dis. 9:1174. doi: 10.1038/s41419-018-1216-3
Blasius, A. L., Giurisato, E., Cella, M., Schreiber, R. D., Shaw, A. S., and Colonna, M. (2006). Bone marrow stromal cell antigen 2 is a specific marker of type I IFN-producing cells in the naive mouse, but a promiscuous cell surface antigen following IFN stimulation. J. Immunol. 177, 3260–3265. doi: 10.4049/jimmunol.177.5.3260
Bonan, N. F., Kowalski, D., Kudlac, K., Flaherty, K., Gwilliam, J. C., Falkenberg, L. G., et al. (2019). Inhibition of HGF/MET signaling decreases overall tumor burden and blocks malignant conversion in Tpl2-related skin cancer. Oncogenesis 8:1. doi: 10.1038/s41389-018-0109-8
Borchert, S., Czech-Sioli, M., Neumann, F., Schmidt, C., Wimmer, P., Dobner, T., et al. (2014). High-affinity Rb binding, p53 inhibition, subcellular localization, and transformation by wild-type or tumor-derived shortened Merkel cell polyomavirus large T antigens. J. Virol. 88, 3144–3160. doi: 10.1128/JVI.02916-13
Bouker, K. B., Skaar, T. C., Riggins, R. B., Harburger, D. S., Fernandez, D. R., Zwart, A., et al. (2005). Interferon regulatory factor-1 (IRF-1) exhibits tumor suppressor activities in breast cancer associated with caspase activation and induction of apoptosis. Carcinogenesis 26, 1527–1535. doi: 10.1093/carcin/bgi113
Britzen-Laurent, N., Lipnik, K., Ocker, M., Naschberger, E., Schellerer, V. S., Croner, R. S., et al. (2013). GBP-1 acts as a tumor suppressor in colorectal cancer cells. Carcinogenesis 34, 153–162. doi: 10.1093/carcin/bgs310
Brunn, D., Turkowski, K., Günther, S., Weigert, A., Muley, T., Kriegsmann, M., et al. (2021). Interferon regulatory factor 9 promotes lung cancer progression via regulation of versican. Cancers (Basel) 13:208. doi: 10.3390/cancers13020208
Burks, J., Reed, R. E., and Desai, S. D. (2014). ISGylation governs the oncogenic function of Ki-Ras in breast cancer. Oncogene 33, 794–803. doi: 10.1038/onc.2012.633
Carbotti, G., Barisione, G., Orengo, A. M., Brizzolara, A., Airoldi, I., Bagnoli, M., et al. (2013). The IL-18 antagonist IL-18–binding protein is produced in the human ovarian cancer microenvironment. Clin. Cancer Res. 19, 4611–4620. doi: 10.1158/1078-0432.CCR-13-0568
Cerezo, M., Guemiri, R., Druillennec, S., Girault, I., Malka-Mahieu, H., Shen, S., et al. (2018). Translational control of tumor immune escape via the eIF4F–STAT1–PD-L1 axis in melanoma. Nat. Med. 24, 1877–1886. doi: 10.1038/s41591-018-0217-1
Chen, H., Cong, X., Wu, C., Wu, X., Wang, J., Mao, K., et al. (2020). Intratumoral delivery of CCL25 enhances immunotherapy against triple-negative breast cancer by recruiting CCR9(+) T cells. Sci. Adv. 6:eaax4690–eaax4690. doi: 10.1126/sciadv.aax4690
Chen, Y., Davidson, N. M., Wan, Y. K., Patel, H., Yao, F., Low, H. M., et al. (2021). A systematic benchmark of nanopore long read RNA sequencing for transcript level analysis in human cell lines. bioRxiv [Preprint]. doi: 10.1101/2021.04.21.440736
Chiang, K.-C., Huang, S.-T., Wu, R.-C., Huang, S.-C., Yeh, T.-S., Chen, M.-H., et al. (2019). Interferon α-inducible protein 27 is an oncogene and highly expressed in cholangiocarcinoma patients with poor survival. Cancer Manag. Res. 11, 1893–1905. doi: 10.2147/CMAR.S196485
Chiao, P. J., Shin, D. M., Sacks, P. G., Hong, W. K., and Tainsky, M. A. (1992). Elevated expression of the ribosomal protein S2 gene in human tumors. Mol. Carcinog. 5, 219–231. doi: 10.1002/mc.2940050309
Co, J. K., Verma, S., Gurjav, U., Sumibcay, L., and Nerurkar, V. R. (2007). Interferon- alpha and – beta restrict polyomavirus JC replication in primary human fetal glial cells: implications for progressive multifocal leukoencephalopathy therapy. J. Infect. Dis. 196, 712–718. doi: 10.1086/520518
Cooper, M. A., Fehniger, T. A., Turner, S. C., Chen, K. S., Ghaheri, B. A., Ghayur, T., et al. (2001). Human natural killer cells: a unique innate immunoregulatory role for the CD56(bright) subset. Blood 97, 3146–3151. doi: 10.1182/blood.v97.10.3146
Czech-Sioli, M., Günther, T., Therre, M., Spohn, M., Indenbirken, D., Theiss, J., et al. (2020). High-resolution analysis of Merkel cell polyomavirus in Merkel cell carcinoma reveals distinct integration patterns and suggests NHEJ and MMBIR as underlying mechanisms. PLoS Pathog. 16:e1008562. doi: 10.1371/journal.ppat.1008562
D’Cunha, J., Knight, E. Jr., Haas, A. L., Truitt, R. L., and Borden, E. C. (1996). Immunoregulatory properties of ISG15, an interferon-induced cytokine. Proc. Natl. Acad. Sci. U. S. A. 93, 211–215. doi: 10.1073/pnas.93.1.211
Desai, S. D., Haas, A. L., Wood, L. M., Tsai, Y.-C., Pestka, S., Rubin, E. H., et al. (2006). Elevated expression of ISG15 in tumor cells interferes with the ubiquitin/26S proteasome pathway. Cancer Res. 66, 921–928. doi: 10.1158/0008-5472.CAN-05-1123
Desai, S. D., Reed, R. E., Burks, J., Wood, L. M., Pullikuth, A. K., Haas, A. L., et al. (2012). ISG15 disrupts cytoskeletal architecture and promotes motility in human breast cancer cells. Exp. Biol. Med. (Maywood) 237, 38–49. doi: 10.1258/ebm.2011.011236
Di Sanzo, M., Gaspari, M., Misaggi, R., Romeo, F., Falbo, L., De Marco, C., et al. (2011). H ferritin gene silencing in a human metastatic melanoma cell line: a proteomic analysis. J. Proteome Res. 10, 5444–5453. doi: 10.1021/pr200705z
Diao, W., Guo, Q., Zhu, C., Song, Y., Feng, H., Cao, Y., et al. (2020). USP18 promotes cell proliferation and suppressed apoptosis in cervical cancer cells via activating AKT signaling pathway. BMC Cancer 20:741. doi: 10.1186/s12885-020-07241-1
Ettahar, A., Ferrigno, O., Zhang, M.-Z., Ohnishi, M., Ferrand, N., Prunier, C., et al. (2013). Identification of PHRF1 as a tumor suppressor that promotes the TGF-β cytostatic program through selective release of TGIF-driven PML inactivation. Cell Rep. 4, 530–541. doi: 10.1016/j.celrep.2013.07.009
Euskirchen, P., Bielle, F., Labreche, K., Kloosterman, W. P., Rosenberg, S., Daniau, M., et al. (2017). Same-day genomic and epigenomic diagnosis of brain tumors using real-time nanopore sequencing. Acta Neuropathol. 134, 691–703. doi: 10.1007/s00401-017-1743-5
Falvey, C. M., O’Donovan, T. R., El-Mashed, S., Nyhan, M. J., O’Reilly, S., and McKenna, S. L. (2017). UBE2L6/UBCH8 and ISG15 attenuate autophagy in esophageal cancer cells. Oncotarget 8, 23479–23491. doi: 10.18632/oncotarget.15182
Fang, C., Zhang, J., Yang, H., Peng, L., Wang, K., Wang, Y., et al. (2019). Leucine aminopeptidase 3 promotes migration and invasion of breast cancer cells through upregulation of fascin and matrix metalloproteinases-2/9 expression. J. Cell Biochem. 120, 3611–3620. doi: 10.1002/jcb.27638
Feng, H., Shuda, M., Chang, Y., and Moore, P. S. (2008). Clonal integration of a polyomavirus in human Merkel cell carcinoma. Science 319, 1096–1100. doi: 10.1126/science.1152586
Feng, Q., Sekula, D., Guo, Y., Liu, X., Black, C. C., Galimberti, F., et al. (2008). UBE1L causes lung cancer growth suppression by targeting cyclin D1. Mol. Cancer Ther. 7, 3780–3788. doi: 10.1158/1535-7163.MCT-08-0753
Fitzgerald, T. L., Dennis, S., Kachare, S. D., Vohra, N. A., Wong, J. H., and Zervos, E. E. (2015). Dramatic increase in the incidence and mortality from Merkel cell carcinoma in the United States. Am. Surg. 81, 802–806. doi: 10.1177/000313481508100819
Fu, Z., Lu, C., Zhang, C., and Qiao, B. (2019). PSMA5 promotes the tumorigenic process of prostate cancer and is related to bortezomib resistance. Anticancer Drugs 30:e0773. doi: 10.1097/CAD.0000000000000773
Gao, J., Pan, H., Zhu, Z., Yu, T., Huang, B., and Zhou, Y. (2020a). Guanine nucleotide-binding protein subunit beta-4 promotes gastric cancer progression via activating Erk1/2. Acta Biochim. Biophys. Sin. (Shanghai) 52, 975–987. doi: 10.1093/abbs/gmaa084
Gao, J., Shi, L. Z., Zhao, H., Chen, J., Xiong, L., He, Q., et al. (2016). Loss of IFN-γ pathway genes in tumor cells as a mechanism of resistance to Anti-CTLA-4 therapy. Cell 167, 397–404.e9. doi: 10.1016/j.cell.2016.08.069
Gao, J., Yu, T., Xuan, Y., and Zhu, Z. (2020b). High expression of GNB4 predicts poor prognosis in patients with Helicobacter pylori -positive advanced gastric cancer. Transl. Cancer Res. 9, 4224–4238.
Gao, Y., Yang, W., Pan, M., Scully, E., Girardi, M., Augenlicht, L. H., et al. (2003). Gamma delta T cells provide an early source of interferon gamma in tumor immunity. J. Exp. Med. 198, 433–442. doi: 10.1084/jem.20030584
Geng, N., Zhang, W., Li, Y., and Li, F. (2019). Aspartyl aminopeptidase suppresses proliferation, invasion, and stemness of breast cancer cells via targeting CD44. Anat. Rec. 302, 2178–2185. doi: 10.1002/ar.24206
Greenwood, C., Metodieva, G., Al-Janabi, K., Lausen, B., Alldridge, L., Leng, L., et al. (2012). Stat1 and CD74 overexpression is co-dependent and linked to increased invasion and lymph node metastasis in triple-negative breast cancer. J. Proteom. 75, 3031–3040. doi: 10.1016/j.jprot.2011.11.033
Gupta, R., Forloni, M., Bisserier, M., Dogra, S. K., Yang, Q., and Wajapeyee, N. (2016). Interferon alpha-inducible protein 6 regulates NRASQ61K-induced melanomagenesis and growth. eLife 5:e16432. doi: 10.7554/eLife.16432
Han, T., Wang, Z., Yang, Y., Shu, T., Li, W., Liu, D., et al. (2015). The tumor-suppressive role of BATF2 in esophageal squamous cell carcinoma. Oncol. Rep. 34, 1353–1360. doi: 10.3892/or.2015.4090
Harms, P. W., Patel, R. M., Verhaegen, M. E., Giordano, T. J., Nash, K. T., Johnson, C. N., et al. (2013). Distinct gene expression profiles of viral- and nonviral-associated Merkel cell carcinoma revealed by transcriptome analysis. J. Invest. Dermatol. 133, 936–945. doi: 10.1038/jid.2012.445
Heath, M., Jaimes, N., Lemos, B., Mostaghimi, A., Wang, L. C., Penas, P. F., et al. (2008). Clinical characteristics of Merkel cell carcinoma at diagnosis in 195 patients: the AEIOU features. J. Am. Acad. Dermatol. 58, 375–381. doi: 10.1016/j.jaad.2007.11.020
Hermann, C., Trowsdale, J., and Boyle, L. H. (2015). TAPBPR: a new player in the MHC class I presentation pathway. Tissue Antigens 85, 155–166. doi: 10.1111/tan.12538
Herold, N., Rudd, S. G., Sanjiv, K., Kutzner, J., Bladh, J., Paulin, C. B. J., et al. (2017). SAMHD1 protects cancer cells from various nucleoside-based antimetabolites. Cell Cycle 16, 1029–1038. doi: 10.1080/15384101.2017.1314407
Hesbacher, S., Pfitzer, L., Wiedorfer, K., Angermeyer, S., Borst, A., Haferkamp, S., et al. (2016). RB1 is the crucial target of the Merkel cell polyomavirus large T antigen in Merkel cell carcinoma cells. Oncotarget 7, 32956–32968. doi: 10.18632/oncotarget.8793
Hong, B., Li, H., Lu, Y., Zhang, M., Zheng, Y., Qian, J., et al. (2014). USP18 is crucial for IFN-γ-mediated inhibition of B16 melanoma tumorigenesis and antitumor immunity. Mol. Cancer 13:132. doi: 10.1186/1476-4598-13-132
Hosono, T., Tanaka, T., Tanji, K., Nakatani, T., and Kamitani, T. (2010). NUB1, an interferon-inducible protein, mediates anti-proliferative actions and apoptosis in renal cell carcinoma cells through cell-cycle regulation. Br. J. Cancer 102, 873–882. doi: 10.1038/sj.bjc.6605574
Houben, R., Shuda, M., Weinkam, R., Schrama, D., Feng, H., Chang, Y., et al. (2010). Merkel cell polyomavirus-infected Merkel cell carcinoma cells require expression of viral T antigens. J. Virol. 84, 7064–7072. doi: 10.1128/JVI.02400-09
House, I. G., Savas, P., Lai, J., Chen, A. X. Y., Oliver, A. J., Teo, Z. L., et al. (2020). Macrophage-derived CXCL9 and CXCL10 are required for antitumor immune responses following immune checkpoint blockade. Clin. Cancer Res. 26, 487–504. doi: 10.1158/1078-0432.CCR-19-1868
Huang, M.-Y., Wang, J.-Y., and Lin, S.-R. (2013). CA9 and CHRNB1 were correlated with perineural invasion in Taiwanese colorectal cancer patients. Biomark. Genom. Med. 5, 84–86. doi: 10.1016/j.bgm.2013.07.001
Huang, P., Liao, R., Chen, X., Wu, X., Li, X., Wang, Y., et al. (2020). Nuclear translocation of PLSCR1 activates STAT1 signaling in basal-like breast cancer. Theranostics 10, 4644–4658. doi: 10.7150/thno.43150
Hung, J.-Y., Chiang, S.-R., Liu, K.-T., Tsai, M.-J., Huang, M.-S., Shieh, J.-M., et al. (2017). Overexpression and proliferation dependence of acyl-CoA thioesterase 11 and 13 in lung adenocarcinoma. Oncol. Lett. 14, 3647–3656. doi: 10.3892/ol.2017.6594
Iacobelli, S., Sismondi, P., Giai, M., D’Egidio, M., Tinari, N., Amatetti, C., et al. (1994). Prognostic value of a novel circulating serum 90K antigen in breast cancer. Br. J. Cancer 69, 172–176. doi: 10.1038/bjc.1994.29
Ikeda, H., Old, L. J., and Schreiber, R. D. (2002). The roles of IFN gamma in protection against tumor development and cancer immunoediting. Cytokine Growth Factor Rev. 13, 95–109. doi: 10.1016/s1359-6101(01)00038-7
Imaoka, M., Tanese, K., Masugi, Y., Hayashi, M., and Sakamoto, M. (2019). Macrophage migration inhibitory factor-CD74 interaction regulates the expression of programmed cell death ligand 1 in melanoma cells. Cancer Sci. 110, 2273–2283. doi: 10.1111/cas.14038
Ip, C. L. C., Loose, M., Tyson, J. R., de Cesare, M., Brown, B. L., Jain, M., et al. (2015). MinION analysis and reference consortium: phase 1 data release and analysis. F1000Res. 4, 1075–1075. doi: 10.12688/f1000research.7201.1
Jain, M., Fiddes, I. T., Miga, K. H., Olsen, H. E., Paten, B., and Akeson, M. (2015). Improved data analysis for the MinION nanopore sequencer. Nat. Methods 12, 351–356. doi: 10.1038/nmeth.3290
Jain, M., Olsen, H. E., Paten, B., and Akeson, M. (2016). The Oxford Nanopore MinION: delivery of nanopore sequencing to the genomics community. Genome Biol. 17, 239–239.
Järvinen, T. A. H., and Prince, S. (2015). Decorin: a growth factor antagonist for tumor growth inhibition. Biomed. Res. Int. 2015, 654765–654765. doi: 10.1155/2015/654765
Ji, X., Zhu, H., Dai, X., Xi, Y., Sheng, Y., Gao, C., et al. (2019). Overexpression of GBP1 predicts poor prognosis and promotes tumor growth in human glioblastoma multiforme. Cancer Biomark. 25, 275–290. doi: 10.3233/CBM-171177
Jia, L., Zhou, Z., Liang, H., Wu, J., Shi, P., Li, F., et al. (2016). KLF5 promotes breast cancer proliferation, migration and invasion in part by upregulating the transcription of TNFAIP2. Oncogene 35, 2040–2051. doi: 10.1038/onc.2015.263
Jiang, A. P., Zhou, D. H., Meng, X. L., Zhang, A. P., Zhang, C., Li, X. T., et al. (2014). Down-regulation of epidermal growth factor receptor by curcumin-induced UBE1L in human bronchial epithelial cells. J. Nutr. Biochem. 25, 241–249. doi: 10.1016/j.jnutbio.2013.11.001
Jin, R., Chow, V. T., Tan, P. H., Dheen, S. T., Duan, W., and Bay, B. H. (2002). Metallothionein 2A expression is associated with cell proliferation in breast cancer. Carcinogenesis 23, 81–86. doi: 10.1093/carcin/23.1.81
John, L. B., and Darcy, P. K. (2016). The double-edged sword of IFN-γ-dependent immune-based therapies. Immunol. Cell Biol. 94, 527–528. doi: 10.1038/icb.2016.37
Jürgens, B., Hainz, U., Fuchs, D., Felzmann, T., and Heitger, A. (2009). Interferon-γ–triggered indoleamine 2,3-dioxygenase competence in human monocyte-derived dendritic cells induces regulatory activity in allogeneic T cells. Blood 114, 3235–3243. doi: 10.1182/blood-2008-12-195073
Kakumu, T., Sato, M., Goto, D., Kato, T., Yogo, N., Hase, T., et al. (2017). Identification of proteasomal catalytic subunit PSMA6 as a therapeutic target for lung cancer. Cancer Sci. 108, 732–743. doi: 10.1111/cas.13185
Kalaora, S., Lee, J. S., Barnea, E., Levy, R., Greenberg, P., Alon, M., et al. (2020). Immunoproteasome expression is associated with better prognosis and response to checkpoint therapies in melanoma. Nat. Commun. 11:896. doi: 10.1038/s41467-020-14639-9
Kasahara, T., Hooks, J. J., Dougherty, S. F., and Oppenheim, J. J. (1983). Interleukin 2-mediated immune interferon (IFN-gamma) production by human T cells and T cell subsets. J. Immunol. 130, 1784–1789.
Kasprzak, A., and Adamek, A. (2019). Insulin-like growth factor 2 (IGF2) signaling in colorectal cancer-from basic research to potential clinical applications. Int. J. Mol. Sci. 20:4915. doi: 10.3390/ijms20194915
Kaufman, H. L., Russell, J., Hamid, O., Bhatia, S., Terheyden, P., D’Angelo, S. P., et al. (2016). Avelumab in patients with chemotherapy-refractory metastatic Merkel cell carcinoma: a multicentre, single-group, open-label, phase 2 trial. Lancet Oncol. 17, 1374–1385. doi: 10.1016/S1470-2045(16)30364-3
Kim, H. G., Kim, J. Y., Han, E. H., Hwang, Y. P., Choi, J. H., Park, B. H., et al. (2011). Metallothionein-2A overexpression increases the expression of matrix metalloproteinase-9 and invasion of breast cancer cells. FEBS Lett. 585, 421–428. doi: 10.1016/j.febslet.2010.12.030
Kito, K., Yeh, E. T., and Kamitani, T. (2001). NUB1, a NEDD8-interacting protein, is induced by interferon and down-regulates the NEDD8 expression. J. Biol. Chem. 276, 20603–20609. doi: 10.1074/jbc.M100920200
Kodigepalli, K. M., Li, M., Liu, S.-L., and Wu, L. (2017). Exogenous expression of SAMHD1 inhibits proliferation and induces apoptosis in cutaneous T-cell lymphoma-derived HuT78 cells. Cell Cycle 16, 179–188. doi: 10.1080/15384101.2016.1261226
Kotowski, U., Erović, B. M., Schnöll, J., Stanek, V., Janik, S., Steurer, M., et al. (2019). Quantitative proteome analysis of Merkel cell carcinoma cell lines using SILAC. Clin. Proteomics 16:42. doi: 10.1186/s12014-019-9263-z
Krasagakis, K., Krüger-Krasagakis, S., Tzanakakis, G. N., Darivianaki, K., Stathopoulos, E. N., and Tosca, A. D. (2008). Interferon-alpha inhibits proliferation and induces apoptosis of Merkel cell carcinoma in vitro. Cancer Invest. 26, 562–568. doi: 10.1080/07357900701816477
Krause, C. D., He, W., Kotenko, S., and Pestka, S. (2006). Modulation of the activation of Stat1 by the interferon-γ receptor complex. Cell Res. 16, 113–123. doi: 10.1038/sj.cr.7310015
Kriegsman, B. A., Vangala, P., Chen, B. J., Meraner, P., Brass, A. L., Garber, M., et al. (2019). Frequent Loss of IRF2 in cancers leads to immune evasion through decreased MHC class I antigen presentation and increased PD-L1 expression. J. Immunol. 203, 1999–2010. doi: 10.4049/jimmunol.1900475
Kwon, C. H., Park, H. J., Choi, Y. R., Kim, A., Kim, H. W., Choi, J. H., et al. (2016). PSMB8 and PBK as potential gastric cancer subtype-specific biomarkers associated with prognosis. Oncotarget 7, 21454–21468. doi: 10.18632/oncotarget.7411
Läubli, H., Alisson-Silva, F., Stanczak, M. A., Siddiqui, S. S., Deng, L., Verhagen, A., et al. (2014). Lectin galactoside-binding soluble 3 binding protein (LGALS3BP) is a tumor-associated immunomodulatory ligand for CD33-related Siglecs. J. Biol. Chem. 289, 33481–33491. doi: 10.1074/jbc.M114.593129
Leger, A., and Leonardi, T. (2019). pycoQC, interactive quality control for Oxford nanopore sequencing. J. Open Source Softw. 4:1236.
Leite-De-Moraes, M. C., Moreau, G., Arnould, A., Machavoine, F., Garcia, C., Papiernik, M., et al. (1998). IL-4-producing NK T cells are biased towards IFN-gamma production by IL-12. Influence of the microenvironment on the functional capacities of NK T cells. Eur. J. Immunol. 28, 1507–1515. doi: 10.1002/(SICI)1521-4141(199805)28:05<1507::AID-IMMU1507>3.0.CO;2-F
Li, J., Song, Y., Yu, B., and Yu, Y. (2020). TNFAIP2 promotes non-small cell lung cancer cells and targeted by miR-145-5p. DNA Cell Biol. 39, 1256–1263. doi: 10.1089/dna.2020.5415
Li, J., Zhang, Z., Wang, L., and Zhang, Y. (2019). The oncogenic role of Wnt10a in colorectal cancer through activation of canonical Wnt/β-catenin signaling. Oncol. Lett. 17, 3657–3664. doi: 10.3892/ol.2019.10035
Li, P., Zhang, Q., and Tang, H. (2019). INPP1 up-regulation by miR-27a contributes to the growth, migration and invasion of human cervical cancer. J. Cell. Mol. Med. 23, 7709–7716. doi: 10.1111/jcmm.14644
Li, S., Xie, Y., Zhang, W., Gao, J., Wang, M., Zheng, G., et al. (2015). Interferon alpha-inducible protein 27 promotes epithelial-mesenchymal transition and induces ovarian tumorigenicity and stemness. J. Surg. Res. 193, 255–264. doi: 10.1016/j.jss.2014.06.055
Li, S. R., Gyselman, V. G., Lalude, O., Dorudi, S., and Bustin, S. A. (2000). Transcription of the inositol polyphosphate 1-phosphatase gene (INPP1) is upregulated in human colorectal cancer. Mol. Carcinog. 27, 322–329. doi: 10.1002/(sici)1098-2744(200004)27:4<322::aid-mc10>3.0.co;2-c
Liberzon, A., Birger, C., Thorvaldsdóttir, H., Ghandi, M., Mesirov, J. P., and Tamayo, P. (2015). The Molecular Signatures Database (MSigDB) hallmark gene set collection. Cell Syst. 1, 417–425. doi: 10.1016/j.cels.2015.12.004
Lin, M., Li, Y., Qin, S., Jiao, Y., and Hua, F. (2020). Ubiquitin-like modifier-activating enzyme 7 as a marker for the diagnosis and prognosis of breast cancer. Oncol. Lett. 19, 2773–2784. doi: 10.3892/ol.2020.11406
Lin, Y., Cui, C., Su, M., Silbart, L. K., Liu, H., Zhao, J., et al. (2021). Identification of TAPBPL as a novel negative regulator of T-cell function. EMBO Mol. Med. 13:e13404. doi: 10.15252/emmm.202013404
Lipnik, K., Naschberger, E., Gonin-Laurent, N., Kodajova, P., Petznek, H., Rungaldier, S., et al. (2010). Interferon gamma-induced human guanylate binding protein 1 inhibits mammary tumor growth in mice. Mol. Med. 16, 177–187. doi: 10.2119/molmed.2009.00172
Liu, F., Liu, J., Zhang, J., Shi, J., Gui, L., and Xu, G. (2020). Expression of STAT1 is positively correlated with PD-L1 in human ovarian cancer. Cancer Biol. Ther. 21, 963–971. doi: 10.1080/15384047.2020.1824479
Liu, M., Guo, S., and Stiles, J. K. (2011). The emerging role of CXCL10 in cancer (Review). Oncol. Lett. 2, 583–589. doi: 10.3892/ol.2011.300
Liu, W., Cao, Y., Guan, Y., and Zheng, C. (2018). BST2 promotes cell proliferation, migration and induces NF-κB activation in gastric cancer. Biotechnol. Lett. 40, 1015–1027. doi: 10.1007/s10529-018-2562-z
Liu, W., Kim, G. B., Krump, N. A., Zhou, Y., Riley, J. L., and You, J. (2020). Selective reactivation of STING signaling to target Merkel cell carcinoma. Proc. Natl. Acad. Sci. U. S. A. 117:13730. doi: 10.1073/pnas.1919690117
Liu, X., Song, C., Yang, S., Ji, Q., Chen, F., and Li, W. (2020). IFI30 expression is an independent unfavourable prognostic factor in glioma. J. Cell. Mol. Med. 24, 12433–12443. doi: 10.1111/jcmm.15758
Liu, Z., Chen, P., Gao, H., Gu, Y., Yang, J., Peng, H., et al. (2014). Ubiquitylation of autophagy receptor optineurin by HACE1 activates selective autophagy for tumor suppression. Cancer Cell 26, 106–120. doi: 10.1016/j.ccr.2014.05.015
Liu, Z., Chu, S., Yao, S., Li, Y., Fan, S., Sun, X., et al. (2016). CD74 interacts with CD44 and enhances tumorigenesis and metastasis via RHOA-mediated cofilin phosphorylation in human breast cancer cells. Oncotarget 7, 68303–68313. doi: 10.18632/oncotarget.11945
Liu, Z., Gu, S., Lu, T., Wu, K., Li, L., Dong, C., et al. (2020). IFI6 depletion inhibits esophageal squamous cell carcinoma progression through reactive oxygen species accumulation via mitochondrial dysfunction and endoplasmic reticulum stress. J. Exp. Clin. Cancer Res. 39:144. doi: 10.1186/s13046-020-01646-3
Liu, Z., Lu, H., Jiang, Z., Pastuszyn, A., and Hu, C. A. (2005). Apolipoprotein l6, a novel proapoptotic Bcl-2 homology 3-only protein, induces mitochondria-mediated apoptosis in cancer cells. Mol. Cancer Res. 3, 21–31.
Lo, B. K., Yu, M., Zloty, D., Cowan, B., Shapiro, J., and McElwee, K. J. (2010). CXCR3/ligands are significantly involved in the tumorigenesis of basal cell carcinomas. Am. J. Pathol. 176, 2435–2446. doi: 10.2353/ajpath.2010.081059
Lobello, N., Biamonte, F., Pisanu, M. E., Faniello, M. C., Jakopin, Ž, Chiarella, E., et al. (2016). Ferritin heavy chain is a negative regulator of ovarian cancer stem cell expansion and epithelial to mesenchymal transition. Oncotarget 7, 62019–62033. doi: 10.18632/oncotarget.11495
Lomonosov, M., Meziane el, K., Ye, H., Nelson, D. E., Randle, S. J., and Laman, H. (2011). Expression of Fbxo7 in haematopoietic progenitor cells cooperates with p53 loss to promote lymphomagenesis. PLoS One 6:e21165. doi: 10.1371/journal.pone.0021165
Long, A., Giroux, V., Whelan, K. A., Hamilton, K. E., Tétreault, M.-P., Tanaka, K., et al. (2015). WNT10A promotes an invasive and self-renewing phenotype in esophageal squamous cell carcinoma. Carcinogenesis 36, 598–606. doi: 10.1093/carcin/bgv025
Love, M. I., Huber, W., and Anders, S. (2014). Moderated estimation of fold change and dispersion for RNA-seq data with DESeq2. Genome Biol. 15:550. doi: 10.1186/s13059-014-0550-8
Luker, K. E., Pica, C. M., Schreiber, R. D., and Piwnica-Worms, D. (2001). Overexpression of IRF9 confers resistance to antimicrotubule agents in breast cancer cells. Cancer Res. 61, 6540–6547.
Ma, Y., Wei, Z., Bast, R. C., Wang, Z., Li, Y., Gao, M., et al. (2016). Downregulation of TRIM27 expression inhibits the proliferation of ovarian cancer cells in vitro and in vivo. Lab. Invest. 96, 37–48. doi: 10.1038/labinvest.2015.132
Maire, C. L., Mohme, M., Bockmayr, M., Fita, K. D., Riecken, K., Börnigen, D., et al. (2020). Glioma escape signature and clonal development under immune pressure. J. Clin. Invest. 130, 5257–5271. doi: 10.1172/JCI138760
Martin, E. M., Gould, V. E., Hoog, A., Rosen, S. T., Radosevich, J. A., and Deftos, L. J. (1991). Parathyroid hormone-related protein, chromogranin A, and calcitonin gene products in the neuroendocrine skin carcinoma cell lines MKL1 and MKL2. Bone Miner. 14, 113–120. doi: 10.1016/0169-6009(91)90088-h
Matsushita, H., Hosoi, A., Ueha, S., Abe, J., Fujieda, N., Tomura, M., et al. (2015). Cytotoxic T lymphocytes block tumor growth both by lytic activity and IFNγ-dependent cell-cycle arrest. Cancer Immunol. Res. 3, 26–36. doi: 10.1158/2326-6066.CIR-14-0098
Meissl, K., Macho-Maschler, S., Müller, M., and Strobl, B. (2017). The good and the bad faces of STAT1 in solid tumours. Cytokine 89, 12–20. doi: 10.1016/j.cyto.2015.11.011
Mojic, M., Takeda, K., and Hayakawa, Y. (2017). The dark side of IFN-γ: its role in promoting cancer immunoevasion. Int. J. Mol. Sci. 19:89. doi: 10.3390/ijms19010089
Mustafa, D. A. M., Pedrosa, R., Smid, M., van der Weiden, M., de Weerd, V., Nigg, A. L., et al. (2018). T lymphocytes facilitate brain metastasis of breast cancer by inducing guanylate-binding protein 1 expression. Acta Neuropathol. 135, 581–599. doi: 10.1007/s00401-018-1806-2
Nghiem, P. T., Bhatia, S., Lipson, E. J., Kudchadkar, R. R., Miller, N. J., Annamalai, L., et al. (2016). PD-1 blockade with pembrolizumab in advanced Merkel-cell carcinoma. N. Engl. J. Med. 374, 2542–2552. doi: 10.1056/NEJMoa1603702
Niess, H., Camaj, P., Mair, R., Renner, A., Zhao, Y., Jäckel, C., et al. (2015). Overexpression of IFN-induced protein with tetratricopeptide repeats 3 (IFIT3) in pancreatic cancer: cellular “pseudoinflammation” contributing to an aggressive phenotype. Oncotarget 6, 3306–3318. doi: 10.18632/oncotarget.2494
Niu, Y., Tang, D., Fan, L., Gao, W., and Lin, H. (2020). CCL25 promotes the migration and invasion of non-small cell lung cancer cells by regulating VEGF and MMPs in a CCR9-dependent manner. Exp. Ther. Med. 19, 3571–3580. doi: 10.3892/etm.2020.8635
Ohsugi, T., Yamaguchi, K., Zhu, C., Ikenoue, T., and Furukawa, Y. (2017). Decreased expression of interferon-induced protein 2 (IFIT2) by Wnt/β-catenin signaling confers anti-apoptotic properties to colorectal cancer cells. Oncotarget 8, 100176–100186. doi: 10.18632/oncotarget.22122
Owusu, B. Y., Galemmo, R., Janetka, J., and Klampfer, L. (2017). Hepatocyte growth factor, a key tumor-promoting factor in the tumor microenvironment. Cancers 9:35. doi: 10.3390/cancers9040035
Pan, Y., Huang, J., Xing, R., Yin, X., Cui, J., Li, W., et al. (2013). Metallothionein 2A inhibits NF-κB pathway activation and predicts clinical outcome segregated with TNM stage in gastric cancer patients following radical resection. J. Transl. Med. 11:173. doi: 10.1186/1479-5876-11-173
Paulson, K. G., Park, S. Y., Vandeven, N. A., Lachance, K., Thomas, H., Chapuis, A. G., et al. (2018). Merkel cell carcinoma: current US incidence and projected increases based on changing demographics. J. Am. Acad. Dermatol. 78, 457–463.e2. doi: 10.1016/j.jaad.2017.10.028
Paulson, K. G., Tegeder, A., Willmes, C., Iyer, J. G., Afanasiev, O. K., Schrama, D., et al. (2014). Downregulation of MHC-I expression is prevalent but reversible in Merkel cell carcinoma. Cancer Immunol. Res. 2, 1071–1079. doi: 10.1158/2326-6066.CIR-14-0005
Payne, A., Holmes, N., Rakyan, V., and Loose, M. (2019). BulkVis: a graphical viewer for Oxford nanopore bulk FAST5 files. Bioinformatics 35, 2193–2198. doi: 10.1093/bioinformatics/bty841
Pflügler, S., Svinka, J., Scharf, I., Crncec, I., Filipits, M., Charoentong, P., et al. (2020). IDO1+ Paneth cells promote immune escape of colorectal cancer. Commun. Biol. 3:252. doi: 10.1038/s42003-020-0989-y
Pidugu, V. K., Wu, M.-M., Yen, A.-H., Pidugu, H. B., Chang, K.-W., Liu, C.-J., et al. (2019). IFIT1 and IFIT3 promote oral squamous cell carcinoma metastasis and contribute to the anti-tumor effect of gefitinib via enhancing p-EGFR recycling. Oncogene 38, 3232–3247. doi: 10.1038/s41388-018-0662-9
Pol, J. G., Le Naour, J., and Kroemer, G. (2020). FLT3LG – a biomarker reflecting clinical responses to the immunogenic cell death inducer oxaliplatin. Oncoimmunology 9, 1755214–1755214. doi: 10.1080/2162402X.2020.1755214
Quick, J., Loman, N. J., Duraffour, S., Simpson, J. T., Severi, E., Cowley, L., et al. (2016). Real-time, portable genome sequencing for Ebola surveillance. Nature 530, 228–232. doi: 10.1038/nature16996
Rea, A., Palmieri, G., Tinari, N., Natoli, C., Tagliaferri, P., Morabito, A., et al. (1994). 90k is a serum marker of poor-prognosis in non-hodgkins-lymphoma patients. Oncol. Rep. 1, 723–725. doi: 10.3892/or.1.4.723
Ritter, C., Fan, K., Paschen, A., Reker Hardrup, S., Ferrone, S., Nghiem, P., et al. (2017). Epigenetic priming restores the HLA class-I antigen processing machinery expression in Merkel cell carcinoma. Sci. Rep. 7:2290. doi: 10.1038/s41598-017-02608-0
Robinson, J., Barker, D. J., Georgiou, X., Cooper, M. A., Flicek, P., and Marsh, S. G. E. (2019). IPD-IMGT/HLA database. Nucleic Acids Res. 48, D948–D955.
Rosen, S. T., Gould, V. E., Salwen, H. R., Herst, C. V., Le Beau, M. M., Lee, I., et al. (1987). Establishment and characterization of a neuroendocrine skin carcinoma cell line. Lab. Invest. 56, 302–312.
Rouette, A., Trofimov, A., Haberl, D., Boucher, G., Lavallée, V.-P., D’Angelo, G., et al. (2016). Expression of immunoproteasome genes is regulated by cell-intrinsic and –extrinsic factors in human cancers. Sci. Rep. 6:34019. doi: 10.1038/srep34019
Sánchez-Martín, D. S., Martínez-Torrecuadrada, J., Teesalu, T., Sugahara, K. N., Alvarez-Cienfuegos, A., Ximénez-Embún, P., et al. (2013). Proteasome activator complex PA28 identified as an accessible target in prostate cancer by in vivo selection of human antibodies. Proc. Natl. Acad. Sci. U. S. A. 110, 13791–13796. doi: 10.1073/pnas.1300013110
Sergushichev, A. A. (2016). An algorithm for fast preranked gene set enrichment analysis using cumulative statistic calculation. bioRxiv [Preprint]. doi: 10.1101/060012
Shao, L., Hou, W., Scharping, N. E., Vendetti, F. P., Srivastava, R., Roy, C. N., et al. (2019). IRF1 inhibits antitumor immunity through the upregulation of PD-L1 in the tumor cell. Cancer Immunol. Res. 7, 1258–1266. doi: 10.1158/2326-6066.CIR-18-0711
Shao, Q., Byrum, S. D., Moreland, L. E., Mackintosh, S. G., Kannan, A., Lin, Z., et al. (2013). A proteomic study of human Merkel cell carcinoma. J. Proteomics Bioinform. 6, 275–282. doi: 10.4172/jpb.1000291
Shoji, T., Kikuchi, E., Kikuchi, J., Takashima, Y., Furuta, M., Takahashi, H., et al. (2020). Evaluating the immunoproteasome as a potential therapeutic target in cisplatin-resistant small cell and non-small cell lung cancer. Cancer Chemother. Pharmacol. 85, 843–853. doi: 10.1007/s00280-020-04061-9
Shuda, M., Feng, H., Kwun, H. J., Rosen, S. T., Gjoerup, O., Moore, P. S., et al. (2008). T antigen mutations are a human tumor-specific signature for Merkel cell polyomavirus. Proc. Natl. Acad. Sci. U. S. A. 105, 16272–16277. doi: 10.1073/pnas.0806526105
Soler, C., Felipe, A., García-Manteiga, J., Serra, M., Guillén-Gómez, E., Casado, F. J., et al. (2003). Interferon-gamma regulates nucleoside transport systems in macrophages through signal transduction and activator of transduction factor 1 (STAT1)-dependent and -independent signalling pathways. Biochem. J. 375, 777–783. doi: 10.1042/BJ20030260
Spranger, S., Spaapen, R. M., Zha, Y., Williams, J., Meng, Y., Ha, T. T., et al. (2013). Up-regulation of PD-L1, IDO, and T(regs) in the melanoma tumor microenvironment is driven by CD8(+) T cells. Sci. Transl. Med. 5:200ra116. doi: 10.1126/scitranslmed.3006504
Starrett, G. J., Marcelus, C., Cantalupo, P. G., Katz, J. P., Cheng, J., Akagi, K., et al. (2017). Merkel cell polyomavirus exhibits dominant control of the tumor genome and transcriptome in virus-associated Merkel cell carcinoma. mBio 8:e02079-16. doi: 10.1128/mBio.02079-16
Steinbach, A., Winter, J., Reuschenbach, M., Blatnik, R., Klevenz, A., Bertrand, M., et al. (2017). ERAP1 overexpression in HPV-induced malignancies: a possible novel immune evasion mechanism. Oncoimmunology 6, e1336594. doi: 10.1080/2162402X.2017.1336594
Stratikos, E. (2014). Modulating antigen processing for cancer immunotherapy. Oncoimmunology 3:e27568. doi: 10.4161/onci.27568
Subramanian, A., Tamayo, P., Mootha, V. K., Mukherjee, S., Ebert, B. L., Gillette, M. A., et al. (2005). Gene set enrichment analysis: a knowledge-based approach for interpreting genome-wide expression profiles. Proc. Natl. Acad. Sci. U. S. A. 102, 15545–15550. doi: 10.1073/pnas.0506580102
Sum, E. Y. M., Segara, D., Duscio, B., Bath, M. L., Field, A. S., Sutherland, R. L., et al. (2005). Overexpression of LMO4 induces mammary hyperplasia, promotes cell invasion, and is a predictor of poor outcome in breast cancer. Proc. Natl. Acad. Sci. U. S. A. 102, 7659–7664. doi: 10.1073/pnas.0502990102
Takata, H., Kudo, M., Yamamoto, T., Ueda, J., Ishino, K., Peng, W. X., et al. (2016). Increased expression of PDIA3 and its association with cancer cell proliferation and poor prognosis in hepatocellular carcinoma. Oncol. Lett. 12, 4896–4904. doi: 10.3892/ol.2016.5304
Tan, Y., Zhou, G., Wang, X., Chen, W., and Gao, H. (2018). USP18 promotes breast cancer growth by upregulating EGFR and activating the AKT/Skp2 pathway. Int. J. Oncol. 53, 371–383. doi: 10.3892/ijo.2018.4387
Tian, S.-Y., Chen, S.-H., Shao, B.-F., Cai, H.-Y., Zhou, Y., Zhou, Y.-L., et al. (2014). Expression of leucine aminopeptidase 3 (LAP3) correlates with prognosis and malignant development of human hepatocellular carcinoma (HCC). Int. J. Clin. Exp. Pathol. 7, 3752–3762.
Wan, Q., Qu, J., Li, L., and Gao, F. (2021). Guanylate-binding protein 1 correlates with advanced tumor features, and serves as a prognostic biomarker for worse survival in lung adenocarcinoma patients. J. Clin. Lab. Anal. 35:e23610. doi: 10.1002/jcla.23610
Wang, B., Li, D., Rodriguez-Juarez, R., Farfus, A., Storozynsky, Q., Malach, M., et al. (2018). A suppressive role of guanine nucleotide-binding protein subunit beta-4 inhibited by DNA methylation in the growth of anti-estrogen resistant breast cancer cells. BMC Cancer 18:817. doi: 10.1186/s12885-018-4711-0
Wang, H., Qiu, X., Lin, S., Chen, X., Wang, T., and Liao, T. (2018). Knockdown of IFI27 inhibits cell proliferation and invasion in oral squamous cell carcinoma. World J. Surg. Oncol. 16:64. doi: 10.1186/s12957-018-1371-0
Wang, J., Zou, K., Feng, X., Chen, M., Li, C., Tang, R., et al. (2017). Downregulation of NMI promotes tumor growth and predicts poor prognosis in human lung adenocarcinomas. Mol. Cancer 16:158. doi: 10.1186/s12943-017-0705-9
Wang, J. L., Lu, F. Z., Shen, X. Y., Wu, Y., and Zhao, L. T. (2014). SAMHD1 is down regulated in lung cancer by methylation and inhibits tumor cell proliferation. Biochem. Biophys. Res. Commun. 455, 229–233. doi: 10.1016/j.bbrc.2014.10.153
Wang, M., Hu, Y., and Stearns, M. E. (2009). RPS2: a novel therapeutic target in prostate cancer. J. Exp. Clin. Cancer Res. 28:6. doi: 10.1186/1756-9966-28-6
Wang, Q., Pan, F., Li, S., Huang, R., Wang, X., Wang, S., et al. (2019). The prognostic value of the proteasome activator subunit gene family in skin cutaneous melanoma. J. Cancer 10, 2205–2219. doi: 10.7150/jca.30612
Wang, W., Wu, S., Guo, M., and He, J. (2016). LMO4 is a prognostic marker involved in cell migration and invasion in non-small-cell lung cancer. J. Thorac. Dis. 8, 3682–3690. doi: 10.21037/jtd.2016.12.22
Wang, X., Ye, M., Wu, M., Fang, H., Xiao, B., Xie, L., et al. (2020). RNF213 suppresses carcinogenesis in glioblastoma by affecting MAPK/JNK signaling pathway. Clin. Transl. Oncol. 22, 1506–1516. doi: 10.1007/s12094-020-02286-x
Wang, Y., Wang, H., Pan, T., Li, L., Li, J., and Yang, H. (2016). Overexpression of PHRF1 attenuates the proliferation and tumorigenicity of non-small cell lung cancer cells. Oncotarget 7, 64360–64370. doi: 10.18632/oncotarget.11842
Wardhani, L. O., Matsushita, M., Iwasaki, T., Kuwamoto, S., Nonaka, D., Nagata, K., et al. (2019). Expression of the IDO1/TDO2-AhR pathway in tumor cells or the tumor microenvironment is associated with Merkel cell polyomavirus status and prognosis in Merkel cell carcinoma. Hum. Pathol. 84, 52–61. doi: 10.1016/j.humpath.2018.09.003
Wen, H., Chen, Y., Hu, Z., Mo, Q., Tang, J., and Sun, C. (2014). Decreased expression of BATF2 is significantly associated with poor prognosis in oral tongue squamous cell carcinoma. Oncol. Rep. 31, 169–174. doi: 10.3892/or.2013.2863
Whitehead, C. M., Nelson, R., Hudson, R., Gore, M., Marcelpoil, R., Morel, D., et al. (2005). Overexpression of the PSMB9 component of the proteasome in early stage, node negative breast cancer is prognostic for an increased risk of early relapse or death. Cancer Res. 65, 740–740.
Wildi, S., Kleeff, J., Maruyama, H., Maurer, C. A., Friess, H., Büchler, M. W., et al. (1999). Characterization of cytokeratin 20 expression in pancreatic and colorectal cancer. Clin. Cancer Res. 5, 2840–2847.
Willmes, C., Adam, C., Alb, M., Völkert, L., Houben, R., Becker, J. C., et al. (2012). Type I and II IFNs inhibit Merkel cell carcinoma via modulation of the Merkel cell polyomavirus T antigens. Cancer Res. 72, 2120–2128. doi: 10.1158/0008-5472.CAN-11-2651
Workman, R. E., Tang, A. D., Tang, P. S., Jain, M., Tyson, J. R., Razaghi, R., et al. (2019). Nanopore native RNA sequencing of a human poly(A) transcriptome. Nat. Methods 16, 1297–1305.
Wu, D.-j, Jiang, Y.-s, He, R.-z., Tao, L.-y., Yang, M.-w., Fu, X.-l., et al. (2018). High expression of WNT7A predicts poor prognosis and promote tumor metastasis in pancreatic ductal adenocarcinoma. Sci. Rep. 8:15792. doi: 10.1038/s41598-018-34094-3
Xing, L., Tang, X., Wu, K., Huang, X., Yi, Y., and Huan, J. (2020). TRIM27 functions as a novel oncogene in non-triple-negative breast cancer by blocking cellular senescence through p21 ubiquitination. Mol. Ther. Nucleic Acids 22, 910–923. doi: 10.1016/j.omtn.2020.10.012
Yan, J., Jiang, Y., Lu, J., Wu, J., and Zhang, M. (2019). Inhibiting of proliferation, migration, and invasion in lung cancer induced by silencing interferon-induced transmembrane protein 1 (IFITM1). Biomed. Res. Int. 2019:9085435. doi: 10.1155/2019/9085435
Yang, R. K., Qing, Y., Jelloul, F. Z., Routbort, M. J., Wang, P., Shaw, K., et al. (2020). Identification of biomarkers of immune checkpoint blockade efficacy in recurrent or refractory solid tumor malignancies. Oncotarget 11, 600–618. doi: 10.18632/oncotarget.27466
Yang, Z., Zhuang, L., Yu, Y., Zhou, W., Lu, Y., Xu, Q., et al. (2015). Overexpression of APOBEC3F in tumor tissues is potentially predictive for poor recurrence-free survival from HBV-related hepatocellular carcinoma. Discov. Med. 20, 349–356.
Yao, N., Chen, Q., Shi, W., Tang, L., and Fu, Y. (2019). PARP14 promotes the proliferation and gemcitabine chemoresistance of pancreatic cancer cells through activation of NF-κB pathway. Mol. Carcinog. 58, 1291–1302. doi: 10.1002/mc.23011
Yen, M. C., Kan, J. Y., Hsieh, C. J., Kuo, P. L., Hou, M. F., and Hsu, Y. L. (2017). Association of long-chain acyl-coenzyme A synthetase 5 expression in human breast cancer by estrogen receptor status and its clinical significance. Oncol. Rep. 37, 3253–3260. doi: 10.3892/or.2017.5610
Yoo, H., Park, S. H., Ye, S. K., and Kim, M. (2011). IFN-γ-induced BST2 mediates monocyte adhesion to human endothelial cells. Cell. Immunol. 267, 23–29. doi: 10.1016/j.cellimm.2010.10.011
Yoshihama, S., Cho, S. X., Yeung, J., Pan, X., Lizee, G., Konganti, K., et al. (2021). NLRC5/CITA expression correlates with efficient response to checkpoint blockade immunotherapy. Sci. Rep. 11:3258. doi: 10.1038/s41598-021-82729-9
Yoshihama, S., Vijayan, S., Sidiq, T., and Kobayashi, K. S. (2017). NLRC5/CITA: a key player in cancer immune surveillance. Trends Cancer 3, 28–38. doi: 10.1016/j.trecan.2016.12.003
Yoshioka, S., King, M. L., Ran, S., Okuda, H., MacLean, J. A. II, McAsey, M. E., et al. (2012). WNT7A regulates tumor growth and progression in ovarian cancer through the WNT/β-catenin pathway. Mol. Cancer Res. 10, 469–482. doi: 10.1158/1541-7786.MCR-11-0177
Yu, F., Xie, D., Ng, S. S., Lum, C. T., Cai, M.-Y., Cheung, W. K., et al. (2015). IFITM1 promotes the metastasis of human colorectal cancer via CAV-1. Cancer Lett. 368, 135–143. doi: 10.1016/j.canlet.2015.07.034
Yu, J., Wei, M., Becknell, B., Trotta, R., Liu, S., Boyd, Z., et al. (2006). Pro- and antiinflammatory cytokine signaling: reciprocal antagonism regulates interferon-gamma production by human natural killer cells. Immunity 24, 575–590. doi: 10.1016/j.immuni.2006.03.016
Zeiner, P. S., Zinke, J., Kowalewski, D. J., Bernatz, S., Tichy, J., Ronellenfitsch, M. W., et al. (2018). CD74 regulates complexity of tumor cell HLA class II peptidome in brain metastasis and is a positive prognostic marker for patient survival. Acta Neuropathol. Commun. 6:18. doi: 10.1186/s40478-018-0521-5
Zhang, D., Wu, P., Zhang, Z., An, W., Zhang, C., Pan, S., et al. (2020). Overexpression of negative regulator of ubiquitin-like proteins 1 (NUB1) inhibits proliferation and invasion of gastric cancer cells through upregulation of p27Kip1 and inhibition of epithelial-mesenchymal transition. Pathol. Res. Pract. 216:153002. doi: 10.1016/j.prp.2020.153002
Zhang, H., Cui, B., Zhou, Y., Wang, X., Wu, W., Wang, Z., et al. (2021). B2M overexpression correlates with malignancy and immune signatures in human gliomas. Sci. Rep. 11:5045. doi: 10.1038/s41598-021-84465-6
Zhang, H., Zhou, Y., Cheng, Q., Dai, Z., Wang, Z., Liu, F., et al. (2020). PDIA3 correlates with clinical malignant features and immune signature in human gliomas. Aging (Albany NY) 12, 15392–15413. doi: 10.18632/aging.103601
Zhang, J., Wang, F., Liu, F., and Xu, G. (2020). Predicting STAT1 as a prognostic marker in patients with solid cancer. Ther. Adv. Med. Oncol. 12:1758835920917558. doi: 10.1177/1758835920917558
Zhang, J.-F., Hua, R., Liu, D.-J., Liu, W., Huo, Y.-M., and Sun, Y.-W. (2014). Effect of CD74 on the prognosis of patients with resectable pancreatic cancer. Hepatobiliary Pancreat. Dis. Int. 13, 81–86. doi: 10.1016/s1499-3872(14)60011-4
Zhang, Y., Feng, Y., Ji, D., Wang, Q., Qian, W., Wang, S., et al. (2018). TRIM27 functions as an oncogene by activating epithelial-mesenchymal transition and p-AKT in colorectal cancer. Int. J. Oncol. 53, 620–632. doi: 10.3892/ijo.2018.4408
Zhang, Z., Sun, T., Chen, Y., Gong, S., Sun, X., Zou, F., et al. (2016). CCL25/CCR9 signal promotes migration and invasion in hepatocellular and breast cancer cell lines. DNA Cell Biol. 35, 348–357. doi: 10.1089/dna.2015.3104
Zhao, J., Dong, Q.-Z., Zhong, F., Cai, L.-L., Qin, Z.-Y., Liu, Y., et al. (2017). NMI promotes hepatocellular carcinoma progression via BDKRB2 and MAPK/ERK pathway. Oncotarget 8, 12174–12185. doi: 10.18632/oncotarget.14556
Zheng, D. L., Huang, Q. L., Zhou, F., Huang, Q. J., Lin, J. Y., and Lin, X. (2012). PA28β regulates cell invasion of gastric cancer via modulating the expression of chloride intracellular channel 1. J. Cell. Biochem. 113, 1537–1546. doi: 10.1002/jcb.24022
Zhou, M.-J., Chen, F.-Z., Chen, H.-C., Wan, X.-X., Zhou, X., Fang, Q., et al. (2017). ISG15 inhibits cancer cell growth and promotes apoptosis. Int. J. Mol. Med. 39, 446–452. doi: 10.3892/ijmm.2016.2845
Keywords: Merkel cell carcinoma (MCC), third generation sequencing, Interferon gamma (IFNγ), immune escape, transcriptome
Citation: Sauerer T, Lischer C, Weich A, Berking C, Vera J and Dörrie J (2021) Single-Molecule RNA Sequencing Reveals IFNγ-Induced Differential Expression of Immune Escape Genes in Merkel Cell Polyomavirus–Positive MCC Cell Lines. Front. Microbiol. 12:785662. doi: 10.3389/fmicb.2021.785662
Received: 29 September 2021; Accepted: 18 November 2021;
Published: 22 December 2021.
Edited by:
Herbert Johannes Pfister, University of Cologne, GermanyReviewed by:
Ugo Moens, Arctic University of Norway, NorwayBarbara Seliger, Martin Luther University of Halle-Wittenberg, Germany
Copyright © 2021 Sauerer, Lischer, Weich, Berking, Vera and Dörrie. This is an open-access article distributed under the terms of the Creative Commons Attribution License (CC BY). The use, distribution or reproduction in other forums is permitted, provided the original author(s) and the copyright owner(s) are credited and that the original publication in this journal is cited, in accordance with accepted academic practice. No use, distribution or reproduction is permitted which does not comply with these terms.
*Correspondence: Julio Vera, Julio.Vera-Gonzalez@uk-erlangen.de; Jan Dörrie, Jan.Doerrie@uk-erlangen.de
†These authors have contributed equally to this work and share first authorship
‡The present work was performed in fulfilment of the requirement for obtaining the degree Dr. rer. nat. at the Friedrich-Alexander-Universität Erlangen-Nürnberg
§These authors share last authorship