- 1School of Environment and Energy, Peking University Shenzhen Graduate School, Shenzhen, China
- 2Laboratory of Municipal Wastewater Treatment and Reutilization Engineering, Shenzhen Water Group, Shenzhen, China
- 3Shenzhen Engineering Research Laboratory for Sludge and Food Waste Treatment and Resource Recovery, Tsinghua Shenzhen International Graduate School, Tsinghua University, Shenzhen, China
- 4College of Chemistry and Environmental Engineering, Shenzhen University, Shenzhen, China
- 5Green Living and Innovation Division, Hong Kong Productivity Council, Hong Kong, Hong Kong SAR, China
- 6Department of Civil and Environmental Engineering, The University of Auckland, Auckland, New Zealand
The anaerobic ammonium oxidation (anammox) by autotrophic anaerobic ammonia-oxidizing bacteria (AnAOB) is a biological process used to remove reactive nitrogen from wastewater. It has been repeatedly reported that elevated nitrite concentrations can severely inhibit the growth of AnAOB, which renders the anammox process challenging for industrial-scale applications. Both denitrifying (DN) and dissimilatory nitrate reduction to ammonium (DNRA) bacteria can potentially consume excess nitrite in an anammox system to prevent its inhibitory effect on AnAOB. However, metabolic interactions among DN, DNRA, and AnAOB bacteria under elevated nitrite conditions remain to be elucidated at metabolic resolutions. In this study, a laboratory-scale anammox bioreactor was used to conduct an investigation of the microbial shift and functional interactions of AnAOB, DN, and DNRA bacteria during a long-term nitrite inhibition to eventual self-recovery episode. The relative abundance of AnAOB first decreased due to high nitrite concentration, which lowered the system’s nitrogen removal efficiency, but then recovered automatically without any external interference. Based on the relative abundance variations of genomes in the inhibition, adaptation, and recovery periods, we found that DN and DNRA bacteria could be divided into three niche groups: type I (types Ia and Ib) that includes mainly DN bacteria and type II and type III that include primarily DNRA bacteria. Type Ia and type II bacteria outcompeted other bacteria in the inhibition and adaptation periods, respectively. They were recognized as potential nitrite scavengers at high nitrite concentrations, contributing to stabilizing the nitrite concentration and the eventual recovery of the anammox system. These findings shed light on the potential engineering solutions to maintain a robust and efficient industrial-scale anammox process.
Introduction
Anaerobic ammonium oxidization (anammox) has gained significant momentum as a highly efficient, cost-effective, and environment-friendly biological nitrogen removal process compared with the conventional nitrification–denitrification processes (Ismail et al., 2019). Anammox is mediated by the autotrophic anaerobic ammonia-oxidizing bacteria (AnAOB), which oxidize ammonium (NH4+) using nitrite (NO2–) as an electron acceptor under anaerobic conditions and produce nitrogen gas (N2) and nitrate (NO3–) (Bonassa et al., 2021). The anammox process has primarily been used to treat ammonium-rich wastewater (Alejandro et al., 2018), such as anaerobic digestion liquid of sludge, landfill leachate, urban domestic sewage, swine wastewater, or monosodium glutamate wastewater. Due to the extremely high ammonia nitrogen content (800–3,000 mg/L) in these types of wastewater, a preliminary step before the implementation of the anammox process is to oxidize approximately half of the ammonium into nitrite, known as partial nitritation, by ammonium oxidizing bacteria in the wastewater (Li et al., 2019). However, the nitritation process is difficult to control, often resulting in an overproduction of nitrite (Tao et al., 2012), leading to the inhibition of the anammox process.
This possible inhibitory effect makes controlling nitrite concentration a focus in implementing the anammox process. The inhibitory nitrite concentrations reported vary from 5 to 750 mg N L–1 (Zekker et al., 2017; Cho et al., 2020) in various anammox-based systems. Although the broad range of inhibitory nitrite concentrations can be attributed to variations in experimental conditions and operating modes (pH, temperature, experimental continuity, etc.), it implies that a mechanistic understanding of nitrite inhibition and nitrite resistance of the anammox microbial communities remains to be elucidated. Furthermore, due to the slow growth of AnAOB (Wang et al., 2016), the recovery from inhibition/death caused by prolonged high nitrite shock was proved to be difficult (van der Star et al., 2007) and puzzling even when a successful recovery did happen (Lotti et al., 2012).
Denitrifying (DN) and the dissimilatory nitrate reduction to ammonium (DNRA) bacteria can metabolize nitrite and potentially promote the recovery process of the anammox system from the nitrite shock. AnAOB is a key player in nitrogen cycling, whereas DN and DNRA bacteria are also important participants of the anammox system (Gonzalez-Gil et al., 2015; Keren et al., 2020). To date, the vast majority of identified AnAOB belongs to Planctomycetes, including six genera that have been reported so far, i.e., Candidatus (Ca.) Brocadia, Ca. Jettenia, Ca. Kuenenia, Ca. Scalindua, Ca. Anammoxoglobus, and Ca. Anammoxomicrobium (Ibrahim et al., 2016). DN and DNRA bacteria are mainly heterotrophs from a variety of phyla, for example, Proteobacteria, Chloroflexi, and Actinobacteria (Zhao et al., 2019; Keren et al., 2020). Many studies have pointed out that autotrophic organisms such as AnAOB can release soluble microbial products and extracellular polymeric substances. Environmental stimuli can cause the immediate release of these substances from the autotrophs (Ma et al., 2012; Chu et al., 2015; Zhang et al., 2016). Heterotrophic bacteria, including DN and DNRA bacteria, can thus live on these substances for survival and cater to important nitrogen cycling processes (Guo et al., 2016; Hou et al., 2017; Zhu et al., 2017). DN bacteria can reduce NO3– and NO2– to N2 (Zhou et al., 2014), whereas DNRA bacteria can reduce them to NH4+. The function of DNRA bacteria under high nitrite concentrations was not clear. DN bacteria from Proteobacteria, however, were reported to aid in reducing nitrite to nitrogen gas. In short, the nitrite denitrification genes in DN bacteria had higher abundance after the inhibition phase, and their cooperation could prevent the nitrite inhibition of anammox bacteria when the influent nitrite concentration was higher (Zhao et al., 2019). Thus, the DN/DNRA bacteria can potentially reduce the NO2– concentration and then balance the NO2–/NH4+ ratio to create a preferable environment for the anammox process (Wang et al., 2018; Zhao et al., 2019). There were a number of studies using amplicon sequencing targeting 16S ribosomal RNA (rRNA) gene to decode the bacterial communities (Ren et al., 2014; Liang et al., 2015), whereas others have used shotgun metagenomic approaches to understand functional genomes in anammox systems (Guo et al., 2016; Ciesielski et al., 2018; Sun et al., 2018; Tang et al., 2018). Overall, the metagenomic analysis is more powerful in revealing microbial functions at the genus or species level (Keren et al., 2020; Li et al., 2020).
To better understand the potential roles of nitrogen removal bacteria in membrane bioreactor (MBR) at high nitrite concentrations, we conducted a long-term nitrite inhibition experiment in MBR by progressively reducing the ammonium loading. Remarkably, an anammox self-recovery event was observed under nitrite inhibition, which has provided a potential solution to mitigate the inhibition of AnAOB in its industrial applications. Both 16S rRNA gene sequencing and genome-resolved metagenomic analysis were implemented to decode the shift of community composition and nitrogen metabolism genes during the nitrite-inhibition and recovery processes in the anammox system. Our finding will enable more stable control of anammox technology and facilitate its widespread application in wastewater treatment plants.
Materials and Methods
Membrane Bioreactor System
A continuous and complete-mix MBR system (Supplementary Figure 1) with an effective volume of 1.5 L and a 10-cm internal diameter was used in this study. The MBR was completely covered with aluminum foil to protect it from light. A hollow fiber ultrafiltration membrane (polyvinylidene fluoride) module was used to filter effluent and retain biomass through pumping. The MBR was mixed using a mechanical stirrer at 150 rpm to achieve a complete mix. A mixture of 95% argon gas and 5% carbon dioxide (50 ml min–1) was fed continuously into the MBR through a steel pipe extending to the bottom of the reactor to eliminate dissolved oxygen and maintain a circumneutral pH range of 6.8–8.0. Dissolved oxygen was monitored by a dissolved oxygen probe in the MBR and controlled at less than 1% air saturation. The entire system was maintained in a 37°C water bath. Mixed liquor volatile suspended solids (MLVSS) mass (0.9 g) of seed anammox sludge was sourced from a laboratory-scale anammox MBR; these anammox sludges were inoculated from an expanded granular sludge bed with carriers initially (Wu et al., 2019). The condition of this laboratory-scale anammox MBR was described as follows: the influent nitrite concentration of laboratory-scale anammox MBR was 1,550 mg N L–1, and the ammonia nitrogen concentration was 1,350 mg N L–1; the corresponding nitrogen removal rate was 1.5 g N L–1 day–1; MLVSS was approximately 3 g/L; the main AnAOB was Ca. Brocadia with a relative abundance of 19%. A synthetic nitrogenous wastewater medium (Supplementary Table 1) was used to cultivate AnAOB. A 24-h hydraulic retention time was maintained throughout the experiment. Nitrite and ammonium were added as needed in the forms of NaNO2 and NH4Cl. Before the commencement of the experiment, N2 was also sparged for 20 min into the medium to remove any dissolved oxygen.
Long-Term Nitrite Inhibition
The anammox MBR was operated for a total of 280 days, which can be divided into two phases (Table 1). Phase I (days 1–110) encompassed the operation of the MBR in the acclimation (days 1–50) and stabilization (days 51–110) periods, whereas phase II (days 110–280) created the nitrite inhibition environment in the MBR. In phase I, AnAOB was acclimated from the seed sludge by continuous feeding of a simulated nitrogenous wastewater medium. The nitrogen loading rate was gradually increased once the nitrogen removal efficiency remained stable. The performance of the MBR was considered stabilized when the influent nitrite concentration reached 1,000 mg N L–1, and the effluent nitrite concentration was below 5 mg N L–1. Acclimation of AnAOB was continued for a further 60 days to maintain stability for subsequent experiments.
Phase II was further subdivided into four periods based on the observed nitrogen removal efficiency, comprising of the perturbation (days 110–127), inhibition (days 127–188), adaptation (days 188–228), and recovery (days 228–280) periods. From days 110 to 127, the effluent nitrite and ammonium concentrations remained unchanged compared with the end of the stabilization period (Figure 1B), but the relative abundance of Ca. Brocadia decreased from 64 to 47% (Figure 2). Therefore, the system was considered disturbed, and days 110–127 were defined as the perturbation period. From the 127th day onward, the effluent nitrite and ammonium concentration (Figure 1B) increased rapidly along with the relative abundance of Ca. Brocadia further decreased to 43% (Figure 2B), suggesting the actual inhibition was happening. We, therefore, defined days 127–180 as the inhibition period. The nitrogen removal efficiency of days 188–228 began to rise, and the nitrogen removal efficiency of days 228–280 was completely restored (Figure 1C), so they were defined as the adaptation period and the recovery period, respectively.
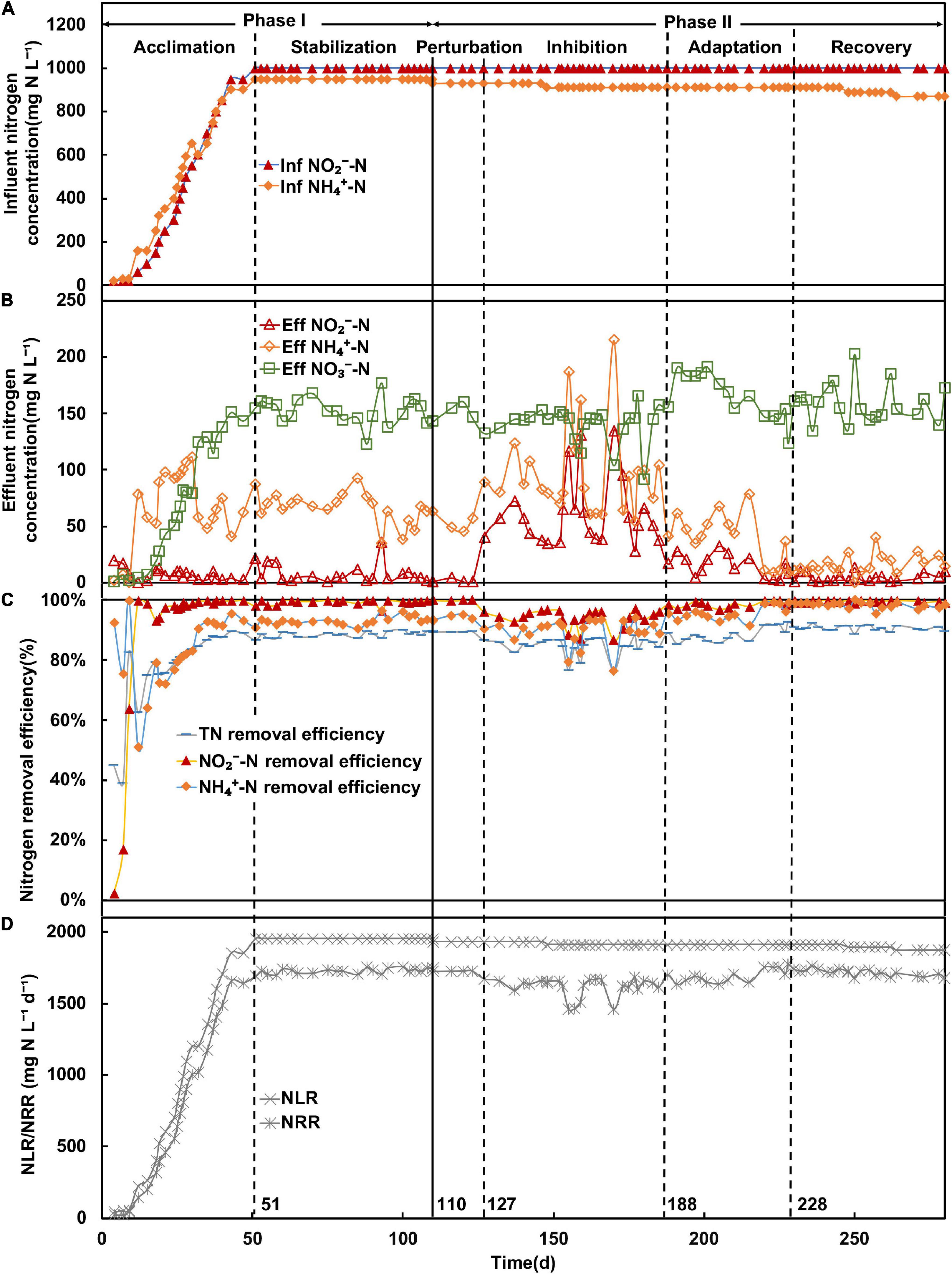
Figure 1. Performance of anammox membrane bioreactor; (A) concentration (mg N L–1) of influent ammonium (NH4+-N) and nitrite (NO2–-N); (B) concentration (mg N L–1) of effluent ammonium, nitrite, and nitrate (NO3–-N); (C) removal efficiency (%) of ammonium, nitrite, and total nitrogen (TN); (D) nitrogen removal rate (NRR) and nitrogen loading rate (NLR) (mg N L–1 day–1).
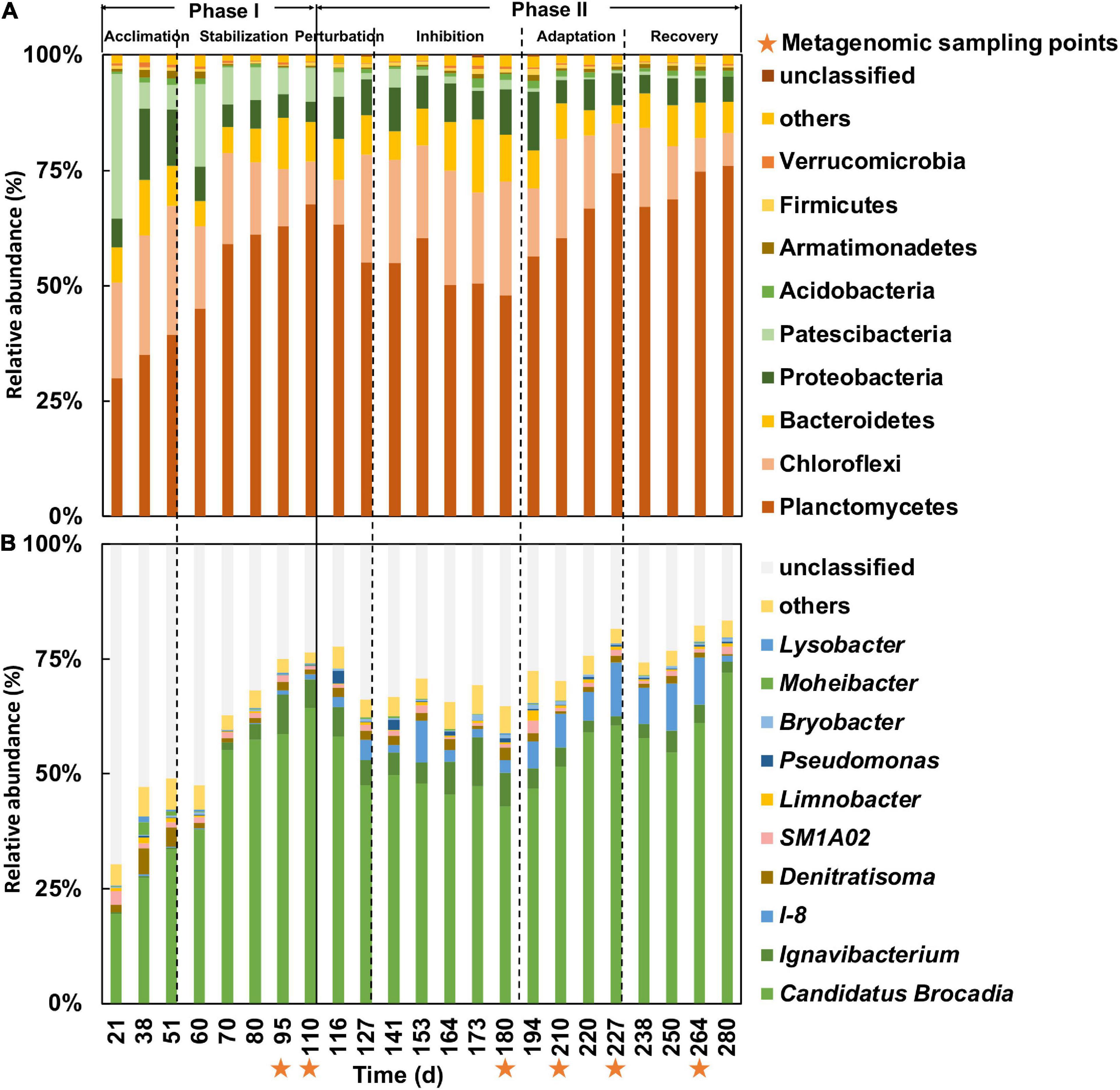
Figure 2. Composition of bacterial community at phylum (A) and genus (B) levels in samples over lifespan of bioreactor based on 16S rRNA OTUs. Orange stars below x-axis indicated sampling time points for metagenomics analysis.
Influent nitrite concentration was kept at 1,000 mg N L–1 throughout, whereas the influent ammonium concentration was gradually decreased from inhibition to recovery to create an imbalanced, nitrite-excess environment to inhibit AnAOB in phase II.
Chemical Analyses
Influent and effluent samples were collected for chemical analyses and filtered through a 0.22-μm membrane filter (JIN TENG, China) two or three times a week. The concentrations of NO2–-N, NH4+-N, and NO3–-N were then measured using standard spectrophotometric methods (APHA, 2012). Four samples in three periods of MLVSS were determined using the standard methods (APHA, 2005): day 1 in the acclimation period, day 100 in the stabilization period, and days 150 and 180 in the recovery period.
Sampling, DNA Extraction, and Sequencing
Sludge samples were collected at 23 different time points from MBR. Each time, CO2 was blown into the MBR to pump out the 50-ml mixed liquor before being collected in a 50-mL falcon tube. The collected sample was centrifuged at 4,000 rpm for 10 min to spin down the cell pellet. The supernatant was discarded, with the pellet aseptically transferred into a sterile 2-ml tube. All samples were stored at -80°C before DNA extraction. The DNA of 23 samples was extracted using the DNeasy Powersoil kit (QIAGEN, Germany) according to the manufacturer’s instructions. The DNA extracts were then subjected to barcoded polymerase chain reaction (PCR) amplification, with the barcodes added to the 5′ end of the PCR primers. The primers 515F (3′-GTGYCAGCMGCCGCGGTAA-5′) and 806R (5′- GGACTACNVGGGTWTCTAAT-3′) were used to amplify the V4 regions of bacterial 16S rRNA genes. PCR reaction solutions (50 μl) were prepared by mixing 25-μl 2 × Premix Taq (Takara Biotechnology, Dalian Co. Ltd., China), 1-μl forward and reverse primers (10 μM), and 3-μl DNA (20 ng/μl) template and nuclease-free water. Thermocycling conditions for PCR were set as follows: 5 min at 94°C for initialization; 30 cycles of 30-s denaturation at 94°C, 30-s annealing at 52°C, and 30-s extension at 72°C; followed by 10-min final elongation at 72°C. PCR products were purified with the TaKaRa MiNiBEST DNA Fragment Purification Kit Ver.4.0 (TaKaRa, Japan) and then quality-checked with gel electrophoresis. Sequencing libraries were generated using NEBNext ® Ultra™ II DNA Library Prep Kit for Illumina ® (New England Biolabs, MA, United States) following manufacturer’s recommendations, and index codes were added. The library quality was assessed on the Qubit@ 2.0 Fluorometer (Thermo Fisher Scientific, MA, United States). At last, the library was sequenced on an Illumina Nova6000 platform, and 250-bp paired-end reads were generated (Guangdong Magigene Biotechnology Co., Ltd., Guangzhou, China).
For shotgun metagenomic sequencing, a subset of six DNA samples were selected around six different time points in the four periods of the experiment: day 95 (D95) and day 110 (D110) in the stabilization period (days 51–110); day 180 (D180) in the inhibition period (days 127–188); day 210 (D210) and day 227 (D227) in the adaptation period (days 188–228); and day 264 (D264) from the recovery period (days 228–280). Each of the six samples is a mixture of the sludge from the days mentioned earlier and 3 days before and after. DNA was extracted and assessed before library preparation and sequenced on an Illumina NovaSeq 6000 platform generating 150-bp paired-end reads (Novogene Co., Ltd., Nanjing, China).
16S Ribosomal RNA Gene Analysis
The 16S rRNA gene sequence analysis was conducted using the Quantitative Insights Into Microbial Ecology (QIIME2) pipeline1 (Bolyen et al., 2019). DADA2 was used to filter low-quality sequences with lengths < 230 bp, remove chimeric sequences and singletons, and join the quality-filtered paired-end reads. Operational taxonomic units (OTUs) at ≥ 97% sequence similarity were generated by clustering sequences with the q2-vsearch method. After selecting a representative sequence for each OTU, sequences were aligned using mafft program, and the taxonomic identity was assigned using the Naive Bayes classifier with the SILVA 16S rRNA gene reference alignment database (release 123) (Pruesse et al., 2007) as the reference database. The taxonomic results were then generated at different levels for further analysis.
Metagenomic Assembly and Binning
For shotgun metagenomic sequences, raw paired-end reads were initially filtered using fastp (Chen et al., 2018). Paired-end reads were filtered when the number of low-quality bases (Q ≤ 5) exceeded 50% in any sequence read. Filtered reads were assembled using MEGAHIT version 1.2.2 (Li et al., 2015), specifying a range of k-mer size values (79–149) in 20-bp intervals and finally reserved contigs > 1,000 bp.
The filtered contigs were processed with BASALT (Binning Across a Series of AssembLies Toolkit) (Yu et al., 2021) to obtain bins. The completeness and contamination of the bins were then estimated using CheckM version 1.0.13 (Parks et al., 2015) with lineage-specific marker genes and default parameters. Bins that meet the MIMAG standard (completeness- 5 * contamination ≥ 50%) (Parks et al., 2017) were retained in this study as metagenome-assembled genomes (MAGs) to infer potential nitrogen metabolism functionalities. Further details of MAGs were described in Supplementary File B.
Taxonomic Assignment, Relative Abundance Calculations, and Functional Annotation of Metagenome-Assembled Genomes
One hundred twenty bacteria-specific protein sequences of conserved maker genes were identified from MAGs using GTDB-Tk version 0.3.2 (Chaumeil et al., 2019). Each protein sequence was individually aligned using hmmalign (Eddy, 2011) with default parameters. Individual alignments were concatenated and used as input to reconstruct the phylogenomic tree using the IQ-TREE version 1.6.12 (Nguyen et al., 2015) with the best-fit model of “LG + F + R10” and at 1,000 times bootstrapping (-b 1,000). Finally, the phylogenetic tree was visualized and edited in the iTOL version 4.4.22 online platform (Letunic and Bork, 2019).
The relative abundance of MAGs was calculated by the average coverage multiplying the length of each MAG divided by the total read base pairs in each sample (Olm et al., 2017), as shown in Eq. (1). The detailed steps were provided in Supplementary File A.
Genes were predicted from the MAGs using Prodigal version 2.6.2 (Hyatt et al., 2010), and predicted amino-acid sequences were annotated against the Kyoto Encyclopedia of Genes and Genomes database via the BLASTP program against the National Center for Biotechnology Information nr database with an E-value cutoff of 10––5. Nitrogen metabolic pathways were constructed using the Kyoto Encyclopedia of Genes and Genomes Mapper3 to visualize results.
Results
Membrane Bioreactor Performance
In the acclimation period, the acclimation of AnAOB was conducted by continuously increasing influent nitrite and ammonium concentrations (Figure 1A), with stable effluent nitrogen concentration observed (Figure 1B). The total nitrogen (TN) removal efficiency (Figure 1C) and nitrogen loading rate (Figure 1D) reached above 80% and 1,950 mg N–1 L–1 day–1 on the 25th day, whereas no significant fluctuation was observed after that, indicating the anammox system was successfully initiated. In this study, the system was maintained under stable conditions from the 51st day with NO2–-N, NH4+-N, and TN removal efficiencies at above 98, 91, and 87%, respectively. The MLVSS increased from 0.6 to 3.2338 g/L from inoculation to day 100. In the stabilization period, the average ratio of consumed nitrite over consumed ammonium (RS) and the ratio of produced nitrate over consumed ammonium (RP) values were 1.123 ± 0.021 and 0.172 ± 0.012, respectively.
In the perturbation period (days 110–127), no nitrite accumulation (Figure 1B) was observed, and the TN removal efficiency remained steady. However, in the inhibition period (days 127–188), the effluent NO2–-N concentration increased to 134 mg N L–1, whereas influent NH4+-N concentration continued to decrease (Figure 1A). The nitrogen removal efficiency was also observed to decline with fluctuations of both NH4+-N and NO2–-N removal efficiencies. In addition, the nitrogen removal rate declined from 1,670 to 1,458 mg N L–1 day–1, and the TN removal efficiency fluctuated within the range of 76–88% and decreased to a minimum of 76%. The NO2–-N and NH4+-N removal efficiencies were also unstable, whereas the NH4+-N removal efficiency generally decreased and reached a minimum of 76%. The MLVSS were 3.1857 and 3.1931 g/L on the 150th and 180th day in the inhibition period, respectively. In the recovery period, the influent ammonium concentration continued to decrease when the nitrite concentration remained steady (below 10 mg N L–1) (Figure 1B). The TN removal efficiency was recovered and maintained at approximately 90%, whereas NH4+-N and NO2–-N removal efficiency reached 96 and 99%, respectively, showing that the performance of MBR was restored.
Characteristic of Microbial Assemblages in the Membrane Bioreactor System
A total of 3,855 OTUs were obtained from the 16S rRNA gene metabarcoding profiling of the 23 MBR samples across 280 days. A total of nine bacterial phyla with an abundance higher than 1% were identified in the samples (Figure 2A). Planctomycetes were found to be the predominant bacterial phylum of the communities in phase I, although their relative abundance fluctuated from 30 to 76% among the samples. After Planctomycetes, the main phyla in descending order of relative abundance are Chloroflexi, Bacteroidetes, and Proteobacteria. Chloroflexi, Bacteroidetes, and Proteobacteria have often been identified alongside Planctomycetes in other bioreactor studies (Lawson et al., 2017; Yang et al., 2018; Keren et al., 2020), which usually contain DN and DNRA bacteria (Guo et al., 2016; Zhao et al., 2019; Pan et al., 2020). The relative abundance of Planctomycetes reached 68% after acclimation in phase I (Figure 2A), which contained a large proportion of AnAOB (Figure 2B). In phase II, the relative abundance of Planctomycetes declined rapidly to 48% in the nitrite inhibition period before bouncing back to 76% in the recovery period. The relative abundance of Chloroflexi, however, increased to a maximum of 25% in the inhibition period and decreased to 7% in the recovery period.
A total number of 215 genera were identified in the samples. Ca. Brocadia, categorized as an AnAOB, was the dominant genus throughout the entire experiment (Figure 2B), whereas their relative abundance increased from 19 to 64% in phase I in concert with high nitrogen removal efficiency in the reactor. These results indicate that AnAOB could properly consume nitrite and ammonium in this phase. On the 180th day of the experiment, the relative abundance of Ca. Brocadia decreased to 43% in concert with high effluent nitrite concentration but then recovered to 72% in the later periods, indicating a disruption occurred due to the high nitrite concentration in the MBR. Apart from Ca. Brocadia, two genera, Ignavibacterium and I-8, were also observed with a combined high relative abundance of 11 and 12% in the inhibition and adaptation periods, respectively.
Nitrogen Cycling Genes in the Metagenomic-Assembled Genomes Suggesting Nitrogen Removal Potentially Functioned in the Membrane Bioreactor
To precisely link microbial abundances to potential functions involved in nitrogen cycling, a metagenomic analysis was conducted to characterize the microbial functions in the whole reactor performance period. A total of 116 bins were recovered with metagenomic binning from six samples, of which 67 bins met the MIMAG standard (completeness - 5 * contamination ≥ 50% MAGs) and were considered as MAGs (Parks et al., 2017). These 67 MAGs were further analyzed and taxonomically classified based on phylogenetic analysis by taxonomic marker genes (Figure 3). The metabolic inference of these high-quality MAGs was then conducted to determine the nitrogen metabolic potential of the bacterial communities. A total of 58 MAGs across 12 phyla were involved in the nitrogen cycling activities. Two MAGs were taxonomically classified as Ca. Brocadia sapporoensis and Ca. Jettenia, respectively, with key functional genes for the anammox pathway observed, including hydrazine synthase (hzsABC) and hydrazine dehydrogenase (hdh), which reduce ammonium to hydrazine (N2H4) first and then N2. Therefore, the two MAGs were considered to represent AnAOB in the MBR. In addition to the AnAOB, 52 of 58 MAGs were found with potential DN functions, such as those with genes related to the first step of nitrite reduction (nirS/nirK), the second step of nitric oxide reduction (norBC), and the third step of nitrous oxide reduction (nosZ). Among these 52 MAGs, 30 MAGs with nirS or nirK genes were considered to be potentially involved in the nitrite reduction process. For the DNRA process, 38 of the 58 MAGs were found with DNRA functional genes (nirBD and nrfAH), which can potentially reduce nitrite to ammonium (Figure 3). Notably, 18 MAGs with potentials can only conduct the DN pathway (Figure 3).
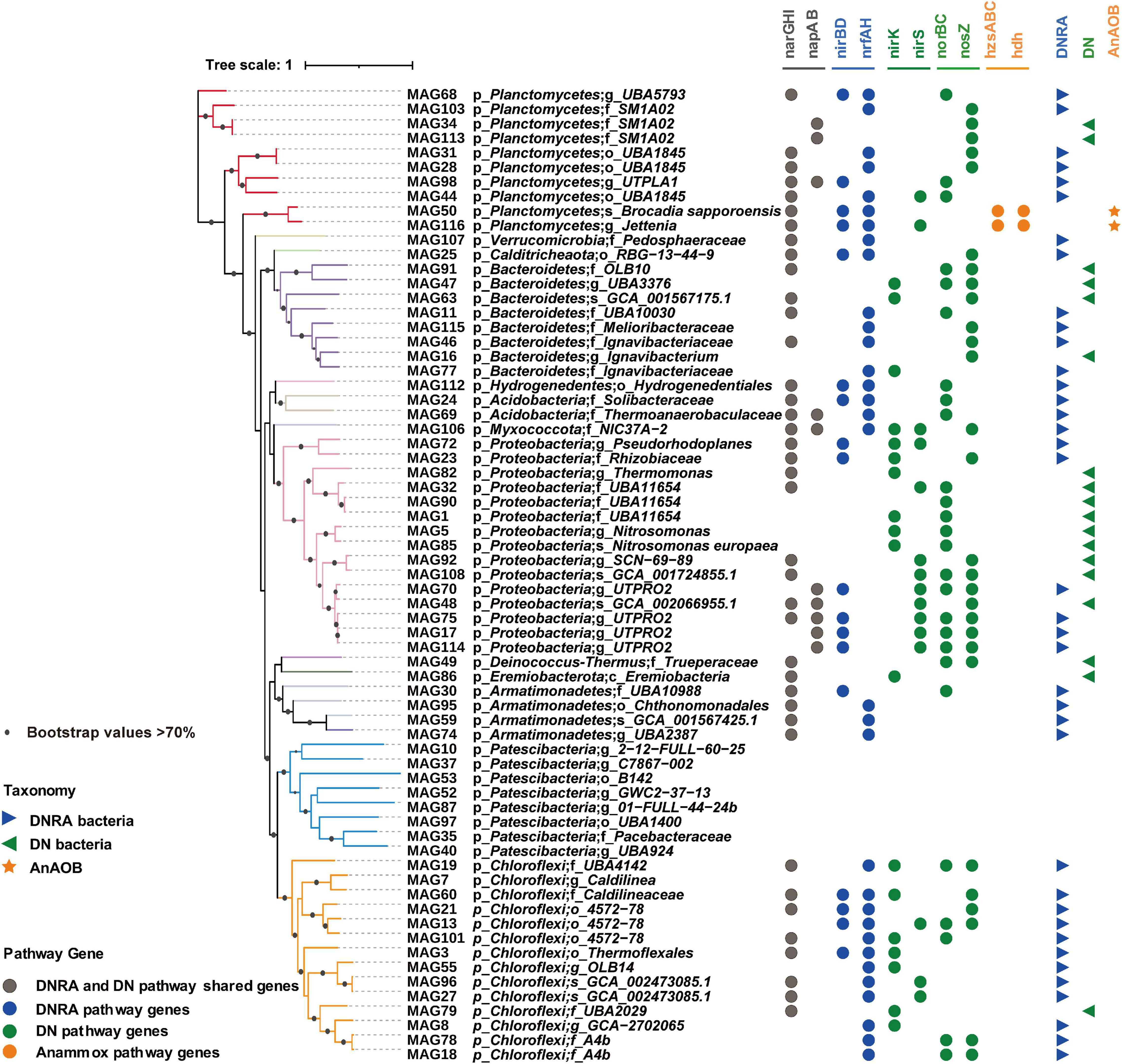
Figure 3. Phylogenomic reconstruction of high-quality MAGs from anaerobic ammonium oxidation (anammox) bioreactor communities. A phylogenetic tree was generated by maximum likelihood (ML) (inferred with IQ-tree and LG + F + R6 model) with 1,000 bootstrap replications. Different colored tree branches indicated different phylum levels. Bootstrap values > 70% were highlighted with black circles. Genes in various metabolic pathways were marked with different colored circles by iTOL version 4.4.2. Consequently, pathway of high-quality MAGs was summarized as follows: AnAOB (orange stars)—MAGs found with anammox genes (hzsABC/hdh), dissimilatory nitrate reduction (DNRA) bacteria (blue triangles pointing right)—MAGs found with DNRA genes (nirBD/nrfAH), and denitrifying only (DN) bacteria (green triangles pointing left)—MAGs only with DN genes (nirS/nirK/norBC/nosZ).
Dynamics of Anaerobic Ammonia-Oxidizing Bacteria, Denitrifying, and Dissimilatory Nitrate Reduction to Ammonium Bacteria in the Inhibition and Recovery Event
To assess the variation of AnAOB, DN, and DNRA throughout the entire experiment, the relative abundances of MAGs were estimated based on metagenomic reads calculations. Among all MAGs, MAG50 and MAG116 were found with AnAOB-related genes, considered representative AnAOB genomes. Although genes required for the DNRA pathway were also found in the two MAGs, the high ammonium removal rate caused by the anammox process makes it safe to assume that the MAG50 and MAG116 bacteria mainly performed the anammox pathway to remove nitrogen (Keren et al., 2020). The relative abundance of MAG116 was less than 1%; therefore, the analysis was focused on MAG50. When the influent ammonium was decreased (Figure 1A), a clear pattern of increasing nitrite and ammonium concentrations was observed (Figure 1B), along with a decreasing relative abundance of MAG50 (Ca. Brocadia sapporoensis) from 39 to 20% (Figure 4C), suggesting the inhibition of the MAG50 species took place. In the later adaptation and recovery periods, we observed a decreasing nitrite concentration (Figure 1B), coupled with the relative abundance of MAG50 bouncing back up to 28% (Figure 4B) and nitrogen removal rate restored, implying that the inhibition effect was mitigated and the performance of the MAG50 species may have recovered along with its potential functions.
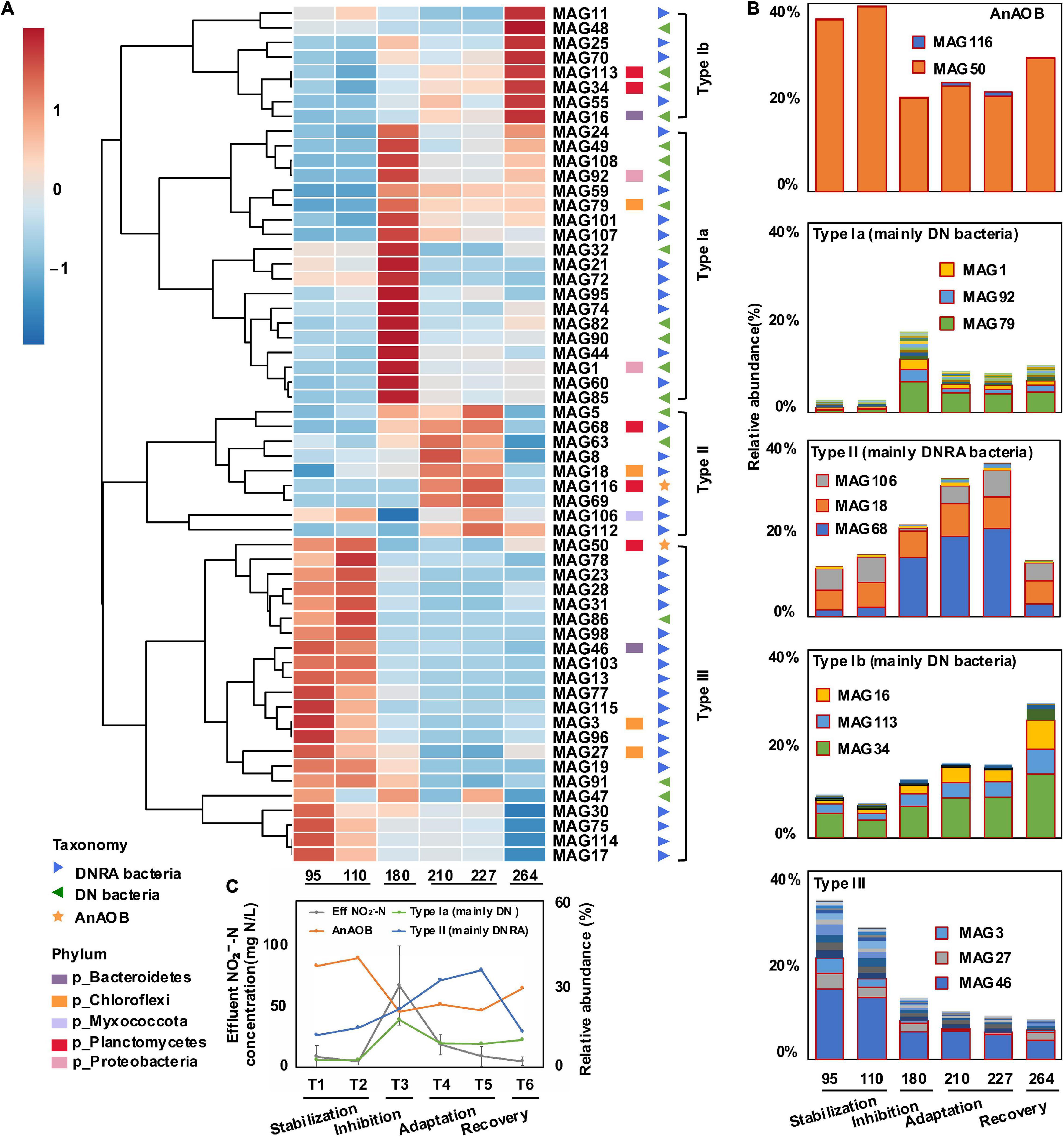
Figure 4. Analysis of bioreactor’s community clustering based on relative abundance of MAGs. A clustering heatmap of anammox (AnAOB), denitrifying (DN) bacteria, and dissimilatory nitrate reduction to ammonium (DNRA)-related metagenome-assembled genomes (MAGs) among experimental phases was generated at six time points (A). Rows were first normalized, and heatmap was created based on a Euclidean distance matrix and clustered with complete method. Color scale was marked with high positive correlation in red and negative correlation in blue. Relative abundance of AnAOB and type Ia, Ib, II, and III bacteria were shown in (B). MAGs with high relative abundance were marked in red boxes. Relative abundance of AnAOB, type Ib and type II bacteria at six time points (day 95, day 110, day 180, day 210, day 227, and day 264) as well as average effluent NO2–-N around sample collection time (T1: days 51–95, T2: days 96–110, T3: days 150–180, T4: days 188–210, T5: days 211–227, and T6: day 228–264) were shown in (C).
To further unfold how AnAOB was recovered and how DN/DNRA bacteria performed in these periods, a correlation analysis that linked nitrogen cycle genes with relative abundances of MAGs was conducted. Based on the dynamics of bacterial populations throughout the four periods, representative MAGs were clustered into three groups: type I (mainly DN bacteria), type II (mainly DNRA bacteria), and type III bacteria (mainly DNRA bacteria) (Figure 4B). Type I bacteria were further separated into two subgroups, types Ia and Ib, based on their rapid increasing time in the inhibition and recovery periods, respectively (Figures 4A,B). The MAG79 (family UBA2029 from Chloroflexi), MAG92 (genus SCN-69-89 from Proteobacteria), and MAG1 (family UBA11654 from Proteobacteria) were found as the three most abundant type Ia MAGs, whereas the MAG 34 (family SM1A02 from phylum Planctomycetes), MAG113 (family SM1A02 from phylum Planctomycetes), and MAG16 (genus Ignavibacterium from Bacterioidetes) were those of type Ib MAGs. Notably, all these three MAGs in the type I group affiliate to DN bacteria. The relative abundance of type II bacteria increased mainly in the adaptation period, including MAG68 (genus UBA5793 from Planctomycetes), MAG18 (family A4B from Chloroflexi), and MAG106 (family NIC37A-2 from Myxococcota) affiliating to DNRA bacteria. Type III bacteria showed a continuous relative abundance decrease from the inhibition to adaptation, containing MAG46 (family Ignavibacteriaceae from Bacteroidetes), MAG27 (GCA_002473085.1 from Chloroflexi), and MAG3 (order Thermoflexales from Chloroflexi) affiliating to DNRA bacteria.
In the inhibition period, the relative abundance of type Ia bacteria increased rapidly from 3 to 17% (Figure 4C), whereas that of other bacterial groups either increased slowly (e.g., type Ib and II bacteria) or decreased (e.g., type III bacteria). In the adaptation period, the relative abundances of AnAOB were 22 and 20% on 210th and 227th day, respectively. The relative abundance of type II bacteria showed a major increase from 20 to 35%, whereas that of type Ia bacteria decreased from 17 to 8% along with a slow increase of that from 12 to 15% for type Ib bacteria. In the recovery period, the relative abundance of type II bacteria dropped from 35 to 13%, the same level as in the stabilization period, whereas that of type Ib bacteria had a rapid increase from 15 to 29% (Figure 4B). The relative abundance of type III bacteria, however, decreased steadily from 34 to 8% (Figure 4B).
Discussion
In this study, we quickly established a laboratory-scale anammox-based nitrogen removal process using the MBR. Overall, a high and stable nitrogen removal rate had been achieved in the MBR after 51 days of operation and lasted in the stabilization period, indicating that high AnAOB activity had been achieved in the MBR. This coincided with the increase and stabilization of the relative abundance of Planctomycetes, especially the genus Ca. Brocadia. In the inhibition period, the low nitrogen removal efficiency and decreased relative abundance of Ca. Brocadia strongly suggested a disrupted anammox system, which is likely attributed to inhibiting AnAOB by a high nitrite concentration (Li et al., 2019; Lin et al., 2020; Pradhan et al., 2020). The concentration of the MLVSS has no significant change between the stabilization and inhibition periods, suggesting that the microbial biomass was maintained at a stable level. Therefore, we confer that the relative abundance of the microbial community at different time points was comparable. At the phylum level, the relative abundance of Chloroflexi increased, which is highly reasonable. Chloroflexi bacteria are highly active in protein degradation, which caters to the metabolism of soluble microbial products and extracellular polymeric substances derived from autotrophic organisms such as AnAOB in the MBR (Kindaichi et al., 2012). Besides, Chloroflexi bacteria play an essential role in enhancing the particle structure of the sludge by producing filamentous biomass networks on the sludge flocs and granules (Kragelund et al., 2011; Guo and Zhang, 2012; Kindaichi et al., 2012). At the genus level, Ignavibacterium was the most abundant except Ca. Brocadia, whose relative abundance variation was also related to the nitrite concentration change. Some Ignavibacterium spp. were previously reported contributing to the nitrogen removal through the nitrite or nitrate pathway (Yang et al., 2017), whereas some other Ignavibacterium spp. were capable of heterotrophic denitrification (Liu et al., 2012; Oren, 2014). In the recovery period, we may imply the recovery of AnAOB by the restoration of nitrogen removal efficiency (Zekker et al., 2015; Zhang et al., 2016). It is critical to explore such a recovery process because it may directly or indirectly be linked with certain bacteria such as DNRA and DN, which can consume the excess nitrite in the inhibition and recovery period. Therefore, we divided these MAGs into three groups: type I separating into two subgroups: types Ia and Ib (mainly DN bacteria), type II (mainly DNRA bacteria), and type III bacteria (mainly DNRA bacteria) (Figure 4B).
Based on these three groups, we further analyzed the dynamics of microbial population in the event of anammox inhibition and restoration to dissect the potential functions of nitrogen cycling bacteria. The results show the relative abundance of type Ia bacteria increased rapidly along with nitrite concentration, whereas other bacterial groups increased slowly or decreased in the inhibition period. We infer that type Ia bacteria are better adapted to the high nitrite concentration (60.55 ± 29.20 mg N L–1). The nitrogen removal efficiency increased from a minimum of 76–89% from days 170 to 188 in the inhibition period (Figure 1C), indicating excess nitrite was consumed. Therefore, type Ia bacteria may serve as pioneers to consume the excess nitrite. Such speculation is supported by a previous study, which revealed DN bacteria from Proteobacteria could aid in reducing nitrite to nitrogen gas. Based on the metagenomic analysis, the nitrite denitrification genes in DN bacteria had higher abundance after the inhibition phase, and their cooperation could prevent the nitrite inhibition of anammox bacteria when the influent nitrite concentration was higher (Zhao et al., 2019). Therefore, we assume that type Ia DN bacteria are important nitrite scavengers at high nitrite concentrations.
In the adaptation period, nitrite concentration rapidly dropped (15.33 ± 9.52 mg N L–1), whereas the relative abundance of AnAOB did not fluctuate significantly, suggesting that the anammox bacteria may not recover under this nitrite concentration. On the other hand, we observed a major increase of type II bacteria, a decrease of type Ia bacteria, and a slow rise in type Ib. This shows that type I bacteria (mainly DN bacteria) were outcompeted by type II bacteria in this period. The competition mechanisms between the two under high nitrite concentration conditions remain to be revealed in future studies. More importantly, nitrite and ammonium concentrations decreased in the adaptation period compared with inhibition. The effluent nitrite concentration maintained at 19 ± 8 mg N L–1, whereas the ammonium concentration was observed with an increase from 41 to 78 mg N L–1 before day 215 in the adaptation period (Figure 1B) (effluent NH4+-N/NO2-N ratio increased from 2.48 to 3.66). As DNRA can provide NH4+ for the anammox process in NH4+-limiting environments (Wang et al., 2018), the ammonium accumulation might be attributed to the DNRA process, which might be critical in triggering the recovery of AnAOB. Therefore, apart from type Ia bacteria that reduce nitrite concentration, type II bacteria might be another key player in assisting the AnAOB recovery.
In the recovery period, the nitrite concentration decreased to a low level of 4.63 ± 3.66 mg N L–1 (Figure 4C), whereas AnAOB and nitrogen removal efficiency (Figure 1C) were observed to have gradually recovered and stabilized. The relative abundance of type II bacteria again dropped to the same level as in the stabilization period. On the other hand, the relative abundance of type III bacteria decreased steadily, and type Ib bacteria increased rapidly, suggesting that type III DNRA bacteria (Figure 4B) were irreversibly stressed under high nitrite concentration (Figure 4C) and replaced by type Ib bacteria. The observations discussed earlier imply that the anammox performance was restored along with the shift of the microbial community. In the restored anammox system, although the relative abundance of type II bacteria remained similar to that under the initial conditions, type III bacteria were substituted by type I bacteria (mostly type Ib). It is indicated that different niches of bacteria may occur after inhibition in the MBR system, which contributes to the stability of the micro-ecosystem.
Conclusion
In this study, a laboratory-scale anammox bioreactor was used to conduct an investigation of the microbial shift and functional interactions of AnAOB, DN, and DNRA bacteria during a long-term nitrite inhibition to eventual self-recovery episode. The relative abundance of AnAOB first decreased due to high nitrite concentration, which lowered the system’s nitrogen removal efficiency but then recovered automatically without any external interference. By analyzing the relationship between the relative abundances of MAGs and their nitrogen metabolism pathways, we found that type Ia (mainly DN) and type II (mainly DNRA) bacteria outcompeted other bacteria in the inhibition and adaptation periods, respectively. They were capable of living under high nitrite concentration conditions and potentially served as a nitrite scavenger that could trigger the recovery of AnAOB from the nitrite inhibition. Our results provide a possible mechanistic explanation for the performance shift of the anammox bioreactor during a long-term nitrite inhibition to the eventual self-recovery episode and advance the stable control of this promising technology.
Data Availability Statement
The datasets presented in this study can be found in online repositories. The names of the repository/repositories and accession number(s) can be found below: https://www.ncbi.nlm.nih.gov/, PRJNA608637.
Author Contributions
XQ: conceptualization, methodology, experimental operation, software, investigation, data analysis, writing—original draft, and visualization. LZha: methodology, experimental operation, software, investigation, and data analysis. ZQ and LW: methodology, data analysis, and revision. YaW and YuW: bioreactor maintenance and operation. CD: methodology and software. JS: methodology. XZ: experimental operation. BL, LZho, AM, and W-QZ: revision. KY: supervision, funding acquisition, resources, and revision. All authors contributed to the article and approved the submitted version.
Funding
This study was financially supported by the National Natural Science Foundation of China (no. 51709005), the Shenzhen Knowledge Innovation Program Basic Research Project (JCYJ20190808183205731) and the Class D project of Shenzhen and Hong Kong Innovation Circle (SGDX2019081623101948). This study was also supported by the Shenzhen Municipal Development and Reform Commission (discipline construction of watershed ecological engineering). We are grateful for the Catalyst: Leaders funding (CHN-UOA1601) provided by the New Zealand Ministry of Business, Innovation, and Employment and administered by the Royal Society Te Apârangi.
Conflict of Interest
The authors declare that the research was conducted in the absence of any commercial or financial relationships that could be construed as a potential conflict of interest.
Publisher’s Note
All claims expressed in this article are solely those of the authors and do not necessarily represent those of their affiliated organizations, or those of the publisher, the editors and the reviewers. Any product that may be evaluated in this article, or claim that may be made by its manufacturer, is not guaranteed or endorsed by the publisher.
Acknowledgments
We are grateful for the support of Peking University’s high-performance computing platform.
Supplementary Material
The Supplementary Material for this article can be found online at: https://www.frontiersin.org/articles/10.3389/fmicb.2021.781156/full#supplementary-material
Footnotes
- ^ https://qiime2.org
- ^ https://itol.embl.de/personal_page.cgi
- ^ http://www.genome.jp/kegg/tool/map_pathway.html
References
Alejandro, G. M., Barbara, M. P., Alejandro, R. S., and Jesus, G. L. (2018). New concepts in anammox processes for wastewater nitrogen removal: recent advances and future prospects. FEMS Microbiol. Lett. 365: fny031.
APHA (2005). Standard Methods for the Examination of Water and Wastewater. Washington DC: American Public Health Association Inc.
APHA (2012). Standard Methods for the Examination of Water and Wastewater. Washington, DC: American Public Health Association.
Bolyen, E., Rideout, J. R., Dillon, M. R., Bokulich, N. A., Abnet, C. C., Al-Ghalith, G. A., et al. (2019). Author correction: reproducible, interactive, scalable and extensible microbiome data science using QIIME 2. Nat. Biotechnol. 37, 1091–1091. doi: 10.1038/s41587-019-0252-6
Bonassa, G., Chiapetti Bolsan, A., Venturin, B., Celant De Prá, M., Goldschmidt Antes, F., Ester Hollas, C., et al. (2021). A new kinetic model to predict substrate inhibition and better efficiency in an airlift reactor on deammonification process. Bioresour. Technol. 319:124158. doi: 10.1016/j.biortech.2020.124158
Chaumeil, P. A., Mussig, A. J., Hugenholtz, P., and Parks, D. H. (2019). GTDB-Tk: a toolkit to classify genomes with the Genome Taxonomy Database. Bioinformatics 36, 1925–1927. doi: 10.1093/bioinformatics/btz848
Chen, S. F., Zhou, Y. Q., Chen, Y. R., and Gu, J. (2018). fastp: an ultra-fast all-in-one FASTQ preprocessor. Bioinformatics 34, 884–890. doi: 10.1093/bioinformatics/bty560
Cho, S., Kambey, C., and Nguyen, V. K. (2020). Performance of anammox processes for wastewater treatment: a critical review on effects of operational conditions and environmental stresses. Water 12:20.
Chu, Z., Wang, K., Li, X., Zhu, M., Yang, L., and Zhang, J. (2015). Microbial characterization of aggregates within a one-stage nitritation–anammox system using high-throughput amplicon sequencing. Chem. Eng. J. 262, 41–48.
Ciesielski, S., Czerwionka, K., Sobotka, D., Dulski, T., and Makinia, J. (2018). The metagenomic approach to characterization of the microbial community shift during the long-term cultivation of anammox-enriched granular sludge. J. Appl. Genet. 59, 109–117. doi: 10.1007/s13353-017-0418-1
Eddy, S. R. (2011). Accelerated profile HMM searches. PLoS Comput. Biol. 7:e1002195. doi: 10.1371/journal.pcbi.1002195
Gonzalez-Gil, G., Sougrat, R., Behzad, A. R., Lens, P. N. L., and Saikaly, P. E. (2015). Microbial community composition and ultrastructure of granules from a full-scale anammox reactor. Microb. Ecol. 70, 118–131. doi: 10.1007/s00248-014-0546-7
Guo, F., and Zhang, T. (2012). Profiling bulking and foaming bacteria in activated sludge by high throughput sequencing. Water Res. 46, 2772–2782. doi: 10.1016/j.watres.2012.02.039
Guo, J., Peng, Y., Fan, L., Zhang, L., Ni, B. J., Kartal, B., et al. (2016). Metagenomic analysis of anammox communities in three different microbial aggregates. Environ. Microbiol. 18, 2979–2993. doi: 10.1111/1462-2920.13132
Hou, X., Liu, S., and Feng, Y. (2017). The autofluorescence characteristics of bacterial intracellular and extracellular substances during the operation of anammox reactor. Sci. Rep. 7:39289. doi: 10.1038/srep39289
Hyatt, D., Chen, G., LoCascio, P. F., Land, M. L., Larimer, F. W., and Hauser, L. J. (2010). Prodigal: prokaryotic gene recognition and translation initiation site identification. BMC Bioinformatics 11:119. doi: 10.1186/1471-2105-11-119
Ibrahim, M., Yusof, N., Mohd Yusoff, M. Z., and Hassan, M. A. (2016). Enrichment of anaerobic ammonium oxidation (anammox) bacteria for short start-up of the anammox process: a review. Desalin. Water Treat. 57, 13958–13978. doi: 10.1080/19443994.2015.1063009
Ismail, S., Elsamadony, M., Elreedy, A., Fujii, M., and Tawfik, A. (2019). Physico-chemical and microbial characterization of compartment-wise profiles in an anammox baffled reactor. J. Environ. Manage. 232, 875–886. doi: 10.1016/j.jenvman.2018.11.134
Keren, R., Lawrence, J. E., Zhuang, W. Q., Jenkins, D., Banfield, J. F., Alvarez-Cohen, L., et al. (2020). Increased replication of dissimilatory nitrate-reducing bacteria leads to decreased anammox bioreactor performance. Microbiology 8:7. doi: 10.1186/s40168-020-0786-3
Kindaichi, T., Yuri, S., Ozaki, N., and Ohashi, A. (2012). Ecophysiological role and function of uncultured Chloroflexi in an anammox reactor. Water Sci. Technol. 66, 2556–2561. doi: 10.2166/wst.2012.479
Kragelund, C., Thomsen, T. R., Mielczarek, A. T., and Nielsen, P. H. (2011). Eikelboom’s morphotype 0803 in activated sludge belongs to the genus Caldilinea in the phylum Chloroflexi. FEMS Microbiol. Ecol. 76, 451–462.
Lawson, C. E., Wu, S., Bhattacharjee, A. S., Hamilton, J. J., McMahon, K. D., Goel, R., et al. (2017). Metabolic network analysis reveals microbial community interactions in anammox granules. Nat. Commun. 8:15416.
Letunic, I., and Bork, P. (2019). Interactive Tree Of Life (iTOL) v4: recent updates and new developments. Nucleic Acids Res. 47, W256–W259.
Li, D., Liu, C., Luo, R., Sadakane, K., and Lam, T. (2015). MEGAHIT: an ultra-fast single-node solution for large and complex metagenomics assembly via succinct de Bruijn graph. Bioinformatics 31, 1674–1676.
Li, W., Zhuang, J. L., Zhou, Y. Y., Meng, F. G., Kang, D., Zheng, P., et al. (2020). Metagenomics reveals microbial community differences lead to differential nitrate production in anammox reactors with differing nitrogen loading rates. Water Res. 169:115279. doi: 10.1016/j.watres.2019.115279
Li, X., Yuan, Y., Huang, Y., Bi, Z., and Lin, X. (2019). Inhibition of nitrite oxidizing bacterial activity based on low nitrite concentration exposure in an auto-recycling PN-Anammox process under mainstream conditions. Bioresour. Technol. 281, 303–308. doi: 10.1016/j.biortech.2019.02.114
Liang, Y. H., Li, D., Zhang, X. J., Zeng, H. P., Yang, Y., and Zhang, J. (2015). Nitrate removal by organotrophic anaerobic ammonium oxidizing bacteria with C2/C3 fatty acid in upflow anaerobic sludge blanket reactors. Bioresour. Technol. 193, 408–414. doi: 10.1016/j.biortech.2015.06.133
Lin, L., Pratt, S., Rattier, M., and Ye, L. (2020). Individual and combined effect of salinity and nitrite on freshwater Anammox bacteria (FAB). Water Res. 169, 114931. doi: 10.1016/j.watres.2019.114931
Liu, Z., Li, D., Yu, L., and Niu, F. (2012). Gallic acid as a cancer-selective agent induces apoptosis in pancreatic cancer cells. Chemotherapy 58, 185–194. doi: 10.1159/000337103
Lotti, T., van der Star, W. R. L., Kleerebezem, R., Lubello, C., and Van Loosdrecht, M. C. M. (2012). The effect of nitrite inhibition on the anammox process. Water Res. 46, 2559–2569. doi: 10.1016/j.watres.2012.02.011
Ma, C., Jin, R., Yang, G., Yu, J., Xing, B., and Zhang, Q. (2012). Impacts of transient salinity shock loads on anammox process performance. Bioresour. Technol. 112, 124–130.
Nguyen, L. T., Schmidt, H. A., von Haeseler, A., and Minh, B. Q. (2015). IQ-TREE: a fast and effective stochastic algorithm for estimating maximum-likelihood phylogenies. Mol. Biol. Evol. 32, 268–274. doi: 10.1093/molbev/msu300
Olm, M. R., Brown, C. T., Brooks, B., Firek, B., Baker, R., Burstein, D., et al. (2017). Identical bacterial populations colonize premature infant gut, skin, and oral microbiomes and exhibit different in situ growth rates. Genome Res. 27, 601–612. doi: 10.1101/gr.213256.116
Oren, A. (2014). “The family Rhodocyclaceae,” in The Prokaryotes: Alphaproteobacteria and Betaproteobacteria, eds E. Rosenberg, E. F. DeLong, S. Lory, E. Stackebrandt, and F. Thompson (Berlin: Springer), 975–998.
Pan, H. W., Yuan, D. D., Liu, W. Y., Pi, Y. X., Wang, S. Y., and Zhu, G. B. (2020). Biogeographical distribution of dissimilatory nitrate reduction to ammonium (DNRA) bacteria in wetland ecosystems around the world. J. Soil Sediments 20, 3769–3778. doi: 10.1007/s11368-020-02707-y
Parks, D. H., Imelfort, M., Skennerton, C. T., Hugenholtz, P., and Tyson, G. W. (2015). CheckM: assessing the quality of microbial genomes recovered from isolates, single cells, and metagenomes. Genome Res. 25, 1043–1055. doi: 10.1101/gr.186072.114
Parks, D. H., Rinke, C., Chuvochina, M., Chaumeil, P.-A., Woodcroft, B. J., Evans, P. N., et al. (2017). Recovery of nearly 8,000 metagenome-assembled genomes substantially expands the tree of life. Nat. Microbiol. 2, 1533–1542. doi: 10.1038/s41564-017-0012-7
Pradhan, N., Swa Thi, S., and Wuertz, S. (2020). Inhibition factors and kinetic model for anaerobic ammonia oxidation in a granular sludge bioreactor with Candidatus Brocadia. Chem. Eng. J. 389:123618. doi: 10.1016/j.cej.2019.123618
Pruesse, E., Quast, C., Knittel, K., Fuchs, B. M., Ludwig, W., Peplies, J., et al. (2007). SILVA: a comprehensive online resource for quality checked and aligned ribosomal RNA sequence data compatible with ARB. Nucleic Acids Res. 35, 7188–7196. doi: 10.1093/nar/gkm864
Ren, Y., Li, D., Li, X., Yang, L., Ding, A., and Zhang, J. (2014). High-rate nitrogen removal and microbial community of an up-flow anammox reactor with ceramics as biomass carrier. Chemosphere 113, 125–131. doi: 10.1016/j.chemosphere.2014.04.113
Sun, Y., Guan, Y., Zeng, D., He, K., and Wu, G. (2018). Metagenomics-based interpretation of AHLs-mediated quorum sensing in Anammox biofilm reactors for low-strength wastewater treatment. Chem. Eng. J. 344, 42–52. doi: 10.1016/j.cej.2018.03.047
Tang, X., Guo, Y. Z., Jiang, B., and Liu, S. T. (2018). Metagenomic approaches to understanding bacterial communication during the anammox reactor start-up. Water Res. 136, 95–103. doi: 10.1016/j.watres.2018.02.054
Tao, Y., Gao, D. W., Fu, Y., Wu, W. M., and Ren, N. Q. (2012). Impact of reactor configuration on anammox process start-up: MBR versus SBR. Bioresour. Technol. 104, 73–80.
van der Star, W. R. L., Abma, W. R., Blommers, D., Mulder, J.-W., Tokutomi, T., Strous, M., et al. (2007). Startup of reactors for anoxic ammonium oxidation: experiences from the first full-scale anammox reactor in Rotterdam. Water Res. 41, 4149–4163. doi: 10.1016/j.watres.2007.03.044
Wang, S. Y., Wang, W. D., Liu, L., Zhuang, L. J., Zhao, S. Y., Su, Y., et al. (2018). Microbial nitrogen cycle hotspots in the plant-bed/ditch system of a constructed wetland with N2O mitigation. Environ. Sci. Technol. 52, 6226–6236.
Wang, Y. Y., Ma, X., Zhou, S., Lin, X. M., Ma, B., Park, H.-D., et al. (2016). Expression of the nirS, hzsA, and hdh genes in response to nitrite shock and recovery in Candidatus Kuenenia stuttgartiensis. Environ. Sci. Technol. 50, 6940–6947. doi: 10.1021/acs.est.6b00546
Wu, Y., Wang, Y. X., De Costa, Y. G., Tong, Z. D., Cheng, J. J., Zhou, L. J., et al. (2019). The co-existence of anammox genera in an expanded granular sludge bed reactor with biomass carriers for nitrogen removal. Appl. Microbiol. Biotechnol. 103, 1231–1242. doi: 10.1007/s00253-018-9494-3
Yang, W., He, S. L., Han, M., Wang, B. B., Niu, Q. G., Xu, Y. J., et al. (2018). Nitrogen removal performance and microbial community structure in the start-up and substrate inhibition stages of an anammox reactor. J. Biosci. Bioeng. 126, 88–95. doi: 10.1016/j.jbiosc.2018.02.004
Yang, Y. D., Zhang, L., Cheng, J., Zhang, S. J., and Peng, Y. Z. (2017). Microbial community evolution in partial nitritation/anammox process: from sidestream to mainstream. Bioresour. Technol. 251, 327–333.
Yu, K., Qiu, Z., Mu, R., Qiao, X., and Zhuang, W. (2021). Recovery of high-qualitied genomes from a deep-inland Salt Lake using BASALT. bioRxiv [Preprint]. doi: 10.1101/2021.03.05.434042
Zekker, I., Rikmann, E., Kroon, K., Mandel, A., Mihkelson, J., Tenno, T., et al. (2017). Ameliorating nitrite inhibition in a low-temperature nitritation–anammox MBBR using bacterial intermediate nitric oxide. Int. J. Environ. Sci. Technol. 14, 2343–2356. doi: 10.1007/s13762-017-1321-3
Zekker, I., Rikmann, E., Tenno, T., Loorits, L., Kroon, K., Fritze, H., et al. (2015). Nitric oxide for anammox recovery in a nitrite-inhibited deammonification system. Environ. Technol. 36, 2477–2487. doi: 10.1080/09593330.2015.1034791
Zhang, Y. L., Niu, Q. G., Ma, H. Y., He, S. L., Kubota, K., and Li, Y. Y. (2016). Long-term operation performance and variation of substrate tolerance ability in an anammox attached film expanded bed (AAFEB) reactor. Bioresour. Technol. 211, 31–40.
Zhao, Y. P., Jiang, B., Tang, X., and Liu, S. T. (2019). Metagenomic insights into functional traits variation and coupling effects on the anammox community during reactor start-up. Sci. Total Environ. 687, 50–60. doi: 10.1016/j.scitotenv.2019.05.491
Zhou, S., Borjigin, S., Riya, S., Terada, A., and Hosomi, M. (2014). The relationship between anammox and denitrification in the sediment of an inland river. Sci. Total Environ. 490, 1029–1036. doi: 10.1016/j.scitotenv.2014.05.096
Keywords: nitrite inhibition, anaerobic ammonium oxidation bacteria, dissimilatory nitrate reduction to ammonium bacteria, nitrogen metabolic pathways, denitrifying bacteria
Citation: Qiao X, Zhang L, Qiu Z, Wang L, Wu Y, Deng C, Su J, Zhang X, Wang Y, Li B, Zhou L, Ma AYW, Zhuang W-Q and Yu K (2022) Specific Denitrifying and Dissimilatory Nitrate Reduction to Ammonium Bacteria Assisted the Recovery of Anammox Community From Nitrite Inhibition. Front. Microbiol. 12:781156. doi: 10.3389/fmicb.2021.781156
Received: 22 September 2021; Accepted: 24 November 2021;
Published: 20 January 2022.
Edited by:
Yifeng Zhang, Technical University of Denmark, DenmarkReviewed by:
Guo-Jun Xie, Harbin Institute of Technology, ChinaYou-Peng Chen, Chongqing University, China
Copyright © 2022 Qiao, Zhang, Qiu, Wang, Wu, Deng, Su, Zhang, Wang, Li, Zhou, Ma, Zhuang and Yu. This is an open-access article distributed under the terms of the Creative Commons Attribution License (CC BY). The use, distribution or reproduction in other forums is permitted, provided the original author(s) and the copyright owner(s) are credited and that the original publication in this journal is cited, in accordance with accepted academic practice. No use, distribution or reproduction is permitted which does not comply with these terms.
*Correspondence: Ke Yu, yuke@pkusz.edu.cn; orcid.org/0000-0001-5039-6056