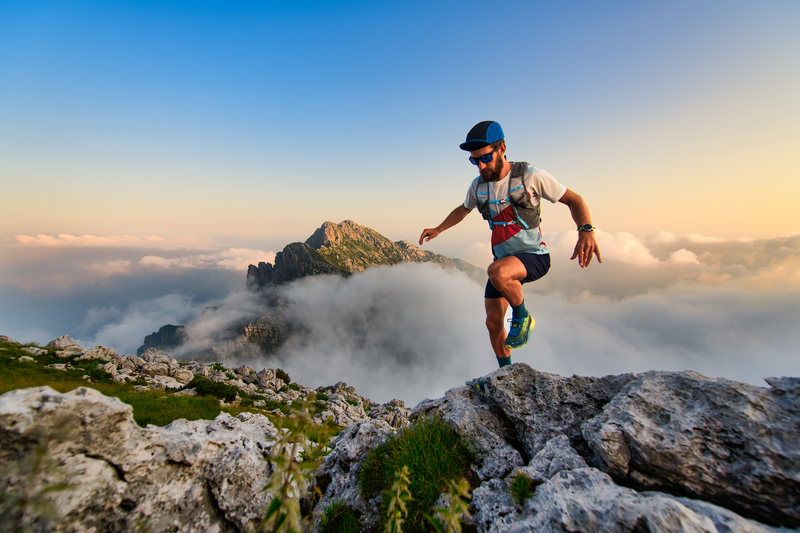
94% of researchers rate our articles as excellent or good
Learn more about the work of our research integrity team to safeguard the quality of each article we publish.
Find out more
ORIGINAL RESEARCH article
Front. Microbiol. , 25 October 2021
Sec. Microorganisms in Vertebrate Digestive Systems
Volume 12 - 2021 | https://doi.org/10.3389/fmicb.2021.759432
Using two successive types of diets (100% concentrate and 67% forage), this study explores the relationship between the ruminal microbiota of 78 Romane lambs and their feed efficiency (residual feed intake trait) or feeding behavior (feeding rate trait). Analysis was carried out phenotypically by correlating feed efficiency or feeding behavior traits with the relative abundance of bacteria at the phylum, family, and genus levels, and then genetically by comparing the microbiota of lambs selected for extreme breeding values for residual feed intake or feeding rate. Our results confirmed the major effect of diet on the ruminal microbiota composition. The microbiota of lambs consuming a forage-based diet was distinguished by higher microbial diversity and also by higher relative abundance of Firmicutes, whereas Bacteriodetes and Actinobacteria were relatively more abundant in the microbiota of lambs consuming a concentrate-based diet. Moreover, the comparison of lambs divergent for residual feed intake breeding values revealed that regardless of diet, more efficient lambs possessed a ruminal microbiota enriched in Coprococcus, Moryella, [Eubacterium] Brachy group, and [Eubacterium] hallii group, but depleted in Lachnospiraceae FD2005 and Shuttleworthia. The connection between microbiota composition and feeding rate was more tenuous, with no link between the abundance of particular genera and lambs genetically divergent for feeding rate.
Feed efficiency is one of the most important phenotypic traits for animal farmers. Residual feed intake (RFI) is the most commonly used method to estimate feed efficiency, defined as the difference between the true and estimated feed intake of animals according to their body weight (BW), average daily gain (ADG), and body composition. A high RFI value characterizes low-efficiency animals since they eat more than the average population, whereas a low RFI value characterizes highly efficient animals. Selecting animals for feed efficiency is a strong lever for agroecological breeding. Individual variations in RFI can be due to a variety of factors, including ruminal digestion and the ruminal microbiome (Løvendahl et al., 2018). Indeed, the latter plays a central role in the nutrition of its host from a digestive and metabolic point of view, which directly affects feed efficiency. In particular, ruminal bacteria produce volatile fatty acids from plant-derived carbohydrates, representing approximately 70% of the animal’s energy needs (Flint et al., 2008). Beyond selection, the ruminal microbiota also provides an opportunity to control feed efficiency through dietary manipulation (Malmuthuge and Guan, 2017), which can be easily applied by farmers. Consequently, understanding host-ruminal microbiota interactions is crucial for improving farming efficiency.
Studies have reported a link between ruminal bacteria and RFI in beef cattle (Carberry et al., 2012; Hernandez-Sanabria et al., 2012; Li and Guan, 2017), dairy cows (Rius et al., 2012; Jami et al., 2014; Jewell et al., 2015), and more recently in sheep (Ellison et al., 2017; Perea et al., 2017). Among these studies, the microbiota of high-RFI (low-efficiency) animals presented increased abundance of some fibrolytic (such as Ruminoccocus, Butyrivibrio, or Fibrobacter) and lactolytic (such as Anaerovibrio or Dialister) genera compared to that of low-RFI animals. Moreover, the effect of RFI on the microbiota was greatly dependent on diet (Carberry et al., 2012; Hernandez-Sanabria et al., 2012; Ellison et al., 2017), although the first two studies used a non-exhaustive approach with gel electrophoresis (DGGE) to analyze the microbiota and the last study compared two groups of lambs that received different diets based on alfalfa pellets or corn. All three studies allocated conventional non-selected animals to their phenotypic RFI; however, the effect of diet was not measured in the same animals.
The feeding behavior of ruminants has been much less explored than RFI in relation to the ruminal microbiota. Breton et al. (2016) demonstrated that multiplication of Escherichia coli in mouse intestinal microbiota modified the animal’s satiety and feeding behavior, leading to anorexia or bulimia. Therefore, it would be interesting to examine the relationship between feeding behavior (especially feeding rate, FR) and ruminal microbiota composition. The development of automatic feeders to record feed intake and feeding duration at the visit level has enabled the analysis of feeding behavior traits. Interestingly, the FR of Romane male lambs, defined as the ratio between feed intake and feeding duration, is a heritable trait (h2 = 0.37 ± 0.06) that seems to be genetically independent of RFI (Rg = 0.08 ± 0.16) (Marie-Etancelin et al., 2019). It is thus possible to study the impact of feeding behavior and feed efficiency on ruminal microbiota without confusion between both traits.
The aim of this study was to analyze the effect of genetic selection for RFI or FR on the ruminal microbiota of Romane lambs fed two different diets, rich either in concentrate or forage.
A total of 78 Romane male lambs, bred at the INRAE Experimental Unit of La Sapinière (UE 332 agreement D18-174-01; Osmoy, France) in 2016, were used in this study. The lambs were reared indoors on deep straw. After weaning at 9 weeks of age, the lambs weighed an average of 26.2 kg and were adapted to a 100% concentrate diet. The experiment began at 12 weeks of age and lasted until 37 weeks of age (Figure 1). During these 26 weeks, the lambs were fed ad libitum by automatic feeders, which recorded individual feed intake and feeding duration at each visit. Each animal underwent two 8-week periods of feeding recording: the first period when lambs were fed concentrate using a concentrate automatic feeder (CAF period) and a second period when lambs were fed total mixed ration (TMR) using a forage automatic feeder (FAF period). The nutritional composition of the commercial concentrates used during both feeding periods is listed in Table 1. The TMR was composed of 67% orchard hay supplemented with FAF concentrate. During the first 2 weeks of each recording period, the data was not used while the lambs were adapted to the automatic feeders. At 12 weeks of age, the lambs began the 8-week CAF period, followed by 2 weeks of dietary transition to the hay diet, and then the 8-week FAF period. Due to limited availability of FAF equipment, only 62 lambs were assessed in the FAF period, which were separated into two groups: 32 lambs were recorded from 22 to 30 weeks of age during the summer and 30 lambs were recorded from 30 to 38 weeks of age during the autumn.
Table 1. Nutritional composition (/kg) of commercial concentrates used during the CAF period (100% concentrates diet) and the FAF period (66% orchard hay diet).
The lambs were weighed four times to obtain a 2-day average BW at the beginning and end of the 8 weeks of recording (Figure 1) for both the CAF and FAF periods. The ADG for each period was calculated from the end (eBW) and beginning BWs. Daily feed intake values for the CAF and FAF periods were averaged over each 8-week testing period to estimate the average daily feed intake (ADFI) with each diet. Muscle depth (MB) and backfat thickness (BFT) were appreciated at the end of the CAF period only, using back ultrasound measurements. The RFI was calculated as the residual value of the multiple linear regression of ADFI, ADG, metabolic BW (eBW0.75), BFT, and MD quantified at the end of the CAF period (Tortereau et al., 2020). Efficient lambs had negative RFI values.
On the last day of the CAF and FAF periods, the ruminal content was sampled for each lamb using a vacuum pump and medical gastric tube. Immobilization of the lamb was performed using a special cage adapted to lambs, and sampling was performed by competent staff. Lambs were trained to accept immobilization during animal husbandry practices. The protocol was approved by the appropriate ethical committee (APAFIS#6076-20 16070809188901).
The feed intake and feeding duration of each individual lamb were recorded for every visit during the CAF and FAF periods. For each period, the feeding behavior traits were calculated using the visit data from the last 10 days, to align as closely as possible with the rumen fluid sampling. Feeding behavior traits were defined per visit and per day. At the visit level, we analyzed the feed intake (FI/V), feeding duration (FD/V), and FR, defined as the ratio between FI/V and FD/V. At the day level, the feed intake (FI/D) and feeding duration (FD/D) were summed over 24 h, and the number of visits per day was deduced (NV/D) (Marie-Etancelin et al., 2019).
Total DNA from 85 μL ruminal fluid sample was extracted and purified using the QIAamp DNA Stool Mini Kit (Qiagen Ltd., West Sussex, United Kingdom) according to the manufacturer’s instructions with a bead-beating step using a FastPrep Instrument (MP Biomedicals, Illkirch-Graffenstaden, France). The V3–V4 region of the 16S rRNA gene was amplified from the purified genomic DNA using forward F343 (5′–CTTTCCCTACACGACGCTCTTCCGATCTACGGRAGGCAG CAG–3′) and reverse R784 (5-GGAGTTCAGACGTGTGCT CTTCCGATCTTACCAGGGTATCTAATCCT–3) primers, and the PCR was performed as described by Zened et al. (2013). Using Illumina MiSeq technology (Illumina, San Diego, CA, United States), we obtained overlapping paired-end 250-bp reads to generate full-length reads of the entire V3 and V4 regions in a single run. Single multiplexing was performed using a 6 bp index, which was added to R784 during a second PCR with 12 cycles, using forward (AATGATACGGCGACCACCGAGATCTACACTCTTTCCCTA CACGAC) and reverse (CAAGCAGAAGACGGCATACGAGAT GTGACTGGAGTTCAGACGTGT) primers. The resulting PCR products were purified, loaded onto an Illumina MiSeq cartridge (Illumina), and analyzed at the Genomic and Transcriptomic Platform (Get-PlaGe, INRAE, Toulouse, France). The raw reads were treated according to the FROGS pipeline (Escudié et al., 2018) as follows: (i) demultiplexing reads, i.e., each pair of reads was assigned to its sample with the help of the previously integrated index; (ii) preprocessing reads, i.e., merging paired-end reads using Vsearch, and removing sequences that did not match the proximal PCR primer sequences, retaining sequences with >397 nucleotides and <432 nucleotides, and removing sequences with at least one ambiguous base; (iii) clustering with the iterative Swarm algorithm executed twice and a local threshold: first with the merged reads and a maximum number of differences between sequences in each step d = 1, and then, with the previous cluster seeds and d = 3; (iv) removing chimeric sequences; (v) filtering operational taxonomic units (OTUs), i.e., only clusters with an abundance > 0.005% of total sequences and present in ≥6 samples were retained; and (vi) aligning sequences using the SILVA database (v. 138) (Quast et al., 2013) via BLAST. OTU affiliations were finally obtained at 3 taxonomic levels: the phylum, family, and genus levels.
Bacterial alpha-diversity was estimated by the total number of observed OTUs (richness) and Shannon and Inverse-Simpson indices using the Phyloseq package in R software (version 3.4.1) (McMurdie and Holmes, 2013). As applied by Martinez Boggio et al. (2021) in dairy ewes, the relative abundance of taxa at the phylum, family, and genus levels was transformed by centered log ratio (CLR) using the Compositions package in R, after imputation of the zero values using the geometric Bayesian method (GBM). The GBM imputation was performed separately for the CAF and FAF periods in order to consider great differences in OTU distribution between the two periods.
The GLM procedure of the sasLM package in R software (version 3.4.1) was performed to determine the significant fixed effects affecting each transformed taxon abundance, alpha-diversity index, feeding behavior, and feed efficiency parameter. We retained the diet/feeding period effect (two levels: CAF vs. FAF) and the pen within feeding period effects (four levels within each feeding period) (model 1). We assumed that the feeding period effect was confounded by the diet effect. The effects of lamb “litter size,” “age” of the lamb at the beginning of the test, and “sampling order” for rumen fluid were also tested. Due to the large number of statistical tests for taxon abundance, raw P-values were corrected using the Benjamini–Hochberg (BH) false-discovery rate correction (Benjamini and Hochberg, 1995) leading to Q-values. Pearson correlations were estimated using residual values from the GLM analysis (model 1) between microbiota traits (relative abundance at the three taxonomic levels and alpha-diversity) and RFI during the CAF period only, or feeding behavior (FR) traits during both periods.
We selected lambs based on their extreme estimated breeding values (eBVs) for RFI or FR. Since the 78 lambs in the current study belonged to a cohort of 951 lambs reported in the genetic study by Tortereau et al. (2020), we determined eBVs for RFI based on this study. Marie-Etancelin et al. (2019) reported the feeding behavior traits of the same cohort of 951 lambs, including FR. Therefore, we calculated eBVs for FR for these 951 lambs using Pest software (Groeneveld, 1990), with a pedigree of 5,216 individuals. We then focused on the 62 lambs measured in both the CAF and FAF periods. The GLM procedure of the sasLM package in R was performed separately for the CAF and FAF periods to transformed taxon abundance and alpha-diversity index, considering the pen effect (4 levels) for the CAF period, and the additional seasonal effect (summer or autumn) for the FAF period (model 2). Twenty divergent lambs with extreme RFI eBVs (10 high and 10 low RFI eBVs) were compared. Additionally, twenty divergent lambs with extreme eBVs for FR (10 high and 10 low FR eBVs) were compared. The effects of the RFI/FR index groups were evaluated on the residual values of taxon abundance, alpha-diversity index, feeding behavior, and feed efficiency parameters using the GLM procedure of the sasLM package in R software. In order to select a small number of relevant OTUs to discriminate the RFI or FR index groups, sparse partial least square discriminant analysis (sPLS-DA) was performed using the mixOmics package in R software (Rohart et al., 2017) on genera residual values (model 2) separately for the CAF and FAF periods. The leave-one-out cross-validation strategy was implemented to assess the optimal numbers of components and variables per component according to the mixOmics tuning function, and to estimate the final model performance using the perf function, according to the balanced error rate (BER).
Descriptive statistics on the performance and feeding behavior traits of the lambs are listed in Table 2. On average, the lambs ingested 1,971 ± 272 g concentrate and 1,656 ± 339 g TMR during the CAF and FAF periods, respectively. Additionally, the lambs grew an average of 402 ± 64 g/d and 113 ± 140 g/d to reach an average BW of 52.6 ± 6.5 kg and 58.6 ± 13.6 kg at the end of the CAP and FAF periods, respectively. These values differed significantly (P < 0.001) between feeding periods, but reflected mixed effects of diet, season, and animal age. At the end of the CAF period, the body composition of the lambs was evaluated by dorsal ultrasound to reveal an average BFT of 7.3 ± 0.9 mm and MD of 2.73 ± 0.18 cm.
With regard to feeding behavior (Table 2), the NV/D (estimated over the last 10 days of each period) did not differ significantly between feeding periods, with average NV/D values of 14.4 ± 5.1 and 15.2 ± 9.8 for the CAF and FAF periods, respectively. The average FI/V value seemed to be higher in the CAF period (161 ± 47 g) than in the FAF period (148 ± 73 g), although this difference was not significant. Moreover, the feeding duration was over 3 times longer during the FAF period than during the CAF period, both for FD/V (16.0 ± 8.3 min vs. 4.1 ± 1.5 min) and FD/D (181.0 ± 37.2 min vs. 54.9 ± 14.2 min). This difference in duration led to significantly (P < 0.001) dissimilar FRs between the two feeding periods, with average FR values of 41.1 ± 12.2 g/min and 9.6 ± 2.1 g/min for the CAF and FAF periods, respectively. Regardless of the trait, the effects of “litter size,” “age” of the lamb at the beginning of the test, and “sampling order” were not significant.
For the 140 samples, 2,808,537 sequences were produced by the Illumina MiSeq technology. After the pre-process step, 2,520,572 sequences were retained and grouped into 336,894 clusters, using Swarm algorithm. The deletion of chimeric sequences and the filtering of clusters with an abundance lower than 0.005% of the total sequences, leads to the deletion of 216,357 and 362,771 sequences corresponding to 122,543 and 212,852 clusters, respectively. Thus, without a rarefying step, we obtained 1,499 OTUs corresponding to 1,941,444 sequences for 140 samples (78 CAF period samples and 62 FAF period samples) that is to say 13,800 sequences per sample on average. 99.5, 99.0, and 98.0% of the 1,499 OTUs were successfully affiliated at the phylum, family, and genus levels, respectively; the 100% complement corresponded to multi-affiliations. Because the affiliation rate dropped <8% at the species level, we decided not to explore this taxonomic rank. Finally, the microbial community was structured into 114 different bacterial genera, which were grouped into 54 families and 10 phyla.
The relative abundance of phyla was greatly affected by diet (Figure 2). Firmicutes was significantly more abundant in the FAF period compared to the CAF period, whereas Bacteroidetes was significantly less abundant in the FAF period (Figure 2A; Q < 0.001). The relative abundance of Actinobacteria and Proteobacteria was significantly decreased when lambs were switched from the concentrate-based to the forage-based diet (CAF to FAF period) (Figure 2B; Q < 0.001). Among other minor phyla (relative abundance ranging from 0.1 to 0.7% of total sequences), Desulfobacterota and Fibrobacterota were slightly affected by diet (Q < 0.05), with increased abundance in the FAF period compared to the CAF period, while Spirochaetes was not affected. The presence of Fusobacteria was specific to the FAF period (i.e., undetected during the CAF period).
Figure 2. Means of major (A) and minor (B) phylum (relative abundance > 1%) significantly (Q < 0.001) affected by the diet/period test: 78 lambs received during a first period a 100% concentrates diet (CAF, ) and then in a second period 62 of them were kept to a 67% hay diet (FAF,
).
Based on the BH-corrected P-values, 41/54 bacterial families and 91/114 bacterial genera were affected by diet (Q < 0.05). The switch from the concentrate-based to the forage-based diet resulted in a sharp reduction in the abundance of Lachnospiraceae (25% to 14%) and Prevotellaceae (53–33%), and a strong increase in Christensenellaceae (1–15%) and Rikenellaceae (1–6%) abundance families (Figure 3).
Figure 3. Means of ruminal dominant families (relative abundance > 1%) significantly (Q < 0.001) affected by the diet: 78 lambs received during a first period a 100% concentrates diet (CAF, ) and then in a second period 62 of them were kept to a 67% hay diet (FAF,
).
The results indicated that microbiota diversity was strongly affected by diet (Figure 4). Feeding lambs with forage significantly (P < 0.001) increased the diversity of the ruminal microbiota considering the evenness of species distribution. Indeed, the Shannon and Inverse Simpson indexes increased from 3.33 to 5.11 and from 14.0 to 78.5 from the CAF to FAF periods, respectively, and the number of observed OTUs increased from 119 to 329.
Figure 4. Least-squares means of alpha-diversity traits of lambs’ rumen microbiota significantly different (P < 0.001) according to the diet: 78 lambs received during a first period a 100% concentrates diet (CAF, ) and then in a second period 62 of them were kept to a 67% hay diet (FAF,
).
Phenotypic correlations with RFI could only be estimated during the CAF period, since RFI was not calculated during the FAF period. RFI was not significantly correlated with microbiota alpha-diversity traits nor abundance, regardless of taxonomic level.
The average difference in eBVs between the two RFI index groups was 146.5 g/d, which is approximately 2 genetic standard deviations (Table 3) since the genetic standard deviation of RFI was estimated at 73 g/d (Tortereau, 2020; personal communication).
The performance traits of the 20 lambs with extreme RFI eBVs during the CAF period are presented in Table 4. As expected, the two RFI index groups differed significantly (P < 0.001) for RFI (difference of 266.6 g/d), ADFI (difference of 309 g/d), and FI/D (difference of 281 g/d). No other traits were differentiated with phenotypic RFI, neither feed efficiency nor feeding behavior traits. Additionally, the RFI index groups did not differ in terms of their microbiota alpha-diversity traits nor their relative abundance during the CAF and FAF periods, regardless of taxonomic level.
Table 4. Performance and feeding behavior traits of lambs for extreme RFI index and FR index during 100% concentrates CAF period.
The sPLS-DA on the GLM residual values of taxa abundance at the genus level enabled discrimination of the RFI index groups (Figure 5A) by selecting three components with 8, 50, and 90 variables per component in the CAF period. The BER of this model was 0.20. On the first axis, the selected genera (Table 5) all belonged to the phylum Firmicutes, with the most efficient lambs (RFI-BV−) having increased abundance of two genera (Coprococcus and Lachnospiraceae Probable genus 1) and decreased abundance in three genera (Colidextribacter, Lachnospiraceae ND3007, and Selenomonas) compared to less efficient lambs. Different phyla were represented on the second axis, with increased abundance of the genera Atopobium, Moryella, [Eubacterium] brachy group, and [Eubacterium] hallii group in efficient lambs, whereas inefficient lambs had increased abundance in the genera Oscillospiraceae UCG-007, Ruminococcaceae UCG-001, Streptococcus, Candidatus soleaferrea, and [Eubacterium] xylanophilum group. For the same lambs during the FAF period, the sPLS-DA on the GLM residual values of taxa abundance at the genus level enabled discrimination of the RFI index groups (Figure 5B) by selecting three components with 75, 1, and 1 variable per component. The BER was 0.10, indicating good prediction ability. On the first axis, the selected taxa were numerous (n = 75). Among those with the highest loading values (Table 5), some genera were associated with more efficient lambs (Sediminispirochaeta, [Eubacterium] brachy group, and Moryella), while some were associated with inefficient lambs (Undibacterium, Lachnospiraceae FD2005, and Prevotellaceae UCG-003). On the second axis, only the genus Oribacterium was selected and associated with efficient lambs. The 3rd axis of the analysis discriminated very little between the RFI index groups during either the CAF or FAF periods.
Figure 5. Sparse partial least squares discriminant analysis of ruminal bacteria abundances at the genus level according to Residual Feed Intake index groups (N = 20) during 100% concentrate CAF (A- sPLS-DA with 3 components, 8, 50, and 90 variables per component and a BER of 0.20) and 67% forage FAF (B- sPLS-DA with 3 components, 75, 1, and 1 variables per component and a BER of 0.10) periods.
Table 5. Sparse partial least squares discriminant analysis of ruminal bacteria abundances at the genus level according to residual feed intake index groups (N = 20) during 100% concentrate CAF (a) and 67% forage FAF (b) periods.
Finally, comparing genera selected in each feeding periods to discriminate the RFI index groups, 19 and 34 genera were specific to the CAF and FAF periods, respectively, while 26 genera were common to both feeding periods (Table 5). Among them, the abundance of 15/26 genera varied in the same manner in the CAF and FAF periods: Coprococcus, Moryella, [Eubacterium] brachy group, [Eubacterium] hallii group, Acetitomaculum, Oscillospiraceae NK4A214, Fibrobacter, Mogibacterium, Family XIII AD3011, Saccharofermentass, Blautia, Ruminococcus, and Prevotellaceae UCG-004 displayed higher abundance in the RFI-BV− group, while Lachnospiraceae FD2005 and Shuttleworthia displayed higher abundance in the RFI-BV+ group. The relative abundance of the remaining 11/26 genera varied in opposite directions in RFI index groups in the CAF and FAF periods (Oscillospiraceae UCG-007, Ruminococcaceae UCG-001, Sediminispirochaeta, [Eubacterium] xylanophilum group, Prevotellaceae Ga6A1, Prevotellaceae UCG-001, Lachnoclostridium, Lachnospiraceae XPB1014, Alloprevotella, [Bacteroides] pectinophilus group, and Defluviitaleaceae UCG-001).
Feeding rate was not significantly correlated with microbiota alpha-diversity traits nor abundance at the phylum, family, or genus levels during the CAF and FAF periods.
The average difference in eBVs between the two FR index groups was 10.90 g/min (Table 3). As this trait was not evaluated in the whole Romane lamb population, it was not possible to transpose this difference into an equivalent genetic standard deviation.
The performance and feeding behavior traits of lambs with extreme FR eBVs are presented in Table 4. As expected, the average FR values differed significantly between the two FR index groups with a ratio of 1.6 (33.7 g/min vs. 55.1 g/min; P < 0.001). Lambs in the FR index groups also significantly diverged for FD/V (3.1 min vs. 4.7 min; P < 0.01) and FD/D (41.1 min vs. 63.2 min; P < 0.001), the highest FR the shortest feeding duration. NV/D values did not differ significantly between the FR index groups. The FR index groups had also similar feed efficiency traits, including RFI. Comparing the ruminal microbiota during the CAF and FAF periods, the FR index groups did not differ in terms of their microbiota alpha-diversity traits nor their relative abundance, regardless of taxonomic level.
The two sPLS-DA on the GLM residual values of taxa abundance at the genus level enabled very little discrimination of the FR index groups for the CAF and FAF periods (Figures 6A,B), since the BERs were 0.40 and 0.45, respectively. During the CAF period, four and six taxa on the first and second axes were selected, respectively (Table 6). Among them, the relative abundance of genera Clostridium sensu stricto 1, Lachnospiraceae NK3A20, and Colidextribacter increased in the FR-BV+ group, whereas the relative abundance of genera [Eubacterium] eligens group, Lachnospiraceae AC2044, and Family XIII AD3011 increased in the FR-BV− group. Only the first axis was used to discriminate the FR index groups during the FAF period, and three genera were retained. The relative abundance of Prevotellaceae UCG-001 increased in the FR-BV− group, whereas that of Lachnospiraceae AC2044 and Prevotellaceae UCG-001 increased in the FR-BV+ group. Among the 11 selected genera, only eight were discriminant in the CAF period, one was specific to the FAF period, and two genera were discriminant in both periods (Lachnospiraceae AC2044 and Prevotellaceae UCG-001), but their abundance varied in opposite manner between FR index groups (Table 6).
Figure 6. Sparse partial least squares discriminant analysis of ruminal bacteria abundances at the genus level according to feeding rate index groups (N = 20) during 100% concentrate CAF (A- sPLS-DA with 2 components, 4 and 6 variables per component and a BER of 0.40) and 67% forage FAF (B- sPLS-DA with 1 component and 3 variables, and a BER of 0.45) periods.
Table 6. Sparse partial least squares discriminant analysis of ruminal bacteria abundances at the genus level according to Feeding Rate index groups (N = 20) during 100% concentrate CAF (a) and 67% forage FAF (b) periods.
In this study, feeding behavior was affected by diet. During the CAF period, lambs ate more and faster than during the FAF period. Such behavior in lambs has been previously reported. For example, Sari et al. (2018) reported increased FI/V and FR with increasing concentrate level in the diet, which may have been due to improved palatability and higher rates of ruminal degradation and passage of concentrates compared to forage. Moreover, the ruminal microbiota is responsible for ruminal degradation of food, which might play a role in the observed differences in eating behavior.
Our results confirmed the major impact of the type of food ingested by the lambs on the composition of their microbiota (Ellison et al., 2017). The three alpha-diversity traits were greatly increased when lambs ingested forage rather than concentrate. Depending on the trait, the alpha-diversity was multiplied a factor of 1.5 to 5. The lower bacterial diversity in the rumen of cattle fed high levels of concentrate compared to high levels of forage has been well established (Mao et al., 2013; Zened et al., 2013; McGovern et al., 2020). Ellison et al. (2014) previously reported substantial changes to sheep microbiota consuming concentrate-based or forage-based diets, with a more diverse microbial ecosystem observed for the forage-based diet. These authors proposed two likely arguments: the ruminant microbiota is more adapted to forage than concentrate digestion, and forage-based diets are more diversified in nutrients than concentrate-based diets.
Diet affected the relative abundance of almost all bacteria, regardless of taxonomic level. Indeed, 9/10 phyla, 41/54 families, and 91/114 genera were significantly impacted. The ruminal ecosystem under the forage-based diet was characterized by increased relative abundance of Firmicutes and Fibrobacteres, whereas that of Bacteroidetes and Actinobacteria decreased compared to the concentrate-based diet. At the family level, the concentrate-based diet led to higher relative abundance of Prevotellaceae and Lachnospiraceae and lower relative abundance of Ruminococcaceae, Christensenellaceae, and Rikenellaceae than the forage-based diet. Some studies reported that Bacteroidetes (Fernando et al., 2010; Zhang et al., 2019) and Prevotella (Henderson et al., 2015) were more abundant in animals fed diets containing concentrate. However, studies have also reported opposite results under high-concentrate diets (Mao et al., 2013; Zened et al., 2013). The literature is also inconsistent concerning Firmicutes, Lachnospiraceae, and Ruminococcaceae. The relative abundance of these bacteria increased with concentrate incorporation in some studies, but other studies reported the opposite effect (Fernando et al., 2010; Mao et al., 2013; Zened et al., 2013; Zhang et al., 2019). These differences could be explained by other features of the diet and sampling conditions, such as the nature of the forage and the sampling site (Deusch et al., 2017). For other groups of bacteria, our results were in accordance with previous studies in which increasing concentrate proportions reportedly led to decreased abundance of Rikenellaceae (Zened et al., 2013; Zhang et al., 2019), Christensenellaceae (Zhang et al., 2019) and Fibrobacteres (Fernando et al., 2010; Zhang et al., 2019), and increased abundance of Actinobacteria (Mao et al., 2013; Zened et al., 2013).
Beyond the major effect of diet on the ruminal microbiota, we tested the impact of selection for RFI or FR. Even if differences between groups of lambs divergent for RFI or FR were significant, the impact on the diversity of the bacterial community appeared to be null. Thus, we did not highlight any link between RFI or FR index groups and microbiota alpha-diversity traits (observed OTUs, or Shannon and Inverse-Simpson indexes). Moreover, no significant phenotypic correlations were obtained between alpha-diversity traits and RFI or FR phenotypes in the whole dataset. Regarding feed efficiency, our results were consistent with those of previous studies (Li and Guan, 2017; McGovern et al., 2018, 2020; Patil et al., 2018) that reported no differences in alpha-diversity traits between low- and high-RFI steers or lambs. However, Shabat et al. (2016) reported significantly lower microbiome richness and a lower Shannon index in efficient dairy cows compared to less efficient cows, regardless of taxonomic level. Li et al. (2016) concluded that bacterial community structures differed between high- and low-RFI steers after applying a weighted UniFrac test to measure beta-diversity. Interestingly, Li and Guan (2017) used the Bray–Curtis dissimilarity test to measure beta-diversity in the same dataset, concluding there was no clear separation between groups of high- and low-RFI steers. McGovern et al. (2020) reported no difference between two groups of steers phenotypically divergent for RFI, regardless of the beta-diversity test used.
Comparing the relative abundance of taxa clearly demonstrated that diet influenced the lamb microbiome more than feed efficiency (RFI) or FR status. In our dataset, diet impacted 91/114 bacterial genera (Q < 0.05), whereas RFI or FR traits did not display significant links with bacterial genera. To a lesser extent, Ellison et al. (2017) previously quantified this disequilibrium between diet type and feed efficiency in 16 sheep, reporting that the abundance of 44/349 OTUs differed with respect to diet while only 11/349 differed with respect to RFI status. In bovines, McGovern et al. (2020) found no difference in microbial taxa between RFI phenotypes, and only ten OTUs were significantly associated with RFI, independent of other factors of variation such as diet, breed, and age.
In the present study, some bacterial genera were impacted by genetic RFI during both feeding periods, with a high ability of prediction (error rates of 0.20 and 0.10 during the CAF and FAF periods, respectively). Our results indicated decreased abundance of Lachnospiraceae FD2005 and Shuttleworthia and increased abundance of Coprococcus, Moryella, Eubacterium Brachy, Eubacterium hallii, Acetitomaculum, Oscillospiraceae NK4A214, Fibrobacter, Mogibacterium, and Family XIII AD3011 in efficient lambs compared to the inefficient lambs, regardless of diet. Notably, among the 26 genera that discriminated the RFI index groups in both the CAF and DAF periods, 11 belonged to the family Lachnospiraceae (Coprococcus, Moryella, Eubacterium Hallii, Acetitomaculum, Eubacterium xylanophilum, Lachnospiraceae FD2005, Lachnoclostridium, Shuttleworthia, Lachnospiraceae XPB1014, Blautia, and Bacteroides pectinophilus) and four belonged to the family Prevotellaceae (Prevotellaceae Ga6A1, Prevotellaceae UCG-003, Prevotellaceae UCG-004, and Alloprevotella), demonstrating opposite correlations with RFI within the family. Differing correlations between RFI and bacterial genera within the same family might explain the lack of significant link between RFI and these bacterial families, particularly Lachnospiraceae. Bovine literature often reports a positive relationship between Lachnospiraceae abundance and RFI, with inefficient animals having higher abundance (Rius et al., 2012; Jewell et al., 2015; Li et al., 2016; Shabat et al., 2016; Li and Guan, 2017; Perea et al., 2017; McGovern et al., 2018). McGovern et al. (2018) reported a correlation of +0.44 between RFI and Lachnospiraceae abundance in the solid ruminal phase only. At the genus level, some of these studies (Jewell et al., 2015; Shabat et al., 2016; McGovern et al., 2018) have also reported that some OTUs belonging to the family Lachnospiraceae are positively or negatively correlated with RFI, which concurred with our results. These inconsistent effects could also depend on diet, as suggested by the opposite links observed in the CAF and FAF periods for the [Eubacterium] xylanophilum group, Lachnoclostridium, Lachnospiraceae XPB1014, and [Bacteroides] pectinophilus group (Table 5). The relationship between the ruminal microbiome and some functional aspects, such as feed efficiency, needs to be further investigated at the genus or OTU level, as was conducted in the most recent studies (Auffret et al., 2020; McGovern et al., 2020; McLoughlin et al., 2020).
At the genus level, we observed increased Coprococcus abundance under both diets in efficient lambs. These results were consistent with the literature (Jewell et al., 2015; Shabat et al., 2016; McGovern et al., 2018), in which a negative relationship was reported between RFI and Coprococcus abundance in the liquid rumen content. Moreover, Shabat et al. (2016) also reported an enrichment of Coprococcus in their efficient cow rumen. Additionally, our study findings indicated a higher abundance of the genus Moryella associated with efficient lambs, as in the study by Hernandez-Sanabria et al. (2012), which was positively linked with RFI in the study by Jami et al. (2014). Further, Acetitomaculum abundance was higher in efficient lambs in the current study. Considering the feed conversion ratio as a measurement of feed efficiency, McLoughlin et al. (2020) reported the same trend. This genus belongs to acetogenic bacteria (Le Van et al., 1998), which can use H2 to reduce CO2 to acetate and thus limit methane emission, leading to decreased energy loss. Blautia was another acetogenic bacterium with increased abundance in efficient lambs in our study, which was also reported by Nakamura et al. (2010). Moreover, we observed a negative link between Fibrobacter and RFI, as reported between the phylum Fibrobacteres (McGovern et al., 2018), the family Fibrobacteraceae (Rius et al., 2012), or Fibrobacter sp. (Perea et al., 2017) and RFI, in that efficient lambs had greater abundance of Fibrobacteres/Fibrobacteraceae. Although McGovern et al. (2018) reported strong correlations (between −0.43 and −0.59) in solid and liquid rumen phases between RFI and Fibrobacteres succinogenes, this link was not corroborated in other studies (Jewell et al., 2015; Shabat et al., 2016). Moreover, McGovern et al. (2020) found that Mogibacterium was negatively associated with phenotypic RFI, which concurred with the results of our study. These bacteria are saccharolytic and thought to be mainly involved in ammonia assimilation (Nakazawa et al., 2000). Rius et al. (2012) reported that efficient cattle (low RFI) had higher nitrogen digestion than inefficient cattle, suggesting a possible link between feed efficiency and ruminal nitrogen metabolism.
McGovern et al. (2018) reported that Ruminococcaceae and Ruminococcus abundance increased in animals with high feed efficiency, in particular Ruminococcus flavefaciens, while their abundance was reportedly increased in low-efficiency animals in the study by Jewell et al. (2015). In a study by Shabat et al. (2016), Ruminococcus albus and R. flavefaciens were associated with H2 (used by Archaea for methane production) and CO2 production, and so, in spite of one strain of R. flavefaciens, their abundance was increased in low-efficiency animals. At the genus level, we highlighted the opposite effects between Ruminococcaceae UCG001 and Ruminococcus abundance and RFI when lambs consumed the concentrate-based diet, but efficient lambs had lower percentages of these two genera in their rumen when they consumed the forage-based diet.
During the FAF period only, the abundance of Oribacterium increased in efficient sheep compared to inefficient sheep, while McGovern et al. (2020) observed a positive link between this genus and RFI regardless of diet. Oribacterium is a genus strongly involved in cell wall degradation (Kang et al., 2019), contributing to one of the ruminal paradoxes. The efficiency of plant cell wall digestion driven by fibrolytic bacteria conditions access to nutrients for all bacteria and increases overall digestion efficiency in the rumen, while increasing parallel energy loss through methane emissions. Such complex phenomena could explain, at least in part, why no clear link could be established between fibrolytic bacteria and feed efficiency. Shuttleworthia was one of two taxa with increased abundance in inefficient lambs regardless of diet, but this result opposes that reported by Jewell et al. (2015). Given the lack of information on this genus, no hypotheses can be drawn.
Shabat et al. (2016) and Auffret et al. (2020) proposed an explanation for the relationship between feed efficiency and particular ruminal microbiota composition: butyrate and lactate producers (and in general bacteria using the acrylate pathway, i.e., Coprococcus) would offer more relevant output metabolites for animals than succinate and acetate/H2 producers (e.g., Ruminoccocus). Although these authors seemed to agree on the higher efficiency of acrylate metabolism compared with succinate metabolism, the butyrate hypothesis remains debated because of the difficulties correlating Lachnospiraceae (butyrate producer) and RFI. Moreover, in growing animals like lambs, the propionate pathway is known to be more efficient for growth than acetate and butyrate. Lactate-producing bacteria, especially prominent during the CAF period, were more abundant in both efficient (e.g., Coprococcus and Atopobium) and inefficient lambs (e.g., Colidextribacter and Streptococcus). Here again, it is difficult to establish a clear link, probably because lactate, although a metabolically efficient nutrient, may also enhance acidogenic rumen conditions, inhibit fibrolytic bacteria, and thus reduce ruminal digestion efficiency. However, the association between Coprococcus and low RFI is most frequently reported in the literature (Jewell et al., 2015; Shabat et al., 2016; McGovern et al., 2018), although sometimes not observed (Jami et al., 2014), and there is currently no report of increased Coprococcus abundance in inefficient animals. Another explanation is the ability of Coprococcus to ferment aromatic compounds into acetate, benzoate, and organic acids (Patel et al., 1981). Moreover, a syntrophic action exists between Coprococcus and Papillibacter, in which Coprococcus ferments benzoate produced by Papillibacter (Defnoun et al., 2003). In our study, Papillibacter was associated with efficient lambs, but only during the FAF period. In humans, such compounds have been shown to reduce obesity in association with changes in gut microbiota and energy metabolism (Van Hul et al., 2018). Since the overall objective is to increase weight gain in growing animals, fermentative bacteria that can utilize aromatic compounds may play a potential role in feed efficiency. Sung et al. (2017) suggested that microbiota sensitive to such compounds (such as Moryella) may, at least in part, act on largely undigested aromatic compounds (i.e., polyphenol) in humans. Aromatic compounds, particularly plant polyphenols, are known to be bioactive and alter ruminal digestion (Vasta et al., 2019). Therefore, the effect of aromatic compounds on rumen bacteria needs to be investigated with respect to feed efficiency.
The impact of genetic FR on microbiota taxa abundance was even smaller than that observed with genetic RFI, and to date has not been reported in the literature. The only relevant study (Schären et al., 2018) did not find a clear association between feeding behavior (volume, duration, and frequency) and rumen OTUs in dairy cows. The relative abundance of rumen bacteria in the current study did not enable discrimination of the FR index groups (error rates of 0.40 and 0.45 during the CAF and FAF periods, respectively). Moreover, none of the bacteria selected by sPLS-DA in the feeding periods were correlated in the same manner in the FR index groups. Therefore, it is difficult to conclude a link between the ruminal microbiota and selection for FR, and further investigation is warranted to advance possible explanations.
Although we did not notice an impact on microbial diversity, feed efficiency was linked with some ruminal microbial genera, particularly bacteria involved in the degradation of aromatic compounds (such as Coprococcus), nitrogen metabolism (such as Mogibacterium), and acetogenesis (such as Actetitomaculum). The link between feed efficiency and plant cell wall digestion efficiency requires further study due to its complex relationship. Surprisingly, genetic FR was only slightly related with a specialized microbiota, which was much less than with genetic RFI. Nevertheless, as expected, diet had a major impact on the ruminal microbial community, and some associations between the microbiota and feed efficiency or feeding behavior could be dependent on diet. Our work continues with divergent RFI selection and a larger number of lambs to explore links between the presence of bacteria in the microbiota and their metabolite production, assaying ruminal and blood metabolites in association with ruminal microbiota analysis.
The datasets presented in this study can be found in online repositories. The names of the repository/repositories and accession number(s) can be found below: https://www.ncbi.nlm.nih.gov/, bioproject/755177.
The animal study was reviewed and approved by the appropriate ethical committee (APAFIS#6076-20 16070809188901). Written informed consent was obtained from the owners for the participation of their animals in this study.
AM, CM-E, and FT designed and monitored the experiments. J-LW and DM implemented the experiments in the P3R unit. BG and M-LD performed the laboratory analysis of the 16S sequencing. GP performed the bioinformatics analysis of the sequences. CM-E performed the statistical analysis of the data with the help of GMB and QL. CM-E drafted the manuscript with contributions from all co-authors. All authors contributed to the article and approved the submitted version.
This experiment was financed by internal GenPhySE funds.
The authors declare that the research was conducted in the absence of any commercial or financial relationships that could be construed as a potential conflict of interest.
All claims expressed in this article are solely those of the authors and do not necessarily represent those of their affiliated organizations, or those of the publisher, the editors and the reviewers. Any product that may be evaluated in this article, or claim that may be made by its manufacturer, is not guaranteed or endorsed by the publisher.
We would like to thank the technicians of the INRAE UE P3R of Bourges (doi: 10.15454/1.5483259352597417E12) for the care given to the lambs, follow-up of the experimental protocol, and zootechnical data collection, as well as ML Chemit for the measurement of feed values for the concentrate and forage. We are grateful to the GenoToul bioinformatics platform Toulouse Occitanie (Bioinfo Genotoul, doi: 10.15454/1.5572369328961167E12) for providing computing and storage resources.
Auffret, M. D., Stewart, R. D., Dewhurst, R. J., Duthie, C. A., Watson, M., and Roehe, R. (2020). Identification of microbial genetic capacities and potential mechanisms within the rumen microbiome explaining differences in beef cattle feed efficiency. Front. Microbiol. 11:1229. doi: 10.3389/fmicb.2020.01229
Benjamini, Y., and Hochberg, Y. (1995). Controlling the false discovery rate: a practical and powerful approach to multiple testing. J. R. Stat. Soc. Series B (Methodological) 57, 289–300.
Breton, J., Tennoune, N., Lucas, N., François, M., Legrand, R., Jacquemot, J., et al. (2016). Gut commensal E. coli proteins activate host satiety pathways following nutrient-induced bacterial growth. Cell Metabol. 23, 324–334. doi: 10.1016/j.cmet.2015.10.017
Carberry, C. A., Kenny, D. A., Han, S., McCabe, M. S., and Waters, S. M. (2012). Effect of phenotypic residual feed intake and dietary forage content on the rumen microbial community of beef cattle. Appl. Environ. Microbiol. 78, 4949–4958. doi: 10.1128/AEM.07759-11
Defnoun, S., Ambrosio, M., Garcia, J.-L., Traoré, A., and Labat, M. (2003). Degradation of cinnamate via β-oxidation to benzoate by a defined, syntrophic consortium of anaerobic bacteria. Curr. Microbiol. 46, 47–52. doi: 10.1007/s00284-002-3759-9
Deusch, S., Camarinha-Silva, A., Conrad, J., Beifuss, U., Rodehutscord, M., and Seifert, J. (2017). A structural and functional elucidation of the rumen microbiome influenced by various diets and microenvironments. Front. Microbiol. 8:1605. doi: 10.3389/fmicb.2017.01605
Ellison, M. J., Conant, G. C., Cockrum, R. R., Austin, K. J., Truong, H., Becchi, M., et al. (2014). Diet alters both the structure and taxonomy of the ovine gut microbial ecosystem. DNA Res. 21, 115–125. doi: 10.1093/dnares/dst044
Ellison, M. J., Conant, G. C., Lamberson, W. R., Cockrum, R. R., Austin, K. J., Rule, D. C., et al. (2017). Diet and feed efficiency status affect rumen microbial profiles of sheep. Small Ruminant Res. 156, 12–19. doi: 10.1016/j.smallrumres.2017.08.009
Escudié, F., Auer, L., Bernard, M., Mariadassou, M., Cauquil, L., Vidal, K., et al. (2018). FROGS: find, rapidly, OTUs with galaxy solution. Bioinformatics 34, 1287–1294. doi: 10.1093/bioinformatics/btx791
Fernando, S. C., Purvis, H. T., Najar, F. Z., Sukharnikov, L. O., Krehbiel, C. R., Nagaraja, T. G., et al. (2010). Rumen Microbial population dynamics during adaptation to a high-grain diet. Appl. Environ. Microbiol. 76, 7482–7490. doi: 10.1128/AEM.00388-10
Flint, H. J., Bayer, E. A., Rincon, M. T., Lamed, R., and White, B. A. (2008). Polysaccharide utilization by gut bacteria: potential for new insights from genomic analysis. Nat. Rev. Microbiol. 6, 121–131. doi: 10.1038/nrmicro1817
Groeneveld, E. (1990). PEST Users’ Manual. Neustadt: Institute of Animal Husbandry and Animal Behaviour Federal Re-search Centre, 80.
Henderson, G., Cox, F., Ganesh, S., Jonker, A., and Young, W. (2015). Rumen microbial community composition varies with diet and host, but a core microbiome is found across a wide geographical range. Sci. Rep. 5:14567. doi: 10.1038/srep14567
Hernandez-Sanabria, E., Goonewardene, L. A., Wang, Z., Durunna, O. N., Moore, S. S., and Guan, L. L. (2012). Impact of feed efficiency and diet on adaptive variations in the bacterial community in the rumen fluid of cattle. Appl. Environ. Microbiol. 78, 1203–1214. doi: 10.1128/AEM.05114-11
Jami, E., White, B. A., and Mizrahi, I. (2014). Potential role of the bovine rumen microbiome in modulating milk composition and feed efficiency. PLoS One 9:e85423. doi: 10.1371/journal.pone.0085423
Jewell, K. A., McCormick, C. A., Odt, C. L., Weimer, P. J., and Suen, G. (2015). Ruminal bacterial community composition in dairy cows is dynamic over the course of two lactations and correlates with feed efficiency. Appl. Environ. Microbiol. 81, 4697–4710. doi: 10.1128/AEM.00720-15
Kang, S., Denman, S., and McSweeney, C. (2019). Draft genome sequence and annotation of oribacterium sp. Strain C9, isolated from a cattle rumen. Microbiol. Resour. Announc. 8, doi: 10.1128/MRA.01562-18
Le Van, T. D., Robinson, J. A., Ralph, J., Greening, R. C., Smolenski, W. J., Leedle, J. A., et al. (1998). Assessment of reductive acetogenesis with indigenous ruminal bacterium populations and Acetitomaculum ruminis. Appl. Environ. Microbiol. 64, 3429–3436. doi: 10.1128/AEM.64.9.3429-3436.1998
Li, F., and Guan, L. L. (2017). Metatranscriptomic profiling reveals linkages between the active rumen microbiome and feed efficiency in beef cattle. Appl. Environ. Microbiol. 83, e61–e17. doi: 10.1128/AEM.00061-17
Li, F., Zhou, M., Ominski, K., and Guan, L. L. (2016). Does the rumen microbiome play a role in feed efficiency of beef cattle? J. Anim. Sci. 94, 44–48. doi: 10.2527/jas.2016-0524
Løvendahl, P., Difford, G. F., Li, B., Chagunda, M. G. G., Huhtanen, P., Lidauer, M. H., et al. (2018). Review: selecting for improved feed efficiency and reduced methane emissions in dairy cattle. Animal 12, s336–s349. doi: 10.1017/S1751731118002276
Malmuthuge, N., and Guan, L. (2017). Understanding host-microbial interactions in rumen: searching the best opportunity for microbiota manipulation. J. Anim. Sci. Biotechnol. 8:8. doi: 10.1186/s40104-016-0135-3
Mao, S., Zhang, R., Wang, D., and Zhu, W. (2013). Impact of subacute ruminal acidosis (SARA) adaptation on rumen microbiota in dairy cattle using pyrosequencing. Anaerobe 24, 12–19. doi: 10.1016/j.anaerobe.2013.08.003
Marie-Etancelin, C., Francois, D., Weisbecker, J. L., Marcon, D., Moreno-Romieux, C., Bouvier, F., et al. (2019). Detailed genetic analysis of feeding behaviour in Romane lambs and links with residual feed intake. J. Anim. Breed Genet. 136, 174–182. doi: 10.1111/jbg.12392
Martinez Boggio, G., Meynadier, A., Daunis-i-Estadella, P., and Marie-Etancelin, C. (2021). Compositional analysis of ruminal bacteria from ewes selected for somatic cell score and milk persistency. PLoS One 16:e0254874. doi: 10.1371/journal.pone.0254874
McGovern, E., Kenny, D. A., McCabe, M. S., Fitzsimons, C., McGee, M., Kelly, A. K., et al. (2018). 16S rRNA sequencing reveals relationship between potent cellulolytic genera and feed efficiency in the rumen of bulls. Front. Microbiol. 9:1842. doi: 10.3389/fmicb.2018.01842
McGovern, E., McGee, M., Byrne, C. J., Kenny, D. A., Kelly, A. K., and Waters, S. M. (2020). Investigation into the effect of divergent feed efficiency phenotype on the bovine rumen microbiota across diet and breed. Sci. Rep. 10:15317. doi: 10.1038/s41598-020-71458-0
McLoughlin, S., Spillane, C., Claffey, N., Smith, P. E., and O’Rourke, T. (2020). Rumen microbiome composition is altered in sheep divergent in feed efficiency. Front. Microbiol. 11:1981. doi: 10.3389/fmicb.2020.01981
McMurdie, P. J., and Holmes, S. (2013). phyloseq: an R Package for reproducible interactive analysis and graphics of microbiome census data. PLoS One 8:e61217. doi: 10.1371/journal.pone.0061217
Nakamura, N., Lin, H. C., McSweeney, C. S., Mackie, R. I., and Gaskins, H. R. (2010). Mechanisms of microbial hydrogen disposal in the human colon and implications for health and disease. Annu. Rev. Food Sci. Technol. 1, 363–395. doi: 10.1146/annurev.food.102308.124101
Nakazawa, F., Sato, M., Poco, S. E., Hashimura, T., Ikeda, T., Kalfas, S., et al. (2000). Description of Mogibacterium pumilum gen. nov., sp. nov. and Mogibacterium vescum gen. nov., sp. nov., and reclassification of Eubacterium timidum (Holdeman et al. 1980) as Mogibacterium timidum gen. nov., comb. nov. Int. J. Syst. Evol. Microbiol. 50, 679–688. doi: 10.1099/00207713-50-2-679
Patel, T., Jure, K. G., and Jones, G. A. (1981). Catabolism of phloroglucinol by the rumen anaerobe coprococcus. Appl. Environ. Microbiol. 42, 1010–1017. doi: 10.1128/aem.42.6.1010-1017.1981
Patil, R. D., Ellison, M. J., Wolff, S. M., Shearer, C., and Wright, A. M. (2018). Poor feed efficiency in sheep is associated with several structural abnormalities in the community metabolic network of their ruminal microbes. J. Anim. Sci. 96, 2113–2124. doi: 10.1093/jas/sky096
Perea, K., Perz, K., Olivo, S. K., Williams, A., and Lachman, M. (2017). Feed efficiency phenotypes in lambs involve changes in ruminal, colonic, and small-intestine-located microbiota. J. Anim. Sci. 95, 2585–2592. doi: 10.2527/jas.2016.1222
Quast, C., Pruesse, E., Yilmaz, P., Gerken, J., Schweer, T., Yarza, P., et al. (2013). The SILVA ribosomal RNA gene database project: improved data processing and web-based tools. Nucleic Acids Res. 41, D590–D596. doi: 10.1093/nar/gks1219
Rius, A. G., Kittelmann, S., Macdonald, K. A., Waghorn, G. C., Janssen, P. H., and Sikkema, E. (2012). Nitrogen metabolism and rumen microbial enumeration in lactating cows with divergent residual feed intake fed high-digestibility pasture. J. Dairy Sci. 95, 5024–5034. doi: 10.3168/jds.2012-5392
Rohart, F., Gautier, B., Singh, A., and Lê Cao, K.-A. (2017). MixOmics: an R package for ‘omics feature selection and multiple data integration. PLoS Comput. Biol. 13:e1005752. doi: 10.1371/journal.pcbi.1005752
Sari, M., Monjezi, Y., and Anoosheh, S. F. (2018). Dietary concentrate level affects the feed sorting behaviour of lambs. J. Anim. Physiol. Anim. Nutr. 102, 892–900. doi: 10.1111/jpn.12914
Schären, M., Frahm, J., Kersten, S., Meyer, U., Hummel, J., Breves, G., et al. (2018). Interrelations between the rumen microbiota and production, behavioral, rumen fermentation, metabolic, and immunological attributes of dairy cows. J. Dairy Sci. 101, 4615–4637. doi: 10.3168/jds.2017-13736
Shabat, S. K., Sasson, G., Doron-Faigenboim, A., Durman, T., and Yaacoby, S. (2016). Specific microbiome-dependent mechanisms underlie the energy harvest efficiency of ruminants. ISME J. 10, 2958–2972. doi: 10.1038/ismej.2016.6
Sung, M. M., Kim, T. T., Denou, E., Soltys, C.-L. M., Hamza, S. M., Byrne, N. J., et al. (2017). Improved glucose homeostasis in obese mice treated with resveratrol is associated with alterations in the gut microbiome. Diabetes 66, 418–425. doi: 10.2337/db16-0680
Tortereau, F., Marie-Etancelin, C., Weisbecker, J. L., Marcon, D., and Bouvier, F. (2020). Genetic parameters for feed efficiency in Romane rams and responses to single-generation selection. Animal 14, 681–687. doi: 10.1017/S1751731119002544
Van Hul, M., Geurts, L., Plovier, H., Druart, C., Everard, A., Stahlman, M., et al. (2018). Reduced obesity, diabetes, and steatosis upon cinnamon and grape pomace are associated with changes in gut microbiota and markers of gut barrier. Am. J. Physiol.-Endocrinol. Metab. 314, E334–E352. doi: 10.1152/ajpendo.00107.2017
Vasta, V., Daghio, M., Cappucci, A., Buccioni, A., Serra, A., Viti, C., et al. (2019) Invited review: plant polyphenols and rumen microbiota responsible for fatty acid biohydrogenation, fiber digestion, and methane emission: experimental evidence and methodological approaches. J. Dairy Sci. 102, 3781–3804. doi: 10.3168/jds.2018-14985
Zened, A., Combes, S., Cauquil, L., Mariette, J., Klopp, C., Bouchez, O., et al. (2013). Microbial ecology of the rumen evaluated by 454 GS FLX pyrosequencing is affected by starch and oil supplementation of diets. FEMS Microbiol. Ecol. 83, 504–514. doi: 10.1111/1574-6941.12011
Keywords: ruminal microbiota, feed efficiency, feeding behavior, lamb, selection
Citation: Marie-Etancelin C, Tortereau F, Gabinaud B, Martinez Boggio G, Le Graverand Q, Marcon D, De Almeida M-L, Pascal G, Weisbecker J-L and Meynadier A (2021) Apart From the Diet, the Ruminal Microbiota of Lambs Is Modified in Relation to Their Genetic Potential for Feed Efficiency or Feeding Behavior. Front. Microbiol. 12:759432. doi: 10.3389/fmicb.2021.759432
Received: 16 August 2021; Accepted: 05 October 2021;
Published: 25 October 2021.
Edited by:
Carl James Yeoman, Montana State University, United StatesReviewed by:
Stephan Schmitz-Esser, Iowa State University, United StatesCopyright © 2021 Marie-Etancelin, Tortereau, Gabinaud, Martinez Boggio, Le Graverand, Marcon, De Almeida, Pascal, Weisbecker and Meynadier. This is an open-access article distributed under the terms of the Creative Commons Attribution License (CC BY). The use, distribution or reproduction in other forums is permitted, provided the original author(s) and the copyright owner(s) are credited and that the original publication in this journal is cited, in accordance with accepted academic practice. No use, distribution or reproduction is permitted which does not comply with these terms.
*Correspondence: Christel Marie-Etancelin, Y2hyaXN0ZWwubWFyaWUtZXRhbmNlbGluQGlucmFlLmZy
Disclaimer: All claims expressed in this article are solely those of the authors and do not necessarily represent those of their affiliated organizations, or those of the publisher, the editors and the reviewers. Any product that may be evaluated in this article or claim that may be made by its manufacturer is not guaranteed or endorsed by the publisher.
Research integrity at Frontiers
Learn more about the work of our research integrity team to safeguard the quality of each article we publish.