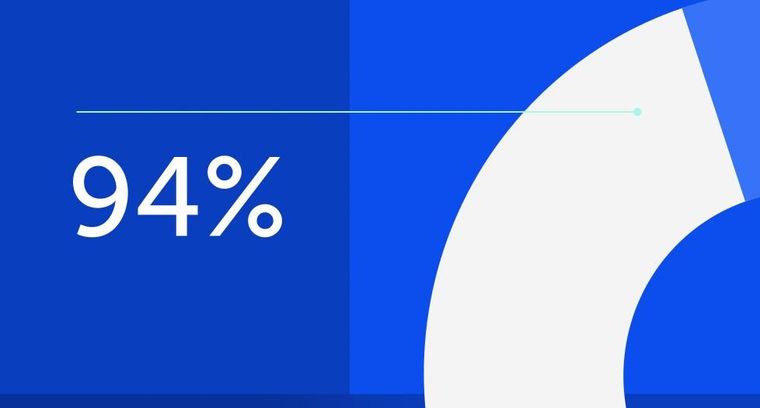
94% of researchers rate our articles as excellent or good
Learn more about the work of our research integrity team to safeguard the quality of each article we publish.
Find out more
ORIGINAL RESEARCH article
Front. Microbiol., 12 November 2021
Sec. Systems Microbiology
Volume 12 - 2021 | https://doi.org/10.3389/fmicb.2021.752674
The predominance of bacterial taxa in the gut, was examined in view of the putative antimicrobial peptide sequences (AMPs) within their proteomes. The working assumption was that compatible bacteria would share homology and thus immunity to their putative AMPs, while competing taxa would have dissimilarities in their proteome-hidden AMPs. A network–based method (“Bacterial Wars”) was developed to handle sequence similarities of predicted AMPs among UniProt-derived protein sequences from different bacterial taxa, while a resulting parameter (“Die” score) suggested which taxa would prevail in a defined microbiome. T he working hypothesis was examined by correlating the calculated Die scores, to the abundance of bacterial taxa from gut microbiomes from different states of health and disease. Eleven publicly available 16S rRNA datasets and a dataset from a full shotgun metagenomics served for the analysis. The overall conclusion was that AMPs encrypted within bacterial proteomes affected the predominance of bacterial taxa in chemospheres.
In terms of species and population, bacterial communities occur in dynamic equilibria related to environmental factors that drive interspecies competition and coexistence. Different species may facilitate their survival in a particular environment (e.g., gut and biofilms) by sharing information on existing sources of food or threats using small molecular messengers (Mukherjee and Bassler, 2019). Apart from aiding each other, bacteria most often compete using toxic molecules. In a close contact intercellular war, they may inject lethal molecules (peptidoglycan hydrolases, phospholipases, pore forming proteins, DNases, RNases, NAD(P)+ hydrolases, and ADP-ribosyltransferase among others), to suppress antagonistic species using the type VI secretion system (Coulthurst, 2019; Ross et al., 2019). Another type of antagonism is the secretion of toxic molecules destined for competitors (Richards et al., 2017). If the toxic effector molecules are proteinaceous they are called bacteriocins (Cotter et al., 2013). The term describes a broad and heterogenous category of molecules that can inhibit bacterial growth while being present in a defined contained environment (chemosphere) (Baquero et al., 2019). They are roughly divided in two categories: one of transmembrane proteins [colicins (Kleanthous, 2010)] and another of peptides (class I bacteriocins) (Riley and Wertz, 2002). Microcins are class I bacteriocins with pluripotent inhibitory actions that may act in synergy with the larger colicins for even broader inhibition effects (Baquero et al., 2019). Bacteriocins have a narrow target range and are designed to benefit the species that produces them over its closely related competitors.
Antimicrobial peptides (AMPs), a term first used for antibacterial peptides of the innate immune response of eukaryotes, are found in all kingdoms of life (Hancock, 2001). Eukaryotic AMPs mostly target and disrupt bacterial membranes at micromolar concentrations, without interacting with receptors (Kumar et al., 2018). They take advantage of the specific lipid constitution of bacterial membranes [very polar parts of the exposed lipid heads (Kumar et al., 2018)] and the curvature of the bacterial membrane (Matsuzaki et al., 1998; Drin and Antonny, 2010) to act as detergents lysing the bacterial cell. AMP-like detergents may constitute part of bacterial defenses against other bacterial species [e.g., class I bacteriocin nisin from Lactococcus lactis (Breukink et al., 1999), mersacidin from Bacillus sp. (Chatterjee et al., 1992), bacteriocins MccV, MccE492, and MccL]. The bacteria generating AMPs are not harmed due to specific immunity mechanisms that include specific AMP pumps (Beis and Rebuffat, 2019), or dedicated immunity proteins embedded within the cellular membrane, that specifically bind to the cognate AMPs on the extracellular side preventing its entrance-damage to the cell (Hassan et al., 2012; Beis and Rebuffat, 2019).
Antimicrobial peptides maintain a balanced microbiome and establish compatibility between bacterial populations in niches such as the human gut (Kerr et al., 2002; Kirkup and Riley, 2004; Majeed et al., 2011). Apart from their role in microbial biodiversity, AMPs have been employed as antimicrobials. Purified AMPs have been used to extend food preservation time, in the treatment of infectious diseases (e.g., skin infections and wounds) (Pfalzgraff et al., 2018) and cancer (Rodrigues et al., 2019). The therapeutic potential of AMPs as a replacement drug candidate for antibiotics has immense potential, in particular for the treatment of pathogens resistant to antibiotics (Yang et al., 2014).
Antimicrobial peptides that form pores are generally considered α-helical amphipathic molecules that may act as detergents on bacterial membranes (Blondelle et al., 1999). The amphipathicity and α-helical secondary structure characteristics have been exploited by a number of prediction tools in order to predict AMPs in a given protein sequence (Gabere and Noble, 2017). Detailed studies on mutations affecting the toxicity of eukaryotic bovine peptide bactenecin 2A (Hilpert et al., 2005) have been used for the formulation of AMPA, a predictor of antimicrobial peptides in a protein sequence (Torrent et al., 2009, 2012). Specifically, AMPA uses a sophisticated algorithm that gives an antimicrobial propensity value for a selected peptide stretch (Torrent et al., 2009). Each amino acid of the stretch is assigned an “antimicrobial index” value derived from high-throughput screening results (antimicrobial IC50 values) concerning amino acid replacements of the AMP bactenecin 2A (Hilpert et al., 2005). AMPA has been thoroughly validated in silico exhibiting accurate prediction of 80–90% of the assessed antimicrobial proteins including human ribonucleases, lysozymes and bacterial bacteriocins (Torrent et al., 2009).
We wondered whether bacterial proteomes might hide antibacterial stretches that could be used for interbacterial competition among different genera. Assuming that sequence similarities of AMPs from the proteomes of different bacteria could form a basis for interspecies competition, a computational method termed Bacterial Wars (BW) was developed and employed herein to explore the relationship between putative AMPs and bacterial predominance. The BW method (i) analyzed and compared putative AMPs hidden in the proteomes of different bacterial taxa and (ii) used these AMPs to predict interbacterial antagonism based on their sequence similarities. Finally, we put the hypothesis to the test by comparing the outcome of BW, to the abundance of bacterial genera in eleven publicly available 16S rRNA derived datasets as well as the species from a high-resolution full shotgun metagenomics dataset. The overall outcome was that AMPs embedded within bacterial proteomes may affect prevalence of individual taxa in the gut. To differentiate this form of competition from previously known specialized mechanisms, the novel concept is coined as “putative AMP defense.”
To detect and analyze putative AMPs from different bacterial species we first created a database (available as a data list – see below for details) that related bacterial species by the sequence similarities of their AMP. The database was then queried to form networks of bacterial interactions based on the number of common AMPs (details below). To achieve these goals and develop the BW method, the following steps were performed: (1) All proteomes from ∼3000 bacterial strains were downloaded from UniProt. Only Swiss-Prot sequences were retained from the proteomes as these are fully curated. (2) The curated protein sets of these species were used as input for the AMPA software (Torrent et al., 2009, 2012), which predicted and assigned a propensity score to all putative AMP sequences. Over 300,000 AMPs were predicted for all bacterial species, with some showing higher numbers of predicted AMPs with respect to others (Figure 1A). Although bacterial putative AMPs were in high numbers they trailed behind the number of putative AMPs from human (Figures 1A,B). Still their numbers were significantly higher than the 200 experimentally verified peptides attributed so far to all bacterial species (Kumar et al., 2018). Certain species (e.g., Buchnera aphidicola) deviated from the fitted dashed regression line, highlighting a disproportional ratio of predicted AMPs (high) with respect to proteins included in the analysis. (3) The resulting bacterial AMPs were next fed into CdHit (Li and Godzik, 2006; Fu et al., 2012) to obtain clusters of highly similar peptides (>80% sequence similarity). This resulted in 109,678 clusters, most of which were comprised of solely one member, namely AMPs which are unique to one species. Further analysis of the unique AMPs revealed that they were encrypted in specific protein categories, especially proteins binding to nucleic acids (Figure 1C). A full list of all the protein description in clusters with unique AMPs are available as Supplementary Table 1. Histograms showing the overall cluster distribution obtained from CdHit also highlight a large portion of unique AMPs (Figure 1D). (4) Next, an adjacency matrix was created by calculating the number of highly similar/common AMPs between all pairs of species in our dataset. (5) This matrix was later used to create an edge list for the construction of the BW database (the edge list is available as Supplementary Table 2). (6) BW utilized this information to construct and visualize genus-to-genus (which can also extend to species-to-species) networks. Network nodes denote species or taxa and edges denote nodes with common AMPs. The weight of the edges is proportional to the number of shared common AMPs. The overall methodology is described schematically in Figure 2.
Figure 1. (A) Normalized AMP counts for bacterial species with the highest number of predicted AMPs (1–25), selected species of interest (26–28) and human. AMP counts were normalized by the number of proteins included in the prediction process (AMP/protein ratio). Due to filtering of proteins (only curated Swiss-Prot taken in consideration) some species had very few remaining proteins. For visualization purposes, only species with at least 140 remaining proteins are shown above. The color scale represents the actual number of AMPs for each organism. (B) Scatter plot showing the number of predicted AMPs against the number of proteins used as input to AMPA software for each organism. Numerical labels correspond to the species in 1a while color groups represent taxa. (C) The 25 most enriched protein categories derived from clusters of size 1 (i.e., unique AMPs). (D) Histogram for all 109.678 clusters showing the distribution of clusters size (i.e., number of AMPs within the cluster – AMPs with >80% sequence similarity). The red bar highlights the large number of unique AMPs (cluster size of 1).
Figure 2. Schematic workflow of the BW methodology. (1) All bacterial proteomes were retrieved from UniProt and filtered to extract the Swiss-Prot curated sequences. (2) AMP perdition was performed using AMPA. (3) CdHit was used to cluster AMPs based on sequence similarity (threshold >80%). (4) Pairwise comparison was performed for all bacterial taxa to obtain the number of common AMPs for each bacterial pair (i.e., creating an adjacency matrix). (5) The adjacency matrix was used to construct an edge list, which, was used to create the BW database of interactions. (6) Querying the BW database for selected taxa allows for networks of interactions to be generated where bacteria taxa are represented as nodes and the number of common peptides as the edge weight.
Following the generation of networks of bacterial relationships based on their common AMPs, a novel network-based score was introduced (termed Die score). The Die score was used for calculating the relative likelihood for any given bacterial species (node) to die in a specific microbiome. We hypothesized that since bacteria are immune to their own AMPs, taxa that shared many common AMPs (high edge weight) would compete less. Therefore, the capacity of bacterial taxa to antagonize each other should be inversely dependent on the similarity of their AMPs. The Die score can quantify this hypothesis using network topology calculations, thus providing measure of how likely a bacterial node will die in the existing network of bacterial interactions. The higher (more positive) the Die score, the more likely for a species to die in the underlining microbiome, while smaller (or negative) Die scores exhibit the opposite trend. A mathematical explanation of the Die score and how it is calculated for all nodes in a given network is described in the section “Materials and Methods.”
Having constructed a methodology (BW) to extract and analyze relationships among different proteomes according to their predicted AMPs, validation of the outcome was performed using MB data from eleven 16S rRNA gene plus 1 shotgun gut microbiome datasets (Duvallet et al., 2017). The MB data provide an accurate representation of actual bacterial abundances in the underlying microbiomes. All bacterial abundances were extracted at the genus level from each 16S rRNA gene MB dataset and at the species level for the shotgun MB dataset. Two parallel approaches were performed using the Die score, one for BW and one for MB–generated networks. A detailed overview of the validation process using one of the microbiomes [Zhu et al. (2013), non-alcoholic steatohepatitis (NASH) vs. Healthy] (Zhu et al., 2013) was performed as a case study (Figure 3). The process involved six steps: (1) a list of bacterial genera was extracted from the Zhu et al. (2013), NASH dataset and used to query the BW database (edge list file) to obtain information on the number of shared common peptides between all pairs of bacterial genera in the microbiome list. (2) A network of bacteria genera, termed the BW network, was constructed using the information available from the BW database. Genera were denoted as network nodes and the number of common AMPs defined their edge weights. Similarly, (3) a network of co-occurrence was created using the abundance data available for the Zhu et al. (2013), NASH dataset, termed the MB network. Co-occurrence networks were constrained by different correlation strengths (rho) including both positive co-occurrence (+rho) and negative co-occurrence (−rho) networks. Different rho cut-offs were set according to the size of the dataset in-hand. The co-occurrence network was used as the validation network. (4) For an examined dataset, Die scores were calculated for all the nodes in both the BW network and the MB network. The MB network did not provide any information on AMPs. To render it comparable with the BW network, we transformed the MB network to a bi-directed network where the outgoing edge weights represented the abundance of the bacterial genus at any given node (see subnetworks in Figure 3, step 4). In the bi-directed MB network, high weight of an outgoing edge (high abundance), denoted greater compatibility within a particular microbiome. Inversely, low weights of an outgoing edge (lower abundance), denoted less compatibility among bacterial genera within the microbiome under investigation. The construction of the bi-directed MB network allowed for the application of the Die score to both BW and MB networks, resulting in two lists of Die scores. (5) An intersection of the two types of networks was obtained, as some genera were not included in the BW database. A partial table with the lists obtained from the intersection of Die scores is shown in step 5 of Figure 3. Supplementary Table 3 shows the full lists of genera and their Die scores, for both the BW and BW networks for the case study by Zhu et al. (2013), as well as all additional datasets analyzed herein. Finally, (6) the correlation between the Die score lists from both networks was calculated to provide a statistical significance for the validity of the working hypothesis (see next section for detailed results).
Figure 3. Schematic workflow of the BW validation process. (1) A list of microbial genera was extracted from microbiome dataset (e.g., Zhu et al. (2013) NASH vs. Control gut MB dataset). (2) The BW database was queried using this list and the relevant BW network was constructed from the results obtained from this query. (3) A MB co-occurrence bi-directed network using a rho = 0.4 and p-value = 0.05 was constructed from the abundance table for the case study dataset. (4) Die scores were calculated for both networks and normalized by subtracting the mean and dividing by the standard deviation. (5) Some bacterial genera were not included in the BW database and therefore only the intersection of Die scores (full list in Supplementary Table 3) from both networks was taken into consideration in step 5. The rows in the table are highlighted in red (maximum, positive Die score) and green (minimum, negative Die score), following the legend shown in the bottom right panel. The transition from red to green denotes genera, which are more or less likely to die, respectively. (6) Pearson’s correlation coefficient was calculated for the BW min/max Die scores obtaining an r-value of 1.
The two Die score lists generated from the taxa of the BW and MB networks (Figure 3 steps 5–6) were compared using the Pearson’s correlation coefficient. A positive correlation would provide evidence in favor of the putative AMP defense hypothesis. Initially, taxa with the highest (maximum) and lowest (minimum) Die scores from a given BW network were compared to the Die scores from the respective MB network.
A complete agreement was observed in the correlation coefficient (r = 1) across all eleven 16S microbiome datasets using this initial assessment (Supplementary Figure 1). However, correlation was not observed for the shotgun metagenomics dataset at the species level. When taxa with the second highest and lowest Die scores were compared, the correlation dropped for some datasets, averaging a correlation coefficient of r = 0.425 across all twelve microbiomes. Similarly, when the comparison was extended to include taxa with the third highest and lowest Die scores, Pearson’s correlations averaged a total of r = 0.325 across all datasets (Supplementary Figure 1). Thus, BW and MB data were in agreement for genera with the highest (maximum) and lowest (minimum) Die scores but not for all taxa with intermediate Die score values and not for individual species.
A correlation coefficient provides an adequate insight of the trend of the data. However, if a specific genus or species achieves a high Die score in both BW and MB networks but does not appear in the same ranking in both networks, then it would not adhere to the correlation trend. This would result in a poor Pearson’s correlation coefficient, even though the underlined genus/species scores were high in both incidents. To obtain insights that a Pearson’s correlation coefficient measure could not provide, the shape of distributions of the Die score values (skewness) was examined. First, taxa were ranked according to their Die scores as derived from the two networks and the absolute distance of the rankings was calculated (see Table 1). The new measure (Dist) provided an indication of the difference in magnitude between the Die scores from the two different networks. Data that were positively skewed with respect to their distances (Dist) indicated that there was a small difference between the taxa rankings based on their Die score. For example, taxa with high or low Die scores in one distribution (i.e., BW), also achieved proportionally high or low Die scores in the second distribution (i.e., MB). This would provide evidence to support the validity of the putative AMP defense hypothesis and would agree with bacterial predominance based on proteome-derived AMPs as a potential mechanism for bacterial prevalence in a specific microbiome. On the other hand, data with a negatively skewed Dist would mean that the Die score rankings had large differences. For example, taxa with high or low Die scores under one distribution, would achieve inversely proportional low and high scores in the other. This would provide evidence against the validity of the putative AMP defense hypothesis. Table 1 shows the procedure of calculating the absolute distance (Dist). A full list of all the taxa can be found as Supplementary Table 3. The use of the Dist measure to investigate skewness for the twelve microbiome datasets showed that they adhered to positively skewed distributions with respect to their Dist measure (Figure 4). Therefore, skewness comparisons showed a notable agreement for the BW and MB networks that previously seemed not to correlate using the Pearson’s correlation coefficient only.
Table 1. Absolute distance (Dist) calculation using Zhu et al. (2013) NASH dataset as a case study.
Figure 4. Visualization of skewness for Die score differences for BW and MB networks. Histogram plots for all the data (disease and control) in twelve microbiomes [including Zhu et al. (2013) dataset – labeled as nash_ob_baker], showing the distribution and skewness of the distances (Dist). Positively skewed distributions of Dist measures were observed for all twelve microbiomes.
Statistical analysis of the Die scores between BW and MB networks was performed by the Wilcoxon signed-rank test. This is a non-parametric statistical test for paired groups and was used to compare the two Die score lists and assessed whether the ranking order of their values differed. The differences in the rankings of the Die scores from the BW and MB networks across all twelve MB datasets (Figure 5), were not statistically significant (p-values > 0.05). In other words, there was no evidence to reject the null hypothesis stating that the two Die score lists have the same continuous distribution. This provides evidence in favor of the putative AMP defense hypothesis, according to which the Die score list distributions between BW and MB networks should be similar.
Figure 5. Wilcoxon signed-rank test for BW and MB networks. The p-values are all >0.05, indicating that the results obtained from the Wilcoxon tests using the ranked Die scores were not statistically significant between the BW and MB networks. This is in favor of the putative AMP defense hypothesis that would expect similar distributions for both types of Die score lists.
Die scores from BW networks and MB co-occurrence networks for twelve gut microbiome datasets were positively correlated (positive skewness for Dist). In these comparisons, all healthy and all diseased state microbiomes for each of the 12 datasets were grouped together (the analyzed MB datasets contained both disease and control samples). Next, we examined whether correlations would be affected upon separation of the MB data into different sample types with greater homogeneity. Therefore, the analysis steps outlined in Figure 3 were repeated after separating the twelve gut MB data to their health and diseased states.
Taxa with the highest (maximum) and lowest (minimum) Die scores from the BW network were positively correlated to the Die scores from the corresponding taxa from the MB network for 10 out of 12 datasets (Table 2A). In addition, the skewness of the different Die scores was also positively skewed for 12 out of 12 (100%) of the MB-control networks when compared to the corresponding BW network’s Die scores (Supplementary Figure 2 and Supplementary Table 4).
Table 2. Top Die score taxa for healthy and disease MB networks and their correlation with BW Die scores.
Performing the same analysis on the diseased state microbiome datasets, taxa with the highest (maximum) and lowest (minimum) Die scores from the BW network were in overall positive correlation (10 out of 12 datasets) with the Die scores from the corresponding taxa from the MB network. An exception was the microbiome data set from the nash_chan dataset (Table 2B). In addition, the different Die scores were also positively skewed for 9 out of 12 (75%) of the MB-diseased state networks (Supplementary Figure 3 and Supplementary Table 4).
In both case studies, taxa with high and low Die scores were different in the healthy and diseased state networks. This highlights the potential of the BW method to follow-up/predict taxa, which are more, or less likely to die in a given gut microbiome. All correlation plots for the datasets analyzed herein can be found as Supplementary Figures 4–6. Diseased state bacterial taxa corroborated by bibliographic information can be viewed in Supplementary Table 5.
In the BW methodology, clustering of highly similar peptides was based on >80% sequence similarity. This high threshold raised the possibility of a similar clustering of AMPs from genera with close phylogenetic origin. To address this issue, a phylogenetic tree of all genera used in the case study for healthy and diseased state microbiomes (Tables 2A,B) was constructed along with the Die score as predicted by BW (Figure 6). According to the presented distribution, bacteria of the same family did not have similar Die scores. This observation demonstrates that the putative AMP defense hypothesis is not biased by bacterial genera with high evolutionary conservation and therefore high genomic sequence similarity. In fact, genera such as Prevotella, Tannarella, and Bacteroides, fared differently within the specific microbiomes presented here, despite all being members of the order Bacteroidales. Prevotella obtained high Die score (more likely to die) while the latter two attained low Die scores (less likely to die). Different Die scores were obtained for Pseudobutyrivibrio, Clostridium, and Eubacterium from Clostridiales and Carnobacterium, Lactobacillus, and Streptococcus of Lactobacillales. These findings demonstrate the extreme specificity of different AMPs for different genera.
Figure 6. Phylogenetic distribution of the bacteria with the maximum (red) and minimum (green) Die scores. Bacterial genera were taken from controls and disease data, Tables 2A,B and were colored according to their BW Die scores. The taxonomic division for the different genera is shown on top and depicted as notches of different shapes on the ancestry lines. Escherichia coli (black color text) is included for comparison. Genera without numbers represent single observations from Tables 2A,B. When present, numbers represent more observations. Salmonella (blue) acquired low and high Die scores in two different microbiomes.
The concept of peptides of bacterial origin as factors of interbacterial antagonism is not new (Baquero and Moreno, 1984). Examples date back to microcin E492 which is secreted by Klebsiella pneumoniae RYC492 and is active against various Enterobacteriaceae (de Lorenzo, 1984; Thomas et al., 2004; Gillor et al., 2008). Some of these peptides [e.g., mersacidin, nisin, subtilin, cinnamycin, duramycin, actagardine, epidermin, gallidermin, lanthiopeptin (Chatterjee et al., 1992)], may require post translational modification [addition of lanthionine (Knerr and van der Donk, 2012)] to stabilize the peptide and enhance the killing effect. Synthetic improvements of hairpin AMPs have also been used (Wu and Hancock, 1999; Liu et al., 2013). Despite the wide diversity of bacterial species, the isolated and characterized AMPs from bacteria are a fraction compared to those from eukaryotes (200 bacterial AMPs compared to 2159 from animals) (Kumar et al., 2018). The herein predicted 3000 putative AMPs for E. coli only (Figure 1B), suggests that the number of bacterial (and human) AMPs may be underestimated. The high number of bacterial AMPs is suggestive of a possible underlying function. Could it be that bacteria (and perhaps other organisms) employ AMPs encrypted in their proteomes to defend themselves against foe, especially in the relatively stable environment of a chemosphere (e.g., gut)? Could it be that a dying or dead bacterium might become a source of AMPs resulting from the proteolysis of its own proteins, to antagonize “enemy” species? This possibility would require the presence of proteases in a chemosphere. Bacterial proteases do exist in the colon (Ruiz-Perez and Nataro, 2014; Sears et al., 2014; Guyton et al., 2019) as well as proteases from other microorganisms (Espinosa-Cantellano and Martínez-Palomo, 2000; Ajjampur and Tan, 2016) to account for such proteolytic activity. Most of the proteins identified as potential sources of AMPs were cationic with nucleic acid binding properties (Figure 1B). Such proteins contain many basic residues (e.g., lysines) and are thus amenable to proteolytic degradation. The concept of AMP homology among bacterial proteomes as being the main prerequisite for bacterial species compatibility cannot be envisaged without proteolysis occurring in a chemosphere that would allow for the continuous production of AMPs. A mechanism as such would relieve the cell from the need of the energy expenses to synthesize de novo AMPs by protein synthesis.
Under the supposition that a bacterial proteome is a potential source of AMPs that are not toxic to the host species and/or species that share AMP homology, a methodology was developed (“Bacterial Wars” or BW) that could predict the outcome of interbacterial competitions. BW (i) detected all putative AMPs embedded in curated proteins from UniProt-derived bacterial proteomes, (ii) clustered the detected AMPs by sequence similarity (iii) created a database of bacterial taxa based on AMP sequence similarities and finally (iv) predicted specific taxa that were more likely to “die” in a given microbiome. The final step was achieved using a novel network-based scoring scheme (termed “Die score”) that provided a measure of how likely a given microbial genus/species was to “die” in a given niche. It should be noted that the Die score is a network measure that may not be restricted to this work. It can be applied to any network that may follow similar assumptions to the bacterial networks generated from the BW method (e.g., co-occurrence networks). The methodology was used to provide evidence in favor of the putative AMP defense hypothesis using eleven 16S rRNA gene datasets as well as one full shotgun metagenomics dataset from the gut microbiome, where the outcomes of the BW method were compared to co-occurrence networks derived from these data. In almost all examined cases, bacterial predominance seemed to be affected by the similarity of encoded AMPs in bacterial proteins.
Co-occurrence networks are subject to change depending on the parameters used during the algorithm employed for their construction (i.e., the choice of correlation metric, the p-value cut-off etc.). This directly impinges on the correlation scores between Die scores for BW and MB networks and although we have tried to be as judicious as possible in the use of these parameters across the twelve datasets assessed in this study, there is still an aspect of subjectivity in the use of these parameters that could potentially affect results. Another concern is the exclusive use of AMPA as a detector of AMPs. It could well be that not all AMPs are detectable by AMPA and not all AMPs predicted by AMPA may have antibacterial properties. All these concerns must be addressed experimentally, which is not a trivial task. We can only state that under the current handling and analysis of proteomes, AMPs and bacterial abundances, putative AMPs seem like a significant factor in the interaction of different bacteria in a chemosphere (putative AMP defense hypothesis).
Numerous databases describe AMPs from prokaryotic and eukaryotic organisms (Seshadri Sundararajan et al., 2012; Gogoladze et al., 2014; Fan et al., 2016; Lin et al., 2016; Pirtskhalava et al., 2016; Waghu et al., 2016; Wang et al., 2016). They host experimentally validated, as well as computationally predicted, AMPs and are fully searchable allowing for queries of multiple search criteria. However, they do not provide any information on the relationship among the different AMPs or some background to explain their efficiency against specific organisms. The herein developed database combined with the BW methodology has the potential of detecting relationships between AMPs in the proteomes of different bacterial taxa and provide such information for further experimentation purposes. Predictions on the predominance and outlook of specific taxa in a given microbiome may lie within the potential of the method.
A first glance on bacterial genome size and AMP count suggests that the two are not related (Figure 1A). The aphid dwelling Buchnera aphidicola with a relatively small genome appears as having the highest number of putative AMPs followed by Wigglesworthia glossinidia, that lives in the gut of tsetse flies and is considered as having one of the smallest known genomes of any living organism. On the other hand, the predatory Bdellovibrio bacteriovorus, and Fusobacterium nucleatum of the oral cavity, seem as having less putative AMPs. E. coli lab strain K12 has a large number of putative AMPs (Figure 1B) but relatively small compared to its proteome (Figure 1A). Could it be that bacteria that live in relatively host-independent conditions (more open chemospheres) may need less AMPs? The relationship between, abiotic conditions of living, surrounding microbiomes and hosts are some of the issues that could be the object of a comparative study with the aim of explaining the different numbers of AMPs in different bacterial taxa.
A large proportion of the putative AMPs were unique as they shared low sequence similarity (<80%) (Figure 1C). This could be viewed as the extreme versatility of the combinatorial capabilities of microorganisms to create diverse AMPs to face an opponent species. Comparison of the Die scores in bacteria with close phylogenetic origin showed that putative AMPs were specific to the level of genera (Figure 6).
In summary, we propose that putative AMPs hidden within the proteome of bacteria may affect their symbiosis with other species in a given chemosphere. In support of the putative AMP defense hypothesis, we provide computational data corroborated by gut microbiome datasets on the abundance of bacterial species in the enterobiomes of different diseases. We believe that our findings may add another jigsaw in the mosaic of bacterial interactions and could perhaps provide leads for the construction of species-specific AMPs.
16S rRNA gene processed OTU tables were used. These represent the abundances (in counts) of specific taxa in eleven gut microbiome datasets. The datasets were downloaded from the MicrobiomeHD database (Duvallet et al., 2017), https://zenodo.org/record/569601#.XdPrYmgzaUk.
The datasets included:
1. EDD_Singh, 2015 enteric diarrheal disease (EDD) – (Singh et al., 2015).
2. CDI_Schubert, 2014 Clostridium difficile infection (CDI) – (Schubert et al., 2014).
3. OB_Zupancic, 2012 Obesity (OB) (Zupancic et al., 2012).
4. HIV_Noguera-Julian, 2016 HIV (Noguera-Julian et al., 2016).
5. HIV_Dinh, 2014 HIV (Dinh et al., 2015).
6. MHE_Zhang, 2013 liver diseases (LIV) (Zhang et al., 2013).
7. PAR_Scheperjans, 2014 Parkinson’s (PAR) (Scheperjans et al., 2015).
8. ASD_KB, 2013 Autism (ASD) (Kang et al., 2013).
9. ASD_Son, 2015 Autism (ASD) (Son et al., 2015).
10. NASH_Chang, 2013, non-alcoholic steatohepatitis (NASH) (Wong et al., 2013).
11. NASH_OB_Baker, 2013, non-alcoholic steatohepatitis (NASH) (Zhu et al., 2013).
Shotgun metagenomics dataset:
1. Parkinson’s disease vs. control metagenomics dataset (Becker et al., 2021).
AMPA (Torrent et al., 2009, 2012), is a publicly available tool for prediction of antimicrobial peptides in a given protein sequence. It is available as a web browser1 but also as a standalone tool. We downloaded and utilized the source code for AMPA written in PERL and available under Linux, in order to perform batch runs for our proteins of interests as detailed in Figure 2. We ran AMPA using the default settings for all input protein sequences.
CdHit was used to cluster AMPs by applying a sequence similarity threshold. AMP similarity was set up to support the hypothesis that bacterial species that share common AMPs (sequence similarity >80%) may coexist more harmoniously in comparison to species that share less or no sequence similarity in their AMPs.
Two different types of networks were constructed using: (i) the BW database (available as an edge list – see Supplementary Table 2) and (ii) MB abundance tables. Network constructions and co-occurrence calculations were performed in R using the following library packages: phyloseq, vegan, ape, WGCNA, igraph, and network. All networks for the 16S data were constructed at the genus level, while the network constructed for the shotgun metagenomics dataset depicts species level associations All networks generated for the BW methodology can be found as Supplementary Figures 7–10. The networks at the genus level were constructed by aggregating all the AMPs found in the species belonging to a specific genus.
The Die score provides a measure of how likely a particular bacterial node (genus/species) is to “die” in a given microbiome environment characterized by a network. It can also be considered as a projection of the relative abundance of bacterial taxa based on their topology in a network. The putative AMP defense hypothesis assumes that bacteria’s capacity to kill each other is inversely dependent on the similarity of their peptides. Therefore, for the BW approach, we created a graph BW with nodes representing different bacterial taxa and edges representing their common peptides.
For a node i in a given graph BW, we calculated the Die score (D) with equation (1):
Where: Si represents the strength of the node (i.e., the weighted degree of the node i) and sums up the number of neighboring bacteria that node i is linked to via an edge (e.g., shares common peptides), as well as the edge weight (e.g., number of common peptides). Ni represents the number of pairs with no edges (e.g., no common peptides). It is calculated using the expression Ni = Np − degi, where degi represents the degree of the node i. Np = (n2 − n)/2 represents the number of all possible pairs in the network.
The Die score can take values in the range of [−1, 1]. A value of 1 means that a particular bacterial taxon (genus/species) is more likely to die, while a value of −1 means exactly the opposite (less likely to die). After the initial calculation using eq. (1), the Die score was normalized by extracting the mean and dividing by the standard deviation. This allowed for a greater range for the Die score for better highlighting differences among the taxa analyzed by this measure.
The Die score formula described above, can also be applied to microbiome (MB) co-occurrence networks (or graphs). In this case, instead of common AMPs, the edges represent the relative abundances of the particular bacterial taxa (represented as nodes). The co-occurrence networks have to be modified to bi-directed networks, whereby the outgoing edge weight represents the relative abundance of the specific node under consideration.
The Dist measure was given by the following eq. (2):
RNK is the ranking function performed, whereby all taxa are ranked according to their position in the Die score vector (the genus/species with the lowest Die was ranked as 1, and increasing rankings denote higher Die scores). The R function named “rank(),” was used to rank the Die scores. In case of ranking ties, the average of the rankings was calculated, accounting for some decimal places in the ranking tables (as shown in Supplementary Table 3). Dist is calculated as the absolute difference between the ranked Die scores from the BW and MB networks, DieBW and DieMB, respectively.
Skewness was calculated using the R function “skewness()” in the e1071 package, according to Joanes and Gill (1998). The equation used is: G_1 = g_1 ∗ sqrt(n(n-1)/(n-2). Positive skewness was determined by any one of the following observations: histograms showing a short left tail and a long right tail, skewness calculations with value >0.4 and/or median of the distribution being closer to the first quartile – as depicted in the boxplots.
Publicly available datasets were analyzed in this study. This data can be found here: https://zenodo.org/record/569601#.XdPrYmgzaUk, https://www.medrxiv.org/content/10.1101/2021.02.07.21251098v1.
AO, MZ, and GMS designed the study. AO and MZ conceived and developed the computational tools. AO, CC, and MT analyzed the data and interpreted the results. GMS and AV-G interpreted the results. UI contributed to code for the computational tools. GPS contributed to the Parkinson’s metagenome dataset. AO and AV-G designed the experiments and wrote the manuscript. AV-G conceived the project. All authors edited the manuscript.
This work was supported by the European Commission Research Executive Agency Grant BIORISE (No. 669026), under the Spreading Excellence, Widening Participation, Science with and for Society Framework, and project “Research Infrastructure on Food Bioprocessing Development and Innovation Exploitation – Food Innovation RI” (MIS 5027222), which is implemented under the Action “Reinforcement of the Research and Innovation Infrastructure,” funded by the Operational Program “Competitiveness, Entrepreneurship and Innovation” (NSRF 2014–2020) and co-financed by Greece and the European Union (European Regional Development Fund). This publication has been financed by the Research Committee of the University of Patras.
The authors declare that the research was conducted in the absence of any commercial or financial relationships that could be construed as a potential conflict of interest.
All claims expressed in this article are solely those of the authors and do not necessarily represent those of their affiliated organizations, or those of the publisher, the editors and the reviewers. Any product that may be evaluated in this article, or claim that may be made by its manufacturer, is not guaranteed or endorsed by the publisher.
The Supplementary Material for this article can be found online at: https://www.frontiersin.org/articles/10.3389/fmicb.2021.752674/full#supplementary-material
Ajjampur, S. S. R., and Tan, K. S. W. (2016). Pathogenic mechanisms in Blastocystis spp. — Interpreting results from in vitro and in vivo studies. Parasitol. Int. 65, 772–779. doi: 10.1016/j.parint.2016.05.007
Baquero, F., and Moreno, F. (1984). The microcins. FEMS Microbiol. Lett. 23, 117–124. doi: 10.1111/j.1574-6968.1984.tb01046.x
Baquero, F., Lanza, V. F., Baquero, M. R., del Campo, R., and Bravo-Vázquez, D. A. (2019). Microcins in Enterobacteriaceae: peptide antimicrobials in the eco-active intestinal chemosphere. Front. Microbiol. 10:2261. doi: 10.3389/fmicb.2019.02261
Becker, A., Schmartz, G. P., Gröger, L., Grammes, N., Galata, V., Philippeit, H., et al. (2021). Effects of resistant starch on symptoms, fecal markers and gut microbiota in Parkinson’s Disease – the resista-PD trial. medRxiv [Preprint] medRxiv: 2021.02.07.21251098,
Beis, K., and Rebuffat, S. (2019). Multifaceted ABC transporters associated to microcin and bacteriocin export. Res. Microbiol. 170, 399–406. doi: 10.1016/j.resmic.2019.07.002
Blondelle, S. E., Lohner, K., and Aguilar, M. I. (1999). Lipid-induced conformation and lipid-binding properties of cytolytic and antimicrobial peptides: determination and biological specificity. Biochim. Biophys. Acta Biomembr. 1462, 89–108. doi: 10.1016/S0005-2736(99)00202-3
Breukink, E., Wiedemann, I., Van Kraaij, C., Kuipers, O. P., Sahl, H. G., and De Kruijff, B. (1999). Use of the cell wail precursor lipid II by a pore-forming peptide antibiotic. Science 286, 2361–2364. doi: 10.1126/science.286.5448.2361
Chatterjee, S., Chatterjee, S., Lad, S. J., Phansalkar, M. S., Rupp, R. H., Ganguli, B. N., et al. (1992). Mersacidin, a new antibiotic from bacillus fermentation, isolation, purification and chemical characterization. J. Antibiot. (Tokyo) 45, 832–838. doi: 10.7164/antibiotics.45.832
Cotter, P. D., Ross, R. P., and Hill, C. (2013). Bacteriocins-a viable alternative to antibiotics? Nat. Rev. Microbiol. 11, 95–105. doi: 10.1038/nrmicro2937
Coulthurst, S. (2019). The type VI secretion system: a versatile bacterial weapon. Microbiology (United Kingdom) 165, 503–515. doi: 10.1099/mic.0.000789
de Lorenzo, V. (1984). Isolation and characterization of microcin E 492 from Klebsiella pneumoniae. Arch. Microbiol. 139, 72–75. doi: 10.1007/BF00692715
Dinh, D. M., Volpe, G. E., Duffalo, C., Bhalchandra, S., Tai, A. K., Kane, A. V., et al. (2015). Intestinal Microbiota, microbial translocation, and systemic inflammation in chronic HIV infection. J. Infect. Dis. 211, 19–27. doi: 10.1093/infdis/jiu409
Drin, G., and Antonny, B. (2010). Amphipathic helices and membrane curvature. FEBS Lett. 584, 1840–1847. doi: 10.1016/j.febslet.2009.10.022
Duvallet, C., Gibbons, S. M., Gurry, T., Irizarry, R. A., and Alm, E. J. (2017). Meta-analysis of gut microbiome studies identifies disease-specific and shared responses. Nat. Commun. 8:1784. doi: 10.1038/s41467-017-01973-8
Espinosa-Cantellano, M., and Martínez-Palomo, A. (2000). Pathogenesis of intestinal amebiasis: from molecules to disease. Clin. Microbiol. Rev. 13, 318–331. doi: 10.1128/CMR.13.2.318-331.2000
Fan, L., Sun, J., Zhou, M., Zhou, J., Lao, X., Zheng, H., et al. (2016). DRAMP: a comprehensive data repository of antimicrobial peptides. Sci. Rep. 6:24482. doi: 10.1038/srep24482
Fu, L., Niu, B., Zhu, Z., Wu, S., and Li, W. (2012). CD-HIT: accelerated for clustering the next-generation sequencing data. Bioinformatics 28, 3150–3152. doi: 10.1093/bioinformatics/bts565
Gabere, M. N., and Noble, W. S. (2017). Empirical comparison of web-based antimicrobial peptide prediction tools. Bioinformatics 33, 1921–1929. doi: 10.1093/bioinformatics/btx081
Gillor, O., Etzion, A., and Riley, M. A. (2008). The dual role of bacteriocins as anti- and probiotics. Appl. Microbiol. Biotechnol. 81, 591–606. doi: 10.1007/s00253-008-1726-5
Gogoladze, G., Grigolava, M., Vishnepolsky, B., Chubinidze, M., Duroux, P., Lefranc, M. P., et al. (2014). DBAASP: database of antimicrobial activity and structure of peptides. FEMS Microbiol. Lett. 357, 63–68. doi: 10.1111/1574-6968.12489
Guyton, K. L., Levine, Z. C., Lowry, A. C., Lambert, L., Gribovskaja-Rupp, I., Hyman, N., et al. (2019). Identification of collagenolytic bacteria in human samples: screening methods and clinical implications for resolving and preventing anastomotic leaks and wound complications. Dis. Colon Rectum. 62, 972–979. doi: 10.1097/DCR.0000000000001417
Hancock, R. E. (2001). Cationic peptides: effectors in innate immunity and novel antimicrobials. Lancet Infect. Dis. 1, 156–164. doi: 10.1016/S1473-3099(01)00092-5
Hassan, M., Kjos, M., Nes, I. F., Diep, D. B., and Lotfipour, F. (2012). Natural antimicrobial peptides from bacteria: characteristics and potential applications to fight against antibiotic resistance. J. Appl. Microbiol. 113, 723–736. doi: 10.1111/j.1365-2672.2012.05338.x
Hilpert, K., Volkmer-Engert, R., Walter, T., and Hancock, R. E. W. (2005). High-throughput generation of small antibacterial peptides with improved activity. Nat. Biotechnol. 23, 1008–1012. doi: 10.1038/nbt1113
Joanes, D. N., and Gill, C. A. (1998). Comparing measures of sample skewness and kurtosis. J. R. Stat. Soc. Ser. D Stat. 47, 183–189. doi: 10.1111/1467-9884.00122
Kang, D. W., Park, J. G., Ilhan, Z. E., Wallstrom, G., LaBaer, J., Adams, J. B., et al. (2013). Reduced incidence of prevotella and other fermenters in intestinal microflora of autistic children. PLoS One 8:e68322. doi: 10.1371/journal.pone.0068322
Kerr, B., Riley, M. A., Feldman, M. W., and Bohannan, B. J. (2002). Local dispersal promotes biodiversity in a real-life game of rock-paper-scissors. Nature 418, 171–174. doi: 10.1038/nature00823
Kirkup, B. C., and Riley, M. A. (2004). Antibiotic-mediated antagonism leads to a bacterial game of rock-paper-scissors in vivo. Nature 428, 412–414. doi: 10.1038/nature02429
Kleanthous, C. (2010). Swimming against the tide: progress and challenges in our understanding of colicin translocation. Nat. Rev. Microbiol. 8, 843–848. doi: 10.1038/nrmicro2454
Knerr, P. J., and van der Donk, W. A. (2012). Discovery, biosynthesis, and engineering of lantipeptides. Annu. Rev. Biochem. 81, 479–505. doi: 10.1146/annurev-biochem-060110-113521
Kumar, P., Kizhakkedathu, J. N., and Straus, S. K. (2018). Antimicrobial peptides: diversity, mechanism of action and strategies to improve the activity and biocompatibility in vivo. Biomolecules 8:4. doi: 10.3390/biom8010004
Li, W., and Godzik, A. (2006). Cd-hit: a fast program for clustering and comparing large sets of protein or nucleotide sequences. Bioinformatics 22, 1658–1659. doi: 10.1093/bioinformatics/btl158
Lin, H., An, Y., Hao, F., Wang, Y., and Tang, H. (2016). Correlations of fecal metabonomic and microbiomic changes induced by high-fat diet in the pre-obesity state. Sci. Rep. 6:21618. doi: 10.1038/srep21618
Liu, Y. F., Xia, X., Xu, L., and Wang, Y. Z. (2013). Design of hybrid β-hairpin peptides with enhanced cell specificity and potent anti-inflammatory activity. Biomaterials 34, 237–250. doi: 10.1016/j.biomaterials.2012.09.032
Majeed, H., Gillor, O., Kerr, B., and Riley, M. A. (2011). Competitive interactions in Escherichia coli populations: the role of bacteriocins. ISME J. 5, 71–81. doi: 10.1038/ismej.2010.90
Matsuzaki, K., Sugishita, K. I., Ishibe, N., Ueha, M., Nakata, S., Miyajima, K., et al. (1998). Relationship of membrane curvature to the formation of pores by magainin 2. Biochemistry 37, 11856–11863. doi: 10.1021/bi980539y
Mukherjee, S., and Bassler, B. L. (2019). Bacterial quorum sensing in complex and dynamically changing environments. Nat. Rev. Microbiol. 17, 371–382. doi: 10.1038/s41579-019-0186-5
Noguera-Julian, M., Rocafort, M., Guillen, Y., Rivera, J., Casadella, M., Nowak, P., et al. (2016). Gut microbiota linked to sexual preference and HIV infection. EBioMedicine 5, 135–146. doi: 10.1016/j.ebiom.2016.01.032
Pfalzgraff, A., Brandenburg, K., and Weindl, G. (2018). Antimicrobial peptides and their therapeutic potential for bacterial skin infections and wounds. Front. Pharmacol. 9:281. doi: 10.3389/fphar.2018.00281
Pirtskhalava, M., Gabrielian, A., Cruz, P., Griggs, H. L., Squires, R. B., Hurt, D. E., et al. (2016). DBAASP v.2: an enhanced database of structure and antimicrobial/cytotoxic activity of natural and synthetic peptides. Nucleic Acids Res 44, 6503. doi: 10.1093/nar/gkw243
Richards, G. P., Watson, M. A., Needleman, D. S., Uknalis, J., Boyd, E. F., and Fay, J. P. (2017). Mechanisms for Pseudoalteromonas piscicidainduced killing of vibrios and other bacterial pathogens. Appl. Environ. Microbiol. 83:e00175–17. doi: 10.1128/AEM.00175-17
Riley, M. A., and Wertz, J. E. (2002). Bacteriocins: evolution, ecology, and application. Annu. Rev. Microbiol. 56, 117–137. doi: 10.1146/annurev.micro.56.012302.161024
Rodrigues, G., Silva, G. G. O., Buccini, D. F., Duque, H. M., Dias, S. C., and Franco, O. L. (2019). Bacterial proteinaceous compounds with multiple activities toward cancers and microbial infection. Front. Microbiol. 10:1690. doi: 10.3389/fmicb.2019.01690
Ross, B. D., Verster, A. J., Radey, M. C., Schmidtke, D. T., Pope, C. E., Hoffman, L. R., et al. (2019). Human gut bacteria contain acquired interbacterial defence systems. Nature 575, 224–228. doi: 10.1038/s41586-019-1708-z
Ruiz-Perez, F., and Nataro, J. P. (2014). Bacterial serine proteases secreted by the autotransporter pathway: classification, specificity, and role in virulence. Cell. Mol. Life Sci. 71, 745–770. doi: 10.1007/s00018-013-1355-8
Scheperjans, F., Aho, V., Pereira, P. A. B., Koskinen, K., Paulin, L., Pekkonen, E., et al. (2015). Gut microbiota are related to Parkinson’s disease and clinical phenotype. Mov. Disord. 30, 350–358. doi: 10.1002/mds.26069
Schubert, A. M., Rogers, M. A., Ring, C., Mogle, J., Petrosino, J. P., Young, V. B., et al. (2014). Microbiome data distinguish patients with clostridium difficile infection and non-C. difficile-associated diarrhea from healthy controls. MBio 5:e01021–14. doi: 10.1128/mBio.01021-14
Sears, C. L., Geis, A. L., and Housseau, F. (2014). Bacteroides fragilis subverts mucosal biology: from symbiont to colon carcinogenesis. J. Clin. Invest. 124, 4166–4172. doi: 10.1172/JCI72334
Seshadri Sundararajan, V., Gabere, M. N., Pretorius, A., Adam, S., Christoffels, A., Lehvaslaiho, M., et al. (2012). DAMPD: a manually curated antimicrobial peptide database. Nucleic Acids Res. 40, D1108–D1112. doi: 10.1093/nar/gkr1063
Singh, P., Teal, T. K., Marsh, T. L., Tiedje, J. M., Mosci, R., Jernigan, K., et al. (2015). Intestinal microbial communities associated with acute enteric infections and disease recovery. Microbiome 3:45. doi: 10.1186/s40168-015-0109-2
Son, J. S., Zheng, L. J., Rowehl, L. M., Tian, X., Zhang, Y., Zhu, W., et al. (2015). Comparison of fecal microbiota in children with autism spectrum disorders and neurotypical siblings in the simons simplex collection. PLoS One 10:e0137725. doi: 10.1371/journal.pone.0137725
Thomas, X., Destoumieux-Garzón, D., Peduzzi, J., Afonso, C., Blond, A., Birlirakis, N., et al. (2004). Siderophore peptide, a new type of post-translationally modified antibacterial peptide with potent activity. J. Biol. Chem. 279, 28233–28242. doi: 10.1074/jbc.M400228200
Torrent, M., Di Tommaso, P., Pulido, D., Nogués, M. V., Notredame, C., Boix, E., et al. (2012). AMPA: an automated web server for prediction of protein antimicrobial regions. Bioinformatics 28, 130–131. doi: 10.1093/bioinformatics/btr604
Torrent, M., Nogués, V. M., and Boix, E. (2009). A theoretical approach to spot active regions in antimicrobial proteins. BMC Bioinformatics 10:373. doi: 10.1186/1471-2105-10-373
Waghu, F. H., Barai, R. S., Gurung, P., and Idicula-Thomas, S. (2016). CAMPR3: a database on sequences, structures and signatures of antimicrobial peptides. Nucleic Acids Res. 44, D1094–D1097. doi: 10.1093/nar/gkv1051
Wang, G., Li, X., and Wang, Z. (2016). APD3: the antimicrobial peptide database as a tool for research and education. Nucleic Acids Res. 44, D1087–D1093. doi: 10.1093/nar/gkv1278
Wong, V. W. S., Tse, C. H., Lam, T. T. Y., Wong, G. L. H., Chim, A. M. L., Chu, W. C. W., et al. (2013). Molecular characterization of the fecal microbiota in patients with nonalcoholic steatohepatitis – a longitudinal study. PLoS One 8:e62885. doi: 10.1371/journal.pone.0062885
Wu, M., and Hancock, R. E. W. (1999). Improved derivatives of bactenecin, a cyclic dodecameric antimicrobial cationic peptide. Antimicrob. Agents Chemother. 43, 1274–1276. doi: 10.1128/aac.43.5.1274
Yang, S. C., Lin, C. H., Sung, C. T., and Fang, J. Y. (2014). Antibacterial activities of bacteriocins: application in foods and pharmaceuticals. Front. Microbiol. 5:241. doi: 10.3389/fmicb.2014.00241
Zhang, Z., Zhai, H., Geng, J., Yu, R., Ren, H., Fan, H., et al. (2013). Large-scale survey of gut microbiota associated with MHE Via 16S rRNA-based pyrosequencing. Am. J. Gastroenterol. 108, 1601–1611.
Zhu, L., Baker, S. S., Gill, C., Liu, W., Alkhouri, R., Baker, R. D., et al. (2013). Characterization of gut microbiomes in nonalcoholic steatohepatitis (NASH) patients: a connection between endogenous alcohol and NASH. Hepatology 57, 601–609. doi: 10.1002/hep.26093
Keywords: putative antimicrobial peptides, interbacterial antagonism, network analysis, bioinformatics analysis, bacterial competition
Citation: Oulas A, Zachariou M, Chasapis CT, Tomazou M, Ijaz UZ, Schmartz GP, Spyrou GM and Vlamis-Gardikas A (2021) Putative Antimicrobial Peptides Within Bacterial Proteomes Affect Bacterial Predominance: A Network Analysis Perspective. Front. Microbiol. 12:752674. doi: 10.3389/fmicb.2021.752674
Received: 03 August 2021; Accepted: 11 October 2021;
Published: 12 November 2021.
Edited by:
Antonios Alekos Augustinos, Hellenic Agricultural Organization “DEMETER”, GreeceReviewed by:
Huaxi Yi, Ocean University of China, ChinaCopyright © 2021 Oulas, Zachariou, Chasapis, Tomazou, Ijaz, Schmartz, Spyrou and Vlamis-Gardikas. This is an open-access article distributed under the terms of the Creative Commons Attribution License (CC BY). The use, distribution or reproduction in other forums is permitted, provided the original author(s) and the copyright owner(s) are credited and that the original publication in this journal is cited, in accordance with accepted academic practice. No use, distribution or reproduction is permitted which does not comply with these terms.
*Correspondence: Anastasis Oulas, YW5hc3Rhc2lvc29AY2luZy5hYy5jeQ==; Alexios Vlamis-Gardikas, YXZsYW1pc0B1cGF0cmFzLmdy
Disclaimer: All claims expressed in this article are solely those of the authors and do not necessarily represent those of their affiliated organizations, or those of the publisher, the editors and the reviewers. Any product that may be evaluated in this article or claim that may be made by its manufacturer is not guaranteed or endorsed by the publisher.
Research integrity at Frontiers
Learn more about the work of our research integrity team to safeguard the quality of each article we publish.