- 1Institute of Life Sciences and Resources, Department of Food Science and Biotechnology, Kyung Hee University, Yongin, South Korea
- 2Department of Molecular Science and Technology, Ajou University, Suwon, South Korea
- 3School of Energy and Chemical Engineering, Ulsan National Institute of Science and Technology (UNIST), Ulsan, South Korea
- 4School of Life Sciences, Ulsan National Institute of Science and Technology (UNIST), Ulsan, South Korea
An accurate diagnostic method for Salmonella serovars is fundamental to preventing the spread of associated diseases. A diagnostic polymerase chain reaction (PCR)-based method has proven to be an effective tool for detecting pathogenic bacteria. However, the gene markers currently used in real-time PCR to detect Salmonella serovars have low specificity and are developed for only a few serovars. Therefore, in this study, we explored the novel unique gene markers for 60 serovars that share similar antigenic formulas and show high prevalence using pangenome analysis and developed a real-time PCR to detect them. Before exploring gene markers, the 535 Salmonella genomes were evaluated, and some genomes had serovars different from the designated serovar information. Based on these analyses, serovar-specific gene markers were explored. These markers were identified as genes present in all strains of target serovar genomes but absent in strains of other serovar genomes. Serovar-specific primer pairs were designed from the gene markers, and a real-time PCR method that can distinguish between 60 of the most common Salmonella serovars in a single 96-well plate assay was developed. As a result, real-time PCR showed 100% specificity for 199 Salmonella and 29 non-Salmonella strains. Subsequently, the method developed was applied successfully to both strains with identified serovars and an unknown strain, demonstrating that real-time PCR can accurately detect serovars of strains compared with traditional serotyping methods, such as antisera agglutination. Therefore, our method enables rapid and economical Salmonella serotyping compared with the traditional serotyping method.
Introduction
The genus Salmonella, the causative agent of foodborne salmonellosis, can infect both animals and humans, leading to public health problems and economic loss (Gand M. et al., 2020a). Most Salmonella infections are caused by consuming contaminated water or food (Kasturi, 2020). Currently, Salmonella is divided into two species and six subspecies. Serotypes are further classified into more than 2,600 serovars following the White–Kauffman–Le Minor scheme, using antigenic agglutination reactions to three cell-surface antigens of somatic O, and flagellar H antigens denoted as H1 and H2 (Grimont and Weill, 2007; Yachison et al., 2017; Zhang et al., 2019; Gand M. et al., 2020a). As a reliable surveillance protocol is critical for detecting outbreaks or preventing their spread, using a differential serotyping method that identifies serogroups and serovars of Salmonella isolates from causative agents is important (Kasturi, 2020).
Traditional serotyping methods require numerous antisera, are labor-intensive, time-consuming, and complicated, and may produce ambiguous results (Hong et al., 2008; Xiong et al., 2017). Some isolates do not express antigens because of a single-nucleotide mutation in the genome, and some require multiple passes through semisolid media to enhance the flagella antigen expression (Ibrahim and Morin, 2018). As a result of these limitations, serotyping by antigenic agglutination and biochemical tests has been replaced by molecular serotyping (Zhang et al., 2019). For the rapid diagnosis or tracking of Salmonella serovars, epidemiological investigations have been conducted by molecular serotyping analysis based on pulsed-field gel electrophoresis, plasmid profiles, and polymerase chain reactions (PCRs) (Ozdemir and Acar, 2014; Gad et al., 2018; Yang et al., 2021). Notably, the PCR-based method is widely used for early diagnosis because it can diagnose a few bacteria in the specimen and can yield rapid results. However, as the PCR-based method for serotyping mainly uses markers within genes responsible for somatic and flagellar antigen expression, these genes do not detect strains that share the same antigenic formula. Thus, although PCR is reasonably rapid and inexpensive compared with conventional serotyping methods, the limiting factors of this assay are that molecular serotyping does not diagnose numerous serovars and focuses mainly on the most common serovars, such as Salmonella Typhimurium and Enteritidis (Ibrahim and Morin, 2018).
Previous studies have identified gene markers, such as fimA, hilA, invA, and ttr, that are useful in detecting Salmonella species (Laing et al., 2017; Gand M. et al., 2020b; Kreitlow et al., 2021). Moreover, for serovar identification, gene markers based on allelic variations in O and H antigen genes were used (Laing et al., 2017; Gand M. et al., 2020b). However, this gene marker cannot distinguish isolates that share the same antigenic formula. To overcome this problem, some studies have identified markers, such as STM0292, STM4200, STM4493, and STM2235 specific to Typhimurium and SEN1392 specific to Enteritidis, using comparative genomics (Akiba et al., 2011; Liu et al., 2012). These gene markers have specificity but have been screened using a limited number of genomes and cannot detect various serovars in a single reaction (Heymans et al., 2018; Ibrahim and Morin, 2018).
Advances in whole-genome sequencing technology have improved the understanding of the species and subtypes of pathogenic bacteria, and can provide information on the virulence of the underlying pathogenesis. Data obtained using whole-genome sequencing technology can be used to confirm paths of disease transmission and provide information on potential outbreaks (Ibrahim and Morin, 2018; Diep et al., 2019; Cooper et al., 2020). Therefore, whole-genome sequencing is currently used as a technique to obtain reliable and rapid serovar information. Recently, multiple in silico tools have been developed to determine Salmonella serovars from whole-genome sequence data (Zhang et al., 2015, 2019; Yoshida et al., 2016). SeqSero and Salmonella In Silico Typing Resource (SISTR), which can infer serovar predictions from analyses of somatic O and flagellar H antigens derived from whole-genome sequence data, are representative in silico analysis tools (Uelze et al., 2020). Whole-genome sequencing and in silico tools have many advantages in serotyping, for example, they reveal detailed genetic information on the characteristics of isolates and accurate serovar predictions, provided the database is sufficient (Ibrahim and Morin, 2018). However, whole-genome sequence-based method must be considered against costs associated with genome sequencing to analyze the many samples. This method also requires trained researchers with a specific skill set (Mellmann et al., 2017). To overcome the limitations of genome sequencing, primer sets for PCR targeting serovar-specific genes were recently developed. They show accuracy for serovar detection but still cannot detect a large number of serovars (Shang et al., 2021; Ye et al., 2021).
The purpose of this study is to evaluate Salmonella genomes by in silico serotyping, to select novel serovar-specific gene markers based on pangenome analysis, and to develop a real-time PCR method that can distinguish between 60 of the most common Salmonella serovars in a single 96-well plate by detecting unique serovar-specific gene markers.
Materials and Methods
In silico Serotyping
This study selected 60 serovars, which have been frequently isolated worldwide, are essential for public health, and are difficult to diagnose using traditional serotyping methods. The target serovars are as follows: Aberdeen, Agona, Albany, Anatum, Bareilly, Berta, Blockley, Braenderup, Brandenburg, Cerro, Choleraesuis, Corvallis, Derby, Dublin, Elisabethville, Enteritidis, Gallinarum, Give, Hadar, Heidelberg, I 4,[5],12:i:-, Infantis, Javiana, Kedougou, Kentucky, Kottbus, Litchfield, Livingstone, London, Manhattan, Mbandaka, Meleagridis, Menston, Minnesota, Mississippi, Montevideo, Muenchen, Muenster, Newington, Newport, Ohio, Oranienburg, Panama, Paratyphi B, Poona, Reading, Rissen, Saintpaul, Schwarzengrund, Senftenberg, Singapore, Stanley, Tennessee, Thompson, Typhi, Typhimurium, Uganda, Vinohrady, Virchow, and Weltevreden. The 535 Salmonella assembled genome sequences representing 60 serovars were obtained from the National Center for Biotechnology Information (NCBI) (Supplementary Table 1). All genomes used in this study were subjected to in silico serotyping using SeqSero2 version 1.2.1 and SISTR version 1.1.1. SeqSero used the assembled genome sequence as the input and the sequence was analyzed using Python code (Zhang et al., 2015, 2019). Also, serotyping and core-genome multilocus sequence typing (cgMLST) of whole-genome sequences were analyzed using a SISTR command-line tool.
Pangenome Analysis and Discovery of Unique Gene Markers
The pangenome was analyzed using workflow for microbial pangenomic analysis with the Anvi’o package version 6.1 (Eren et al., 2015). Briefly, the assembled genome sequences were used as input and clustered based on the similarity of the amino acid sequences by the Markov cluster algorithm and NCBI’s blastp algorithm according to the developer recommendations (Kayansamruaj et al., 2019). Then the pangenome result was visualized using anvi-display-pan code of Anvi’o, and the genomes were organized based on the pan gene cluster frequencies.
The unique gene of each serovar was obtained using a Bacterial Pan Genome Analysis (BPGA) version 1.3 (Chaudhari et al., 2016). The annotated protein in fasta-format file was used as input, and the pangenome was analyzed with the default value (cut-off: 50%). All genomes were compiled into separate local databases, which include the core-genome composed of proteins common to genomes of the target serovar, and the pangenome is composed of entire proteins of all genomes. The unique genes of each serovar were gathered by comparing the pan and core-genome databases. The extracted unique genes were aligned with 65,815,883 sequences using the Basic Local Alignment Search Tool (BLAST). To verify the unique gene markers, the unique gene of each serovar was aligned with 535 Salmonella genomes using USEARCH version 9.0 (Edgar, 2010). Afterward, serovar-specific primer pairs were designed from selected gene markers. To increase the efficiency of primer pairs, the length was 18–30 base pairs, the guanine–cytosine (GC) content was designed to be 45–60%, and the melting temperature (Tm) value was 52–58°C (Abd-Elsalam, 2003). Representativeness of newly designed primer pairs were evaluated by in silico PCR. PCR was run from a web-based in silico PCR amplification1 software using 625 Salmonella genomes (Supplementary Table 2). The genomes used in the in silico PCR come from NCBI and EnteroBase.
Cultured Bacterial Strains and DNA Extraction
The 199 Salmonella strains, 33 Salmonella species or Salmonella enterica strains, and 29 non-Salmonella strains used in this study are presented in Supplementary Table 3. All bacterial strains were grown in TSB at 37°C for 18 h under aerobic conditions. The cultured strains were collected by centrifugation at 13,600 × g for 5 min. Then, genomic DNA was extracted from the pellet using the DNeasy Blood & Tissue Kit (QIAGEN, Hilden, Germany), according to the manufacturer’s instructions. The concentration and purity of the genomic DNA extracted were measured using the MaestroNano® spectrophotometer (Maestrogen, Las Vegas, NV, United States).
Specificity and Accuracy for Developed Primer Pairs
Each 20-μl real-time PCR reaction mixture contained 500 nM of each primer pair, 10 μl of 2× Thunderbird SYBR® qPCR Mix (Toyobo, Osaka, Japan), and 20 ng of template DNA. To avoid false-negative results, an internal standard targeting the bacterial 16S rRNA gene fragment was used (Aboutalebian et al., 2021). Amplification was conducted in a 7500 Real-Time PCR System (Applied Biosystems, Foster City, CA, United States), with an initial denaturation for 2 min at 95°C, followed by 30 cycles of 95°C for 5 s and 60°C for 30 s. The melting curve was generated according to the following conditions: 95°C for 15 s, 60°C for 1 min, 95°C for 30 s, and 60°C for 15 s. The specificity of the primer pairs developed was tested against each Salmonella strain as well as each non-Salmonella reference strain. Primer pairs cross reactivity across all of the serovars were evaluated. To evaluate the accuracy, genomic DNA for each serovar were serially diluted, and then standard curves were generated in triplicate using diluted DNA from 0.0002 to 20 ng. The results obtained were analyzed using 7500 software version 2.3 (Applied Biosystems).
Evaluation of Real-Time Polymerase Chain Reaction With a Single 96-Well Plate
A real-time PCR method was developed that can distinguish between 60 of the most common Salmonella serovars in a single 96-well plate. The real-time PCR was designed so that each primer pair was run independently in a single 96-well plate (Supplementary Figure 1). Each well contained different primer pair, and the genomic DNA from a single isolate was added to each well. The serovar of strain was determined as serovar corresponding to the primer pair included in the well in which amplification occurred. The real-time PCR developed in this study was evaluated using 189 Salmonella strains whose serovars were confirmed through antisera agglutination and 33 strains whose serovars were unknown. Each strain was tested against each of the primer pairs. For serovar diagnosis of isolates, genomic DNA of the isolate was added to each well of the reaction plate containing different primer pair and 2× Thunderbird SYBR® qPCR Mix (Toyobo). Then, real-time PCR was conducted in the 7500 Real-Time PCR System (Applied Biosystems). The conditions were the same as those described in the previous “Specificity and Accuracy for Developed Primer Pairs” section.
Traditional Serotyping Using Antisera Agglutination
The antigenic formulas of strains were determined by the World Health Organization Collaborating Center for Reference and Research on Salmonella, located at the Pasteur Institute, and the serovar name was assigned by the White–Kauffmann–Le Minor scheme (Grimont and Weill, 2007). All strains were cultured in brain heart infusion (BD Difco, Sparks, MD, United States) and motility GI medium (BD Difco) to determine somatic and flagellar antigens. The somatic antigen was confirmed by the slide agglutination reaction using antisera (BD Difco). The flagellar phase was activated by the motility test and fixed by treatment with 0.6% formalin. The flagellar antigen was determined by an aggregation reaction in glass test tubes by mixing with an antisera solution (BD Difco). The serotyping results of antisera agglutination were compared with the results of the real-time PCR method.
Results and Discussion
In silico Serotyping
Whole-genome sequencing data have been used for serotype diagnosis or subtyping of Salmonella (Zhang et al., 2019; Uelze et al., 2020). SeqSero is a web-based serotyping tool that can predict many Salmonella serovars using whole-genome sequence data based on a database of Salmonella serovar determinants (Ibrahim and Morin, 2018). This tool extracts the relevant genomic regions of cell-surface antigens, such as the rfb gene cluster to determine the O antigen and the fliC and fljB genes to determine H1 and H2 antigens, from the genome assemblies or raw sequencing reads and aligns these genes to the curated database using BLAST (Zhang et al., 2015; Ibrahim and Morin, 2018). This software then determines the serovar according to the White–Kauffmann–Le Minor scheme.
In this study, 511 genomes (95.51%) were predicted as correct serovars by SeqSero, and the remaining 24 genomes (4.49%) were predicted as incorrect serovars (Supplementary Table 4). Of the 24 genomes that produced incorrect serovars, eight genomes were predicted to be serotypes inconsistent with serovar nomenclature reported to NCBI, and 13 genomes indicated two or more serotypes (Table 1). This shows that as the serotype by SeqSero is determined only by the O and H antigens, these genomes show that two or more serovars were indicated for similar serovars, such as Gallinarum or Enteritidis, Paratyphi C or Choleraesuis or Typhisuis, and Albany or Duesseldorf. Additionally, some genomes (n = 4) were determined to be partial serovars lacking O or H1 or H2-antigens, and accurate serovar information could not be extracted. The reason for this appears to be the failure to extract the serovar determinant from the assembled genomes (Zhang et al., 2015).
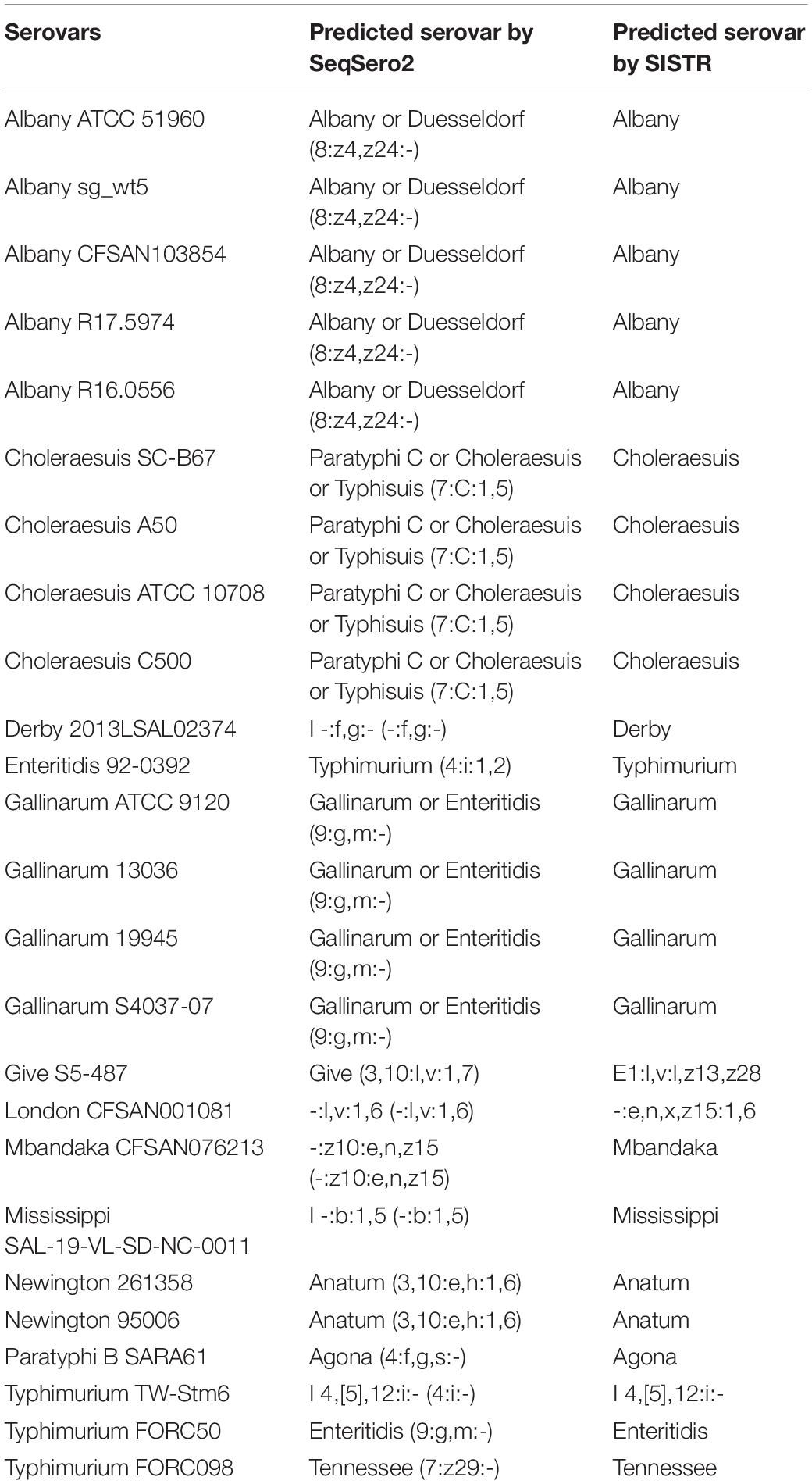
Table 1. Genomes predicted to incorrect serovar among 535 Salmonella genomes by in silico serotyping.
Salmonella In Silico Typing Resource also determines the serovar according to the White–Kauffmann–Le Minor scheme, based on their databases of Salmonella serotype determinants (wzx/wzy, fliC, and fljB alleles) (Yoshida et al., 2016). To resolve the ambiguous serovar designations resulting from antigen determination, SISTR uses the novel 330 locus cgMLST analysis, and together, these two determinants are used to provide an overall serovar prediction (Yoshida et al., 2016). As a result of SISTR analysis, 526 genomes (98.32%) were predicted as correct serovars (Supplementary Table 5), whereas nine genomes (1.68%) were predicted as incorrect serovars (Table 1).
In silico serotyping analyses of the genomes obtained from the NCBI showed 95.51 and 98.32% accuracy on SeqSero and SISTR platforms, respectively. These data are consistent with the success rates of each platform reported in previous studies (Zhang et al., 2015; Yoshida et al., 2016). However, some limitations of whole-genome sequencing also exist. Previous studies have reported that SeqSero provided two possible serovars that share the same antigenic formula but differed in the minor O antigen factor (Ibrahim and Morin, 2018). In this study, serovars such as Anatum (3,10:e,h:1,6) and Newington (Anatum var. 15+, 3,10,15:e,h:1,6) shared similar antigenic formulas, yielding only Anatum serovars. Moreover, in silico serotyping tools could not predict some genomes. These unpredicted serovars may infrequently be separated, or possibly there were some gaps in their databases (Ibrahim and Morin, 2018).
Genome Evaluation
Previous studies have reported that the NCBI has often misclassified genomes, so the genome evaluation should be conducted before using the genome obtained from the NCBI (Kim et al., 2020, 2021). Therefore, the genomes used in this study were evaluated to prevent incorrect results obtained in selecting unique serovar genes.
As a result of phylogeny based on the pangene cluster frequencies among the 535 genomes, most genomes were clustered according to serovar types (Figure 1). Serovars with similar antigenic formula types, such as Typhimurium and I 4,[5],12:i:-, were adjacent but clustered into different groups. These two serovars differ by only one flagellar antigen (1,4,[5],12:i:1,2 vs. 4,[5],12:i:-) in their antigenic formulas. However, some Enteritidis, Typhimurium, and Paratyphi B strains were clustered with different serovars. Paratyphi B SARA61 was clustered into Agona; Typhimurium TW-Stm6, FORC50, and FORC098 were clustered into I 4,[5],12:i:-, Enteritidis, and Tennessee, respectively; Enteritidis 92-0392 was clustered into Typhimurium. Most genomes were clustered with the same serovar group because of in silico serotyping analyses. This is consistent with a previous study that reported that similar or rare serotypes could produce unpredicted serovar results using the SeqSero database (Ibrahim and Morin, 2018).
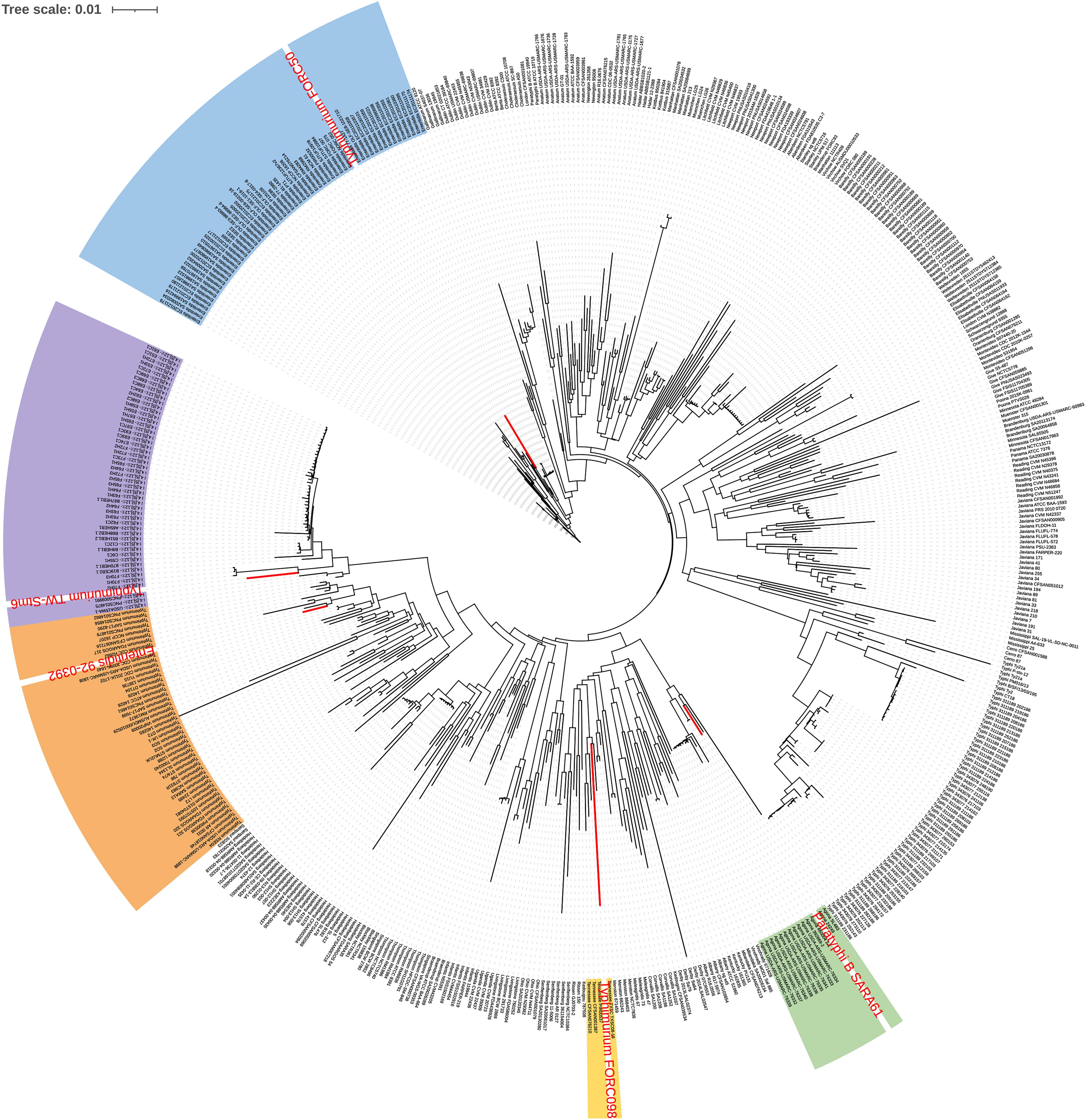
Figure 1. Pangenome distribution of the 535 Salmonella genomes. The red letters in the circular dendrogram indicates the strains predicted by the incorrect serovars. The orange, purple, blue, green, and yellow backgrounds represent Typhimurium, I 4,[5],12:i:-, Enteritidis, Agona, and Tennessee, respectively.
Identification of Serovar-Specific Gene Markers
A total of 2,440,535 genes yielded a pangenome size of 15,853 genes. The core genome comprises 1,215 genes; the accessory genome, 11,156 genes; and the unique genome, 3,482 genes. The core genomes for each serovar included from 3,065 to 4,702 genes, and unique genomes contained from 1 to 160 genes. The unique genes considered specific for each serovar were identified by BLAST analysis, and genes rarely present in other bacteria and specific to the serovar were selected. Further, a unique gene marker of each serovar was finally selected considering the GC content and sequence length suitable for the primer design. The 60 gene markers selected by the analysis were confirmed to be genes present in all target serovars and absent in other Salmonella serovars. Information on these gene markers is shown in Table 2.
The specificity of gene markers was evaluated using 535 genomes by in silico analysis. As a result, gene markers were present in the genomes of most target serovars (Figure 2). Notably, most of the gene markers shared 99–100% of the sequence identified in the target serovars, and 0–50% of the sequence identified against other serovars. In contrast, for the serovar Enteritidis, 60 genomes showed 99–100% identity, but in one genome, the Enteritidis gene marker was not found. Among them, Enteritidis 92-0392 contained the Typhimurium gene marker (100% identity) instead of the Enteritidis gene marker, and this genome was determined as Typhimurium in the pangenome analysis and in silico serotyping. For the Typhimurium gene marker, 46 genomes showed 99–100% identity, but in three genomes, the Typhimurium-specific gene marker could not be found. Typhimurium TW-Stm6, FORC50, and FORC098 showed 100% identity to I 4,[5],12:i:-, Enteritidis, and Tennessee-specific gene markers instead of the Typhimurium-specific gene marker, respectively. For serovar Paratyphi B, two genomes showed 100% identity, but in one genome, the Paratyphi B-specific gene marker could not be found. Paratyphi B SARA61 showed 100% identity to Agona gene markers instead of the Paratyphi B gene marker. As mentioned above, misclassified genomes were reclassified. Therefore, Agona, I 4,[5],12:i:-, and Tennessee had more genomes with corresponding gene markers than the number of genomes analyzed (Figure 2). In contrast, misclassified genome absent the corresponding gene markers, so some serovar had more analyzed genomes than the number of corresponding gene markers. Based on the pangenome analysis, serovar-specific primer pairs that can accurately detect Salmonella serovars were developed (Table 3). An Infantis-specific primer pair was developed in the previous study (Yang et al., 2021).
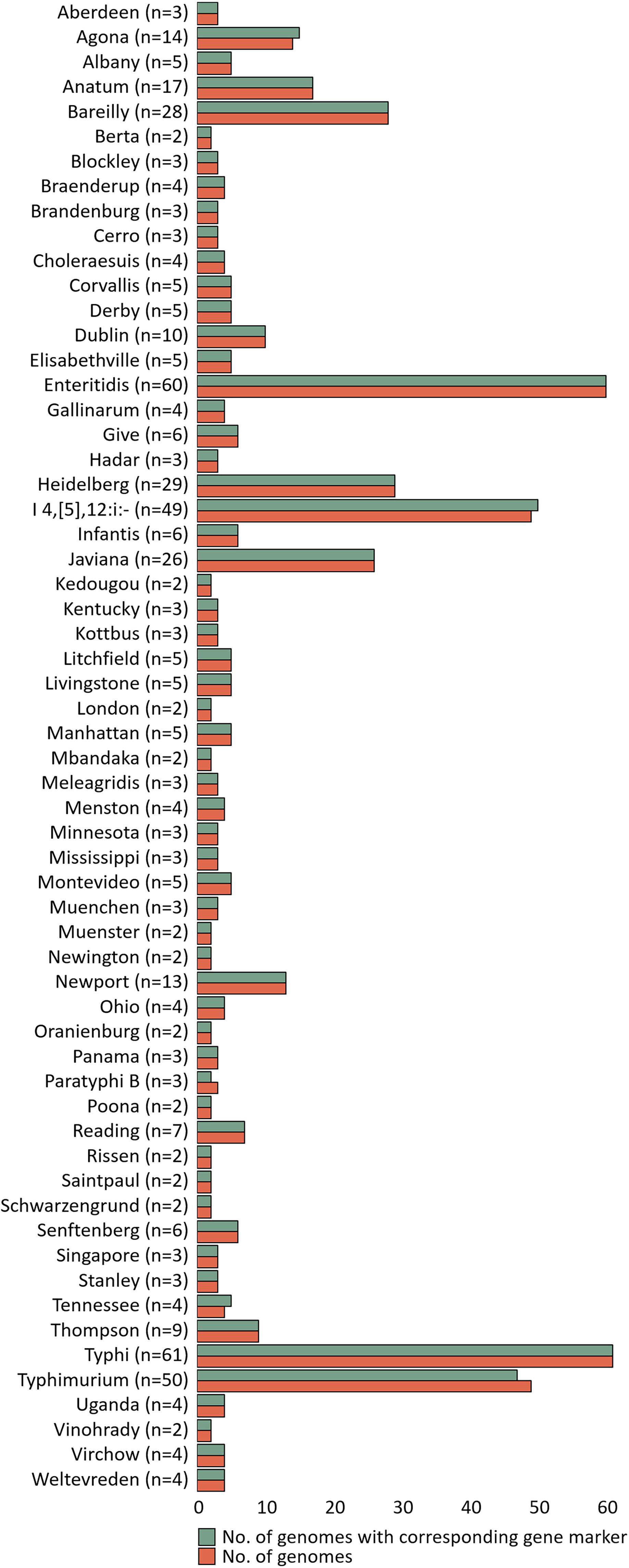
Figure 2. Evaluation of specificity unique gene markers. The figure shows the presence or absence of unique gene markers in 535 genomes. The number of analyzed genomes for each serovar is shown in the green bar, and the number of genomes with gene marker corresponding to that serovar is shown in the red bar.
In this study, our method was applied against 232 Salmonella strains. However, since the number of strains included in some serovars (e.g., Aberdeen, Berta, Cerro, Hadar, Kedougou, etc.) is rarely isolated, only a small number of strains were analyzed. In silico PCR was performed using the 625 genomes to determine whether marker genes are representative of each serovar and whether the accuracy can maintain high enough once it is applied to more strains of these serovars. All primer pairs were successfully amplified for corresponding genome sequences (Supplementary Table 2). Therefore, in silico PCR results revealed that the marker gene was representative for each serovar.
Specificity and Accuracy of the Developed Real-Time Polymerase Chain Reaction
Pangenome analysis based on the whole-genome sequence can efficiently select serovar-specific gene markers using large-scale genomes. The classification of pathogenic bacteria to their correct taxonomy using whole-genome sequencing shows reproducibility and accuracy, but is expensive and requires additional bioinformatics analysis (Ibrahim and Morin, 2018). In contrast, the PCR-based method can rapidly detect pathogenic bacteria with high accuracy and sensitivity (Abubakar et al., 2007; Chen et al., 2010). This method can cost-effectively detect many isolates with relatively simple procedures; it also has potent sensitivity and specificity (Hoorfar, 2011; Xiong et al., 2018). It is crucial to develop the primer pair with high specificity as the accuracy of these PCR-based assays depends mainly on the specific gene or primer pair. Therefore, in this study, a real-time PCR method was developed for serotyping by detecting unique gene markers obtained through pangenome analysis.
A total of 199 Salmonella strains and 29 non-Salmonella strains were used to develop a real-time PCR method that is specific and accurate. Amplification plots for the most frequently isolated six serovars worldwide are shown in Figure 3, and the result on the remaining serovars is in Supplementary Table 6. The genomic DNA across serovars yielded a detectable amplicon for the target primer pair, whereas those from all non-target Salmonella did not generate any signal (Figure 3). The Ct value ranges were 11.49–18.07 for each Salmonella serovar strain (Supplementary Table 6). Thus, primer pairs developed in this study were considered specific for the identification and detection of individual Salmonella serovars. Serial dilution was used on the genomic DNA of Salmonella reference strains to confirm the accuracy of the real-time PCR assay. All Salmonella serovar-specific primer pairs showed a linear relationship over the range of 0.002–20 ng. The slopes for the primer pairs of Bareilly, Enteritidis, I 4,[5],12:i:-, Montevideo, Typhi, and Typhimurium were −3.589, −3.395, −3.66, −3.457, −3.677, and −3.61, respectively, and the R2 values were ≥0.997 (Figure 4). The primer pairs for the remaining 54 serovars also showed that slope values were −3.19 to −3.683, and the R2 values were ≥0.996 (Supplementary Table 7). To show high efficiency, the slope value should be −3.1 to −3.9, and the R2 value ≥ 0.996 for the standard curve (Broeders et al., 2014). The slope and R2 values of all primer pairs developed in this study were within these ranges.
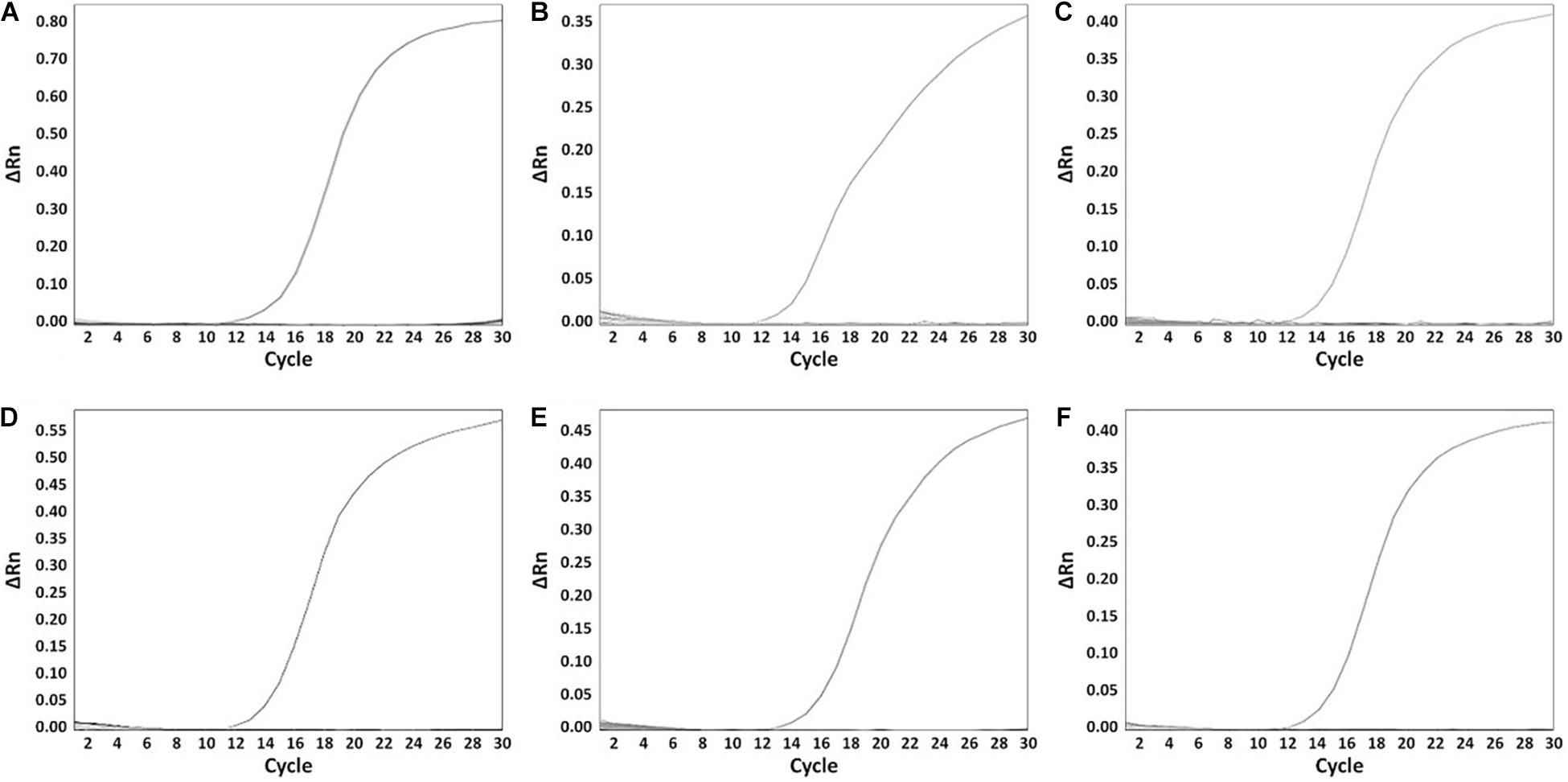
Figure 3. The specificity of serovar-specific primers. (A) Specificity of Bareilly primer pair, amplification curve: Bareilly MFDS 1007637; (B) Specificity of Enteritidis primer pair, amplification curve: Enteritidis MFDS 1010897; (C) I 4,[5],12:i:- primer pair, amplification curve: MFDS 1004858; (D) Specificity of Montevideo specific primer pair, amplification curve: CCARM 8189; (E) Specificity of Typhi primer pair, amplification curve: ATCC 33459; (F) Specificity of Typhimurium primer pair, amplification curve: ATCC 19585. ΔRn value means Rn (fluorescent signal from SYBR Green) value of an experimental response minus the Rn value of the baseline signal.
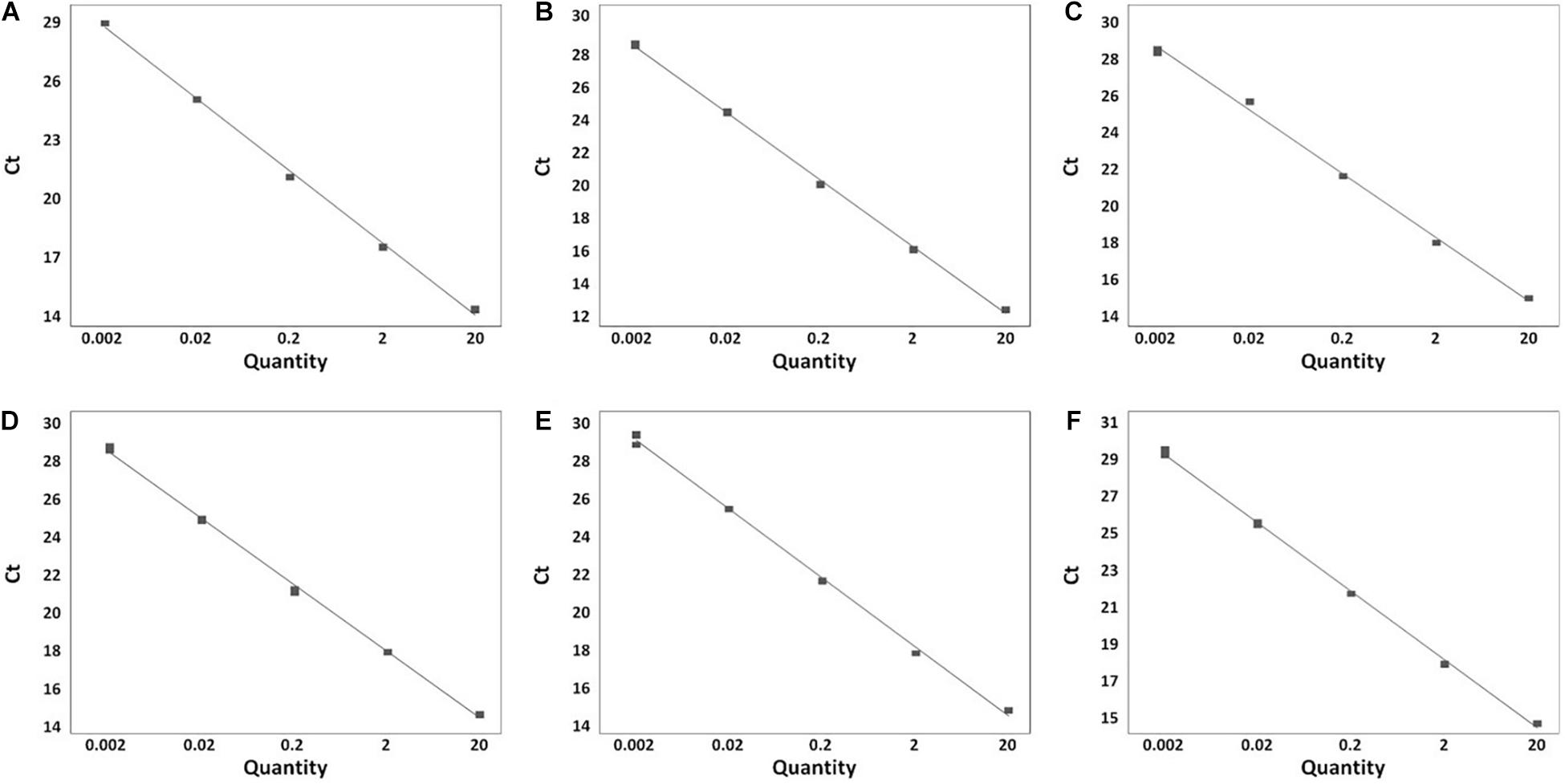
Figure 4. Real-time PCR standard curve. (A) Bareilly MFDS 1007637 standard curve (y = –3.58 x + 29.779, R2 = 0.998); (B) Enteritidis MFDS 1010897 standard curve (y = –3.39 x + 19.106, R2 = 0.999); (C) I 4,[5],12:i:- MFDS 1004858 standard curve (y = –3.66 x + 18.591, R2 = 0.997); (D) Montevideo CCARM 8189 standard curve (y = –3.45 x + 19.009, R2 = 0.999); (E) Typhi ATCC 33459 standard curve (y = –3.67 x + 21.768, R2 = 0.999); (F) Typhimurium ATCC 19585 standard curve (y = –3.61 x + 19.372, R2 = 0.999).
Evaluation of Real-Time Polymerase Chain Reaction and Validation by Traditional Serotyping
The real-time PCR method developed in this study was evaluated in a single 96-well plate using 222 Salmonella strains. Moreover, serovars of some strains were identified using the antisera agglutination method and compared with real-time PCR results. Real-time PCR competency was checked using an internal standard. Of 222 strains, 189 strains are known at the serovar level, and the remaining 33 were strains with unknown serovars. The real-time PCR result determined that the corresponding serovars were detected when amplified in a well containing a serovar-specific primer pair. All strains were amplified in wells containing a specific primer pair and internal standard primer pair, whereas no amplification was observed in the other wells (Supplementary Table 8). The internal standard primer pair was amplified in 222 Salmonella strains and 29 non-Salmonella, and the Ct value ranged from 10.03 to 14.95. Serovars of all strains were identified accurately by real-time PCR, and the result was identical to the serotyping result of the antisera agglutination method (Table 4). Anatum and Newington presented similar antigenic formulas as the result of antisera agglutination, but two serovars were distinguishable in real-time PCR. To verify our real-time PCR method, serovars were determined for 33 isolates identified down to the genus or species level obtained from the Korea Veterinary Culture Collection (KVCC) (Table 5). As a result, 33 isolates were determined as 13 different serovars, such as I 4,[5],12:i:-, Enteritidis, Blockley, Hadar, Livingstone, Mbandaka, Montevideo, Rissen, Typhimurium, Infantis, Virchow, London, and Senftenberg. Therefore, our results suggest that the 60 primer pairs and real-time PCR method developed in this study are 100% accurate in detecting Salmonella serovars. However, in this study, few strains were used in some serovars that may influence the identification of the real marker genes and detecting accuracy.
This method not only can clearly distinguish between two serovars presenting similar antigenic formulas, but also alleviates the time and cost required for traditional serotyping method. This method has the limitation of lack of replicates in a sample run of 60 serovars in 96-well plates, but may be necessary for application in the field as it is evaluated in a single plate.
Conclusion
In this study, novel serovar-specific gene markers were discovered through pangenome analysis of whole-genome sequences. The pangenome analysis could identify gene markers for 60 Salmonella serovars present in genomes of target serovars and absent in genomes of other serovars. Furthermore, in silico analyses confirmed that some genomes deposited in the public database, such as the NCBI, were incorrectly designated. The real-time PCR method, designed to detect serovar-specific gene markers using a single 96-well plate, successfully detected 222 strains, thus validating the specificity and effectiveness of the assay. Additionally, the traditional serotyping method yielded ambiguous results for strains that share similar antigenic formulas but were accurately identified as one serovar using real-time PCR. These results suggest that the efficient real-time PCR assay developed could be used as a high-throughput diagnostic tool to identify 60 serovars. The real-time PCR method developed in this study is useful in diagnosing Salmonella infections and has applications in food safety and human health.
Data Availability Statement
The original contributions presented in the study are included in the article/Supplementary Material, further inquiries can be directed to the corresponding author/s.
Author Contributions
S-MY and H-YK contributed to the conception and design of this study. S-MY, EK, SK, and DoK performed the analysis of in silico serotyping and pangenome. S-MY, EK, DaK, and H-BK performed the real-time PCR. JB and HY performed the serotyping using antisera agglutination. S-MY and EK prepared a draft manuscript. H-YK reviewed and edited the manuscript. All authors contributed to manuscript revision, read, and approved the submitted version.
Funding
This research was supported by a grant (19162MFDS042) from Ministry of Food and Drug Safety in 2021.
Conflict of Interest
The authors declare that the research was conducted in the absence of any commercial or financial relationships that could be construed as a potential conflict of interest.
Publisher’s Note
All claims expressed in this article are solely those of the authors and do not necessarily represent those of their affiliated organizations, or those of the publisher, the editors and the reviewers. Any product that may be evaluated in this article, or claim that may be made by its manufacturer, is not guaranteed or endorsed by the publisher.
Supplementary Material
The Supplementary Material for this article can be found online at: https://www.frontiersin.org/articles/10.3389/fmicb.2021.750379/full#supplementary-material
Footnotes
References
Abd-Elsalam, K. A. (2003). Bioinformatic tools and guideline for PCR primer design. Afr. J. Biotechnol. 2, 91–100. doi: 10.5897/ajb2003.000-1019
Aboutalebian, S., Ahmadikia, K., Fakhim, H., Chabavizadeh, J., Okhovat, A., Nikaeen, M., et al. (2021). Direct Detection and Identification of the Most Common Bacteria and Fungi Causing Otitis Externa by a Stepwise Multiplex PCR. Front. Cell. Infect. Microbiol. 11:644060. doi: 10.3389/fcimb.2021.644060
Abubakar, I., Irvine, L., Aldus, C. F., Wyatt, G. M., Fordham, R., Schelenz, S., et al. (2007). A systematic review of the clinical, public health and cost-effectiveness of rapid diagnostic tests for the detection and identification of bacterial intestinal pathogens in faeces and food. Health Technol. Assess. 11, 1–216. doi: 10.3310/hta11360
Akiba, M., Kusumoto, M., and Iwata, T. (2011). Rapid identification of Salmonella enterica serovars, Typhimurium, Choleraesuis, Infantis, Hadar, Enteritidis, Dublin and Gallinarum, by multiplex PCR. J. Microbiol. Methods 85, 9–15. doi: 10.1016/j.mimet.2011.02.002
Broeders, S., Huber, I., Grohmann, L., Berben, G., Taverniers, I., Mazzara, M., et al. (2014). Guidelines for validation of qualitative real-time PCR methods. Trends Food Sci. Technol. 37, 115–126. doi: 10.1016/j.tifs.2014.03.008
Chaudhari, N. M., Gupta, V. K., and Dutta, C. (2016). BPGA-an ultra-fast pan-genome analysis pipeline. Sci. Rep. 6:24373. doi: 10.1038/srep24373
Chen, J., Zhang, L., Paoli, G. C., Shi, C., Tu, S. I., and Shi, X. (2010). A real-time PCR method for the detection of Salmonella enterica from food using a target sequence identified by comparative genomic analysis. Int. J. Food Microbiol. 137, 168–174. doi: 10.1016/j.ijfoodmicro.2009.12.004
Cooper, A. L., Low, A. J., Koziol, A. G., Thomas, M. C., Leclair, D., Tamber, S., et al. (2020). Systematic Evaluation of Whole Genome Sequence-Based Predictions of Salmonella Serotype and Antimicrobial Resistance. Front. Microbiol. 11:549. doi: 10.3389/fmicb.2020.00549
Diep, B., Barretto, C., Portmann, A. C., Fournier, C., Karczmarek, A., Voets, G., et al. (2019). Salmonella Serotyping; Comparison of the Traditional Method to a Microarray-Based Method and an in silico Platform Using Whole Genome Sequencing Data. Front. Microbiol. 10:2554. doi: 10.3389/fmicb.2019.02554
Edgar, R. C. (2010). Search and clustering orders of magnitude faster than BLAST. Bioinformatics 26, 2460–2461. doi: 10.1093/bioinformatics/btq461
Eren, A. M., Esen, O. C., Quince, C., Vineis, J. H., Morrison, H. G., Sogin, M. L., et al. (2015). Anvi’o: an advanced analysis and visualization platformfor ’omics data. PeerJ. 3:e1319. doi: 10.7717/peerj.1319
Gad, A. H., Abo-Shama, U. H., Harclerode, K. K., and Fakhr, M. K. (2018). Prevalence, serotyping, molecular typing, and antimicrobial resistance of Salmonella isolated from conventional and organic retail ground poultry. Front. Microbiol. 9:2653. doi: 10.3389/fmicb.2018.02653
Gand, M., Mattheus, W., Roosens, N., Dierick, K., Marchal, K., Bertrand, S., et al. (2020a). A genoserotyping system for a fast and objective identification of Salmonella serotypes commonly isolated from poultry and pork food sectors in Belgium. Food Microbiol. 91:103534. doi: 10.1016/j.fm.2020.103534
Gand, M., Mattheus, W., Roosens, N. H. C., Dierick, K., Marchal, K., De Keersmaecker, S. C. J., et al. (2020b). A multiplex oligonucleotide ligation-PCR method for the genoserotyping of common Salmonella using a liquid bead suspension assay. Food Microbiol. 87:103394. doi: 10.1016/j.fm.2019.103394
Grimont, P., and Weill, F.-X. (2007). Antigenic formulae of the Salmonella servovars: WHO Collaborating Centre for Reference and Research on Salmonella. 9th Edition. Paris: Institut Pasteur.
Heymans, R., Vila, A., van Heerwaarden, C. A. M., Jansen, C. C. C., Castelijn, G. A. A., van der Voort, M., et al. (2018). Rapid detection and differentiation of Salmonella species, Salmonella Typhimurium and Salmonella Enteritidis by multiplex quantitative PCR. PLoS One 13:e0206316. doi: 10.1371/journal.pone.0206316
Hong, Y., Liu, T., Lee, M. D., Hofacre, C. L., Maier, M., White, D. G., et al. (2008). Rapid screening of Salmonella enterica serovars Enteritidis, Hadar, Heidelberg and Typhimurium using a serologically-correlative allelotyping PCR targeting the O and H antigen alleles. BMC Microbiol. 8:178. doi: 10.1186/1471-2180-8-178
Hoorfar, J. (2011). Rapid detection, characterization, and enumeration of foodborne pathogens. APMIS Suppl. 119, 1–24. doi: 10.1111/j.1600-0463.2011.02767.x
Ibrahim, G. M., and Morin, P. M. (2018). Salmonella serotyping using whole genome sequencing. Front. Microbiol. 9:2993. doi: 10.3389/fmicb.2018.02993
Kasturi, K. N. (2020). A real-time PCR for rapid identification of Salmonella enterica Gaminara serovar. J. Microbiol. Methods 169:105729. doi: 10.1016/j.mimet.2019.105729
Kayansamruaj, P., Soontara, C., Unajak, S., Dong, H. T., Rodkhum, C., Kondo, H., et al. (2019). Comparative genomics inferred two distinct populations of piscine pathogenic Streptococcus agalactiae, serotype Ia ST7 and serotype III ST283, in Thailand and Vietnam. Genomics 111, 1657–1667. doi: 10.1016/j.ygeno.2018.11.016
Kim, E., Kim, H. B., Yang, S. M., Kim, D., and Kim, H. Y. (2021). Real-time PCR assay for detecting Lactobacillus plantarum group using species/subspecies-specific genes identified by comparative genomics. LWT 138:110789. doi: 10.1016/j.lwt.2020.110789
Kim, E., Yang, S. M., Cho, E. J., and Kim, H. Y. (2020). Novel real-time PCR assay for Lactobacillus casei group species using comparative genomics. Food Microbiol. 90:103485. doi: 10.1016/j.fm.2020.103485
Kreitlow, A., Becker, A., Schotte, U., Malorny, B., Plötz, M., and Abdulmawjood, A. (2021). Evaluation of different target genes for the detection of Salmonella sp. by loop-mediated isothermal amplification. Lett. Appl. Microbiol. 72, 420–426. doi: 10.1111/lam.13409
Laing, C. R., Whiteside, M. D., and Gannon, V. P. J. (2017). Pan-genome analyses of the species Salmonella enterica, and identification of genomic markers predictive for species, subspecies, and serovar. Front. Microbiol. 8:1345. doi: 10.3389/fmicb.2017.01345
Liu, B., Zhou, X., Zhang, L., Liu, W., Dan, X., Shi, C., et al. (2012). Development of a novel multiplex PCR assay for the identification of Salmonella enterica Typhimurium and Enteritidis. Food Control 27, 87–93. doi: 10.1016/j.foodcont.2012.01.062
Mellmann, A., Andersen, P. S., Bletz, S., Friedrich, A. W., Kohl, T. A., Lilje, B., et al. (2017). High interlaboratory reproducibility and accuracy of next-generation-sequencing-based bacterial genotyping in a ring trial. J. Clin. Microbiol. 55, 908–913. doi: 10.1128/JCM.02242-16
Ozdemir, K., and Acar, S. (2014). Plasmid profile and pulsed-field gel electrophoresis analysis of Salmonella enterica isolates from humans in Turkey. PLoS One 9:e95976. doi: 10.1371/journal.pone.0095976
Shang, Y., Ye, Q., Wu, Q., Pang, R., Xiang, X., Wang, C., et al. (2021). PCR identification of Salmonella serovars for the E serogroup based on novel specific targets obtained by pan-genome analysis. LWT 145:110535. doi: 10.1016/j.lwt.2020.110535
Uelze, L., Borowiak, M., Deneke, C., Szabó, I., Fischer, J., Tausch, S. H., et al. (2020). Performance and accuracy of four open-source tools for in silico serotyping of Salmonella spp. Based on whole-genome short-read sequencing data. Appl. Environ. Microbiol. 86, e02265–19. doi: 10.1128/AEM.02265-19
Xiong, D., Song, L., Pan, Z., and Jiao, X. (2018). Identification and Discrimination of Salmonella enterica Serovar Gallinarum Biovars Pullorum and Gallinarum Based on a One-Step Multiplex PCR Assay. Front. Microbiol. 9:1718. doi: 10.3389/fmicb.2018.01718
Xiong, D., Song, L., Tao, J., Zheng, H., Zhou, Z., Geng, S., et al. (2017). An efficient multiplex PCR-based assay as a novel tool for accurate inter-serovar discrimination of Salmonella Enteritidis, S. Pullorum/Gallinarum and S. Dublin. Front. Microbiol. 8:420. doi: 10.3389/fmicb.2017.00420
Yachison, C. A., Yoshida, C., Robertson, J., Nash, J. H. E., Kruczkiewicz, P., Taboada, E. N., et al. (2017). The validation and implications of using whole genome sequencing as a replacement for traditional serotyping for a national Salmonella reference laboratory. Front. Microbiol. 8:1044. doi: 10.3389/fmicb.2017.01044
Yang, S. M., Baek, J., Kim, E., Kim, H. B., Ko, S., Kim, D., et al. (2021). Development of a genoserotyping method for Salmonella Infantis detection on the basis of pangenome analysis. Microorganisms 9:67. doi: 10.3390/microorganisms9010067
Ye, Q., Shang, Y., Chen, M., Pang, R., Li, F., Xiang, X., et al. (2021). Identification of Novel Sensitive and Reliable Serovar-Specific Targets for PCR Detection of Salmonella Serovars Hadar and Albany by Pan-Genome Analysis. Front. Microbiol. 12:605984. doi: 10.3389/fmicb.2021.605984
Yoshida, C. E., Kruczkiewicz, P., Laing, C. R., Lingohr, E. J., Gannon, V. P. J., Nash, J. H. E., et al. (2016). The Salmonella in silico typing resource (SISTR): an open web-accessible tool for rapidly typing and subtyping draft Salmonella genome assemblies. PLoS One 11:e0147101. doi: 10.1371/journal.pone.0147101
Zhang, S., den Bakker, H. C., Li, S., Chen, J., Dinsmore, B. A., Lane, C., et al. (2019). SeqSero2: rapid and improved Salmonella serotype determination using whole-genome sequencing data. Appl. Environ. Microbiol. 85, e01746–19. doi: 10.1128/AEM.01746-19
Keywords: Salmonella, serotyping, pangenome analysis, detection, real-time PCR, gene marker
Citation: Yang S-M, Kim E, Kim D, Kim H-B, Baek J, Ko S, Kim D, Yoon H and Kim H-Y (2021) Rapid Real-Time Polymerase Chain Reaction for Salmonella Serotyping Based on Novel Unique Gene Markers by Pangenome Analysis. Front. Microbiol. 12:750379. doi: 10.3389/fmicb.2021.750379
Received: 30 July 2021; Accepted: 02 September 2021;
Published: 21 September 2021.
Edited by:
Kwangcheol Casey Jeong, University of Florida, United StatesReviewed by:
Peixin Fan, University of Florida, United StatesChristopher Baker, University of Arkansas, United States
Copyright © 2021 Yang, Kim, Kim, Kim, Baek, Ko, Kim, Yoon and Kim. This is an open-access article distributed under the terms of the Creative Commons Attribution License (CC BY). The use, distribution or reproduction in other forums is permitted, provided the original author(s) and the copyright owner(s) are credited and that the original publication in this journal is cited, in accordance with accepted academic practice. No use, distribution or reproduction is permitted which does not comply with these terms.
*Correspondence: Hae-Yeong Kim, aHlraW1Aa2h1LmFjLmty