- Department of Soil, Plant and Food Sciences, University of Bari, Bari, Italy
Brown rot, caused by different Monilinia species, is a most economically important disease of pome and stone fruits worldwide. In Europe and in Italy, the quarantine pathogen M. fructicola was recently introduced and rapidly spread and, by competing with the main indigenous species Monilinia fructigena and Monilinia laxa, caused relevant changes in Monilinia populations. As a result, in most areas, the pathogen almost replaced M. fructigena and now coexists with M. laxa. The availability of specific and easy-of-use quantification methods is essential to study the population dynamics, and in this work, a new method for the simultaneous quantification of M. fructicola and M. laxa based on droplet digital PCR (ddPCR) technique was established. Under the optimized reaction conditions, consisting of 250/500 nM of primers/probe sets concentration, 58°C as annealing temperature and 50 PCR cycles, the duplex-ddPCR assay was 200-fold more sensitive than duplex-real-time quantitative PCR (qPCR) assay, quantifying < 1 copy μL–1 of target DNA in the PCR mixture. The results obtained with the validation assay performed on apricot and peach fruits, artificially inoculated with conidial suspensions containing different ratios of M. fructicola and M. laxa, showed a high correlation (R2 = 0.98) between the relative quantity of DNA of the two species quantified by ddPCR and qPCR and a more accurate quantification by ddPCR compared to qPCR at higher concentrations of M. fructicola. The herein described method represents a useful tool for the early detection of Monilinia spp. on stone fruits and for the improving knowledge on the epidemiology of brow rot and interactions between the two prevalent Monilinia species.
Introduction
Monilinia fructicola (G. Winter) Honey and Monilinia laxa (Aderh. & Ruhland) Honey are the main fungal pathogens causing brown rot on peach, apricot, and other stone fruit (De Cal et al., 2009), whereas Monilinia fructigena (Pers.) Honey is prevalently found on pome fruit (Lane, 2002). The disease may be responsible for severe yield losses in pre- and postharvest, with early infections appearing as blossom and twig blight or cankers and later infections causing rot on ripening fruit in the field and in postharvest.
Monilinia laxa is considered indigenous to Europe, while M. fructicola was first introduced in 2001 in France (OEPP/EPPO, 2002), reported in 2009 in Northern Italy (OEPP/EPPO, 2009; Pellegrino et al., 2009), and later also in other regions of the country (Abate et al., 2018). It is currently included in the A2 list of pathogens recommended for regulation as quarantine pests in the EPPO areas.1
Monitoring programs carried out in Mediterranean countries, such as Spain (Villarino et al., 2013), Greece (Papavasileiou et al., 2015), and South of Italy (Abate et al., 2018), over the last decade showed changes in the composition of Monilinia populations with M. fructicola almost completely displacing the indigenous species M. fructigena and coexisting with M. laxa (Papavasileiou et al., 2015; Abate et al., 2018). The shift caused by M. fructicola introduction in new geographic areas could be explained by its faster growth rate, infection, and adaptation to hot–dry conditions, associated with more abundant sporulation compared with M. laxa and M. fructigena (Byrde and Willetts, 1977; Lichtemberg et al., 2014; Villarino et al., 2016; Abate et al., 2018).
Different Monilinia species cause very similar symptoms on infected plant tissues and the identification based on morphological features is labor and time consuming and requires well-trained personal with mycological skills.
Several molecular tools based on the polymerase chain reaction (PCR) of species-specific genetic markers are available and can assist in quick and accurate identification of Monilinia species (Hughes et al., 2000; Ioos and Frey, 2000; Côté et al., 2004; Gell et al., 2007; Van Brouwershaven et al., 2010; Hu et al., 2011).
Both quantitative real-time PCR (qPCR) and droplet-digital PCR (ddPCR) are highly sensitive molecular techniques for the quantitative determination of target DNA sequences and represent a significant advancement with respect to conventional PCR (Hindson et al., 2013). In particular, qPCR is widely used for the detection and quantification of plant pathogenic fungi (Schena et al., 2004; Atallah et al., 2007). It allows the quantification of target DNA by monitoring the progression of the reaction using a variety of fluorescent reporter chemistries, also in the same reaction, thus allowing to analyze more than one target (multiplex assays) (Rahman et al., 2013). Quantitative data are obtained by comparing the quantification cycle (Cq) of each sample with those of reference samples containing known DNA amounts to generate a calibration curve (Hindson et al., 2011). qPCR-based methods have been developed for rapid and sensitive detection and quantification of single or multiple Monilinia species (Van Brouwershaven et al., 2010; Wang et al., 2018; Ortega et al., 2019). In our previous work, a TaqMan duplex-qPCR assay using species-specific primers/probe sets was developed for simultaneous detection and quantification both of M. fructicola and M. laxa (Abate et al., 2018). ddPCR, on the other hand, is considered an emerging and efficient molecular technology that provides an alternative, highly sensitive method for absolute quantification of nucleic acids, even with very low abundant targets, being not dependent from calibration (Hindson et al., 2011; Pinheiro et al., 2012). In brief, the ddPCR mixture is partitioned into thousands of water-in-oil droplets, containing zero, one, or more copies of the target nucleic acid, assorted in a random fashion, and each droplet represents an independent nano-PCR event (Yang et al., 2014). After PCR amplification, at the endpoint, the fluorescence of each droplet is individually measured and defined as positive (presence of PCR product) or negative (absence of PCR product). The absolute number of target DNA copies in a sample can then be calculated directly from the ratio of positive events to total partitions using binomial Poisson statistics (Pinheiro et al., 2012).
A wide range of research and diagnostic sectors can benefit from ddPCR applications (Huggett et al., 2013; Gutiérrez-Aguirre et al., 2015; Morcia et al., 2020). ddPCR assays achieved higher accuracy and precision than preexisting methods for the diagnosis of cancer (Olmedillas-López et al., 2017), environmental analyses, controls of food safety, detection of genetically modified organisms (BogožalecKošir et al., 2019), expression analysis (Taylor et al., 2017), and detection and quantification of human and animal parasites and pathogens (Li et al., 2018). Recently, ddPCR has been used for the diagnosis of various plant pathogens such as viruses (Zhang et al., 2017; Liu et al., 2019), phytoplasma (Bahar et al., 2018), and bacteria (Dreo et al., 2014; Dupas et al., 2019). For phytopathogenic fungi, ddPCR has been used to quantify population dynamics of Aspergillus species, including major mycotoxin-producing fungi (Palumbo et al., 2016), and recently for the measurement of fungal abundance in soil and plant tissues of Ilyonectria liriodendri, associated with black foot disease (del Pilar Martínez-Diz et al., 2020); Cadophora luteo-olivacea, associated with grapevine Petri disease and esca (Maldonado-González et al., 2020); and Tilletia spp., responsible for bunt of wheat (Liu et al., 2020; Xu et al., 2020). Compared to conventional and qPCR, ddPCR assays were reported to be more sensitive and less prone to negative interference by PCR inhibitors (e.g., polyphenols, polysaccharides, and pectin) contained in fruits and other plant parts, food, soil, or water, which can lead to false negative results or an underestimation of the pathogen abundance in the analyzed samples (Zhao et al., 2016; Dupas et al., 2019).
A deeper understanding of brown rot epidemiology and multiple interactions among M. fructicola, M. laxa, their host plants, and the environment may generate important benefits to the disease management. The aim of the present work was to develop a duplex-ddPCR assay to improve simultaneous and quantitative detection of M. fructicola and M. laxa in peach (Prunus persica) and apricot (Prunus armeniaca) fruits. The qPCR assay developed by Abate et al. (2018) was adapted and optimized to ddPCR format, and the performance of the two methods was comparatively assessed in terms of sensitivity, specificity, and replicability. The qPCR and ddPCR assays were then applied to determine relative abundance of the two Monilinia species, at different time points, in peach and apricot fruits artificially inoculated with one or both pathogens at different ratios.
Materials and Methods
Fungal Isolates and Growing Condition
Monilinia fructicola strain Mfrc123 (CBS 144850) and M. laxa strain Mlax297 (CBS 144852) used in this work were originally isolated from the fruit of Prunus avium collected from commercial orchards in South Italy (Apulia region). Both strains were stored in aqueous 10% glycerol at −80°C and revitalized on potato dextrose agar medium (PDA: infusion from 200 g peeled and sliced potatoes kept at 60°C for 1 h, 20 g dextrose, adjusted at pH 6.5, and 20 g agar Oxoid No. 3 per liter) to obtain fresh cultures. For conidia production, they were grown on PDA supplemented with 25% of commercial vegetable juice (V8®, Campbell Soup Company, Camden, NJ, United States) (PDA-V8) and incubated at 25°C ± 1°C in the darkness for the first 3 days and then exposed to a combination of two daylight (Osram, L36W/640) and two near-UV (Osram, L36/73) lamps with a 12 h light/dark photoperiod.
Fruit Inoculation
Healthy fruits of peach cv. Andros and apricot cv. Pellecchiella, with no visual defects were collected from Apulian orchards conducted according to organic farming. The stage of maturity was verified on 10 apricot and peach fruits, representative of the samples, by using a digital refractometer (Hanna Instruments, Italy) to determine the Brix degrees (peach, 11.5 ± 1.1; apricot, 17.2 ± 1.0) and, only for peaches, a digital penetrometer (8 mm tip, FM200, PCE Italia srl, Italy) to measure the flesh firmness (2.9 ± 0.5 kg/0.5 cm2).
Conidia suspensions of M. fructicola and M. laxa obtained in water added with 0.01% of Tween 20 (Sigma-Aldrich, St. Louis, MO, United States) from 10-day-old cultures were filtered through a layer of Miracloth (Calbiochem, San Diego, CA, United States) to remove mycelium fragments and adjusted to 1–2 × 106 conidia ml–1 by using a hemocytometer. The vitality of conidia was assessed, evaluating the conidia germination on PDA disks after 24 h of incubation at 24°C ± 1°C in darkness.
All fruits were decontaminated by immersion in 2% sodium hypochlorite for 3 min, washed twice with sterilized distilled water, and air dried at room temperature before use.
To evaluate the within-host competition between the two Monilinia species, fruits were wounded by a sterile needle (5 mm deep) and inoculated with mixtures of conidia suspensions in different M. fructicola/M. laxa ratios (100:0, 90:10, 50:50, 10:90, and 0:100). Aliquots of 25 μL (apricot, 2 × 106 conidia ml–1) or 50 μL (peach, 106 conidia ml–1) of each mixture were spotted onto four and six inoculation points of each wounded apricot and peach fruit, respectively. Fruits were then placed on decontaminated Nestipacks (Nespak, Massa Lombarda, Italy) and incubated in a moist chamber at 25°C ± 1°C in darkness. Three fruits for each of three independent replications (in total nine replicate fruits) were used for each combination of fruit and conidia ratios. Fruits inoculated with sterile distilled water with 0.01% of Tween 20 were used as negative control. At 24, 48, and 72 h postinoculation (hpi), the diameters (mm) of rotted areas were recorded. For PCR analyses, at each time point, the areas around the inoculation points were collected from all fruits of each replication with the help of a sterile corkborer (5 mm deep and 10 mm diameter), put together in a tube, immediately frozen with liquid nitrogen, and stored at −80°C until further processing.
DNA Extraction
Genomic DNA was extracted from 3-day-old mycelium from pure cultures of the two Monilinia strains grown at 25°C ± 1°C on cellophane disks overlaid on PDA according to De Miccolis Angelini et al. (2010). For apricot and peach fruits, a modified cetyltrimethyl ammonium bromide (CTAB) protocol for rapid DNA extraction from plant tissues (Li et al., 2008) was used. Briefly, about 2.5 g of fruit was placed into extraction bags (Bioreba, Reinach, Switzerland) with a plastic intermediate layer, homogenized in 10 ml of CTAB extraction buffer [100 mM Tris–Cl, pH 8.0; 1.4 M NaCl; 20 mM ethylenediaminetetraacetic acid (EDTA), pH 8.0; 2% cetyltrimethylammonium bromide (w/v)] using the semiautomated Homex 6 apparatus (Bioreba). An aliquot of 1 ml of each homogenized sample was then transferred into a new tube and incubated at 65°C ± 1°C for 30 min. After extraction with 1 vol of cold chloroform/isoamyl alcohol (24:1, v/v) solution, clear supernatant was precipitated with 0.7 vol of isopropanol at −20°C per 30 min. The tube was then centrifuged at 14,000 rpm for 25 min at 4°C, and the pellet was washed with 500 μL of cold 70% ethanol, air-dried, and dissolved in 50 μL of ultrapure water. DNA quality and concentration were assessed using NanoDrop 2000 spectrophotometer (Thermo Fischer Scientific Inc., Wilmington, DE) and Qubit 2.0 fluorometer (Life Technologies Ltd., Paisley, United Kingdom) with dsDNA BR Assay kit (Thermo Fisher Scientific Inc., Wilmington, DE, United States).
Optimization of the Droplet Digital PCR Assay and Comparison With the Quantitative PCR Assay
The same primers/TaqMan probe sets targeting M. fructicola and M. laxa were used in both qPCR and ddPCR assays (Table 1; Abate et al., 2018). All primers and probes were custom synthesized and high-performance liquid chromatography (HPLC) purified by external service (Macrogen, Seoul, South Korea).
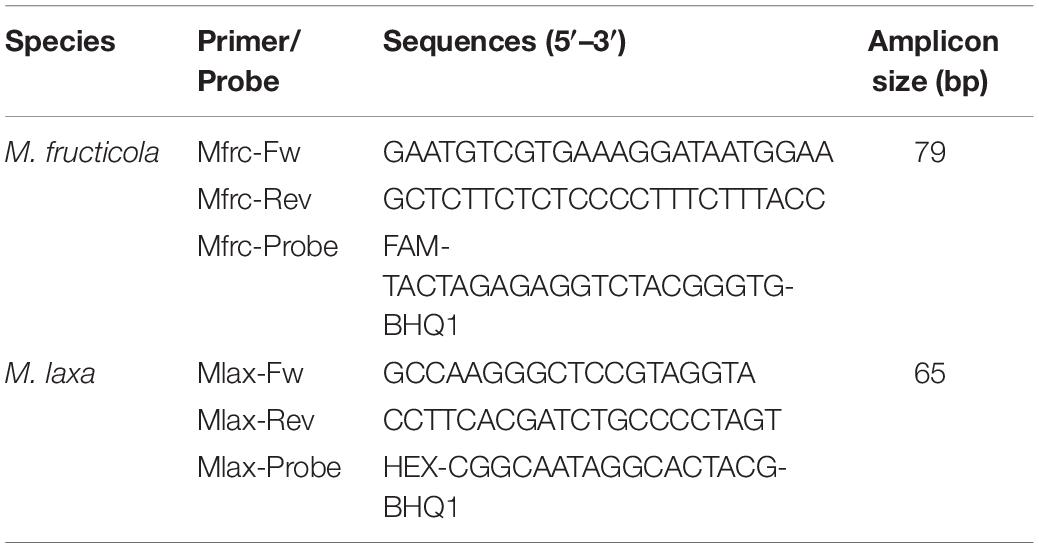
Table 1. Primers/TaqMan probe sets (Abate et al., 2018).
ddPCR was performed on QX200 Droplet Digital PCR System (Bio-Rad, Hercules, CA, United States), according to the manufacturer’s instructions. To optimize the ddPCR conditions, two primers/probe concentrations (240/160 nM and 500/250 nM), a thermal gradient with annealing temperature ranging from 56 to 62°C, and different numbers of PCR cycles were tested on samples containing 25, 2.5, and 0.25 ng of M. fructicola and M. laxa DNA, in simplex or duplex assays.
ddPCR reaction mixture contained 1 × ddPCR™ Supermix for probes (No dUTP) (Bio-Rad), primers and probes labeled with 6′FAM/BHQ-1 (M. fructicola) or 6′HEX/BHQ-1 (M. laxa), 2 μL of DNA template, and ultrapure water up to 22 μL. A volume of 20 μL of the total mixture was used to generate droplets with the automated Droplet Generator in an eight-channel DG8 cartridge and cartridge holder with 70 μL of Droplet Generation Oil for probes (Bio-Rad). A volume of 40 μL of the generated emulsion was carefully transferred into a 96-well PCR plate (Bio-Rad), heat sealed with pierceable foil using a PX1™ PCR plate sealer (Bio-Rad), and amplified in a T100™ Thermal Cycler (Bio-Rad). PCR amplification was performed with the following cycling parameters: initial denaturation at 95°C for 10 min, followed by 40 or 50 cycles (at a temperature rate of 2°C s–1) of denaturation at 94°C for 30 s and a combined annealing/extension step at 56–62°C for 1 min, and a final step at 98°C for 10 min, ending at 4°C. After amplification, the plate was directly transferred to the Droplet Reader, and the QuantaSoft™ software (version 1.7.4, Bio-Rad) was used for data acquisition and data analysis. For each experiment, a fluorescence amplitude threshold line was manually set up to discriminate positive droplets, with higher fluorescent signals, and negative droplets, with lower fluorescent signals, the latter considered as background, in accordance with negative controls. ddPCR reactions with fewer than 10,000 generated droplets were excluded from the analysis, and a reaction was considered positive if more than two positive droplets were counted. Poisson statistics was used to calculate the absolute copy number concentration of target DNAs in each sample. All the experiments were carried out in triplicate.
The specificity of the ddPCR assay was verified testing DNA from a panel of fungal species commonly associated to stone fruits, i.e., Alternaria sp., Aspergillus niger, Botrytis cinerea, Cladosporium sp., Colletotrichum sp., Fusarium sp., Monilinia fructigena, Monilinia polystroma, Mucor sp., Penicillium rubens, Penicillium expansum, Phomopsis amygdali, Sclerotinia sclerotiorum, Trichoderma sp., and Wilsonomyces carpophilus. The analytical sensitivity for both M. fructicola and M. laxa was assessed testing 10-fold serial dilutions of fungal DNA (from 25 to 0.05 pg). The linear regression of the ddPCR assay was determined by plotting the log10 of known DNA amounts against log10 of the number of DNA copies μL–1 quantified. Negative controls (NC, healthy peach, or apricot fruit DNAs) and no template control (NTC, ultrapure water) were always included in the experiments. To evaluate the reproducibility of the ddPCR assays, triplicate experiments were performed as independent replicates using DNA samples from fruit spiked with serial dilutions of fungal DNA (25, 2.5, and 0.25 ng) of M. fructicola and M. laxa.
ddPCR was compared with qPCR. The qPCR amplifications were performed in a CFX96™ Real-Time PCR Detection System Thermal Cycler (Bio-Rad), according to Abate et al. (2018). The amplification mixture consisted of 1 × Sso Advanced™ Universal Probes Supermix (Bio-Rad), 240 nM of each primer and 160 nM of each probe, 2 μL of DNA template, and ultrapure water up to 12.5 μL. Cycling conditions were 95°C for 3 min, followed by 30 cycles of 95°C for 10 s and 64°C for 30 s. Calibration curves were constructed for 10-fold serial dilutions of DNA (from 25 to 0.05 ng) of M. fructicola and M. laxa. Appropriate controls (i.e., NC and NTC) were included in each run. Every sample was measured in replicates. The Cq values, efficiency (E) of the reaction, coefficient of determination (R2), and slope were calculated using the CFX Manager™ software (version 3.1, Bio-Rad Laboratories, RRID: SCR_017251).
The performances of optimize ddPCR and qPCR assays in detecting M. fructicola and M. laxa in fruit samples were compared. In detail, the same DNA extracts from fruits artificially inoculated with different ratios of M. fructicola and M. laxa conidia were used as templates in the two PCR assays, and fungal quantities measured as amount (qPCR) or copy numbers (ddPCR) of each target DNA (M. fructicola and M. laxa) were used to estimate the percent proportion of the two fungi in the analyzed sample. For both ddPCR and qPCR, each sample was amplified in duplicate in the same PCR run.
Statistical Analysis
The data of fruit lesion diameters were analyzed by ANOVA followed by Tukey’s honestly significant different (HSD) test at the significance levels p ≤ 0.05 and p ≤ 0.01, using CoStat-software (CoHort Software, Monterey, CA, United States). Repeatability and reproducibility of ddPCR and qPCR were evaluated by measuring the intra- and interassay coefficients of variation (CVs).
The ratios of M. fructicola and M. laxa quantified by ddPCR were compared with those obtained by qPCR by using χ2-test. To compare the two PCR assays, Pearson correlation, linear regression, and the related probability value for each fungal species (M. fructicola and M. laxa), fruits (apricot and peach), and sampling times (24, 48, and 72 hpi) were calculated in GraphPad version 6.01 software (La Jolla, CA, United States, RRID: SCR_000306).
Results
Optimization of the Duplex-Droplet Digital PCR Assay
The ddPCR assay was initially set up to identify the optimal primers and probe concentrations, annealing temperature, and cycle number. Figure 1 shows the one-dimensional plots of fluorescence amplitudes at FAM channel, used for M. fructicola-specific detection, and at the HEX channel, used for M. laxa-specific detection. Droplet separation in the FAM channel was visibly better than in the HEX channel. Almost complete saturation of positive droplets was always obtained at 25 ng of target DNA. For both the primers/probe sets, increased concentrations up to 500/250 nM compared to those used in qPCR (240/160 nM) resulted in higher fluorescence amplitude and better separation between positive and negative droplets, which was clearly visible for M. fructicola but not M. laxa (Figure 1A). A further increase in concentration of primers up to 900 nM, as recommended by suppliers (Droplet Digital™ PCR Applications Guide), did not improve the droplet patterns in the ddPCR assay and DNA quantification (Supplementary Table 1). To determine the optimal annealing temperature in separating positive and negative droplets, four different annealing temperatures in thermal gradient, including 56, 58, 60, and 62°C, were compared. The best droplet separation for both targets was observed at the lowest tested temperatures, and therefore, 58°C was identified as the most suitable annealing temperature, since it presented slightly less droplet rain between positive and negative droplets compared to 56°C (Figure 1B). Different numbers of PCR cycles were also tested to improve cluster separation for M. laxa quantification. An increase in cycle numbers up to 50 cycles yielded higher fluorescence values than 40 cycles and always largest differences in fluorescence signals between positive and negative droplets without inducing false positive results, since no positive droplets were observed for negative controls (NC and NTC) (Figure 1C). Similar results were obtained using different amounts of DNA targets (2.5 and 0.25 ng).
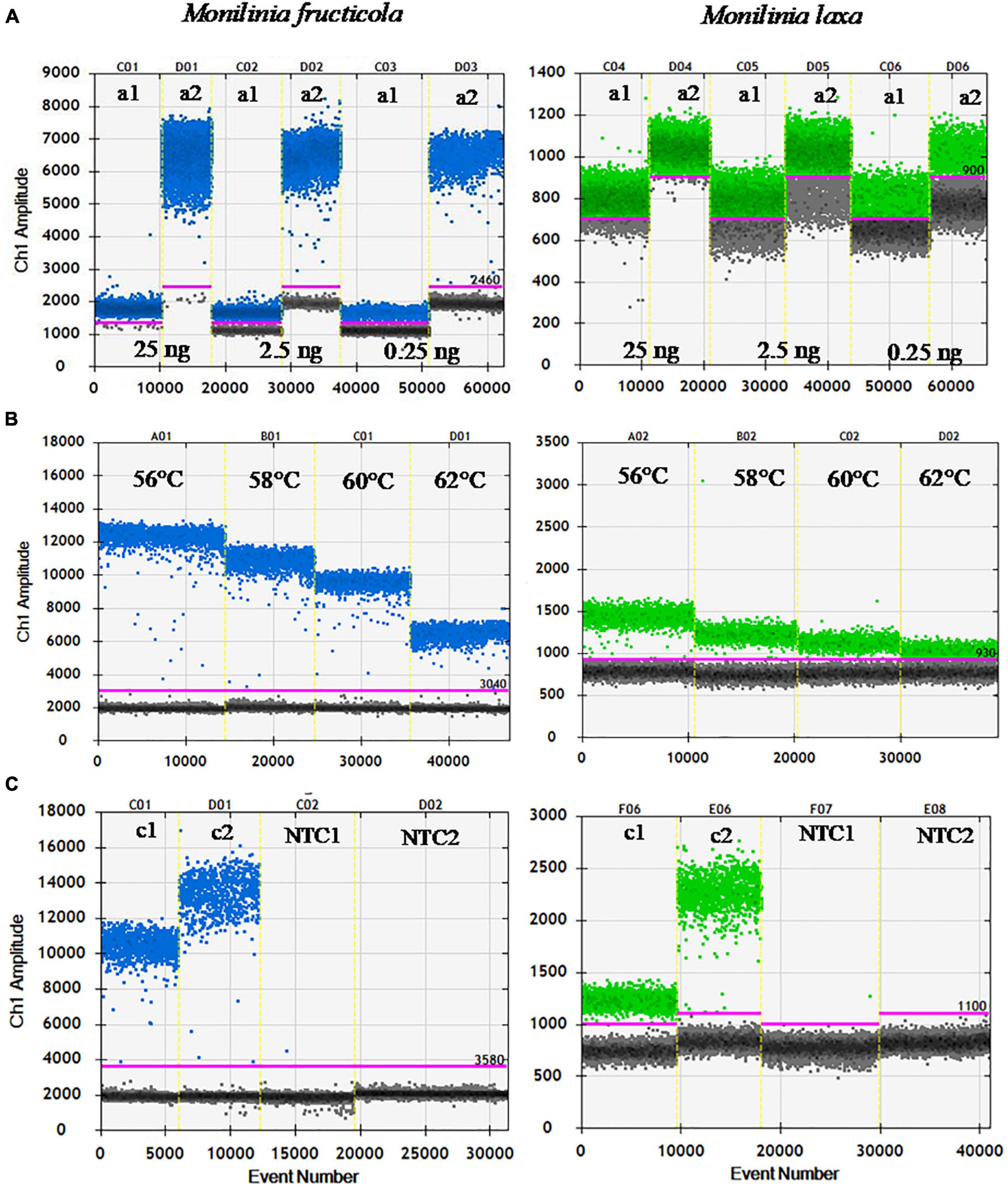
Figure 1. Optimization of ddPCR parameters. (A) Primers/probe concentration [240/160 nM (a1) and 500/250 nM (a2)], using 25, 2.5, and 0.25 ng of M. fructicola (Mfrc) and M. laxa (Mlax) DNAs, at 62°C of annealing temperature, and 40 PCR cycles. (B) Annealing temperatures (56, 58, 60, and 62°C) using 2.5 ng of Mfrc and Mlax DNA, 500/250 nM of primers/probe concentration, and 40 PCR cycles. (C) PCR cycles number [40 (c1) and 50 (c2)] using 2.5 ng of target DNA, 500/250 nM of primers/probe concentration and 58°C as annealing temperature. NTC, no template control. Blue and green dots represent the positive droplets, above the pink horizontal threshold, for ddPCR with FAM (Mfrc) and HEX (Mlax) probes, respectively. Gray dots represent the negative droplets.
Therefore, the best results for ddPCR were obtained by the combination of 500/250 nM primers/probe concentration, an annealing temperature of 58°C, and 50 PCR cycles. These conditions were used in subsequent ddPCR experiments.
To exclude cross-reactions among primers, probe sets, and the targeted DNAs in duplex ddPCR assay, the optimized protocol was validated using templates containing M. fructicola and M. laxa DNAs (25–0.25 ng), in both singleplex and duplex formats, with no significant differences in cluster patterns and in the number of DNA copies detected (data not shown). Detection and quantification of fungal DNAs were successful and produced similar results when 2 μL of DNA extract from either peach or apricot healthy fruit was added to the reaction mixture (Supplementary Table 1).
Performance of the Duplex-Droplet Digital PCR Assay and Comparison With Quantitative PCR Assays
As to duplex-ddPCR specificity, only samples containing DNA from M. fructicola and/or M. laxa proved positive, while no positive fluorescence signals were detected when using DNAs from non-target Monilinia species (e.g., M. fructigena and M. polystroma) and other pathogenic and non-pathogenic microorganisms commonly associated with stone fruits.
The performance of duplex-ddPCR was compared with qPCR for analytical sensitivity, linearity, and dynamic range. Quantitative linearity of both methods was assessed by quantification of serial dilutions (25 ng–0.05 pg) of M. fructicola and M. laxa DNAs. The sensitivity of detection of qPCR was 0.05 ng for both fungal species. In ddPCR, the lowest detectable DNA amount with reliable positive results for both targets was 0.25 pg, corresponding to 0.34 copies μL–1 for M. fructicola and 0.44 copies μL–1 for M. laxa in the PCR mix. Thus, compared to qPCR, ddPCR increased analytical sensitivity by 200-fold. A linear regression model was used to compare for both Monilinia species the log10-transformed copy numbers (c) of DNA measured by ddPCR against the corresponding log10-transformed DNA amounts (Figure 2A). For both pathogens, droplets were positively saturated in samples containing 25 ng of target DNA, making the Poisson algorithm invalid and causing a loss of linearity, while the dynamic range of quantification was from 2.5 ng to 0.25 pg with R2 values of 0.997 and 0.999 (p < 0.0001) for M. fructicola and M. laxa, respectively. The qPCR assay exhibited a good linearity (R2 = 0.994 for M. fructicola and R2 = 0.998 for M. laxa, p < 0.0001) over the dynamic range from 25 to 0.05 ng. The slopes were −3.22 and −3.37, equivalent to an average PCR efficiency of 104.2 and 99.9% for M. fructicola and M. laxa, respectively (Figure 2B).
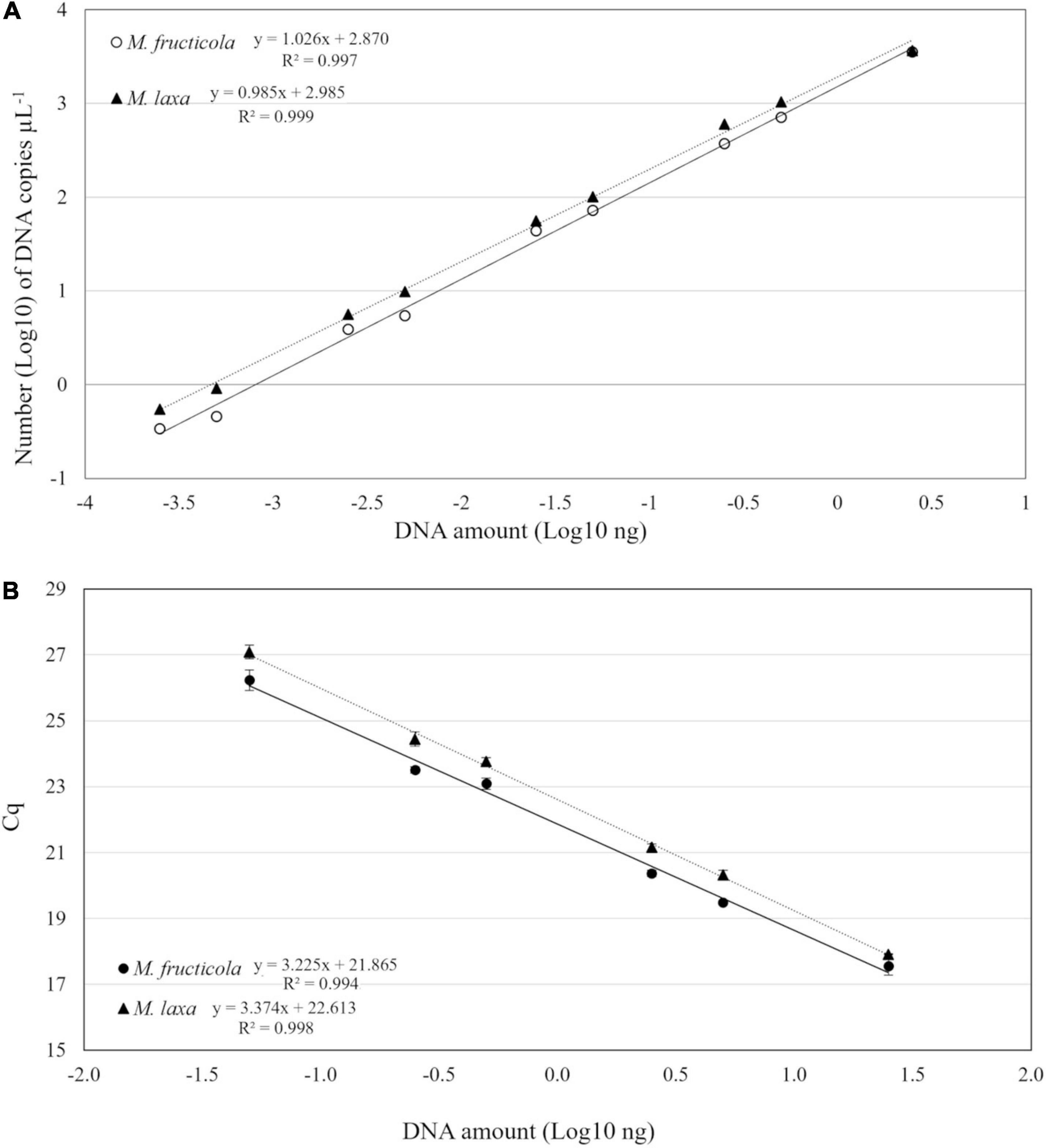
Figure 2. Linear regression of droplet digital PCR (ddPCR) copy numbers (A) and real-time PCR assay Cq values (B) vs. genomic DNA of Monilinia fructicola and Monilinia laxa.
To evaluate ddPCR repeatability and reproducibility, templates containing different amounts of fungal target DNA were tested in triplicate in one run and in three independent experiments. The intra-assay CV was included between 0.01 and 0.10, for target DNA templates of both targets ranging from 0.5 ng to 25 pg, while CV values increased (0.14–0.21) when extreme, highest (2.5 ng) or lowest (5 pg) DNA quantities were used. The interassay CV ranged between 0.06 and 0.09 for M. fructicola and between 0.07 and 0.11 for M. laxa.
Quantification of M. fructicola and M. laxa in Artificially Inoculated Apricot and Peach Fruits
At 8 hpi, the percentages of germinated conidia on PDA were 15% (M. fructicola) and 20% (M. laxa) with the length of the germ tube of 3.42 ± 0.16 μm (M. fructicola) and 4.14 ± 0.25 μm (M. laxa). At 24 hpi, all conidia germinated on PDA disks without differences among the two species. At that time, no rotting areas were observed on inoculated apricot and peach fruits. In the subsequent assessments, lesions expanded faster on apricot compared to peach fruits, likely due to higher degree of fruit ripeness in apricot compared to peach. In details, at 48 hpi, the lesion ranged from 9.8 to 15.3 mm in diameter on apricot fruits and from 5.3 to 14.2 mm on peach fruits; at 72 hpi, it ranged from 42.2 to 44.6 mm on apricot and from 9.8 to 20.8 mm on peach fruits. At 48 hpi, fruits of apricot and peach inoculated with M. fructicola alone or coinoculated with M. laxa in the Mfrc/Mlax ratios of 90:10 and 50:50 exhibited a diameter of lesions statistically (p ≤ 0.01) smaller than fruits inoculated with M. laxa alone or with a large abundance of M. laxa (Mfrc/Mlax 10:90). At 72 hpi, the diameter of lesions on peach fruits inoculated with M. fructicola were statistically (p ≤ 0.01) lower than that caused by M. laxa inoculated alone or coinoculated in different ratios with M. fructicola, while no statistical difference was observed on apricot (Table 2 and Figure 3).
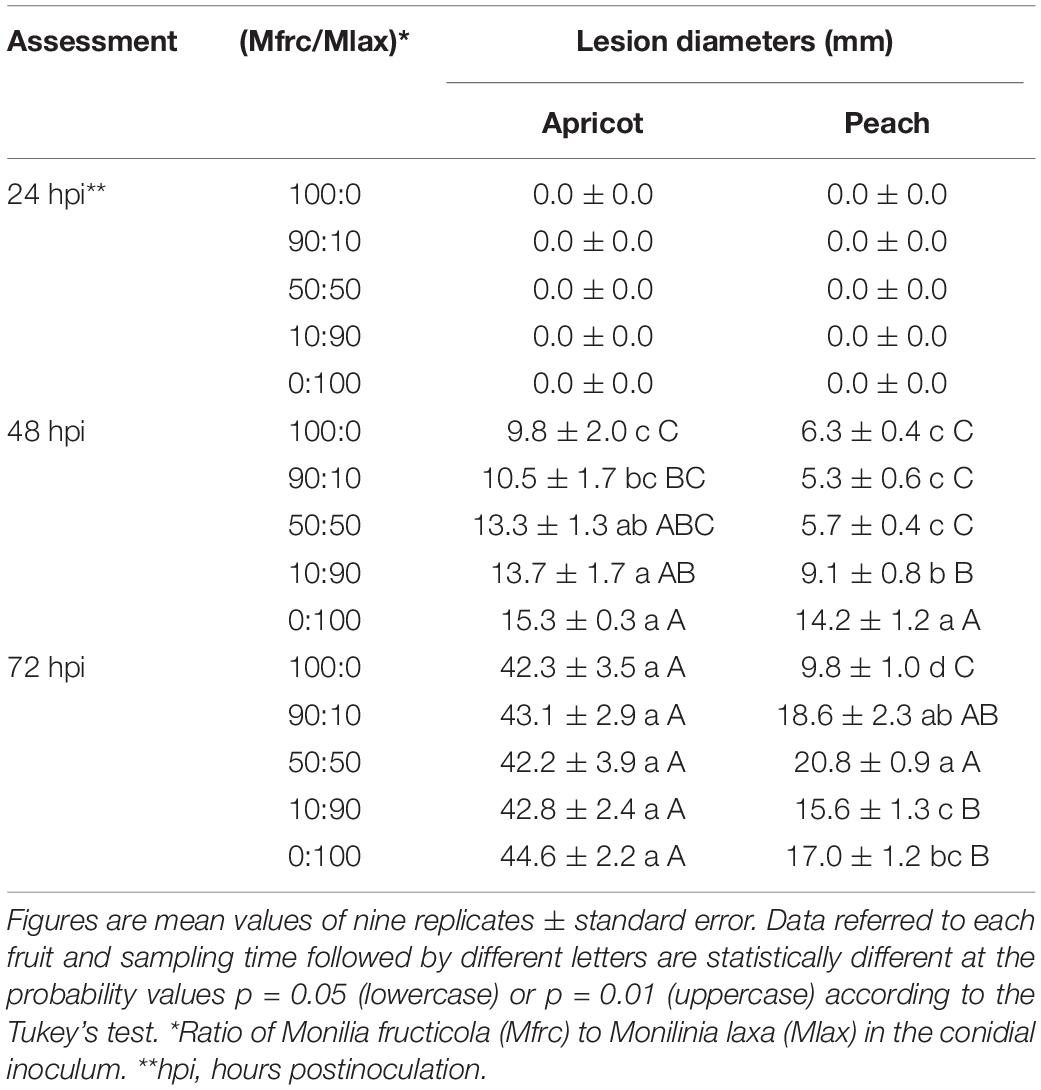
Table 2. Lesion diameters on apricot and peach fruits artificially inoculated with different M. fructicola and M. laxa conidial suspension ratios.
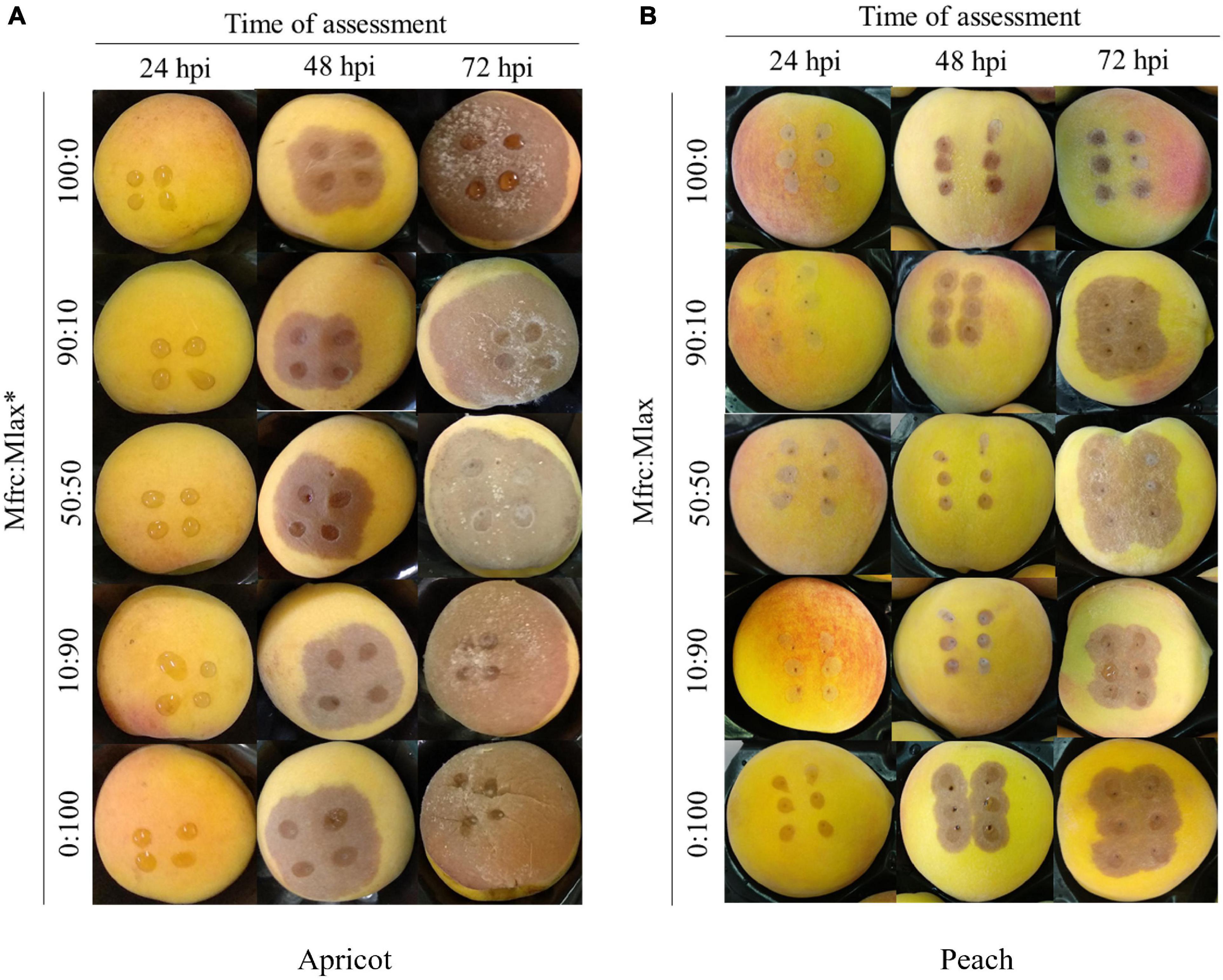
Figure 3. Apricot (A) and peach (B) fruits artificially inoculated with different Monilinia fructicola (Mfrc) and M. laxa (Mlax) conidial suspension ratios (*).
At each time points, DNA extracted from fruit infected areas were analyzed using both ddPCR and qPCR, and the quantitative results obtained by the two methods were compared (Table 3). M. fructicola and M. laxa were detectable by both the assays in all fruit samples inoculated with each of the two Monilinia species alone or together at various ratios and different time points corresponding to different levels of fruit infection and colonization. When M. fructicola or M. laxa were inoculated alone (Mfrc/Mlax 100:0 or 0:100), no positive reaction with the no-target species was confirmed, strengthening the species specificity of the assays.
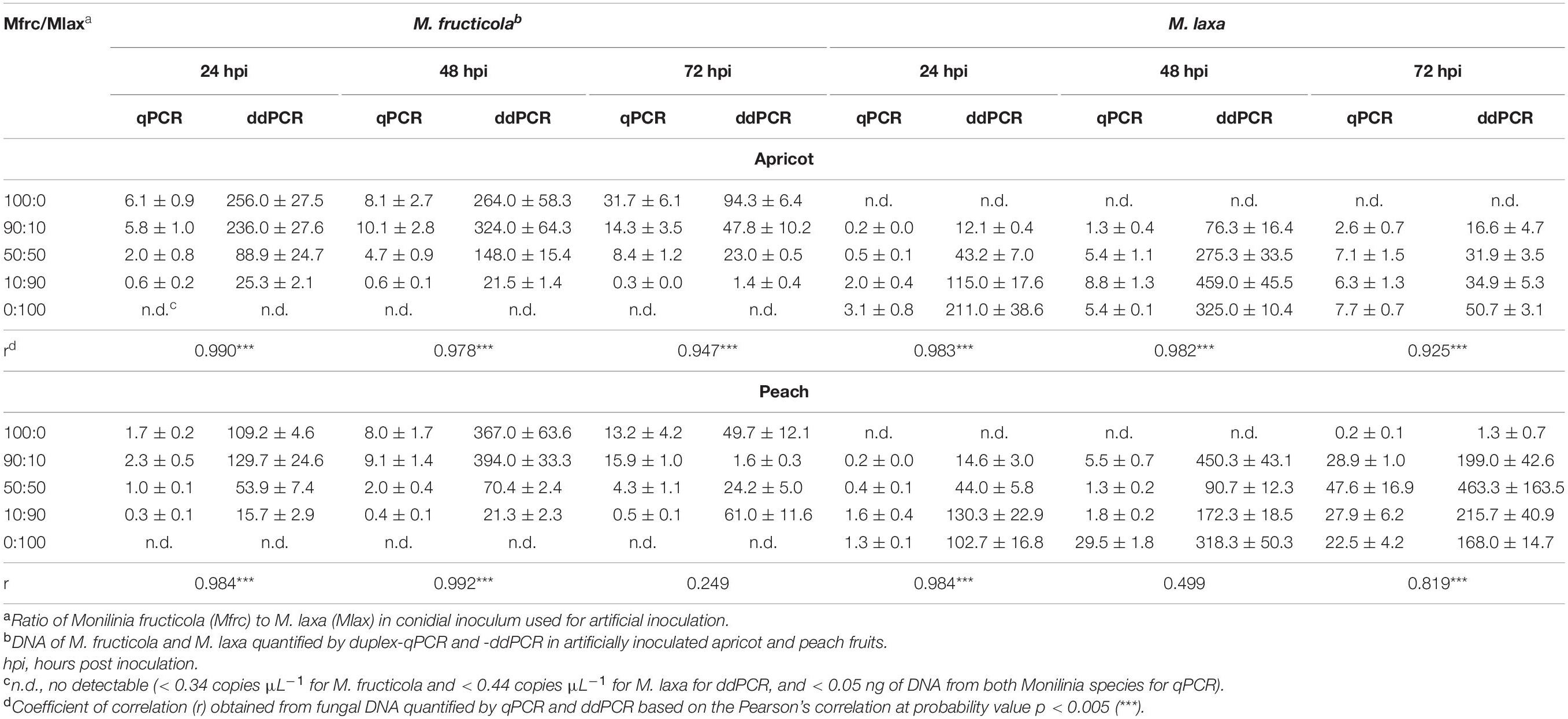
Table 3. Comparison among quantity of Monilinia fructicola and M. laxa DNAs in duplex-qPCR (ng) and duplex-ddPCR (copies μL–1) assays on artificially inoculated apricot and peach fruits.
The correlation values between the ddPCR and qPCR quantification data for the two pathogens (Table 3) were significant (p < 0.005) with r ≥ 0.978 at 24 and 48 hpi, and r ≥ 0.819 at 72 hpi, with only few exceptions (M. fructicola at 72 hpi and M. laxa at 48 hpi on peach). The CV mean values among replicates of quantification data were 0.32 (ranging from 0.03 to 0.71) ng for qPCR and 0.26 (ranging from 0.07 to 0.61) copies μL–1 for ddPCR.
On both apricot and peach fruit, and at all the sampling times (24, 48, and 72 hpi), linear regression analysis indicated significant accordance between ddPCR and qPCR based on Pearson’s correlation (R2 = 0.981 and p < 0.0001) for the estimation of M. fructicola to M. laxa DNA ratios in infected fruits (Figure 4).
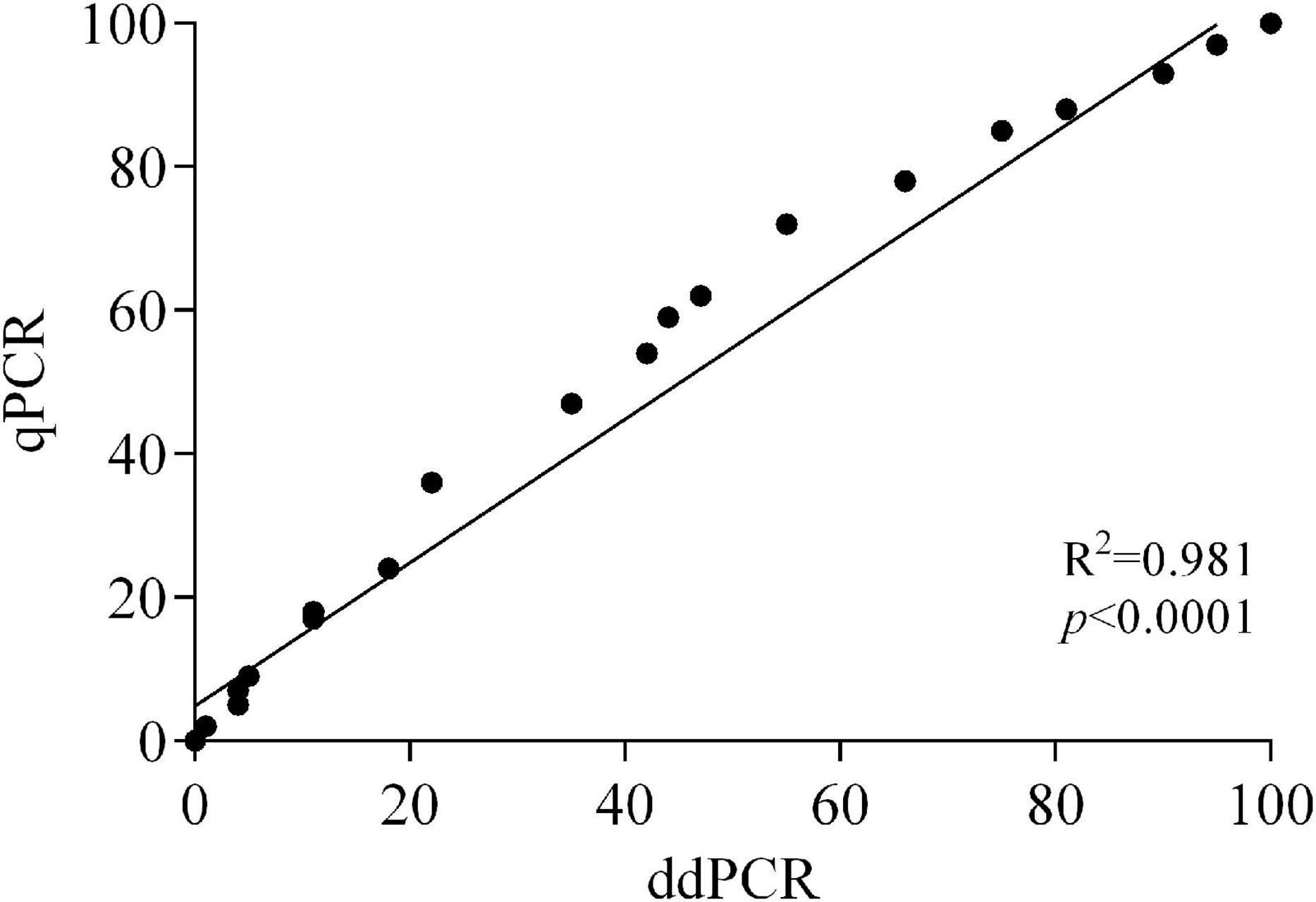
Figure 4. Correlation between ddPCR and qPCR data of Monilinia fructicola vs. Monilinia laxa ratios in artificially inoculated fruits of apricot and peach.
According to the chi-square test, statistically significant differences (p ≤ 0.05) between data of M. fructicola to M. laxa ratios determined by ddPCR and qPCR were observed at 24 and 48 hpi (50:50 ratio) and at 48 and 72 hpi (90:10 ratio) on both fruits and at 72 hpi (50:50 ratio) only on apricot (Table 4). qPCR slightly overestimated (up to 17%) M. fructicola compared to M. laxa in all the coinoculated fruit samples, as a possible consequence of the efficiency of M. fructicola quantification that was 103.0% (apricot) and 105.4% (peach), while that for M. laxa was 100.0% (apricot) and 96.9% (peach). At 24 hpi, the estimated proportions of fungal DNAs showed higher percentages of M. fructicola (up to 16%) in the Mfrc/Mlax ratios compared to those in the conidial inoculum used for coinoculations of the two species on both apricot and peach fruits. An increase in the M. laxa DNA proportion was generally recorded starting from 48 hpi on both fruits, more markedly on peach (up to 43%) than on apricot (up to 31%). On apricot fruits, the DNA ratios between the two Monilinia species at 72 hpi remained more stable, while on peach, a further relative increase in M. laxa DNA proportion was observed (up to 39%).
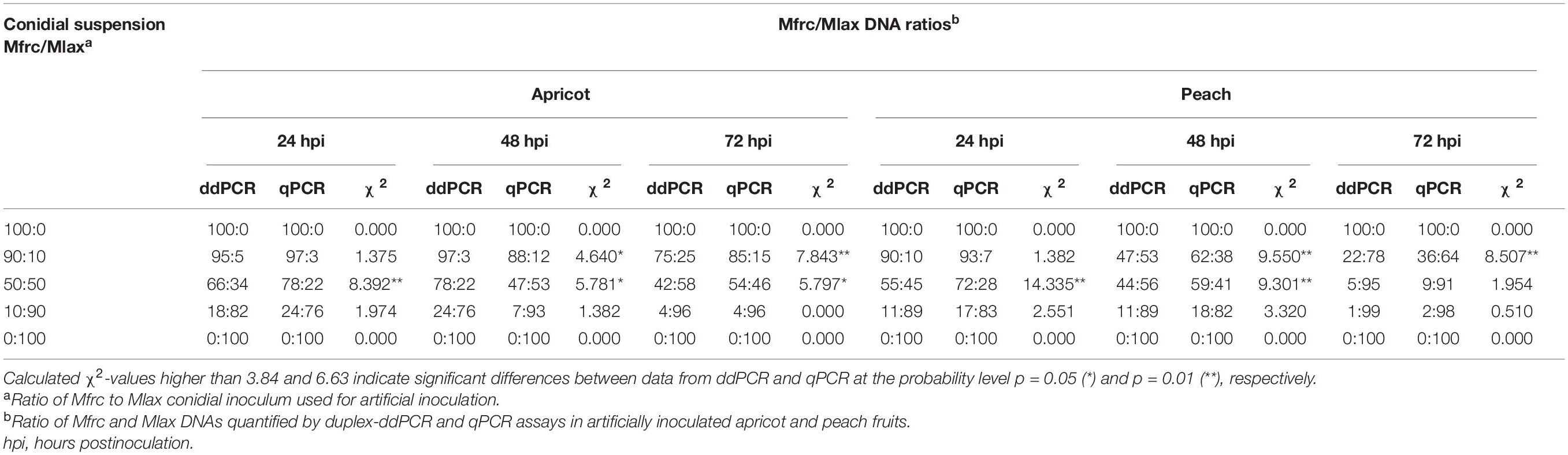
Table 4. Comparison among ratios of Monilinia fructicola (Mfrc) and M. laxa (Mlax) DNAs quantified by duplex-ddPCR and duplex-qPCR assays in apricot and peach fruits artificially inoculated with mixed conidial suspensions (1–2 × 106 mL–1) containing different ratios of conidia of the two pathogens.
Discussion
The introduction of the quarantine species M. fructicola into new areas caused significant changes in Monilinia populations responsible for brown rot on stone fruit (Villarino et al., 2013; Abate et al., 2018), so intensive and careful monitoring programs are crucial for evaluating the effectiveness of integrated crop protection strategies and whether adaptation is need (Côté et al., 2004).
The recent availability of ddPCR for the quantification of fungal pathogens allows the absolute quantification of the target, without the use of a standard curve, and an improvement in accuracy and sensitivity as compared with qPCR assays (Palumbo et al., 2016; del Pilar Martínez-Diz et al., 2020). In the present study, a duplex-ddPCR assay for the simultaneous quantification of both M. fructicola and M. laxa, the main agents of brown rot on stone fruits in Italy and many other Countries, was set up, optimized, and compared to the qPCR assay previously developed by Abate et al. (2018) and successfully applied to quantify the relative proportions of the two species in artificially inoculated apricot and peach fruits. All the experiments meet the minimum requirements for qPCR (Bustin et al., 2009) and digital PCR (Huggett et al., 2013; dMIQE Group and Huggett, 2020) data.
The concentrations of primers/TaqMan probe sets efficiently used in qPCR (240/160 nM), first tested in ddPCR resulted in low amplitude signals of droplets individually analyzed by using a two-color detection system set to detect FAM- and HEX-labeled probes, specific for M. fructicola and M. laxa, respectively. An increase in the concentrations up to 500/250 nM resulted in a higher fluorescence amplitude for both primers/probe sets and better separation between positive and negative droplets for FAM detection. Moreover, a thermal gradient was used to optimize reaction conditions that allowed to identify 58°C as the best annealing temperature improving droplet separation for both FAM and HEX detection. To further improve the known limited separation between positive and negative droplets with the HEX probe, the number of cycles routinely set up to 40 in ddPCR assays was increased up to 50 cycles as suggested by Huggett et al. (2013) and dMIQE Group and Huggett (2020).
A good and comparable linearity (R2 ≥ 0.997) in ddPCR vs. qPCR was ascertained for both the fungal species M. fructicola and M. laxa. In addition, ddPCR was about 200-fold more sensitive than qPCR. The quantification limit using ddPCR was 0.25 pg for both M. fructicola and M. laxa, while 50 pg of DNA was the quantification limit using qPCR for both pathogens. In details, the detection threshold values of ddPCR on M. fructicola and M. laxa DNA was of 0.34 and 0.44 copies μL–1 in the PCR mix, respectively, for the two species, corresponding to 7.5 and 9.7 copies of the target DNA in the analyzed sample. The results herein reported are consistent with those obtained in other research studies on other plant pathogens (Zhao et al., 2016; Maheshwari et al., 2017; del Pilar Martínez-Diz et al., 2020). Nevertheless, ddPCR failed to quantify more than 4,000 copies μL–1 of DNA target corresponding to about 7.5 ng, due to complete saturation of positive droplets at high concentrations of DNA template.
The ddPCR assay showed relatively low intra-/interassay variation, indicating that it can provide a repeatable and reproducible quantification of M. fructicola and M. laxa in fruits, especially for samples with concentrations of target DNA in the reaction mixture ranging from 25 pg to 0.5 ng corresponding to a number of copies μL–1 from 44 (M. fructicola) or 56 (M. laxa) to 711 (M. fructicola) or 1,037 (M. laxa).
To validate the duplex-ddPCR assay, apricot and peach fruits were artificially inoculated with different M. fructicola/M. laxa conidial suspension ratios, and the DNA of both fungal species was quantified by both ddPCR and qPCR and used to estimate the relative Monilinia ratios. A high correlation between the two molecular techniques was observed. However, differences in the amplification efficiency of DNA quantification from the two species by duplex qPCR caused an overestimation of M. fructicola vs. M. laxa and consequently less accurate estimation of their proportion in infected fruits. Indeed, in our qPCR experiments, the average efficiency of the M. laxa calibration curve was 98.4%, whereas the efficiency of the M. fructicola calibration curve was higher than 100% (104.2%), likely due to interfering compounds in DNA extracts. Therefore, application of ddPCR for detection and quantification of M. fructicola and M. laxa in stone fruits was more sensitive and reliable. The ddPCR assay is hence a reliable method with enhanced sensitivity and accuracy in detection of the two species even without visible symptoms on fruits and could be reliably applied to analyze latent Monilinia infections in plant tissues. No inhibition or loss of sensitivity was indeed observed when Monilinia DNA was extracted from peach and apricot fruits.
At all sampling times (24, 48, and 72 hpi) in our experiments, M. fructicola and M. laxa species were quantified, taking into consideration the initial ratios of the conidial suspension used for the inoculation. A general increase in M. laxa DNA was recorded starting from 48 hpi on both apricot and peach fruits, and at 72 hpi on peach became prevalent over M. fructicola as compared to the starting ratio. Moreover, the lesions observed on the artificially inoculated fruits with conidial suspensions including M. laxa conidia were always higher than those observed on fruits inoculated with M. fructicola alone. These results agree with those obtained by Hu et al. (2011) who observed on peach fruits a slower growing of M. fructicola compared to M. laxa, although they proved a fastest colony growth rate on PDA medium. Thus, the fitness edge proved for M. fructicola vs. M. laxa in the monitoring programs (Villarino et al., 2013; Papavasileiou et al., 2015; Abate et al., 2018; Ortega et al., 2019) seems to be not strictly related to the pathogenicity, and other competitive factors, such as vegetative vigor and abundance of conidia production, are likely responsible for the replacement of the indigenous species M. laxa and M. fructigena (Villarino et al., 2013).
A preliminary evaluation of the possible application of the new ddPCR method was carried out on 20 naturally infected fruits of peach, apricot, and cherry from different orchards and three regions of Southern Italy (Apulia, Basilicata, and Campania), and 8 fruits were infected by M. laxa and 12 by M. fructicola and no mixed infections were found. Currently, the method is applied in more extensive monitoring programs on Monilinia populations on stone fruit in different geographic areas.
In conclusion, with this study, a duplex-ddPCR assay for the quantification of M. fructicola and M. laxa was optimized, validated, and its performance compared with the duplex-qPCR assay. To the best of our knowledge, this is the first study on the application of ddPCR in the quantitative detection of Monilinia species. ddPCR could produce highly sensitive and quantitative results. The method could be useful in the future in monitoring programs on Monilinia populations, especially at low levels of infection and in the presence of high levels of PCR inhibitors in plants, although the high cost of ddPCR still represents a drawback for its use as a high throughput method. The monitoring of Monilinia species present in single orchard or a growing area would support the growers in the implementation of more sustainable and effective crop protection strategies by more appropriate choices of plant protection products against brown rot pathogens during the cropping season, as differences in fungicide responses have been reported for different Monilinia species (Egüen et al., 2016; Abate et al., 2018). Based on the results observed through the validation of the assay on artificially inoculated apricot and peach fruits, the methods can be applied to investigate the behavior of the two fungal species and their population dynamics even when they coexist and share the same ecological niche.
Data Availability Statement
The raw data supporting the conclusions of this article will be made available by the authors, without undue reservation.
Author Contributions
CRa designed the experiments, performed the main experimental activities, collected and analyzed the data, and wrote the main manuscript. DG performed some experiments, contributed to data analysis, and to the writing of the main manuscript. SP, RD, and CRo designed and performed some experiments, analyzed the data, and wrote the main manuscript. PR analyzed the data. FF contributed to the experiments design, supervised, and complemented the writing and coordinated the collaboration of the authors. All authors reviewed the manuscript.
Funding
This work was supported by the University of Bari Aldo Moro for the project “Epidemiology, Genetics of Plant Pathogens and Development of Molecular Diagnostic Methods” and from Apulia Region, PO FESR 2007–2013—Axis I, Line of intervention 1.2., Action 1.2.1 for the project “Laboratory Network for the Selection, Characterization and Conservation of Germplasm and for Preventing the Spread of Economically Relevant and Quarantine Pests (SELGE) No. 14”.
Conflict of Interest
The authors declare that the research was conducted in the absence of any commercial or financial relationships that could be construed as a potential conflict of interest.
Publisher’s Note
All claims expressed in this article are solely those of the authors and do not necessarily represent those of their affiliated organizations, or those of the publisher, the editors and the reviewers. Any product that may be evaluated in this article, or claim that may be made by its manufacturer, is not guaranteed or endorsed by the publisher.
Acknowledgments
We thank Domenico Netti for his contribution to the work. ddPCR assays were performed by using the facilities of the “Laboratory for Animal Welfare, Productive and Reproductive Efficiency in Farm Animals (LA.B.E.R.P.A.R.) No. 67” of the University of Bari Aldo Moro.
Supplementary Material
The Supplementary Material for this article can be found online at: https://www.frontiersin.org/articles/10.3389/fmicb.2021.747560/full#supplementary-material
Footnotes
References
Abate, D., Pastore, C., Gerin, D., De Miccolis Angelini, R. M., Rotolo, C., Pollastro, S., et al. (2018). Characterization of Monilinia spp. populations on stone fruit in South Italy. Plant Dis. 102, 1708–1717. doi: 10.1094/PDIS-08-17-1314-RE
Atallah, Z. K., Bae, J., Jansky, S. H., Rouse, D. I., and Stevenson, W. R. (2007). Multiplex real-time quantitative PCR to detect and quantify Verticillium dahliae colonization in potato lines that differ in response to Verticillium wilt. Phytopathology 97, 865–872. doi: 10.1094/PHYTO-97-7-0865
Bahar, M. H., Wist, T. J., Bekkaoui, D. R., Hegedus, D. D., and Olivier, C. Y. (2018). Aster leafhopper survival and reproduction, and Aster yellows transmission under static and fluctuating temperatures, using ddPCR for phytoplasma quantification. Sci. Rep. 8, 1–9. doi: 10.1038/s41598-017-18437-0
BogožalecKošir, A., Demšar, T., Štebih, D., Žel, J., and Milavec, M. (2019). Digital PCR as an effective tool for GMO quantification in complex matrices. Food Chem. 294, 73–78. doi: 10.1016/j.foodchem.2019.05.029
Bustin, S. A., Benes, V., Garson, J. A., Hellemans, J., Huggett, J., Kubista, M., et al. (2009). The MIQE guidelines: minimum information for publication of quantitative real-time PCR experiments. Clin.Chem. 55, 611–622. doi: 10.1373/clinchem.2008.112797
Byrde, R. J. W., and Willetts, H. J. (1977). The Brown Rot Fungi Of Fruit: Their Biology And Control. London: Pergamon Press. doi: 10.1016/C2013-0-02715-6
Côté, M. J., Tardif, M. C., and Meldrum, A. J. (2004). Identification of Monilinia fructigena, M. fructicola, M. laxa, and Monilia polystroma on inoculated and naturally infected fruit using multiplex PCR. Plant Dis. 88, 1219–1225. doi: 10.1094/PDIS.2004.88.11.1219
De Cal, A., Gell, I., Usall, J., Viñas, I., and Melgarejo, P. (2009). First report of brown rot caused by Monilinia fructicola in peach orchards in Ebro Valley, Spain. Plant Dis. 93:763. doi: 10.1094/PDIS-93-7-0763A
De Miccolis Angelini, R. M., Habib, W., Rotolo, C., Pollastro, S., and Faretra, F. (2010). Selection, characterization and genetic analysis of laboratory mutants of Botryotinia fuckeliana (Botrytis cinerea) resistant to the fungicide boscalid. Eur. J. Plant Pathol. 128, 185–199. doi: 10.1007/s10658-010-9643-8
del Pilar Martínez-Diz, M., Andrés-Sodupe, M., Berbegal, M., Bujanda, R., Díaz-Losada, E., and Gramaje, D. (2020). Droplet digital PCR technology for detection of Ilyonectria liriodendri from grapevine environmental samples. Plant Dis. 104, 1144–1150. doi: 10.1094/PDIS-03-19-0529-RE
dMIQE Group, and Huggett, J. F. (2020). The digital MIQE guidelines update: minimum information for publication of quantitative digital PCR experiments for 2020. Clin Chem. 66, 1012–1029. doi: 10.1093/clinchem/hvaa125
Dreo, T., Pirc, M., Ramšak, Ž, Pavšič, J., Milavec, M., Žel, J., et al. (2014). Optimising droplet digital PCR analysis approaches for detection and quantification of bacteria: a case study of fire blight and potato brown rot. Anal. Bioanal. Chem. 406, 6513–6528. doi: 10.1007/s00216-014-8084-1
Dupas, E., Legendre, B., Olivier, V., Poliakoff, F., Manceau, C., and Cunty, A. (2019). Comparison of real-time PCR and droplet digital PCR for the detection of Xylella fastidiosa in plants. J. Microbiol. Methods 162, 86–95. doi: 10.1016/j.mimet.2019.05.010
Egüen, B., Melgarejo, P., and De Cal, A. (2016). The effect of fungicide resistance on the structure of Monilinia laxa populations in Spanish peach and nectarine orchards. Eur. J. Plant Pathol. 145, 815–827. doi: 10.1007/s10658-016-0871-4
Gell, I., Cubero, J., and Melgarejo, P. (2007). Two different PCR approaches for universal diagnosis of brown rot and identification of Monilinia spp. in stone fruit trees. J. Appl. Microbiol. 103, 2629–2637. doi: 10.1111/j.1365-2672.2007.03495.x
Gutiérrez-Aguirre, I., Rački, N., Dreo, T., and Ravnikar, M. (2015). Droplet digital PCR for absolute quantification of pathogens. Methods Mol. Biol. 1302, 331–347. doi: 10.1007/978-1-4939-2620-6_24
Hindson, B. J., Ness, K. D., Masquelier, D. A., Belgrader, P., Heredia, N. J., Makarewicz, A. J., et al. (2011). High-throughput droplet digital PCR system for absolute quantitation of DNA copy number. Anal. Chem. 83, 8604–8610. doi: 10.1021/ac202028g
Hindson, C. M., Chevillet, J. R., Briggs, H. A., Gallichotte, E. N., Ruf, I. K., Hindson, B. J., et al. (2013). Absolute quantification by droplet digital PCR versus analog real-time PCR. Nat. Methods 10:1003. doi: 10.1038/nmeth.2633
Hu, M. J., Cox, K. D., Schnabel, G., and Luo, C. X. (2011). Monilinia species causing brown rot of peach in China. PLoS One 6:e24990. doi: 10.1371/journal.pone.0024990
Huggett, J. F., Foy, C. A., Benes, V., Emslie, K., Garson, J. A., Haynes, R., et al. (2013). The digital MIQE guidelines: minimum information for publication of quantitative digital PCR experiments. Clin Chem. 59, 892–902. doi: 10.1373/clinchem.2013.206375
Hughes, K. J. D., Fulton, C. E., McReynolds, D., and Lane, C. R. (2000). Development of new PCR primers for identification of Monilinia species. EPPO Bull. 30, 507–511. doi: 10.1111/j.1365-2338.2000.tb00938.x
Ioos, R., and Frey, P. (2000). Genomic variation within Monilinia laxa, M. fructigena and M. fructicola, and application to species identification by PCR. Eur. J. Plant Pathol. 106, 373–378. doi: 10.1023/A:1008798520882
Lane, C. R. (2002). A synoptic key for differentiation of Monilinia fructicola, M. fructigena and M. laxa, based on examination of cultural characters. EPPO Bull. 32, 489–493. doi: 10.1046/j.1365-2338.2002.00595.x
Li, H., Bai, R., Zhao, Z., Tao, L., Ma, M., Ji, Z., et al. (2018). Application of droplet digital PCR to detect the pathogens of infectious diseases. Biosci. Rep. 38:BSR20181170. doi: 10.1042/BSR20181170
Li, R., Mock, R., Huang, Q., Abad, J., Hartung, J., and Kinard, G. (2008). A reliable and inexpensive method of nucleic acid extraction for the PCR-based detection of diverse plant pathogens. J. Virol. Methods. 154, 48–55. doi: 10.1016/j.jviromet.2008.09.008
Lichtemberg, P. S. F., Silva, F. A., Zeviani, W. M., and May De Mio, L. L. (2014). Comparison of macro-morphological and physiological methods for Monilinia species identification in Paraná State, Brazil. Can. J. Plant Pathol. 36, 38–47. doi: 10.1080/07060661.2013.864710
Liu, J., Li, C., Muhae-Ud-Din, G., Liu, T., Chen, W., Zhang, J., et al. (2020). Development of the droplet digital PCR to detect the teliospores of Tilletia controversa Kühn in the soil with greatly enhanced sensitivity. Front. Microbiol. 11:4. doi: 10.3389/fmicb.2020.00004
Liu, Y., Wang, Y., Wang, Q., Zhang, Y., Shen, W., Li, R., et al. (2019). Development of a sensitive and reliable reverse transcription droplet digital PCR assay for the detection of citrus yellow vein clearing virus. Arch. Virol. 164, 691–697. doi: 10.1007/s00705-018-04123-7
Maheshwari, Y., Selvaraj, V., Hajeri, S., and Yokomi, R. (2017). Application of droplet digital PCR for quantitative detection of Spiroplasma citri in comparison with real time PCR. PLoS One 12:e0184751. doi: 10.1371/journal.pone.0184751
Maldonado-González, M. M., Del Pilar Martínez-Diz, M., Andrés-Sodupe, M., Bujanda, R., Díaz-Losada, E., and Gramaje, D. (2020). Quantification of Cadophora luteo-olivacea from grapevine nursery stock and vineyard soil using droplet digital PCR. Plant Dis. 104, 2269–2274. doi: 10.1094/PDIS-09-19-2035-RE
Morcia, C., Ghizzoni, R., Delogu, C., Andreani, L., Carnevali, P., and Terzi, V. (2020). Digital PCR: what relevance to plant studies? Biology 9:433. doi: 10.3390/biology9120433
OEPP/EPPO (2002). First Report of Monilinia fructicola in France. EPPO Reporting Service 2002/003: No. 1. Available online at: www.eppo.org (accessed February 8, 2021).
OEPP/EPPO (2009). First Report of Monilinia fructicola in Italy. EPPO Reporting Service 2009/05: No. 2009/091. Available online at: www.eppo.org (accessed February 8, 2021).
Olmedillas-López, S., García-Arranz, M., and García-Olmo, D. (2017). Current and emerging applications of droplet digital PCR in oncology. Mol. Diagn. Ther. 21, 493–510. doi: 10.1007/s40291-017-0278-8
Ortega, S. F., del Pilar Bustos López, M., Nari, L., Boonham, N., Gullino, M. L., and Spadaro, D. (2019). Rapid detection of Monilinia fructicola and Monilinia laxa on peach and nectarine using loop-mediated isothermal amplification. Plant Dis. 103, 2305–2314. doi: 10.1094/PDIS-01-19-0035-RE
Palumbo, J. D., O’keeffe, T. L., Ho, Y. S., and Fidelibus, M. W. (2016). Population dynamics of Aspergillus section Nigri species on vineyard samples of grapes and raisins. J. Food Prot. 79, 448–453. doi: 10.4315/0362-028X.JFP-15-437
Papavasileiou, A., Testempasis, S., Michailides, T. J., and Karaoglanidis, G. S. (2015). Frequency of brown rot fungi on blossoms and fruit in stone fruit orchards in Greece. Plant Pathol. 64, 416–424. doi: 10.1111/ppa.12264
Pellegrino, C., Gullino, M. L., Garibaldi, A., and Spadaro, D. (2009). First report of brown rot of stone fruit caused by Monilinia fructicola in Italy. Plant Dis. 93:668. doi: 10.1094/PDIS-93-6-0668A
Pinheiro, L. B., Coleman, V. A., Hindson, C. M., Herrmann, J., Hindson, B. J., Bhat, S., et al. (2012). Evaluation of a droplet digital polymerase chain reaction format for DNA copy number quantification. Anal Chem. 84, 1003–1011. doi: 10.1021/ac202578x
Rahman, M. T., Uddin, M. S., Sultana, R., Moue, A., and Setu, M. (2013). Polymerase Chain Reaction (PCR): a short review. Anwer Khan Mod. Med. Coll. J. 4, 30–36. doi: 10.3329/akmmcj.v4i1.13682
Schena, L., Nigro, F., Ippolito, A., and Gallitelli, D. (2004). Real-time quantitative PCR: a new technology to detect and study phytopathogenic and antagonistic fungi. Eur. J. Plant Pathol. 110, 893–908. doi: 10.1007/s10658-004-4842-9
Taylor, S. C., Laperriere, G., and Germain, H. (2017). Droplet Digital PCR versus qPCR for gene expression analysis with low abundant targets: from variable nonsense to publication quality data. Sci. Rep. 7:2409. doi: 10.1038/s41598-017-02217-x
Van Brouwershaven, I. R., Bruil, M. L., Van Leeuwen, G. C. M., and Kox, L. F. F. (2010). A real-time (TaqMan) PCR assay to differentiate Monilinia fructicola from other brown rot fungi of fruit crops. Plant Pathol. 59, 548–555. doi: 10.1111/j.1365-3059.2009.02220.x
Villarino, M., Egüen, B., Lamarca, N., Segarra, J., Usall, J., Melgarejo, P., et al. (2013). Occurrence of Monilinia laxa and M. fructigena after introduction of M. fructicola in peach orchards in Spain. Eur. J. Plant Pathol. 137, 835–845. doi: 10.1007/s10658-013-0292-6
Villarino, M., Melgarejo, P., and De Cal, A. (2016). Growth and aggressiveness factors affecting Monilinia spp. survival peaches. Int. J. Food Microbiol. 227, 6–12. doi: 10.1016/j.ijfoodmicro.2016.01.023
Wang, J. R., Guo, L. Y., Xiao, C. L., and Zhu, X. Q. (2018). Detection and identification of six Monilinia spp. causing brown rot using TaqMan real-time PCR from pure cultures and infected apple fruit. Plant Dis. 102, 1527–1533. doi: 10.1094/PDIS-10-17-1662-RE
Xu, T., Yao, Z., Liu, J., Zhang, H., Din, G. M. U., Zhao, S., et al. (2020). Development of droplet digital PCR for the detection of Tilletia laevis, which causes common bunt of wheat, based on the SCAR marker derived from ISSR and real-time PCR. Sci. Rep. 10:16106. doi: 10.1038/s41598-020-72976-7
Yang, R., Paparini, A., Monis, P., and Ryan, U. (2014). Comparison of next-generation droplet digital PCR (ddPCR) with quantitative PCR (qPCR) for enumeration of Cryptosporidium oocysts in faecal samples. Int. J. Parasitol. 44, 1105–1113. doi: 10.1016/j.ijpara.2014.08.004
Zhang, Y., Huang, J., Wang, X., Zhou, H., Li, X., Fu, W., et al. (2017). Detection of Potato virus S (PVS) by droplet digital PCR (ddPCR). J. Agric. Biotechnol. 25, 1721–1728. doi: 10.3969/j.issn.1674-7968.2017.10.019
Keywords: ddPCR, quantitative PCR (qPCR), brown rot, detection, plant pathogens, fungi, stone fruit
Citation: Raguseo C, Gerin D, Pollastro S, Rotolo C, Rotondo PR, Faretra F and De Miccolis Angelini RM (2021) A Duplex-Droplet Digital PCR Assay for Simultaneous Quantitative Detection of Monilinia fructicola and Monilinia laxa on Stone Fruits. Front. Microbiol. 12:747560. doi: 10.3389/fmicb.2021.747560
Received: 26 July 2021; Accepted: 04 November 2021;
Published: 29 November 2021.
Edited by:
Matthias Sipiczki, University of Debrecen, HungaryReviewed by:
Raymond Yokomi, Agricultural Research Service, United States Department of Agriculture, United StatesVijayanandraj Selvaraj, National Botanical Research Institute (CSIR), India
Liangfen Yin, Huazhong Agricultural University, China
Copyright © 2021 Raguseo, Gerin, Pollastro, Rotolo, Rotondo, Faretra and De Miccolis Angelini. This is an open-access article distributed under the terms of the Creative Commons Attribution License (CC BY). The use, distribution or reproduction in other forums is permitted, provided the original author(s) and the copyright owner(s) are credited and that the original publication in this journal is cited, in accordance with accepted academic practice. No use, distribution or reproduction is permitted which does not comply with these terms.
*Correspondence: Stefania Pollastro, c3RlZmFuaWEucG9sbGFzdHJvQHVuaWJhLml0