- 1Facultad de Medicina, Centro Nacional de Secuenciación Genómica - CNSG, Sede de Investigación Universitaria - SIU, Universidad de Antioquia -UdeA, Medellín, Colombia
- 2Centro de Innovación y Tecnología ICP, Ecopetrol S.A, Gerencia de Operaciones, Bucaramanga, Colombia
- 3Grupo SISTEMIC, Ingeniería Electrónica, Facultad de Ingeniería, Universidad de Antioquia - UdeA, Medellín, Colombia
Microorganisms are capable of colonizing extreme environments like deep biosphere and oil reservoirs. The prokaryotes diversity in exploited oil reservoirs is composed of indigenous microbial communities and artificially introduced microbes. In the present work, high throughput sequencing techniques were applied to analyze the microbial community from the injected and produced water in a neotropical hyper-thermophile oil reservoir located in the Orinoquia region of Colombia, South America. Tepidiphilus is the dominant bacteria found in both injection and produced waters. The produced water has a higher microbial richness and exhibits a Tepidiphilus microdiversity. The reservoir injected water is recycled and treated with the biocides glutaraldehyde and tetrakis-hydroxymethyl-phosphonium sulfate (THPS) to reduce microbial load. This process reduces microbial richness and selects a single Tepidiphilus genome (T. sp. UDEAICP_D1) as the dominant isolate. Thermus and Hydrogenobacter were subdominants in both water systems. Phylogenomic analysis of the injection water dominant Tepidiphilus positioned it as an independent branch outside T. succinatimandens and T. thermophilus lineage. Comparative analysis of the Tepidiphilus genomes revealed several genes that might be related to the biocide-resistant phenotype and the tolerance to the stress conditions imposed inside the oil well, like RND efflux pumps and type II toxin-antitoxin systems. Comparing the abundance of Tepidiphilus protein-coding genes in both water systems shows that the biocide selected Tepidiphilus sp. UDEAICP_D1 genome has enriched genes annotated as ABC-2 type transporter, ABC transporter, Methionine biosynthesis protein MetW, Glycosyltransferases, and two-component system NarL.
Introduction
Oil reservoirs exhibit extreme environmental conditions for microbial life such as high temperature, salinity, pressure, anoxic conditions, and presence of heavy metals (Pannekens et al., 2019; Roumagnac et al., 2020). Nevertheless, the crude oil, the aqueous phase, and solid surfaces in the well can harbor complex microbial communities with the capability to thrive in these extreme environments (Liu et al., 2018; Pannekens et al., 2019). Sulfate-reducing bacteria, nitrate-reducing bacteria, fermentative bacteria, syntrophic bacteria, methanogens, and many more microorganisms can be found in oil reservoirs (Liang et al., 2018). The structure of natural microbial communities can be explained by niche-based mechanisms (Yuan et al., 2021), and differences in the abundance of taxa reflect the differences in the environmental factors (Gao et al., 2016). Among these, temperature has been considered the highest theoretical limiting factor controlling microbial growth in petroleum reservoirs (Magot et al., 2000).
Indigenous and introduced microorganisms have several effects in petroleum reservoirs and oil exploitation (Ren et al., 2015); some of them are detrimental producing hydrogen sulfide (souring), inducing corrosion or leading to oil pipelines clogging (Okoro et al., 2016; Dolfing and Hubert, 2017; Vigneron et al., 2017). Chemical and physical treatments are widely applied to reduce the microbial load introduced in the secondary oil recovery strategies (Jurelevicius et al., 2021). In the oil industry, the biocide treatment can be applied continuously or in cycles on weekly basis (Korenblum et al., 2010). Oxidizing (chlorine and ozone), or non-oxidizing biocides (quaternary ammonium salts, aldehydes, and tetrakis -hydroxymethyl- phosphonium sulfate-THPS) are commonly applied (Gieg et al., 2011). However, this practice eventually leads to the selection of biocide-resistant microbes (Kahrilas et al., 2015).
The microbial diversity in high-temperature reservoirs includes the thermophilic genera Thermodesulfovibrio, Hydrogenophilus, Thermodesulforhabdus, Pseudomonas, Thermovirga, Thermoanaerobacteraceae, Thermus, Thermodesulfobacteriaceae, and others (Zhou et al., 2020). Members of Tepidiphilus have been reported in low proportion in oil reservoirs and described as a nitrate-reducing bacterium (NRB) belonging to the family Hydrogenophylaceae (Zhang X. et al., 2020). Nowadays, members of genus the Thepidiphilus have been reported in the produced water from thermophilic oil reservoirs, thermophilic anaerobic digesters, and hot springs from Australia, China, and India (Zhang X. et al., 2020. So far there are no reports of reference genomes for this genus in the Neotropic realm.
To date, there is limited knowledge regarding the genomic characteristics of the Tepidiphilus genus and its molecular capabilities to resist typical biocide treatment regimens used in the oil industry. In this work, we report and describe the genomic characteristics of a novel Tepidiphilus isolate found in a hyperthermophile oil well in the Orinoquia region, in Colombia, that endures the treatment with glutaraldehyde and THPS.
Materials and Methods
Water Samples Collection and Physicochemical Measurements
The studied oil field is in the Orinoquia region of Colombia, near the municipality of Castilla la nueva, Meta. The GPS coordinates are 3°52′46.0″N 73°37′53.0″W. The temperature in the water recovered from the oil well is estimated to be around 93°C. After sampling, the temperature of the produced water samples went down to the environmental temperature, around 26°C. The water recycling system works at environmental temperature. Before biocide-treated recycled water is reinjected into the oil well, the injection water samples were taken. About 10 L of water was taken from each sampling point and this volume was filtered on 0.45-micron diameter nitrocellulose membranes to retain the bacterial community in the filter matrix. The membranes of the filtered water samples were transferred refrigerated (4–8°C) to the laboratory for DNA extraction. Chemical analyses performed to the waters included dissolved oxygen, carbon dioxide, iron, chloride ion, and sulfate. All these tests were performed with the colorimetric kit Chemetrics (Virginia, United States): K-1910 (CO2), K-7350S (dissolved oxygen), K-6210 (iron), K-2020 (chloride ion), K-9510 (sulfate).
Bacterial Community Metataxonomic Analysis
The DNA was extracted using the PowerSoil® DNA Isolation Kit (QIAGEN) according to the manufacturer’s instructions. The DNA QC was performed by combining quantitation with PicoGreen (Invitrogen P11496) and gel electrophoresis (1% agarose) to evaluate the DNA degradation. In the produced water samples, we observed some solid particles that stacked the membrane with smaller volumes. This situation was associated with low DNA yield.
Illumina libraries were prepared and sequenced in a MiSeq (Illumina) instrument producing 300 bp paired-end reads at Macrogen Inc. (Seoul, Republic of Korea) following their recommendations. The V3-V4 hypervariable regions of bacterial and archaeal 16S rDNA gene were targeted using PCR with the primers Bakt_341F (5′-CCTACGGGNGGCWGCAG-3′) and Bakt_805R (5′-GACTACHVGGGTATCTAATCC-3′) (Herlemann et al., 2011). Amplicon libraries yielded between 128,450 and 212,074 raw reads. The amplicon reads were analyzed with the Mothur pipeline v.1.44.3 (Schloss et al., 2009). Paired-end (PE) reads were merged using Mothur’s command “make.contigs.” Sequences with homopolymers longer than 6, with ambiguous bases, or more than 466 bases in length were filtered out.
The filtered amplicon sequences were aligned to the SILVA 16S rDNA reference database (Quast et al., 2013) with the command align.seqs and only those that matched the hypervariable regions V3/V4 were retained. Next, VSEARCH (Rognes et al., 2016) was used to detect chimeric sequences. Non-bacterial lineages were removed with the command remove.lineage (chloroplasts, mitochondria, and eukaryotes). Read clustering to operational taxonomic units was performed with the subroutine “dist.seqs” at a distance limit of 0.03. Library size for each sample was normalized with the “totalgroup” method. Rare (supported by < 3 reads) OTUs were removed for downstream analyzes. The phylogenetic classification was carried out with the RDP classifier tool (bootstrap threshold set to 80) (Wang et al., 2007) and the SILVA v132 16S database. Microbial richness and diversity and indices were calculated with the R (R Development Core Team, 2018) packages Phyloseq (McMurdie and Holmes, 2013), Vegan (Oksanen et al., 2020), and Microbiome (Lahti and Shetty, 2012–2019).
Shotgun Metagenome Analysis
For the shotgun metagenome sequencing experiment, a set of 4 DNA samples (obtained from 4 independent filters) were pooled and concentrated with Amicon Ultra Centrifugal Filters (Millipore) of each type of water: injection water-IW or produced water-PW. With each pool, one WGS library was prepared and sequenced at Macrogen Korea in one Illumina Novaseq 6000 instrument producing 150 bases paired end reads. The shotgun library was prepared with the kit TruSeq Nano DNA Kit (Illumina). Fragmentation was performed with sonication. Around 100 ng of metagenomic DNA was used for the library preparation.
The produced water WGS library yielded 77,796,614 PE reads, while the injection water library yielded 84,810,002. The reads were cleaned with CUTADAPT software removing adapters and poor-quality reads (<Q30) with flags -j 20 -q 30 -m 70 –max-n 0 (v 2.10) (Martin, 2011). Reads that were shorter than 70 bases or singletons were excluded for further analysis.
Clean reads and scaffolds were classified with MEGAN software v.6.19.9 (Huson et al., 2007) comparing the sequences using DIAMOND v2.0.11.149 (Buchfink et al., 2014) against the NCBI’s NR (Agarwala et al., 2018) protein database (February 2021). The shotgun metagenome assembly was performed with MetaSPADES version v3.14.1 (flags -t 40 -m 160) testing Kmer lengths (-k) of 21, 33, 55, 77, and 99 bases (Nurk et al., 2017).
Injection Water Dominant Tepidiphilus Genome Analysis
Shotgun metagenome-assembled scaffolds were analyzed based on their nucleotide lengths and sequencing depths calculated by the assembler SPADES. Taxonomical assignment for each scaffold was obtained from MEGAN used with default settings.
The binning of the scaffolds into individual genomes was performed with VAMB version 3.0.2 (Nissen et al., 2021) with default parameters. The dominant Tepidiphilus genome was binned in a group of 23 scaffolds, 10 of them had nucleotide lengths below 1,000 bases, which were removed for subsequent genomic analysis. The 13 remaining scaffolds set summed 2,237,770 bp. Next, these scaffolds were submitted for genome annotation (CDSs prediction) to the DFAST web server1 (Tanizawa et al., 2016) activating the FastANI (Jain et al., 2018) taxonomic analysis option as well as the CHECKM v1.1.3 (Parks et al., 2015) completeness analysis. Taxonomic analysis using the digital DNA-DNA hybridization approach was performed in the TYGS website2 (Meier-Kolthoff and Göker, 2019). Predicted putative peptides were submitted for annotation at the KEGG database using the KAAS tool (Moriya et al., 2007) using the BBH method and the GHOSTX algorithm.
For the Tepidiphilus genome mixture analysis, shotgun reads of the injection and produced waters were mapped to the IW dominant Tepidiphilus genome using bowtie2 v. 2.4.1 (Langmead and Salzberg, 2012). The resulting SAM files were processed to obtain sorted BAM using SAMTOOLS v. 1.10 (Li et al., 2009) and variants were called with BCFTOOLS v. 1.10.2 (Li, 2011) filtering called variant with a minimum quality of 30.
The relative abundance of the predicted CDSs was calculated with the program KALLISTO 1.10.2 (Bray et al., 2016) which normalizes the library counts to each reference sequence using the TPM measurement. The fold change for each CDS was calculated with the formula Y/X – 1; being X the TPM counts for the PW shotgun reads, and Y the IW TPM counts for each CDS.
To confirm the genomic position of the CDSs with the highest fold changes, the annotated genomes were load into the ARTEMIS (Carver et al., 2012) genome browser for manual inspection and curation of the enriched CDSs. The intergenic regions were counted with ARTEMIS selecting and extracting the respective regions of interest.
Orthologous Gene and Phylogenomic Analysis
Orthologous gene analysis was performed with SONICPARANOID program v1.3 (Cosentino and Iwasaki, 2019), using the respective predicted peptides as queries. Single and multicopy orthologous groups were detected in the Tepidiphilus genomes (GenBank assembly accession): T. succinatimandens (GCA_006503695.1), T. thermophilus (GCA_001418245.1), T. margaritifer (GCA_000425565.1), T. sp. J10 (GCA_006980785.1), T. sp. J18 (GCA_006980705.1), T. baoligensis B18 (GCA_012911495.1).
The above-mentioned six reference Tepidiphilus genomes were annotated with DFAST in the same conditions already described elsewhere.
For the phylogenomic analysis 26, single copy coding genes, commonly selected for taxonomic purposes in proteobacteria, were used: gyrB, infC, rplA, rplB, rplC, rplD, rplE, rplF, rplK, rplL, rplM, rplN, rplO, rplP, rplT, rpmA, rpoB, rpsC, rpsE, rpsG, rpsH, rpsK, rpsL, rpsM, rpsS, and secY.
A Maximum-likelihood (ML) tree was calculated with IQTREE2 v. 2.1.32 (Minh et al., 2020). Orthologous CDSs were extracted from each genome. Then, an individual alignment (one per gene) was performed using MAFFT (version v7.475) (Katoh and Standley, 2013) with parameters –inputorder –adjustdirection –anysymbol –auto. All the aligned genes in fasta format were concatenated to form a single aligned nucleotide matrix. The alignment matrix comprises 19002 sites of 9 taxa, seven Tepidiphilus and two outgroups, Azoarcus communis (GCA_003111645.1) and Hydrogenophilus thermoluteolus (GCA_003574215.1). The matrix was organized in two partitions with different substitutions models selected according to BIC (Bayesian Information Criterion) (Lanfear et al., 2014); as follows:
GTR + F + R2: gyrB + infC + rplE + rplL + rpoB + rpsG + rpsL, and TVM + F + I: rplA + rplB + rplC + rplD + rplF + rplK + rplM+ rplN + rplO + rplP + rplT + rpmA + rpsC + rpsE + rpsH + rpsK+ rpsM + rpsS + secY.
One thousand pseudo replicates of SH- aLRT and ultra-fast bootstrap were performed, and consensus tree generated was edited in FigTree v1.4.4.3 Maximum likelihood total tree length was 1.2276.
Statistical Analysis and Graph Preparation
Statistical tests and graphical representation of data were performed with R version 4.0.4 (R Development Core Team, 2018) and R studio Version 1.2.1335. Additional packages used were: ggplot2, dplyr, ggExtra, and tidyverse.
Next Generation Sequencing Data and Assembly Accession Numbers
Metagenome WGS raw sequences were deposited in the SRA NCBI database under the bioproject access number PRJNA727370 (SRR14424469 and SRR14424468).
Metataxonomic 16S V3-V4 amplicon sequences were deposited at the SRA database under the bioproject access number PRJNA727592 (SRR12532400, SRR12532399, SRR12532398, and SRR12532397). Tepidiphilus sp. UdeAICP_D1 MAG (Metagenome assembled genome) was deposited in GenBank under the accession number JAIQIL000000000. Genome and annotation in GFF format were included as Supplementary Material.
Results
Experiment Design and Geochemical Characterization of the Oilfield
The samples were collected in June 2020 in an oil field located in the Orinoquia region of Colombia in the Municipality of Castilla la Nueva, Meta. The reservoir has been flooded for 3 years and the produced water was taken directly from the system of crude treatment that recovers the oil/water mixture from the reservoir. The injection water was taken at the exit of the water treatment system that includes the sequential addition of glutaraldehyde and tetrakis hydroxymethyl phosphonium sulfate (THPS). The in situ reservoir temperature is around 93°C. The physical properties of the samples are described in Supplementary Table 1. The pH values were close to the neutral ranging from 6.9 to 7.5 for produced water (PW) and from 6.7 and 7.1 for injection water (IW). The dissolved oxygen concentration indicates an anaerobic condition in both samples, with IW having a lower oxygen concentration. Both water samples have low salinity according to the ionic constituents.
Microbial Community Structure Based on 16S Amplicon Sequencing
The MiSeq amplicon sequencing experiment yielded between 128,450 and 212,074 read pairs for all tested samples. After filtering short/low-quality reads and chimeras, an average of 42,291 sequences per library was kept for further analysis (Supplementary Table 2). We excluded the replicate 16SPWICP2 due to aberrant results in both richness indices and taxonomic results. The sequences were assigned to 410 OTUs with a nucleotide identity threshold of 97%. The alpha diversity indices showed a higher richness in the PW samples (Figure 1). This observation was supported by the Kruskal-Wallis test (p < 0.05) in the ACE, Chao1, and observed OTUs indices (Figure 1). Shannon and Simpson diversity indices didn’t show any statistical significance (Figures 1D,E). The Good’s coverage values were ≥ 99.8% for all samples. The NMDS ordination revealed a clear separation in the IW and PW samples. The independent clustering of the different microbial communities present in the IW and PW was supported by the Adonis test (p < 0.05) (Figure 2A).
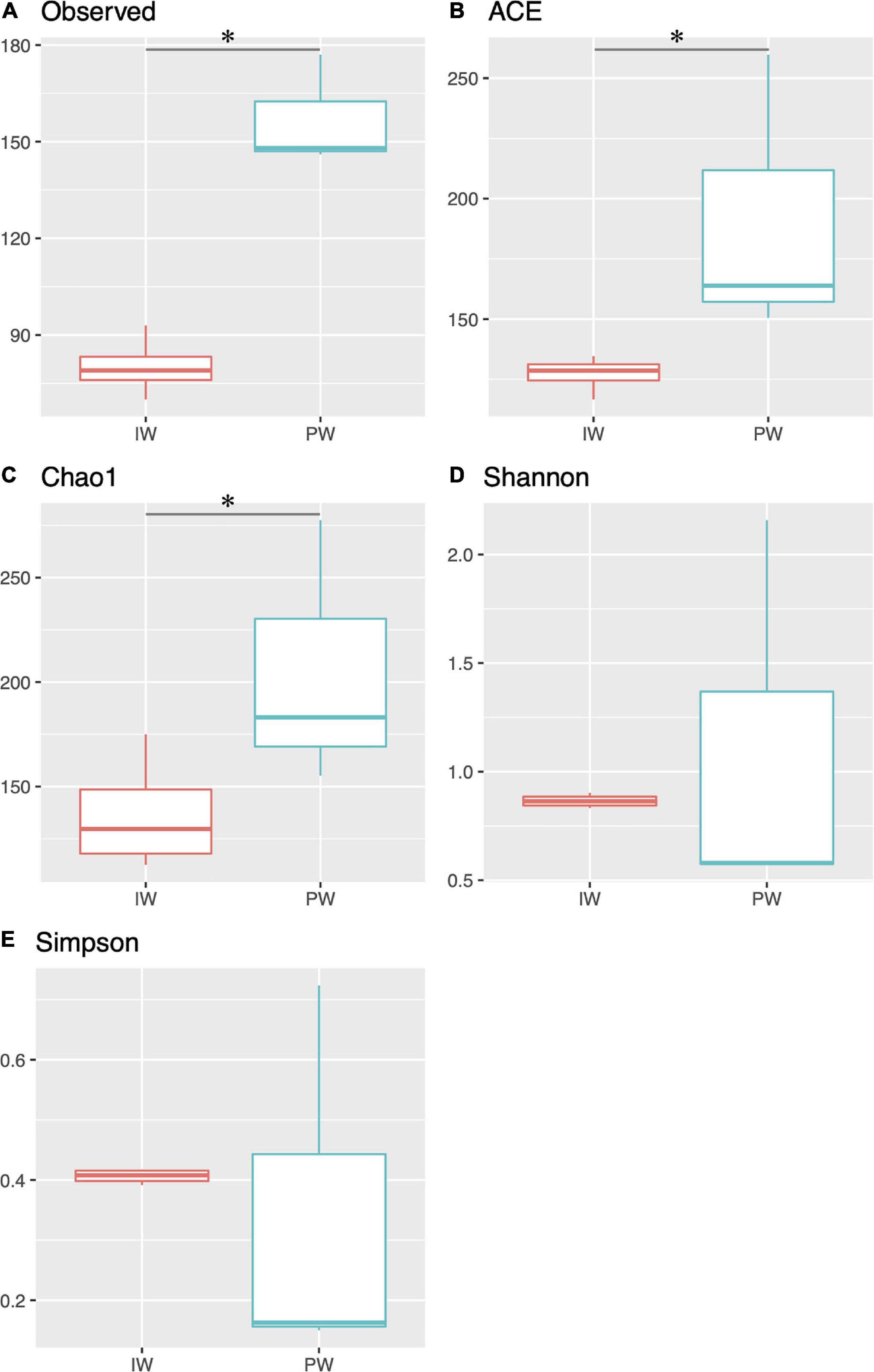
Figure 1. Alpha diversity analysis. Box plot comparing richness and diversity indices of the injection water (IW) and produced water (PW). (A) Observed OTUs. (B) Ace index. (C) Chao1 index. (D) Shannon Index. (E) Simpson Index. Asterix denotes statistical significance in the compared groups (p < 0.05).
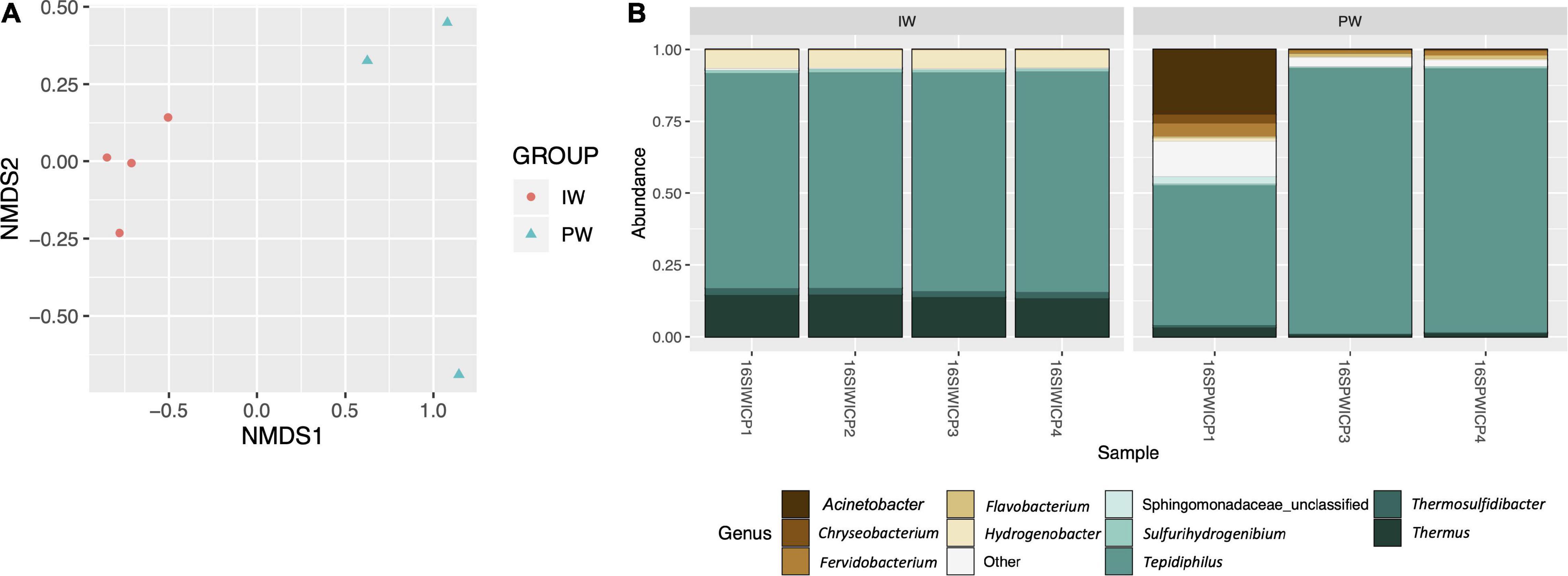
Figure 2. (A) Beta diversity analysis. NMDS ordination analysis comparing the structure of the microbial communities of the injection (IW) and produced waters (Adonis test p < 0.05). (B) Bacterial genus frequencies. Stacked histogram depicting the normalized relative abundance of the top 10 most frequent bacterial genera detected in the metataxonomic analysis of the injection (IW) and produced waters (PW).
Most of the sequences (97.8%) were effectively classified in the genus category. A total of 26 bacterial phyla were identified. Proteobacteria (75.7% ± 0.87 IW and 90.9% ± 5.34 PW), Deinococcus-Thermus (14.06% ± 0.65 IW and 1.77% ± 1.28), and Aquificae (7.48% ± 0.19 IW and 1.11% ± 0.46) were the dominant phyla accounting for more than 90% of the whole bacterial community in both samples. In the produced water, the phyla Thermotogae (2.5%) and Bacteroidetes (2.26%) were in higher proportions (Supplementary Figure 1). At the genus level, a total of 218 taxa were classified (Figure 2B). Tepidiphilus, belonging to Hydrogenophilalia class, was the dominant genus in both samples, 75.6% ± 0.9 and 77.6% ± 25.06 IW and PW, respectively. Thermus and Hydrogenobacter were, respectively, the subdominant genera in the IW and PW samples accounting for 14.04% ± 0.63 and 6.38% ± 0.16.
A core community of 9 bacterial OTUs was detected for the IW and PW representing between 48.5 and 92.2% of all assigned sequences. This core community consisted of Tephidiphilus, Thermus, Thermosulfidibacter, Hydrogenobacter, Thermodesulfovibrio, Thermodesulforhabdus, Sulfurihydrogenibium, Fervidobacterium, and Dictyoglomus (Figure 3).
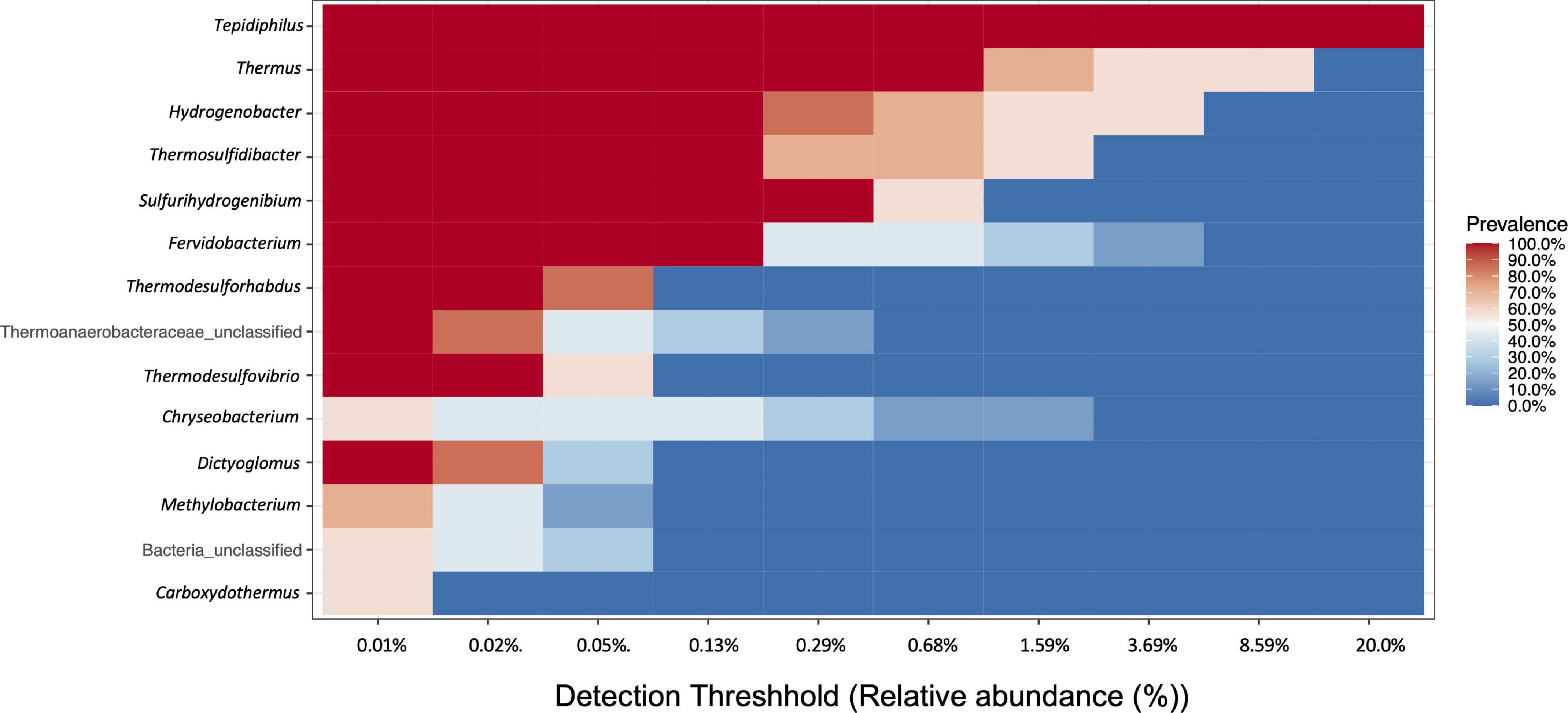
Figure 3. Core community analysis. Heat map representing the genera with prevalence in both water samples (IW and PW). In the X-axis is represented the prevalence of each genus with different detection thresholds.
Genome Analysis of the Dominant Microorganism in the Injection Water
Microbes selected in the injection waters show a resilient phenotype to the biocides THPs and glutaraldehyde applied in the oil reservoir recycled water. To gain insights into the genome structure of the dominant Tepidiphilus of the injection water, complementary genomic/metagenomic analyses were performed.
The metagenome of the injection water was de novo assembled, yielding 38,342,519 total bases contained in 38,581 scaffolds. Analysis of the nucleotide length, average sequencing depth, and the taxonomic assignation of each scaffold show that sequences of the same microorganism tend to cluster in a narrow sequencing depth range (Figure 4). Moreover, the taxonomic data (color of the dots) supports the related taxonomic origin. In concordance with the previous metataxonomic and taxonomic analysis of the shotgun reads, we observed a clear dominance of Tepidiphilus (orange dots), which exhibits the longest scaffold assembled (695,641 bases). It is also evident that two clusters of Tepidiphilus scaffolds were present, one that exceeds the 1,000X average sequencing depth and another at around 16X with lower nucleotide length counts.
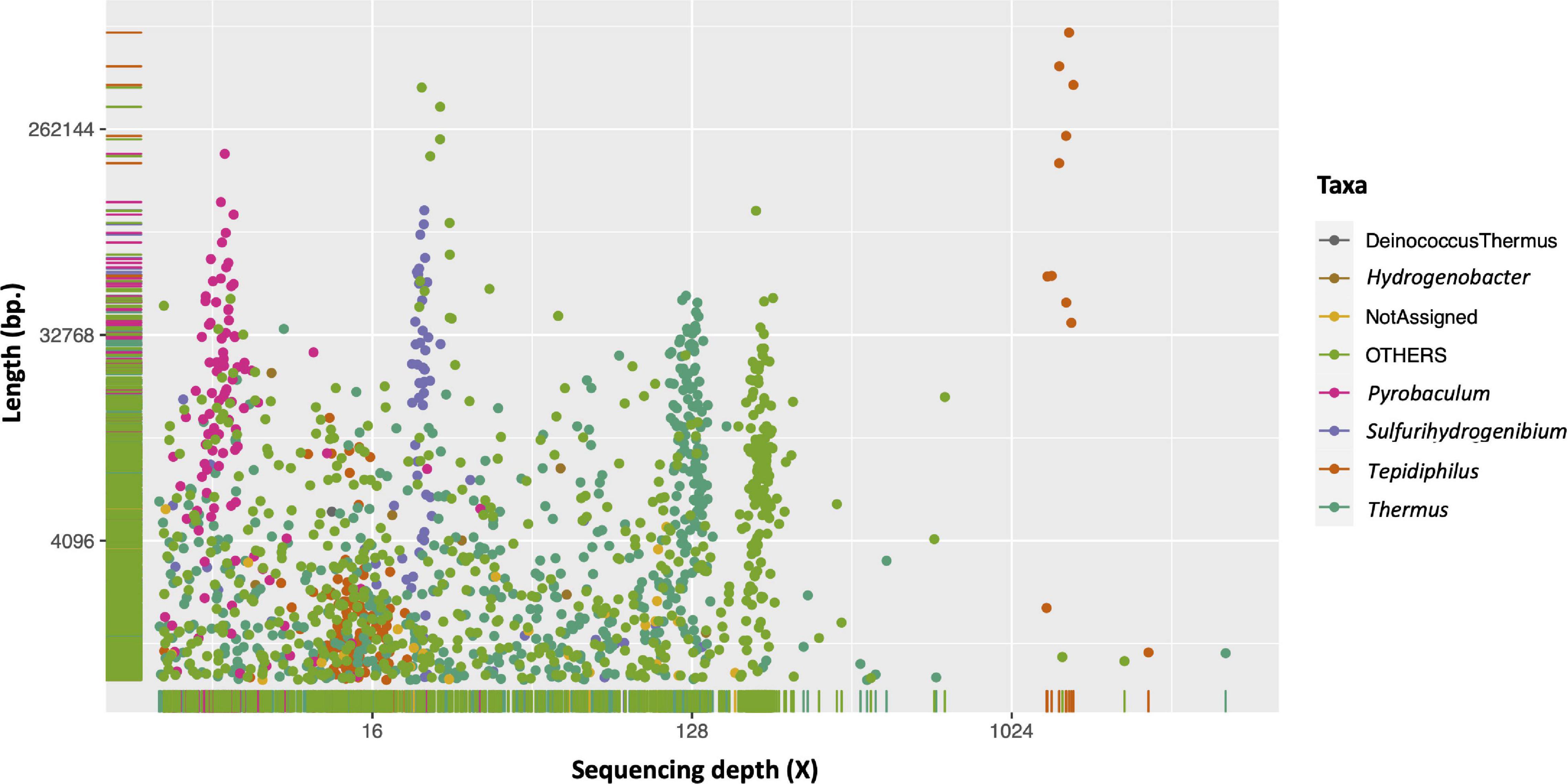
Figure 4. Injection water metagenome analysis of scaffold depth, length, and taxonomy. The scaffold average sequencing depth (Depth, X-axis) and nucleotide size (Length, Y-axis) are plotted using log2 transformation. The color scheme represents the respective taxa assignment for each scaffold based on the MEGAN results.
Other relevant microorganisms observed in this metagenome are Thermus and Sulfurihydrogenibium, and the Archaea Pyrobaculum.
The genome of the IW dominant Tepidiphilus, from now on termed UdeAICP_D1, was isolated using the software VAMP. To achieve this, the shotgun reads were mapped to the metagenome-assembled scaffolds, and then the reads that link different scaffolds allow the clustering of sequences that comes from the same chromosome. Scaffolds shorter than 1,000 bases were excluded from the subsequent genomic analysis. The isolated genome comprises 2,237,527 bp in 13 scaffolds. The assembly N50 value was 494,759 bases, and it exhibits a GC content of 66%. Genome annotation identified 2,108 CDS sequences along with 2 rRNA genes, 51 tRNAs genes, and one CRISPR gene. The average protein length was 333 amino acids, and the coding ratio was 94.1%. The gap ratio was estimated to be 0.004469%. Genome completeness analysis using CHECKM for proteobacteria gave a 100% score and a contamination signal below 1%.
Taxonomic analysis using the FastANI tool indicates that Tepidiphilus sp. UdeAICP_D1 is a species closely related to Tepidiphilus succinatimandens (ANI score 98.3291) and Tepidiphilus thermophilus (ANI score of 98.322) with a nearly equal score for both species. Digital DNA-DNA hybridization analysis classified as Tepidiphilus sp. UDEAICP_D1 as T. thermophilus with a dDDH score of 83.7%. It should be mentioned that Tepidiphilus succinatimandens is also above the dDDH species threshold with a dDDH 82.7%.
Taxonomic assignment confirmation was performed using a phylogenomic analysis based on 26 single copy conserved protein-coding genes. The concatenated alignment comprises 19,002 sites. Azoarcus communis and Hydrogenophilus thermoluteolus were selected as outgroups. The best evolution model (mixed) was selected according to BIC (GTR and TVM). The best maximum likelihood tree had a log-likelihood value of −49969.3879.
The tree shows two well-supported (100% UFB) clades, one that comprises the isolates J10, J18, and B18, and another that comprises T. succinatimandens, T. thermophilus, and Tepidiphilus UdeAICP_D1. Furthermore, T. margaritifer is positioned outside both clades with slightly lower support (90%). The tree also indicates that Tepidiphilus sp. UdeAICP_D1 is an independent branch outside T. succinatimandens and T. thermophilus with 100% UFB support (Figure 5).
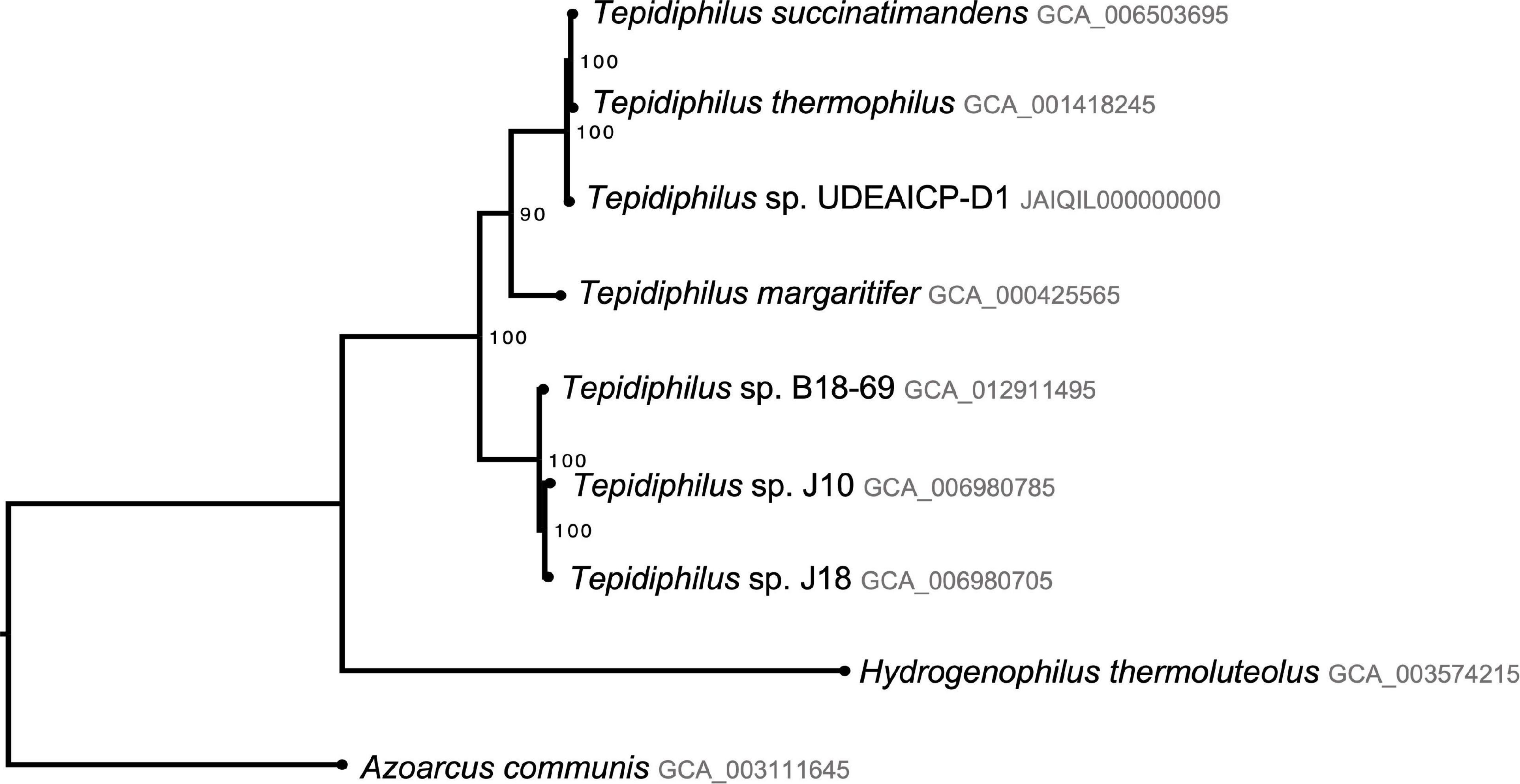
Figure 5. Phylogenomic analysis of the Tepidiphilus species based on 26 single copy conserved protein-coding genes. Maximum likelihood tree with 1,000 pseudoreplicates of ultrafast bootstrap.
Metabolic Potential of Tepidiphilus
The genome of Tepidiphilus UdeAICP_D1 recovered from the injected water was annotated, and key metabolic pathways were predicted with the KEGG (Kyoto Encyclopedia of Genes and Genomes) database. The annotation comparison with the Tepidiphilus genomes deposited in public databases identified a virtual proteome that ranged from 2,033 and 2,288 peptides. Orthologous protein analysis clustered Tepidiphilus proteomes into 2,370 orthologous groups. Most of the Tepidiphilus putative pan proteome is shared among the studied species since more than 92% of the predicted proteins are shared by at least 2 genomes. The core proteome, shared by all seven Tepidiphilus genomes, comprises 1,621 orthologous protein groups, of which 1,596 are single-copy genes. All Tepidiphilus isolates have unique proteins, with no detected orthologous, with counts that ranged between 58 (Tepidiphilus thermophilus) and 183 (Tepidiphilus succinatimandens). Tepidiphilus UdeAICP_D1 has 90 specific putative proteins. Singleton protein count (with no orthologous) in the other species were T. baoligensis B18, n = 156; T. sp. J18, n = 170; T. sp. J10, n = 75; and T. margaritifer, n = 149 (Supplementary Table 3).
The Tepidiphilus sp. UdeAICP_D1 putative proteome functional annotation showed 51 complete modules and putative proteins that are part of 227 KEGG pathways. Moreover, Tepidiphilus sp. UdeAICP_D1 encodes the enzymes required for the biosynthesis of the amino acids: threonine, cysteine, isoleucine, lysine, ornithine, arginine, and proline. The carbon fixation was related to acetate production. Enzymes involved in the assimilatory sulfate reduction (CysND, CysC, CysH, and CysJI) and the denitrification pathway were also detected.
Several Tepidiphilus sp. UdeAICP_D1 genes could be involved in its biocide and stress-resistant phenotype. Five efflux pumps modules were identified: MexAB-OprM, MexJK-OprM, MexXY-OprM, AcrEF-TolC, and MdtEF-TolC. These efflux pumps are present in all seven Tepidiphilus genomes. Additionally, five type II toxin-antitoxin (TA) systems were found in Tepidiphilus chromosomes: YhaV-PrlF, YoeB-YefM, ParE-ParD, HigB-HigA, and FitB-FitA. Other genes related to the response to stress were annotated in this genome, namely PhoR-PhoB (phosphate starvation response), EnvZ-OmpR (osmotic stress response), CusS-CusR (copper tolerance), and QseC-QseB (quorum sensing regulator B and C related with the motility).
Tepidiphilus Genome Biocide Selection
The metagenomic analysis showed that Tepidiphilus dominated injection and produced water systems, although the injection water was a more restrictive environment due to the biocide treatment. To gain insights into the possible mixture of different Tepidiphilus genomes present in both samples and the selection process exerted by the biocide regime, a mapping, and single nucleotide variant (SNV) detection analysis was performed. To this end, the isolated genome of the Tepidiphilus sp. UDEAICP_D1 was used as a reference, and the shotgun metagenomic reads of both IW and PW were mapped to identify nucleotide variants that reflect the genomic diversity of this bacteria within the studied industrial waters.
The genome mapping analysis with IW reads identified only 95 well-supported (≥Q30) positions with an alternate nucleotide. This result indicates that the isolated genome scaffolds of Tepidiphilus sp. UDEAICP_D1 represents an accurate genome model and is somehow pure due to the low number of genomic variations (>0.005%). However, the PW read mapping analysis shows a mixture of Tepidiphilus genomes since more than 32,567 SNVs were detected with very diverse coverage (Figure 6). In the PW reads, it was also possible to discern some positions with a well-supported (>Q30) third SNV.
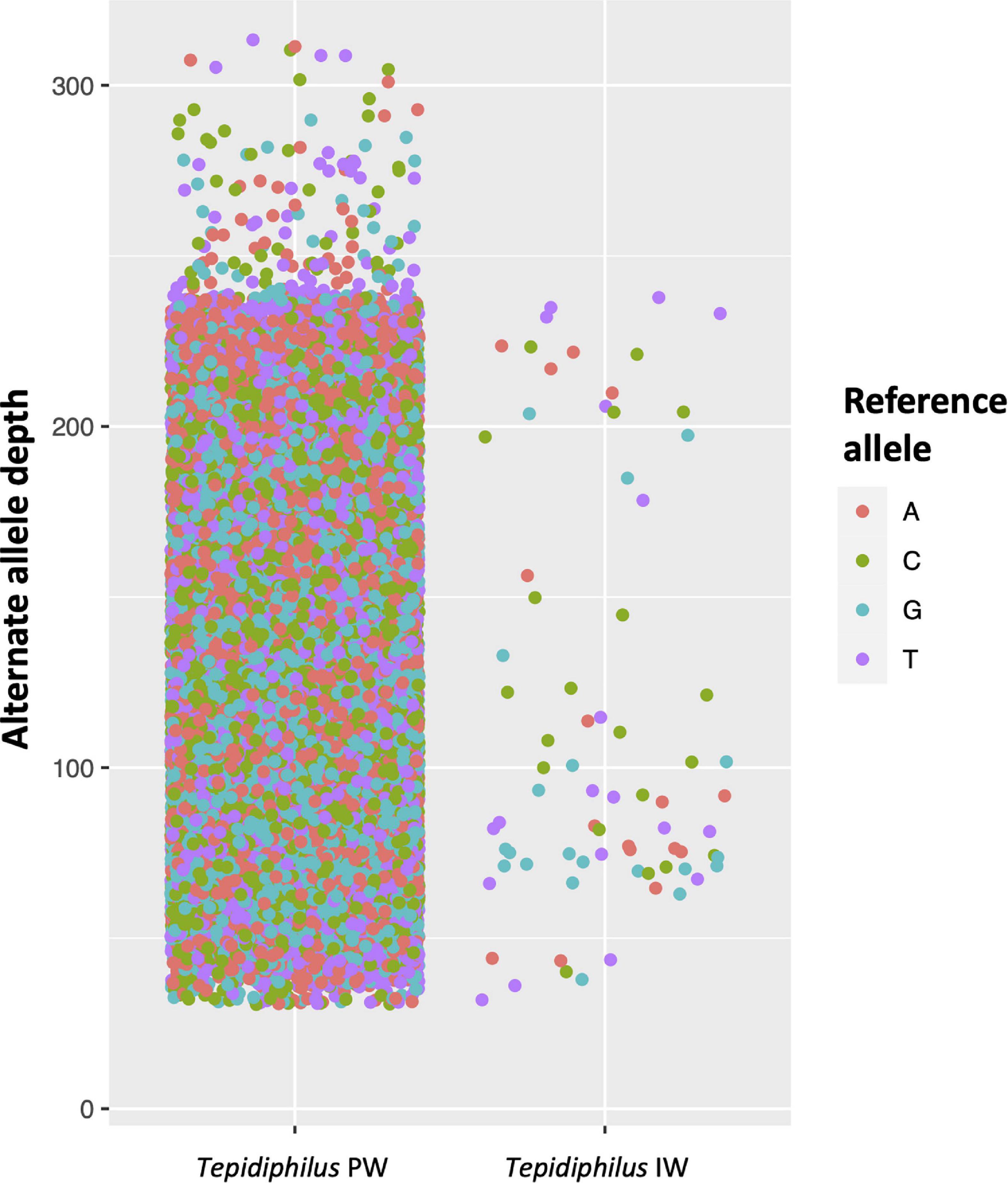
Figure 6. Genomic variations among Tepidiphilus genomes present within the injection (IW) and produced waters (PW). Detection of single nucleotide variants -SNVs- in the Tepidiphilus genomes. Each dot represents high-quality SNVs detected in the shotgun reads of the PW and IW. The respective reference base (Reference Allele) is represented as different colors. The Y-axis depicts the read depth for each SNV observed. In the IW, 95 alternative bases were detected while in the PW 32,567 high-quality alternate alleles were encountered.
To gain insights into the genomic characteristics of the Tepidiphilus sp. UDEAICP_D1 genome selected in the IW, an analysis of the enriched coding regions was performed. To this end, using as reference the annotated genome of the Tepidiphilus sp. UDEAICP_D1, the shotgun reads of both IW and PW libraries were mapped to the annotated CDSs and then counted, normalized, and plotted (Figure 7). The normalized counts (TPMs) obtained for the PW reads are depicted in the X-axis, whereas in the Y-axis are the IW TPMs. In addition, fold-change measurements were calculated with the aim to compare the relative abundance of each CDS between both metagenomes. Most of the CDSs form a cluster above the 256 TPM values on both samples. This result indicates that most genes are shared among the different Tepidiphilus genomes. The interesting CDSs are those with unbalanced TPM values in both metagenomes. The CDSs sequences with values above 256 (Y-axis) and below the 16 value (X-axis) display a strong enrichment within the Tepidiphilus sp. UDEAICP_D1 genome.
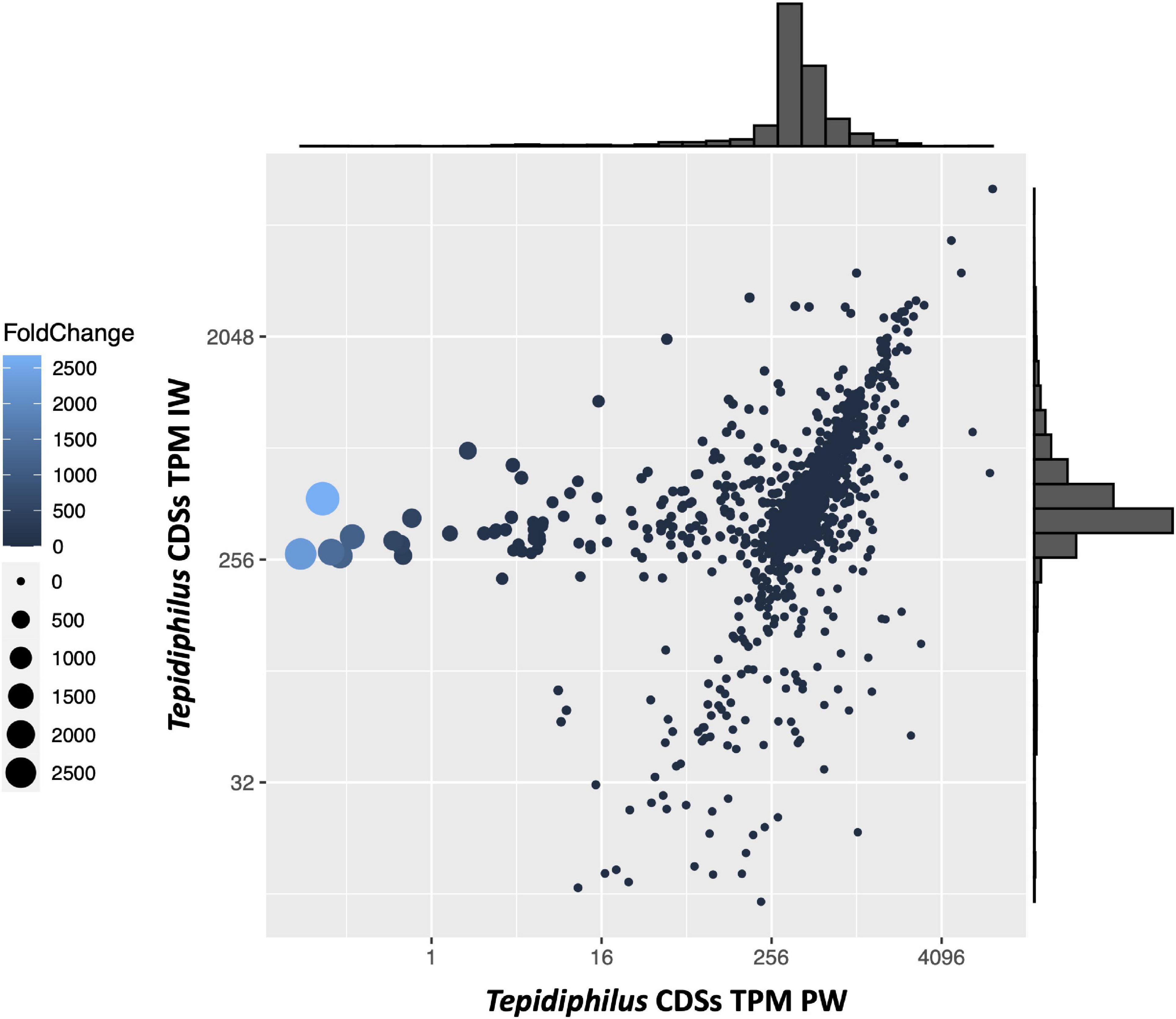
Figure 7. Tepidiphilus CDS relative abundance using TPM. The X and Y-axes represent the calculated TPM for each CDS for the PW and IW, respectively. Both axes are log2 transformed. Dot size and the lighter color are proportional to the CDS fold change: The bigger the dots or lighter the color, means higher fold change. Outer histograms represent the frequency of scaffolds in each region of the graph.
Enriched genes in the IW Tepidiphilus sp. UDEAICP_D1 genome were explored using a fold-change metric. This analysis pointed to a group of six genes with the highest fold-change values, between 500 and 2,600 (Supplementary Table 4, TepidiUDEAICP_19830-80). Interestingly, these genes are organized as a cluster in the same strand and with short intergenic regions of 10 or fewer bases. Unfortunately, the annotation tools retrieve no hits for most of them. Just one gene had a weak hit with a proteobacterial Heat shock 70 kDa protein.
The following enriched group consists of four genes, also organized in a tandem structure, and located in the same strand with very short intergenic regions (<5 bp.). Their fold-change ranged between 20 and 569 (Supplementary Table 4, TepidiUDEAICP_09260-90). The annotation results obtained for these proteins indicated putative functions as ABC-2 type transporter, ABC transporter, Methionine biosynthesis protein MetW, and Glycosyltransferases group. Another group of five genes with a high fold-change was related to the two-component system NarL (Supplementary Table 4, TepidiUDEAICP_11610-50), which is sensible to nitrate/nitrite ligands. This system is absent in the Tepidiphilus isolates B18, J10, and J18. Finally, it was possible to identify yet another group of 7 genes organized in an operon-like structure with fold-change values that ranged between 52 and 68 (Supplementary Table 4, TepidiUDEAICP_00090-150). The annotation results for this gene cluster indicate that they are related to enzymes with functions like: Short-chain dehydrogenase reductase, reversible phosphatidyl group transfer, DNA methylase, tRNA cytidylyltransferase, and Superfamily II DNA RNA helicases.
Discussion
The metataxonomic and metagenomic analysis performed in this work unveiled the microbial diversity present in a hyperthermophile oil reservoir of the Orinoquia region in Colombia, South America. In the oil industry, it is often common to recycle oil well water to be used again to flood the reservoir. This procedure is named secondary recovery and allows for more oil extraction. Recycled water is treated with biocides to prevent the undesired effect of the native of introduced microbiota in the oil recovery process.
The produced water samples might reflect a closer image of the native oil reservoir microbiota, whereas the injection water (recycled water with added biocides) microbiota contains indigenous biocide resilient microbes and those artificially introduced, which are also resistant to biocides, and that are capable of colonizing piping systems. As expected, our results show that the biocide regime dramatically reduces microbial richness and wipes out certain microbes in the injection water, but some bacteria endure.
The dominant bacterial genera observed as indigenous microbes in this oil reservoir has already been described in other thermophilic environments: Tepidiphilus, Thermus, Thermosulfidibacter, and Hydrogenobacter. These bacterial genera have been isolated from the produced water from oilfields that use secondary recovery by water injection with temperatures, in situ, above 55°C (Ren et al., 2015; Pannekens et al., 2019). However, it is still a matter of discussion if all these microorganisms are indigenous or some are introduced artificially with the injected water used for the secondary oil recovery (Vigneron et al., 2017).
The genus Thermus has been previously isolated from thermal environments, including oil reservoirs. This bacterium is associated with alterations of the physicochemical properties of crude petroleum and with the degradation of hydrocarbons (Nikolova and Gutierrez, 2020). Thermosulfidibacter is a thermophilic anaerobic bacterium initially isolated from deep-sea hydrothermal vents. In oil reservoirs, this bacterium is related to sulfur reduction (Nunoura et al., 2008). Hydrogenobacter is a hydrogen-oxidizing bacterium that is part of the thermophilic deep terrestrial water microbial community (Takai et al., 2001).
Tepidiphilus dominated all the water samples analyzed in this industrial system, injection, and produced, despite the recycling and biocide treatment. This genus has been reported in hot springs, thermophilic anaerobic digesters, and produced water from oil reservoirs (Zhang X. et al., 2020). This bacterium is described as a rod-shaped cell, gram-negative, motile by polar flagella, and capable of anaerobic growth in the presence of nitrate (Poddar et al., 2014).
Our genomic analysis demonstrated the existence of a Tepidiphilus microdiversity within the water recovered from the oil reservoir (produced water). After the water recycling and biocide addition, this microdiversity disappears, and only one clonal Tepidiphilus is selected. Due to the strong selection of this Tepidiphilus in the injection water, the metagenomic analysis allowed us to easily isolate and characterize its metagenome-assembled genome.
The phylogenomic analysis confirmed its genus but showed that Tepidiphilus sp. UdeAICP_D1 is an independent, and well-supported branch (100% UFB) outside T. succinatimandens and T. thermophilus clade. This analysis also showed very low distances between these three organisms. The ANI and dDDH approaches showed conflicting results among them and with our phylogenomic results. Nonetheless, the phylogenomic analysis is the most theoretically rigorous approach available to resolve evolutionary relationships between organisms. Adequate bacterial taxonomical methods should rely on the evolutionary relationship between the species (Parks et al., 2018). Further analysis, including more genomes, should be performed to support this finding.
When individual genomes are isolated from metagenomic data, one utmost concern arises and is related to the possibility of generating mixed/chimeric scaffolds (Meziti et al., 2021). To overcome this limitation, mapping analyses were performed to identify mixed nucleotide signals in the isolated reference genome of Tepidiphilus sp. UdeAICP_D1. With this strategy, we were able to spot only 95 SNVs along the whole genome. This result indicated that shotgun reads support a pure clonal genome. Additionally, the completeness and contamination analysis reported by CHECKM reported values of 100% and < 1%, respectively, confirming the quality of this metagenome-assembled genome (MAG) from the injection water. By contrast, the mapping and the CDS depth analysis revealed a complex mixture of closely related Tepidiphilus genomes within the produced water. Nonetheless, specific genomic blocks, encompassing certain genes, differentiate these different Tepidiphilus genomes. In the end, it seems that only one genome carries the necessary gene repertoire to endure and thrive in the harsh conditions imposed by physicochemical stress-induced outside the oil well.
Comparative genomic analysis revealed highly conserved genomes within the Tepidiphilus genus. Most of the virtual proteome is shared (92%) by at least two members. Nonetheless, all the analyzed species presented several specific CDSs. Tepidiphilus core proteome is organized in 1,621 orthologous groups present in all the studied species.
The dominance of Tepidiphilus sp. UDEAICP_D1 within the injection water might suggest that it is the most adapted microbe to the stringent conditions of this recycled water. Its genome comprises a combination of genes that help withstand biocide, metabolic and competence challenges like ABC transporters, NarL two-component systems, and TA-systems. Furthermore, Tepidiphilus sp. UDEAICP_D1 also encompasses genes related to toxic chemical resistance and stress response.
The microbial species present in the oil reservoirs are exposed to different types of selective pressure, such as chemical treatment (biocides) or high concentrations of lipids and heavy metals. This scenario might play a role in the prevalence of multidrug efflux transporters in the indigenous Tepidiphilus community. The multidrug efflux pumps MexJK-OprM, MexXY-OprM, MexAB-OprM, AcrEF-TolC, and MdtEF-TolC, were observed in all Tepidiphilus genomes. They belong to the resistance–nodulation–cell division (RND) superfamily. These molecular pump systems encompass three elements: an inner membrane protein component with proton motive function, a channel-forming outer membrane factor (OMF), and a periplasmic membrane fusion protein (MFP) (Delmar et al., 2014). RND complexes are commonly found in Proteobacteria and have been extensively studied in human infecting species, but the information in non-infectious environmental prokaryotes is scarce (Rahman et al., 2017).
The efflux pumps AcrEF-TolC and MdtEF-TolC have been extensively studied in Escherichia coli. They are associated with resistance to antibiotics like quinolones, Erythromycin, DNA intercalating agents like ethidium bromide, and anionic detergents like Sodium dodecyl sulfate SDS (Anes et al., 2015). Additionally, MdtEF-TolC is shown to protects E. coli cells from the toxicity of nitrosative stress during anaerobic respiration of nitrate. A similar effect could be expected in Tepidiphilus in the underground thermophilic anaerobic environment (Zhang Y. et al., 2011).
In general terms, RND systems confer resistance to a wide spectrum of antibiotics like aminoglycosides, fluoroquinolones, and beta-lactams. Also confer resistance to lipids, toxins, detergents, herbicides, dyes, and biocides (Venter et al., 2015; Tsutsumi et al., 2019).
Moreover, RND efflux pumps have been associated with glutaraldehyde resistance. In Pseudomonas, Vikram et al. (2015) found that efflux pumps contribute to glutaraldehyde endurance since RND inhibitors can potentiate glutaraldehyde activity. In P. aeruginosa, a famous microbe due to its wide-spectrum resistance to antimicrobial agents (Poole, 2011), four RND pumps have been described (Morita et al., 2001). Likewise, we found five of these molecular pump systems encoded in the Tepidiphilus genome.
The several toxin-antitoxin (TA) systems detected in the genome of Tepidiphilus sp. UdeAICP_D1 might contribute, as observed in other bacterial taxa, to bacterial stress responses, biofilm formation, virulence, antibiotic tolerance, and persistence bacteria formation (Zhang S.-P. et al., 2020). The TA system consists of pairs of genes located in one operon: a toxic protein, and a labile antitoxin. These systems can be activated in response to stress conditions and are crucial to compete and thrive in harsh conditions inhibiting translation or DNA replication of bacterial competitors (Harms et al., 2018). YhaV and YoeB are ribonuclease toxins of the RelE family. Both can arrest cell growth and are inhibited by their respective antitoxin PrlF and yefM. This TA system was initially described in E. coli (Schmidt et al., 2007), yet RelE toxins have been found on other Proteobacteria as well as in Firmicutes (Leplae et al., 2011). YefM-YoeB is one of the most frequent type II TA systems detected in pathogenic bacteria. This system is activated during thermal stress and confers oxidative resistance, biofilm formation, and antibiotic resistance (Ma et al., 2021). In the ParE-ParD TA system described in the Enterobacteriaceae family, ParE is a gyrase inhibitor that blocks DNA replication and provides antibiotic and heat tolerance. Tepidiphilus also carries a gene coding for the HigB mRNA endonuclease toxin which is inhibited by its antitoxin HigA. This TA system belongs to the HigA superfamily, which is found in Proteobacteria, Firmicutes, and Cyanobacteria (Leplae et al., 2011). FitB-FitA is another TA system that belongs to the VapB superfamily and that it is present in Bacteria and Archaea (Senissar et al., 2017). FitA, the antitoxin, is a DNA-binding protein with a putative ribbon-helix-helix (RHH) motif that neutralizes the toxin FitB through protein–protein interaction (Senissar et al., 2017).
Previous studies have reported that the ParE-ParD system stimulates bacterial growth at high temperatures and the biofilm formation in E. coli (Kamruzzaman and Iredell, 2019). ParE is a toxin that inhibits DNA gyrase inhibiting chromosome replication (Dalton and Crosson, 2010).
Conclusion
In conclusion, Tepidiphilus displays several type II TA systems and RND efflux pumps. In combination, both improve bacterial fitness and extrude toxic compounds that might be present in the oil reservoir or related to the biocides regime. Five type II TA systems were annotated in all Tepidiphilus genomes. These systems are associated with biofilm formation and stress response (Wang and Wood, 2011). It is demonstrated that bacterial communities organized under biofilm structures show higher resilience to biocide treatment (Mah and O’Toole, 2001). Additionally, five RND efflux pumps are carried by the Tepidiphilus genomes, and these systems are associated with the bacterial ability to withstand glutaraldehyde treatment (Vikram et al., 2015).
We consider that there are, at least, two potential areas that should be addressed shortly for Tepidiphilus. One involves the revision of the taxonomy of the members of the genus, and the other is related to targeted gene annotation. Sequencing of more Tepidiphilus genomes is necessary to validate, through phylogenomic analyses, the actual species scheme. On the other hand, in vitro biochemical analysis should be performed in order to gain insights into the function of the highly enriched genes found in Tepidiphilus sp. UDEAICP_D1. The current annotation programs and databases fail to assign a function to their respective gene products. The annotation of these genes will help to clarify not only its role in Tepidiphilus fitness, but also in other Proteobacterial species.
Data Availability Statement
The datasets presented in this study can be found in the NCBI SRA under accession numbers PRJNA727370 and PRJNA727592 (https://www.ncbi.nlm.nih.gov/). The MAG Tepidiphilus genome is also available under accession JAIQIL000000000.
Author Contributions
KB: wetlab experiments, DNA extraction and QC, bioinformatics and statistical analyses, and manuscript writing and review. JN: wet lab experiment design, sample collection, and processing, manuscript review. JA: wet lab experiment design and manuscript review. RJ-P: wet lab experiment design, sample collection and processing, and manuscript review. FC: bioinformatics and statistical analyses, and manuscript writing and review. JFA: project design and coordination, analysis planning, bioinformatics and statistical analyses, and manuscript writing and review. All authors contributed to the article and approved the submitted version.
Funding
This work was supported by the Instituto Colombiano del Petroleo (ICP).
Conflict of Interest
The authors declare that the research was conducted in the absence of any commercial or financial relationships that could be construed as a potential conflict of interest.
Publisher’s Note
All claims expressed in this article are solely those of the authors and do not necessarily represent those of their affiliated organizations, or those of the publisher, the editors and the reviewers. Any product that may be evaluated in this article, or claim that may be made by its manufacturer, is not guaranteed or endorsed by the publisher.
Supplementary Material
The Supplementary Material for this article can be found online at: https://www.frontiersin.org/articles/10.3389/fmicb.2021.741555/full#supplementary-material
Footnotes
- ^ https://dfast.ddbj.nig.ac.jp/
- ^ https://tygs.dsmz.de/
- ^ http://tree.bio.ed.ac.uk/software/figtree/
References
Agarwala, R., Barrett, T., Beck, J., Benson, D. A., Bollin, C., Bolton, E., et al. (2018). Database resources of the National Center for Biotechnology Information. Nucleic Acids Res. 46, D8–D13. doi: 10.1093/nar/gkx1095
Anes, J., McCusker, M. P., Fanning, S., and Martins, M. (2015). The ins and outs of RND efflux pumps in Escherichia coli. Front. Microbiol. 6:587. doi: 10.3389/fmicb.2015.00587
Bray, N. L., Pimentel, H., Melsted, P., and Pachter, L. (2016). Near-optimal probabilistic RNA-seq quantification. Nat. Biotechnol. 34, 525–527. doi: 10.1038/nbt.3519
Buchfink, B., Xie, C., and Huson, D. H. (2014). Fast and sensitive protein alignment using DIAMOND. Nat. Methods 12, 59–60. doi: 10.1038/nmeth.3176
Carver, T., Harris, S. R., Berriman, M., Parkhill, J., and McQuillan, J. A. (2012). Artemis: An integrated platform for visualization and analysis of high-throughput sequence-based experimental data. Bioinformatics 28, 464–469. doi: 10.1093/bioinformatics/btr703
Cosentino, S., and Iwasaki, W. (2019). SonicParanoid: Fast, accurate and easy orthology inference. Bioinformatics 35, 149–151. doi: 10.1093/bioinformatics/bty631
Dalton, K. M., and Crosson, S. (2010). A conserved mode of protein recognition and binding in a ParD-ParE toxin - antitoxin complex. Biochemistry 49, 2205–2215. doi: 10.1021/bi902133s
Delmar, J. A., Su, C. C., and Yu, E. W. (2014). Bacterial multidrug efflux transporters. Annu. Rev. Biophys. 43, 93–117. doi: 10.1146/annurev-biophys-051013-022855
Dolfing, J., and Hubert, C. R. J. (2017). Using thermodynamics to predict the outcomes of nitrate-based oil reservoir souring control interventions. Front. Microbiol. 8:1–9. doi: 10.3389/fmicb.2017.02575
Gao, P., Tian, H., Wang, Y., Li, Y., Li, Y., Xie, J., et al. (2016). Spatial isolation and environmental factors drive distinct bacterial and archaeal communities in different types of petroleum reservoirs in China. Sci. Rep. 6, 1–12. doi: 10.1038/srep20174
Gieg, L. M., Jack, T. R., and Foght, J. M. (2011). Biological souring and mitigation in oil reservoirs. Appl. Microbiol. Biotechnol. 92, 263–282. doi: 10.1007/s00253-011-3542-6
Harms, A., Brodersen, D. E., Mitarai, N., and Gerdes, K. (2018). Toxins, Targets, and Triggers: An Overview of Toxin-Antitoxin Biology. Mol. Cell 70, 768–784. doi: 10.1016/j.molcel.2018.01.003
Herlemann, D. P. R., Labrenz, M., Ju, K., Bertilsson, S., Waniek, J. J., and Andersson, A. F. (2011). Transitions in bacterial communities along the 2000 km salinity gradient of the Baltic Sea. ISME J. 5, 1571–1579. doi: 10.1038/ismej.2011.41
Huson, D. H., Auch, A. F., Qi, J., and Schuster, S. C. (2007). MEGAN analysis of metagenomic data. Genome Res. 17, 377–386. doi: 10.1101/gr.5969107
Jain, C., Rodriguez-R, L. M., Phillippy, A. M., Konstantinidis, K. T., and Aluru, S. (2018). High throughput ANI analysis of 90K prokaryotic genomes reveals clear species boundaries. Nat. Commun. 9, 1–8. doi: 10.1038/s41467-018-07641-9
Jurelevicius, D., Ramos, L., Abreu, F., Lins, U., de Sousa, M. P., dos Santos, V. V. C. M., et al. (2021). Long-term souring treatment using nitrate and biocides in high-temperature oil reservoirs. Fuel 288:119731. doi: 10.1016/j.fuel.2020.119731
Kahrilas, G. A., Blotevogel, J., Stewart, P. S., and Borch, T. (2015). Biocides in hydraulic fracturing fluids: A critical review of their usage, mobility, degradation, and toxicity. Environ. Sci. Technol. 49, 16–32. doi: 10.1021/es503724k
Kamruzzaman, M., and Iredell, J. (2019). A ParDE-family toxin antitoxin system in major resistance plasmids of Enterobacteriaceae confers antibiotic and heat tolerance. Sci. Rep. 9, 1–12. doi: 10.1038/s41598-019-46318-1
Katoh, K., and Standley, D. M. (2013). MAFFT Multiple Sequence Alignment Software Version 7: Improvements in Performance and Usability. Mol. Biol. Evol. 30, 772–780. doi: 10.1093/molbev/mst010
Korenblum, E., Valoni, É, Penna, M., and Seldin, L. (2010). Bacterial diversity in water injection systems of Brazilian offshore oil platforms. Appl. Microbiol. Biotechnol. 85, 791–800. doi: 10.1007/s00253-009-2281-4
Lanfear, R., Calcott, B., Kainer, D., Mayer, C., and Stamatakis, A. (2014). Selecting optimal partitioning schemes for phylogenomic datasets. BMC Evol. Biol. 14:1–14. doi: 10.1186/1471-2148-14-82
Langmead, B., and Salzberg, S. L. (2012). Fast gapped-read alignment with Bowtie 2. Nat. Methods 9, 357–359. doi: 10.1038/nmeth.1923
Leplae, R., Geeraerts, D., Hallez, R., Guglielmini, J., Drze, P., and Van Melderen, L. (2011). Diversity of bacterial type II toxin-antitoxin systems: A comprehensive search and functional analysis of novel families. Nucleic Acids Res. 39, 5513–5525. doi: 10.1093/nar/gkr131
Li, H. (2011). A statistical framework for SNP calling, mutation discovery, association mapping and population genetical parameter estimation from sequencing data. Bioinformatics 27, 2987–2993. doi: 10.1093/bioinformatics/btr509
Li, H., Handsaker, B., Wysoker, A., Fennell, T., Ruan, J., Homer, N., et al. (2009). The Sequence Alignment/Map format and SAMtools. Bioinformatics 25, 2078–2079. doi: 10.1093/bioinformatics/btp352
Liang, B., Zhang, K., Wang, L. Y., Liu, J. F., Yang, S. Z., Gu, J. D., et al. (2018). Different diversity and distribution of archaeal community in the aqueous and oil phases of production fluid from high-temperature petroleum reservoirs. Front. Microbiol. 9:00841. doi: 10.3389/fmicb.2018.00841
Liu, Y. F., Galzerani, D. D., Mbadinga, S. M., Zaramela, L. S., Gu, J. D., Mu, B. Z., et al. (2018). Metabolic capability and in situ activity of microorganisms in an oil reservoir. Microbiome 6, 1–12. doi: 10.1186/s40168-017-0392-1
Ma, D., Gu, H., Shi, Y., Huang, H., Sun, D., and Hu, Y. (2021). Edwardsiella piscicida YefM-YoeB: A Type II Toxin-Antitoxin System That Is Related to Antibiotic Resistance, Biofilm Formation, Serum Survival, and Host Infection. Front. Microbiol. 12:646299. doi: 10.3389/fmicb.2021.646299
Magot, M., Ollivier, B., and Patel, B. K. C. (2000). Microbiology of petroleum reservoirs. Antonie van Leeuwenhoek Int. J. Gen. Mol. Microbiol. 77, 103–116. doi: 10.1023/A:1002434330514
Mah, T. F. C., and O’Toole, G. A. (2001). Mechanisms of biofilm resistance to antimicrobial agents. Trends Microbiol. 9, 34–39. doi: 10.1016/S0966-842X(00)01913-2
Martin, M. (2011). Cutadapt removes adapter sequences from high-throughput sequencing reads. EMBnet J. 17:10. doi: 10.14806/ej.17.1.200
McMurdie, P. J., and Holmes, S. (2013). Phyloseq: An R Package for Reproducible Interactive Analysis and Graphics of Microbiome Census Data. PLoS One 8:e61217. doi: 10.1371/journal.pone.0061217
Meier-Kolthoff, J. P., and Göker, M. (2019). TYGS is an automated high-throughput platform for state-of-the-art genome-based taxonomy. Nat. Commun. 10, 1–10. doi: 10.1038/s41467-019-10210-3
Meziti, A., Rodriguez-R, L. M., Hatt, J. K., Peña-Gonzalez, A., Levy, K., and Konstantinidis, K. T. (2021). The Reliability of Metagenome-Assembled Genomes (MAGs) in Representing Natural Populations: Insights from Comparing MAGs against Isolate Genomes Derived from the Same Fecal Sample. Appl. Environ. Microbiol. 87, 1–15. doi: 10.1128/AEM.02593-20
Minh, B. Q., Schmidt, H. A., Chernomor, O., Schrempf, D., Woodhams, M. D., von Haeseler, A., et al. (2020). IQ-TREE 2: New Models and Efficient Methods for Phylogenetic Inference in the Genomic Era. Mol. Biol. Evol. 37, 1530–1534. doi: 10.1093/molbev/msaa015
Morita, Y., Komori, Y., Mima, T., Kuroda, T., Mizushima, T., and Tsuchiya, T. (2001). Construction of a series of mutants lacking all of the four major mex operons for multidrug efflux pumps or possessing each one of the operons from Pseudomonas aeruginosa PAO1: MexCD-OprJ is an inducible pump. FEMS Microbiol. Lett. 202, 139–143. doi: 10.1111/j.1574-6968.2001.tb10794.x
Moriya, Y., Itoh, M., Okuda, S., Yoshizawa, A. C., and Kanehisa, M. (2007). KAAS: An automatic genome annotation and pathway reconstruction server. Nucleic Acids Res. 35, 182–185. doi: 10.1093/nar/gkm321
Nikolova, C., and Gutierrez, T. (2020). Use of Microorganisms in the Recovery of Oil From Recalcitrant Oil Reservoirs: Current State of Knowledge, Technological Advances and Future Perspectives. Front. Microbiol. 10:02996. doi: 10.3389/fmicb.2019.02996
Nissen, J. N., Johansen, J., Allesøe, R. L., Sønderby, C. K., Armenteros, J. J. A., Grønbech, C. H., et al. (2021). Improved metagenome binning and assembly using deep variational autoencoders. Nat. Biotechnol. 39, 555–560. doi: 10.1038/s41587-020-00777-4
Nunoura, T., Oida, H., Miyazaki, M., and Suzuki, Y. (2008). Thermosulfidibacter takaii gen. nov., sp. nov., a thermophilic, hydrogen-oxidizing, sulfur-reducing chemolithoautotroph isolated from a deep-sea hydrothermal field in the Southern Okinawa Trough. Int. J. Syst. Evol. Microbiol. 58, 659–665. doi: 10.1099/ijs.0.65349-0
Nurk, S., Meleshko, D., Korobeynikov, A., and Pevzner, P. A. (2017). MetaSPAdes: A new versatile metagenomic assembler. Genome Res. 27, 824–834. doi: 10.1101/gr.213959.116
Okoro, C., Ekun, O. A., Nwume, M. I., and Lin, J. (2016). Molecular analysis of microbial community structures in Nigerian oil production and processing facilities in order to access souring corrosion and methanogenesis. Corros. Sci. 103, 242–254. doi: 10.1016/j.corsci.2015.11.024
Oksanen, J., Blanchet, F. G., Friendly, M., Kindt, R., Legendre, P., McGlinn, D., et al. (2020). vegan: Community Ecology Package. Vienna: R Core Team.
Pannekens, M., Kroll, L., Müller, H., Mbow, F. T., and Meckenstock, R. U. (2019). Oil reservoirs, an exceptional habitat for microorganisms. N. Biotechnol. 49, 1–9. doi: 10.1016/j.nbt.2018.11.006
Parks, D. H., Chuvochina, M., Waite, D. W., Rinke, C., Skarshewski, A., Chaumeil, P. A., et al. (2018). A standardized bacterial taxonomy based on genome phylogeny substantially revises the tree of life. Nat. Biotechnol. 36:996. doi: 10.1038/nbt.4229
Parks, D. H., Imelfort, M., Skennerton, C. T., Hugenholtz, P., and Tyson, G. W. (2015). CheckM: Assessing the quality of microbial genomes recovered from isolates, single cells, and metagenomes. Genome Res. 25, 1043–1055. doi: 10.1101/gr.186072.114
Poddar, A., Lepcha, R. T., and Das, S. K. (2014). Taxonomic study of the genus Tepidiphilus: Transfer of Petrobacter succinatimandens to the genus Tepidiphilus as Tepidiphilus succinatimandens comb. nov., emended description of the genus Tepidiphilus and description of Tepidiphilus thermophilus sp. nov. Int. J. Syst. Evol. Microbiol. 64, 228–235. doi: 10.1099/ijs.0.056424-0
Poole, K. (2011). Pseudomonas aeruginosa: Resistance to the max. Front. Microbiol. 2:65. doi: 10.3389/fmicb.2011.00065
Quast, C., Pruesse, E., Yilmaz, P., Gerken, J., Schweer, T., Yarza, P., et al. (2013). The SILVA ribosomal RNA gene database project: improved data processing and web-based tools. Nucleic Acids Res. 41:D590. doi: 10.1093/nar/gks1219
R Development Core Team (2018). A Language and Environment for Statistical Computing. Vienna: R Core Team.
Rahman, T., Yarnall, B., and Doyle, D. A. (2017). Efflux drug transporters at the forefront of antimicrobial resistance. Eur. Biophys. J. 46, 647–653. doi: 10.1007/s00249-017-1238-2
Ren, H., Xiong, S., Gao, G., Song, Y., Cao, G., Zhao, L., et al. (2015). Bacteria in the injection water differently impacts the bacterial communities of production wells in high-temperature petroleum reservoirs. Front. Microbiol. 6:00505. doi: 10.3389/fmicb.2015.00505
Rognes, T., Flouri, T., Nichols, B., Quince, C., and Mahé, F. (2016). VSEARCH: a versatile open source tool for metagenomics. PeerJ 4:e2584. doi: 10.7717/peerj.2584
Roumagnac, M., Pradel, N., Bartoli, M., Garel, M., Jones, A. A., Armougom, F., et al. (2020). Responses to the Hydrostatic Pressure of Surface and Subsurface Strains of Pseudothermotoga elfii Revealing the Piezophilic Nature of the Strain Originating From an Oil-Producing Well. Front. Microbiol. 11:588771. doi: 10.3389/fmicb.2020.588771
Schloss, P. D., Westcott, S. L., Ryabin, T., Hall, J. R., Hartmann, M., Hollister, E. B., et al. (2009). Introducing mothur: open-source, platform-independent, community-supported software for describing and comparing microbial communities. Appl. Environ. Microbiol. 75, 7537–7541. doi: 10.1128/AEM.01541-09
Schmidt, O., Schuenemann, V. J., Hand, N. J., Silhavy, T. J., Martin, J., Lupas, A. N., et al. (2007). prlF and yhaV Encode a New Toxin-Antitoxin System in Escherichia coli. J. Mol. Biol. 372, 894–905. doi: 10.1016/j.jmb.2007.07.016
Senissar, M., Manav, M. C., and Brodersen, D. E. (2017). Structural conservation of the PIN domain active site across all domains of life. Protein Sci. 26, 1474–1492. doi: 10.1002/pro.3193
Takai, K., Komatsu, T., and Horikoshi, K. (2001). Hydrogenobacter subterraneus sp. nov., an extremely thermophilic, heterotrophic bacterium unable to grow on hydrogen gas, from deep subsurface geothermal water. Int. J. Syst. Evol. Microbiol. 51, 1425–1435. doi: 10.1099/00207713-51-4-1425
Tanizawa, Y., Fujisawa, T., Kaminuma, E., Nakamura, Y., and Arita, M. (2016). DFAST and DAGA: Web-based integrated genome annotation tools and resources. Biosci. Microbiota Food Heal. 35, 173–184. doi: 10.12938/bmfh.16-003
Tsutsumi, K., Yonehara, R., Ishizaka-Ikeda, E., Miyazaki, N., Maeda, S., Iwasaki, K., et al. (2019). Structures of the wild-type MexAB–OprM tripartite pump reveal its complex formation and drug efflux mechanism. Nat. Commun. 10, 1–10. doi: 10.1038/s41467-019-09463-9
Venter, H., Mowla, R., Ohene-Agyei, T., and Ma, S. (2015). RND-type drug efflux pumps from Gram-negative bacteria: Molecular mechanism and inhibition. Front. Microbiol. 6:377. doi: 10.3389/fmicb.2015.00377
Vigneron, A., Alsop, E. B., Lomans, B. P., Kyrpides, N. C., Head, I. M., and Tsesmetzis, N. (2017). Succession in the petroleum reservoir microbiome through an oil field production lifecycle. ISME J. 11, 2141–2154. doi: 10.1038/ismej.2017.78
Vikram, A., Bomberger, J. M., and Bibby, K. J. (2015). Efflux as a glutaraldehyde resistance mechanism in Pseudomonas fluorescens and Pseudomonas aeruginosa biofilms. Antimicrob. Agents Chemother. 59, 3433–3440.
Wang, Q., Garrity, G. M., Tiedje, J. M., and Cole, J. R. (2007). Naïve Bayesian Classifier for Rapid Assignment of rRNA Sequences into the New Bacterial Taxonomy. Appl. Environ. Microbiol. 73:5261. doi: 10.1128/AAC.05152-14
Wang, X., and Wood, T. K. (2011). Toxin-antitoxin systems influence biofilm and persister cell formation and the general stress response. Appl. Environ. Microbiol. 77, 5577–5583. doi: 10.1128/AEM.00062-07
Yuan, Y., Ziyu, G., Yu, C., Xuefeng, T., Peike, G., Guoquiang, L., et al. (2021). Disentangling the distinct mechanisms shaping the subsurface oil reservoir bacterial and archaeal communities across northern China. Sci. Total Environ. 789:148074. doi: 10.1128/AEM.05068-11
Zhang, X., Wang, G., Ma, X., Yu, J., You, J., Xue, Y., et al. (2020). Tepidiphilus baoligensis sp. nov., a Novel Bacterium of the Family Hydrogenophilaceae Isolated from an Oil Reservoir. Curr. Microbiol. 77, 1939–1944. doi: 10.1016/j.scitotenv.2021.148074
Zhang, S.-P., Wang, Q., Quan, S.-W., Yu, X.-Q., Wang, Y., Guo, D.-D., et al. (2020). Type II toxin–antitoxin system in bacteria: activation, function, and mode of action. Biophys. Rep. 6, 68–79. doi: 10.1007/s00284-020-01983-8
Zhang, Y., Xiao, M., Horiyama, T., Zhang, Y., Li, X., Nishino, K., et al. (2011). The multidrug efflux pump MdtEF protects against nitrosative damage during the anaerobic respiration in Escherichia coli. J. Biol. Chem. 286, 26576–26584. doi: 10.1007/s41048-020-00109-8
Zhou, L., Lu, Y. W., Wang, D. W., Zhang, S. L., Tang, E. G., Qi, Z. Z., et al. (2020). Microbial community composition and diversity in production water of a high-temperature offshore oil reservoir assessed by DNA- and RNA-based analyses. Int. Biodeterior. Biodegrad. 151:104970. doi: 10.1074/jbc.M111.243261
Keywords: extremophile, comparative genomics, metagenomics, Tepidiphilus, thermophile, biocide resistance
Citation: Bedoya K, Niño J, Acero J, Jaimes-Prada R, Cabarcas F and Alzate JF (2021) Metagenomic Analysis of Biocide-Treated Neotropical Oil Reservoir Water Unveils Microdiversity of Thermophile Tepidiphilus. Front. Microbiol. 12:741555. doi: 10.3389/fmicb.2021.741555
Received: 14 July 2021; Accepted: 04 October 2021;
Published: 01 November 2021.
Edited by:
Edgardo Donati, National University of La Plata, ArgentinaReviewed by:
Grégoire Michoud, École Polytechnique Fédérale de Lausanne, SwitzerlandStepan Toshchakov, Kurchatov Institute, Russia
Copyright © 2021 Bedoya, Niño, Acero, Jaimes-Prada, Cabarcas and Alzate. This is an open-access article distributed under the terms of the Creative Commons Attribution License (CC BY). The use, distribution or reproduction in other forums is permitted, provided the original author(s) and the copyright owner(s) are credited and that the original publication in this journal is cited, in accordance with accepted academic practice. No use, distribution or reproduction is permitted which does not comply with these terms.
*Correspondence: Juan F. Alzate, amZlcm5hbmRvLmFsemF0ZUB1ZGVhLmVkdS5jbw==